- 1 Rotman Research Institute, Baycrest Hospital, Toronto, ON, Canada
- 2 Department of Psychology, University of California at Berkeley, Berkeley, CA, USA
The most recently encountered information is often most easily remembered in psychological tests of memory. Recent investigations of the neural basis of such “recency effects” have shown that activation in the lateral inferior parietal cortex (LIPC) tracks the recency of a probe item when subjects make recognition memory judgments. A key question regarding recency effects in the LIPC is whether they fundamentally reflect the storage (and strength) of information in memory, or whether such effects are a consequence of task difficulty or an upswing in resting state network activity. Using functional magnetic resonance imaging we show that recency effects in the LIPC are independent of the difficulty of recognition memory decisions, that they are not a by-product of an increase in resting state network activity, and that they appear to dissociate from regions known to be involved in verbal working memory maintenance. We conclude with a discussion of two alternative explanations – the memory strength and “expectancy” hypotheses, respectively – of the parietal lobe recency effect.
Introduction
When presented with a list of words to recall, people are more likely to recall the first and last few items in the list than they are to recall items from the middle of the list. These twin phenomena, known as the “primacy” and “recency” effects, have been observed in a variety of experimental contexts and paradigms, in tests of recall and recognition, and over short and long time scales (Crowder, 1976). While there is disagreement as to the exact mechanistic basis of recency effects (Davelaar et al., 2005; Brown et al., 2007), many would agree that some combination of temporal factors such as time-based decay (Peterson and Peterson, 1959; Portrat et al., 2008) and interference (Nairne, 2002) conspire to make older memories more difficult to retrieve than more recent ones.
A number of functional neuroimaging studies of recognition memory have shown that activity in the lateral inferior parietal cortex (LIPC) during recognition memory tracks the recency of the probe item (Dudukovic and Wagner, 2007; Nee and Jonides, 2008; Greve et al., 2010; Huijbers et al., 2010; Buchsbaum et al., 2011b). We have previously shown that in a verbal continuous recognition memory task, activation in the LIPC is maximal at a lag of 1 (immediate repetition) and falls off at a roughly exponential rate before reaching an asymptotic lower bound after approximately four or five items (Buchsbaum et al., 2011b). This finding seems to be consistent with the idea that the LIPC plays a role short-term memory storage – or at least that the activation observed in the region for short lag repetitions is a genuine marker of neural processes that underlie recency phenomenon in recognition memory.
An alternative hypothesis, however, is that because recognition memory for short lag trials is easier – both in terms of response time (RT) and accuracy (e.g., Rubin et al., 1999) – than for longer lags, the observed pattern of activation in LIPC might be generically attributable to “task difficulty.” Indeed, at least a portion of the LIPC belongs to a network of regions that are often activated at rest (the so-called “resting state” or “default mode” network; Raichle et al., 2001), and typically show an inverse correlation with task difficulty in a variety of experimental paradigms (Mckiernan et al., 2003; Huijbers et al., 2010; Kayser et al., 2010). Thus, the observations that we and others have made of recency-related activity in the LIPC might not reflect memory processes per se, but rather an increase in default mode activity that commonly accompanies manipulations of task difficulty.
The goal of the present study is to test whether recency effects in the LIPC persist even in the case where lag and difficulty are no longer strictly confounded. To achieve this decoupling between item lag and item difficulty in recognition memory, we adopted a paradigm that involved the presentation of two continuous streams of words – one in the visual modality, the relevant stream – and one in the auditory modality, the irrelevant stream. Words from the relevant stream are sometimes repeated in the visual stream but subjects nevertheless have to classify those items as “new” (see Figure 1). As we knew from a behavioral pilot study, accuracy in rejecting these “lure” probes (i.e., probes that had previously appeared only in the unattended stream) does not strictly decrease as a function of lag as is seen for items that repeat in the attended stream. Thus, by examining the pattern of responses as a function of lag for both visual repetitions (VRs) and lures, we can examine the extent to which recency-related activation in LIPC is dissociable from item difficulty. If we find that activity in LIPC tracks the performance profile across lag for relevant and irrelevant items, we may conclude that the effect is indeed a trivial consequence of task difficulty. On the contrary, if activity in LIPC is monotonically related to recency in both conditions, the evidence will rather support a genuine link between LIPC activity and mnemonic recency as we have previously hypothesized (Buchsbaum et al., 2011b).
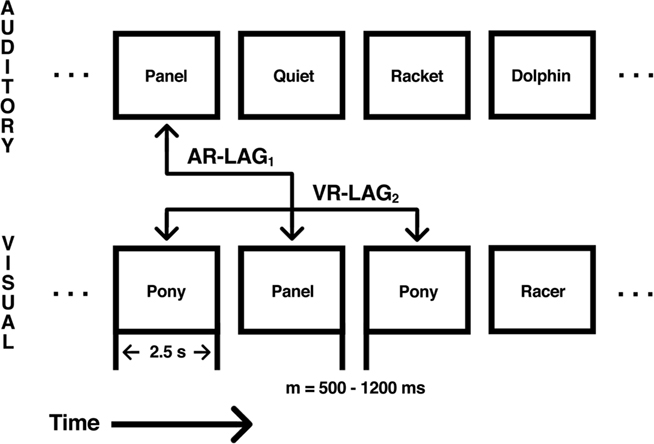
Figure 1. Illustration of the dual-modality continuous recognition task. Each level represents either the auditory or visual stream as labeled. Each box represents a stimulus presented in either the auditory or visual modality. Stimuli were presented for 2.5-s with an intertrial interval (ITI) between 800 and 1200 ms, with a mean ITI of 1000 ms. Repetition occurred across modality as auditory repetitions (AR) between the auditory stream and the visual stream or as visual repetitions (VR) within the visual modality. Repetition intervals of AR and VR trials varied between five levels, from 1 to 16 (1, 2, 4, 8, and 16). Repetition modality was crossed with repetition interval to yield 10 distinct repetition conditions.
A second aim of this study is to demonstrate that the region of the LIPC that is sensitive to recency in recognition memory is anatomically distinct from a nearby functional area in the temporoparietal zone that has consistently been associated with subvocal rehearsal in working memory (for a review, see Buchsbaum and D’Esposito, 2008). Functional neuroimaging studies involving tasks that require covert verbal rehearsal in the service of working memory consistently implicate a region in the more ventral extent of the LIPC in the posterior portion of the planum temporale (Area Spt; Sylvian–parietal–temporal; Hickok et al., 2003; Buchsbaum and D’Esposito, 2008). Activity in Spt is thought be important for phonological working memory, and damage to the area is associated with conduction aphasia, a disorder marked by an impairment in auditory–verbal repetition and short-term memory (Buchsbaum et al., 2011b). It might be argued that recency effects in recognition memory are related to subvocal rehearsal, and that activation in LIPC during short lag repetitions is simply a reflection of maintenance processes in working memory. We addressed this possibility by administering a verbal working memory localizer task that reliably activates area Spt, thereby offering a way to independently assess whether recency effects and subvocal rehearsal co-localize to the same area of cortex in the temporoparietal zone.
Materials and Methods
Participants
Sixteen healthy subjects (six females, 18–38 years) gave informed consent according to procedures approved by the University of California Institutional Review Board. All were right handed, were native English speakers, had normal hearing, had normal or corrected-to-normal vision, and had at least 12 years of education. None of the subjects reported any history of neurological or psychiatric disease nor were they taking any psychoactive medications.
Experimental Stimuli
A total of 860 novel bi-syllabic words were selected from the MRC psycholinguistic database (Wilson, 1988). Auditory recordings of 720 of those words were made using a human male voice.
