- 1 Department of Psychology, Koç University, Istanbul, Turkey
- 2 Department of Cognitive, Linguistic and Psychological Sciences, Brown University, Providence, RI, USA
- 3 Brown Institute for Brain Sciences, Brown University, Providence, RI, USA
Proactive interference (PI), in which irrelevant information from prior learning disrupts memory performance, is widely viewed as a major cause of forgetting. However, the hypothesized spontaneous recovery (i.e., automatic retrieval) of interfering information presumed to be at the base of PI remains to be demonstrated directly. Moreover, it remains unclear at what point during learning and/or retrieval interference impacts memory performance. In order to resolve these open questions, we employed a machine-learning algorithm to identify distributed patterns of brain activity associated with retrieval of interfering information that engenders PI and causes forgetting. Participants were scanned using functional magnetic resonance imaging during an item recognition task. We induced PI by constructing sets of three consecutive study lists from the same semantic category. The classifier quantified the magnitude of category-related activity at encoding and retrieval. Category-specific activity during retrieval increased across lists, consistent with the category information becoming increasingly available and producing interference. Critically, this increase was correlated with individual differences in forgetting and the deployment of frontal lobe mechanisms that resolve interference. Collectively, these findings suggest that distributed patterns of brain activity pertaining to the interfering information during retrieval contribute to forgetting. The prefrontal cortex mediates the relationship between the spontaneous recovery of interfering information at retrieval and individual differences in memory performance.
Introduction
Why do we forget? Human memory is a remarkably powerful and efficient system for storing and retrieving information. But, we are, perhaps, most aware of our memory when it fails, and we find ourselves unable to remember a particular name, event, or fact. The reasons for such forgetting have long been a source of debate.
One major hypothesized cause of forgetting is proactive interference (PI). In general, interference refers to conditions in which the information one wants to retrieve is blocked, suppressed, or otherwise suffers from competition with other information also active in memory (Crowder, 1976; Anderson and Neely, 1996). PI refers to cases when prior learning interferes with the subsequent encoding and/or retrieval of newer information (Keppel and Underwood, 1962; Wickens, 1970; Gardiner et al., 1972; Watkins and Watkins, 1975; Tehan and Humphreys, 1996). Though viewed as a major source of forgetting, the nature of PI and the neural mechanisms by which it is resolved remain controversial. In particular, PI may diminish the quality of memory formation (i.e., encoding; Wickens, 1970; Watkins and Watkins, 1975), or alternatively disrupt subsequent retrieval (Gardiner et al., 1972; Crowder, 1976; Tehan and Humphreys, 1996). Whether PI is assumed to be a learning phenomenon, a retrieval phenomenon, or both affects theorizing about its mechanisms of action and resolution. Consequently, resolving this controversy is fundamental to our basic understanding of PI-induced forgetting.
Importantly, to date, the presence of interference and its effect on memory performance has been inferred from behavior (e.g., accuracy and/or response time measures) rather than demonstrated directly. This is because the hypothesized latent states that are the source of interference have not been accessible to measurement. Consequently, it has been difficult to directly demonstrate the presence of interference, its dynamics, at which stage of processing it is elicited, and how it is modulated by cognitive and neural mechanisms that help resolve its negative impact on memory performance.
Recently, Kuhl et al. (2011) demonstrated a relationship between the strength of retrieved information from memory and the degree of its reactivation assessed by multi-voxel pattern classification analysis (MVPA). Following a similar logic, we sought to elicit forgetting due to PI during fMRI scanning, and then index the degree to which interfering information is activated in the brain during encoding and retrieval using MVPA. During fMRI scanning, participants were shown lists of words, presented one at a time. Then, following a brief delay, participants decided whether a probe word was in the preceding list (“old”) or not (“new”). They then repeated the process again, receiving a new list of words followed by a delay and probe word. To investigate PI, we used the “release from PI” paradigm (Wickens, 1970; Watkins and Watkins, 1975), in which PI is induced by manipulating the semantic similarity of the consecutive word lists. In particular, consecutive list-delay-probe cycles were grouped into sets of three across which all words came from the same semantic category (e.g., animals). When items from the same category are studied for several consecutive lists, PI gradually builds up and leads to a decline in memory performance at the probe across the lists. Importantly, both the encoding and retrieval accounts of PI can offer an explanation for forgetting in this task.
At retrieval, PI might reduce the discriminability of items (Crowder, 1976). The common semantic category is increasingly evoked and available in memory because of its repetition over lists. But, it is “non-diagnostic” because it does not indicate whether an item was on a recent list. It follows that changing the category results in more efficient retrieval, as the target memory now has unique features that it does not share with other recent memories. As such, interference can be resolved during retrieval by engaging in controlled retrieval/selection operations that direct retrieval to item-specific, diagnostic information (Tehan and Humphreys, 1996; Badre and Wagner, 2005; Öztekin and McElree, 2007; Öztekin et al., 2009).
An alternative account is that PI affects the quality of encoding (Wickens, 1970; Watkins and Watkins, 1975). From this perspective, the repetition of the same semantic category results in the emphasis on the salient, but non-diagnostic feature (i.e., the semantic category) at the expense of the sufficient encoding of distinctive features of the individual target items, which results in a decline in memory performance (Chechile, 1987). Alternatively, an encoding account could also pose that the repetition of the semantic category might spontaneously elicit recovery of related items and thus render pattern separation more difficult due to the high degree of overlap in the context.
While there has been some indirect behavioral evidence supporting the contention that PI primarily affects retrieval (Gardiner et al., 1972; Tehan and Humphreys, 1996; Öztekin and McElree, 2007), there are also data to suggest it may affect encoding as well (Chechile and Butler, 1975; Chechile, 1987). To date, a direct demonstration of how PI builds up and leads to forgetting has not been provided. Accordingly, we sought to use MVPA to index the degree to which the interfering information that causes PI is represented in the distributed activity in the brain during encoding and retrieval stages.
