Electrophysiological indicators of surprise and entropy in dynamic task-switching environments
- 1Department of Neurology, Hannover Medical School, Hannover, Germany
- 2Cognitive Neurology, Technische Universität Braunschweig, Braunschweig, Germany
- 3Department of Research Methods and Biopsychology, Technische Universität Braunschweig, Braunschweig, Germany
This event-related brain potential (ERP) study aimed at bridging two hitherto widely separated domains of cognitive neuroscience. Specifically, we combined the analysis of cognitive control in a cued task-switching paradigm with the fundamental question of how uncertainty is encoded in the brain. Two functional models of P3 amplitude variation in cued task-switching paradigms were put to an empirical test: (1) According to the P3b surprise hypothesis, parietal P3b waveforms are related to surprise over switch cues. (2) According to the P3a entropy hypothesis, frontal P3a waveforms are associated with entropy over switch outcomes. In order to examine these hypotheses, we measured the EEG while sixteen healthy young participants performed cued task-switching paradigms closely modeled to the Wisconsin Card Sorting Test (WCST). We applied a factorial design, with number of tasks (two vs. three viable tasks), cue explicitness (task cuing vs. transition cuing), and cue contingency (prospectively-signaled cuing vs. feedback-based cuing) as independent variables. The ERP results replicated the commonly reported P3b effect associated with task switches, and further showed that P3a amplitudes were related to the entropy of switch outcomes, thereby supporting both hypotheses. Based on these ERP data, we suggest that surprise over task switches, and entropy over switch outcomes, constitute dissociable functional correlates of P3b and P3a ERP components in task-switching paradigms, respectively. Finally, a theoretical integration of the findings is proposed within the framework of Sokolov's (1966) entropy model of the orienting response (OR).
Introduction
In task-switching paradigms, one of several viable stimulus-response mappings (also referred to as task sets) needs to be executed at any one time, based on contextual information provided by instructions or by other stimuli (cued task-switching; see Monsell, 2003; Kiesel et al., 2010; for reviews). Task-switching paradigms thus provide dynamic environments which involve frequent state transitions, and which thereby probe contextual adjustment by the performers. Task-switching paradigms are often considered benchmark in research on neurocognitive mechanisms of executive control (Shallice et al., 2008; Nyhus and Barceló, 2009; Kopp and Wessel, 2011) since deficient contextual adjustment represents one of the hallmarks of executive dysfunctions which occur in some brain-lesioned patients (Kopp, 2012).
In principle, there exist two categories of models of cognitive control in task-switching paradigms, which we here refer to as meta-level models and task-level models (see Mayr et al., 2013, for a more detailed discussion). Meta-level models assume that a task switch “… necessitates a process (or a set of processes) that operates on an abstract, hierarchically higher level than that of specific tasks” (Mayr et al., 2013; p. 491). However, Kiesel et al. (2010) concluded that the necessity of higher-level control has not yet been settled in the relevant literature. Task-level models assume that task control can be established exclusively through mutual competition between task sets, without any requirement of higher-level processes which should be related to the need to switch back and forth between task sets (e.g., Gilbert and Shallice, 2002; Logan and Bundesen, 2003).
Conceptualizing meta-level task control as an all-or-none phenomenon may be an inadequate oversimplification of a continuously variable phenomenon. For example, the dynamics of task control might necessitate the contribution of meta-level processes to the degree that decisions about task sets are uncertain (Kepecs and Mainen, 2012). Figure 1 depicts two state transition diagrams which illustrate task switching under certainty and under uncertainty. Specifically, inspection of Figure 1 reveals that transitions between task sets can be conducted with certainty on two-task paradigms, whereas three-task paradigms imply uncertain transitions between task sets. This consideration led to the present study which focuses on task-set uncertainty. Our study thereby touches the fundamental question of how uncertainty influences behavior and how it is encoded in the brain (Bach and Dolan, 2012; Badre et al., 2012).
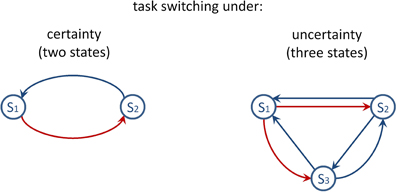
Figure 1. Task-switching paradigms considered as dynamic environments which involve frequent state transitions. Left panel: The simple diagram shows state transitions when two tasks are viable. The red arrow indicates that S1 passes on to S2 (i.e., switching between task sets consists of a one-alternative choice). The blue arrow illustrates the alternative switch between task sets. Right panel: The diagram shows state transitions when three tasks are viable. The red arrows indicate that S1 passes on to S2 or to S3 (i.e., switching between task sets consists of a two-alternative choice). The blue arrows illustrate possible other task switches.
The Wisconsin Card Sorting Test (WCST; Berg, 1948; Grant and Berg, 1948; Heaton et al., 1993) represents a clinical benchmark task-switching paradigm (Milner, 1963; Nyhus and Barceló, 2009; Kopp and Wessel, 2011), since patients with frontal brain damage commit more errors on the WCST than patients with non-frontal damage (Barceló and Knight, 2002; Demakis, 2003). The WCST possesses two highly specific, yet important characteristics: (1) WCST stimuli differ with regard to the color, shape, and number of depicted objects, and these three stimulus dimensions define three viable tasks. (2) Further, to cite the original articles: “As the S [subject] sorted the response cards he was informed whether he was ‘right’ or ‘wrong’.” (Berg, 1948, p. 16; Grant and Berg, 1948, p. 405). Thus, solely “wrong”-cues, following erroneous sorts, signal the need to switch task sets, but they do not indicate the currently prevailing task on the WCST. Taken together, there are at least three hypothetical explanations for why the WCST is found to be one of the few task-switching paradigms with documented sensitivity for frontal lobe dysfunction (Barceló and Knight, 2002; Demakis, 2003). (1) The WCST may place high load on working memory (the memory load hypothesis). (2) Successful performance on the WCST may hinge on efficient action-outcome monitoring (the contingency hypothesis). (3) The WCST may challenge task switching under uncertainty (the uncertainty hypothesis). The rationale of each of these three hypotheses will be briefly discussed in the following paragraphs.
The memory load hypothesis rests on the fact that contextual cues on the WCST are transition cues, indicating that task sets need to be changed, without specifying which of the three tasks is currently effective, whereas task cues would provide explicit information about the currently effective task. Schneider and Logan (2007) suggested that transition cues place greater demands on the retrieval of task sets in comparison to task cues. Forstmann et al. (2005) showed functional activation of lateral prefrontal areas by transition cues, but not by task cues. Further, based on event-related brain potential (ERP) recordings, West et al. (2011) reported that transition cues evoked more pronounced frontal positivities than task cues did. This analysis led to one of our experimental manipulations (cue explicitness) in which labels such as “switch” (rather than “wrong” as in the original WCST) and “repeat” (rather than “right” as in the original WCST) were used for transition-cuing, whereas the labels “color,” “shape,” and “number” constituted the stimulus material for explicit task cuing.
The contingency hypothesis focuses on the fact that contextual cuing rests on feedback stimuli on the WCST, such that successful performance requires efficient action-outcome monitoring (Kopp and Wessel, 2011). Action-outcome prediction (Alexander and Brown, 2010, 2011) has been considered to form the basis of performance monitoring, a widely studied frontal function (Gehring and Knight, 2000; Ullsperger et al., 2002; Ridderinkhof et al., 2004; Wessel et al., 2012). Throughout this article, we call the feedback-based method of contextual cuing feedback-based (F-B) cuing. In contrast to F-B cuing, many task-switching paradigms provide information about the currently effective task by delivering contextual cues before the appearance of the imperative stimuli. Throughout this article, this contextual cuing method will be called prospectively-signaled (P-S) cuing. This analysis led to another experimental manipulation (cue contingency), in which we compared the effects of F-B cues with the effects of P-S cues, as further described below.
Figure 2 illustrates event structures over time within the initial three trials of task runs, separately for P-S cuing and for F-B cuing. It can be seen that cues (e.g., C1; read: “cue on trial 1”) precede targets (e.g., T1; read: “target on trial 1”) on P-S cuing conditions, such that R1 (read: “response on trial 1”) will be correct (in the “ideal” performer). In contrast, because targets (e.g., T1) precede cue/feedback events (e.g., C/FB1; read: “cue/feedback on trial 1”) on F-B cuing conditions, R1 will necessarily be incorrect (even in the “ideal” performer). As a corollary, while first trials are state-transition trials on both cuing conditions, set shifts are expected to occur on first trials on P-S cuing conditions, whereas set shifts are expected to occur on second trials on F-B cuing conditions (see Table 1).