Functional Magnetic Resonance Imaging
Functional images were acquired during seven runs lasting 445 s each. T2*-weighted echo-planar images (EPI) sensitive to blood oxygenation level-dependent (BOLD) contrast were acquired using a 3-Tesla Siemens (Berlin, Germany) MAGNETOM Trio and a 12-channel transverse electromagnetic send-and-receive radiofrequency head coil system. Images were acquired using a two-shot gradient-echo EPI sequence (22.5 cm × 22.5 cm field of view with a 96 × 96 matrix size, resulting in an in-place resolution of 2.5 mm × 2.5 mm for each of twenty-four 3.5-mm axial slices with a 1-mm interslice gap; repetition time, 1.37 s; echo time, 27 ms; flip angle, 62°). High-resolution gradient-echo multislice T1-weighted scans, coplanar with the EPIs, as well as whole-brain MP-RAGE three-dimensional T1-weighted scans were acquired for anatomical localization.
All stimuli were presented using E-Prime 2.0 (Psychological Software Tools, Inc.). Visual stimuli were presented in a 120-point Arial font with a liquid crystal display projection system onto a screen approximately 4 m away. Subjects viewed the screen through a mirror mounted on the radiofrequency head coil, located approximately 20 cm from the screen. Sound stimuli were delivered through an Avotec headphone system. Subjects wore earplugs for sound attenuation of scanner background noise. Auditory word stimuli were presented at approximately 10–15 db above the scanner noise.
Dual-Modality Continuous Recognition Task
Subjects performed a continuous recognition paradigm with simultaneous presentation of auditory and visual stimuli (Figure 1). For each of six scanning runs, subjects were presented with a total of 120 visual words and 120 auditory words. Auditory stimuli were presented with a simultaneous onset to visual stimuli. Visual stimuli were presented for 2.5 s. The stimulus onset asynchrony (SOA) varied between 800 to 1200 ms with a mean of 1000 ms. During the entire task, subjects were instructed to ignore the auditory stimuli. For each visually presented word, subjects made a judgment as to whether the current item had been previously presented in the visual modality. Subjects pressed the left button if they judged that an item was a previously presented word (old) or if the item was new. In each run, 40 (33.3%) of the visually presented words were novel (NOVEL), 40 (33.3%) were repeated words first presented as a novel visual word (VR), and the remaining 40 (33.3%) were repeated words that had first been presented auditorally (auditory repetition, AR), but had never been presented visually. Auditory items never repeated in the auditory modality but did repeat in the visual modality (see Figure 1). Subjects were made aware of each of the three possibilities and reminded to ignore the auditory stimuli. Thus, subjects were told that sometimes an item that first appeared in the auditory modality could later appear in the visual modality and that in such cases the correct response to the visual probe would be “new”; in other words, only items that were repeated in the visual modality were to be considered old. AR trials therefore constituted “lures” in the sense that these items had previously been encountered in the ignored modality, but should nevertheless be rejected as an old item.
Subjects were also instructed that no word ever repeated across scanning runs. VR and AR trials were manipulated by varying the number of words between initial presentation and repeated presentation. VR and AR trials followed the initial presentation immediately (LAG1), after 1 intervening word (LAG2), after 3 intervening words (LAG4), after 7 intervening words (LAG8), or after 15 intervening words (LAG16). The 40 VR trials were distributed equally across all five LAG levels, with eight trials per level. The 40 AR trials followed the same distribution across levels of LAG. Subjects were informed that repeats would occur within the relatively recent past, but were not told the exact maximum number of intervening words between initial presentation and subsequent repetition of any given stimulus.
Verbal Working Memory Localizer Task
On the first run of the scanning session all subjects performed a 7-min working memory localizer task. Subjects performed an auditory Sternberg recognition memory task with letter stimuli that were recorded by a male voice and presented through headphones during functional magnetic resonance imaging (fMRI) scanning. On each of 14 trials, six letters drawn randomly from the set of consonants (except for W) were presented with an SOA of 800 ms. Stimulus presentation was followed by a 10-s delay period in which subjects were instructed to keep the letters in memory by silently rehearsing them. After the 10-s delay period, a probe letter was presented (duration: 400–500 ms) auditorally and subjects decided whether it was or was not a member of the memory set.
Data Processing
For each run, 325 EPI images were obtained in DICOM format and exported using AFNI (Cox, 1996) into NIFTI format. For each subject, images were motion-corrected and realigned to the first image of the first run of the session using the AFNI program 3dvolreg. Functional images were then smoothed with a 5-mm full-width at half-maximum Gaussian kernel. All statistical analyses were performed on these smoothed and realigned images.
Each subject’s high-resolution anatomical MP-RAGE scan was normalized to Montreal Neurological Institute (MNI) stereotaxic space with a non-linear transformation using FSL programs FLIRT and FNIRT. An additional six-parameter rigid-body registration between each subject’s mean functional image, derived from the first function run, was performed to derive a transformation between each subject’s native EPI space and the normalized template space. Spatial normalization for the purpose of random effects group analyses was carried out on individual t-statistic maps generated from regression analyses performed on native space EPI time series data.
Functional Magnetic Resonance Imaging Statistical Analysis
Single-subject regression modeling was carried out using the AFNI program 3dDeconvolve. Each of the 10 repetition conditions [VR, AR × LAG(1,2,4,8,16)] was modeled as a separate regressor generated by convolving a gamma function with a binary vector indicating the event onset times for each condition (1 indicating event onset, 0 otherwise). Regressors for novel trials were generated in the same manner. An additional set of nuisance regressors (constant term plus linear, quadratic, and cubic polynomial terms) were included for each scanning run to model low-frequency noise in the time series data. Statistical contrasts at the single subject level were computed as weighted sums of the estimated beta coefficients divided by an estimate of the standard error, yielding a t-statistic for each voxel in the image volume. Random effects group analyses were then carried out on the spatially normalized single subject t-statistics using repeated measures analysis of variance (ANOVA) for hypotheses involving more than one level and with a one-sample t-test (against 0) for simple hypotheses.
The same analysis stream was also applied to the working memory localizer time series data. For the regression analysis, the task was divided in to three phases: encoding (5 s), delay (10 s), and probe (2 s). These phases were modeled with three regressors, which were constructed by convolving a block impulse (with duration equal to the length of the respective task phase) with a standard gamma hemodynamic convolution function. Regression analysis with 3dDeconvolve produced beta estimates and corresponding t-statistics for each of the three task components (encoding, delay, and probe).
ROI Selection Procedure
To define region of interests (ROIs) for area Spt, we used peak activation coordinates derived from a meta-analysis of five fMRI studies, including 107 subjects, of verbal working memory maintenance (Buchsbaum et al., 2011a) to define the center of a spherical ROI (x = −51, y = −43, z = 20; search radius = 12 mm) in MNI space centered around area Spt. This spherical ROI was then used to constrain the search space for the definition subject-specific Spt ROIs derived from the independent working memory localizer acquired on the first scanning run for each of the subjects in the present study. Note that 12 mm represents the maximum allowable distance between the center of the ROI search space and the peak voxel in any of the individual subject images; the average distance is in practice substantially less than 12 mm for each of the three ROIs defined in this manner (see below).
As in previous work (Hickok et al., 2003; Buchsbaum et al., 2005), we defined Spt using a conjunction (Nichols et al., 2005) of the auditory encoding (auditory presentation of six letters) contrast and the delay period maintenance contrast [Z(encoding) > 2.55 ∩ Z(delay) > 2.55]. This contrast isolates the region of temporoparietal cortex that is responsive during perception of auditory–verbal stimuli and also shows sustained activity during covert verbal rehearsal. For each subject, we searched for the maximum voxel within 12 mm of the meta-analysis peak coordinate and then selected the top eight voxels in the connected neighborhood surrounding the maximum (mean MNI coordinates: −58, −38, 25). The average distance from the peak coordinate in the single subject ROI to the center of the search sphere was 4.1 mm (see Figure 5, left panel to see spatial distribution of the single subject ROIs). We then examined these ROIs overlaid on each subject’s high-resolution MRI to ensure that the selected voxels lay within the gray matter of the posterior planum temporale or planum parietale, and thus were consistent with the functional–anatomical definition of area Spt (see Hickok and Poeppel, 2007; Buchsbaum and D’Esposito, 2008).
