- 1 The Mind Research Network, Albuquerque, NM, USA
- 2 Department of Electrical and Computer Engineering, University of New Mexico, Albuquerque, NM, USA
- 3 Olin Neuropsychiatry Research Center, Hartford, CT, USA
- 4 Department of Psychiatry, Yale University, New Haven, CT, USA
- 5 Department of Neurobiology, Yale University, New Haven, CT, USA
Schizophrenia (SZ) is one of the most cryptic and costly mental disorders in terms of human suffering and societal expenditure (van Os and Kapur, 2009). Though strong evidence for functional, structural, and genetic abnormalities associated with this disease exists, there is yet no replicable finding which has proven accurate enough to be useful in clinical decision making (Fornito et al., 2009), and its diagnosis relies primarily upon symptom assessment (Williams et al., 2010a). It is likely in part that the lack of consistent neuroimaging findings is because most models favor only one data type or do not combine data from different imaging modalities effectively, thus missing potentially important differences which are only partially detected by each modality (Calhoun et al., 2006a). It is becoming increasingly clear that multimodal fusion, a technique which takes advantage of the fact that each modality provides a limited view of the brain/gene and may uncover hidden relationships, is an important tool to help unravel the black box of schizophrenia. In this review paper, we survey a number of multimodal fusion applications which enable us to study the schizophrenia macro-connectome, including brain functional, structural, and genetic aspects and may help us understand the disorder in a more comprehensive and integrated manner. We also provide a table that characterizes these applications by the methods used and compare these methods in detail, especially for multivariate models, which may serve as a valuable reference that helps readers select an appropriate method based on a given research question.
Brief Introduction to Schizophrenia
Schizophrenia is a chronic, disabling mental disorder diagnosed on the basis of a constellation of clinical psychiatric symptoms and longitudinal course. The disease impairs multiple cognitive domains including memory, attention, and executive function (Heinrichs and Zakzanis, 1998). Although the causes and mechanisms of schizophrenia are still unclear, a hypothesis of neural network “disconnection” has been proposed (Friston and Frith, 1995). This hypothesis proposes that schizophrenia arises from dysfunctional integration of a distributed network of brain regions or a misconnection of neural circuitry leading to an impairment in the smooth coordination of mental processes, sometimes described as “cognitive dysmetria” (Andreasen et al., 1998). A number of studies have been published which have tried to delineate the underlying neural mechanisms of schizophrenia using functional magnetic resonance imaging (fMRI; Pearlson, 1997; Loeber et al., 1999; Curtis et al., 2001; McIntosh et al., 2008b; Yu et al., 2011a), structural MRI (sMRI; Giuliani et al., 2005; Strasser et al., 2005; Douaud et al., 2007), diffusion tensor imaging (DTI; McIntosh et al., 2008a; Kubicki et al., 2009; Sussmann et al., 2009), and genetics (Bahn, 2002; Williams et al., 2010b; Ripke et al., 2011). For example, in fMRI studies, deficits in dorsolateral prefrontal cortex (DLPFC; Hamilton et al., 2009) and the temporal lobe (Calhoun et al., 2008) have often been implicated in schizophrenia. In addition, DTI studies have found reduced integrity of the anterior limb of the internal capsule, uncinate fasciculus (UF), and anterior thalamic radiation (ATR) regions in schizophrenia (Kubicki et al., 2005b; Bellani et al., 2009; Sussmann et al., 2009). Finally, in genome wide association (GWAS) studies (Bahn, 2002; Shifman et al., 2004; Williams et al., 2009; Ripke et al., 2011), gene expression alterations have been reported in CACNA1C, ANK3, MIR137, and DISC1 recently. Given the breadth of findings across modalities, it is natural to evaluate the inter-relationship among them within a larger context.
Why Multimodal
Recently, collecting multiple types of brain data from the same individual using various non-invasive imaging techniques [MRI, DTI, electro-encephalography (EEG), MEG, etc.] has become common practice. Each imaging technique provides a different view of brain function or structure. For example, fMRI measures the hemodynamic response related to neural activity in the brain dynamically; sMRI provides information about the tissue type of the brain [gray matter (GM), white matter (WM), cerebrospinal fluid (CSF)]. DTI can additionally provide information on structural connectivity among brain networks. Another useful measure of brain function is EEG, which measures brain electrical activity with higher temporal resolution than fMRI (and lower spatial resolution). Typically these data are analyzed separately; however separate analyses do not enable the examination of the joint information between the modalities. In addition, focusing on a single modality in brain connectivity estimation can sometimes lead to contradicting conclusions (Plis et al., 2011).
Based on many previous findings in brain connectivity (Olesen et al., 2003; Rykhlevskaia et al., 2008; Camara et al., 2010; Yu et al., 2011b), it is plausible to assume covariation between brain function and structure. In addition, approximately 80% of total assayed genes display some cellular expression in brain (Lein et al., 2007). Therefore, it is reasonable to assume that these very diverse data may share certain underlying contributions to the etiopathology of brain disorders such as schizophrenia and jointly analyzing multimodal data may uncover previously hidden relationships that can only be partially detected in each modality alone. A motivating example is shown in Figure 1, in which a fiber bundle provides input to a distant location in varying degrees in two participants whose particular single nucleotide polymorphisms (SNPs) also differ, thus fMRI activity is different at the output location and possibly in functional connectivity (fMRI activity at the input is unaffected). The interactions between modalities (Figure 1B) would not be revealed by traditional separate analysis whereas a joint analysis (Figure 1C) would detect the underlying associations.
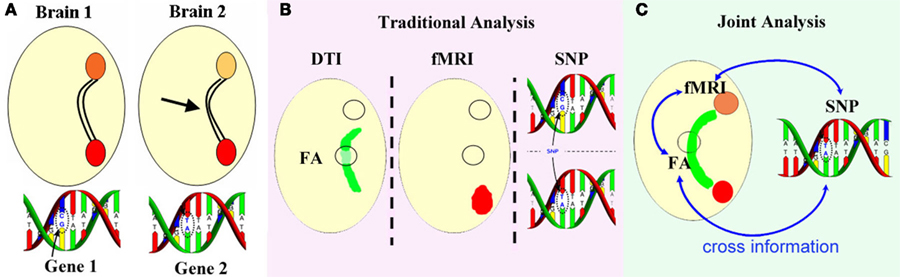
Figure 1. Motivating example for multimodal joint analysis. (A) Fiber bundle [fractional anisotropy (FA) from DTI] provides varied input to a distant region in two participants whose particular SNPs also differ, thus fMRI activity is different at the output location (orange circles) and possibly in functional connectivity, while fMRI activity at the input (red circles) is unaffected. Traditional separate analysis of each modality (B) would not reveal such modal interactions, whereas a joint analysis (C) may detect the underlying associations.
A key motivation for multimodal fusion is to take advantage of the cross-information provided by multiple imaging techniques, which in turn can be useful for identifying dysfunctional regions or potential biomarkers for many diseases. Basically, multimodal fusion refers to the use of a common symmetric model that explains different sorts of data (Friston, 2009). This is a complicated endeavor, and can generate results that are not obtainable using traditional approaches which focus upon a single data type or processing multiple datasets individually. However, in the real world, challenges often come from the fact that conclusions need to be drawn from high dimensional and noisy brain imaging data from only a limited number of subjects. Hence efficient and appropriate methods should be developed and chosen carefully.
In addition, multimodal brain imaging data has shown increasing utility in answering both scientifically interesting and clinically relevant questions. As well as providing the conceptual glue to bind together data from multiple types or levels of analysis, the related computational methods are also valuable for clinical research on the mechanisms of disease progression. For example, researchers often look for reliable relationships between few summary values from each modality, and certain behavioral dependent variables, e.g., the symptom scores of the patients with disorders, which may allow assessment of functional links between brain dynamics and human cognitive process. The incorporation of behavioral/cognitive data into multimodal fusion analysis which take the entire data into account and are not limited to a single region will not only improve our physiological understanding of brain diseases, but also provide insight into the neural bases of these human cognition or behavior (Makeig et al., 2009).
