- 1Institut de Neurosciences de la Timone, Centre National de la Recherche Scientifique - Aix-Marseille University, Marseille, France
- 2Autism Resources Center, Sainte Marguerite Hospital, AP-HM, Marseille, France
- 3Adolescent Psychiatry Unit, Salvator Hospital, AP-HM, Marseille, France
- 4Institute for Cognitive Systems, Technische Universität München, München, Germany
Mentalizing is defined as the inference of mental states of fellow humans, and is a particularly important skill for social interactions. Here we assessed whether activity in brain areas involved in mentalizing is specific to the processing of mental states or can be generalized to the inference of non-mental states by comparing brain responses during the interaction with an intentional and an artificial agent. Participants were scanned using fMRI during interactive rock-paper-scissors games while believing their opponent was a fellow human (Intentional agent, Int), a humanoid robot endowed with an artificial intelligence (Artificial agent, Art), or a computer playing randomly (Random agent, Rnd). Participants' subjective reports indicated that they adopted different stances against the three agents. The contrast of brain activity during interaction with the artificial and the random agents didn't yield any cluster at the threshold used, suggesting the absence of a reproducible stance when interacting with an artificial intelligence. We probed response to the artificial agent in regions of interest corresponding to clusters found in the contrast between the intentional and the random agents. In the precuneus involved in working memory, the posterior intraparietal suclus, in the control of attention and the dorsolateral prefrontal cortex, in executive functions, brain activity for Art was larger than for Rnd but lower than for Int, supporting the intrinsically engaging nature of social interactions. A similar pattern in the left premotor cortex and anterior intraparietal sulcus involved in motor resonance suggested that participants simulated human, and to a lesser extend humanoid robot actions, when playing the game. Finally, mentalizing regions, the medial prefrontal cortex and right temporoparietal junction, responded to the human only, supporting the specificity of mentalizing areas for interactions with intentional agents.
Introduction
“In considering the functions of the mind or the brain we find certain operations which we can explain in purely mechanical terms. This we say does not correspond to the real mind: it is a sort of skin which we must strip off if we are to find the real mind. But then in what remains we find a further skin to be stripped off, and so on. Proceeding in this way do we ever come to the “real” mind, or do we eventually come to the skin which has nothing in it? In the latter case the whole mind is mechanical.”
Alan T. Turing, 1950
Is the human mind mechanical? This question mirrors another asked by Alan M. Turing, one of the founders of artificial intelligence: “Can machine think?” (Turing, 1950). In his article, Turing introduced an imitation game to test whether artificial intelligence can equal human intelligence, hence “think.” In this game, a human judge communicates with two agents hidden in other rooms through a teleprinter. One of the agents is a fellow human, the other is an artificial intelligence, and the judge has to decide which of the two agents is the computer. The artificial intelligence “passes” the “Turing Test” if it can fool the judge into believing it is the human. In that case the computer can reproduce the relevant aspects of humans' intelligent conversation using its computations (Turing, 1950). In contrast, the inability of machines to pass the Turing Test, that is to imitate a human being in a conversation, implies that our interactions with fellow humans have something that can not be captured by an artificial agent, that philosopher Daniel Dennett called the “intentional stance” (Dennett, 1987). In his view, no matter how complex the computations an artificial intelligence can accomplish it will not be intentional.
But how would we react if intentional and artificial agents were indistinguishable, but we were aware of their nature? The current experiment was designed to investigate whether we use the same neural mechanisms, in particular in brain regions involved in the processing of fellow humans' mental states, when believing we interact with a natural or with an artificial intelligence. It could not be implemented as a free rolling conversation with humans and artificial agents, if only because today's artificial intelligence systems' attempts to pass the Turing Test are still inconclusive. But as our focus was on how laymen in computer sciences and robotics think machines think, and not how the machine actually thinks, we were able to restrict the interactions to a highly controllable environment: participants believed they played with a fellow human or a humanoid robot endowed with an artificial intelligence, while the interaction with both agents was effectively similar.
A humanoid robot was used to provide embodiment to the artificial intelligence to the otherwise disembodied game. Humanoid robots are also interesting as a technology on the verge of becoming commonplace. While recent advances have indeed provided increasingly complex and interactive anthropomorphic robots, little is known about how human social cognition mechanisms adapt to these new interactive partners (Chaminade and Cheng, 2009). Research on how humans interact with these artificial agents is, therefore, increasingly important as human-robot interactions will impact issues of public concern in the near future, in particular when assistive technologies for education and healthcare will be concerned (Billard et al., 2007; Dautenhahn, 2007; Mataric et al., 2009; Chaminade and Kawato, 2011).
Recent neuroimaging research focused on the effect of robots' appearance and motion on brain activity (Chaminade et al., 2010; Cross et al., 2011; Saygin et al., 2011), emphasising the consequences of artificial agents' anthropomorphism without informing the observers of the algorithms controlling their behavior. Another line of research compared the neural bases of social interactions using economic games played against a human to the same games played against a computer (McCabe et al., 2001; Rilling et al., 2004), but didn't provide information about the algorithm controlling the computer's output. The computer was considered as the low-level control for intentional human behaviours. In one experiment a humanoid and a functional robot were used to induce different stances in participants playing the prisoner's dilemma game (Krach et al., 2008), but the authors didn't explicitly induce the belief that any of these robots had been endowed with a specific artificial intelligence.