The recency-sensitive portion of LIPC was identified using a similar procedure. A spherical ROI (radius = 12 mm) in the left LIPC using the peak activation for the conjunction of (negative) linear lag effects for the AR and VR conditions (ARlinear ∩ VRlinear). Within each subject the peak voxel within this spherical ROI was selected, and again the top eight voxels in the connected neighborhood surrounding the maximum were used to define the subject-specific ROI (mean MNI coordinates: −54, −61, 29). The average distance from the peak coordinate in the single subject ROI to the center of the search sphere was 5.5 mm (see Figure 5, middle panel).
The left posterior inferior parietal cortex (PIPC) ROIs were defined by first identifying the peak coordinate in the posterior parietal lobe in the full group for the baseline > (delay + encoding) contrast (one-sample t-test; p < 0.0001 uncorrected) on the verbal working memory maintenance task. This contrast identified regions that were less active during the encoding and maintenance of verbal information than during the baseline. A spherical search (12 mm) radius was again defined and the peak voxels within this area were identified for each subject and the eight values in the connected neighborhood were identified (mean MNI coordinates: −46, −72, 31). The average distance from the peak coordinate in the single subject ROI to the center of the search sphere was 7.7 mm (see Figure 5, right panel).
The above selection procedure produced ROIs that could vary in location across subject but were constrained by the peak coordinate at the group level; it therefore increased the functional homogeneity of the ROI at the subject level. The across-subject spatial scatter in ROI location is shown on an average cortical surface in Figure 5, which shows an anterior–posterior progression for the three ROIs: Spt to LIPC to PIPC.
Results
Behavioral Performance
Subjects rejected new items with a high level of accuracy [percent correct = 97.8%, SD = 2.2%, mean response time (RT) = 856.5 ms, SD = 137.5 ms]. VRs were correctly identified as “old” 83.1% of the time (SD = 11%), whereas ARs (lures) were correctly rejected on 94.5% of trials (SD = 3.4%). As expected, the false alarm rate for AR lures was greater (5.5%) than for new items (2.2%), a difference that was statistically significant, t(14) = 6.1316, p < 0.0001. A two-way within-subject ANOVA with percent correct as the dependent variable and repetition modality (VR/AR) and lag [LAG(1,2,4,8,16)] as independent variables was carried out. There was a main effect of repetition modality [F(1,14) = 14.27, p = 0.002], a main effect of lag [F(4,56) = 15.7, p < 0.0001], and a repetition modality by lag interaction [F(4,56) = 5.107, p = 0.001]. As can be seen in Figure 2, accuracy for VRs smoothly declined as a function of lag until lag 8, after which performance leveled off. In contrast, accuracy for ARs (plotted as false alarm rate in Figure 2) was best for lags 1 and 2, worst for lags 4 and 8, and intermediate at lag 16. This differential performance pattern as a function of lag accounts for the reliable repetition modality by lag interaction. A second two-way within-subjects ANOVA was also carried out for RT. Here there was no main effect of repetition modality [F(1,14) = 1.46], but there was a main effect of lag [F(4,56) = 9.1, p < 0.0001] and there was a condition by lag interaction [F(4,56) = 6.92, p = 0.0001]. As is apparent in Figure 2, the shape of the RT curve across lag differs in the VR and AR conditions. Trend analysis using linear and quadratic contrasts shows that for the AR condition, there was a significant quadratic trend [t(14) = −2.42, p < 0.03] and a non-significant linear trend [t(14) = 0.41, p = 0.41]. For the VR condition both linear [t(14) = 5.4, p < 0.001] and quadratic [t(14) = −3.99, p < 0.01] trends were significant. A direct contrast of linear trends (VR–AR) was also significant [t(14) = 4.13, p < 0.001]. Compared to previous results (e.g., Rubin et al., 1999) the increase in RT as a function of lag for the VR condition was atypically rapid, reaching a rough asymptote by lag 3.
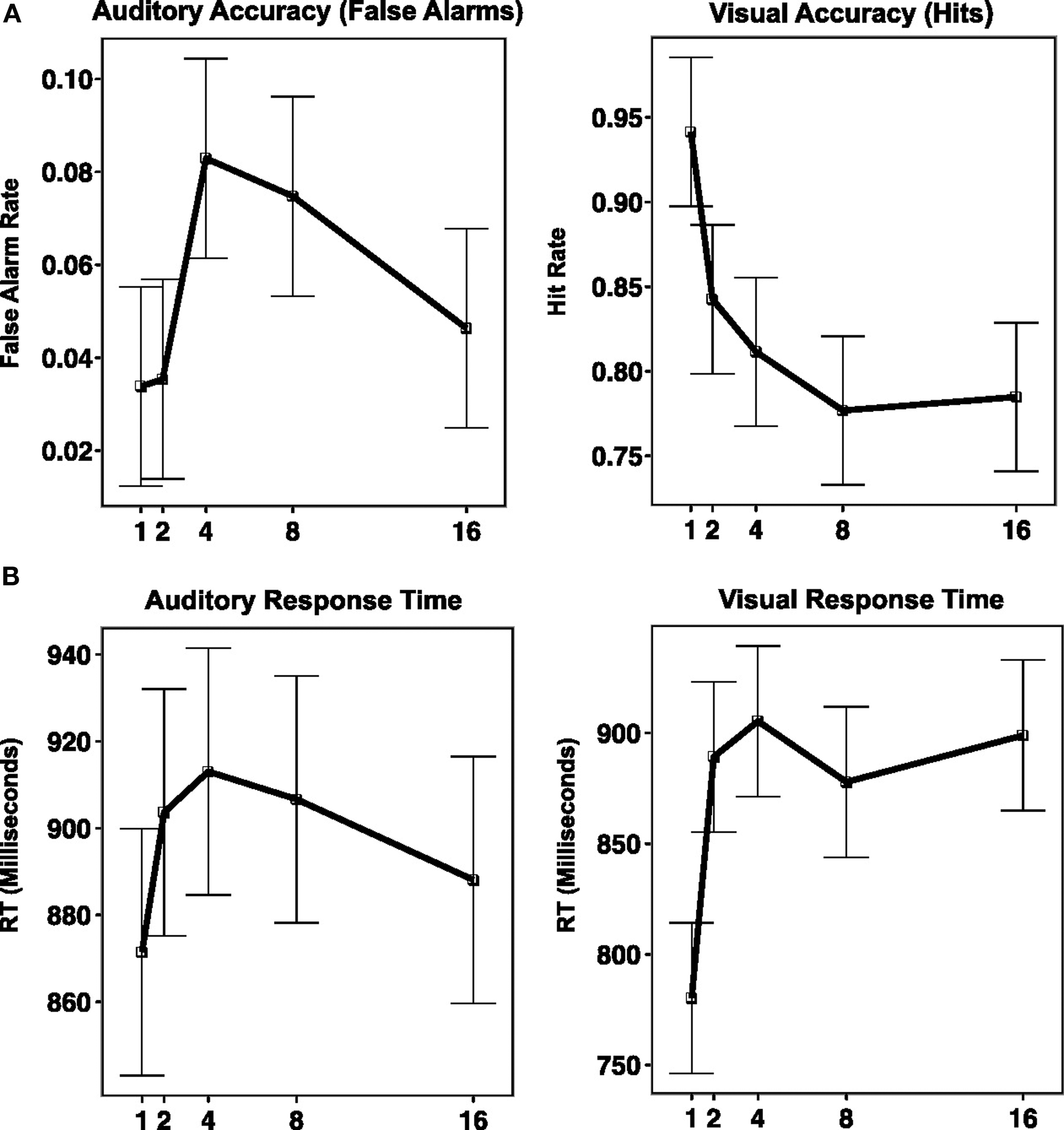
Figure 2. Behavioral performance on dual-modality continuous recognition task plotted against repetition interval (along x-axis, as LAG). (A) Graph of accuracy (along y-axis, measured as percent correct for VR condition, and percent false alarms for AR condition). (B) Graph of response time (along y-axis, measure in millisecond).