For schizophrenia, though strong evidences exist for functional, structural, and genetic abnormalities in each single-modality analysis, there is still lack of a diagnostic “gold standard” which is specific and sensitive enough to guide clinical decisions (Fornito et al., 2009). Even though GWAS studies have identified several risk genes that may influence brain functions affected in schizophrenia, they have not illuminated the etiology of the disease as they were perhaps anticipated to (Ripke et al., 2011; Williams et al., 2011). Therefore, it is likely at least in part, that a lack of consistent findings results from most studies favoring only one data type or not combining modalities in an integrated manner (Calhoun et al., 2006a).
Next, we will review several multimodal fusion applications in schizophrenia applied to different data types. Note that many fusion applications rely on studying correlations between highly distilled measures, e.g., from small regions of interest (Tregellas et al., 2007; Bates et al., 2009; Foucher et al., 2011). However the applications we reviewed mostly examine more complete relationships among the data types based on multivariate methods. Finally, we classify these applications based on the fusion models used, in order to provide a framework for selection of methods in future applications.
Multimodal Fusion Applications in Schizophrenia
Function–function
Functional magnetic resonance imaging–EEG (ERP)
The combination of fMRI and EEG is the most frequently used brain imaging fusion examples. Because it is believed that the spatial precision of fMRI can be complemented by the temporal precision of EEG (Friston, 2009) so that the neurovascular coupling mechanism can capture both neuronal and hemodynamic activity as two important components. One approach used for fMRI–EEG fusion is to constrain one modality with another, as reported in Dale and Halgren (2001), Eichele et al. (2005), Henson et al. (2010). While these are powerful techniques, a common limitation is that the potentially unrealistic assumptions of a fundamentally different nature than the known modality are imposed on the constrained data. For example, constraining EEG sources to lie within fMRI activated regions makes the implicit assumption that both signals have the same origin, or if present should be at least correlated, which is not always true. The fMRI signal is undoubtedly related to changes in blood follow, but it is by no means related in a simple ways to neuronal activity. Furthermore, transient EEG activity might not induce a detectable hemodynamic effect, and intense neuronal activity that is de-synchronized may lead to strong hemodynamic effects with on detectable average electrical activity (Jbabdi, 2009). By contrast, data-driven fusion methods are attractive for exploring data sets more fully, as they do not require prior hypotheses about the connection of interest.
Calhoun et al. (2006b, 2011) first proposed a joint independent component analysis (jICA) model (which assumes that two or more modalities share the same mixing matrix) to fuse together fMRI and EEG data, and aimed to identify specific differences in the neuronal chronometry of target detection for chronic schizophrenia patients compared to healthy controls (HC). One group-discriminative joint component was found via two-sample t-test (p < 0.0001), showing a clear difference in fMRI at bilateral fronto-temporal regions and in the event-related potentials (ERPs) during the N2–P3 latency range (ERPs are labeled based on their ordinal position following the stimulus onset, e.g., P3 for the third positive peak or N2 for the second negative peak). Note that both the hemodynamic and electro-physiologic phenomena were jointly expressed in this source, which have been previously implicated in schizophrenia, but no prior study had showed that changes in these two modalities were linked.
Correa et al. (2010b) also tried to differentiate schizophrenia from controls by applying multimodal canonical correlation analysis (mCCA) to fMRI–EEG data during performance of an auditory oddball (AOD) task (Kiehl and Liddle, 2001). Significant group differences were found in the bilateral temporal lobe/middle anterior cingulate region in fMRI, associated with the N2 and P3 peak in EEG. Multimodal CCA allows a different mixing matrix for each modality and is able to find a transformed coordinate system that maximizes inter-subject covariation across two or more data sets (Li et al., 2009). As shown in Figure 2, a set of linked components derived from a three-way fusion of sMRI, fMRI, and EEG were detected (Correa et al., 2010a), which significantly discriminate schizophrenia patients from controls. On examining the inter-subject modulation in conjunction with the spatial and temporal components, the results imply that patients with schizophrenia have less functional activity and less GM in the motor and temporal areas and also in part of the ERP N2/P3 complex. The comparison of t-tests for three modality (fMRI, sMRI, and EEG) versus two modality (fMRI and sMRI) analyses for this set of components is also listed, showing that the three-way analysis was more significant than a two- or one-way analysis, which further validates our motivation for multimodal fusion.
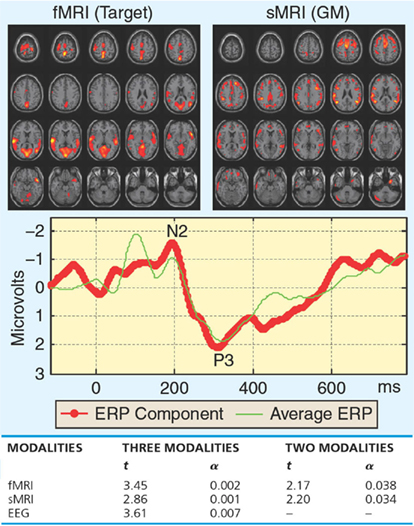
Figure 2. Functional magnetic resonance imaging/EEG/sMRI fusion by mCCA from Correa et al. (2010a): a set of associated components estimated by mCCA that showed significantly different loading for schizophrenia patients versus controls, as well as the t-test comparison between three-way and two-way fusion.
Structure–Structure
Gray matter–white matter
Using joint ICA, Xu et al. also identified four joint sources that were significantly associated with schizophrenia. The linked GM–WM regions identified in each of the joint sources included: (1) temporal – corpus callosum, (2) occipital/frontal – inferior fronto-occipital fasciculus, (3) frontal/parietal/occipital/temporal –superior longitudinal fasciculus, and (4) parietal/frontal – thalamus (Xu et al., 2009), which include a large number of brain regional networks and reflecting the widespread nature of the disease. A complex pattern of regional increases and decreases in schizophrenia were reflected. For example, for joint source 1, linkage between less GM in temporal lobe and the less WM in corpus callosum is interesting and may be related to the posterior corpus callosum connections to temporal lobe (Woodruff et al., 1993; Downhill et al., 2001). While for joint source 4, larger thalamic WM concentrations were shown in schizophrenia, suggesting that GM difference in the parietal and frontal lobe are associated with WM difference in thalamus, consistent with the role of the thalamus as a relay station (Wolfarth et al., 1985). In summary, jICA provides a unified framework to identify joint GM–WM sources that show group differences, which can cover as much neurological ground in a single computational model as achieved by many traditional separate analyses (Shenton et al., 2001).
Moreover, the inter-relationship between GM and WM can be analyzed to identify the tissue distribution abnormalities in schizophrenia (Xu et al., 2011) by utilizing novel features called structural phase and magnitude images. Where the phase image indicates the relative contribution of GM and WM, and the magnitude image reflects the overall tissue concentration. Six networks were identified showing significantly lower WM-to-GM in schizophrenia, including thalamus, right precentral–postcentral, left pre-/post-central, parietal, right cuneus–frontal, and left cuneus–frontal sources. Such findings demonstrate that structural phase and magnitude images can naturally and efficiently summarize the associated relationship between GM and WM.
Function–Structure
Cognitive dysfunction present in schizophrenia is often thought to be driven in part by disorganized connections between higher-order cortical fields, thus the combination of function and structure may provide more informative insights into altered brain connectivity (Rykhlevskaia et al., 2008).
Functional magnetic resonance imaging–GM
Figure 3 (Calhoun et al., 2006a) shows analyzed data collected from groups of schizophrenia patients and HCs using the jICA approach. The main finding was that group differences in bilateral parietal and frontal as well as posterior temporal regions in GM distinguished groups. A finding of less patient GM and less hemodynamic activity for target detection in these bilateral anterior temporal lobe regions was consistent with previous work. An unexpected corollary to this finding was that, in the regions showing the largest group differences, GM concentrations were larger in patients versus controls, suggesting that more GM may be related to less functional connectivity during performance of an auditory oddball task. Correa et al. (2008) also showed an interesting joint relationship between fMRI and GM by mCCA, with patients with schizophrenia showing more functional activity in motor areas and less activity in temporal areas associated with less GM as compared to HCs.