In the present experiment, participants played a computer version of the rock-paper-scissors game, as in Gallagher et al. (2002), against a fellow human (Intentional agent, Int) or against a humanoid robot (Artificial agent, Art), both agents actively playing in order to beat the participants. The robot was presented as endowed with an artificial intelligence developed to play the game actively and efficiently by relying on a specifically developed computer algorithm. In a control condition the opponent was presented as a computer playing randomly (Random agent, Rnd), as in Krach et al. (2008); its responses couldn't be anticipated so that no strategy could be developed to beat it. Importantly, the games sequences, in terms of wins and losses, were prepared in advance and exactly similar across the three opponents, so that only the stance adopted by the scanned participant when playing against the three agents, the fellow human, the robot endowed with artificial intelligence and the random computer, changed.
Our hypotheses were two-fold. First, we expected that playing against the intentional or the artificial agent would yield similar responses in specific regions of the brain. As participants were made to believe that both active opponents, but not the random computer, followed a strategy, they attempted to understand these strategies in order to increase the number of games won, similar processes should be engaged when playing against the intentional and artificial agents. Practically, when sensorimotor aspects of the game are removed by subtraction of condition Rnd, both Int and Art should require similar cognitive mechanisms, such as keeping track of the opponent games (working-memory), attempting to find regularities in the previous games (problem-solving) and choosing the next response accordingly (response selection), all functions subtended by the dorsolateral prefrontal cortex (Petrides, 2005). We, therefore, predicted that clusters in the frontal cortex would respond similarly to the two active opponents compared to the random computer.
Second, as the adopted stance is supposed to differ depending on whether one is interacting with a human or with an artificial agent, the cognitive and neural mechanisms recruited when playing against these two opponents should yield differences, with brain areas specifically involved in the interaction with each one. Humans particularly developed social skills have been the focus of intense investigations in functional neuroimaging, which have revealed two mechanisms that play an important role. Motor resonance is the generalization of the finding of mirror neurons, that discharge both when a macaque monkey performs an action and when it sees another agent performing an action (Rizzolatti and Craighero, 2004), and it is believed to play a role in action perception, action understanding, imitation, and social bonding in human cognition (Chaminade and Decety, 2001; Rizzolatti and Craighero, 2004). At the brain level, the inferior parietal lobule including the anterior intraparietal sulcus and the premotor cortex are the main locations of motor resonance. No activity in these areas was predicted in the present experiment provided that interaction with, and not observation of, the interactive agents was investigated.
Mentalizing is the inference of the hidden mental states, such as intentions, desires and beliefs, that cause intentional agents' behavior, and it is particularly important for social interactions (Frith and Frith, 1999). Its neural correlates include prominently the medial prefrontal cortex and the temporoparietal junction (Frith and Frith, 1999). Increased response in these regions (Saxe and Kanwisher, 2003; Amodio and Frith, 2006) has been repeatedly reported for interacting with an intentional agent, including in experimental settings similar to the one used here (Gallagher et al., 2002; Krach et al., 2008). It was proposed that the medial prefrontal cortex “supports a general mechanism for the integration of complex representations of possible actions and anticipated outcomes […] particularly relevant to the domain of social cognition” (Amodio and Frith, 2006). Here we assessed whether activity in these regions is specific to the processing of mental states or can be generalized to the inference of non-mental hidden states. Response of the temporoparietal junction and medial prefrontal cortex activated during the interaction with human fellow was, therefore, examined during the interaction with the artificial intelligence, characterized by hidden states that are not intentional but computational. The finding of an activation in mentalizing areas when interacting with an artificial intelligence would parallel the finding that motor resonance generalizes to the perception of anthropomorphic robots (Chaminade and Cheng, 2009), implying that similar cognitive mechanisms are at play when manipulating mental or mechanistic hidden states during an interaction. Alternatively, interacting with an artificial intelligence could engage areas involved in calculus and rule-solving computations, in the left intraparietal sulcus (Simon et al., 2002) and anterior part of the prefrontal cortex (Koechlin et al., 2003; Badre, 2008), respectively.
Methods
Participants
Nineteen healthy male volunteers (mean age 21.5 years, SD 4.9 years), with no history of neurological or psychiatric diseases according to self-report, gave informed consent and were indemnified 40€ to participate to this fMRI experiment, that was approved by the local ethics committee “CPP Sud-Marseille-1,” approval number 2010-A00508-31. One participant was excluded due to his inability to perform the task and excessive movements. All were students in local universities and engineering schools. Right-handedness was confirmed by the questionnaire of Edinburgh (Oldfield, 1971).
Experimental Paradigm
The experimental paradigm used the game Rock-Paper-Scissors similar to that used in Gallagher et al. (2002), and a briefing procedure similar to that used in Krach et al. (2008). As support for the allegedly live interaction, participants were playing a computerized version of the game (Figure 1): the three choices, paper, scissors, and stone were presented on screen during a “2–1–0” countdown, each frame lasting 2/3 of a second, and participants were asked to respond during the “0” frame by clicking on one of three buttons with the thumb, index or middle fingers of their right hand, respectively. The key-response mapping was kept constant to avoid the recruitment of attentional resources for sensorimotor remapping during the experiment. The next screen indicated the result of the game, with the participant's response on the left, the opponent's response on the right, and a color frame around each response providing the result of the game (green for win, red for loss and yellow for tie). Series of five games were played consecutively in a round against a given opponent, and five rectangular markers at the top of the screen kept track of games results as the round unravelled (–: not played, X: loss, +: gain, O: tie). For each given 15 s round, a 2 s video before and a 2.5 s video feedback after showed the opponent being played against. Different videos were used for each individual game. No video or image of the opponent was shown when the games were actually being played. Three 9 min functional runs, consisting each of seven games against each of the three opponents, for a total of 21 games against each opponent, were scanned. Unbeknown to the participants, all games' results and sequences of videos were prerecorded in an experimental script in order to provide an equal number of gains and losses across all opponents, spanning the entire spectrum of possibilities (from 5 wins to 5 losses), according to their likelihood in a random sequence of five games (in the script, an overall tie is four times more likely—and happens four times—while 5 losses or 5 wins happens only once). The experimental paradigm and the synchronisation with fMRI recordings were accomplished with an ad-hoc LabView 8.6 program running on a National Instrument PXI-1031 chassis computer.
