- 1Brain Research Unit and MEG Core, O.V. Lounasmaa Laboratory, School of Science, Aalto University, Espoo, Finland
- 2BioMag Laboratory, HUSLAB, Helsinki University Central Hospital, Helsinki, Finland
Social interactions fill our everyday life and put strong demands on our brain function. However, the possibilities for studying the brain basis of social interaction are still technically limited, and even modern brain imaging studies of social cognition typically monitor just one participant at a time. We present here a method to connect and synchronize two faraway neuromagnetometers. With this method, two participants at two separate sites can interact with each other through a stable real-time audio connection with minimal delay and jitter. The magnetoencephalographic (MEG) and audio recordings of both laboratories are accurately synchronized for joint offline analysis. The concept can be extended to connecting multiple MEG devices around the world. As a proof of concept of the MEG-to-MEG link, we report the results of time-sensitive recordings of cortical evoked responses to sounds delivered at laboratories separated by 5 km.
Introduction
Humans spend a considerable amount of time interacting with other people, for example, communicating by verbal and nonverbal means and performing joint actions. Impressively, most persons deal with the ever-changing and intermingling conversations and tasks effortlessly. Various aspects of social interaction have been studied extensively in social sciences, for example by conversation analysis, but they have also recently started to gain interest in systems neuroscience and brain imaging communities (for reviews, see Hari and Kujala, 2009; Becchio et al., 2010; Dumas, 2011; Dumas et al., 2011; Hasson et al., 2012; Singer, 2012). However, many current approaches for studying the brain basis of social interaction are still methodologically clumsy, mainly because of the lack of suitable recording setups and analysis tools for simultaneous recordings of two persons.
Consequently, most brain imaging studies on social interaction have concentrated on recording brain activity of one participant at a time in “pseudo-interactive” situations (e.g., Schippers et al., 2009, 2010; Stephens et al., 2010; Anders et al., 2011). For example, a few-second-time-scale synchronization between the speaker's and listener's brain was demonstrated with functional magnetic resonance imaging (fMRI) by first recording one person's brain activity while she was narrating a story and later on scanning other persons while they listened to this story (Stephens et al., 2010). With near-infrared spectroscopy (NIRS), one person's brain activity was monitored during face-to-face communication with a time resolution of several seconds (Suda et al., 2010). With magnetoencephalography (MEG), more rapid changes were demonstrated, as the dominant coupling of the listener's cortical signals to the reader's voice occurred around 0.5 Hz and 4–6 Hz (Bourguignon et al., 2012).
However, in the above-mentioned studies, the data were obtained in measurements of one person at a time. For example, in the fMRI study by Stephens et al. (2010), brain data from the speaker and the listeners were obtained in separate measurements. In the MEG study, the interaction was more natural as two persons were present all the time, although only the listener's brain activity was measured. However, in these experimental setups, the flow of information was unidirectional, which is not typical for natural real-time social interaction. In addition, if only one subject is measured at a time, the complex pattern of mutually dependent neurophysiological or hemodynamic activities cannot be appropriately addressed.
Real-time two-person neuroscience (Hari and Kujala, 2009; Dumas, 2011; Hasson et al., 2012) requires accurate quantification of both behavioral and brain-to-brain interactions. In fact, brain functions have already been studied simultaneously from two or more participants during common tasks. The first demonstration of this type of “hyperscanning” was by Montague et al. (2002) who connected two fMRI scanners, located in different cities, via the Internet to study the brain activity of socially engaged individuals. No real face-to-face contact was possible as the subjects were neither visually nor auditorily connected and the communication was mediated through button press. This approach has been applied to e.g., a trust game where the time lags inherent to fMRI are not problematic (King-Casas et al., 2005; Tomlin et al., 2006; Chiu et al., 2008; Li et al., 2009).
However, the sluggishness of the hemodynamics limits the power of fMRI in unraveling the brain basis of fast social interaction, such as turn-taking in conversation, that occurs within tens or hundreds of milliseconds. The same temporal limitations apply to NIRS which has been used for studying two persons at the same time (Cui et al., 2012). Thus, methods with higher temporal resolution, such as electroencephalography (EEG) or MEG, are called for in studies of rapid natural social interaction.