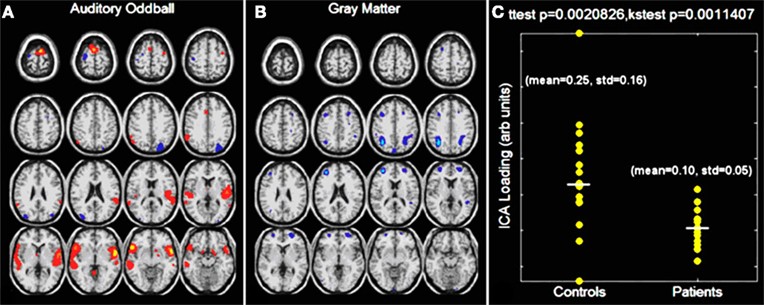
Figure 3. Auditory oddball/gray matter jICA analysis. Only one component demonstrated a significant difference between patients and controls. The joint source map for the auditory oddball fMRI data (A) and gray matter (B) data is presented along with the loading parameters for patients and controls (C).
Michael et al. (2010) introduced a method to identify inter-correlations among GM and fMRI voxels within the whole brain by reducing the cross-correlation matrix into histograms. Results show that the linkage between GM and functional activation in an auditory sensorimotor task (Schroder et al., 1999) is stronger in HCs than patients with schizophrenia. Specifically, GM regions in the cerebellum show more significant positive correlations with functional regions in HC. The cross-correlation can also be reduced to brain clusters (Michael et al., 2011) by fusing GM and fMRI contrast maps of a working memory task (Manoach et al., 1999). The maximum group difference occurred in medium difficulty working memory load. Particularly, the inter-cluster GM-Probe correlations for this load were positive in controls but negative in schizophrenia. While within one group, the inter-cluster correlation comparisons show no differences in controls but in patients with schizophrenia, indicating that the function-structure integrity during the recognition phrase is aberrant in schizophrenia.
Functional magnetic resonance imaging–DTI
Functional magnetic resonance, as a well-established neuroimaging technology, can act as a reference framework for validating conclusions derived from the relatively newer DTI method (Reinges et al., 2004), which can provide information regarding the integrity of WM tracts. Other recent work has shown that brain anatomical and functional connectivity are both altered in schizophrenia (Skudlarski et al., 2010).
Schlosser et al. (2007) observed a direct correlation in schizophrenia between frontal fractional anisotropy (FA) reduction and fMRI activation in regions in prefrontal and occipital cortices, which highlights a potential relationship between anatomical changes in a frontal-temporal anatomical circuit and functional alterations in the prefrontal cortex.
Sui et al. (2011) applied a blind data-driven model, mCCA + jICA, optimized for identifying correspondence across modalities, to real fMRI–DTI datasets from 164 subjects, including 62 HC, 54 SZ, and 48 bipolar disorder (BP) subjects. Only one joint group-discriminating component was detected between SZ and HC, including DLPFC and motor regions in fMRI of an auditory oddball detection task as well as parts of the ATR, SLF, and IFO WM tracts. The loading parameters of each modality also showed significant correlations with age. AOD_IC1 represents activations mainly in motor cortex, accompanied by a functional asymmetry with left dominance; see Figure 4A, consistent with the fact that the AOD task design required participants to push the button with fingers on their right hand. Controls had a very significant correlation r = 0.5, p = 4e − 5, while patient groups did not (p does not pass correction for multiple comparisons), implying that the motor regions of HC are normally more involved in the task with increasing age (Bennett et al., 2010), whereas schizophrenia patients have no such trend due to presumed motor system deficits (Rogowska et al., 2004).
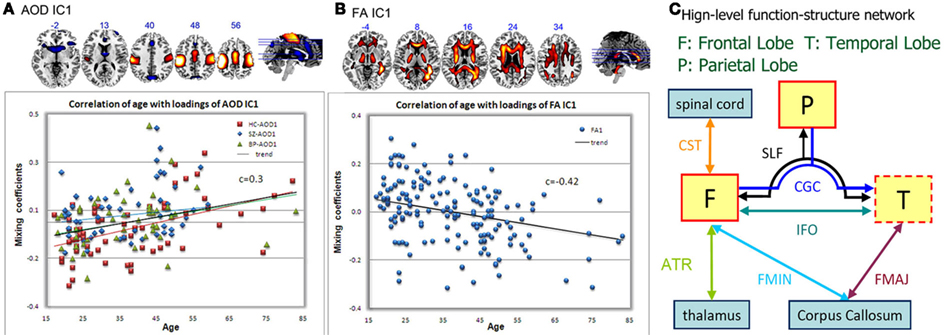
Figure 4. Joint fMRI/FA component that is HC–SZ discriminative, from Sui et al. (2011). Spatial maps of the identified functional blobs (A) and WM regions (B) are displayed with the correlation plot between subjects’ loadings and ages. Specifically, HC in red line, SZ in blue line, BP in green line, and trend of all subjects in black line. (C) Shows a high-level brain interaction diaphragm according to the joint component. Functional region with a red solid line frame indicates a major portion activation and the dotted line frame indicates that only small part of it is activated. Abbreviations are defined below, SLF, superior longitudinal fasciculus; CST, corticospinal tract; IFO, inferior fronto-occipital fasciculus; ILF, inferior longitudinal fasciculus; ATR, anterior thalamic radiation; CGC, cingulum; FMAJ, forceps major; FMIN, forceps minor.
Fractional anisotropy_IC1, as shown in Figure 4B, as a joint component of AOD_IC1, also demonstrated a significant (p = 2e − 08), but anti-correlation with age. Note that all groups had low p values for this component, thus using one marker, suggesting all subjects have a general age-related decrease in WM integrity, in agreement with (Sullivan and Pfefferbaum, 2003; Grieve et al., 2007). We are also able to provide insights into the high-level brain function-structure network, which verified that the linked (joint) components do correspond to FA changes in known tracts and functional changes in distant regions connected to that tract (Figure 4C). Note that we are not directly performing fiber tractography; a strength of mCCA + jICA is that it can detect complicated FA/fMRI relationships without requiring a directly detected link.
Brain Imaging–Genotype
Understanding genetic influences on both healthy and disordered brain function/structure is a major focus of psychiatric neuroimaging (“imaging genomics”) and may provide important additional information. For example, using combined genetic and fMRI data can achieve better classification accuracy than using either alone (Yang et al., 2010), indicating that genetic and brain function represent different, but partially complementary aspects.
Functional magnetic resonance imaging–SNP
Liu et al. (2009) first proposed parallel ICA to investigate correlations between brain dysfunctional regions and putative disease susceptibility SNPs, as shown in Figure 5. A correlation of 0.38 between one fMRI component and one SNP component was found; both showed significant differences in loading parameters between the schizophrenia and control groups (p = 0.0006 for the fMRI; p = 0.001 for SNP). The fMRI component consisted of regions in parietal lobe, right temporal lobe, and bilateral frontal lobe. The relevant SNP component was contributed to significantly by 10 SNPs located in genes including those coding for the nicotinic alpha-7 cholinergic receptor (CHRNA7), aromatic amino acid decarboxylase (AADC), and disrupted in schizophrenia 1 (DISC1). The findings provide a proof-of-concept that genomic SNP factors can be investigated by using phenotypic imaging findings in a multivariate format.