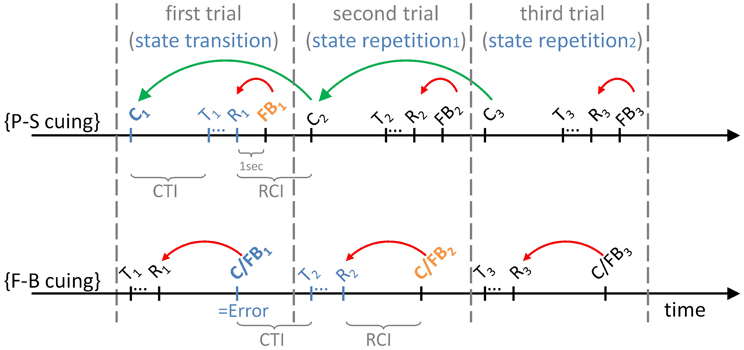
Figure 2. The temporal sequence of events on P-S cuing and on F-B cuing conditions. The figure illustrates the initial three trials of task runs. Cx, task cue on trial x{x = 1 … 3}; FBx, feedback stimulus on trial x{x = 1 … 3}; C/FBx, hybrid cue/feedback stimulus on trial x{x = 1 … 3}; Tx, target stimulus on trial x{x = 1 … 3}; Rx, response on trial x{x = 1 … 3}. CTI, cue-target interval (3 s); RCI, response-cue interval (3 s). Curved arrows indicate the contingencies: Cx-cues inform about possible state transitions, i.e., the currently effective task relative to the task that was effective on the previous trial on the P-S cuing condition; additional FBx-feedback stimuli inform about the correctness of the response, Rx, on the P-S cuing condition. Specifically, C1-cues (shown in blue) require set shifts, and FB1-feedback stimuli (shown in orange) enable evaluating the task-set decision. C/FBx-hybrid stimuli inform about the correctness of the response, Rx, on the F-B cuing condition. In the absence of other information, this feedback simultaneously serves as contextual information about possible state transitions. Specifically, a set shift is required when C/FB1-stimuli (shown in blue) signal that R1 was erroneous, whereas C/FB2-stimuli (shown in orange) enable evaluating the task-set decision.
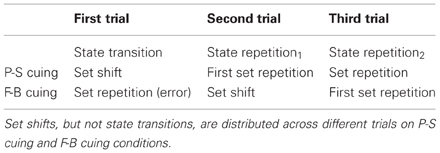
Table 1. The table shows the initial three trials of task runs for clarification of our nomenclature.
Further, the green curved arrows in Figure 2 indicate that P-S cues inform about the occurrence of state transitions vs. repetitions, irrespective of performance accuracy. Feedback information about performance accuracy is solely provided by additional feedback stimuli in P-S cuing paradigms (red curved arrows). Thus, task cues and feedback stimuli are dispersed in time within each trial on P-S cuing conditions. This characteristic of P-S cuing differs from F-B cuing where stimuli concomitantly serve as task cues and feedback stimuli (hence the notation C/FB, indicating that these events are hybrid cue and feedback events; red curved arrows).
The simplest way to evaluate the uncertainty hypothesis is to manipulate the number of viable tasks (cf. Figure 1). Subjects need to handle three viable task sets on the WCST, (i.e., to inhibit the previous set, e.g., “shape,” and to consider the other two sets for responding, e.g., “color” and “number”), whereas the use of two tasks is common in many task-switching experiments (Kiesel et al., 2010). Thus, as illustrated in Figure 1, whereas the WCST incorporates two-alternative choice (2AC) task-set decisions, many task-switching paradigms demand one-alternative choice (1AC) task-set decisions (i.e., to inhibit the previous set, e.g., “shape,” and to consider the other set for responding, e.g., “color,” when “number” is not a viable alternative). Note further that explicit task cuing generally implies 1AC task-set decisions, irrespective of the number of viable rules, because explicit task cues (e.g., “color”) specify the correct task directly.
The factorial combination of cue explicitness (task cuing vs. transition cuing), cue contingency (P-S vs. F-B cuing), and number of tasks (two vs. three tasks) led to differential dynamics of task-set decisions, as shown in Table 2. Decisions (D) to switch task sets follow C1, and the presentation of FB1 (read: “feedback on trial 1”) allows to evaluate (E) their correctness within the first trial on P-S cuing conditions. In contrast, decisions to switch sets task sets follow C/FB1 (which indicates erroneous performance), and evaluating their correctness is not possible before C/FB2 on the second trial on F-B cuing conditions. Table 2 offers an overview over the various possibilities, basically showing the occurrence of 1AC task-set decisions, and their evaluation, on all explicit task-cuing conditions (i.e., irrespective of the number of possible tasks) as well as on transition-cuing conditions with only two viable tasks. The sole exception from this pattern emerges on transition-cuing conditions with three viable tasks, where 2AC task-set decisions, and their evaluation, are required. Thus, 2AC task-set decisions are required on three-task transition-cuing conditions (task-switching under uncertainty), whereas 1AC task-set decisions are sufficient on all remaining conditions (task switching under certainty).
In the remainder of our introduction, we apply two classical measures of uncertainty, i.e., surprise and entropy, to task-switching. Specifically, we formalize surprise over switch cues and entropy over negative outcomes in order to prepare the derivation of hypotheses about the functional significance of two ERP measures which are consistently found in task-switching paradigms. To begin with, it is well-known that surprise, I(X),
and entropy, H(X),
for a binary random variable X represent two different measures of uncertainty (Shannon and Weaver, 1948). Figure 3 shows surprise and entropy as a function of probability for a binary random variable X [as in (1), (2)]. Note that the binary entropy function reaches a maximum at equal probabilities (i.e., at P = 0.5), whereas maximum surprise is associated with rareness or improbability (i.e., when P → 0.0).
Let
denote a subjective probability estimate that a state transition will occur on trial n ϵ {1, …, N}, given a sequence t(n − 1) = t(1), t(2), …, t(n − 1) of n − 1 former trials. Note that trial t(n) in (3) has not yet been observed, therefore, a subjective probability distribution Ps(n) for all possibilities {s, ns} on t(n) is of interest. However, once s has been observed on t(n) (which is only a single value s out of set {s, ns}), the respective subjective probability Ps(n) can be used to calculate the degree of surprise (Shannon and Weaver, 1948; Kolossa et al., 2013)
Let
denote a subjective probability estimate that a “wrong”-outcome will occur on trial t(n), given a sequence of n − 1 former trials. Note that the outcome on t(n) has not yet been observed, therefore, a subjective probability distribution Pw(n) for all possibilities {w, r} on t(n) is of interest. However, once w has been observed on t(n), the respective subjective probability Pw(n) can be used to calculate the degree of entropy (Shannon and Weaver, 1948; Kolossa et al., 2013)
Figure 4 illustrates that the subjective probability estimates Pw(n) need to be further conditioned on the serial position of t(n) within task runs. There are only two instances where Pw(n) will deviate from either P = 0.0 or P = 1.0, i.e., on the first trial on uncertain P-S cuing conditions, and on the second trial on uncertain F-B cuing conditions. Table 3 presents these conditional probabilities Pw(n) as they result in an ideal performer.
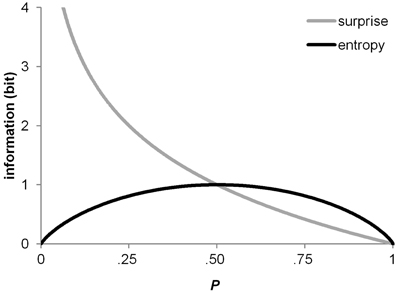
Figure 3. The surprise function and the entropy function as a function of probability over a binary random variable.