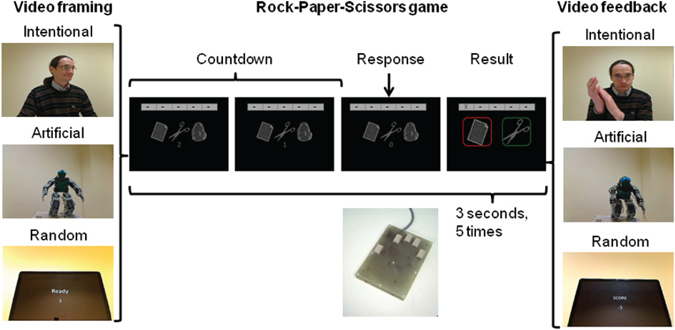
Figure 1. Experimental paradigm. Each game of Rock-Paper-Scissors consisted of a countdown, a response screen and a result screen, and lasted 3 s. Five games were played for a round against one given opponent, and each round was preceded by a 2 s video of the opponent (video framing) and was followed by a 2.5 s video of the opponent providing a feedback on the results of the game (video feedback).
Participants' Briefing
All participants underwent an extensive verbal presentation of the experimental setting and procedures in the control room adjoining the MRI scanner. The three opponents presented to the participants were the experimenter TC, described as a human agent playing with the usual strategies of a human agent (hence “Intentional agent” Int), a small humanoid robot endowed with an artificial intelligence algorithm specially developed to attempt to win the games by taking into account results of previous games (hence “Artificial agent” Art), and a random number generator embedded in a computer that only kept track of the results to calculate a score at the end of each round of games (hence “Random agent” Rnd). Participants were explicitly explained that as both the intentional and artificial agents used strategies in order to win a maximum of games they should also try to develop a strategy to beat them. In contrast, as the random number generator didn't have any strategy, they shouldn't be able to defeat it. After this presentation they underwent 5 or 10 rounds of training for the Rock-Paper-Scissors game on a test computer to familiarize themselves with the motor requirements of the task.
Then, participants were shown the “game control room” in which the three opponents would be localized during fMRI scanning: the experimenter playing with a keypad similar to the one used in the MRI setting, a humanoid Bioloid robot from Robotis plugged to a computer which hosts the artificial intelligence algorithm, that plays with three arm movements corresponding to the three responses, and a computer providing the random responses. They were further shown the webcam, at the centre of the table, that would allegedly be used to provide them with live feedback of the opponent before and after each round, and shown live examples of what they would see for the three opponents. For the human opponent, the video preceding the game was a gestural invitation to play, and the video following the game displayed an emotional expression relative to the experimenter's score; for the robot opponent, the robot standing up, and arms movements (up for victory, down for defeat); for the computer opponent, the number 3 starting the countdown for the first game of the round, and its score between −5 and 5. While all was presented to make participants believe they were interacting live with the three opponents, all video stimuli were prerecorded in the exact same setting prior to the experiment.
After fMRI scanning participants were given a questionnaire about their habits in computer-related hobbies (use of internet, of social networks, and of computer games) as well as questions about their perception of the games they just played. In particular we asked to what extent they thought they were successful against each of the three opponents, and whether they thought they adopted an efficient strategy against each the three opponents, both on a 5-point Likert scale. Analyses of variance were run on the Z-score transform of participants' ratings for the two questions to assess the effect of the agent. When an effect of the agent was identified, three planned pairwise comparisons between agents (Int-Rnd, Art-Rnd, Int-Art) were calculated.
MRI Acquisition
Data were collected with a 3T BRUKER MEDSPEC 30/80 AVANCE scanner running ParaVision 3.0.2 at Marseille Cerebral Functional MRI centre. Participants lying supine in the scanner were instructed to remain still during the course of the experiment. Stimuli were projected on a mirror located in front of participants' eyes through a mirror and projector located in the back of the scanner. Responses were recorded with a right-hand 5-digit ergonomic MRI-compatible keypad.
After a localizer ensured the participants were correctly positioned in the magnetic field, five scanning runs were performed. First a fieldmap using a double echo FLASH sequence recorded distortions in the magnetic field (FLASH, FOV 192 × 192 × 192 mm3, voxel size 3 × 3 × 3 mm3, TR 30.0 ms, TE 3.700 ms, α = 30°). Three functional runs (EPI, FOV 192 × 192 mm2, pixel size 3 × 3 mm2, 36 interleaved ascending axial slices and each were 3 mm thick without gap, TR 2400.0 ms, TE 30.000 ms, α = 81.6, 232 repetitions, scanning time 9 min 16 s), using the same spatial parameters as the fieldmap, covering the whole-brain parallel to the AC-PC plane, were recorded. Finally a high-resolution T1-weighted 3D image was acquired for each participant (MPRAGE, FOV 256 × 256 × 180 mm3, voxel size 1 × 1 × 1 mm3, TR 9.4 ms, TE 4.424 ms, α = 30°).
fMRI Data Processing
The FieldMap toolbox in SPM8 was used to determine voxel displacements in the EPI image. After discarding the first five EPI images to allow for initial T1-equilibrium, realignment, and unwarping procedures were applied to fMRI time series to correct for both the static distortions of the magnetic distortions with the voxel displacement map obtained from the fieldmap and the movement-induced distortions of the time series (Hutton et al., 2002). The mean image created during realignment was coregistered with the high-resolution anatomical image, that was normalized to SPM8 T1 template, and the convolved normalization and coregistration transformations were applied to the realigned EPI time series. A 9 mm FWHM Gaussian kernel smoothing was applied to EPI images prior to statistical analysis.