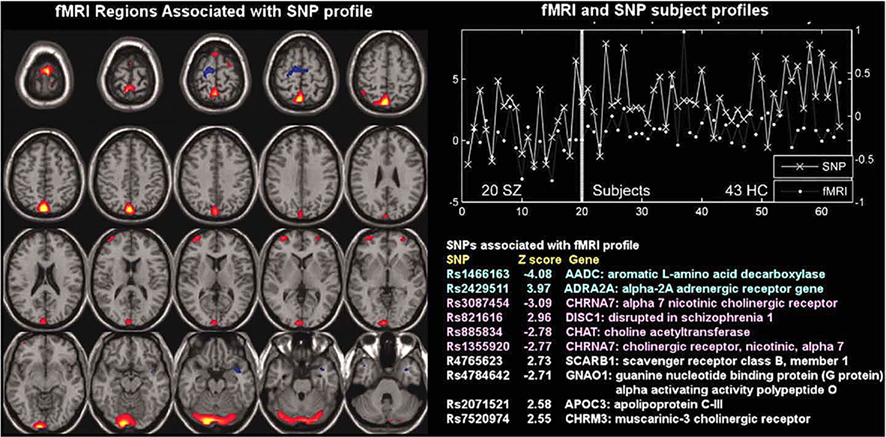
Figure 5. Functional magnetic resonance imaging/SNP parallel ICA from Liu et al. (2009): parallel ICA provides an fMRI part (left) and a SNP part (bottom right) in addition to a correlated subject profile for both fMRI and SNP data (top right).
Meda et al. (2010) further extended the use of parallel ICA by simultaneously analyzing fMRI and 24 SNP markers that previously had been associated with schizophrenia. Three fMRI components (including PFC, ACC, STG, and MTG) correlated significantly with two distinct gene components including DAT and SLC6A4_PR, revealing specific interactions between schizophrenia risk genes on imaging phenotypes that represent brain function in attention/working memory/goal directed behavior, establishing a useful methodology to probe multivariate genotype–phenotype relationships.
Structural MRI–SNP
Jamadar et al. (2011) further adopted parallel ICA to examine the relationship between GM volumes and 16 SNPs spanning FOXP2 and four Reading Disability-related genes: DCDC2, DYX1C1, KIAA0319, and TTRAP. Five such GM–SNP relationships were identified. The superior prefrontal, temporal, and occipital networks were positively related to DCDC2 in the schizophrenia, but not control group. In addition, the identified networks closely correspond to the known distribution of language processes in the cortex. Thus, reading and language difficulties in schizophrenia may be related to distributed cortical structural abnormalities associated with Reading Disability-related genes.
Similarly, Jagannathan et al. (2010) linked sMRI and genetic (SNP) components using parallel ICA, and identified a sMRI component that significantly correlated with a genetic component (r = −0.54, p < 0.00005), which also distinguished SZ from HC. The GM deficits pointed to brain regions including frontal/temporal lobes and thalamus (p < 0.01), which are consistently implicated in previous reports. These deficits were related to SNPs from 16 genes associated with schizophrenia risk and/or involved in normal central nervous system development, such as AKT, PI3K, SLC6A4, and DRD2.
As to the neurodevelopmental and life span factors in schizophrenia, first episode and longitudinal computed tomography (CT), and MRI studies have shown that brain abnormalities in schizophrenia are present at onset of psychosis and are non-progressive (Nasrallah et al., 1986; Wood et al., 2009). These and other findings support the idea that schizophrenia is a developmental rather than a degenerative condition (e.g., Rund, 2009). Furthermore, the presence of ventriculomegaly and diminished hemispheric asymmetry in familial schizophrenics and in those of their relatives who appear to be transmitting the disorder, implies involvement of the genes controlling neurodevelopment (Frangou and Murray, 1996). All these evidence suggests that genetic vulnerability, environmental factors, and cerebral structural disturbances can act in combination to result in clinically manifest schizophrenia (Harrison and Owen, 2003). Fusion approaches can be used to characterize such changes by relating the fusion parameters with variables such as age (Sui et al., 2011).
Summary of Multivariate Methods
In summary, studies featuring multimodal combinations prove to be more informative in understanding brain activity and disorders and the complexities of schizophrenia make this disease an appropriate test bed for exploration using joint information derived from multimodal datasets. The existing multivariate fusion methods have different optimization priorities and limitations. Each method presents a different view in interpreting and connecting the multiple datasets based on their various hypotheses. In Table 1, we listed several multivariate fusion methods that have been applied in schizophrenia study. The following aspects are compared in detail, including feasible combinations, optimization assumptions, purpose of the analysis, requirement of priors, the number of the modalities that can be combined and the input data types, which may serve as a guideline on method selection based on a given research and data.
In addition, for most of the above mentioned models, their inputs are from outputs of the general linear regression model. Since the statistical testing being performed is on the loading parameters within a regression framework, the statistical issues are similar to any regression analysis. Since small sample sizes may not generate enough statistical power, one major means to improve our results is to analyze data from more subjects, perhaps via multisite analyses. For example, in Sui et al. (2011), at least 48 SZ and 37 BP are needed to detect differences from HCs at a power of 0.8. More generally, when the effect size is 0.5, at least 64 subjects are needed per group (N = 2) to reach a statistical power of 0.8 in detecting group differences (Kenny, 1987).
Note that there are several other multivariate methods that have been successfully applied to brain imaging data fusion in other mental illnesses, such as Alzheimer’s disease, but have not yet been applied to schizophrenia. These methods include but not limited to partial least squares (Chen et al., 2009), linked ICA (Groves et al., 2011), and dynamic Bayesian networks (Plis et al., 2010). Hence, a future direction may lie in utilizing such approaches to explore schizophrenia.
In conclusion, the use of data fusion is a powerful technique. Selecting which fusion model to use should be done carefully but can be somewhat daunting, as there are many approaches available already. We have attempted to characterize and compare some of the available models in this paper. The use of data fusion approaches can help better elucidate the relationship among multiple modalities and facilitate new discoveries in brain disorders. The most promising avenues for future schizophrenia study may rest on developing better models that can complement and exploit the richness of the various data sets (Friston, 2009) and may enable a broader neuroscience perspective to be applied to neuroimaging so that key questions can be addressed in a theoretically grounded fashion.
Finally, certain caveats must also be considered in reviewing the evidence summarized in this paper. Schizophrenia is a clinically defined syndrome currently lacking specific biologic markers (biomarkers), though some robust deficits have been frequently identified in specific modalities, e.g., the DLPFC and superior temporal gyrus regions in MRI (Calhoun et al., 2004; Kim et al., 2009; Xu et al., 2009; Correa et al., 2010b), the ATR in WM tracts (Kubicki et al., 2005a; McIntosh et al., 2008a; Sui et al., 2011), and genetic differences in CACNA1C, DISC1, etc. (Liu et al., 2009; Williams et al., 2009). Thus, it is certainly possible that “schizophrenia” represents an agglomeration of biologically distinct diseases gathered by clinicians into a clinically convenient but biologically unrelated category of convenience, analogous to “dropsy.” To that extent this is true, no analyses will likely detect a uniform set of biomarkers to delineate a single disorder, because there are multiple disorders. The second caveat is that the overwhelming majority of schizophrenia subjects studied in the studies summarized are treated with powerful psychotropic medications that potentially alter both brain structure and function, so that commonalities detected in these imaging studies may in part result from such treatments for the disorder rather than being primary; this issue can only be resolved by studying first-episode drug-naïve subjects.
Conflict of Interest Statement
The authors declare that the research was conducted in the absence of any commercial or financial relationships that could be construed as a potential conflict of interest.
Acknowledgments
This work was supported by the National Institutes of Health grants R01EB 006841 and R01EB 005846 (to Vince D. Calhoun), and by the National Sciences Foundation grants 1016619 (to Vince D. Calhoun).
References
Andreasen, N. C., Paradiso, S., and O’Leary, D. S. (1998). “Cognitive dysmetria” as an integrative theory of schizophrenia: a dysfunction in cortical-subcortical-cerebellar circuitry? Schizophr. Bull. 24, 203–218.
