- 1School of Psychology, Behavioural Brain Sciences Centre, University of Birmingham, Birmingham, UK
- 2Department of Neurology, Radboud University Medical Centre, Radboud University, Nijmegen, Netherlands
- 3Donders Institute for Brain, Cognition and Behaviour, Radboud University, Nijmegen, Netherlands
There is recent EEG evidence describing task-related changes of theta power in spatial attention and reaching/pointing tasks. Here, we aim to better characterize this theta activity and determine whether it is associated with visuospatial memory or with visuospatial selection functions of the frontoparietal cortex. We recorded EEG from 20 participants during a movement precuing task with center-out joystick movements. Precues displayed 1, 2, or 4 potential targets and were followed (stimulus onset asynchrony 1.2 s) by a central response cue indicating the movement-target. Remembering the precued target location(s) was mandatory in one and optional in a second version of the task. Analyses evaluated two slow brain potentials (CNV, contingent negative variation and CDA, contralateral delay activity) and task-related power changes. Results showed a differential modulation of frontal CNV and parietal CDA, consistent with earlier described set-size effects on motor preparation and visual short-term memory. Short-lived phases of theta event-related synchronization (ERS) were found 150–500 ms after precue and response cue presentation, exhibiting parietal and frontal maxima. The increase of frontoparietal theta power following response cue presentation was strongly modulated by target load, i.e., absent for 1-target (when the movement-target could be selected in advance), contrasting with a robust 20–50% ERS response in 2- and 4-target conditions. The scalp distribution, the timing, and the modulation by set-size suggest a role of theta activity in movement-target selection. The results support a recently proposed view of theta as emerging around behavioral decision points, linked to the evaluation of choice-relevant information.
Introduction
Theta oscillations are commonly associated with memory function and spatial representation, not only in the hippocampus and in the interaction of hippocampus and prefrontal cortex (for review see Düzel et al., 2010), but also in frontoparietal circuitry serving visuospatial memory (Sauseng et al., 2008). In concert with fast gamma oscillations, theta oscillations may encode multiple items in a defined order or spatial arrangement (Lisman and Buzsáki, 2008), and set up or control the information flow between distant regions (Jensen, 2005; Sauseng et al., 2008). Beyond their relevance to memory, these properties of theta oscillations are also well-suited to the visuospatial and visuomotor functions of the frontoparietal cortex. Accordingly, there is emerging evidence for task-related theta synchronization associated with reaching and pointing movements (Praamstra et al., 2009; Tombini et al., 2009; Perfetti et al., 2011; Cruikshank et al., 2012).
Praamstra et al. (2009) used a movement precueing task to investigate how the number and spatial proximity of potential movement-targets affect movement preparatory EEG activity in the delay-period and during the selection of a response. During response selection, in a time window following the response cue, they found variations in theta power over the parietal scalp region. Delay-period activity in frontal and parietal cortex is often framed in terms of the frontal cortex carrying a prospective representation of future actions and the parietal cortex maintaining a retrospective representation of sensory information (Curtis and D'Esposito, 2006; Lindner et al., 2010). Theta activity elicited following the response cue that terminates the delay-period may be considered from the same perspective. That is, this activity could serve a memory function and reflect the integration of current perceptual information with a memory representation of visuospatial cue information. In line with such a view, parietal theta modulation has been considered a frequency domain correlate of the memory updating process assumed to underlie the P300 (Klimesch et al., 1994; Makeig et al., 2004). Alternatively or additionally, theta activity elicited by a response cue might reflect spatial aspects of action planning, as supported by a hypothesized role of theta activity in coordinating sensory and motor brain activity (Bland and Oddie, 2001; Caplan et al., 2003).
Building on this background, the present study explored which of these two mechanisms takes precedence in the modulation of frontoparietal theta activity during response selection, that is, whether theta power synchronization is associated with visuospatial memory or with the visuospatial selection of a target for a motor response. We used two task versions of a delayed-response pointing task in which precues, i.e., a brief display of potential movement-targets (1, 2, or 4 potential targets) primed spatial representation and movement preparatory processes. Remembering the precued target location(s) was mandatory in one (Memory search version) and optional in a second version (Visual search version) of the task. We hypothesized that if theta synchronization following the cue stimulus is related to visual spatial memory function, it should be of higher power and exhibit stronger load effects in the Memory search than in the Visual search task. If, by contrast, no such task differences are obtained, we infer that the theta synchronization, and load effects upon the theta synchronization, are related to movement-target selection. The results revealed theta synchronization effects over posterior parietal and premotor regions; scalp distribution, timing, and modulation by task-version and set-size support a role of theta activity in movement-target selection.
Materials and Methods
Participants
Twenty right-handed (by self-report) participants took part in the experiment (eight males; mean age 29 years ± 8 years). All had normal or corrected-to-normal vision. Data from two additional participants were excluded due to an excessive number of errors. The participants were paid volunteers from the post-graduate community at the University of Birmingham. The study had been approved by the South Birmingham Research Ethics Committee. Participants gave written informed consent following a full explanation of the study.
Task and Hypotheses
The experiment consisted of two precued choice-reaction tasks using center-out joystick movements. In both tasks, participants were presented with a precue indicating 1, 2, or 4 movement-targets within a circular array of 12 placeholders. Following a short delay-period, a cue (response stimulus) was presented that signaled which of the movement-targets had to be captured with a pointing movement of the joystick. The tasks differed in the following way. In one task, the response stimulus also re-displayed the movement-targets displayed earlier in the precue stimulus, whereas in the second task this was not the case. Hence, in the latter task, it was mandatory to memorize the locations of precued movement-targets during the delay-period. Upon presentation of the response stimulus, participants searched the visual display in the first task, whereas in the second task they had to search their visual spatial memory to identify the target corresponding in color to the response cue, before they could generate a joystick movement. The two task versions are referred to as the “visual search” (Vis search) and “memory search” (Mem search) tasks, respectively. If theta synchronization following the cue stimulus serves a visual spatial memory function, it should be of higher power and exhibit stronger load effects in the Mem search than in the Vis search task. In the absence of such task differences, theta synchronization and load effects on theta synchronization are more likely related to movement-target selection.
Note that the use of contrasting visual search and memory search tasks resembles recent experimental approaches of investigating the nature of visual short-term memory representation with the N2pc ERP component (Kuo et al., 2009; Dell'Acqua et al., 2010). This work does not predict the outcome of the present study, as it used target present/absent button press responses instead of directional pointing movements to capture a perceived or remembered target.