A neural net classifier was trained to distinguish the 10 semantic categories employed in the experiment based on neural activation in the lateral temporal cortex (LTC). LTC has been previously implicated in storage and retrieval of semantic information (Damasio, 1990; Badre and Wagner, 2002; Thompson-Schill, 2003; Badre et al., 2005). Following previous research, the classifier was trained during encoding and was tested at retrieval. Importantly, the encoding and retrieval phases were separated by a 14-s distractor period, allowing us to independently estimate each phase. This served to test the amount of category-specific activity at retrieval, and track changes in the amount of category-related activity across levels of PI. On another iteration, the classifier was trained during the retrieval phase and tested at encoding. In addition, we investigated neural activation patterns in two regions that have been previously implicated during PI resolution in this specific paradigm; the left ventrolateral prefrontal cortex (lVLPFC), including the mid and anterior VLPFC subregions (Badre and Wagner, 2007), and the medial temporal lobe (MTL), including the hippocampus and the parahippocampal cortex (Öztekin et al., 2009).
Materials and Methods
Participants
Twenty-two right-handed adults (16 female, ages 18 to 29) participated in the experiment. Participants had normal or corrected-to-normal vision and were native speakers of English. All participants were screened for use of CNS affecting drugs, for psychiatric or neurological conditions, and for contraindications for MRI. Informed consent was obtained in accordance with the Research Protections Office at Brown University. Participants were compensated for their participation.
Design and Stimuli
Stimuli consisted of 21 instances of 10 semantic categories from the category norms of (Van Overschelde et al., 2004). The experiment consisted of eight 10-min runs. Each run contained 30 experimental trials, in which participants studied a five-item list, solved four math problems, and made a recognition judgment to a test word. PI was manipulated by employing a release from PI paradigm: words from the same semantic category were presented for three consecutive trials. Semantic categories were pseudo-randomly selected from the 10 categories, with the constraint that no category was repeated within a run.
Participants were tested with positive and negative probes equally often. Positive test probes were randomly chosen from the five members of the study list. Negative probes consisted of lures that were drawn from members of the same semantic category of the studied items, but had not been presented within the current run. That is, the negative probes used in the first run were novel, and in the following runs, a negative probe could be a word presented in the previous runs. Importantly, however, the likelihood of repetition of a word from a prior run was equal across the three repetitions of the same category within a run.
This design structure (illustrated in Figure 1A) yielded 80 experimental trials for each of the three lists (with 40 positive and 40 negative test trials in each list) upon completion of the experiment.
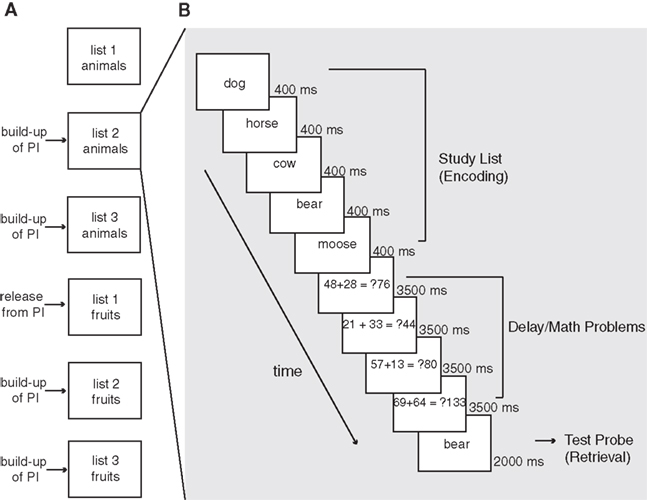
Figure 1. Illustration of the proactive interference (PI) manipulation (A) and an example trial sequence (B). Each trial proceeded with the presentation of a 5-word study list sequentially for 400 ms each. Following the fifth word, participants solved four math problems (3500 ms each) consisting of addition or subtraction of two randomly selected two-digit numbers. Participants indicated whether the solution presented next to the math problem was accurate by pressing either the middle or index finger on the button box. Following the fourth math problem, participants were presented with a test word and indicated whether the word was a member of the current study list. Participants had 2000 ms to respond to the test probe. Proactive interference was manipulated by employing a release from PI paradigm: words from the same semantic category were presented for three consecutive trials, during which we expected a decline in memory performance as a result of build up of PI. The category was switched on the upcoming trial, where performance is expected to return to its original level (i.e., release from PI).
Procedure
Figure 1B presents sequence of events within a single experimental trial. Each trial proceeded with the presentation of a 5-word study list sequentially for 400 ms each. Following the fifth word, participants solved four math problems consisting of addition or subtraction of two randomly selected two-digit numbers. Participants indicated whether the solution presented next to the math problem was accurate by pressing either the middle or index finger on the button box. Participants had 3500 ms to respond to each math problem. Following the fourth math problem, participants were presented with a test word and indicated whether the word was a member of the current study list. Participants had 2000 ms to respond to the test probe. The inter-trial interval consisted of presentation of a fixation cross on the center of the screen for variable duration (ranging from 0 to 8000 ms).
fMRI Protocol
Whole-brain imaging was performed on a Siemens 3T TIM Trio MRI system. Functional images were acquired using a gradient-echo echo-planar sequence (TR = 2 s; TE = 30 ms; flip angle = 90°; 35 axial slices, 3 mm × 3 mm × 3 mm with 0.6 mm interslice gap). Following the functional runs, high-resolution T1-weighted (MP-RAGE) anatomical images were collected for visualization. Head motion was restricted using firm padding that surrounded the head. Visual stimuli were projected onto a screen, and viewed through a mirror attached to a standard head coil.