Astolfi, L., Cincotti, F., Mattia, D., Salinari, S., Babiloni, C., Basilisco, A., Rossini, P. M., Ding, L., Ni, Y., He, B., Marciani, M. G., and Babiloni, F. (2004). Estimation of the effective and functional human cortical connectivity with structural equation modeling and directed transfer function applied to high-resolution EEG. Magn. Reson. Imaging 22, 1457–1470.
Bahn, S. (2002). Gene expression in bipolar disorder and schizophrenia: new approaches to old problems. Bipolar Disord. 4(Suppl. 1), 70–72.
Bates, A. T., Kiehl, K. A., Laurens, K. R., and Liddle, P. F. (2009). Low-frequency EEG oscillations associated with information processing in schizophrenia. Schizophr. Res. 115, 222–230.
Bellani, M., Yeh, P. H., Tansella, M., Balestrieri, M., Soares, J. C., and Brambilla, P. (2009). DTI studies of corpus callosum in bipolar disorder. Biochem. Soc. Trans. 37, 1096–1098.
Bennett, I. J., Madden, D. J., Vaidya, C. J., Howard, D. V., and Howard, J. H. (2010). Age-related differences in multiple measures of white matter integrity: a diffusion tensor imaging study of healthy aging. Hum. Brain Mapp. 31, 378–390.
Calhoun, V. D., Adali, T., Giuliani, N. R., Pekar, J. J., Kiehl, K. A., and Pearlson, G. D. (2006a). Method for multimodal analysis of independent source differences in schizophrenia: combining gray matter structural and auditory oddball functional data. Hum. Brain Mapp. 27, 47–62.
Calhoun, V. D., Adali, T., and Liu, J. (2006b). “A feature-based approach to combine functional MRI, structural MRI and EEG brain imaging data,” in Proceedings of the 28th IEEE EMBS Annual International Conference, New York City, 3672–3675.
Calhoun, V. D., Kiehl, K. A., Liddle, P. F., and Pearlson, G. D. (2004). Aberrant localization of synchronous hemodynamic activity in auditory cortex reliably characterizes schizophrenia. Biol. Psychiatry 55, 842–849.
Calhoun, V. D., Maciejewski, P. K., Pearlson, G. D., and Kiehl, K. A. (2008). Temporal lobe and “default” hemodynamic brain modes discriminate between schizophrenia and bipolar disorder. Hum. Brain Mapp. 29, 1265–1275.
Calhoun, V. D., Wu, L., Kiehl, K., Eichele, T., and Pearlson, G. (2011). Aberrant processing of deviant stimuli in schizophrenia revealed by fusion of fMRI and EEG data. Acta Neuropsychiatr. 22, 127–138.
Camara, E., Rodriguez-Fornells, A., and Munte, T. F. (2010). Microstructural brain differences predict functional hemodynamic responses in a reward processing task. J. Neurosci. 30, 11398–11402.
Chen, K., Reiman, E. M., Huan, Z., Caselli, R. J., Bandy, D., Ayutyanont, N., and Alexander, G. E. (2009). Linking functional and structural brain images with multivariate network analyses: a novel application of the partial least square method. Neuroimage 47, 602–610.
Correa, N. M., Adali, T., Li, Y. O., and Calhoun, V. D. (2010a). Canonical correlation analysis for data fusion and group inferences: examining applications of medical imaging data. IEEE Signal Process. Mag. 27, 39–50.
Correa, N. M., Eichele, T., Adali, T., Li, Y. O., and Calhoun, V. D. (2010b). Multi-set canonical correlation analysis for the fusion of concurrent single trial ERP and functional MRI. Neuroimage 50, 1438–1445.
Correa, N. M., Li, Y. O., Adali, T., and Calhoun, V. D. (2008). Canonical correlation analysis for feature-based fusion of biomedical imaging modalities and its application to detection of associative networks in schizophrenia. IEEE J. Sel. Top. Signal Process. 2, 998–1007.
Curtis, V. A., Dixon, T. A., Morris, R. G., Bullmore, E. T., Brammer, M. J., Williams, S. C., Sharma, T., Murray, R. M., and McGuire, P. K. (2001). Differential frontal activation in schizophrenia and bipolar illness during verbal fluency. J. Affect. Disord. 66, 111–121.
Dale, A. M., and Halgren, E. (2001). Spatiotemporal mapping of brain activity by integration of multiple imaging modalities. Curr. Opin. Neurobiol. 11, 202–208.
Douaud, G., Smith, S., Jenkinson, M., Behrens, T., Johansen-Berg, H., Vickers, J., James, S., Voets, N., Watkins, K., Matthews, P. M., and James, A. (2007). Anatomically related grey and white matter abnormalities in adolescent-onset schizophrenia. Brain 130, 2375–2386.
Downhill, J. E. Jr., Buchsbaum, M. S., Hazlett, E. A., Barth, S., Lees Roitman, S., Nunn, M., Lekarev, O., Wei, T., Shihabuddin, L., Mitropoulou, V., Silverman, J., and Siever, L. J. (2001). Temporal lobe volume determined by magnetic resonance imaging in schizotypal personality disorder and schizophrenia. Schizophr. Res. 48, 187–199.
Eichele, T., Calhoun, V. D., and Debener, S. (2009). Mining EEG-fMRI using independent component analysis. Int. J. Psychophysiol. 73, 53–61.
Eichele, T., Specht, K., Moosmann, M., Jongsma, M. L., Quiroga, R. Q., Nordby, H., and Hugdahl, K. (2005). Assessing the spatiotemporal evolution of neuronal activation with single-trial event-related potentials and functional MRI. Proc. Natl. Acad. Sci. U.S.A. 102, 17798–17803.
Fornito, A., Yucel, M., and Pantelis, C. (2009). Reconciling neuroimaging and neuropathological findings in schizophrenia and bipolar disorder. Curr Opin Psychiatry 22, 312–319.
Foucher, J. R., Luck, D., Marrer, C., Pham, B. T., Gounot, D., Vidailhet, P., and Otzenberger, H. (2011). fMRI working memory hypo-activations in schizophrenia come with a coupling deficit between arousal and cognition. Psychiatry Res 194, 21–29.
Franco, A. R., Ling, J., Caprihan, A., Calhoun, V. D., Jung, R. E., Heileman, G. L., and Mayer, A. R. (2008). Multimodal and multi-tissue measures of connectivity revealed by joint independent component analysis. IEEE J. Sel. Top. Signal Process. 2, 986–997.
Frangou, S., and Murray, R. M. (1996). Imaging as a tool in exploring the neurodevelopment and genetics of schizophrenia. Br. Med. Bull. 52, 587–596.
Friston, K. J. (2009). Modalities, modes, and models in functional neuroimaging. Science 326, 399–403.
Friston, K. J., and Frith, C. D. (1995). Schizophrenia: a disconnection syndrome? Clin. Neurosci. 3, 89–97.
Giuliani, N. R., Calhoun, V. D., Pearlson, G. D., Francis, A., and Buchanan, R. W. (2005). Voxel-based morphometry versus region of interest: a comparison of two methods for analyzing gray matter differences in schizophrenia. Schizophr. Res. 74, 135–147.
Grieve, S. M., Williams, L. M., Paul, R. H., Clark, C. R., and Gordon, E. (2007). Cognitive aging, executive function, and fractional anisotropy: a diffusion tensor MR imaging study. AJNR Am. J. Neuroradiol. 28, 226–235.
Groves, A. R., Beckmann, C. F., Smith, S. M., and Woolrich, M. W. (2011). Linked independent component analysis for multimodal data fusion. Neuroimage 54, 2198–2217.
Hamilton, L. S., Altshuler, L. L., Townsend, J., Bookheimer, S. Y., Phillips, O. R., Fischer, J., Woods, R. P., Mazziotta, J. C., Toga, A. W., Nuechterlein, K. H., and Narr, K. L. (2009). Alterations in functional activation in euthymic bipolar disorder and schizophrenia during a working memory task. Hum. Brain Mapp. 30, 3958–3969.