Stimuli and Procedure
Figure 1 shows a timeline and sample stimuli for the two tasks. The precue stimuli were identical for the two tasks, consisting of color coded target locations within a permanently displayed background consisting of 12 circular placeholders (Ø 0.5° visual angle) equidistantly positioned on a virtual circle (Ø 4.5° visual angle) around a central fixation circle. Placeholders and fixation circle were drawn in gray (RGB: 120, 120, 120) onto a darker homogenous gray screen (RGB: 177, 177, 177). Possible target colors were blue (RGB: 28, 132, 199), red (RGB: 240, 69, 60), yellow (RGB: 244, 236, 29), or green (RGB: 15, 148, 71). In both tasks, the precue stimulus was followed by a response stimulus after 1200 ms (SOA—stimulus onset asynchrony), indicating the movement-target by means of the color of the fixation signal. Only in the Vis search task, the response stimulus also displayed the movement-targets earlier shown in the precue stimulus.
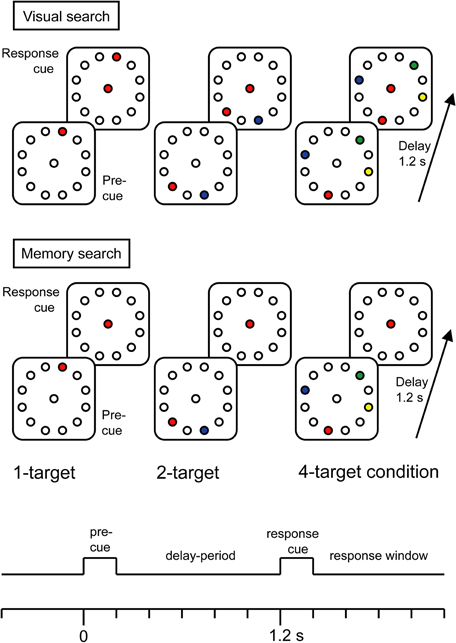
Figure 1. Trial timeline and sample precue and response stimuli of Visual search (top) and Memory search task versions (bottom). The color of the central color cue provides the information where to move with a joystick cursor. The color of the central color cue varied from trial to trial.
Movement-targets were positioned at random locations within the array of placeholders, with the following constraints. In the 1-target condition, there was an equal number of trials with targets left and targets right from fixation. In the 2- and the 4-target conditions, there were always equal numbers of targets on both sides of fixation in order to avoid stimulus- and attention-related EEG asymmetries. The two alternative targets in the 2-target condition were never located at adjacent placeholders directly left and right to the midline. Likewise, in the 4-target condition there was always a minimum of 1 unfilled placeholder between two targets within a hemifield. These constraints helped to separate target selection errors from movement inaccuracies. The precue and response stimuli were each presented for 200 ms on a 17 inch CRT monitor placed 100 cm from the participants' eyes. Responses were made with a joystick mounted on a table in front of the participant. Joystick movements moved a cursor on the screen, leaving a black trace (RGB: 5, 6, 7) that provided visual feedback. The distance travelled by the cursor was ∼4 cm, corresponding to 2.25° of visual angle separating fixation circle and targets. Participants were instructed to respond as soon as possible and to make ballistic movements that were allowed to overshoot the target. They were instructed not to follow the trajectory of the cursor with their eyes, but to maintain fixation on the center of the screen. The intertrial interval varied between 1750 and 2250 ms. In order to keep the task versions as similar as possible, we did not implement feed-back information in the memory search task version.
The experiment consisted of one practice block and six consecutive experimental blocks for each of the two tasks. The order of the two tasks was counterbalanced. Practice blocks had 36 trials and experimental blocks 72 trials, divided equally between 1-, 2-, and 4-target conditions. This yielded 144 trials per condition in each of the two tasks. The duration of an experimental block was ∼6 min. The experiment was run in a quiet normally illuminated room.
Data Acquisition
Joystick
Manual pointing responses were made with a Hall effect joystick (SureGrip Controls Inc.; JM Series), with the handle (11 cm, Ø 26 mm) held in hand grip. Joystick data were AD converted with a 16-bit resolution USB-1608FS DAQ device (Measurement Computing Corporation, Norton, MA) at 1 kHz. The data were continuously streamed to a PC and processed using the Matlab data acquisition toolbox (The MathWorks, Natick, MA) and a custom Matlab application. Response data were acquired from just before the response signal and continuously thereafter for 1200 ms.
EEG
EEG was recorded continuously with BioSemi ActiveTwo DC amplifiers from 132 Ag/AgCl scalp electrodes relative to the common mode sense (CMS) and driven right leg (DRL) electrodes, which were placed adjacent to the Cz (vertex) electrode location. Electrodes were placed according to the 10–5 extension of the international 10–20 electrode system (Oostenveld and Praamstra, 2001), using a carefully positioned elastic nylon cap. Electrooculography (EOG) electrodes were positioned lateral to the left and right eyes in order to monitor horizontal eye-movements. EEG and EOG signals were sampled at 512 Hz (anti-alias filter −3 dB at 102 Hz).
Data Processing and Analysis
Joystick trajectory data
Reaction times were calculated on the basis of movement onset, which was defined as the time when the screen cursor movement in any direction exceeded 4 mm from the screen center, which corresponded to the joystick's neutral position. Error analyses were based on an off-line trial-by-trial inspection of the movement trajectories. Trials without response and trials with reaction times <100 ms or >1000 ms were counted as error. Trajectories were allowed to overshoot the target and allowed to miss the target by at most half the distance separating two adjacent placeholders. Thus, trials where the movement trajectory deviated from the target to a degree that it came closer to one of the adjacent placeholders were counted as error. These criteria led to exclusion of two participants with >40% errors.
EEG slow brain potentials
Complementary to time-frequency analyses of the EEG data, we extracted slow brain potentials developing in the delay-period between precue and response cue, i.e., the contingent negative variation (CNV). Amplitude differences of the CNV provided information on the degree of (movement) preparation during the delay-period and thus helped assess whether subjects used precue information to perform the tasks. Analyses of the CNV were also aimed to replicate similar analyses in Praamstra et al. (2009). To extract slow brain potentials, the continuous EEG data were re-referenced to an average reference and subsequently subjected to an eye-blink and eye-movement correction based on a source modeling approach (Berg and Scherg, 1994) implemented in BESA (Brain Electrical Source Analysis 5.1.8; MEGIS software GmbH). Prior to the eye-movement correction, we established that there was no difference between VIS and MEM search tasks in the tendency to make eye-movements following the screen cursor. The continuous data were subsequently segmented into epochs from 500 ms before to 2500 ms after the precue stimulus for those trials with a correct behavioral response. Based on the segmented and artifact-corrected individual trials, averaged data were created for each participant and condition separately. The scalp distributions of slow brain potentials were inspected and evaluated on the basis of voltage distributions and on the basis of current source density (CSD) distributions. CSD analyses compute the second spatial derivative of the scalp voltage distribution and attenuate the contribution of distant sources to the measurements at the scalp, yielding scalp topographies that are more representative of local current flow. CSD analyses were performed after spherical spline interpolation of the scalp voltage distribution using BrainVision Analyzer (BrainProducts GmbH, Munich, Germany). We adopted standard parameter values for the interpolation (order of splines 4; maximum degree of Legendre polynomials 10). Based on evaluation of voltage and CSD scalp distributions, regions of interest (ROIs) were defined over posterior parietal and premotor cortex, consisting of electrodes P1, P3, PPO3h, CPP3h, and electrodes FC1, FCz, FFC1h, FCC1h, respectively.