It is likely that this rapid increase in RT with lag occurred because of the two-fold increase in interference caused by the items from the irrelevant auditory stream.
fMRI Analyses
Effect of repetition lag for attended and ignored words
To replicate our previous findings (Buchsbaum and D’Esposito, 2009; Buchsbaum et al., 2011b) showing that activity in the LIPC declines with increasing lag during verbal recognition memory, we performed a one-sample t-test on the linear contrast of lag for the VR condition. We confirmed that the bilateral LIPC shows decreasing activity as a function of lag, whereas regions in the superior parietal lobe/IPS, the middle frontal gyrus (MFG) and the anterior insula showed increasing activity with lag (Figure 3). To test whether these patterns were also present for lure probes, we performed another one-sample t-test for the linear effect of lag in the AR condition (Figure 3B). We then calculated a conjunction map showing areas where both visual (VR) and auditory (AR) conditions revealed reliable lag-related trends that were in the same direction (e.g., both of them positive or both of them negative). The conjunction confirmed that the bilateral LIPC shows a negative effect of lag for both VR and AR conditions (see Figure 3C). Finally, to isolate regions that show different lag activation profiles depending on whether the item was a lure (AR condition) or a true positive (VR conditions), we examined the lag × repetition modality interaction using repeated measures ANOVA. This contrast revealed significant effects (false discovery rate = 0.05) in the bilateral MFG, the bilateral anterior insula, and the left IPS (see Figure 3D).
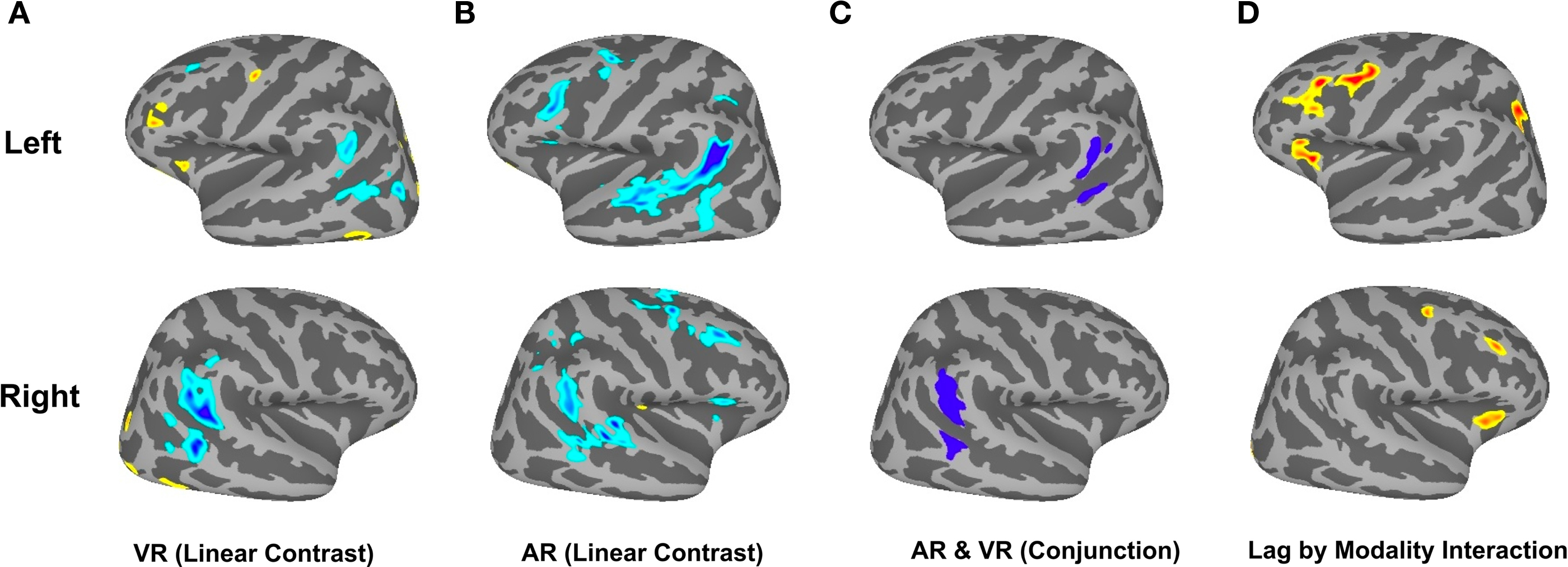
Figure 3. Brain Activation in Dual-Modality Continuous Recognition Task. (A) Shows linear effect of lag for the VR condition (cool colors indicate decreasing activation with increasing lag; warm colors indicate increasing activation with increasing lag). (B) Linear effect of lag for AR condition. (C) Conjunction of negative linear effect for AR and VR conditions. (D) Interaction of Lag and source modality.
To examine the precise pattern of effects in several of the regions showing either lag × repetition modality interaction or in the conjunction of (negative) linear trends across lag (ARlinear ∩ VRlinear), we extracted the top eight contiguous voxels surrounding the coordinate of maximal activation in the relevant group contrast map (Figure 4). These ROIs were then used to select voxels from the individual t-statistic contrast maps in each subject and for all of the experimental conditions (e.g., all combinations of lag and repetition modality – 2 × 5 = 10 conditions). The mean value for each condition was then computed (Masson and Loftus, 2003) and plotted with standard errors for each ROI in Figure 4. The purpose of this ROI extraction was not for hypothesis-testing but rather to show the shape of parametric effect of lag across as a function of repetition modality. Five ROIs – bilateral MFG, bilateral anterior insula, and the left IPS – were selected from the lag × source modality group level interaction map, while two ROIs (bilateral LIPC) were taken from the lag conjunction analysis (negative linear effect of lag; ARlinear ∩ VRlinear). As one can see from Figure 4, there is a similar pattern of effects for the interaction ROIs, where there is a negative trend (flattening out at about lag 8) for the AR condition and nearly a inverted effect for the VR condition – except that in the VR conditions, activation changes more precipitously, flattening out in its upward course as early as lag 2 for the MFG and IPS ROIs. In contrast, LIPC activation patterns for the VR and AR conditions have the same general form, with maximal activity at lag 1, which flattens out at approximately lag 4 or 8. In summary, the ROI plots show that while the bilateral LIPC region tracks recency independent of whether the probe is a hit (VR) or a lure (AR), the anterior insula, IPS, and MFG show an effect whereby the pattern of activity as a function of lag sharply diverges depending on the status of the memory probe.