Image Processing
Image processing and data analysis were performed using SPM21. Following quality assurance procedures to assess outliers or artifacts in volume and slice-to-slice variance in the global signal, functional images were corrected for differences in slice acquisition timing by resampling all slices in time to match the first slice, followed by motion correction across all runs (using sinc interpolation). Functional data were then normalized based on MNI stereotaxic space using a 12-parameter affine transformation along with a non-linear transformation using cosine basis functions. Images were resampled into 2-mm cubic voxels and then spatially smoothed with an 8-mm FWHM isotropic Gaussian kernel. Data for MVPA analyses underwent all of these preprocessing steps in addition to detrending to account for baseline shifts across runs, and for scanner drift across the entire session.
fMRI Data Analysis
Data analysis was conducted under the assumptions of the general linear model as implemented in SPM2. Separate regressors were generated for each condition [encoding for each of the three lists, distractor period (collapsed across lists), recognition probes for each of the three lists] and were modeled using a canonical hemodynamic response function and its temporal derivative. Data across runs were concatenated and modeled as one session with mean signal and scanner drift entered as covariates. For each participant, statistical effects were estimated using a subject-specific fixed-effects model.
Percent BOLD signal change in ROIs was derived using the MarsBaR region of interest toolbox for SPM2. The lVLPFC and the MTL ROIs were initially structurally defined. In light of previously observed functional dissociations between anterior and midVLPFC (see Badre and Wagner, 2007), separate anterior and midVLPFC ROIs were defined. Specifically, the anterior VLPFC ROI was restricted to the pars orbitalis portion of the left inferior frontal gyrus, located ventrally to the horizontal Sylvian ramus, and the midVLPFC ROI was restricted to the pars triangularis portion, located between the inferior frontal sulcus, the horizontal ramus of the Sylvius, and the ascending ramus of Sylvius. The MTL ROIs consisted of the hippocampus and the parahippocampal regions. The hippocampus region contained the dendate gyrus, the uncus, and the hippocampus proper. It was limited caudally by the parahippocampal ramus of the collateral fissure. The parahippocampal region contained the parahippocampal gyrus and parahippocampal uncus (including both the entorhinal and the perirhinal cortices), and was limited caudally by the parieto-occipital sulcus, and ventrally by the collateral sulcus (Tzourio-Mazoyer et al., 2002). Active voxels within these predefined anatomical boundaries from a contrast that assessed retrieval-based activation (i.e., probe phase greater than baseline) for each participant at an uncorrected threshold of p < 0.001. Each individual participant’s ROI was further restricted to the most active 20 voxels within each region. This approach provided unbiased estimates of fMRI signal change in these a priori hypothesized regions. Percent signal change (PSC) activation across participants was subjected to mixed-effect ANOVAs, treating Condition and Time (TRs) as repeated measures and Subjects as a random effect. These effects were followed by additional comparisons to reveal the statistical pattern across conditions. For these comparisons, the peak time point (point of maximum PSC) and the two adjacent time points (peak time point ±1 TR) were averaged to account for potential differences in time to peak across conditions.
Multi-Voxel Pattern Classifier Analysis
Multi-voxel pattern classifier analysis were carried out using a two-layer neural net classifier3 (see Norman et al., 2006, for a detailed overview). A classifier was trained to distinguish the 10 semantic categories used in the experiment based on the distributed activation in LTC. The LTC ROI was structurally defined, and consisted of the left middle and left inferior temporal gyri (see Figure 3A). The time courses from these voxels were normalized across runs to z scores. As feature selection step, voxels were further constrained by employing a one-way ANOVA that determined the voxels that exhibited an omnibus reliability across the 10 classification conditions (e.g., Polyn et al., 2005). Then, a set of regressors that assigned each functional scan to a particular classification condition (i.e., the specific semantic category) assuming a lagged hemodynamic response function was determined for each individual participant. Consistent with previous research (Polyn et al., 2005), onsets were shifted forward by three points to account for the hemodynamic response lag. The classifier was trained on the preprocessed imaging data. In one iteration, the classifier was trained from scans during the encoding phase (i.e., presentation of the study list) of trials from all lists and was then tested independently at retrieval (i.e., during the test probe presentation). On a second iteration, the classifier was trained during retrieval and tested at encoding. Training was achieved using a two-layer neural network consisting of k input units and m output units, where k equals the number of selected voxels within the temporal cortex ROI and m equals the number of conditions to be classified (i.e., the 10 semantic categories). Each input unit was connected to each of the 10 output units through a weighted feed-forward connection, thereby relating distributed activation across voxels to each semantic category. Weights were initialized randomly throughout the network and then adjusted over the course of training using a backpropagation algorithm that adjusts the weights to minimize prediction error. At test, scans from the test trials served as input to the classifier, and the classifier assigned each data point to one of the 10 semantic categories based on the highest activation value among output nodes.
For each participant, classifier’s success was determined by the proportion of correct category classifications across the test trials. After establishing that the classifier can successfully predict distributed neural activation pertaining to the correct semantic category, the magnitude of category-level activity – derived from the activation values that the classifier assigns for the correct category – was further assessed across the three lists where the same semantic category was presented. This served as an index of whether build up of PI could be tracked from the category-related distributed patterns of neural activation in the brain.
Results
Performance on the memory task was consistent with the build up of PI across lists of the same category. Memory accuracy was assessed via scaling each participant’s hit rates against false alarm rates to obtain (equal variance Gaussian) d’ measures. There was a reliable decline in accuracy across the three lists [F(2,21) = 8.86, p < 0.001; shown in Figure 2A]. Pair-wise comparisons indicated a significant decrease in memory performance at the probe from list 1 to lists 2 and 3 [t(21) = 2.57, p < 0.02; t(21) = 4.41, p < 0.01], and an increase in reaction time (RT), evident in a difference between lists 1 and 3 [t(21) = 2.09, p < 0.05; illustrated in Figure 2B]. There was a positive correlation between RT and False Alarm rates [r = 0.425, p < 0.049], ruling out a speed–accuracy trade-off account of the PI effect.