EEG time-frequency analysis
Time-frequency analyses were performed using BESA and the Matlab toolbox FieldTrip (http://www.ru.nl/fcdonders/fieldtrip). Following segmentation and artifact correction, each trial was transformed in the time-frequency domain using complex demodulation set to a frequency resolution of 1 Hz and temporal resolution of 50 ms in the frequency range 3–50 Hz. Time-frequency representations per channel, condition and subject were created by averaging spectral density amplitude over trials, after subtracting the averaged evoked-response. This subtraction ensured that time-frequency differences between conditions were not due to differences in evoked activity related to the different number of movement-targets. Inspection of the individual subject and grand average time-frequency representations enabled the identification of a time-frequency window defining the theta activity. Event-related effects on theta power relative to the pre-stimulus baseline theta power were evaluated with cluster-level randomization tests in FieldTrip, developed to handle the multiple comparison problem inherent in the statistical evaluation of high-density EEG and MEG data (Maris and Oostenveld, 2007). The cluster-level randomization method first identifies electrodes where the difference between two conditions (here event-related theta power vs. baseline theta power) exceeds a chosen significance level. Adjacent electrodes where the difference between conditions is significant are combined into clusters for each of which the cluster-level t-value is the sum of all t-values within the cluster. Then a null-distribution is created by randomly assigning paired observations to one of the two conditions for a user-specified number of times, and computing the cluster-level statistics for each randomization. Finally, the observed cluster-level test statistic is compared against the null-distribution. When the observed statistic falls in one of the 2.5th percentiles of the null-distribution, the effect is considered significant. We used default parameters for the definition of a cluster (electrodes within 4 cm distance and a minimum of two neighbor channels). The number of random draws for reference distributions was set at 500. This analysis required the baseline interval to be equal in duration to the test interval. The baseline interval hence started at a duration before precue onset that depended on the analysis.
Statistical analyses of EEG potentials and response times were performed using GLM (General Linear Model) repeated measures analyses in SPSS and post-hoct-tests with Bonferroni correction for multiple comparisons where appropriate. The Greenhouse-Geisser procedure was applied to all repeated measures with more than one degree of freedom. The adjusted p-values are reported, in combination with the original degrees of freedom and the Greenhouse–Geisser correction factor ε. As effect size measure, partial eta squared was used (η2p). No additional filtering of EEG data was performed prior to statistical analyses. By contrast, for illustration purposes the waveforms in illustrations were smoothed by a low-pass filter (12 Hz, 12 dB).
Results
Behavioral Performance
Response times and percentage of errors were analyzed using GLM repeated measures analyses with within-subject factors Task (Visual search, Memory search) and Target load (1,2,4). The results are summarized in Figure 2, showing a straightforward and expected pattern. Analyses of mean response times revealed a significant main effect of Task [F(1, 19) = 15.62, p = 0.001, η2p = 0.451], due to faster reaction times in the Vis search than in the Mem search task. There was also a significant main effect of Target load, due to an increase of reaction time with the number of targets [F(2, 38) = 89.27, ε = 0.576, p < 0.001, η2p = 0.826]. A significant interaction between Task and Target load [F(2, 38) = 8.29, ε = 0.678, p < 0.005, η2p = 0.304] was due to a much smaller (non-significant) difference between the 2-target load conditions than between the 1- and 4-target load conditions.
The effect of Target load was further analyzed for each task version separately. In the Vis search task, RTs scaled with target load: 1-target 384 ± 83 ms, 2-target 479 ± 55 ms, and 4-target 534 ± 57 ms. Bonferroni corrected pairwise t-tests between the 1- and 2- and between the 2- and 4-target conditions confirmed both pairwise differences as significant (p < 0.001). In the Mem search task, RTs were: 1-target 454 ± 96 ms, 2-target 500 ± 56 ms, and 4-target 604 ± 49 ms. Here, the pairwise differences between 1- and 2- and between 2- and 4-target conditions were also significant (p < 0.02 and p < 0.001, respectively).
Error analyses revealed that more errors occurred in the Mem search task (10%) than in the Vis search task (2%), yielding a significant main effect of Task [F(1, 19) = 40.5, p < 0.001, η2p = 0.680]. In addition, there was a significant main effect of Target load [F(2, 38) = 22.1, ε = 0.605, p < 0.001, η2p = 0.538]. A significant interaction between Task and Target load [F(2, 38) = 35.7, ε = 0.563, p < 0.001, η2p = 0.652] was due to the fact that an increase of errors from the 1- to the 4-target condition was solely accounted for by the Memory search task. Pairwise comparisons between the 1- and 2- and between the 2- and 4-target conditions were carried out separately for the two task versions. In the Visual search task, the error percentages were 4 ± 4%, 2 ± 2%, and 3 ± 3%, for the 1, 2, and 4 target conditions, respectively. There were no significant differences between pairs. The numerically highest number of errors in the 1-target condition was due to early responses <100 ms. In the Memory search task version, the error percentages were 6 ± 6%, 11 ± 6%, and 25 ± 16%, with significant differences both between the 1- and 2-, and between the 2- and 4-target conditions (ps < 0.001).
These analyses confirm anticipated differences between the task versions. Searching for a target in visual working memory is markedly slower than visual search and subject to capacity limitations of visual working memory, as demonstrated by a steep increase of errors from the 2- to the 4-target condition.
EEG Analyses
Delay-period slow brain potentials
An inspection of the slow brain potential topography during the delay-period revealed separate scalp maxima over the left frontal and left parietal scalp areas. As shown in Figure 3, the frontal and parietal maxima are more distinctive in CSD topographies than in scalp voltage representation. Based on visual assessment of the scalp distribution in both tasks separately, frontal and parietal ROIs were defined, each comprising the same four electrodes in both tasks. CSD values for these ROIs for a time window of 600–900 ms were entered in a repeated measures GLM analysis with factors Task (2), Area (2), and Load (3). The time window was defined to coincide with the most conspicuous modulation of the parietal slow brain potentials. The analysis showed no main effect of Task [F(1, 19) < 1], no interaction of Task by Target load [F(2, 38) < 1, ε = 0.945, η2p = 0.043], and no interaction of Task by Area [F(1, 19) = 2.1, p = 0.16, η2p = 0.101]. Hence, Figure 3 shows results averaged across both tasks. There was a significant effect of Area [F(1, 19) = 13.1, p = 0.002, η2p = 0.408] and a significant interaction of Area by Load [F(2, 38) = 16.7, ε = 0.636, p < 0.001, η2p = 0.468]. As is clear from Figure 3, the effect of Area is due to higher amplitudes for the frontal than for the parietal ROI. The interaction is due to Load effects of opposite direction in the frontal and parietal ROIs. For clarity of exposition, we further report separate analyses for the frontal and parietal ROIs. We note that similar analysis results were obtained with voltage instead of CSD values entered into the analysis.