In the single-subject analyses, rounds of interaction with each of the three opponents were modelled as 15 s boxcar functions blocks synchronised with the onset of the first countdown image, and videos presented before and after each round of games were modelled with 2 s and 2.5 s boxcar functions respectively, for each of the three opponents. The condition regressors were convolved with the canonical hemodynamic response function and a high pass filter with a cut-off of 128 s removed low-frequency drifts in BOLD signal.
Statistical Analysis
Contrast images corresponding to the main effect of the rounds of interaction with each of the three opponents and for each of the three recording sessions were used in a second-level repeated-measure analysis of variance across the 18 participants, using session as a repeated factor of no-interest. Contrasts between conditions were thresholded at p < 0.05 family wise error (FWE) corrected and extent k superior to 25 voxels (200 mm3). When possible anatomical localisations were performed using the Anatomy toolbox in SPM8 (Eickhoff et al., 2007), otherwise using Duvernoy's brain atlas (Duvernoy, 1999). Renders on an average inflated brain were performed using surfrend for SPM8 and freesurfer. Percent signal change was extracted in clusters taking into account all voxels using MarsBAR SPM toolbox implemented in SPM8, and ANOVAs were computed using SPSS to assess the effect of the opponent on percent signal change using sessions as repeated-measures of no interest, as in the whole-brain analysis. Planned pairwise comparisons between agents (Int-Rnd, Art-Rnd, Int-Art) were reported as highly significant (p < 0.001) or significant (p < 0.05).
Results
Debriefing
For logistical reasons debriefing questionnaires were only obtained in the last 12 of the 18 scanned participants. The main observation is that, when considering responses to both questions, about their subjective feeling of success and of efficacy of the strategy used, none of the participants gave the same rate for the three opponents. Informal debriefing with the first six participants indicated that they felt differences in their success rate against the three opponents. Altogether individual responses thus support that each participant perceived differently their success and strategy efficiency against Int, Art, and Rnd.
Group ANOVA indicated no significant effect of the agent being interacted with on the perceived success [F(2, 22) = 0.890, p = 0.428], supporting the absence of a systematic perception of gain or loss toward any of the opponents. On the efficacy of the strategy being used, ANOVA revealed a marginally significant effect [F(2, 22) = 3.553, p = 0.058]. Participants were more confident (p = 0.040) about their strategy when interacting with the human (Int) than the computer (Rnd). Pairwise comparisons between Art and Int and Art and Rnd were both not significant p > 0.1.
Whole-Brain Analysis: Effect of Agents
Repeated-measure whole-brain analysis of variance was used to compute pairwise comparisons between the three agents (Figure 2 and Table 1). The contrast Int-Rnd yielded response in expected brain areas on the basis of our hypotheses, namely in the frontal cortex (left superior frontal, middle frontal and precentral gyrus, right medial prefrontal cortex), in the parietal cortex (precuneus bilaterally, left anterior and posterior intraparietal sulcus, right temporoparietal junction) and well as in the anterior part of the right thalamus.
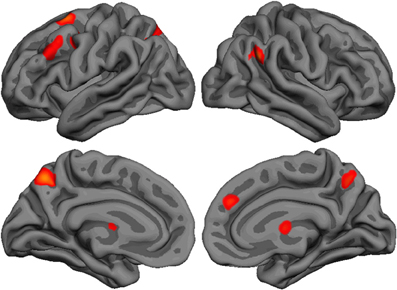
Figure 2. Lateral and medial brain renders (rendered with freesurfer) showing activated clusters (p < 0.05 FWE corrected, k > 25 voxels) for the contrast Int-Rnd.
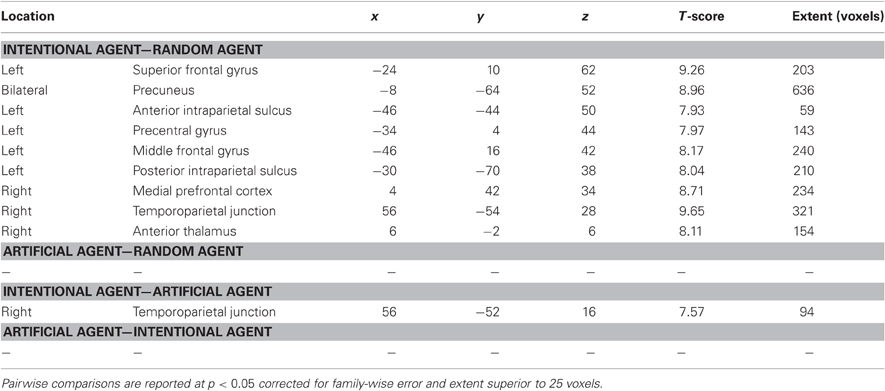
Table 1. Regions of increased BOLD response in comparisons between believing the Rock-Paper-Scissors game is being played against an intentional agent (Int), an artificial agent (Art), and a random number generator (Rnd).
Using the same thresholds, the contrast Art-Rnd yielded no activated cluster. As activated clusters could be found with the more lenient threshold p < 0.001 uncorrected, another approach was used to check whether brain areas responded to the artificial intelligence only with a more lenient threshold: the contrast Art-Rnd (p < 0.001 uncorrected) was exclusively masked with the contrast Int-Rnd (p < 0.001 uncorrected). No region was identified with this approach, supporting the conclusion that no brain region responded specifically to the interaction with the artificial intelligence.