EEG has previously been recorded from two to four interacting subjects to study inter-brain synchrony and connectivity during competition and coordination in different types of games (Babiloni et al., 2006, 2007; Astolfi et al., 2010a,b), playing instruments together (Lindenberger et al., 2009), and spontaneous nonverbal interaction and coordination (Tognoli et al., 2007; Dumas et al., 2010). This type of EEG hyperscanning enables visual contact between the participants who can all be placed in the same room.
EEG and MEG provide the same excellent millisecond-range temporal resolution, but MEG may offer other benefits as it enables a more straightforward identification of the underlying neuronal sources (for a recent review, see Hari and Salmelin, 2012). Here we introduce a novel MEG dual-scanning approach to provide both excellent temporal resolution and convenient source identification. In our setup, two MEG devices, located in separate MEG laboratories about 5 km apart, are synchronized and connected via the Internet. The subjects can communicate with each other via telephone lines. The feasibility of the developed MEG-to-MEG link was tested by recording time-sensitive cortical auditory evoked fields to sounds delivered from both MEG sites.
Methods
General
Figure 1 (top) shows the experimental setup. MEG signals were recorded with two similar 306-channel neuromagnetometers—one at the MEG Core, Brain Research Unit (BRU), Aalto University, Espoo, and the other at BioMag laboratory (BioMag) at the Helsinki University Central Hospital, Helsinki; both devices are located within high-quality magnetically shielded rooms (MSRs), and the sites are separated by 5 km.
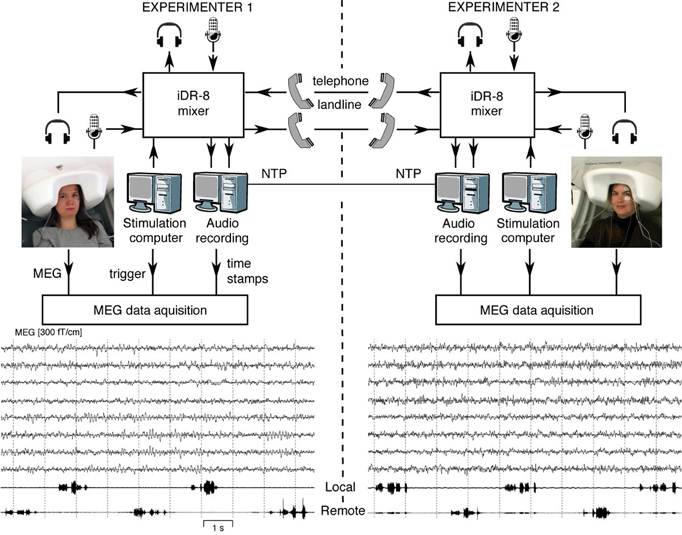
Figure 1. Schematics of the MEG-to-MEG link and examples of ongoing MEG signals. Subjects seated in laboratories 5 km apart are communicating via landline phones during the MEG measurement. The experimenters at both sites can monitor online both data acquisition audio communication. The audio recording computer sends digital timing signals to the MEG data acquisition computers at both sites. Examples of the 10 s MEG signals from four temporal-lobe and four occipital-lobe gradiometers are given below, passband 0.1–40 Hz. The two lowest traces show the audio recording of speech while the participants counted numbers in alternation.
We constructed a short-latency audio communication system that enables connecting two MEG recording sites. Specifically, the system allows:
- Free conversation between the two subjects located at the two laboratories.
- Instructing both subjects by an experimenter at either site.
- Presentation of acoustic stimuli from either laboratory to both subjects.
Each laboratory is equipped with a custom-built system for recording the incoming and outgoing audio streams. The audio recording systems of both sites are synchronized with the local MEG devices and to each other, allowing millisecond-range alignment of the MEG and audio data streams.
Audio-Communication System
We devised a flexible audio-communication system for setting up audio communication between the subjects in the MSRs and/or experimenters in the MEG control rooms at the two laboratories. The system comprises two identical sets of hardware at the two sites, each including:
- An optical microphone (Sennheiser MO2000SET; Sennheiser, Wedemark, Germany) used for picking up the voice of the subject inside the MSR. The microphone is MEG-compatible and provides good sound quality.
- Insert earphones with plastic tubes between the ear pieces and the transducer (Etymotic ER-2, Etymotic Research, Elk Grove Village, IL, USA) to deliver the sound to the subject.