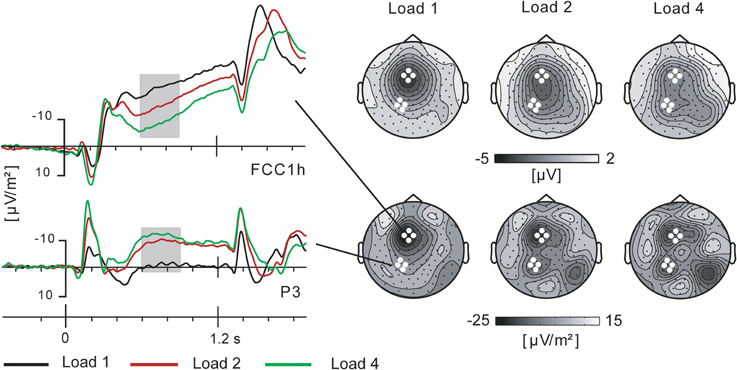
Figure 3. Slow brain potentials over (pre) motor cortex and parietal cortex during the cue-target interval. Scalp distributions shown as voltage maps (top) and current source density (CSD) maps (bottom). Grand average data across tasks.
Based on previous work, it was expected that delay-period preparatory activity in (pre)motor cortex, i.e., the frontal ROI, would be influenced by the number of potential movement-targets indicated by the cue. This was robustly confirmed by an amplitude modulation of the CNV at electrode locations overlying (pre)motor cortex (see Figure 3). The amplitude was highest in the 1-target condition and lowest in the 4-target condition. Statistical evaluation yielded a significant main effect of Target load [F(2, 38) = 13.7, ε = 0726, p < 0.001, η2p = 0.419]. Bonferroni-corrected pair-wise comparisons between the 1- and 2-target condition and between the 2- and 4-target condition showed each pair to be significantly different (p < 0.029 and p < 0.008). The robust effect of Target load was not different between tasks [interaction Task by Target load F(2, 38) < 1, ε = 0.976, η2p = 0.026]. Nor was there a main effect of Task [F(1, 19) = 1.6, p = 0.22, η2p = 0.077].
Based on previous work on visual working memory, it was no surprise that at parietal electrode sites the load effect on slow brain potentials was inversed relative to the frontal ROI. As shown in Figure 3, here the amplitude was lowest for the 1-target condition, and considerably higher for the 2- and 4-target condition, giving a main effect of Target load [F(2, 38) = 13.0, ε = 0.650, p < 0.001, η2p = 0.406]. Bonferroni-corrected pair-wise comparisons showed only the 1 vs. 2-target and 1 vs. 4-target comparisons to be significant (p < 0.001 and p < 0.008). The effect of Target load was not different between tasks [interaction Task by Target load F(2, 38) < 1, ε = 0.791, η2p = 0.024]. Nor was there a main effect of Task [F(1, 19) = 1.5, p = 0.24, η2p = 0.072].
Given the distinctly left-lateralized parietal activity, these analyses were performed for a left parietal ROI. Figure 3 does show an additional right posterior focus of activity, but this is located over occipito-temporal cortex rather than parietal. Noteworthy, a median-split separating the participants on the basis of their performance in the 4-target condition of the Mem search task (with the highest memory load), suggested that the best performing subjects did produce right parietal activity, yielding more symmetrical parietal activity altogether. Supplementary analyses including a homologous right parietal ROI did not, however, yield significant effects attributable to differences in memory capacity.
Together, these analyses confirm (pre)motor cortex activity during the delay-period to be influenced by the number of potential movement-targets, in both tasks. Hence, the precue information was utilized not only when it was mandatory (Mem search), but also when it was not strictly required (Vis search). The target load effects on left parietal slow potentials parallel the behavior of the contralateral delay activity (CDA), a recognized marker of visual working memory (Vogel and Machizawa, 2004), as we will further explain in the Discussion. Here as well, the identical amplitude modulation across task versions indicates that possible target locations were encoded and maintained during the delay-period, whether or not this was needed to perform the task.
Time-frequency analyses—theta activity
Analyses of task-related modulations in oscillatory power were performed in a frequency range of 3–50 Hz. Our main interest was in the modulation of frontoparietal theta activity (4–7 Hz) following the presentation of the response signal. That is, when the participant starts a movement that was already planned (1-target condition), or selects one target/movement direction from several previously cued alternatives (2- and 4-target condition) and then starts movement. However, there was a very similar modulation of theta activity following the precue stimulus. For the sake of completeness, we analyze both the first phase of theta synchronization following the precue (200–400 ms) and the synchronization following the response stimulus (1450–1700 ms). A first inspection of the data revealed that the two theta synchronization phases have similar distributions over left parietal and frontal scalp areas, and that the parietal activity peaks earlier than the frontal activity (see Figures 4 and 5).
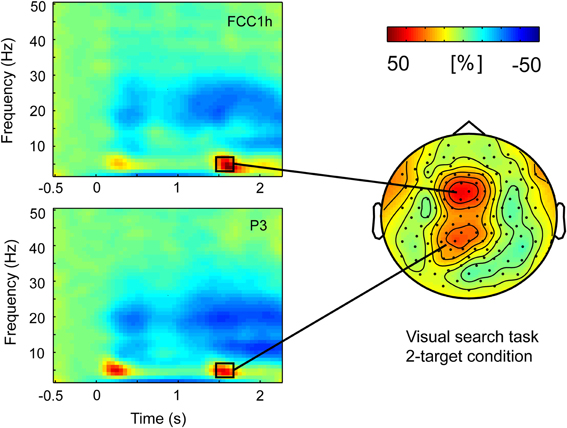
Figure 4. Task-related theta-power during pointing target selection (displayed in time-frequency spectra on the left) was distributed over frontal and parietal scalp regions. Grand average data from Visual search task version in the 2-target condition.
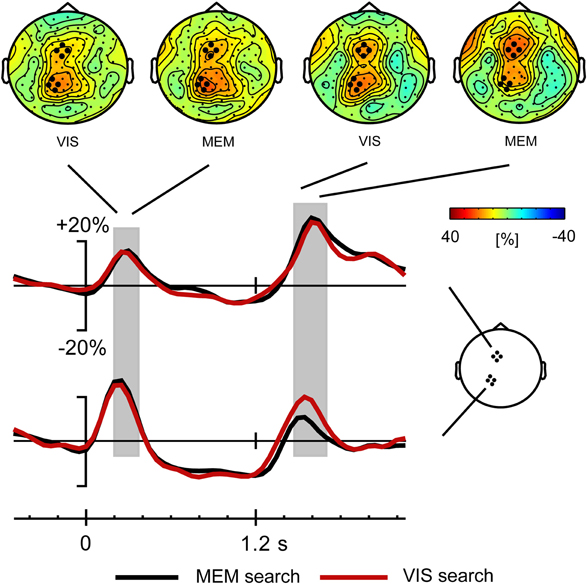
Figure 5. Time course of theta activity for Visual search and Memory search task versions, with scalp distributions of the theta synchronization phases following precue and response cue; grand average data across all subjects. The theta synchronization following the response cue is due to the 2- and 4-target conditions only (see Figure 6). The gray blocks indicate the time windows for analysis of the theta synchronization.