Harrison, P. J., and Owen, M. J. (2003). Genes for schizophrenia? Recent findings and their pathophysiological implications. Lancet 361, 417–419.
Heinrichs, R. W., and Zakzanis, K. K. (1998). Neurocognitive deficit in schizophrenia: a quantitative review of the evidence. Neuropsychology 12, 426–445.
Henson, R. N., Flandin, G., Friston, K. J., and Mattout, J. (2010). A parametric empirical Bayesian framework for fMRI-constrained MEG/EEG source reconstruction. Hum. Brain Mapp. 31, 1512–1531.
Jagannathan, K., Calhoun, V. D., Gelernter, J., Stevens, M. C., Liu, J., Bolognani, F., Windemuth, A., Ruano, G., Assaf, M., and Pearlson, G. D. (2010). Genetic associations of brain structural networks in schizophrenia: a preliminary study. Biol. Psychiatry 68, 657–666.
Jamadar, S., Powers, N. R., Meda, S. A., Gelernter, J., Gruen, J. R., and Pearlson, G. D. (2011). Genetic influences of cortical gray matter in language-related regions in healthy controls and schizophrenia. Schizophr. Res. 129, 141–148.
Jbabdi, S. (2009). “Imaging structure and function,” in Diffusion MRI: From Quantitative Measurement to In Vivo Neuroanatomy, eds H. Johansen-Berg and T. E. J. Behrens (Elsevier Inc.), 470–471.
Kenny, D. A. (1987). Statistics for the Social and Behavioral Sciences. Boston, MA: Little Brown and Company, 215.
Kiehl, K. A., and Liddle, P. F. (2001). An event-related functional magnetic resonance imaging study of an auditory oddball task in schizophrenia. Schizophr. Res. 48, 159–171.
Kim, D. I., Mathalon, D. H., Ford, J. M., Mannell, M., Turner, J. A., Brown, G. G., Belger, A., Gollub, R., Lauriello, J., Wible, C., O’Leary, D., Lim, K., Toga, A., Potkin, S. G., Birn, F., and Calhoun, V. D. (2009). Auditory oddball deficits in schizophrenia: an independent component analysis of the fMRI multisite function BIRN study. Schizophr. Bull. 35, 67–81.
Kim, D. I., Sui, J., Rachakonda, S., White, T., Manoach, D. S., Clark, V. P., Ho, B. C., Schulz, S. C., and Calhoun, V. D. (2010). Identification of imaging biomarkers in schizophrenia: a coefficient-constrained independent component analysis of the mind multi-site schizophrenia study. Neuroinformatics 8, 213–229.
Kubicki, M., McCarley, R. W., Nestor, P. G., Huh, T., Kikinis, R., Shenton, M. E., and Wible, C. G. (2003). An fMRI study of semantic processing in men with schizophrenia. Neuroimage 20, 1923–1933.
Kubicki, M., Niznikiewicz, M., Connor, E., Nestor, P., Bouix, S., Dreusicke, M., Kikinis, R., McCarley, R., and Shenton, M. (2009). Relationship between white matter integrity, attention, and memory in schizophrenia: a diffusion tensor imaging study. Brain Imaging Behav. 3, 191–201.
Kubicki, M., Park, H., Westin, C. F., Nestor, P. G., Mulkern, R. V., Maier, S. E., Niznikiewicz, M., Connor, E. E., Levitt, J. J., Frumin, M., Kikinis, R., Jolesz, F. A., McCarley, R. W., and Shenton, M. E. (2005a). DTI and MTR abnormalities in schizophrenia: analysis of white matter integrity. Neuroimage 26, 1109–1118.
Kubicki, M., Westin, C. F., McCarley, R. W., and Shenton, M. E. (2005b). The application of DTI to investigate white matter abnormalities in schizophrenia. Ann. N. Y. Acad. Sci. 1064, 134–148.
Lein, E. S., Hawrylycz, M. J., Ao, N., Ayres, M., Bensinger, A., Bernard, A., Boe, A. F., Boguski, M. S., Brockway, K. S., Byrnes, E. J., Chen, L., Chen, T. M., Chin, M. C., Chong, J., Crook, B. E., Czaplinska, A., Dang, C. N., Datta, S., Dee, N. R., Desaki, A. L., Desta, T., Diep, E., Dolbeare, T. A., Donelan, M. J., Dong, H. W., Dougherty, J. G., Duncan, B. J., Ebbert, A. J., Eichele, G., Estin, L. K., Faber, C., Facer, B. A., Fields, R., Fischer, S. R., Fliss, T. P., Frensley, C., Gates, S. N., Glattfelder, K. J., Halverson, K. R., Hart, M. R., Hohmann, J. G., Howell, M. P., Jeung, D. P., Johnson, R. A., Karr, P. T., Kawal, R., Kidney, J. M., Knapik, R. H., Kuan, C. L., Lake, J. H., Laramee, A. R., Larsen, K. D., Lau, C., Lemon, T. A., Liang, A. J., Liu, Y., Luong, L. T., Michaels, J., Morgan, J. J., Morgan, R. J., Mortrud, M. T., Mosqueda, N. F., Ng, L. L., Ng, R., Orta, G. J., Overly, C. C., Pak, T. H., Parry, S. E., Pathak, S. D., Pearson, O. C., Puchalski, R. B., Riley, Z. L., Rockett, H. R., Rowland, S. A., Royall, J. J., Ruiz, M. J., Sarno, N. R., Schaffnit, K., Shapovalova, N. V., Sivisay, T., Slaughterbeck, C. R., Smith, S. C., Smith, K. A., Smith, B. I., Sodt, A. J., Stewart, N. N., Stumpf, K. R., Sunkin, S. M., Sutram, M., Tam, A., Teemer, C. D., Thaller, C., Thompson, C. L., Varnam, L. R., Visel, A., Whitlock, R. M., Wohnoutka, P. E., Wolkey, C. K., Wong, V. Y., Wood, M., Yaylaoglu, M. B., Young, R. C., Youngstrom, B. L., Yuan, X. F., Zhang, B., Zwingman, T. A., and Jones, A. R. (2007). Genome-wide atlas of gene expression in the adult mouse brain. Nature 445, 168–176.
Li, Y. O., Adali, T., Wang, W., and Calhoun, V. D. (2009). Joint blind source separation by multi-set canonical correlation analysis. IEEE Trans. Signal Process. 57, 3918–3929.
Liu, J., Pearlson, G., Windemuth, A., Ruano, G., Perrone-Bizzozero, N. I., and Calhoun, V. (2009). Combining fMRI and SNP data to investigate connections between brain function and genetics using parallel ICA. Hum. Brain Mapp. 30, 241–255.
Loeber, R. T., Sherwood, A. R., Renshaw, P. F., Cohen, B. M., and Yurgelun-Todd, D. A. (1999). Differences in cerebellar blood volume in schizophrenia and bipolar disorder. Schizophr. Res. 37, 81–89.
Makeig, S., Gramann, K., Jung, T. P., Sejnowski, T. J., and Poizner, H. (2009). Linking brain, mind and behavior. Int. J. Psychophysiol. 73, 95–100.
Manoach, D. S., Press, D. Z., Thangaraj, V., Searl, M. M., Goff, D. C., Halpern, E., Saper, C. B., and Warach, S. (1999). Schizophrenic subjects activate dorsolateral prefrontal cortex during a working memory task, as measured by fMRI. Biol. Psychiatry 45, 1128–1137.
McIntosh, A. M., Maniega, S. M., Lymer, G. K., McKirdy, J., Hall, J., Sussmann, J. E., Bastin, M. E., Clayden, J. D., Johnstone, E. C., and Lawrie, S. M. (2008a). White matter tractography in bipolar disorder and schizophrenia. Biol. Psychiatry 64, 1088–1092.
McIntosh, A. M., Whalley, H. C., McKirdy, J., Hall, J., Sussmann, J. E., Shankar, P., Johnstone, E. C., and Lawrie, S. M. (2008b). Prefrontal function and activation in bipolar disorder and schizophrenia. Am. J. Psychiatry 165, 378–384.