- Microphones and headphones for the experimenter in the control room.
- Two ISDN landline phone adapters enabling communication between the laboratories.
- An 8-channel full-matrix digital mixer (iDR-8; Allen & Heath, Cornwall, United Kingdom) connected to all the audio sources and destinations described above. Additionally, the mixer is connected to the local audio recording system and the stimulus computer.
To eliminate the problem of crosstalk between the incoming and outgoing audio streams, each of the two ISDN telephone landlines was devoted for streaming the audio in one direction only.
In “free” conversation experiments, the two subjects can talk to each other and the experimenters at both sites can listen to the conversation. In a simple auditory stimulation experiment (reported below), sounds can be delivered from the stimulus computer at one site to both subjects.
Latencies of Sound Transmission
We examined the delays introduced by our setup into the audio streams:
- The silicone tubes used for delivering the sound to subject's ear introduced a constant delay of 2.0 ms.
- Each mixer introduced a constant delay of 2.7 ms from any input to any output.
- The delay of the telephone landlines was stable and free of jitter. We estimated this delay before each experiment by measuring the round-trip time of a brief audio signal presented over a loop including the two phone lines and the two mixers; the round-trip time was consistently 16 ms.
In sum, the total local transmission delay from the stimulus computer to the local participant at each laboratory was 2.0 + 2.7 = 4.7 ms.
The lab-to-labtransfer time to the remote laboratory—computed from the local stimulus computer to the participant at the remote laboratory—was 12.7 ms (4.7 ms local transmission delay + 8 ms remote mixer and phone line delay). As the local transmission delays (4.7 ms) were identical for each participant, only the lab-to-lab transfer time was taken into account in the analysis of the two MEG datasets (see below).
Audio Recording
At each site, the audio signals were recorded locally using a dedicated PC (Dell OptiPlex 980) running Ubuntu Linux 10.04 and in-house custom-built audio-recording software. Each PC was equipped with an E-MU 1616 m soundcard (E-MU Systems, Scotts Valley, California, USA), and it recorded the incoming and outgoing audio streams at a sampling rate of 22 kHz. The same audio signals were also recorded by the local MEG system as auxiliary analog input signals (at a rate of 1 kHz) for additional verification of the synchronization.
Synchronization
The audio and MEG data sets were synchronized locally by means of digital timing signals, generated by the audio-recording software and fed from the audio recording computer's parallel port to a trigger channel of the MEG device. To time-lock data from the two sites, the real-time clocks of the audio-recording computers at the two sites were synchronized via the Network Time Protocol (NTP). To pass through the hospital firewall (at BioMag), the NTP protocol was tunneled over a secure shell (SSH) connection established between the sites.
The achieved local audio–MEG synchronization accuracy was about 1 ms. The typical latency of the network connection between the two sites (as measured by the “ping” command) was about 1 ms, and the NTP synchronization accuracy, as reported by the “ntpdate” command, was typically better than 1 ms. Thus we were able to achieve about 2–3 ms end-to-end synchronization accuracy between the two MEG devices. We did not observe any significant loss of the NTP synchronization in a 4.5 h test run.
Stimulation for Auditory Evoked Fields
For recording of cortical auditory evoked fields, 500 Hz 50 ms tone pips (10 ms rise and fall times) were generated with a stimulation PC (Dell Optiplex 755) running Windows XP and the Presentation software (Neurobehavioral Systems Inc., CA, USA; www.neurobs.com; version 14.8 at BRU and version 14.7 at BioMag). The sound level was adjusted to be clearly audible but comfortable for both participants. During each recording session, stimuli were generated at one laboratory and presented to both subjects (locally to the local subject and over the telephone line to the subject at the remote site). Stimulation was synchronized locally by recording the stimulation triggers generated by the Presentation software.
The interstimulus interval was 2005 ms, and each block comprised 120 tones. The stimuli were delivered in two blocks from each site.