Visual inspection was followed by cluster randomization analyses comparing theta power in the windows of 200–400 ms (following the cue) and 1450–1700 ms (following the response signal) against pre-stimulus baseline theta power. In the early time window, the theta synchronization was significantly different from pre-stimulus theta power in a large frontal-central-parietal cluster of electrodes. The size of the cluster varied across conditions in both tasks, ranging between 39 and 114 electrodes. In the late time window following the response signal, the theta synchronization was also found to be significantly different from baseline in a large cluster of electrodes (size 47–78 electrodes) in the 2- and 4-target conditions of both tasks. In the 1-target condition, by contrast, theta activity was not significantly raised from baseline in the Vis search version of the task, while only a small (18 electrodes) frontal cluster was present in the Mem search task version. Importantly, within the large clusters of parietofrontal theta activity, separate frontal and parietal maxima could be discerned (see Figure 4).
In order to better compare theta power across tasks and conditions, we defined a frontal and a parietal ROI. Since the maxima of task-related theta power nearly coincided with the parietal and frontal maxima of slow brain potentials, we selected the same ROIs as used for slow brain potentials, each encompassing four electrodes. Power at these frontal and parietal ROIs was entered in a repeated measures GLM analysis with within-subjects factors Task (2), Area (2) and Load (3). We first describe the analysis results of the early theta synchronization phase. As is clear from Figure 5, showing the time course of theta activity separately for both task versions (collapsed across loads), there was no difference in theta power between tasks. By contrast, a main effect of Area [F(1, 19) = 4.66, p < 0.044, η2p = 0.197] was due to higher theta power over the parietal than over the frontal cortex, which is also evident in Figure 5. The time course of task-related theta modulation is shown separately for the different load conditions in Figure 6. Load had a significant effect on the first theta synchronization phase [F(2, 38) = 16.59, ε = 0.877, p < 0.001, η2p = 0.466], more so parietally than in the frontal ROI, as expressed in a significant Load x Area interaction [F(2, 38) = 3.97, ε = 0.806, p < 0.037, η2p = 0.173].
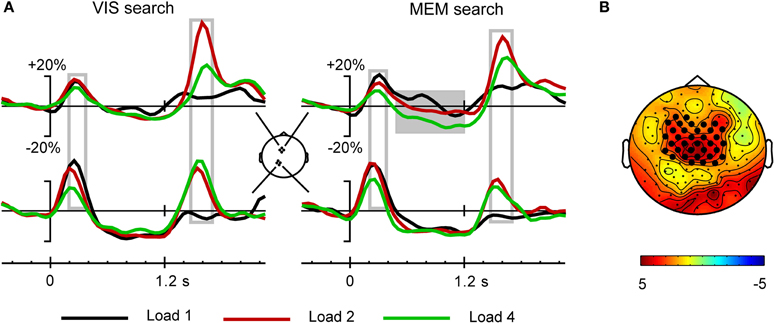
Figure 6. (A) Grand average time course of theta activity for the different target load conditions. Note the absence of significant theta following the response signal for the 1-target condition. Theta power differentiates 2- and 4-target conditions over the premotor cortex, but not the parietal cortex. (B) In the Memory search task, sustained theta activity in the interval 0.5–1.2 s (gray block in A) differentiates the 4-target condition from the 1- and 2-target conditions. The scalp map shows the significant electrode cluster that differentiates 4- and 1-target conditions, comprising 35 frontocentral electrodes.
The same analysis applied to the theta synchronization in the later time window after the response stimulus, yielded a significant main effect of Area [F(1, 19) = 7.4, p < 0.014, η2p = 0.279]. As illustrated in Figure 5, this was related to higher theta power in the frontal compared to the parietal ROI, i.e., the opposite of what was found in the early time window. In addition, a Task by Area interaction [F(1, 19) = 14.6, p < 0.001, η2p = 0.434] was due to higher parietal theta power in the Vis compared to the Mem search task version (see Figure 5). Target load also had a significant effect on theta power [F(2, 38) = 45.3, ε = 0.701, p < 0.001, η2p = 0.704], which was modulated by an Area by Load interaction [F(2, 38) = 11.5, ε = 0.833, p < 0.001, η2p = 0.378], evident in Figure 6. This was due to a divergence in theta power for the 1-, 2-, and 4-target conditions at the frontal ROI, while power was identical for the 2- and 4-target conditions at the parietal ROI.
Together, these analyses show that the brief theta synchronization phases following precue and response cue were influenced very little by the different mnemonic demands of the two task versions. In view of the pronounced difference in performance in the two task versions, contrasting with the minor difference in theta synchronization phases, we also performed an analysis of the sustained theta activity during the delay-period, in the time window from 0.5 to 1.2 s. While Figure 5 reveals no difference between tasks for the delay-period, Figure 6 suggests a load effect at the frontal ROI for the Memory search task version. Cluster randomization analysis confirmed a difference between the Load 4 and Load 1 conditions, consisting of a frontocentral cluster of 35 electrodes. Similarly, a comparison of the Load 4 with the Load 2 conditions yielded a smaller cluster of 23 electrodes. The load-dependent depression of theta during the memory delay, in a frontal distribution, agrees with findings of Bastiaansen et al. (2002), who proposed that delay-period frontal desynchronization of theta activity is related to visuospatial working memory function. The delay-period theta effects thus corroborate that performance in the memory search task draws upon visual spatial working memory in a way that is not the case for the visual search task version. Since our main interest is in the theta activity following the response cue, this delay-period effect will not be further addressed.
Discussion
The results of this study show novel effects on theta oscillatory power that broadly support a role of theta activity in the spatial selection of movement-targets. Event-related changes in theta power are most prominently expressed in brief theta synchronization phases following precue and response cue, and are discretely localized over left frontal and parietal cortex. Theta power at these frontal and parietal loci is differentially modulated during the encoding of possible movement-targets and during the retrieval/movement-target selection phase. Crucially, the difference in mnemonic demands between the tasks had a very limited effect on the theta synchronization phases, and effects of target load on the theta synchronization phases are difficult to account for in terms of visual working memory load. Interestingly, the phasic changes in theta power following precue and response cue are identical in scalp distribution with visual working memory-related slow brain potentials and with movement-preparatory slow brain potentials bridging the intervening delay-period. We discuss the event-related effects on theta power against the background of the modulation of slow brain potentials.