Direct comparisons between the two allegedly interactive opponents yielded only one cluster in the right temporoparietal junction for Int-Art, included in the temporoparietal junction cluster reported in Int-Rnd. No cluster survived at the thresholds used for the reverse comparison Art-Int, nor for the comparisons Rnd-Art and Rnd-Int.
Region of Interest Analysis
To characterize brain responses to artificial intelligence, BOLD percent signal change was extracted in all clusters identified in the contrast Int-Rnd and the response to Art investigated with ANOVAs and t-tests to test the hypothesis that similar neural resources are used to play against the two allegedly active opponents (Figure 3). As expected from the selection of ROIs, there was a highly significant effect of the agent [all F(2, 34) > 20, p < 0.001], and comparisons between Intentional and Random agents were all highly significant (at p < 0.001). More interestingly comparisons between intentional and artificial agents were significant in all ROIs (ps < 0.001) while the significance of the comparison between Artificial and Random agents depended on the ROI: it was highly significant in the anterior intraparietal sulcus and precentral gyrus (both p = 0.001), significant at p < 0.05 in the precuneus and posterior intraparietal sulcus, in the left superior and middle frontal gyrus and in the thalamus, and not significant in the medial prefrontal cortex (p = 0.105) and the right temporoparietal junction (p = 0.207).
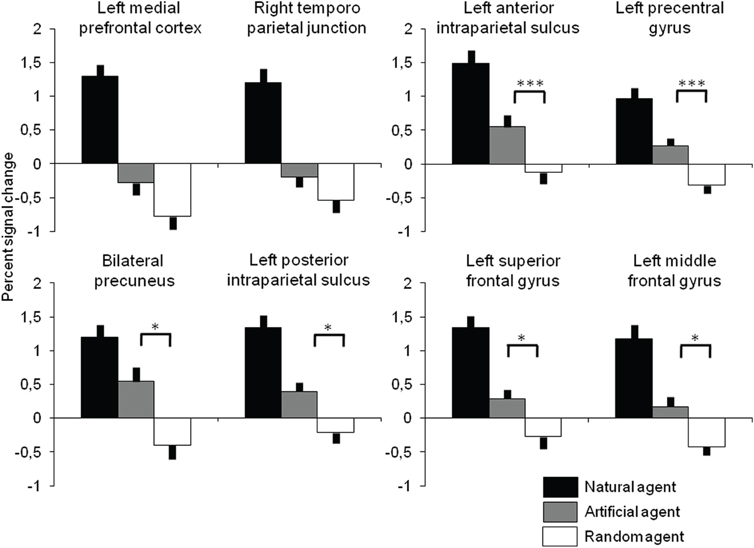
Figure 3. Percent signal change (error bar: standard error) as a function of the opponent extracted in all cortical regions identified in Int-Rnd (see Table 1 and Figure 2). All pairwise comparisons between Intentional agent and the other two opponents are significant at p < 0.001 (not represented). Significant pairwise comparisons between Artificial and Random agents are presented at significant (*p < 0.05) and highly significant (***p < 0.001) thresholds.
Discussion
In this experiment we compared the effect of one's beliefs about the nature of the agent he is interacting with while all other aspects of the interaction, in particular sensorimotor transformations, are controlled. The two actively playing opponents were the experimenter with its intentional intelligence (Int) and a humanoid robot endowed with an artificial intelligence algorithm developed to win the game (Art). A third opponent, a computer providing random responses which couldn't be anticipated (Rnd), was used as a control. Taken individually, each participant considered that his strategy or success rate differed between the three opponents, suggesting that he really believed that there were three different opponents. However, there was no significant effect of the agent on perceived success in group analysis, suggesting there was no consensus on the perception of gain or loss toward the opponents. In contrast, the adopted strategy was considered better against the human than the computer, in agreement with the information given in the briefing that the human was using a strategy and could, therefore, be beaten, while it was not possible to adopt a strategy against a random number generator. As feedbacks from all opponents were controlled experimentally to be similar, these findings clearly indicate that the induction by verbal briefing and the allegedly live videos used during scanning worked efficiently. Local differences in brain activity observed between the three experimental conditions are, therefore, undoubtedly related to differences in the participants' different stances when playing against the three alleged agents.
Increased Involvement in Human-Human Interactions
The fMRI contrast between human playing with intentional intelligence and random player yielded expected results, in the medial prefrontal cortex, the left superior frontal, middle frontal and precentral gyrus, the precuneus and right temporoparietal junction (Krach et al., 2008). As the briefing incited participants to actively look for the opponent strategy when playing against the human and the robot, our null hypothesis was that brain areas involved in playing the game should respond similarly to the two active opponents.
The dorsal precuneus reported here has been associated with working-memory retrieval (Cabeza et al., 2002), and in particular when the content to be retrieved is highly imageable (Cavanna and Trimble, 2006). Keeping track of previous games, depicted as images for the three possible responses, during one round in order to anticipate the forthcoming action by the opponent requires a strong involvement of imagery-based working-memory. The precuneus may also be involved in changing the rules adopted to win (Nagahama et al., 1999): the precuneus was activated when a “wrong” feedback was provided in a card sorting task and the sorting rule had to be adapted by focusing on another aspect of the cards (“color” vs. “shape”). The posterior intraparietal sulcus, that shows a similar effect of agents, is in the human homolog of monkey's lateral intraparietal area LIP (Sereno et al., 2001) involved in eye movement (Simon et al., 2002) and in attentional shifts (Sereno et al., 2001), and could participate together with the precuneus, in orienting attention to the participant and the opponent games results during a round. Keeping track of players' responses and victories is essential to understand the rules behind the opponent's choices.