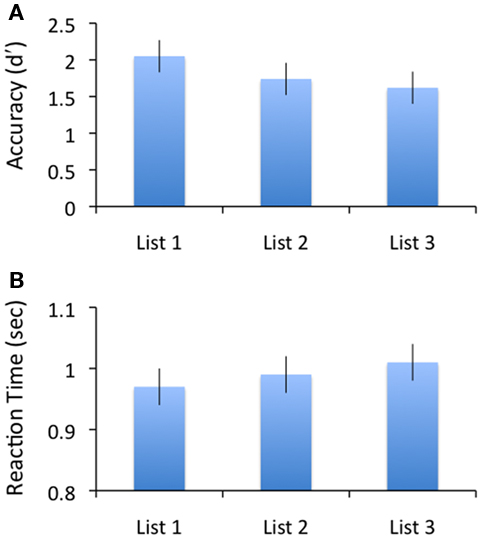
Figure 2. Illustration of the behavioral data. (A) Accuracy (measured in d’ units) across levels of PI. (B) Reaction time (in second) across levels of PI.
Average (across participants) classifier success, assessed as the proportion of correct classifications, was 0.215 (ranging from 0.155 to 0.265 across participants). This performance was better than chance (p < 0.001) in all participants as assessed via a non-parametric statistical test (see Polyn et al., 2005) that generates a null distribution of performance values by repeatedly generating scrambled versions of the classifier output.
We next examined whether the magnitude of the category-related neural activity changed as a function of PI across the three lists. At retrieval, the classifier’s estimate of the magnitude of category-related activity increased across the lists [F(2,21) = 21.32, p < 0.001], with a significant difference between List 1 vs. Lists 2–3 [t (21) = 5.96, p < 0.001]. Pair-wise comparisons indicated a significant increase from List 1 to List 2 [t(21) = 5.94, p < 0.001]. As was the case for accuracy, there were no reliable differences in category activity between List 2 and List 3 (shown in Figure 3A). Notably, rerunning the MVPA analyses employing a standard N-minus-one (leave-one-out) run-by-run cross-validation replicated the reported effects above, with a mean classification success of 0.19 (ranging from 0.16 to 0.24 across participants), and a reliable increase in classification success from List 1 to List 2 [t(21) = 2.26, p < 0.035]. Importantly, this analysis rules out a possible concern that the changes in classification success may be due to non-independence arising from the hemodynamic lag between encoding and retrieval in our experimental paradigm.
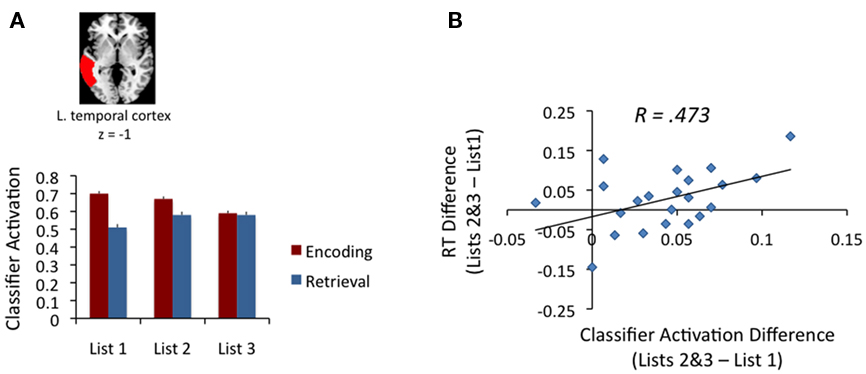
Figure 3. (A) Classifier’s estimates of the amount of category-specific neural activity at encoding (red bars) and retrieval (blue bars) across the three lists where PI builds up. The activity at encoding exhibit a decline across levels of PI during, while the activity at retrieval show an increase across PI. Hence, amount of category-specific activity at retrieval tracks the build of PI. (B) The relationship between the changes in category-specific neural activity during retrieval (i.e., the increase in category-related activity for Lists 2–3 compared to List 1) and the decline in memory performance (i.e., the amount of reaction time increase for Lists 2–3 compared to List 1) across individuals. The figure shows a reliable positive relationship, indicating that the increase in neural activity pertaining to the interfering information in the brain is directly linked to individual differences in the memory performance as a function of PI.
The encoding account predicts PI to build up during encoding via biasing encoding of the common category at the expense of more distinctive item-specific details or via the greater elicitation of related items in memory. Thus, the encoding account predicts category-specific activity to increase specifically during encoding as PI builds up. However, in contrast to retrieval, classifier weights during encoding exhibited a decline across the lists as PI built up [F(2,21) = 7.75, p < 0.001]4. The opposite pattern of classifier weights during encoding and retrieval was confirmed with a reliable Phase (Encoding vs. Retrieval) × PI interaction [F(2,21) = 15.28, p < 0.001; Figure 3A].
To assess the relationship between activation of the interfering semantic category items across phases and memory performance, the classifier success at encoding and retrieval was correlated with the RT interference effect across lists. At retrieval, the increase in RT as a function of PI (Lists 2–3 vs. List 1) was positively correlated with the corresponding increase in classifier’s estimate of the magnitude of category-related activity in LTC [r(21) = 0.473, p < 0.029; Figure 3B]. By contrast, there was no relationship between the level of category-specific activity during encoding and behavioral measures of forgetting (r = −0.01). The correlation with accuracy was not reliable during encoding or retrieval (r = 0.16 for retrieval, and r = −0.19 for encoding)5.