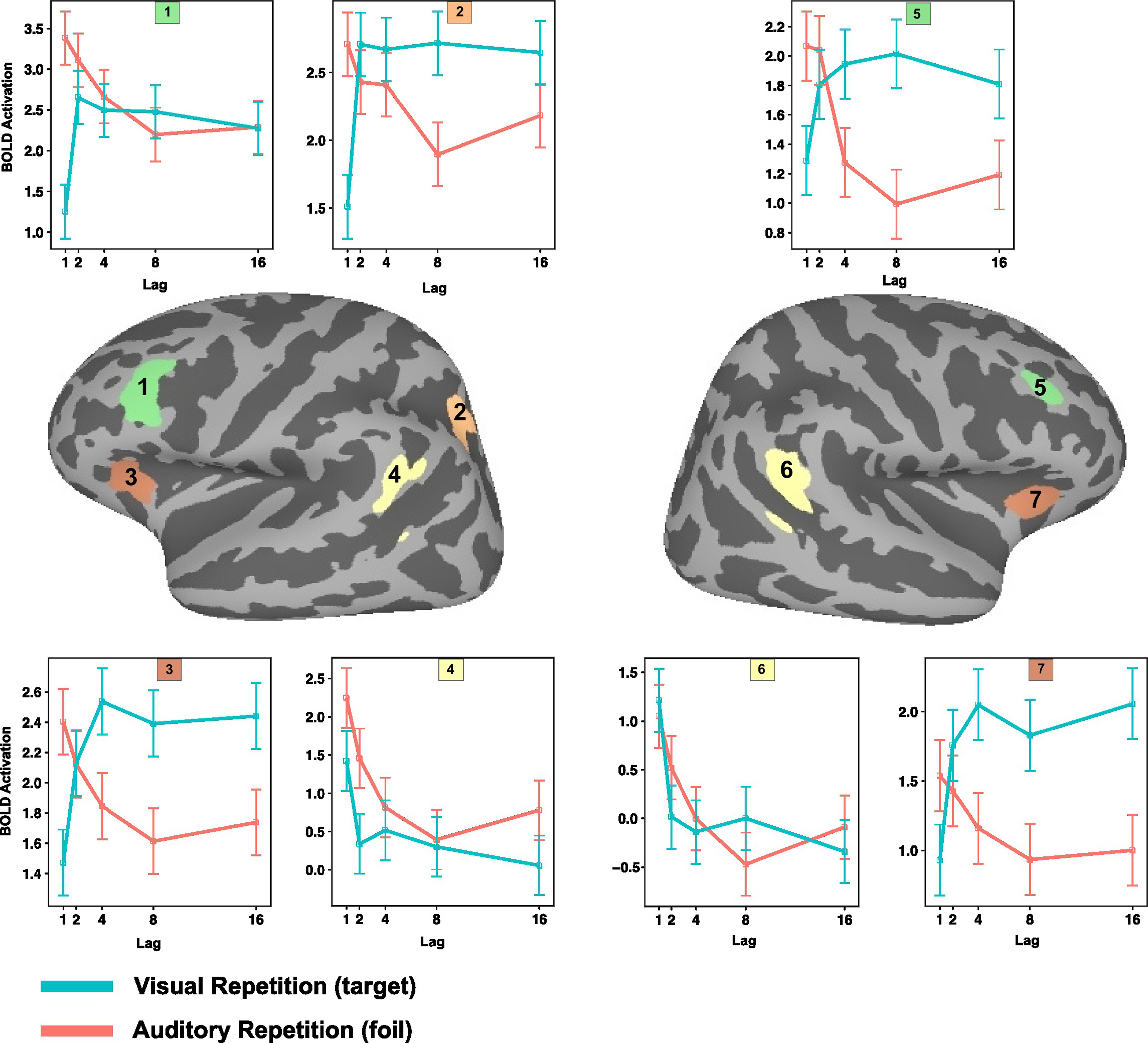
Figure 4. Mean level of activation as a function of lag and source modality in selected regions of interest (1–7). 1. Left dorsolateral prefrontal cortex. 2. Left intra parietal sulcus. 3. Left anterior insula. 4. Left inferior parietal lobe. 5. Right dorsolateral prefrontal cortex. 6. Right inferior parietal lobe. 7. Right anterior insula.
Comparison of activation in Spt and IPL during verbal maintenance and recognition memory
A second key aim of this study was to establish whether area Spt, a region that has repeatedly been shown active during maintenance of verbal information (Hickok et al., 2003; Buchsbaum et al., 2005; Buchsbaum and D’Esposito, 2008; Koelsch et al., 2009), also shows differential activity as a function of lag during recognition memory; and, likewise, to establish whether the LIPC, which shows lag effects during recognition memory, is also active during verbal working memory maintenance. The third goal was to establish whether the location of the lag-sensitive LIPC region area is contained by, or overlaps with, the area of the PIPC that commonly deactivates during cognitive tasks and is considered part of the “default network.” To answer these questions, the following strategy was adopted. ROIs were defined for the three regions in a manner that allowed for some spatial variation in the location of peak activation across subjects (see Materials and Methods for details). These ROIs were then used to extract time courses for the verbal working memory task and the pattern of lag effects for the recognition memory task, respectively. ROIs for Spt and PIPC (default network node) were defined on the basis of activation in the working memory maintenance task, while the LIPC ROI was defined using the lag contrast in the recognition memory task. This ROI-selection strategy allowed for an unbiased assessment of activation for the task that was not used to define the ROI. For the purposes of symmetry and quality assurance, however, we extracted data and displayed activation patterns (Figure 5) for both the biased (ROI-defined) and unbiased ROI data. We only report statistics, however, for data extracted using an ROI defined on an independent data set.
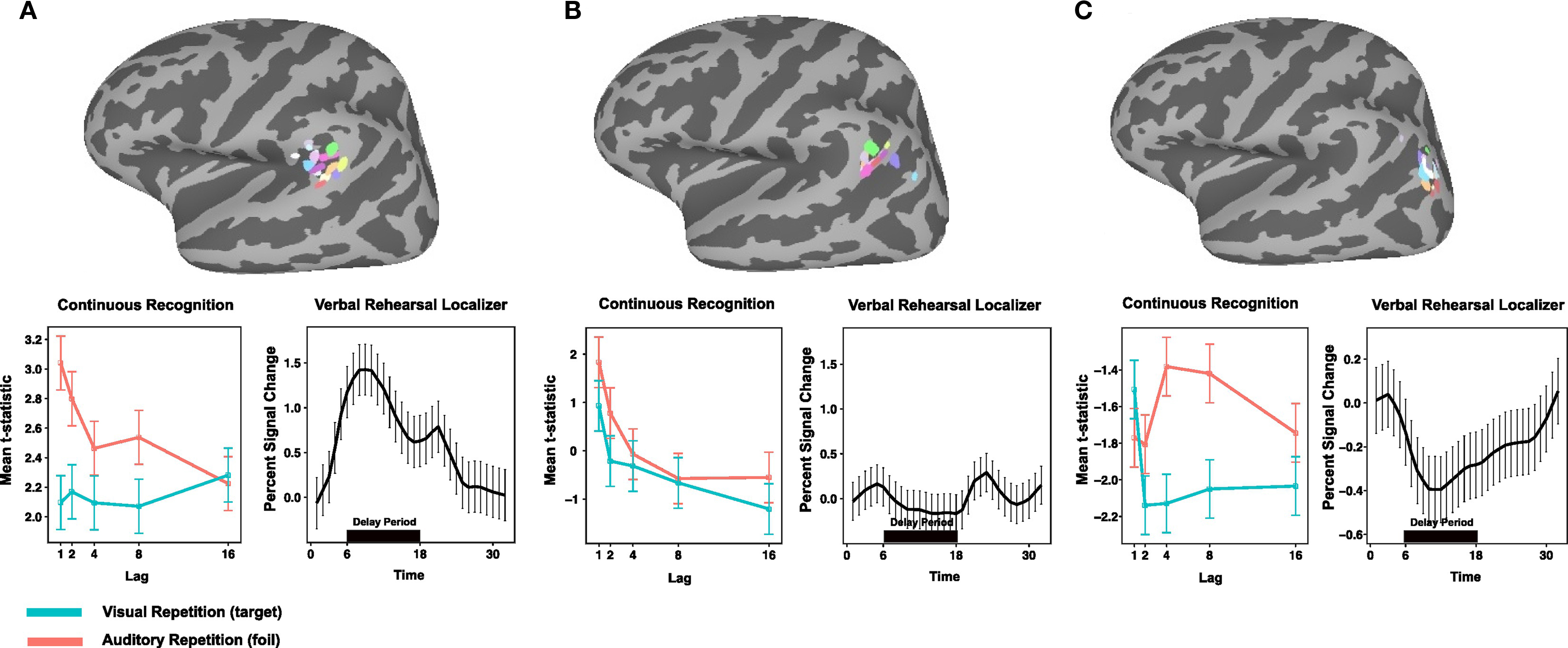
Figure 5. Comparison of Spt, LIPC, and PIPC for during verbal working memory maintenance and recognition memory. (A) Top, peak activation coordinates in the posterior planum temporale (Spt) for 14 subjects during delay period of verbal working memory localizer. Bottom, left: Activation plotted as a function of lag for AR and VR conditions in dual-modality recognition memory task for each ROI; right, time course of activation during verbal working memory maintenance task for each ROI. (B) Top, peak activation coordinates for conjunction of negative linear effects (ARlinear ∩ VRlinear) in the left LIPC. (C) Top, peak activation coordinates for PIPC region of task deactivation during encoding and delay periods of the verbal working memory localizer task.