Data Acquisition
The MEG signals were recorded with two similar 306-channel neuromagnetometers by Elekta Oy (Helsinki, Finland): Elekta Neuromag® system at BRU and Neuromag Vectorview system at BioMag. Both devices comprise 204 orthogonal planar gradiometers and 102 magnetometers on a similar helmet-shaped surface. However, despite the slightly different electronics and data acquisition systems, the sampling rates were the same within 0.16%. Both devices were situated within high-quality MSRs (at BRU, a three-layer room by Imedco AG, Hägendorf, Switzerland; at BioMag, a three-layer room by Euroshield/ETS Lindgren Oy, Eura, Finland). During the recording, the participants were sitting with their eyes open and their heads were covered by the MEG sensor arrays (see Figure 1).
In addition to the MEG channels, vertical electro-oculogram, stimulus triggers, digital timing signals for synchronization, and audio signals were recorded simultaneously into the MEG data file. All channels of the MEG data file were filtered to 0.03–330 Hz, sampled at 1000 Hz and stored locally.
The position of the subject's head with respect to the sensor helmet was determined with the help of four head-position-indicator (HPI) coils, two attached to mastoids and two attached to the forehead of both hemispheres. Before the measurement, the locations of the coils with respect to three anatomic landmarks (nasion and left and right preauricular points) were determined using a 3-D digitizer before the measurement. The HPI coils were activated before each stimulus block, and the head position with respect to the sensor array was determined on the basis of the signals picked up by the MEG sensors.
External interference on MEG recordings was reduced offline with the signal-space separation (SSS) method (Taulu et al., 2004). Averaged evoked responses were low-pass filtered at 40 Hz. The 900 ms analysis epochs included a 200 ms pre-stimulus baseline.
Data Analysis
For comparable analysis of the two data sets, the 8 ms remote mixer and phone line delay to the remote laboratory had to be taken into account. First, the two datasets were synchronized according to the real-time stamps recorded during the measurement. Thereafter, the triggers in the remote data were shifted forward by 8 ms. With the applied 1000 Hz sampling rate, the accuracy of the correction was 1 ms.
The magnetic field patterns of the auditory evoked responses were modeled with equivalent current dipoles, one per hemisphere. The dipoles were found by a least-squares fit to best explain the variance of 28 planar gradiometer signals over each temporal lobe.
Results
The lower part of Figure 1 shows, for both subjects, eight unaveraged MEG traces from temporal-lobe and occipital-lobe gradiometers. The two lowest channels below the MEG traces illustrate both the local and remote audio streams, in this case indicating alternate counting of numbers by the two subjects.
Figure 2 shows the source waveforms for the auditory evoked fields modeled as current dipoles located in the supratemporal auditory cortices of each hemisphere. For both subjects, N100m peak latencies were similar for tones presented locally (black lines) and over the auditory link (red lines). Response amplitudes were well replicable both for the local and the remote presentations, as is evident from the superimposed two traces for both conditions; Table 1 shows source strengths and peak latencies for both subjects and stimulus repetitions.
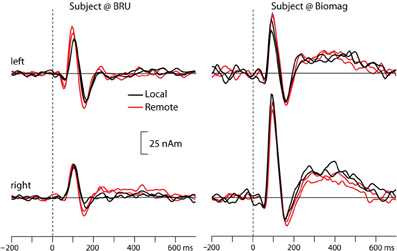
Figure 2. Source waveforms of averaged auditory evoked fields from both participants to tones presented locally (black lines) and remotely (red lines), separately for the left and right hemisphere. The superimposed traces illustrate replications of the same stimulus block. Please note that we did not rigorously control the sound intensities in this proof-of-the-concept experiment, and thus the early difference between local and remote sound presentations in the left hemisphere of the BRU subject likely reflects differences in sound quality.
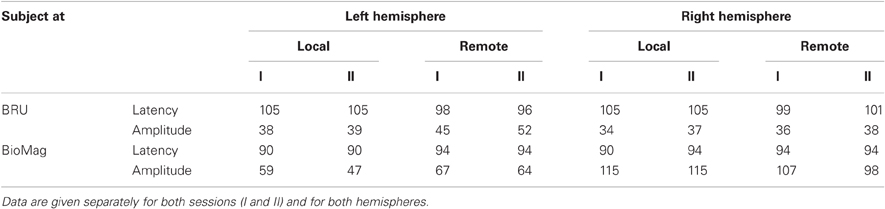
Table 1. Source strengths (in nAm) and peak latencies (in ms) of auditory evoked magnetic fields elicited by tones presented locally or remotely to the subjects located at BRU (top panel), and at BioMag laboratory (bottom panel).