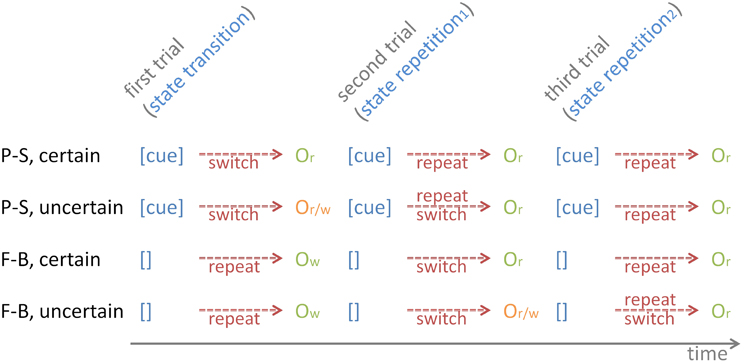
Figure 4. The temporal sequence of contextual cues and outcome stimuli on P-S cuing and on F-B cuing conditions, separately for certain and uncertain conditions. The figure illustrates the initial three trials of task runs. Contextual cues are provided on P-S cuing, but not on F-B cuing (depicted as []), which leads to erroneous task-set repetitions on state-transition trials, and concomitantly to “wrong”-outcomes (Ow). Note the uncertainty of outcomes (“right” or “wrong,” Or/w) on uncertain P-S state-transition trials and on uncertain F-B state-repetition1 trials. Depending on the outcome valence, task-set repetitions or task-set switches are required on subsequent trials.
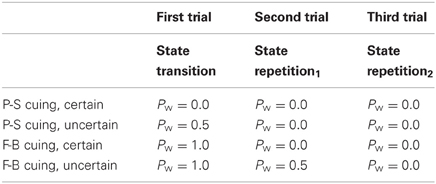
Table 3. Conditional probabilities for negative trial outcomes in an ideal performer, separately for P-S cuing and F-B cuing as well as for certain and uncertain cuing conditions, further conditioned on the serial position of trials within task runs.
The current study made use of ERP measures to examine hypothetical explanations for the documented frontal lobe sensitivity of the WCST. We now briefly discuss the P3b and P3a variants of the P300 ERP component (see for Polich, 2007; Duncan et al., 2009, for reviews) which are associated with uncertainty (see Kopp, 2008, for a conceptual review) and which play major roles in WCST-like task switching (Barceló, 2003). Barceló et al. (2002) used a modified version of the WCST and analyzed ERPs to the cue/feedback tones which were presented after every trial. ERPs to switch cues/feedbacks showed large frontal positivities (350–400 ms) as well as large later parietal positivities (550–600 ms) which decreased in amplitude across task runs. These ERP effects were interpreted as modulation of parietal P3b and frontal P3a waveforms as a result of switching task sets.
The observation of switch-related enhancements of parietal P3b amplitudes is a ubiquitous finding in the task-switching literature (Rushworth et al., 2002, 2005; Karayanidis et al., 2003, 2010; Kieffaber and Hetrick, 2005; Nicholson et al., 2005; Astle et al., 2006, 2008; Swainson et al., 2006; Jost et al., 2008; Travers and West, 2008; Periáñez and Barceló, 2009; Wylie et al., 2009; Gajewski and Falkenstein, 2011). Further, it is well-recognized that “surprising events elicit a large P300 component” (Donchin, 1981, p. 498) as specified in Donchin's surprise hypothesis of P3b amplitude modulations (see also Kolossa et al., 2013). Applied to switch-related enhancements of parietal P3b amplitudes, cue-locked P3b amplitudes should be proportional to surprise I(n), i.e.,
with I(n) as defined in (4), i.e., the surprise over task-set switches.
Barceló et al. (2002) found a rapid decline of frontal P3a amplitudes across task runs which they interpreted as being related to task-set uncertainty. Note that prominent P3a waveforms in response to cue/feedback events were also found in other WCST-like task-switching studies (Kopp et al., 2005, 2006; Barceló et al., 2006; Cunillera et al., 2012). Barceló et al. (2006) put forward the hypothesis that modulations of P3a amplitudes are generally related to the entropy which is conveyed by eliciting stimuli. Applied to switch-related enhancements of frontal P3a amplitudes, feedback-locked P3a amplitudes should be proportional to entropy H(n), i.e.,
with H(n) as defined in (6) and in Table 3, i.e., the entropy over outcomes, conditioned on task-set (un-)certainty and serial position within task runs.
We started our analysis of WCST-like task switching with the formulation of three hypotheses. The memory load hypothesis led us to expect strong frontal activities which should be evoked by transition cues, as indicated by frontal P3a waveforms. In contrast, the contingency hypothesis led us to expect strong frontal activities which should be evoked by F-B cues, again as indicated by frontal P3a waveforms. Finally, the uncertainty hypothesis led to two predictions. First, surprise over task-set switches, being related to their rareness or improbability, should modulate the parietal P3b. Second, entropy over switch outcomes should modulate the frontal P3a, such that P = 0.5 outcomes should elicit enhanced P3a amplitudes in comparison to both, P = 1.0 and P = 0.0 outcomes, which should evoke less prominent P3a waveforms.
Materials and Methods
Participants
A group of sixteen healthy undergraduate psychology students (M = 21.5 years; range 19–26 years; 14 females; 5 left-handed) participated for course credit. All had normal or corrected-to-normal vision. Nobody showed impairment in set switching abilities as indicated by the time they required to complete Trail Making Test A, 23.7 (M) ± 1.8 (SE) sec, and Trail Making Test B, 52.7 (M) ± 3.7 (SE) sec (Army Individual Test Battery, 1944).
The authors confirm that the research has been conducted according to all ethical standards imposed by their Ethics Committee at the Technische Universität Braunschweig, who approved the study. The study conforms to the Declaration of Helsinki. Written informed consent was obtained by all participants, according to the procedures imposed and approved by the above Ethics Committee.
Stimulus Materials and Procedure
The experiment was controlled by the Presentation® software (Albany, CA; http://www.neurobs.com). Target displays consisted of four key cards per trial which appeared invariantly above one stimulus card, all configured around the center of a computer screen against white background (Eizo FlexScan T766 19″; Hakusan, Ishikawa, Japan; http://www.eizo.com/global). The stimulus arrangement subtended a visual angle of 4° horizontally and 2.5° vertically at a viewing distance of 1.5 m. Participants indicated their sorting choice by pressing one of four keys on a standard computer keyboard which were mapped to the spatial position of the key cards on the screen (“C,” left middle finger, equaling choice of the outside left key card; “V,” left index finger, equaling choice of inside left key card; “N,” right index finger, equaling choice of inside right key card; “M,” right middle finger, equaling choice of outside right key card). Target displays remained on screen until a response was recognized. We selected only those stimulus cards of the original 64-card version of the WCST which share one and only one attribute with each of the four key cards (i.e., the stimulus cards which match one key card by color, another keycard by shape, and a third key card by number). Twenty-four stimulus cards fulfill this criterion (Nelson, 1976).
One single sequence of trials was generated pseudo-randomly by one of the authors (Florian Lange). This particular sequence of trials was utilized repeatedly throughout all experimental conditions. Thus, the succession of stimulus cards was exactly identical for all experimental conditions. Each stimulus card was presented five times per condition, adding up to a total of 120 trials. The sequence of trials included 31 rule switches, yielding an average run length of 3.8 trials, with a range of three to five trials per run. The succession of runs was identical for all two-task experimental conditions such that these four conditions differed solely with regard to cue explicitness and cue contingency. Likewise, the succession of runs was identical for all three-task experimental conditions were the sequence of trials consisted of eleven runs of “color” and “shape” sorting, respectively, as well as ten runs of trials which required sorting according to the “number” of objects displayed on the stimulus cards.
Depending on the experimental condition, target stimuli were differentially surrounded in time by cue and feedback stimuli (see Figure 2). On P-S cuing conditions, cues (C) preceded target stimuli, indicating the effective rule explicitly or implicitly. The time interval between cue onset and target onset amounted to 3.000 ms (cue-target interval, CTI). Subsequent cues were presented another 3.000 ms after responses to the targets (response-cue interval, RCI). Further, additional feedback stimuli (FB) were presented during the RCI (i.e., 1.000 ms after the responses) on P-S cuing conditions. Feedback was provided by displaying the German words for “correct” (“richtig”) and “wrong” (“falsch”), respectively, in black capital letters at the center of the screen. On F-B cuing conditions, responses were followed by a 3.000 ms time interval (RCI) until the onset of hybrid C/FB stimuli. This stimulus informed, explicitly or implicitly, whether the applied set was correct or not. The onset of C/FB stimuli was followed by another interval of 3.000 ms until the onset of the subsequent target stimuli (CTI). On transition-cuing conditions, C or C/FB stimuli were composed of the German words for “repeat” (“bleiben”) and “switch” (“wechseln”), respectively, superimposed on a black rectangle. On task-cuing conditions, these stimuli consisted of the German words for “color” (“Farbe”), “shape” (“Form”) and “number” (“Zahl”). All word stimuli were of equal size (2° horizontal visual angle, font: Arial 28), and their presentation duration amounted to 200 ms. Note that the two (i.e., color, shape) or three (i.e., color, shape, number) task cues occurred about equally often (i.e., in 50% vs. 50% or in 33% vs. 33% vs. 33% of the trials, respectively) on their respective two-task or three-task experimental conditions. In contrast, “switch”-cues occurred less frequently than “repeat”-cues on transition-cuing conditions [i.e., “switch”-cues on around 25% (31 out of 120) of the trials vs. “repeat”-cues on around 75% (89 out of 120) of the trials], irrespective of the number of viable task rules on the particular experimental condition.