Considerable evidence has indicated that lVLPFC is critical for PI resolution (D’Esposito et al., 1999; Jonides et al., 2000; Thompson-Schill et al., 2002; Badre and Wagner, 2005; Feredoes et al., 2006). Moreover, a study investigating the release from PI task also implicated MTL activation in association with successful resolution of interference (Öztekin et al., 2009). Accordingly, we assessed PSC in neural activation levels in the lVLPFC, testing both anterior and midVLPFC subregions (see Materials and Methods), and hippocampal and parahippocampal regions (Figure 4). Among the subregions of lVLPFC, the anterior lVLPFC showed a reliable increase from Lists 1 to 3 [t(21) = 2.19, p < 0.04]. MidVLPFC exhibited a similar increase in activation levels across lists, but this effect did not reach statistical significance (p > 0.32). Within the MTL, both the hippocampus and the parahippocampal cortex showed a quantitative increase due to PI. However, this effect was reliable only within the parahippocampal cortex [t(21) = 2.04, p < 0.04 for parahippocampal cortex; p > 0.26 for hippocampus]. Accordingly, further analysis of retrieval-related effects in lVLPFC was restricted to left anterior VLPFC and in MTL to parahippocampal cortex.
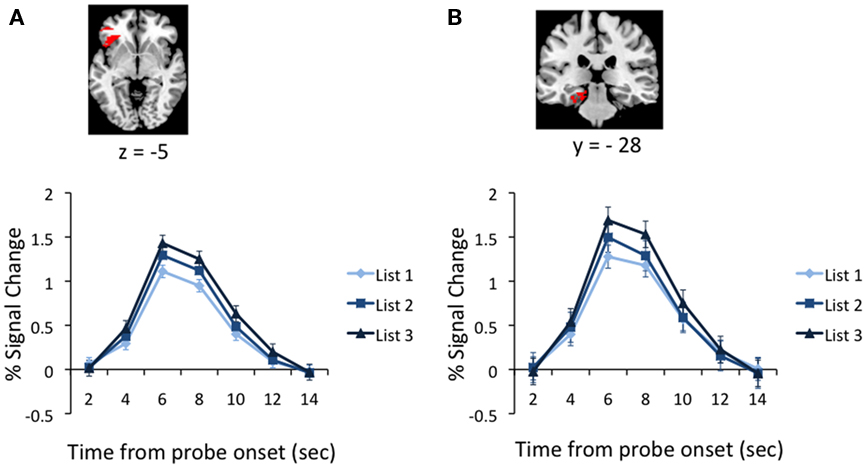
Figure 4. (A) Percent BOLD signal change in the left anterior ventrolateral prefrontal cortex during retrieval (starting from the recognition probe onset) across levels of PI. (B) Percent BOLD signal change in the left parahippocampal cortex during retrieval across levels of PI. Both figures indicate a reliable increase in neural activation in these regions as PI builds up, consistent with their role in resolving interference.
In contrast to retrieval, PSC during encoding in both left anterior VLPFC and parahippocampus exhibited a decline as PI built up. The decline from List 1 to Lists 2–3 was reliable in parahippocampal cortex [t(21) = 2.55, p < 0.019], and marginal in hippocampus [t(21 = 1.97, p < 0.062], but not anterior or midVLPFC (p > 0.169 for anterior VLPFC; p > 0.352 for midVLPFC). In addition, neural activation in the anterior VLPFC and the parahippocampal cortex predicted subsequent memory. That is, activation during encoding for subsequently correctly remembered trials was greater than incorrect trials [Fs > 8.6, ps < 0.01].
We next examined whether there is a relationship between neural activation during retrieval in left anterior VLPFC and individual differences in behavioral RT measures [r = −0.525, p < 0.01]. Thus, memory performance was better to the extent that the lVLPFC was engaged, consistent with the notion that this region plays a critical role in successfully resolving interference.
Having established a reliable correlation between amount of category-related activity and RT measures, as well as a reliable correlation with lVLPFC activation, we next evaluated whether lVLPFC mediates the relationship between the amount of interfering information elicited in the brain, and its resultant impact on memory performance (see Figure 5). Importantly, the relationship between category-specific activity and the resultant behavioral RT at retrieval were significantly mediated by the magnitude of activation in lVLPFC [Goodman test statistic = −1.96, p < 0.05]. That is the effect of category-specific activity on changes in RT measures were mediated by the degree to which lVLPFC was engaged.
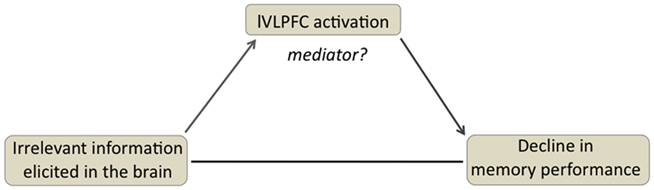
Figure 5. Illustration of the mediation analysis, testing the hypothesis that the left VLPFC mediates the relationship between the amount of interfering information elicited in the brain, and the corresponding decline in memory performance.
Discussion
The present results provide new insights into the nature of PI, both in terms of its build up during encoding and retrieval and its resolution by the prefrontal cortex. The current MVPA approach goes beyond prior work using strictly behavioral measures or standard univariate activation-based fMRI in that it permits us to measure changes in the availability of specific category information in the activation across voxels and to relate that availability to performance. Thus, using this approach, we provide the first demonstration of a direct relationship between patterns of brain activity that mark the activation of interfering representations during memory retrieval and individual differences in behavioral measures of forgetting. In addition, we provide evidence that lVLPFC may support interference resolution mechanisms that ameliorate its negative effects on remembering.