Discussion
We introduced a novel MEG-to-MEG setup to study two interacting subjects' brain activity with good temporal and reasonable spatial resolution. The impetus for this work derives from the view that dyads rather than individuals form the proper analysis units in studies of the brain basis of social interaction (Hari and Kujala, 2009; Dumas, 2011). Within this kind of “two-person neuroscience” framework, it is evident that one cannot obtain all the necessary information by studying just one person at a time, and simultaneous recordings of the two interacting persons' brain function are required.
It is well known that just the presence of another person affects our behavior. Daily social life comprises various types of interactions, from unfocused encounters happening on busy streets (where the main obligation is not to bump into strangers, and—should it happen—to politely apologize) to focused face-to-face interactions with colleagues, friends, and family members. We spend much time observing other people's lives that intrude into our homes via audiovisual media and literature. Normal social interaction is, however, more symmetric and mutual so that information flows in both directions, with verbal and nonverbal cues tightly coupled.
Social interaction is characterized by its rapid and poorly predictable dynamics. One important issue for any hyperscanning approach is thus the required time resolution. The facial expression of a speaker can change clearly even during a single phoneme (Peräkylä and Ruusuvuori, 2006), and to pick up the brain effects of such fleeting nonverbal cues requires a temporal resolution not worse than a hundred or tens of milliseconds (Hari et al., 2010); similar time scales would be also needed for monitoring of brain events related to turn-taking in a conversation (Stivers et al., 2009).
Moreover, brain rhythms that have been hypothesized to play an important role in social interaction (Wilson and Wilson, 2005; Tognoli et al., 2007; Schroeder et al., 2008; Lindenberger et al., 2009; Scott et al., 2009; Dumas et al., 2010; Hasson et al., 2012) are very fast (5–20 Hz) compared with hemodynamic variations and can be only picked up by electrophysiological methods. However, the below 1 Hz modulations of neuronal signals have clear correlates in the hemodynamics (Magri et al., 2012), meaning that the electrophysiological (MEG/EEG) and hemodynamic (fMRI/NIRS) approaches complement each other in the study of the brain basis of social interaction.
Compared with EEG, the rather straightforward source analysis of MEG is beneficial for pinpointing the generators of both evoked responses and spontaneous activity. For example, the differentiation of the rolandic mu rhythm from the parieto-occipital alpha rhythm (for a review, Hari and Salmelin, 1997), appearing in overlapping frequency bands, is easy with MEG—often evident just by examining the spatial distributions of the signals at the sensor level—but the corresponding differentiation is strenuous with EEG because extracerebral tissues smear the potential distribution that is also affected by the site of the reference electrode (Hari and Salmelin, 2012).
Our MEG-to-MEG setup, with its high temporal resolution and reasonable spatial resolution, therefore, provides a promising tool for studying the brain basis of social interaction. In the following, we discuss the technical aspects and future applications of the established MEG-to-MEG link.
Technical Performance of the MEG-to-MEG Link
Our major technical challenge in building the MEG-to-MEG link was to create a stable and short-latency audio connection between two laboratories. Both these criteria were met. The obtained 12.7 ms lab-to-lab transmission time corresponds to sound lags during normal conversation between participants about 4 m apart. Thus, our subjects were not able to perceive the delays of the audio connection.
High sound quality was another central requirement, and the selected optical microphones and the telephone-line bandwidth were sufficient for effortless speech communication.
Moreover, it was crucial to accurately synchronize the MEG datasets of the two laboratories. We achieved offline alignment accuracy of 3 ms by synchronizing the computers at the two sites to a real-time clock via NTP, and by recording the digital timing signal to both MEG data files. As a result, the millisecond temporal resolution of MEG was preserved in the analysis of the two subjects' brain signals in relation to each other.
Recording of auditory evoked cortical responses, used as a “physiological test” of the connection, also endorsed the good quality of the established MEG-to-MEG link: the prominent 100 ms deflections were similar in amplitude and latency when the stimuli were presented from either laboratory.
Further Development and Applications
The current setup with combined MEG and audio recordings could be extended to multi-person interaction studies with only a few extra steps, even connecting subjects located in various parts of the world. As the major part of human-to-human interaction is nonverbal, one evident further development is the implementation of an accurate video connection that, however, will inherently involve longer time lags than does the audio connection.