Participants were instructed that their task would be to match the stimulus card with one of the four key cards in accordance with the appropriate sorting rule. They were further informed that the prevailing sorting rule would change from time to time in an unpredictable manner. They were told that cue stimuli or feedback stimuli, respectively, conveyed information about the correct sorting rule. Participants were discouraged to guess whether or not task rules might have changed on the current trial. They were further instructed to prioritize response accuracy over response speed. Prior to each experimental condition, participants' understanding of the particular task at hand was ascertained by running fifteen practice trials before the corresponding 120-trial sequence was initiated.
Experimental Design
The combination of number of viable task rules (two vs. three rules), cue contingency (P-S cuing vs. F-B cuing), and cue explicitness (task cuing vs. transition cuing) provided a 2 × 2 × 2 factorial design. Participants were examined on two separate days (time lag: M = 6 days ± 0.9 (SE), range 1–13 days) since each participant had to complete all eight experimental conditions (adding to 960 trials per participant). The sequential design of the experiment was balanced across participants, as follows: (1) 50% of the participants (N = 8) started with all two-task conditions on the first day, and 50% of the participants (N = 8) started with all three-task conditions on the first day. (2) Within each day, 50% of the participants (N = 4) started with P-S cuing, while 50% of the participants (N = 4) started with F-B cuing. (3) Finally, within each day and within each cue contingency condition, the order of task cuing and transition cuing conditions was balanced across participants, with 50% of the participants (N = 2) starting with task cuing, and 50% of the participants (N = 2) starting with transition cuing. Thus, the number of viable task rules constituted the slowest-changing factor, whereas cue explicitness formed the fastest-changing factor.
Electroencephalographic (EEG) Recordings
Continuous EEG was recorded by means of a QuickAmps-72 amplifier (Brain Products, Gilching, Germany; www.brainproducts.com) and the BrainVision Recorder® Version 1.02 software (Brain Products, Gilching, Germany; www.brainproducts.com) from frontal (F7, F3, Fz, F4, F8), central (T7, C3, Cz, C4, T8), parietal (P7, P3, Pz, P4, P8), occipital (O1, O2), and mastoid (M1, M2) sites. Ag-AgCl EEG electrodes were used. They were mounted on an EasyCap (EasyCap, Herrsching-Breitbrunn, Germany; www.easycap.de). Electrode impedance was kept below 10 kΩ. All EEG electrodes were referenced to average reference. Participants were informed about non-cerebral artifacts and asked to avoid eye and limb movements as well as bucco-facial muscle activities (Picton et al., 2000). Ocular artifacts were monitored by means of bipolar pairs of electrodes positioned at the sub- and supraorbital ridges (vertical electrooculogram, vEOG) and at the external ocular canthi (horizontal electrooculogram, hEOG). The EEG and EOG channels were amplified with a band-pass of 0.01–40 Hz and digitized at 250 Hz.
Off-line analysis was performed by means of the BrainVision Analyzer® Version 2.0 software (Brain Products, Gilching, Germany; www.brainproducts.com). The contribution of ocular artifacts to the EEG was eliminated by applying independent component analysis. A digital high-pass filter was applied to the data (0.33 Hz, 24 db/oct) in order to eliminate low-frequency variations in the EEG signal which were associated with the occasional occurrence of electro-dermal artifacts. Further artifacts were removed semi-automatically [maximum allowed voltage step per sampling point: 50 μ V; maximum allowed amplitude difference: 70 μ V; lowest allowed activity (max-min, interval length 100 ms): 0.5μ V]. In a second step, the accuracy of the initially automatic artifact rejection was approved by visual inspection. By applying these rejection criteria, less than one percent of trials had to be discarded.
The EEG was then divided into epochs of 1.100 ms duration, starting 100 ms before onset of the events of interest (see below). A 100 ms pre-stimulus baseline was subtracted from the sampling points before the EEG was averaged off-line. Further, all EEG electrodes were re-referenced to the algebraic average of both mastoid electrodes, (M1 + M2)/2. However, since the resulting ERP waveforms did not differ substantially between the two reference methods, analyses and results are reported for average reference only.
Data Analysis
SPSS 13.0 served for statistical analyses (IBM, Armonk, NY; http://www-01.ibm.com/software/analytics/spss). Statistical significance level was set at p < 0.01.
Behavioral Data
For the eight experimental conditions, median response times (RTs) were computed for each participant, separately for set-shift trials and for first set-repetition trials (see Table 1). Comparing response latencies across these trials allows quantifying behavioral switch costs (Kiesel et al., 2010). These median RTs were subjected to a 2 × 2 × 2 × 2 analysis of variance (ANOVA) with number of viable task rules (two vs. three), cue contingency (P-S cuing vs. F-B cuing), cue explicitness (task cuing vs. transition cuing), and trial (set-shift trial vs. first set-repetition trial, Table 1) as within-subject factors. Only error-free trial sequences were selected for analysis since we aimed at eliminating confounding contributions of potential post-error slowing (Rabbitt, 1966). In addition, mean error percentages across the initial three trials of task runs were calculated (Table 1).
EEG Data Analysis
ERP measures were primarily derived from stimulus-locked averages of error-less trials (which are denoted here as COR). Averaging was generally conducted for Cx and FBx:cor, x = 1 … 3, stimuli, i.e., separately for individual trials of task runs on P-S cuing conditions (Figure 1). Likewise, on F-B cuing conditions, averaging was conducted for C/FBy:cor, y = 2 … 3, stimuli. In addition, ERPs were analyzed in response to C/FB1:inc stimuli on all F-B cuing conditions (which served as switch cues). Finally, ERPs evoked by FB1:inc on P-S cuing conditions and those elicited by C/FB2:inc on F-B cuing conditions were analyzed when three rules were viable and cuing was implicit (i.e., on transition cuing). Note that sufficiently large numbers of error trials for analysis were only available in these, but not in other, conditions (Table 4).
Mean P3a amplitudes were measured at electrode Fz in a 120 ms (±60 ms) interval around P3a peak latencies for each participant. P3a peak latencies corresponded to the largest positive deflection in individual ERP waveforms within the latency range between 340 and 440 ms following stimulus onset. P3a peak detection was performed on low-pass filtered individual ERP waveforms (12 Hz, 48 db/octave) which showed prominent P3a waves. Specifically, individual ERP waveforms evoked by FB1:cor and FB1:inc stimuli on three-task P-S transition cuing conditions and by C/FB2:cor and C/FB2:inc stimuli on three-task F-B transition cuing conditions (i.e., the 2AC trials, Table 2) served for determining individual P3a peak latencies. Averaged individual P3a peak latencies were calculated for comparisons with mean P3a amplitudes obtained from the remaining conditions which did not show prominent P3a waves. Specifically, the average latency, avP − S = (latency FB1:cor + latency FB1:inc)/2 on three-task P-S transition cuing conditions served as the midpoint of the 120 ms (±60 ms) latency window on the remaining P-S cuing conditions, whereas the average latency, avF − B = (latency C/FB2:cor + latency C/FB2:inc)/2 on three-task F-B transition cuing conditions served as the midpoint of the 120 ms (±60 ms) latency window on the remaining F-B cuing conditions. Mean P3b amplitudes were generally measured at electrode Pz in the interval between 450 ms and 700 ms following stimulus onset since the P3b did not show obvious peaks in individual ERP waveforms.
Results
Behavioral Results
As can be seen by inspection of Table 4, response accuracy generally increased across the initial three trials of task runs (Table 1). Error proportions on these trials demonstrated strong differences between P-S cuing and F-B cuing (Figure 2) as well as between conditions involving 1AC and 2AC task-set decisions (Table 2). Participants switched rarely to the wrong task set when confronted with 1AC decisions on first trials on P-S cuing conditions, whereas F-B cuing conditions produced errors on first trials by default. As expected, set-shift trials (Table 1) provoked around 50% erroneous responses when they were associated with 2AC task-set decisions (Table 2), i.e., on transition-cuing conditions with three viable tasks, irrespective of cue contingency (i.e., on P-S cuing and on F-B cuing conditions).