The present results provide direct evidence for spontaneous recovery of interfering information at retrieval as a source of PI. Specifically, we observed an increase in distributed patterns of activity representing the interfering category across lists. It is important to note that this increase is not due to any difference across lists in the probe itself, as a single word is always presented and the specific probe words are counterbalanced across individuals. This change in rate of classifier success is specifically related to the strength of the distributed category representation in the brain as a function of the encountering consecutive lists from the same category. Importantly, the increase in activity for the interfering category information during retrieval correlated with individual differences in behavioral measures of forgetting. Thus, these data are largely consistent with the retrieval discriminability account (Crowder, 1976).
Our results complement a recent study by Kuhl et al. (2011) that also employed MVPA in order to assess the relationship between the strength of information retrieved from memory and the degree of its reactivation. Their findings indicated lower reactivation values for target (to-be-retrieved) information were associated with an increased likelihood of competing memories to be later remembered. In the present study, we show that the increase in the reactivation of the common semantic category (which can be interpreted similarly to the competitors in Kuhl et al., 2011) was associated with the resultant decrease in memory performance. Thus, our results are consistent with and complementary to those reported in Kuhl et al. (2011).
It is notable that classifier success could be affected by both activation of semantic category information and specific item information (such as through successful retrieval of the associated list items). However, when our results are considered collectively, they suggest that changes in the availability of category information drives the classifier performance changes, at least as they relate to behavior. First, item recognition performance declined, while the classifier weights increased. Second, the increase in classifier’s estimate was correlated with the increase in response time measures. This relationship with behavior is counter to successful item-level retrieval. Finally, our mediation analyses rules out a possible alternative explanation suggesting that the slow down of retrieval might be arising from top down influence of signals from the prefrontal cortex. Rather, as discussed in more detail below, this analysis suggests that the engagement of the prefrontal cortex control mechanisms is deployed reactively to mitigate interference from category information.
In contrast to retrieval, the distributed representation of the interfering category decreased at encoding across study lists. One potential item-level account of this decrease is that repetition of lists from a common semantic category results in diminished attention or adaptation (i.e., repetition suppression). And indeed, repetition induced decreases in attention at encoding is one potential source of subsequent forgetting (c.f., Feredoes and Postle, 2010). Another category-level account, is that the decline reflects an active attempt to avoid attending to the common semantic category during encoding. However, the present experiment did not find a correlation between these decreases at encoding and subsequent behavioral markers of forgetting. Thus, unlike retrieval, the present data set did not point to conclusive evidence for the influence of PI at encoding. Future research would be useful in providing additional insight into the underlying theoretical mechanisms that lead to this decrease during encoding.
A second key set of findings in the present study concerned the role of lVLPFC in resolving interference. Considerable evidence has associated lVLPFC with resolution of competition during memory retrieval (Thompson-Schill et al., 1997; Badre et al., 2005; Badre and D’Esposito, 2007), including during elicitation of PI (Badre and Wagner, 2005; Jonides and Nee, 2006; Öztekin et al., 2009). Consistent with these previous findings, we observed an increase in lVLPFC as PI built up across lists, and activation in this region was negatively correlated with behavioral measures of forgetting. And, lVLPFC activation reliably mediated the relationship between the amount of interfering information represented in the brain during retrieval (i.e., classifier weights) and the resultant decline in memory performance.
These results extend an extensive literature implicating lVLPFC in the resolution of interference arising from episodic familiarity. In these studies, interference is induced using the recent probe paradigm in which a lure drawn from a previous study list engenders longer response times and/or higher false alarm rates (Monsell, 1978; McElree and Dosher, 1989; Öztekin and McElree, 2007). Neuroimaging studies have consistently implicated the lVLPFC during resolution of interference in this paradigm (Jonides et al., 1998, 2000; Postle and Brush, 2004; Badre and Wagner, 2005; Öztekin et al., 2009). Event-related fMRI has suggested that the lVLPFC may resolve familiarity-based PI at retrieval, consistent with the present results (D’Esposito et al., 1999). And, disruption of lVLPFC, either due to stroke (Thompson-Schill et al., 2002) transcranial magnetic stimulation, has been shown to impair PI resolution in the recent probes task.
Accounts of lVLPFC contributions to recency-based interference resolution have proposed that lVLPFC supports a domain general selection mechanism (e.g., Thompson-Schill et al., 1997) that operates via biased competition (Desimone and Duncan, 1995; Miller and Cohen, 2001; Badre and Wagner, 2006; Thompson-Schill and Botvinick, 2006). In these accounts, interference resolution operates at the level of retrieved episodic details (Badre and Wagner, 2005; Öztekin and McElree, 2007; Öztekin et al., 2009) or classification/response criteria (Jonides and Nee, 2006; Feredoes and Postle, 2010). Thus, the present results appear largely consistent with the extant literature on familiarity-induced PI, and perhaps speak to the domain generality of lVLPFC mechanisms (also see Öztekin et al., 2009).
However, it is also notable that the precise locus of activation in lVLPFC that was the focus of the present paper is rostral to that commonly observed during the recency paradigm. Specifically, previous work has consistently located activation in midlVLPFC (inferior frontal gyrus pars triangularis; ∼BA 45/44) during recency conditions of the episodic PI task. Badre and Wagner (2007) noted that this was consistent with the involvement of left midVLPFC in a wide range of tasks that require domain general selection to overcome competition in memory. In the present study, a quantitative effect of PI was observed in midVLPFC, but did not reach significance. Interestingly, however, the reliable PI effect in lVLPFC was observed rostral to midVLPFC, in the pars orbitalis subdivision of the inferior frontal gyrus (∼BA 47). Prior work has generally associated this anterior lVLPFC subregion with semantic processing, particularly under conditions in which cognitive control is required during retrieval (Poldrack et al., 1999; Wagner et al., 2001; Badre et al., 2005; reviewed in Badre and Wagner, 2007). The domain specificity (i.e., semantic-level) of anterior lVLPFC and its putative retrieval operation functionally distinguished this region from the domain general midVLPFC during episodic retrieval (Badre et al., 2005; Dobbins and Wagner, 2005; Badre and Wagner, 2007). Thus, the activation of anterior lVLPFC during the present PI task may reflect the semantic nature of the interference in this task; whereas the absence of a semantic component in the standard recency task may explain why this subregion is not typically observed during this task (though see Atkins and Reuter-Lorenz, 2011).