Pattern of effects in Spt, LIPC, and PIPC ROIs
ROI analyses were conduction using a selection procedure (see Materials and Methods) that used a peak coordinate defined from a group analysis in MNI space to constrain the search (within a 12-mm radius) for subject-specific activation clusters. The end result was the definition of three sets of ROIs across the group: Spt, the (recency-sensitive portion of) left LIPC, and the PIPC. The location of these ROIs and the subject-to-subject variability can be seen on the three cortical surface maps depicted in the top row of Figure 5.
Using the Spt ROIs to examine activation as a function of lag (see Figure 5, bottom left) during recognition memory reveals a marginally significant lag effect for the AR condition [F(2,54) = 2.97, p = 0.059; ANOVA with lag parameterized with linear and quadratic trends] but not for the VR condition (p > 0.08); while the lag by modality interaction failed to reach significance [F(2,54) = 2.17, p = 0.12]. Area Spt showed sustained delay-period activation during working memory maintenance – a pattern that reflects the fact that the ROIs were selected on the basis of the delay-period contrast (see Figure 5, bottom, second graph from left). These findings show that when Spt, defined by a working memory maintenance localizer, is used to examine lag effects on the main recognition memory experiment, there is evidence for a small recency effect for the AR condition only. This pattern diverges from the corresponding pattern in LIPC, which shows strong and consistent recency effects across both VR and AR conditions.
The second question was whether the lag-sensitive portion of the LIPC, as defined on the recognition memory task, would show delay-period activity on the verbal working memory maintenance task. As can be seen in Figure 5, the LIPC showed no delay-period activity [t(13) = −1.977, p = 0.07] during working memory maintenance. It was, however, reliably active during the presentation of the probe item in the recognition phase of the working memory task [t(13) = 3.71, p = 0.002]. The results confirmed that the LIPC, which shows a recency effect in verbal recognition memory, does not activate during the delay period of a typical verbal working memory maintenance task.
Lastly, we examined whether the region of the PIPC that deactivated (e.g., baseline > task) during the working memory task showed an effect of lag in the recognition memory task. The goal of this analysis was to assess the extent to which the recency effects observed in the recognition memory tasks might be trivially explained as a modulation of the “default state” such that greater activation would be observed for shorter lags merely because they required less attention or cognitive load and therefore gave rise to more rest-related, or default, activity. As can be seen in Figure 5 (bottom, right), the pattern of activation in the PIPC default node does not show a main effect of lag as is evident in the LIPC ROI; rather, there is a cross-over interaction [ANOVA, lag × modality; F(2,54) = 3.3517, p = 0.014] such that activity for visual items is maximal at lag 1, drops precipitously at lag 2, and levels off thereafter. In contrast, for auditory items, the activation pattern is U-shaped and maximal at lag 4 (the most “difficult” condition in the AR condition as measured by accuracy – with approximately 8% false alarms; see Figure 2). We conclude therefore that the lag effect observed in the LIPC, which shows a recency effect for both target and lure probes, has a distinct functional profile from that observed in the nearby PIPC region that showed task-related deactivation for the working memory localizer.
Discussion
In the present study we have shown that activity in the inferior parietal lobe is modulated by short-term recency in a manner that cannot be explained by “task difficulty” alone. We further showed that that this recency-sensitive part of the LIPC is functionally and anatomically dissociable from the adjacent temporoparietal region (area Spt; Hickok et al., 2003; Buchsbaum et al., 2005) that has been consistently associated with covert verbal rehearsal and phonological short-term memory. Lastly, we have shown that both of these regions (LIPC and Spt) are functionally and anatomically dissociable from a third, more posteriorly situated, parietal area (PIPC) that typically deactivates during effortful cognitive tasks and is a core member of the “default” network. In the following sections, we discuss each of these related findings in more detail.
Parietal Lobe Recency Effects are Independent of Task Difficulty and Response Type
A number of previous studies have shown that activity in the LIPC is strongly correlated with item recency in the context of recognition memory (Dudukovic and Wagner, 2007; Buchsbaum and D’Esposito, 2009; Buchsbaum et al., 2011b; Greve et al., 2010; Oztekin et al., 2010). In light of the correlation between item lag and general task difficulty – i.e., subjects are faster and more accurate at correctly recognizing an immediately repeated item as old than in so identifying an item that was presented a minute ago (Rubin et al., 1999) – as well as the apparent proximity of this inferior parietal region to a canonical member of the default network, we sought to dissociate mnemonic recency from general task difficulty. The dissociation was achieved by adding an irrelevant stream of auditory items that would occasionally reappear (as lures) in the relevant visual stream. Thus, items that had previously been presented in the irrelevant channel might be assumed to give rise to interference and conflict, requiring an increase in the deployment of cognitive control processes and increase in behavioral indices of task difficulty. Indeed, that is precisely the pattern we found. While accuracy and RT for VRs showed a pattern of decreasing accuracy and increasing RT as a function of lag (although roughly plateauing at lag 3), repetitions from the unattended stream showed a U-shaped (or inverted U-shape, if plotted as proportion of false alarms) pattern for accuracy and an inverted U-shaped curve for RT. Thus, the relationship between lag and difficulty reliably differed between the VR and AR conditions. Despite the behavioral difference between the AR and VR conditions, the pattern of activity as a function of lag in the LIPC was nearly identical, suggesting that the nature of the signal in this area is genuinely associated with item recency, as opposed to mere item difficulty. If the activation in LIPC were driven purely by task difficulty we would have expected a pattern that tracked accuracy and RT (which themselves had corresponding profiles for both VR and AR conditions) – that is, a U-shaped pattern for the AR condition and a decreasing function for the VR condition.
The lag by repetition modality interaction effect observed in the MFG, anterior insula, and IPS for the two probe conditions suggests that the strong memory signal associated with recent lures (e.g., lags 1 and 2) needed to be overridden through the action of prefrontal and parietally mediated control processes, such as source retrieval and/or interference resolution (Jonides and Nee, 2006; Badre and Wagner, 2007; Kuhl et al., 2008; Raye et al., 2008). Furthermore, we have shown that the recency-sensitive portion of the LIPC is functionally and anatomically distinct from the more posteriorly situated region of parietal cortex that deactivated during the working memory maintenance task. While this region did show a recency effect that resembled the corresponding pattern in LIPC for VR items, it did not show a recency effect for AR items. Thus, activation in this region did not consistently covary with recency as it did in the LIPC.