Comparing RTs by means of a 2 × 2 × 2 × 2 ANOVA with number of viable tasks (two vs. three), cue contingency (P-S cuing vs. F-B cuing), cue explicitness (task cuing vs. transition cuing), and trial (set-shift trial vs. first set-repetition trial) as within-subject factors revealed a significant effect of the trial factor [F(1, 15) = 14.94, p < 0.01], indicating the general occurrence of RT switch costs across experimental conditions (set-shift trials M = 1.109 ms; first set-repetition trials M = 1.009 ms), since no other main or interaction effect proved significant (all p > 0.02).
ERP Results
The analysis of the ERP waveforms has three main parts: (1) confirmatory evaluation of the P3b surprise hypothesis, (2) confirmatory evaluation of the P3a entropy hypothesis, and (3) exploratory evaluation of outcome valence effects.
Evaluation of the P3b Surprise Hypothesis
Switch-related effects on cue-locked ERP waveforms can be gleaned from inspection of Figure 5 by comparing ERPs (a) evoked by C1 (switch) cues vs. those elicited by C2 and C3 (repeat) cues on P-S cuing conditions, and (b) evoked by C/FB1:inc (switch) stimuli vs. those elicited by C/FB2:cor and C/FB3:cor (repeat) stimuli on F-B cuing conditions (Figure 2).
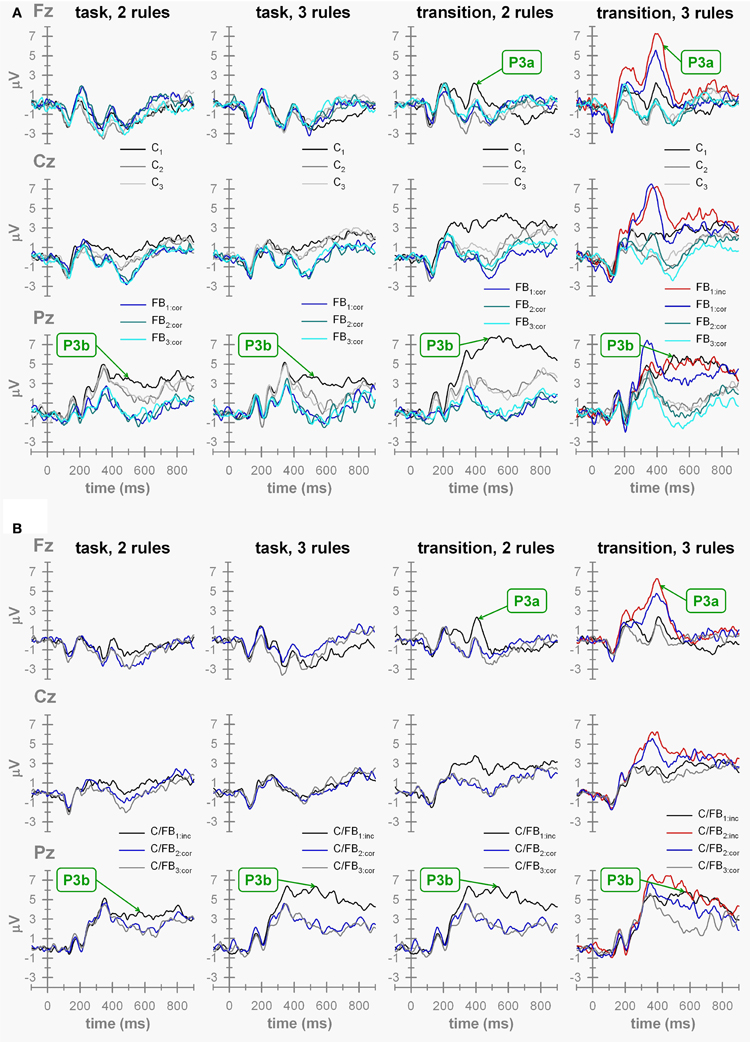
Figure 5. ERP waveforms. (A) ERP waveforms obtained on P-S cuing conditions. “task,” task-cuing conditions (two-task, three-task conditions) with Cx, task cue on trial x{x = 1 … 3}; FBx:cor, correct feedback stimulus on trial x{x = 1 … 3}. “transition”, transition-cuing conditions (two-task, three-task conditions) with Cx = task cue on trial x{x = 1 … 3}; FBx:cor = correct feedback stimulus on trial x{x = 1 … 3} and FB1:inc, incorrect feedback stimulus on trial 1. (B) ERPs waveforms obtained on F-B cuing conditions. “task,” task-cuing conditions (two-task, three-task conditions) with C/FBx, hybrid cue/feedback stimulus on trial x{x = 1 … 3; “cor,” correct; “inc,” incorrect}. “transition,” transition-cuing conditions (two-task, three-task conditions) with C/FBx, hybrid cue/feedback stimulus on trial x{x = 1 … 3; “cor,” correct; “inc,” incorrect}.
As a proxy for switch-related effects, we compared C1-locked vs. C3-locked P3b waveforms on P-S cuing conditions and C/FB1:inc-locked vs. C/FB3:cor-locked P3b waveforms on F-B cuing conditions. The resulting 2 × 2 × 2 × 2 ANOVA with number of viable tasks (two vs. three), cue contingency (P-S cuing vs. F-B cuing), cue explicitness (task cuing vs. transition cuing), and trial (first trial vs. third trial) as within-subject factors showed a significant explicitness by trial interaction, F(1, 15) = 29.01, p < 0.01, indicating more pronounced P3b amplitude switch-related effects on transition cuing conditions compared to task cuing conditions. Separate analyses for the two levels of cue explicitness revealed that P3b amplitudes showed significant switch-related effects on both conditions, F(1, 15) = 25.33, p < 0.01 (task-cuing condition), F(1, 15) = 166.51, p < 0.01 (transition-cuing condition). Thus, we found evidence for switch-related effects on parietal P3b amplitudes as they are consistently reported in the task-switching literature (Karayanidis et al., 2010). Over and above this often replicated finding, the switch-related effects on parietal P3b amplitudes were modulated by cuing method such that more pronounced switch-related effects occurred on transition-cuing conditions.
Evaluation of the P3a Entropy Hypothesis
Two ANOVAs were conducted to evaluate the hypothesis. The first ANOVA targeted the effects of post-switch outcome uncertainty (i.e., FB1-locked P3a amplitudes on P-S cuing conditions and C/FB2-locked P3a amplitudes on F-B cuing conditions) across all experimental conditions (Figure 5). Inspection of the ERP waveforms reveals that pronounced P3a waveforms were solely evoked by FB1- and by C/FB2-feedback stimuli on three-task transition-cuing conditions, i.e., when the correctness of task-set decisions was unpredictable. This impression was corroborated by the results of the 2 × 2 × 2 ANOVA with number of viable tasks (two vs. three), cue contingency (P-S cuing vs. F-B cuing) and cue explicitness (task cuing vs. transition cuing) as within-subject factors. This ANOVA showed significant effects of number of tasks, F(1, 15) = 27.89, p < 0.01, cue explicitness, F(1, 15) = 45.01, p < 0.01, as well as of the number of tasks by cue explicitness interaction, F(1, 15) = 24.92, p < 0.01, with all remaining F-values < 1. The interaction between number of viable tasks and cue explicitness is illustrated in Figure 6 (left panel). Separate ANOVAs were performed on each number of tasks condition to further parse the two-way interaction. These analyses revealed that P3a amplitudes showed significant cue explicitness effects on three-task conditions, F(1, 15) = 37.13, p < 0.01 (task cuing: M = −0.98 μV, transition cuing: M = 5.00 μV), but not on two-task conditions, F(1, 15) = 5.83, p > 0.01 (task cuing: M = −1.40 μV, transition cuing: M = −0.60 μV). Thus, post-switch outcome events evoked enhanced P3a amplitudes specifically when three viable tasks were combined with transition cues.