Face-to-face interaction, obtainable with such a video link, gives immediate feedback about the success and orientation of the interaction. Fleeting facial expressions that uniquely color verbal messages in a conversation are impossible to be mimicked in a conventional brain-imaging setting where one prefers to study all participants in as equal conditions as possible.
The MEG-to-MEG connection can be further enriched by adding, e.g., eye tracking and/or measures of the autonomic nervous system. Just glancing at another person, even briefly, during the interaction gives information about the mutual understanding between them; for example, too sluggish reactions would be interpreted as lack of presence of the partner. Eye gaze also informs about turn-taking times in conversation, and gaze directed to the same object tells about shared attention. Eye-gaze analysis has already given interesting results on the synchronization of two persons' behavior (Kendon, 1967; Richardson et al., 2007).
It has to be emphasized that analysis of the two-person datasets still remains the bottleneck in dual scanning experiments. The analysis approaches attempted so far range from looking at the similarities between the participants' brain signals, searching for inter-subject coupling at different time scales, and combining the two persons' data to obtain a more integrative view of the whole situation. In a recent joint improvisation task—applying a mirror game where two persons follow and lead each other without any pre-set action goals—the participants entered in smooth co-leadership states in which they did not know who was leading and who was following (Noy et al., 2011). Thus any causality measures trying to quantify information flow from one brain to another during a real-life-like interaction likely run into problems. This example also illustrates the uniqueness of real-life interaction: it would be impossible to recreate exactly the same states even if the same participants would be involved again. Thus measuring the brain activity of both interaction partners at the same time is crucial for tracking down any coupling between their brain activities.
One may try to predict one person's brain activity with the data of the other or to use, e.g., machine-learning algorithms to “decode” from brain signals joint states of social interaction, such as turn-taking in a conversation. Beyond these data-driven approaches, this field of research calls for better conceptual basis for the experiments, analysis, and interpretations. One of the first steps is, however, the acquisition of reliable data, to which purpose the current work contributes.
Conflict of Interest Statement
The authors declare that the research was conducted in the absence of any commercial or financial relationships that could be construed as a potential conflict of interest.
Acknowledgments
This study was financially supported by European Research Council (Advanced Grant #232946), the Academy of Finland (National Centers of Excellence Programme 2006–2011, grant #129678; #218072, #131483, and grant LASTU #135198), the aivoAALTO research project of Aalto University (http://www.aivoaalto.fi), the Finnish Graduate School of Neuroscience, and the Jenny and Antti Wihuri Foundation. Special thanks go to Ronny Schreiber for his insightful technical contributions. We also thank Helge Kainulainen, Jussi Nurminen, and Petteri Räisänen for expert technical help, and Cristina Campi, Aapo Hyvärinen, Sami Kaski, and Arto Klami for discussions about signal-analysis issues of the two-person data sets, as well as Antti Ahonen and Erik Larismaa at Elekta Oy for support.
References
Anders, S., Heinzle, J., Weiskopf, N., Ethofer, T., and Haynes, J. D. (2011). Flow of affective information between communicating brains. Neuroimage 54, 439–446.
Astolfi, L., Cincotti, F., Mattia, D., De Vico Fallani, F., Salinari, S., Vecchiato, G., Toppi, J., Wilke, C., Doud, A., Yuan, H., He, B., and Babiloni, F. (2010a). Imaging the social brain: multi-subjects EEG recordings during the “Chicken's game”. Conf. Proc. IEEE Eng. Med. Biol. Soc. 2010, 21734–1737.
Astolfi, L., Toppi, J., De Vico Fallani, F., Vecchiato, G., Salinari, S., Mattia, D., Cincotti, F., and Babiloni, F. (2010b). Neuroelectrical hyperscanning measures simultaneous brain activity in humans. Brain Topogr. 23, 243–256.
Babiloni, F., Cincotti, F., Mattia, D., De Vico Fallani, F., Tocci, A., Bianchi, L., Salinari, S., Marciani, M., Colosimo, A., and Astolfi, L. (2007). High resolution EEG hyperscanning during a card game. Conf. Proc. IEEE Eng. Med. Biol. Soc. 2007, 4957–4960.