A second possibility is that interference in the present task may particularly necessitate controlled retrieval – at the item level – to a greater extent than the more widely studied recency paradigm. In particular, the release from PI paradigm requires directing retrieval and any strategic or elaborative processing to the item-level rather than the highly available, but non-diagnostic, common semantic category. Thus, controlled retrieval of weakly associated information with items may be a key demand in this task. This interpretation is partially supported by evidence from the application of the speed–accuracy trade-off procedure (e.g., Öztekin and McElree, 2007) that indicates interference effects during release from PI are resolved by slow retrieval processes required to bring to mind diagnostic recollective details. However, directed future research will need to further explore the contexts under which anterior and midVLPFC are engaged during PI resolution and to understand their dynamics of action with respect to anticipated vs. elicited control demands.
Conflict of Interest Statement
The authors declare that the research was conducted in the absence of any commercial or financial relationships that could be construed as a potential conflict of interest.
Acknowledgments
This research was supported by the National Institute of Neurological Disease and Stroke (R01 NS065046 awarded to David Badre), the Alfred P. Sloan Foundation (awarded to David Badre), and a Marie Curie International Reintegration grant (PIRG08-GA-2010-277016 awarded to Ilke Öztekin).
Footnotes
- ^http://www.fil.ion.ucl.ac.uk/spm/
- ^http://marsbar.sourceforge.net/
- ^http://www.csbmb.princeton.edu/mvpa/
- ^Rerunning this analysis with N-minus-one (leave-one-out) run-by-run cross-validation yielded consistent results, with a decline in classifier success across the lists during encoding, however this decline did not reach statistical significance (p > 0.13).
- ^There may be two explanations for the lack of a reliable relationship with accuracy. First, this could reflect differences in variance between RT and accuracy, and thus be a null result. Alternatively, the selective impact on RT could be related to the engagement of cognitive control and the fact that left VLPFC makes selection of the diagnostic details from memory more efficient, but given sufficient time, the correct response will be generated. This is potentially consistent with previous research that has indicated a reliable relationship between left VLPFC activation and RT measures, but not accuracy during resolution of PI in the release from PI paradigm employed in our study (e.g., Öztekin et al., 2009).
References
Anderson, M. C., and Neely, J. H. (1996). “Interference and inhibition in memory retrieval,” in Handbook of Perception and Memory, Vol. 10: Memory, eds E. L. Bjork and R. A. Bjork (San Diego: Academic Press), 237–313.
Atkins, A. S., and Reuter-Lorenz, P. A. (2011). Neural mechanisms of semantic interference and false recognition in short-term memory. Neuroimage 56, 1726–1734.
Badre, D., and D’Esposito, M. (2007). Functional magnetic resonance imaging evidence for a hierarchical organization of the prefrontal cortex. J. Cogn. Neurosci. 19, 2082–2099.
Badre, D., Poldrack, R. A., Pare-Blagoev, E. J., Insler, R. Z., and Wagner, A. D. (2005). Dissociable controlled retrieval and generalized selection mechanisms in ventrolateral prefrontal cortex. Neuron 47, 907–918.
Badre, D., and Wagner, A. D. (2002). Semantic retrieval, mnemonic control, and prefrontal cortex. Behav. Cogn. Neurosci. Rev. 1, 206–218.
Badre, D., and Wagner, A. D. (2005). Frontal lobe mechanisms that resolve proactive interference. Cereb. Cortex 15, 2003–2012.
Badre, D., and Wagner, A. D. (2006). Computational and neurobiological mechanisms underlying cognitive flexibility. Proc. Natl. Acad. Sci. U.S.A. 103, 7186–7191.
Badre, D., and Wagner, A. D. (2007). Left ventrolateral prefrontal cortex and the cognitive control of memory. Neuropsychologia 45, 2883–2901.
Chechile, R., and Butler, K. (1975). Storage and retrieval changes that occur in development and release of PI. J. Mem. Lang. 14, 430–437.
Damasio, A. R. (1990). Category-related recognition defects as a clue to the neural substrates of knowledge. Trends Neurosci. 13, 95–98.
Desimone, R., and Duncan, J. (1995). Neural mechanisms of selective visual attention. Annu. Rev. Neurosci. 18, 193–222.
D’Esposito, M., Postle, B. R., Jonides, J., and Smith, E. E. (1999). The neural substrate and temporal dynamics of interference effects in working memory as revealed by event-related functional MRI. Proc. Natl. Acad. Sci. U.S.A. 96, 7514–7519.
Dobbins, I. G., and Wagner, A. D. (2005). Domain-general and domain-sensitive prefrontal mechanisms for recollecting events and detecting novelty. Cereb. Cortex 15, 1768–1778.
Feredoes, E., and Postle, B. R. (2010). Prefrontal control of familiarity and recollection in working memory. J. Cogn. Neurosci. 22, 323–330.
Feredoes, E., Tononi, G., and Postle, B. R. (2006). Direct evidence for a prefrontal contribution to the control of proactive interference in verbal working memory. Proc. Natl. Acad. Sci. U.S.A. 103, 19530–19534.
Gardiner, J. M., Craik, F. I. M., and Birstwistle, J. (1972). Retrieval cues and release from proactive inhibition. J. Mem. Lang. 11, 778–783.