Frontal regions could be involved in other high-order aspects of the competitive game grouped under the heading of executive functions. One mechanism, the maintenance of a representation of the stimuli for active processing according to the task at hand, involves the middle frontal gyrus (Petrides, 2005), complementary to representing the stimuli in working-memory in the medial parietal cortex (Cabeza et al., 2002). The exact function of the superior frontal gyrus in executive functions is not well established, but a lesion study confirmed it participates to domain-independent working-memory (du Boisgueheneuc et al., 2006). The finding of these areas in Int-Rnd could be explained by the increased executive demands of interacting with an active opponent: “given the results of the previous games (working-memory) and my knowledge about the nature of the opponent (adopted stance), I anticipate that it is more likely to play scissors, eventually stone, at the next game (problem-solving) and I should play stone to win or avoid to lose (response selection).” Within this framework, regions involved in the attentional and executive aspects of the task should have been similarly activated for Int and Art, while results indicate that parietal and prefrontal regions were more activated when the opponent was the intentional than the artificial agent. Increased response in regions devoted to attentional and executive functions when interacting with a peer compared to an artificial intelligence signals an increased involvement in the interaction. A cluster of activity in the thalamus was reported when participants anticipated a social reward in a simple reaction time task [6, 0, 3 in Spreckelmeyer et al. (2009), 6, −2, 6 here], supporting the idea that the increased involvement in the task when playing against the intentional, compared to the artificial, agent is caused by the rewarding value of human-human interactions (Krach et al., 2010).
Motor Resonance when Interacting with Artificial Intelligence
The two brain areas in which the difference between response to Art and Rnd was highly significant in the ROI analysis were the anterior intraparietal sulcus and precentral gyrus of the left hemisphere. Both areas have been reported in previous experiments of human and robot action observation (Chaminade et al., 2010; Saygin et al., 2011), and discussed within the framework of motor resonance. Motor resonance is the mechanism by which the neural substrates involved in the internal representation of actions performed by the self are also recruited when perceiving another individual performing the same action. Artificial agents have been used to gain knowledge on how anthropomorphism affects neural markers of motor resonance (Chaminade and Kawato, 2011), and we reported a similar response to observing a human or a humanoid robot in the right premotor area (Chaminade et al., 2010) comparable to the left precentral gyrus found here, and a large repetition priming effect in response to an android's actions in a left anterior intraparietal sulcus area (Saygin et al., 2011), believed to play a specific role in the perception of action goals (Hamilton and Grafton, 2006). In the present experiment activity in these regions can't be due to action observation as no video feedback was given during the actual games, but may be explained by the participants imagining his or his opponent's actions. As William James put it (James, 1890), “Every representation of a movement awakens in some degree the actual movement which is its object,” which could correspond in the present experiment to a simulation of the opponent's actions, as activity depends on the nature of the agent being played. Interestingly, the result, based on ROI analysis, that both the increase from random to artificial and from artificial to intentional agents are significant parallels the previous finding that observing a robot performing an action induces a reduced motor resonance compared to observing a human agent (Chaminade and Kawato, 2011).
Alternatively, activity in a parietopremotor circuit in the left hemisphere could be caused by higher attentional demands or demands of response selection for Int than for Art. As in the interpretation previously proposed for the parietal and lateral prefrontal clusters, an increased involvement of participants when interacting with the human than with the humanoid robot endowed with an artificial intelligence would cause increased response in brain areas controlling attention and/or the motor response. This interpretation is parsimonious in the sense that it makes use of a conclusion already supported by the response in other regions, namely a greater involvement in interactions with an intentional compared to an artificial agent. Further experiments are required to test whether the parietopremotor circuit discussed in this section should be interpreted as sustaining motor resonance or attentional and motor aspects of the interaction game.
No Intentional Stance against the Artificial Agent
The medial prefrontal cortex was reported in Int-Rnd, though in a more dorsal location that the paracingulate cortex reported for the same contrast in a previous PET experiment using a similar stone-paper-scissors interactive game to investigate the intentional stance (Gallagher et al., 2002). The present cluster was closer to the cluster reported in the left hemisphere for the contrast between playing against a human and rule-solving in Gallagher et al. (2002), while the rule-solving condition was more similar, in terms of the stance adopted, to the present artificial intelligence. The opponent responses were presented as depending on explicit mathematical rules in Gallagher et al. (2002), while they were implicit in the present experiment. Moreover, this was only a cover story in the present experiment, in which all trials were truly random, but partly true in Gallagher et al. (2002): three possible rules could be alternated and all runs had a fully random sequence of trials (10 out of 30) in their midst. It is possible that these differences in experimental conditions or in the neuroimaging technique used (PET vs. fMRI) explain the changes in the exact location of the medial prefrontal cluster associated with adopting an intentional stance. Yet both fall inside the same subdivision of the medial prefrontal cortex as parcelled in Amodio and Frith (2006), the anterior rostral medial prefrontal cortex, presented as specific to mentalizing, that is, thinking about other people's mental states. Furthermore, the same region is found when interacting with a human is compared to a control, non-interactive, condition [x, y, z coordinates 4, 31, 41 in Krach et al. (2008), 4, 42, 34 here] while the interactive game is not competitive but cooperative (prisoner's dilemma).
Similarly the right temporoparietal junction was also reported not only for the contrast between human and control, but as correlated with anthropomorphism [55, −53, 21 in Krach et al. (2008), 56, −54, 28 here]. Altogether, response of the main areas involved in mentalizing, the medial prefrontal cortex and right temporoparietal junction (Frith and Frith, 1999), reproduced existing results from the literature, confirming that the induction of a different stance between interacting with a human intentional agent and with a random number generator was successful. Region of interest analysis indicated that, of all regions found in the contrast Int-Rnd, only these two, the medial prefrontal cortex and the right temporoparietal junction, were not significantly more active in response to the humanoid robot endowed with artificial intelligence than to the random player. As both regions play a central role in mentalizing, that is adopting an intentional stance in an interaction (Frith and Frith, 1999; Gallagher et al., 2002; Amodio and Frith, 2006), our data clearly demonstrate that when participants' belief about the intentional nature of their opponent was manipulated, brain areas involved in adopting an intentional stance in a social interaction were not recruited when interacting with an artificial intelligence.