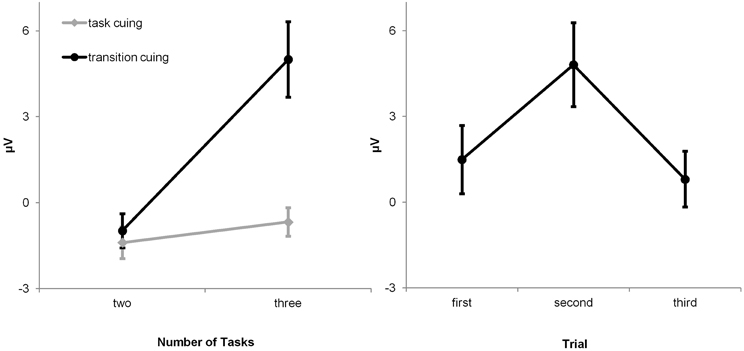
Figure 6. Mean (standard error) P3a amplitudes at Fz. Left panel: The interaction between cue explicitness and number of tasks reveals that P3a amplitudes vary as a function of post-switch outcome uncertainty which is high on three-task transition-cuing conditions and low on all remaining conditions. Right panel: P3a amplitudes follow the entropy function over outcome events across the initial three trials of task runs on the uncertain F-B cuing condition.
The second ANOVA was performed as a direct test for the P3a entropy hypothesis. Inspection of Table 4 reveals that negative-outcome probabilities across task runs on the three-task F-B transition cuing condition approached the P = 1.0, P = 0.5, and P = 0.0 values that were predicted for ideal performers (Table 3). Inspection of Figure 3 reveals that the P3a entropy hypothesis predicts enhanced P3a amplitudes elicited by P = 0.5 outcomes in comparison to both, P = 1.0 and P = 0.0 outcomes, which should indistinguishably evoke less prominent P3a waveforms. The omnibus ANOVA on P3a amplitudes evoked by C/FB2 (with Pw → 0.5), C/FB1 (with Pw → 1.0), and C/FB3 (with Pw → 0.0), outcomes, F(1, 15) = 21.39, p < 0.01, was followed by planned Helmert contrasts, i.e., (1) C/FB2 vs. (C/FB1 + C/FB3)/2, F(1, 15) = 35.72, p < 0.01, and (2) C/FB1 vs. C/FB3, F(1, 15) = 1.20, p > 0.01, respectively. These results are shown in Figure 6 (right panel), and they reveal that P3a amplitudes follow the predicted entropy pattern. Taken together, both ANOVAs devoted to the evaluation of the P3a entropy hypothesis showed that amplitude variability in P3a was related to outcome uncertainty, and more specifically, to the entropy over switch outcomes.
Analysis of Outcome Valence Effects
An exploratory comparison of ERP waveforms evoked by (roughly equiprobable, cf. Table 4) positive and negative outcomes was possible by comparing FB1:cor and C/FB2:cor against FB1:inc and C/FB2:inc, respectively. The resulting 2 × 2 ANOVAs with cue contingency (P-S cuing vs. F-B cuing) and outcome valence (positive vs. negative) as within-subject factors showed significant valence effects, F(1, 15) = 14.68, p < 0.01, for the P3b, but not for the P3a, F(1, 15) = 5.83, p > 0.01, indicating enhanced P3b, but not P3a, amplitudes evoked by negative outcomes compared to positive outcomes. Implications of this finding will be considered in the discussion section.
Discussion
This study explored relationships between various aspects of uncertainty and neuronal correlates of cognitive control in several modifications of a cued task-switching paradigm. Subjects switched between two or three task sets; contextual cues were either (explicit) task cues or (implicit) transition cues, and contextual cuing was either prospectively-signaled or feedback-based in WCST-like task-switching paradigms (Barceló, 2003). The manipulated variables of our experimental design exerted negligible influence on behavioral switch costs, as evidenced by their general and indistinguishable presence on all eight experimental conditions. There were two separable ERP components which could be easily distinguished by their topography. More importantly, they were dissociated by their antecedent conditions. The switch-related parietal P3b was commonly evoked by switch-cues on all experimental conditions, with the switch-related variability in P3b amplitude being more pronounced on transition cuing. Further, the P3b distinguished negative and positive outcomes. A frontal P3a was evoked by feedback stimuli, and it distinguished certain from uncertain task switching such that solely uncertain switch outcomes elicited a prominent P3a, irrespective of their valence. Each of these ERP components is discussed in turn.
Switch-Related Amplitude Variability in P3b
The switch-related parietal P3b has been present in many different task-switching experiments (see Karayanidis et al., 2010 for review), so that it has been difficult to assign any specific functional role to it. As one possibility, the switch-related P3b may index the updating of “attentional set” in our study, i.e., the updating of the relevant features of those stimuli which later determine action (Rushworth et al., 2005). However, since the switch-related P3b has so often been observed in a diversity of task-switching experiments, we prefer to interpret its occurrence not as an index of switching between attentional sets, but rather as indicating being unprepared to update (and, conversely, being prepared to repeat) task sets, irrespective of the nature of the particular sets concerned.
This idea was dubbed P3b surprise hypothesis in the introduction to this article; it has the advantage of being generally applicable to any kind of study. In fact, the historical roots of the P3b surprise hypothesis (Donchin, 1981) lie in oddball paradigms (e.g., Squires et al., 1976), and it is one of the major functional models of variability in parietal P3b amplitude (Kolossa et al., 2013). The predictions of the surprise model are clear in terms of how absolute switch probability should affect switch-related variability in P3b amplitude. As revealed by inspection of Figure 3, surprise shows an asymmetrical course over binary probability distributions, with maximal surprise when P → 0.0 and minimal surprise when P → 1.0. Thus, surprise over switches (P = 0.25) exceeds surprise over repetitions (P = 0.75) throughout all conditions of our experiment, thereby offering an explanation for the switch-related amplitude variability in P3b. In contrast to this, the switch-related amplitude variability in P3b cannot be accommodated to entropy over switches and repetitions, due to the symmetrical course of entropy over binary probability distributions. It is further of importance to note that a P3b occurred in response to all switch cues, irrespective of the level switch uncertainty.
It should be kept in mind, however, that our study does not directly pertain to the P3b surprise model since we did not manipulate absolute switch probability. How is task-switching affected by switch probability? In one of the few studies of this type, (Monsell and Mizon 2006; Experiment 4) manipulated switch probability (P = 0.25, P = 0.5, or P = 0.75) and showed that behavioral switch costs decreased as switches became more frequent. Further, Nessler et al. (2012) recently found that switch-related amplitude variability in P3b disappeared completely when switches and repetitions were equiprobable, and when the sequential succession of switches and repetitions was highly predictable. With regard to this, equiprobable switches and repetitions are, in and of itself, not sufficient for eliminating surprise over task-set switches (Barceló et al., 2006). Consider the possible succession of task-set switches (s) and task repetitions (r) over three consecutive trials (n-2, n-1, n), given switch probability P = 0.5. The succession of two task repetitions, i.e. r(n − 1)r(n), is equivalent to the succession of a particular task (with tasks denoted a or b), a(n − 2)a(n − 1)a(n), and a repetition after a switch, s(n − 1)r(n), equals the task sequence, b(n − 2)a(n − 1)a(n). Thus, if a cue on (n) is the eliciting event, the short-term task probability for task a on the preceding two trials, (n − 2) and (n − 1), equals P(a) = 0.75. However, the succession of two task-set switches, i.e. s(n − 1)s(n), is equivalent to a(n − 2)b(n − 1)a(n), and a switch after a repetition, r(n − 1)s(n), equals the task sequence, b(n − 2)b(n − 1)a(n). Thus, if a cue on (n) is the eliciting event, the short-term task probability for task a on the preceding two trials (n − 2) and (n − 1) equals P(a) = 0.25. The confound between switch probability (P = 0.5) and short-term task probability (P(a(n − 2)(n − 1)/r(n)) = 0.75, P(a(n − 2)(n − 1)/s(n)) = 0.25) will affect amplitude variability in P3b (Squires et al., 1976; Kolossa et al., 2013).
The switch-related variability in P3b amplitude was more pronounced on transition cuing. This modulation may be related to the higher memory load which is associated with transition cues in comparison to task cues (Schneider and Logan, 2007). However, the P3b surprise hypothesis offers an alternative explanation for this result. Whereas switch probability was held constant across transition-cuing and task-cuing conditions, stimulus probability distinguished between the two conditions. Specifically, task cuing implies physically identical switch cues and repeat cues, their sole difference lying in their serial position within task runs (switch cues initiate new task runs, e.g., … bba … (switch cue underlined), whereas repeat cues do not, … aaa … (repeat cues underlined)). This is different if one considers stimuli on transition-cuing conditions, with physically different switch cues and repeat cues (labeled switch and repeat), e.g., … repeat repeat switch … (switch cue underlined). Given switch probabilities of P = 0.25, transition switch cues occurred less frequently than transition repeat cues. Thus, the sensory surprise over switch cues on transition-cuing conditions exceeds the sensory surprise over switch cues on task-cuing conditions, and it is well known that sensory surprise contributes to the switch-related variability in P3b amplitude (Nicholson et al., 2006; Jost et al., 2008; Periáñez and Barceló, 2009.