Jonides, J., Marshuetz, C., Smith, E. E., Reuter-Lorenz, P. A., Koeppe, R. A., and Hartley, A. (2000). Age differences in behavior and PET activation reveal differences in interference resolution in verbal working memory. J. Cogn. Neurosci. 12, 188–196.
Jonides, J., and Nee, D. E. (2006). Brain mechanisms of proactive interference in working memory. Neuroscience 139, 181–193.
Jonides, J., Smith, E. E., Marshuetz, C., Koeppe, R. A., and Reuter-Lorenz, P. A. (1998). Inhibition in verbal working memory revealed by brain activation. Proc. Natl. Acad. Sci. U.S.A. 95, 8410–8413.
Keppel, G., and Underwood, B. J. (1962). Proactive inhibition in short-term retention of single items. J. Mem. Lang. 1, 153–161.
Kuhl, B. A., Rissman, J., Chun, M. M., and Wagner, A. D. (2011). Fidelity of neural reactivation reveals competition between memories. Proc. Natl. Acad. Sci. U.S.A. 108, 5903–5908.
McElree, B., and Dosher, B. A. (1989). Serial position and set size in short-term-memory – the time course of recognition. J. Exp. Psychol. Gen. 118, 346–373.
Miller, E. K., and Cohen, J. D. (2001). An integrative theory of prefrontal cortex function. Annu. Rev. Neurosci. 24, 167–202.
Monsell, D. (1978). Recency, immediate recognition memory, and reaction time. Cogn. Psychol. 10, 465–501.
Norman, K. A., Polyn, S. M., Detre, G. J., and Haxby, J. V. (2006). Beyond mind-reading: multi-voxel pattern analysis of fMRI data. Trends Cogn. Sci. (Regul. Ed.) 10, 424–430.
Öztekin, I., Curtis, C. E., and McElree, B. (2009). The medial temporal lobe and the left inferior prefrontal cortex jointly support interference resolution in verbal working memory. J. Cogn. Neurosci. 21, 1967–1979.
Öztekin, I., and McElree, B. (2007). Proactive interference slows recognition by eliminating fast assessments of familiarity. J. Mem. Lang. 57, 126–149.
Poldrack, R. A., Wagner, A. D., Prull, M. W., Desmond, J. E., Glover, G. H., and Gabrieli, J. D. (1999). Functional specialization for semantic and phonological processing in the left inferior prefrontal cortex. Neuroimage 10, 15–35.
Polyn, S. M., Natu, V. S., Cohen, J. D., and Norman, K. A. (2005). Category-specific cortical activity precedes retrieval during memory search. Science 310, 1963–1966.
Postle, B. R., and Brush, L. N. (2004). The neural bases of the effects of item-nonspecific proactive interference in working memory. Cogn. Affect. Behav. Neurosci. 4, 379–392.
Tehan, G., and Humphreys, M. S. (1996). Cuing effects in short-term recall. Mem. Cognit. 24, 719–732.
Thompson-Schill, S. L. (2003). Neuroimaging studies of semantic memory: inferring “how” from “where.” Neuropsychologia 41, 280–292.
Thompson-Schill, S. L., and Botvinick, M. M. (2006). Resolving conflict: a response to Martin and Cheng (2006). Psychon. Bull. Rev. 13, 402–408; discussion 409–411.
Thompson-Schill, S. L., D’Esposito, M., Aguirre, G. K., and Farah, M. J. (1997). Role of left inferior prefrontal cortex in retrieval of semantic knowledge: a reevaluation. Proc. Natl. Acad. Sci. U.S.A. 94, 14792–14797.
Thompson-Schill, S. L., Jonides, J., Marshuetz, C., Smith, E. E., D’Esposito, M., Kan, I. P., Knight, R. T., and Swick, D. (2002). Effects of frontal lobe damage on interference effects in working memory. Cogn. Affect. Behav. Neurosci. 2, 109–120.
Tzourio-Mazoyer, N., Landeau, B., Papathanassiou, D., Crivello, F., Etard, O., Delcroix, N., Mazoyer, B., and Joliot, M. (2002). Automated anatomical labeling of activations in SPM using a macroscopic anatomical parcellation of the MNI MRI single-subject brain. Neuroimage 15, 273–289.
Van Overschelde, J., Rawson, K., and Dunlosky, J. (2004). Category norms: an updated and expanded version of the Battig and Montague (1969) norms. J. Mem. Lang. 50, 289–335.
Wagner, A. D., Maril, A., Bjork, R. A., and Schacter, D. L. (2001). Prefrontal contributions to executive control: fMRI evidence for functional distinctions within lateral prefrontal cortex. Neuroimage 14, 1337–1347.
Watkins, O. C., and Watkins, M. J. (1975). Build-up of proactive inhibition as a cue overload effect. J. Exp. Psychol. Hum. Learn. Mem. 104, 442–452.
Keywords: proactive interference, memory retrieval, fMRI, multi-voxel pattern analysis, VLPFC
Citation: Öztekin I and Badre D (2011) Distributed patterns of brain activity that lead to forgetting. Front. Hum. Neurosci. 5:86. doi: 10.3389/fnhum.2011.00086
Received: 02 June 2011;
Accepted: 03 August 2011;
Published online: 22 August 2011.
Edited by:
Russell A. Poldrack, University of California, USACopyright: © 2011 Öztekin and Badre. This is an open-access article subject to a non-exclusive license between the authors and Frontiers Media SA, which permits use, distribution and reproduction in other forums, provided the original authors and source are credited and other Frontiers conditions are complied with.
*Correspondence: Ilke Öztekin, Department of Psychology, Koç University, Rumeli Feneri Yolu, Sariyer 34450, Istanbul, Turkey. e-mail:aW96dGVraW5Aa3UuZWR1LnRy