Parietal and Frontal Slow Brain Potentials
The CNV developing in the delay-period showed separate frontal and parietal scalp maxima (see Figure 3). The former reflects (pre)motor cortex activity associated with movement preparation, the latter parietal activity associated with visual working memory. Working memory-related slow brain potentials are usually derived in terms of a subtraction between signals at occipitotemporal electrodes ipsi- and contralateral to the visual hemifield containing items that have to be memorized (Klaver et al., 1999; Vogel and Machizawa, 2004). The resulting subtraction potential is named CDA or SPCN (sustained posterior contralateral negativity) and represents a sensitive index of working memory storage and working memory capacity (McCollough et al., 2007). The design of our study did not allow the derivation of the CDA proper, as potential movement-targets were displayed in both hemifields in the 2- and 4-target conditions. Nevertheless, the behavior, the morphology, and the antecedent conditions of the left-lateralized parietal slow brain potentials in our study are such that there is no doubt that they represent the same process as the CDA (McCollough et al., 2007; Robitaille et al., 2010). Noteworthy, parietal CDA-like slow brain potentials were not evident in related previous work where we used endogenous arrow cues (Praamstra et al., 2009). This reveals an important difference between spatial working memory processes invoked by exogenous cues, directly representing potential movement-targets, and by endogenous cues, which merely point to the location of potential movement-targets.
The CDA-like slow brain potentials did not differ between the Memory search and Visual search task versions. This suggests that multiple movement-targets, irrespective of whether their locations need to be memorized, are committed to visual spatial working memory to enable preparation of movements to capture the targets (Meyer et al., 2007). The behavior of the CDA-like potentials was characterized by a marginal increase in amplitude from the 2- to the 4-target condition. This corresponds with the behavior of the CDA, which increases in amplitude with memory set-size and then plateaus at a set-size that predicts individual working memory capacity (Vogel and Machizawa, 2004). The relatively low set-size at which the potentials plateau in the present study is probably influenced by factors such as display duration of the targets, which was short relative to memory studies. Recall that we explored whether there were differences in visual working memory-related slow brain potentials between participants with good and with poor performance in the 4-target condition. Such differences did not reach significance, however. The fact that the parietal slow potentials failed to separate good and bad performers suggests that the CDA proper, derived relative to the contralateral visual field, is more sensitive.
Whereas the parietal CDA-like slow brain potentials increased in amplitude with target load, the frontal potentials demonstrated a modulation in opposite direction, with the highest amplitude for the 1-target condition. The inverse scaling with Target load accords with the pattern of reaction times. Not reported here, we found a related scaling of beta power decreases in the present data set, similar to that described by Tzagarakis et al. (2010). According to the “affordance competition model” (Cisek, 2007) multiple potential movements are simultaneously encoded in premotor cortex through competitive interactions between the underlying neural populations. Conceivably, competitive interactions between multiple potential movement plans could result in the observed scaling of movement preparatory activity (Praamstra et al., 2009). However, it is difficult to discount an alternative explanation of such effects, known as Hick's law in the reaction time literature (Hick, 1952), in terms of response uncertainty (Lee and Keller, 2008; Tzagarakis et al., 2010).
Event-Related Theta Power—Parietal and Frontal Localization
The modulation of theta power in the form of relatively short-lasting phases of theta synchronization, following the precue and the response signal, was most pronounced at frontal and parietal scalp locations that coincided with the electrode sites where we found the most conspicuous modulation of slow brain potentials. This convergence elegantly supports the recruitment of a parietofrontal network and underscores the relevance of analyzing slow brain potentials as well as oscillatory activity (Düzel et al., 2010). Note, however, that theta power during the delay-period was very low and that the main analyses of theta activity and those of slow brain potentials concern complementary time windows. Hence, within the delineated network, slow brain potentials and theta activity are likely associated with distinct, albeit linked functions.
The presence of phasic theta synchronization effects following precue and response signal is similar to what has been observed in association with encoding and retrieval stages in studies of episodic and working memory (Klimesch, 1999; Burgess and Gruzelier, 2000; Sauseng et al., 2010). Likewise, memory studies have found higher power theta synchronization with retrieval than with encoding (Klimesch et al., 2001). However, the discrete parietal and frontal localization and the time course with parietal theta slightly preceding the frontal synchronization peak have not been described in memory tasks. Similarly, in recent reports of theta activity in motor tasks, it is predominantly distributed either parietally (Perfetti et al., 2011) or frontally (Tombini et al., 2009; Cruikshank et al., 2012). The combined frontal and parietal distribution, the timing, and the temporal order of parietal and frontal theta effects revealed in the present data, is reminiscent of theta synchronization described in cued spatial attention tasks following an endogenous arrow cue pointing to left or right hemispace (Green and McDonald, 2008; Green et al., 2011). The similarity is not surprising, as indeed spatial orienting can be regarded as a functional ingredient of the encoding of relevant spatial locations and of movement-target selection (Cisek and Kalaska, 2010). While the parietal cortex theta synchronization leads the frontal theta after the precue as well as after the response signal, the amplitude relation reverses. That is, there is a stronger parietal change in power following the precue and stronger frontal synchronization following the response signal. This may be attributed to parietal dominance in the processing of visuospatial information and premotor cortex predominance in visuomotor processing (Curtis et al., 2004; Curtis and D'Esposito, 2006; Mars et al., 2008). Accordingly, encoding of spatial precue information regarding multiple movement-targets taxes especially the parietal cortex, while direct or memory-guided visuomotor selection is more dependent on premotor cortex. Note that this visuomotor selection must be separate from actual execution processes or else the frontal theta synchronization for the 1-target condition would not have been virtually absent.
Event-Related Theta Power—Task and Load Effects
Theta synchronization phases following precue and response signal were identical between tasks, except for a lower power parietal synchronization following the response signal in the Memory compared to the Visual search task (Figure 5). The lower power theta synchronization in the Memory search task argues against an identification of this theta synchronization phase with short-term memory retrieval. An alternative account that potentially reconciles the evidence from both tasks takes the theta synchronization more broadly as related to the retrieval of choice-relevant information (Womelsdorf et al., 2010, see below), in this case spatial information, either from visual spatial memory or from an actual visual display. Relevant in this context is recent evidence involving the N2pc event-related potential, associated with visual selection of a target in a multi-element array. Just as we find theta synchronization in visual and memory search task versions, the N2pc is present not only in visual search, but also with search in visual short-term memory (Kuo et al., 2009; Dell'Acqua et al., 2010), albeit of lower amplitude. The attenuated amplitude of the N2pc in visual spatial memory search matches the attenuated theta synchronization we found in the memory search task. This is not to say that the N2pc is the time-domain equivalent of the theta synchronization; the N2pc has an occipito-temporal scalp distribution (Praamstra and Kourtis, 2010), distinct from the parietal theta effect studied here1.