Babiloni, F., Cincotti, F., Mattia, D., Mattiocco, M., De Vico Fallani, F., Tocci, A., Bianchi, L., Marciani, M. G., and Astolfi, L. (2006). Hypermethods for EEG hyperscanning. Conf. Proc. IEEE Eng. Med. Biol. Soc. 1, 3666–3669.
Becchio, C., Sartori, L., and Castiello, U. (2010). Toward you: the social side of actions. Curr. Dir. Psychol. Sci. 19, 183–188.
Bourguignon, M., De Tiege, X., Op De Beeck, M., Ligot, N., Paquier, P., Van Bogaert, P., Goldman, S., Hari, R., and Jousmäki, V. (2012). The pace of prosodic phrasing couples the reader's voice to the listener's cortex. Hum. Brain Mapp. [Epub ahead of print].
Chiu, P. H., Kayali, M. A., Kishida, K. T., Tomlin, D., Klinger, L. G., Klinger, M. R., and Montague, P. R. (2008). Self-responses along cingulate cortex reveal quantitative neural phenotype for high-functioning autism. Neuron 57, 463–473.
Cui, X., Bryant, D. M., and Reiss, A. L. (2012). NIRS-based hyperscanning reveals increased interpersonal coherence in superior frontal cortex during cooperation. Neuroimage 59, 2430–2437.
Dumas, G., Lachat, F., Martinerie, J., Nadel, J., and George, N. (2011). From social behaviour to brain sychronization: review and perspectives in hyperscanning. IRBM 32, 48–53.
Dumas, G., Nadel, J., Soussignan, R., Martinerie, J., and Garnero, L. (2010). Inter-brain synchronization during social interaction. PLoS One 5:e12166. doi: 10.1371/journal.pone.0012166
Hari, R., and Kujala, M. V. (2009). Brain basis of human social interaction: from concepts to brain imaging. Physiol. Rev. 89, 453–479.
Hari, R., Parkkonen, L., and Nangini, C. (2010). The brain in time: insights from neuromagnetic recordings. Ann. N.Y. Acad. Sci. 1191, 89–109.
Hari, R., and Salmelin, R. (1997). Human cortical oscillations: a neuromagnetic view through the skull. Trends Neurosci. 20, 44–49.
Hari, R., and Salmelin, R. (2012). Magnetoencephalography: from SQUIDs to neuroscience Neuroimage 20th Anniversary Special Edition. Neuroimage [Epub ahead of print].
Hasson, U., Ghazanfar, A. A., Galantucci, B., Garrod, S., and Keysers, C. (2012). Brain-to-brain coupling: a mechanism for creating and sharing a social world. Trends Cogn. Sci. 16, 114–121.
King-Casas, B., Tomlin, D., Anen, C., Camerer, C. F., Quartz, S. R., and Montague, P. R. (2005). Getting to know you: reputation and trust in a two-person economic exchange. Science 308, 78–83.
Kendon, A. (1967). Some functions of gaze-direction in social interaction. Acta Psychol. (Amst.) 26, 22–63.
Li, J., Xiao, E., Houser, D., and Montague, P. R. (2009). Neural responses to sanction threats in two-party economic exchange. Proc. Natl. Acad. Sci. U.S.A. 106, 16835–16840.
Lindenberger, U., Li, S. C., Gruber, W., and Muller, V. (2009). Brains swinging in concert: cortical phase synchronization while playing guitar. BMC Neurosci. 10, 22.
Magri, C., Schridde, U., Murayama, Y., Panzeri, S., and Logothetis, N. K. (2012). The amplitude and timing of the BOLD signal reflects the relationship between local field potential power at different frequencies. J. Neurosci. 25, 1395–1407.
Montague, P. R., Berns, G. S., Cohen, J. D., McClure, S. M., Pagnoni, G., Dhamala, M., Wiest, M. C., Karpov, I., King, R. D., Apple, N., and Fisher, R. E. (2002). Hyperscanning: simultaneous fMRI during linked social interactions. Neuroimage 16, 1159–1164.
Noy, L., Dekel, E., and Alon, U. (2011). The mirror game as a paradigm for studying the dynamics of two people improvising motion together. Proc. Natl. Acad. Sci. U.S.A. 108, 2047–20952.