Expectancy, Distinctiveness, and Memory Strength
Although the current data seem to eliminate a trivial task-difficulty explanation of recency effects in the LIPC, some question remains as to the interpretation of the apparent memory-related effects observed in this area. For instance, while some authors have suggested that activation in the LIPC is a direct index of memory strength – either as a multimodal representational space (or “episodic buffer”; Vilberg and Rugg, 2009b) or as a signal accumulator in the context of recognition decisions (Wagner et al., 2005) – other, less mnemnocentric, accounts have recently appeared. O’Connor (O’Connor et al., 2009) used a Posner cueing paradigm adapted for a recognition memory to show that activation in both the angular gyrus (AG) and supramarginal gyrus (SMG) of the LIPC, is more sensitive to whether a person expected an item to be old or new than whether the item actually was old or new. Thus, despite the many studies showing a relation between LIPC and some measure of “retrieval success” (Hutchinson et al., 2009), it is possible that these findings can be explained by task expectancies and the relative salience of a perceptual or mnemonic event. For instance, even in simple visual target detection tasks that lack an explicit recognition memory component, activity in the AG is often found during the detection of an oddball (McCarthy et al., 1997; Downar et al., 2001), an invalidly cued spatial target (Doricchi et al., 2010), or a deviant event embedded in a learned sequential pattern (Bubic et al., 2009, 2010). The results of the present study may also be interpreted from the standpoint of expectancy. Although all “lag” conditions in the continuous recognition task were approximately equiprobable from trial to trial, subjects may have adopted a task set that was optimal for the discrimination of old items with a weak memory strength (e.g., lag 4 or greater) from new items. Such an orientation would treat high strength items – lags 1 or 2 – as “deviant” events that require a temporary suspension of the default response orientation, and perhaps a reallocation or shifting of attention to active (active due to their recency) memory representations (Cabeza et al., 2008; Ciaramelli et al., 2008). Indeed, a recent study by Huijbers et al. (2010) that used a continuous recognition memory task showed in a similar parietal area (MNI: 45, −66, 24) and using lags (1,2,3,4,7,15, and 31) that activity was maximal for early lags (1,2,3) and late lags (15,31). This “V-shaped” pattern differs from the decreasing pattern we found, however, but might be explained by assuming that the shortest and longest lag events were most “deviant” and therefore constituted violations of expectancy.
The tendency of subjects to adopt the default expectation that items will be “weak” (longer lag or new) as opposed to strong (recent or short lag) is compatible with the idea that memory strength in tasks with serial presentation is to a great degree determined by the “temporal distinctiveness” of a memory representation (Glenberg and Swanson, 1986; Brown et al., 2007). As stimuli in a memory list recede in time, the relative discriminability between two items that appeared in close succession tends to decrease as a logarithmic function of their respective temporal distances (Crowder, 1976; Brown et al., 2007). We note that mean accuracy and mean level of activity in the LIPC both declined non-linearly as a function of lag, a finding that is compatible with the temporal ratio formulation of the decline in memory strength. Thus, the exponential decline in memory strength as a function of lag produces a skewed distribution of memory strength, where lags 1 and 2 are effectively outliers and lags 4 and above are increasingly indistinct from one another other. From the standpoint of subjective memory strength, then, very recent items are much more distinctive – and therefore may lead to the kind of expectation violation that has previously been associated with activation in the LIPC.
If the observed recency effects observed in the present study reflect a violation in “expectedness” as opposed to something inherent to the representation of memory (O’Connor et al., 2009), then examination of activity in the LIPC in tasks that do not involve variation in task expectancy would be revealing. For instance, if activity in the LIPC is greater for more recent items because of its role in memory storage, i.e., as an “episodic buffer” (Vilberg and Rugg, 2009a), then one might expect the LIPC to be engaged during the online maintenance of information in memory. To that end, we used an LIPC ROI defined as showing recency effects during the continuous recognition task to test whether the same functional region is also active during verbal memory maintenance. The result was clear: the LIPC showed no activation during the maintenance of verbal information over a 12-s delay. This finding is difficult to reconcile with the idea that the LIPC plays a role in the temporary storage of retrieved information. One could, of course, counter that the LIPC will only be involved in the buffering of information that has been specifically retrieved from long-term memory. This does not, however, answer why the LIPC shows large recency effects in a task such as the current one in which memory was tested as early as 3 s after initial stimulus presentation. Taken together, the evidence from the present study and others suggests that the LIPC is unlikely to be involved in the direct representation of the content of memory – as would be implied by the concept of a buffer – but nevertheless may reflect or otherwise index mnemonic signals that arise in the context of decision-making tasks such as recognition memory. After all, even if LIPC activity was entirely due do violations of expectancy, such violations could not be categorized as such without access to some quantitative measure of memory strength. In other words, the expectancy account of recency effects in the LIPC cannot avoid the necessity of the existence of an input signal, perhaps from sensory-perceptual regions, indicating mnemonic strength (or recency) of the current item – otherwise, how would the region distinguish unexpected (recent items) from expected events?
Finally, we showed that the temporoparietal region most often associated with phonological short-term maintenance, area Spt in the posterior planum temporale, is functionally and anatomically dissociable from the region of the LIPC that is sensitive to recency in short-term recognition memory. We further showed that area Spt, which was defined using a verbal working memory maintenance localizer, showed a recency effect on the recognition memory task, although this effect was modest and only present in the auditory modality. This is consistent with our previous finding of increased sensitivity in this area only for the recognition of auditory items in a bi-modal continuous recognition memory task (Buchsbaum and D’Esposito, 2009). These results show that while area Spt seems to be involved in both verbal maintenance and recognition memory the LIPC does not appear to be important for at least a particular type of verbal short-term memory tasks that stress maintenance and subvocal rehearsal.
Conflict of Interest Statement
The authors declare that the research was conducted in the absence of any commercial or financial relationships that could be construed as a potential conflict of interest.
References
Badre, D., and Wagner, A. D. (2007). Left ventrolateral prefrontal cortex and the cognitive control of memory. Neuropsychologia 45, 2883–2901.
Brown, G. D., Neath, I., and Chater, N. (2007). A temporal ratio model of memory. Psychol. Rev. 114, 539–576.
Bubic, A., von Cramon, D. Y., Jacobsen, T., Schroger, E., and Schubotz, R. I. (2009). Violation of expectation: neural correlates reflect bases of prediction. J. Cogn. Neurosci. 21, 155–168.
Bubic, A., von Cramon, D. Y., and Schubotz, R. I. (2010). Prediction, cognition and the brain. Front. Hum. Neurosci. 4:25. doi: 10.3389/fnhum.2010.00025
Buchsbaum, B. R., and D’Esposito, M. (2008). The search for the phonological store: from loop to convolution. J. Cogn. Neurosci. 20, 762–778.
Buchsbaum, B. R., and D’Esposito, M. (2009). Repetition suppression and reactivation in auditory–verbal short-term recognition memory. Cereb. Cortex 19, 1474–1485.
Buchsbaum, B. R., Baldo, J., Okada, K., Berman, K. F., Dronkers, N., D’Esposito, M., and Hickok, G. (2011a). Conduction aphasia, sensory-motor integration, and phonological short-term memory – an aggregate analysis of lesion and fMRI data. Brain Lang. doi: 10.1016/j.bandl.2010.12.001. [Epub ahead of print].
Buchsbaum, B. R., Padmanabhan, A., and Berman, K. F. (2011b). The neural substrates of recognition memory for verbal information: spanning the divide between short- and long-term memory. J. Cogn. Neurosci. 23, 978–991.
Buchsbaum, B. R., Olsen, R. K., Koch, P., and Berman, K. F. (2005). Human dorsal and ventral auditory streams subserve rehearsal-based and echoic processes during verbal working memory. Neuron 48, 687–697.
Cabeza, R., Ciaramelli, E., Olson, I. R., and Moscovitch, M. (2008). The parietal cortex and episodic memory: an attentional account. Nat. Rev. Neurosci. 9, 613–625.