The most prominent load effect is the absent theta synchronization phase after the response signal in the 1-target condition. This absence sets the 1-target condition apart from the other conditions and is consistent with the fact that only in this condition subjects can—already after the precue—select the movement-target and prepare the response, preempting such a selection phase following the response signal. The theta synchronization thus only occurs with those load conditions where a decision or selection must be made, i.e., the 2- and 4-target conditions. The theta synchronization, therefore, seems to reflect, beyond the retrieval of choice-relevant information, also the decision process. How does the behavior of the theta synchronization in the 2- and 4-target conditions fit with this interpretation? The behavior after the response signal is characterized by identical changes in power over the parietal cortex, contrasting with higher synchronization for the 2- than the 4-target condition over the frontal cortex. One might explain this divergence in load effects on parietal and frontal theta activity again in terms of a distinction between visuospatial and visuomotor processing (e.g., Curtis et al., 2004; Curtis and D'Esposito, 2006; but see Lindner et al., 2010). Thus, the parietal theta would be involved in a process of activating a visuospatial representation, but relatively insensitive to the complexity of that representation, while the frontal theta is sensitive to the load difference by virtue of its visuomotor selection function.
Conclusions
Recent work describing modulations of parietal (Perfetti et al., 2011) and frontal (Tombini et al., 2009) theta activity in motor tasks has tentatively linked their observations to recruitment of cognitive or attentional resources. Cruikshank et al. (2012), by contrast, attribute frontally distributed theta synchronization, during initiation of reaching movements, to sensorimotor integration processes. The present report extends this recent work on theta synchronization in motor tasks by revealing parietal and frontal theta synchronization effects in a pointing task. The effects are not likely explained in terms of memory processes. What the present and the previous work on theta synchronization in motor tasks have in common is that they required spatial (attentional) selection of a target in order to capture it with a movement. In our task, full precue information on the location of the movement-target in the 1-target condition preempted theta synchronization following the response cue, supporting the proposal that the synchronization is genuinely related to a selection or decision process and not to movement per se. The theta activity elicited by such a selection or decision process may or may not be specific to the domain of sensorimotor integration. A unifying account of theta activity was recently proposed by Womelsdorf et al. (2010), describing its function as structured retrieval of choice-relevant information around behavioral decision points. The relevant retrieval operations may concern information from different brain areas, proceed within just a few cycles, and involve communication of top-down and bottom-up information (Womelsdorf et al., 2010). This account seems particularly appropriate to the theta synchronization in reaching and pointing tasks that rely on fast sensorimotor integration.
The present results show parietal and frontal theta synchronization in overlapping time windows. Further progress in determining its mechanistic and functional role may be expected from analyses that address their temporal coordination to evaluate functional interactions between parietal and frontal cortex. Likewise, analyses of theta-gamma coupling are relevant to elucidate the local computations carried out in parietal and frontal cortex separately. Such approaches may help close the gap with monkey studies on parietal and premotor cortex contributions to movement-target selection (e.g., Balan et al., 2008; Cohen et al., 2009; Gail et al., 2009).
Conflict of Interest Statement
The authors declare that the research was conducted in the absence of any commercial or financial relationships that could be construed as a potential conflict of interest.
Acknowledgments
R. Chris Miall was funded by the Wellcome Trust.
Footnotes
- ^Although not reported here, we did analyze the N2pc associated with visual search and memory search in the present data. Like Kuo et al. (2009), we found a considerably lower amplitude for the N2pc in the Memory compared to the Visual search task. In contrast to these authors, we also found a load effect on the N2pc amplitude (reduction amplitude with increasing load), as there should be according to Dell'Acqua et al. (2010). In both tasks, the N2pc had a well-defined occipito-temporal distribution.
References
Balan, P. F., Oristaglio, J., Schneider, D. M., and Gottlieb, J. (2008). Neuronal correlates of the set-size effect in monkey lateral intraparietal area. PLoS Biol. 6:e158. doi: 10.1371/journal.pbio.0060158
Bastiaansen, M. C., Posthuma, D., Groot, P. F., and de Geus, E. J. (2002). Event-related alpha and theta responses in a visuo-spatial working memory task. Clin. Neurophysiol. 113, 1882–1893.
Berg, P., and Scherg, M. (1994). A multiple source approach to the correction of eye artifacts. Electroencephalogr. Clin. Neurophysiol. 90, 229–241.
Bland, B. H., and Oddie, S. D. (2001). Theta band oscillation and synchrony in the hippocampal formation and associated structures: the case for its role in sensorimotor integration. Behav. Brain Res. 127, 119–136.
Burgess, A. P., and Gruzelier, J. H. (2000). Short duration power changes in the EEG during recognition memory for words and faces. Psychophysiology 37, 596–606.
Caplan, J. B., Madsen, J. R., Schulze-Bonhage, A., Aschenbrenner-Scheibe, R., Newman, E. L., and Kahana, M. J. (2003). Human theta oscillations related to sensorimotor integration and spatial learning. J. Neurosci. 23, 4726–4736.
Cisek, P. (2007). Cortical mechanisms of action selection: the affordance competition hypothesis. Philos. Trans. R. Soc. Lond. B Biol. Sci. 362, 1585–1599.
Cisek, P., and Kalaska, J. F. (2010). Neural mechanisms for interacting with a world full of action choices. Annu. Rev. Neurosci. 33, 269–298.
Cohen, J. Y., Heitz, R. P., Woodman, G. F., and Schall, J. D. (2009). Neural basis of the set-size effect in frontal eye field: timing of attention during visual search. J. Neurophysiol. 101, 1699–1704.
Crammond, D. J., and Kalaska, J. F. (2000). Prior information in motor and premotor cortex: activity during the delay period and effect on pre-movement activity. J. Neurophysiol. 84, 986–1005.
Cruikshank, L. C., Singhal, A., Hueppelsheuser, M., and Caplan, J. B. (2012). Theta oscillations reflect a putative mechanism for human sensorimotor integration. J. Neurophysiol. 107, 65–77.
Curtis, C. E., and D'Esposito, M. (2006). Selection and maintenance of saccade goals in the human frontal eye fields. J. Neurophysiol. 95, 3923–3927.
Curtis, C. E., Rao, V. Y., and D'Esposito, M. (2004). Maintenance of spatial and motor codes during oculomotor delayed response tasks. J. Neurosci. 24, 3944–3952.
Dell'Acqua, R., Sessa, P., Toffanin, P., Luria, R., and Jolicoeur, P. (2010). Orienting attention to objects in visual short-term memory. Neuropsychologia 48, 419–428.
Düzel, E., Penny, W. D., and Burgess, N. (2010). Brain oscillations and memory. Curr. Opin. Neurobiol. 20, 143–149.
Gail, A., Klaes, C., and Westendorff, S. (2009). Implementation of spatial transformation rules for goal-directed reaching via gain modulation in monkey parietal and premotor cortex. J. Neurosci. 29, 9490–9499.