The use of Karayanidis et al. (2009) “switch-to” (equivalent to explicit task cues) vs. “switch away” (equivalent to transition cues) cuing may help to circumvent the sensory surprise confound when comparisons between switches under task cuing and switches under transition cuing are under scrutiny. In this paradigm, task cuing relies on mapping tasks with particular spatial positions. “Switch-to” cuing is mapped to the spatial position of one particular task (thereby unambiguously defining a task-set), whereas two viable tasks are cued by indicating spatial positions with overlapping task mappings in “switch away” cuing (thereby ambiguously defining two task sets).
Feedback-Related Amplitude Variability in P3a
One of the basic findings of the study was that the frontal P3a was evoked by feedback stimuli rather than by cue stimuli. The appearance of a feedback-locked P3a is a common denominator of prospectively-signaled and feedback-based task switching. These data are in agreement with earlier work in this area. It has repeatedly been reported that C/FB-events in WCST-like task-switching paradigms evoke prominent P3a waveforms (Barceló et al. (2002); Barceló, 2003; Kopp et al., 2005, 2006). However, Cunillera et al. (2012) recently reported that switch cues (C1-cues) and feedback events (FB1-events) evoked prominent P3a waveforms of similar amplitudes on a prospectively-signaled, three-task transition task-switching paradigm, seemingly contradicting our finding of specifically outcome-evoked amplitude variability in P3a. While the data converge with regard to the P3a evoked by FB1-events, they seem to diverge with regard to the P3a evoked by C1-cues. The contradictory pattern of results can be explained by the instrumental character of C1-cues in Cunillera et al.'s (2012) study in which they signaled the need for task-set updating, akin to the role of FB1:INC-events in both studies. Note that task-set switches are only one prerequisite for task-set updating; the need for task-set updating also emerges when an error occurred on the preceding trial. In contrast, C1-cues in our study indicated task-set switches, irrespective of foregone errors, as detailed above (cf. Figure 2). Thus, C1-cues provided information about task-set switches and/or foregone errors in Cunillera et al.'s study (i.e., they served as hybrid C/FB-stimuli). In contrast, C1-cues solely informed about task-set switches, irrespective of foregone errors, in the current study (i.e., they served as pure C-stimuli).
There were some differences between the experimental conditions which, apparently, exerted only minor effects on amplitude variability in P3a. First, the duration of the response-stimulus intervals differed between prospectively-signaled (one second) and feedback-based (three seconds) cuing conditions (cf. Figure 2). Second, the semantic framing of feedback-stimuli differed between prospectively-signaled (wrong, right) and feedback-based (switch, stay) cuing conditions. Future studies should keep these variables constant, or they should address their role by manipulating them systematically, despite the current evidence of their seemingly negligible role for amplitude variability in P3a.
The frontal P3a which was evoked by feedback stimuli on both cuing-contingency conditions distinguished certain from uncertain task switching such that solely uncertain switch outcomes elicited a prominent P3a. Specifically, the entropy over switch outcomes modulated the frontal P3a, such that uncertain switch outcomes (Pw = 0.5) elicited enhanced P3a amplitudes in comparison to certain switch outcomes (i.e., Pw = 1.0 and Pw = 0.0). Importantly, the P3a data thereby lend support to the P3a entropy hypothesis according to which modulations of P3a amplitudes are generally related to the entropy which is conveyed by eliciting stimuli (Barceló et al., 2006).
Neither the memory load hypothesis (Schneider and Logan, 2007) nor the contingency hypothesis (Kopp and Wessel, 2011) received support from the P3a results obtained in this study. Inspection of Figure 5 reveals that transition switch cues, but not task switch cues, evoked small enhancements of frontal P3a waves. However, the main effect of cue explicitness in the corresponding ANOVA is difficult to interpret, given the significant interaction between the number of tasks and cue explicitness. Further, the non-significance of cue explicitness effects on two-task conditions suggests that cue explicitness exerts rather marginal effects on P3a amplitude variability, and that they may be difficult to obtain (but see West et al., 2011). The contingency hypothesis (Kopp and Wessel, 2011) was incorrect insofar as it held that P3a amplitude enhancements should be restricted to C/FB-stimuli on feedback-based task switching. This prediction was clearly disconfirmed, since FB-stimuli on prospectively-signaled task switching evoked P3a waves of similar amplitude. This result indicates that the unique combination of task information and performance feedback by C/FB-stimuli is not necessary for obtaining a prominent P3a in response to switch outcome events. In conclusion, the main P3a findings of this study are that amplitude variability in P3a is (a) feedback-locked, and (b) related to the uncertainty of switch outcomes.
A Note on the Effects of Outcome Valence on ERP Waveforms
Amplitude variability in the feedback-related negativity (FRN) is a frequent subject in ERP studies of outcome processing and feedback-guided learning (see San Martín, 2012, for review). The FRN is a frontally distributed negative ERP component (around 250 ms after outcome presentation) which tends to be larger for negative than for positive outcomes (i.e., the outcome valence effect on amplitude variability in FRN). Holroyd and Coles (2002) proposed that the FRN is a scalp-recorded index of a neuronal system for reinforcement learning (RL; Sutton and Barto, 1998). However, inspection of Figure 5 reveals that outcome valence (which could only be analyzed in the two uncertain switching conditions) did obviously not evoke FRN-like amplitude variability in the current study.
In this study, a P3b, rather than a FRN, outcome valence effect was observed (with a larger P3b elicited by negative outcomes). San Martín (2012) concluded that there are inconsistent findings in the literature regarding the relationship between outcome valence and amplitude variability in P3b. We suggest that the effect of outcome valence on the P3b might result from another variable, since negative, but not positive, outcomes signal the need for additional task-set switches (see Table 2). Thus, negative outcomes, but not positive outcomes, serve as switch cues, and the apparent outcome valence effect may simply be another instance of switch-related amplitude variability in P3b.
Toward a Theoretical Integration of Uncertainty and Cognitive Control
The results of our study imply that switch-related P3b and P3a are dissociable, with regard to their scalp topography and to their antecedent conditions (P3b: cue-locked and modified by switch surprise, irrespective of switch entropy; P3a: feedback-locked and modified by switch entropy). These ERP data await comprehensive theoretical treatment, which is outlined below.
The theoretical fundament is provided by Sokolov's (1966) entropy model of the orienting response (OR), which analysed the dependence of the OR (a) on the statistical properties of the signals and (b) on their information content (see also Velden, 1974). Let et denote an event e at time t, P(et/Sj) be the probability of e at t in case of one of several hypothetical states Sj{with j = 1,…, n}, P(Sj/et) be the posterior probability of a particular state Sj given e at t, and P(Sj) be the prior probability of the state Sj. Then, according to Bayes' theorem
Thus, Sokolov's model is first of all a Bayesian model (Knill and Pouget, 2004; Doya et al., 2007; Friston, 2010; Vilares and Körding, 2011; Penny, 2012) of the OR. Further, the degree of uncertainty is determined by the entropy over the probability distribution of posterior probabilities of all hypothetical states, i.e.,
According to Sokolov (1966), the OR arises when the uncertainty of the situation, depending on the number of hypothetical states, reaches the threshold value, i.e., when H(Sj = 1…n/et) > threshold, and the OR lasts until the uncertainty is eliminated and H(Sj = 1… n/et) < threshold1. Further, Sokolov introduced the term “enquiries” to describe conditioned orienting reactions to information-carrying signals, i.e., a “selective concentration of attention at definite moments in time” (Sokolov, 1966, p.353). Conditioned orienting reactions are not evoked by the level of entropy as such (which is nevertheless necessarily required), “but its change anticipated at a given moment in time” (Sokolov, 1966, p.353).