Peräkylä, A., and Ruusuvuori, J. (2006). “Facial expression in an assessment,” in Video Analysis: Methodology and Methods eds Knoblauch, H., Schnettler, B., Raab, J., Soeffner, H. G. (Frankfurt am Main: Peter Lang), 127–142.
Richardson, D. C., Dale, R., and Kirkham, N. Z. (2007). The art of conversation is coordination: common ground and the coupling of eye movements during dialogue. Psychol. Sci. 18, 407–413.
Schippers, M. B., Gazzola, V., Goebel, R., and Keysers, C. (2009). Playing charades in the fMRI: are mirror and/or mentalizing areas involved in gestural communication? PLoS One 4:e6801. doi: 10.1371/journal.pone.0006801
Schippers, M. B., Roebroeck, A., Renken, R., Nanetti, L., and Keysers, C. (2010). Mapping the information flow from one brain to another during gestural communication. Proc. Natl. Acad. Sci. U.S.A. 107, 9388–9393.
Schroeder, C. E., Lakatos, P., Kajikawa, Y., Partan, S., and Puce, A. (2008). Neuronal oscillations and visual amplification of speech. Trends Cogn. Sci. 12, 106–113.
Scott, S. K., McGettigan, C., and Eisner, F. (2009). A little more conversation, a little less action–candidate roles for the motor cortex in speech perception. Nat. Rev. Neurosci. 10, 295–302.
Singer, T. (2012). The past, present, and future of social neuroscience: an European perspective. Neuroimage [Epub ahead of print].
Stephens, G. J., Silbert, L. J., and Hasson, U. (2010). Speaker–listener neural coupling underlies successful communication. Proc. Natl. Acad. Sci. U.S.A. 107, 14425–14430.
Stivers, T., Enfield, N. J., Brown, P., Englert, C., Hayashi, M., Heinemann, T., Hoymann, G., Rossano, F., De Ruiter, J. P., Yoon, K. E., and Levinson, S. C. (2009). Universals and cultural variation in turn-taking in conversation. Proc. Natl. Acad. Sci. U.S.A. 106, 10587–10592.
Suda, M., Takei, Y., Aoyama, Y., Narita, K., Sato, T., Fukuda, M., and Mikuni, M. (2010). Frontopolar activation during face-to-face conversation: an in situ study using near-infrared spectroscopy. Neuropsychologia 48, 441–447.
Taulu, S., Simola, J., and Kajola, M. (2004). MEG recordings of DC fields using the signal space separation method (SSS). Neurol. Clin. Neurophysiol. 2004, 35.
Tognoli, E., Lagarde, J., Deguzman, G. C., and Kelso, J. A. (2007). The phi complex as a neuromarker of human social coordination. Proc. Natl. Acad. Sci. U.S.A. 104, 8190–8195.
Tomlin, D., Kayali, M. A., King-Casas, B., Anen, C., Camerer, C. F., Quartz, S. R., and Montague, P. R. (2006). Agent-specific responses in the cingulate cortex during economic exchanges. Science 312, 1047–1050.
Keywords: dual recording, magnetoencephalography, MEG, social interaction, auditory cortex, synchronization
Citation: Baess P, Zhdanov A, Mandel A, Parkkonen L, Hirvenkari L, Mäkelä JP, Jousmäki V and Hari R (2012) MEG dual scanning: a procedure to study real-time auditory interaction between two persons. Front. Hum. Neurosci. 6:83. doi: 10.3389/fnhum.2012.00083
Received: 14 February 2012; Paper pending published: 15 March 2012;
Accepted: 22 March 2012; Published online: 10 April 2012.
Edited by:
Chris Frith, University College London, UKReviewed by:
Ivana Konvalinka, Aarhus University, DenmarkJames Kilner, University College London, UK
Copyright: © 2012 Baess, Zhdanov, Mandel, Parkkonen, Hirvenkari, Mäkelä, Jousmäki and Hari. This is an open-access article distributed under the terms of the Creative Commons Attribution Non Commercial License, which permits non-commercial use, distribution, and reproduction in other forums, provided the original authors and source are credited.
*Correspondence: Riitta Hari, Brain Research Unit, O.V. Lounasmaa Laboratory, School of Science, Aalto University, P.O. Box 15100, FI-00076 AALTO, Espoo, Finland. e-mail:cmlpdHRhLmhhcmlAYWFsdG8uZmk=