This raises questions about the absence of specific local brain activity in the contrast between artificial intelligence and random player. This absence is not only due to the stringent threshold used, similar to the one used in a previous experiment (Krach et al., 2008), as the use of a more lenient threshold but with a masking procedure to exclude regions also responding to intentional intelligence also failed to reveal any cluster responding exclusively to the artificial intelligence. While neuroimaging results clearly support that induction worked for interaction with the intentional intelligence, they fail to yield significant results for the interaction with the artificial intelligence. As the same procedure was used during the briefing for the two opponents, it is unlikely that induction only worked for the human opponent. It is also unlikely that participants adopted a similar stance when playing against the artificial intelligence and the random player, as one would expect similar differences in Int-Rnd and Int-Art while these contrasts didn't yield similar activation patterns.
It is possible that in contrast to mentalizing, that forms the cornerstone of social interactions from childhood (Frith and Frith, 1999), and randomness, that can be seen as the absence of causality or as a folk psychology concept like chance, participants did not have an existing representation of what an artificial intelligence is, how it works, and how to interact with it. More generally, while a computer and a humanoid robot are straightforward objects, today's laymen don't have a clear representation of the inner mechanisms of artificial intelligence, and therefore can't develop a cognitive strategy to interact with them. Alternatively, participants could have adopted different stances toward the artificial intelligence depending on their personal views of the inner mechanisms, so that no brain areas would have survived group analysis. Altogether, these results suggest that our sample didn't adopt an intentional stance or any other type of design stance [e.g., problem-solving reflected in the anterior frontal cortex activity (Badre, 2008), arithmetic calculation in the intraparietal sulcus (Simon et al., 2002)] when interacting with the artificial agent (Dennett, 1987). A similar conclusion is supported by the analysis of post experiment questionnaires. Participants reported that their strategy against the intentional human was better than against the random agent, suggesting that they were in different states of mind against the two opponents and adopted an intentional stance when playing against the human. In contrast, there was no consensus on their efficacy against the robot, indicating that there was no consensus between participants' subjective perception of their interaction with the artificial agent.
Conclusion
The contrast between playing stone-paper-scissors against an intentional and a random agent identified brain areas in which we subsequently assessed the response to a humanoid robot endowed with an artificial intelligence. Two types of responses were found. The medial prefrontal and temporoparietal junction responded exclusively to the human agent, while we observed an increase from random to artificial and form artificial to intentional agents in parietal and lateral frontal areas. If the later result supports a greater involvement of participants interacting with a natural than an artificial agent, maybe due to the intrinsically rewarding nature of social interactions, the former result suggests that mentalizing is exclusive to the manipulation of mental, compared to computational, states.
Conflict of Interest Statement
The authors declare that the research was conducted in the absence of any commercial or financial relationships that could be construed as a potential conflict of interest.
Acknowledgments
We thank the volunteers who participated to this research. We thank Jean-Luc Anton, Muriel Roth and Rym Djouri from Marseille fMRI centre (irmfmrs.free.fr) for their support. We thank reviewers for useful comments on a previous version of the manuscript. This study was supported by a Cognisud grant.
References
Amodio, D. M., and Frith, C. D. (2006). Meeting of minds: the medial frontal cortex and social cognition. Nat. Rev. Neurosci. 7, 268–277.
Badre, D. (2008). Cognitive control, hierarchy, and the rostro-caudal organization of the frontal lobes. Trends Cogn. Sci. 12, 193–200.
Billard, A., Robins, B., Nadel, J., and Dautenhahn, K. (2007). Building Robota, a mini-humanoid robot for the rehabilitation of children with autism. Assist. Technol. 19, 37–49.
Cabeza, R., Dolcos, F., Graham, R., and Nyberg, L. (2002). Similarities and differences in the neural correlates of episodic memory retrieval and working memory. Neuroimage 16, 317–330.
Cavanna, A. E., and Trimble, M. R. (2006). The precuneus: a review of its functional anatomy and behavioural correlates. Brain 129, 564–583.
Chaminade, T., and Cheng, G. (2009). Social cognitive neuroscience and humanoid robotics. J. Physiol. Paris 103, 286–295.
Chaminade, T., and Decety, J. (2001). A common framework for perception and action: neuroimaging evidence. Behav. Brain Sci. 24, 879–882.
Chaminade, T., and Kawato, M. (2011). “Mutual benefits of using humanoid robots in social neuroscience,” in Handbook of Social Neuroscience, eds J. Decety and J. T. Cacioppo (Oxford: Oxford University Press USA), 974–991.
Chaminade, T., Zecca, M., Blakemore, S. J., Takanishi, A., Frith, C. D., Micera, S., Dario, P., Rizzolatti, G., Gallese, V., and Umilta, M. A. (2010). Brain response to a humanoid robot in areas implicated in the perception of human emotional gestures. PLoS One 5:e11577. doi: 10.1371/journal.pone.0011577
Cross, E. S., Liepelt, R., de, C. H. A. F., Parkinson, J., Ramsey, R., Stadler, W., and Prinz, W. (2011). Robotic movement preferentially engages the action observation network. Hum. Brain Mapp. doi: 10.1002/hbm.21361. [Epub ahead of print].