Green, J. J., and McDonald, J. J. (2008). Electrical neuroimaging reveals timing of attentional control activity in human brain. PLoS Biol. 6:e81. doi: 10.1371/journal.pbio.0060081
Green, J. J., Doesburg, S. M., Ward, L. M., and McDonald, J. J. (2011). Electrical neuroimaging of voluntary audiospatial attention: evidence for a supramodal attention control network. J. Neurosci. 31, 3560–3564.
Jensen, O. (2005). Reading the hippocampal code by theta phase-locking. Trends Cogn. Sci. 9, 551–553.
Jensen, O., and Tesche, C. D. (2002). Frontal theta activity in humans increases with memory load in a working memory task. Eur. J. Neurosci. 15, 1395–1399.
Klaver, P., Talsma, D., Wijers, A. A., Heinze, H. J., and Mulder, G. (1999). An event-related brain potential correlate of visual short-term memory. Neuroreport 10, 2001–2005.
Klimesch, W. (1999). EEG alpha and theta oscillations reflect cognitive and memory performance: a review and analysis. Brain Res. Brain Res. Rev. 29, 169–195.
Klimesch, W., Doppelmayr, M., Stadler, W., Pöllhuber, D., Sauseng, P., and Röhm, D. (2001). Episodic retrieval is reflected by a process specific increase in human electroencephalographic theta activity. Neurosci. Lett. 302, 49–52.
Klimesch, W., Schimke, H., and Schwaiger, J. (1994). Episodic and semantic memory: an analysis in the EEG theta and alpha band. Electroencephalogr. Clin. Neurophysiol. 91, 428–441.
Kuo, B.-C., Rao, A., Lepsien, J., and Nobre, A. C. (2009). Searching for targets within the spatial layout of visual short-term memory. J. Neurosci. 29, 8032–8038.
Lee, K. M., and Keller, E. L. (2008). Neural activity in the frontal eye fields modulated by the number of alternatives in target choice. J. Neurosci. 28, 2242–2251.
Lindner, A., Iyer, A., Kagan, I., and Andersen, R. A. (2010). Human posterior parietal cortex plans where to reach and what to avoid. J. Neurosci. 30, 11715–11725.
Lisman, J., and Buzsáki, G. (2008). A neural coding scheme formed by the combined function of gamma and theta oscillations. Schizophr. Bull. 34, 974–980.
Makeig, S., Delorme, A., Westerfield, M., Jung, T. P., Townsend, J., Courchesne, E., and Sejnowski, T. J. (2004). Electroencephalographic brain dynamics following manually responded visual targets. PLoS Biol. 2:e176. doi: 10.1371/journal.pbio.0020176
Maris, E., and Oostenveld, R. (2007). Nonparametric statistical testing of EEG- and MEG-data. J. Neurosci. Methods 164, 177–190.
Mars, R. B., Coles, M. G., Hulstijn, W., and Toni, I. (2008). Delay-related cerebral activity and motor preparation. Cortex 44, 507–520.
McCollough, A. W., Machizawa, M. G., and Vogel, E. K. (2007). Electrophysiological measures of maintaining representations in visual working memory. Cortex 43, 77–94.
Medendorp, W. P., Beurze, S. M., Van Pelt, S., and Van Der Werf, J. (2008). Behavioral and cortical mechanisms for spatial coding and action planning. Cortex 44, 587–597.
Meyer, T., Qi, X. L., and Constantinidis, C. (2007). Persistent discharges in the prefrontal cortex of monkeys naive to working memory tasks. Cereb. Cortex 17 (Suppl. 1), i70–i76.
Oostenveld, R., and Praamstra, P. (2001). The five percent electrode system for high resolution EEG and ERP measurements. Clin. Neurophysiol. 112, 713–719.
Perfetti, B., Moisello, C., Landsness, E. C., Kvint, S., Pruski, A., Onofrj, M., Tononi, G., and Ghilardi, M. F. (2011). Temporal evolution of oscillatory activity predicts performance in a choice-reaction time reaching task. J. Neurophysiol. 105, 18–27.
Praamstra, P., and Kourtis, D. (2010). An early parietal ERP component of the frontoparietal system: EDAN ≠ N2pc. Brain Res. 1317, 203–210.
Praamstra, P., Kourtis, D., and Nazarpour, K. (2009). Simultaneous preparation of multiple potential movements: opposing effects of spatial proximity mediated by premotor and parietal cortex. J. Neurophysiol. 102, 2084–2095.
Robitaille, N., Marois, R., Todd, J., Grimault, S., Cheyne, D., and Jolicoeur, P. (2010). Distinguishing between lateralized and nonlateralized brain activity associated with visual short-term memory: fMRI, MEG, and EEG evidence from the same observers. Neuroimage 53, 1334–1345.
Sauseng, P., Griesmayr, B., Freunberger, R., and Klimesch, W. (2010). Control mechanisms in working memory: a possible function of EEG theta oscillations. Neurosci. Biobehav. Rev. 34, 1015–1022.
Sauseng, P., Klimesch, W., Gruber, W. R., and Birbaumer, N. (2008). Cross-frequency phase synchronization: a brain mechanism of memory matching and attention. Neuroimage 40, 308–317.
Tombini, M., Zappasodi, F., Zollo, L., Pellegrino, G., Cavallo, G., Tecchio, F., Guglielmelli, E., and Rossini, P. M. (2009). Brain activity preceding a 2D manual catching task. Neuroimage 47, 1735–1746.
Tzagarakis, C., Ince, N. F., Leuthold, A. C., and Pellizzer, G. (2010). Beta-band activity during motor planning reflects response uncertainty. J. Neurosci. 30, 11270–11277.
Vogel, E. K., and Machizawa, M. G. (2004). Neural activity predicts individual differences in visual working memory capacity. Nature 428, 748–751.
Keywords: movement preparation, reaching, motor cortex, parietal cortex, theta oscillations
Citation: Rawle CJ, Miall RC and Praamstra P (2012) Frontoparietal theta activity supports behavioral decisions in movement-target selection. Front. Hum. Neurosci. 6:138. doi: 10.3389/fnhum.2012.00138
Received: 12 February 2012; Accepted: 30 April 2012;
Published online: 18 May 2012.
Edited by:
Shuhei Yamaguchi, Shimane University, JapanReviewed by:
Jun'Ichi Katayama, Kwansei Gakuin University, JapanKeiichi Onoda, Shimane University, Japan
Copyright: © 2012 Rawle, Miall and Praamstra. This is an open-access article distributed under the terms of the Creative Commons Attribution Non Commercial License, which permits non-commercial use, distribution, and reproduction in other forums, provided the original authors and source are credited.
*Correspondence: Peter Praamstra, Department of Neurology, Radboud University Medical Centre, Radboud University, PO Box 9101, 6500 HB Nijmegen, Netherlands. e-mail: p.praamstra@neuro.umcn.nl