Meda, S. A., Jagannathan, K., Gelernter, J., Calhoun, V. D., Liu, J., Stevens, M. C., and Pearlson, G. D. (2010). A pilot multivariate parallel ICA study to investigate differential linkage between neural networks and genetic profiles in schizophrenia. Neuroimage 53, 1007–1015.
Michael, A. M., Baum, S. A., White, T., Demirci, O., Andreasen, N. C., Segall, J. M., Jung, R. E., Pearlson, G., Clark, V. P., Gollub, R. L., Schulz, S. C., Roffman, J. L., Lim, K. O., Ho, B. C., Bockholt, H. J., and Calhoun, V. D. (2010). Does function follow form? Methods to fuse structural and functional brain images show decreased linkage in schizophrenia. Neuroimage 49, 2626–2637.
Michael, A. M., King, M. D., Ehrlich, S., Pearlson, G., White, T., Holt, D. J., Andreasen, N. C., Sakoglu, U., Ho, B. C., Schulz, S. C., and Calhoun, V. D. (2011). A data-driven investigation of gray matter-function correlations in schizophrenia during a working memory task. Front. Hum. Neurosci. 5:71. doi:10.3389/fnhum.2011.00071
Nasrallah, H. A., Olson, S. C., McCalley-Whitters, M., Chapman, S., and Jacoby, C. G. (1986). Cerebral ventricular enlargement in schizophrenia. A preliminary follow-up study. Arch. Gen. Psychiatry 43, 157–159.
Olesen, P. J., Nagy, Z., Westerberg, H., and Klingberg, T. (2003). Combined analysis of DTI and fMRI data reveals a joint maturation of white and grey matter in a fronto-parietal network. Brain Res. Cogn. Brain Res. 18, 48–57.
Pearlson, G. D. (1997). Superior temporal gyrus and planum temporale in schizophrenia: a selective review. Prog. Neuropsychopharmacol. Biol. Psychiatry 21, 1203–1229.
Plis, S. M., Calhoun, V. D., Weisend, M. P., Eichele, T., and Lane, T. (2010). MEG and fMRI fusion for non-linear estimation of neural and BOLD signal changes. Front. Neuroinform. 4:114. doi:10.3389/fninf.2010.00114
Plis, S. M., Weisend, M. P., Damaraju, E., Eichele, T., Mayer, A., Clark, V. P., Lane, T., and Calhoun, V. D. (2011). Effective connectivity analysis of fMRI and MEG data collected under identical paradigms. Comput. Biol. Med. 41, 1156–1165.
Reinges, M. H., Krings, T., Kranzlein, H., Hans, F. J., Thron, A., and Gilsbach, J. M. (2004). Functional and diffusion-weighted magnetic resonance imaging for visualization of the postthalamic visual fiber tracts and the visual cortex. Minim. Invasive Neurosurg. 47, 160–164.
Ripke, S., Sanders, A. R., Kendler, K. S., Levinson, D. F., Sklar, P., Holmans, P. A., Lin, D. Y., Duan, J., Ophoff, R. A., Andreassen, O. A., Scolnick, E., Cichon, S., St Clair, D., Corvin, A., Gurling, H., Werge, T., Rujescu, D., Blackwood, D. H., Pato, C. N., Malhotra, A. K., Purcell, S., Dudbridge, F., Neale, B. M., Rossin, L., Visscher, P. M., Posthuma, D., Ruderfer, D. M., Fanous, A., Stefansson, H., Steinberg, S., Mowry, B. J., Golimbet, V., De Hert, M., Jonsson, E. G., Bitter, I., Pietilainen, O. P., Collier, D. A., Tosato, S., Agartz, I., Albus, M., Alexander, M., Amdur, R. L., Amin, F., Bass, N., Bergen, S. E., Black, D. W., Borglum, A. D., Brown, M. A., Bruggeman, R., Buccola, N. G., Byerley, W. F., Cahn, W., Cantor, R. M., Carr, V. J., Catts, S. V., Choudhury, K., Cloninger, C. R., Cormican, P., Craddock, N., Danoy, P. A., Datta, S., de Haan, L., Demontis, D., Dikeos, D., Djurovic, S., Donnelly, P., Donohoe, G., Duong, L., Dwyer, S., Fink-Jensen, A., Freedman, R., Freimer, N. B., Friedl, M., Georgieva, L., Giegling, I., Gill, M., Glenthoj, B., Godard, S., Hamshere, M., Hansen, M., Hansen, T., Hartmann, A. M., Henskens, F. A., Hougaard, D. M., Hultman, C. M., Ingason, A., Jablensky, A. V., Jakobsen, K. D., Jay, M., Jurgens, G., Kahn, R. S., Keller, M. C., Kenis, G., Kenny, E., Kim, Y., Kirov, G. K., Konnerth, H., Konte, B., Krabbendam, L., Krasucki, R., Lasseter, V. K., Laurent, C., Lawrence, J., Lencz, T., Lerer, F. B., Liang, K. Y., Lichtenstein, P., Lieberman, J. A., Linszen, D. H., Lonnqvist, J., Loughland, C. M., Maclean, A. W., Maher, B. S., Maier, W., Mallet, J., Malloy, P., Mattheisen, M., Mattingsdal, M., McGhee, K. A., McGrath, J. J., McIntosh, A., McLean, D. E., McQuillin, A., Melle, I., Michie, P. T., Milanova, V., Morris, D. W., Mors, O., Mortensen, P. B., Moskvina, V., Muglia, P., Myin-Germeys, I., Nertney, D. A., Nestadt, G., Nielsen, J., Nikolov, I., Nordentoft, M., Norton, N., Nothen, M. M., O’Dushlaine, C. T., Olincy, A., Olsen, L., O’Neill, F. A., Orntoft, T. F., Owen, M. J., Pantelis, C., Papadimitriou, G., Pato, M. T., Peltonen, L., Petursson, H., Pickard, B., Pimm, J., Pulver, A. E., Puri, V., Quested, D., Quinn, E. M., Rasmussen, H. B., Rethelyi, J. M., Ribble, R., Rietschel, M., Riley, B. P., Ruggeri, M., Schall, U., Schulze, T. G., Schwab, S. G., Scott, R. J., Shi, J., Sigurdsson, E., Silverman, J. M., Spencer, C. C., Stefansson, K., Strange, A., Strengman, E., Stroup, T. S., Suvisaari, J., Terenius, L., Thirumalai, S., Thygesen, J. H., Timm, S., Toncheva, D., van den Oord, E., van Os, J., van Winkel, R., Veldink, J., Walsh, D., Wang, A. G., Wiersma, D., Wildenauer, D. B., Williams, H. J., Williams, N. M., Wormley, B., Zammit, S., Sullivan, P. F., O’Donovan, M. C., Daly, M. J., and Gejman, P. V. (2011). Genome-wide association study identifies five new schizophrenia loci. Nat. Genet. 43, 969–976.
Rogowska, J., Gruber, S. A., and Yurgelun-Todd, D. A. (2004). Functional magnetic resonance imaging in schizophrenia: cortical response to motor stimulation. Psychiatry Res. 130, 227–243.
Rykhlevskaia, E., Gratton, G., and Fabiani, M. (2008). Combining structural and functional neuroimaging data for studying brain connectivity: a review. Psychophysiology 45, 173–187.
Schlosser, R. G., Nenadic, I., Wagner, G., Gullmar, D., von Consbruch, K., Kohler, S., Schultz, C. C., Koch, K., Fitzek, C., Matthews, P. M., Reichenbach, J. R., and Sauer, H. (2007). White matter abnormalities and brain activation in schizophrenia: a combined DTI and fMRI study. Schizophr. Res. 89, 1–11.