Dautenhahn, K. (2007). Socially intelligent robots: dimensions of human-robot interaction. Philos. Trans. R. Soc. Lond. B Biol. Sci. 362, 679–704.
du Boisgueheneuc, F., Levy, R., Volle, E., Seassau, M., Duffau, H., Kinkingnehun, S., Samson, Y., Zhang, S., and Dubois, B. (2006). Functions of the left superior frontal gyrus in humans: a lesion study. Brain 129, 3315–3328.
Duvernoy, H. M. (1999). The Human Brain: Surface, Blood Supply, and Three-Dimensional Anatomy, 2nd edn, completely revised. Wien New York, NY: Springer-Verlag.
Eickhoff, S. B., Paus, T., Caspers, S., Grosbras, M. H., Evans, A. C., Zilles, K., and Amunts, K. (2007). Assignment of functional activations to probabilistic cytoarchitectonic areas revisited. Neuroimage 36, 511–521.
Gallagher, H., Jack, A., Roepstorff, A., and Frith, C. (2002). Imaging the intentional stance in a competitive game. Neuroimage 16, 814.
Hamilton, A. F., and Grafton, S. T. (2006). Goal representation in human anterior intraparietal sulcus. J. Neurosci. 26, 1133–1137.
Hutton, C., Bork, A., Josephs, O., Deichmann, R., Ashburner, J., and Turner, R. (2002). Image distortion correction in fMRI: a quantitative evaluation. Neuroimage 16, 217–240.
Koechlin, E., Ody, C., and Kouneiher, F. (2003). The architecture of cognitive control in the human prefrontal cortex. Science 302, 1181–1185.
Krach, S., Hegel, F., Wrede, B., Sagerer, G., Binkofski, F., and Kircher, T. (2008). Can machines think? Interaction and perspective taking with robots investigated via fMRI. PLoS One 3:e2597. doi: 10.1371/journal.pone.0002597
Krach, S., Paulus, F. M., Bodden, M., and Kircher, T. (2010). The rewarding nature of social interactions. Front. Behav. Neurosci. 4:22. doi: 10.3389/fnbeh.2010.00022
Mataric, M., Tapus, A., Winstein, C., and Eriksson, J. (2009). Socially assistive robotics for stroke and mild TBI rehabilitation. Stud. Health Technol. Inform. 145, 249–262.
McCabe, K., Houser, D., Ryan, L., Smith, V., and Trouard, T. (2001). A functional imaging study of cooperation in two-person reciprocal exchange. Proc. Natl. Acad. Sci. U.S.A. 98, 11832–11835.
Nagahama, Y., Okada, T., Katsumi, Y., Hayashi, T., Yamauchi, H., Sawamoto, N., Toma, K., Nakamura, K., Hanakawa, T., Konishi, J., Fukuyama, H., and Shibasaki, H. (1999). Transient neural activity in the medial superior frontal gyrus and precuneus time locked with attention shift between object features. Neuroimage 10, 193–199.
Oldfield, R. C. (1971). The assessment and analysis of handedness: the Edinburgh inventory. Neuropsychologia 9, 97–113.
Petrides, M. (2005). Lateral prefrontal cortex: architectonic and functional organization. Philos. Trans. R. Soc. Lond. B Biol. Sci. 360, 781–795.
Rilling, J. K., Sanfey, A. G., Aronson, J. A., Nystrom, L. E., and Cohen, J. D. (2004). The neural correlates of theory of mind within interpersonal interactions. Neuroimage 22, 1694–1703.
Rizzolatti, G., and Craighero, L. (2004). The mirror-neuron system. Annu. Rev. Neurosci. 27, 169–192.
Saxe, R., and Kanwisher, N. (2003). People thinking about thinking people. The role of the temporo-parietal junction in “theory of mind”. Neuroimage 19, 1835–1842.
Saygin, A. P., Chaminade, T., Ishiguro, H., Driver, J., and Frith, C. (2011). The thing that should not be: predictive coding and the uncanny valley in perceiving human and humanoid robot actions. Soc. Cogn. Affect. Neurosci. doi: 10.1093/scan/nsr025. [Epub ahead of print].
Sereno, M. I., Pitzalis, S., and Martinez, A. (2001). Mapping of contralateral space in retinotopic coordinates by a parietal cortical area in humans. Science 294, 1350–1354.
Simon, O., Mangin, J. F., Cohen, L., Le Bihan, D., and Dehaene, S. (2002). Topographical layout of hand, eye, calculation, and language-related areas in the human parietal lobe. Neuron 33, 475–487.
Keywords: social cognition, neuroscience, artificial intelligence, fMRI
Citation: Chaminade T, Rosset D, Da Fonseca D, Nazarian B, Lutcher E, Cheng G and Deruelle C (2012) How do we think machines think? An fMRI study of alleged competition with an artificial intelligence. Front. Hum. Neurosci. 6:103. doi: 10.3389/fnhum.2012.00103
Received: 31 December 2011; Accepted: 08 April 2012;
Published online: 08 May 2012.
Edited by:
Leonhard Schilbach, Max-Planck-Institute for Neurological Research, GermanyReviewed by:
Nicole David, University Medical Center Hamburg-Eppendorf, GermanyBojana Kuzmanovic, Research Center Juelich, Germany
Copyright: © 2012 Chaminade, Rosset, Da Fonseca, Nazarian, Lutcher, Cheng and Deruelle. This is an open-access article distributed under the terms of the Creative Commons Attribution Non Commercial License, which permits non-commercial use, distribution, and reproduction in other forums, provided the original authors and source are credited.
*Correspondence: Thierry Chaminade, Institut de Neurosciences de la Timone, Centre National de la Recherche Scientifique - Aix-Marseille University, 31 Chemin Jospeh Aiguier, CEDEX 20 Marseille, France. e-mail: tchamina@gmail.com