Ciaramelli, E., Grady, C. L., and Moscovitch, M. (2008). Top-down and bottom-up attention to memory: a hypothesis (AtoM) on the role of the posterior parietal cortex in memory retrieval. Neuropsychologia 46, 1828–1851.
Cox, R. W. (1996). AFNI: software for analysis and visualization of functional magnetic resonance neuroimages. Comput. Biomed. Res. 29, 162–173.
Davelaar, E. J., Goshen-Gottstein, Y., Ashkenazi, A., Haarmann, H. J., and Usher, M. (2005). The demise of short-term memory revisited: empirical and computational investigations of recency effects. Psychol. Rev. 112, 3–42.
Doricchi, F., Macci, E., Silvetti, M., and Macaluso, E. (2010). Neural correlates of the spatial and expectancy components of endogenous and stimulus-driven orienting of attention in the Posner task. Cereb. Cortex 20, 1574–1585.
Downar, J., Crawley, A. P., Mikulis, D. J., and Davis, K. D. (2001). The effect of task relevance on the cortical response to changes in visual and auditory stimuli: an event-related fMRI study. Neuroimage 14, 1256–1267.
Dudukovic, N. M., and Wagner, A. D. (2007). Goal-dependent modulation of declarative memory: neural correlates of temporal recency decisions and novelty detection. Neuropsychologia 45, 2608–2620.
Glenberg, A. M., and Swanson, N. G. (1986). A temporal distinctiveness theory of recency and modality effects. J. Exp. Psychol. Learn Mem. Cogn. 12, 3–15.
Greve, A., Doidge, A. N., Evans, C. J., and Wilding, E. L. (2010). Functional neuroanatomy supporting judgments of when events occurred. J. Neurosci. 30, 7099–7104.
Hickok, G., and Poeppel, D. (2007). The cortical organization of speech processing. Nat. Rev. Neurosci. 8, 393–402.
Hickok, G., Buchsbaum, B., Humphries, C., and Muftuler, T. (2003). Auditory-motor interaction revealed by fMRI: speech, music, and working memory in area Spt. J. Cogn. Neurosci. 15, 673–682.
Huijbers, W., Pennartz, C. M., and Daselaar, S. M. (2010). Dissociating the “retrieval success” regions of the brain: effects of retrieval delay. Neuropsychologia 48, 491–497.
Hutchinson, J. B., Uncapher, M. R., and Wagner, A. D. (2009). Posterior parietal cortex and episodic retrieval: convergent and divergent effects of attention and memory. Learn. Mem. 16, 343–356.
Jonides, J., and Nee, D. E. (2006). Brain mechanisms of proactive interference in working memory. Neuroscience 139, 181–193.
Kayser, A. S., Buchsbaum, B. R., Erickson, D. T., and D’Esposito, M. (2010). The functional anatomy of a perceptual decision in the human brain. J. Neurophysiol. 103, 1179–1194.
Koelsch, S., Schulze, K., Sammler, D., Fritz, T., Muller, K., and Gruber, O. (2009). Functional architecture of verbal and tonal working memory: an FMRI study. Hum. Brain Mapp. 30, 859–873.
Kuhl, B. A., Kahn, I., Dudukovic, N. M., and Wagner, A. D. (2008). Overcoming suppression in order to remember: contributions from anterior cingulate and ventrolateral prefrontal cortex. Cogn. Affect. Behav. Neurosci. 8, 211–221.
Masson, M., and Loftus, G. (2003). Using confidence intervals for graphically based data interpretation. Can. J. Exp. Psychol. 57, 203–220.
McCarthy, G., Luby, M., Gore, J., and Goldman-Rakic, P. (1997). Infrequent events transiently activate human prefrontal and parietal cortex as measured by functional MRI. J. Neurophysiol. 77, 1630–1634.
McKiernan, K. A., Kaufman, J. N., Kucera-Thompson, J., and Binder, J. R. (2003). A parametric manipulation of factors affecting task-induced deactivation in functional neuroimaging. J. Cogn. Neurosci. 15, 394–408.
Nairne, J. S. (2002). Remembering over the short-term: the case against the standard model. Annu. Rev. Psychol. 53, 53–81.
Nee, D. E., and Jonides, J. (2008). Neural correlates of access to short-term memory. Proc. Natl. Acad. Sci. U.S.A. 105, 14228–14233.
Nichols, T., Brett, M., Andersson, J., Wager, T., and Poline, J. B. (2005). Valid conjunction inference with the minimum statistic. Neuroimage 25, 653–660.
O’Connor, A. R., Han, S., and Dobbins, I. G. (2009). The inferior parietal lobule and recognition memory: expectancy violation or successful retrieval? J. Neurosci. 30, 2924–2934.
Oztekin, I., Davachi, L., and McElree, B. (2010). Are representations in working memory distinct from representations in long-term memory?: neural evidence in support of a single store. Psychol. Sci. 21, 1123–1133.
Peterson, L. R., and Peterson, M. J. (1959). Short-term retention of individual verbal items. J. Exp. Psychol. 58, 193–198.
Portrat, S., Barrouillet, P., and Camos, V. (2008). Time-related decay or interference-based forgetting in working memory? J. Exp. Psychol. Learn. Mem. Cogn. 34, 1561–1564.
Raichle, M. E., MacLeod, A. M., Snyder, A. Z., Powers, W. J., Gusnard, D. A., and Shulman, G. L. (2001). A default mode of brain function. Proc. Natl. Acad. Sci. U.S.A. 98, 676–682.
Raye, C. L., Mitchell, K. J., Reeder, J. A., Greene, E. J., and Johnson, M. K. (2008). Refreshing one of several active representations: behavioral and functional magnetic resonance imaging differences between young and older adults. J. Cogn. Neurosci. 20, 852–862.
Rubin, D. C., Hinton, S., and Wenzel, A. (1999). The precise time course of retention. J. Exp. Psychol. Learn. Mem. Cogn. 25, 1161–1176.
Vilberg, K. L., and Rugg, M. D. (2009a). Functional significance of retrieval-related activity in lateral parietal cortex: evidence from fMRI and ERPs. Hum. Brain Mapp. 30, 1490–1501.
Vilberg, K. L., and Rugg, M. D. (2009b). Left parietal cortex is modulated by amount of recollected verbal information. Neuroreport 20, 1295–1299.
Wagner, A. D., Shannon, B. J., Kahn, I., and Buckner, R. L. (2005). Parietal lobe contributions to episodic memory retrieval. Trends Cogn. Sci. 9, 445–453.
Keywords: recognition memory, parietal lobe, working memory, recency effect, fMRI, serial position
Citation: Buchsbaum BR, Ye D and D’Esposito M (2011) Recency effects in the inferior parietal lobe during verbal recognition memory. Front. Hum. Neurosci. 5:59. doi: 10.3389/fnhum.2011.00059
Received: 15 October 2010; Accepted: 07 June 2011;
Published online: 15 July 2011.
Edited by:
Neal J. Cohen, University of Illinois, USAReviewed by:
Joel Voss, University of Illinois at Urbana-Champaign, USASander Daselaar, Duke University, USA
Copyright: © 2011 Buchsbaum, Ye and D’Esposito. This is an open-access article subject to a non-exclusive license between the authors and Frontiers Media SA, which permits use, distribution and reproduction in other forums, provided the original authors and source are credited and other Frontiers conditions are complied with.
*Correspondence: Bradley R. Buchsbaum, Rotman Research Institute, Baycrest Hospital, 3560 Bathurst Street, Toronto, ON, Canada M6A 2E1. e-mail:YmJ1Y2hzYmF1bUByb3RtYW4tYmF5Y3Jlc3Qub24uY2E=