Schroder, J., Essig, M., Baudendistel, K., Jahn, T., Gerdsen, I., Stockert, A., Schad, L. R., and Knopp, M. V. (1999). Motor dysfunction and sensorimotor cortex activation changes in schizophrenia: a study with functional magnetic resonance imaging. Neuroimage 9, 81–87.
Shenton, M. E., Dickey, C. C., Frumin, M., and McCarley, R. W. (2001). A review of MRI findings in schizophrenia. Schizophr. Res. 49, 1–52.
Shifman, S., Bronstein, M., Sternfeld, M., Pisante, A., Weizman, A., Reznik, I., Spivak, B., Grisaru, N., Karp, L., Schiffer, R., Kotler, M., Strous, R. D., Swartz-Vanetik, M., Knobler, H. Y., Shinar, E., Yakir, B., Zak, N. B., and Darvasi, A. (2004). COMT: a common susceptibility gene in bipolar disorder and schizophrenia. Am. J. Med. Genet. B Neuropsychiatr. Genet. 128B, 61–64.
Skudlarski, P., Jagannathan, K., Anderson, K., Stevens, M. C., Calhoun, V. D., Skudlarska, B. A., and Pearlson, G. (2010). Brain connectivity is not only lower but different in schizophrenia: a combined anatomical and functional approach. Biol. Psychiatry 68, 61–69.
Strasser, H. C., Lilyestrom, J., Ashby, E. R., Honeycutt, N. A., Schretlen, D. J., Pulver, A. E., Hopkins, R. O., Depaulo, J. R., Potash, J. B., Schweizer, B., Yates, K. O., Kurian, E., Barta, P. E., and Pearlson, G. D. (2005). Hippocampal and ventricular volumes in psychotic and nonpsychotic bipolar patients compared with schizophrenia patients and community control subjects: a pilot study. Biol. Psychiatry 57, 633–639.
Sui, J., Adali, T., Pearlson, G. D., and Calhoun, V. D. (2009). An ICA-based method for the identification of optimal fMRI features and components using combined group-discriminative techniques. Neuroimage 46, 73–86.
Sui, J., Pearlson, G., Caprihan, A., Adali, T., Kiehl, K. A., Liu, J., Yamamoto, J., and Calhoun, V. D. (2011). Discriminating schizophrenia and bipolar disorder by fusing fMRI and DTI in a multimodal CCA+ joint ICA model. Neuroimage 57, 839–855.
Sullivan, E. V., and Pfefferbaum, A. (2003). Diffusion tensor imaging in normal aging and neuropsychiatric disorders. Eur. J. Radiol. 45, 244–255.
Sussmann, J. E., Lymer, G. K., McKirdy, J., Moorhead, T. W., Maniega, S. M., Job, D., Hall, J., Bastin, M. E., Johnstone, E. C., Lawrie, S. M., and McIntosh, A. M. (2009). White matter abnormalities in bipolar disorder and schizophrenia detected using diffusion tensor magnetic resonance imaging. Bipolar Disord. 11, 11–18.
Tregellas, J. R., Davalos, D. B., Rojas, D. C., Waldo, M. C., Gibson, L., Wylie, K., Du, Y. P., and Freedman, R. (2007). Increased hemodynamic response in the hippocampus, thalamus and prefrontal cortex during abnormal sensory gating in schizophrenia. Schizophr. Res. 92, 262–272.
Williams, H. J., Craddock, N., Russo, G., Hamshere, M. L., Moskvina, V., Dwyer, S., Smith, R. L., Green, E., Grozeva, D., Holmans, P., Owen, M. J., and O’Donovan, M. C. (2010a). Most genome-wide significant susceptibility loci for schizophrenia and bipolar disorder reported to date cross-traditional diagnostic boundaries. Hum. Mol. Genet. 20, 387–391.
Williams, H. J., Norton, N., Dwyer, S., Moskvina, V., Nikolov, I., Carroll, L., Georgieva, L., Williams, N. M., Morris, D. W., Quinn, E. M., Giegling, I., Ikeda, M., Wood, J., Lencz, T., Hultman, C., Lichtenstein, P., Thiselton, D., Maher, B. S., Malhotra, A. K., Riley, B., Kendler, K. S., Gill, M., Sullivan, P., Sklar, P., Purcell, S., Nimgaonkar, V. L., Kirov, G., Holmans, P., Corvin, A., Rujescu, D., Craddock, N., Owen, M. J., and O’Donovan, M. C. (2010b). Fine mapping of ZNF804A and genome-wide significant evidence for its involvement in schizophrenia and bipolar disorder. Mol. Psychiatry 16, 429–441.
Williams, H. J., Craddock, N., Russo, G., Hamshere, M. L., Moskvina, V., Dwyer, S., Smith, R. L., Green, E., Grozeva, D., Holmans, P., Owen, M. J., and O’Donovan, M. C. (2011). Most genome-wide significant susceptibility loci for schizophrenia and bipolar disorder reported to date cross-traditional diagnostic boundaries. Hum. Mol. Genet. 20, 387–391.
Williams, H. J., Owen, M. J., and O’Donovan, M. C. (2009). Schizophrenia genetics: new insights from new approaches. Br. Med. Bull. 91, 61–74.
Wolfarth, S., Kolasiewicz, W., and Ossowske, K. (1985). Thalamus as a relay station for catalepsy and rigidity. Behav. Brain Res. 18, 261–268.
Wood, S. J., Pantelis, C., Yung, A. R., Velakoulis, D., and McGorry, P. D. (2009). Brain changes during the onset of schizophrenia: implications for neurodevelopmental theories. Med. J. Aust. 190, S10–S13.
Woodruff, P. W., Pearlson, G. D., Geer, M. J., Barta, P. E., and Chilcoat, H. D. (1993). A computerized magnetic resonance imaging study of corpus callosum morphology in schizophrenia. Psychol. Med. 23, 45–56.
Xu, L., Pearlson, G., and Calhoun, V. D. (2009). Joint source based morphometry identifies linked gray and white matter group differences. Neuroimage 44, 777–789.
Xu, L., Tulay, A., Schretlen, D., Pearlson, G. D., and Calhoun, V. D. (2011). Structural angle and power images reveal interrelated gray and white matter abnormalities in schizophrenia. Neurol. Res. Int. 2012, 1–18.
Yang, H., Liu, J., Sui, J., Pearlson, G. D., and Calhoun, V. D. (2010). A hybrid machine learning method for fusing fMRI and genetic data: combining both improves classification of schizophrenia. Front. Hum. Neurosci. 4:192. doi:10.3389/fnhum.2010.00192
Yu, Q., Sui, J., Rachakonda, S., He, H., Gruner, W., Pearlson, G., Kiehl, K. A., and Calhoun, V. D. (2011a). Altered topological properties of functional network connectivity in schizophrenia during resting state: a small-world brain network study. PLoS ONE 6, e25423. doi:10.1371/journal.pone.0025423
Keywords: multimodal fusion, schizophrenia, MRI, DTI, EEG, SNP, ICA, CCA
Citation: Sui J, Yu Q, He H, Pearlson GD and Calhoun VD (2012) A selective review of multimodal fusion methods in schizophrenia. Front. Hum. Neurosci. 6:27. doi: 10.3389/fnhum.2012.00027
Received: 04 December 2011;
Accepted: 08 February 2012;
Published online: 24 February 2012.
Edited by:
Kenneth Hugdahl, University of Bergen, NorwayReviewed by:
Kenneth Hugdahl, University of Bergen, NorwayPatricia E. Cowell, University of Sheffield, UK
Copyright: © 2012 Sui, Yu, He, Pearlson and Calhoun. This is an open-access article distributed under the terms of the Creative Commons Attribution Non Commercial License, which permits non-commercial use, distribution, and reproduction in other forums, provided the original authors and source are credited.
*Correspondence: Jing Sui, The Mind Research Network, 1101 Yale Blvd. Northeast, Albuquerque, NM 87106, USA. e-mail:anN1aUBtcm4ub3JnLA==dmNhbGhvdW5AdW5tLmVkdQ==