Sokolov's (1966) entropy model of conditioned orienting reactions can be applied directly to uncertain task switching. Here, uncertain switch cues induce entropy, whereas uncertain switch outcomes eliminate entropy. Thus, viewed from the perspective of Sokolov's (1966) model, the elicitation of a P3a by uncertain switch outcomes is equivalent to a conditioned orienting reaction, an attentional focusing at that moment in time when an information-carrying (entropy-eliminating) signal was anticipated. In that context, it is of interest that the P3a component of the ERP has often been considered as indicating the brain's OR (e.g., Friedman et al., 2001; Barceló et al. (2002); Nieuwenhuis et al., 2011). Further, our analysis is very similar to Barcelü et al.'s (2006) P3a entropy hypothesis. However, a subtle difference between these two models lies in the fact that our model does not relate the P3a in uncertain task-switching paradigms to stimuli which convey entropy (i.e., uncertain switch cues), but rather to stimuli which eliminate entropy (i.e., uncertain switch outcomes).
Figure 7 shows a possible application of Sokolov's (1966) entropy model in the context of a hierarchical model of task control (Mayr et al., 2013), which specifies task-level and meta-level components. As an initial approximation, a simple actor-critic architecture (Sutton and Barto, 1998) is assumed at the task level (with P3b being related to the surprise over switches, FRN being related to negative outcomes). Perception of event e at time t (et) activates processing at the task level, and it simultaneously initiates processing in the multi-faceted orienting system (OS), which leads under two different conditions to an OR: (1) If et is entropy-inducing (ei) and unexpected, i.e., P(ei(t)) < α (with α → 0), under a non-entropic measure at time t, i.e., Ht ≤ γ. (2) If et is entropy-eliminating (ee) and expected, i.e., P(ee(t)) > β (with β → 1), under an entropic measure at delayed time t-1, i.e., Ht − 1 > γ. In case (1), a reactive orienting response (rOR) is evoked, whereas a proactive orienting response (pOR) is released in case (2). rORs and pORs may activate ORs by partly different neuronal mechanisms. Their final common pathway involves “selective concentration of attention at definite moments in time” (Sokolov, 1966, p.353), applied to et, and measurable as P3a.
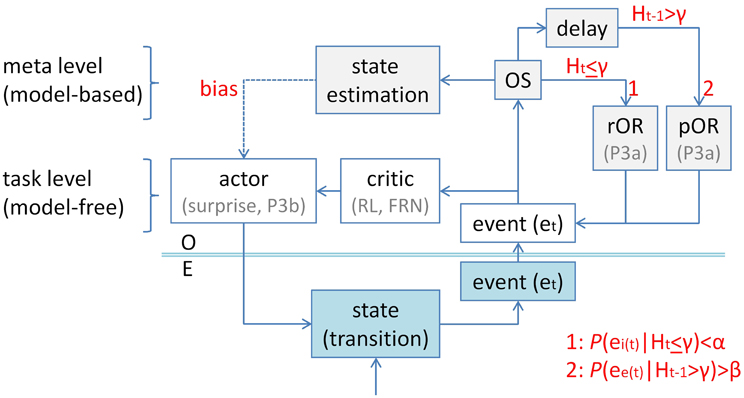
Figure 7. An application of Sokolov's (1966) entropy model of the orienting response to task switching. An actor-critic architecture (Sutton and Barto, 1998) is assumed at the task level (white frames; with P3b being related to the surprise over switches, FRN being related to negative outcomes). Perception of event e at time t (et) activates processing at the task level, and it initiates processing in the multi-faceted orienting system (OS; light gray frames), which leads under two different conditions to an orienting response (OR): (1) If et is entropy-inducing (ei) and unexpected, i.e., P(ei(t)) < α (with α → 0), under a non-entropic measure at time t, i.e., Ht < γ. (2) If et is entropy-eliminating (ee) and expected, i.e., P(ee(t)) > β (with β → 1), under an entropic measure at delayed time t-1, i.e., Ht − 1 > γ. In case (1), a reactive orienting response (rOR) is evoked, whereas a proactive orienting response (pOR) is released in case (2). Further, the OS serves as a switch from task control at the task level to task control at the meta level. One of the functions of meta-level processing is to estimate the current state of the environment from a number of hypothetical states, i.e., to dynamically model state transitions which occur in the environment (turquoise frames). Hence, the meta level is considered being “model-based,” whereas the task level is considered being “model-free.” Computationally, state estimation is equivalent to solving inverse problems. Once a state has been estimated, the meta level of task control is able to bias the actor toward adequate stimulus-response mappings. In its application to task switching, task control is exerted at the task level as long as Ht < γ (e.g., during task runs). When P(ei(t)) < α is not met, entropy-inducing switch cues fail to evoke a rOR. However, entropy-eliminating switch outcomes are expected to occur at time t, i.e., P(ee(t)) > β, under the delayed entropic measure, Ht − 1 > γ, which is a consequence of the occurrence of entropy-inducing switch cues at time t-1. Therefore, the uncertainty-related P3a qualifies as a pOR (also labeled conditioned orienting reaction, cf. Sokolov, 1966). O, organism; E, environment; RL, reinforcement learning; FRN, feedback-related negativity.
Further, the OS serves as a switch from task control at the task level to task control at the meta level (Nelson and Narens, 1990; Dosenbach et al., 2008; Tsujimoto et al., 2011; Fleming and Dolan, 2012; Kepecs and Mainen, 2012; Petersen and Posner, 2012; Yeung and Summerfield, 2012). One of the functions of meta-level processing is to estimate the current state of the environment from a number of hypothetical states, i.e., to dynamically model state transitions which occur in the environment. Hence, the meta level is considered being “model-based,” whereas the task level is considered being “model-free” (Johnson and Donchin, 1982; Daw et al., 2005; Behrens et al., 2007; Gläscher et al., 2010; Summerfield et al., 2011; Pearson and Platt, 2012). Computationally, state estimation is equivalent to solving inverse problems (Dayan et al., 1995; Friston, 2010; Kopp, 2012), putatively by Bayesian probability inversion (Sokolov, 1966; Kopp, 2008). Once a state has been estimated, the meta level of task control is able to bias the actor toward adequate stimulus-response mappings (Nelson and Narens, 1990).
In its application to task switching, task control is exerted at the task level as long as Ht ≤ γ (e.g., during task runs). When P(ei(t)) < α is not met, entropy-inducing switch cues fail to evoke a rOR. However, entropy-eliminating switch outcomes are expected to occur at time t, i.e., P(ee(t)) > β, under the delayed entropic measure, Ht − 1 > γ, which is a consequence of the occurrence of entropy-inducing switch cues at time t-1. Therefore, the uncertainty-related P3a qualifies as a pOR (also labeled conditioned orienting reaction, cf. Sokolov, 1966).
Shimamura (2008) mapped task-level and meta-level processing to distinct cortical regions. Specifically, he assigned task-level control to areas within the posterior cortex (PC), whereas meta-level control should be associated with areas within the prefrontal cortex (PFC). Meta-level cognitive processes, placed at the top of the cognitive hierarchy (Kopp, 2012), are expected to be supported by the anterior PFC, a region being a possible top level node of task control because progressively rostral PFC regions support progressively higher levels of the cognitive hierarchy (Koechlin et al., 2003; Badre, 2008; Badre et al., 2009, 2010).
The frontopolar cortex (FPC) is the most anterior part of the PFC. An understanding of FPC cognitive functions remains elusive, yet the FPC has been implicated in several cognitive functions, such as prospective memory (Simons et al., 2006), gating external and internal influences on cognition (Burgess et al., 2007), establishing task sets (Sakai, 2008), decision making (Soon et al., 2008) and evaluating outcomes of decisions (Ramnani et al., 2004; Boorman et al., 2009). Tsujimoto et al. (2010) reported single-cell activity in FPC, demonstrating that some FPC neurons encoded decisions when feedback approached, thereby suggesting a role of FPC in evaluating decisions. According to Fleming and co-workers, individual differences in structure (Fleming et al., 2010) and function (Fleming et al., 2012) of the right rostral PFC correlated with the accuracy of confidence reports in perceptual decision making paradigms. Fleming et al. suggested that this region of the anterior PFC re-represents decision uncertainty, to facilitate reportable confidence in task performance. Here we propose, albeit speculatively, that neuronal activity in the FPC contributes to amplitude variability in uncertainty-related P3a which in turn is associated with pOR-supported elimination of entropy over hypothetical states, as described above.
Conclusion
P3b and P3a waveforms represent separate electrophysiological markers of dissociable aspects of cognitive control in task-switching paradigms. Whereas parietal P3b waveforms are related to surprise over switches at the task level, frontally distributed P3a waveforms seem to be specifically related to the processing of uncertain switch outcomes at the meta level (functionally described as proactive orienting responses). P3a activities may computationally be related to the formation of inverse models of dynamic environments, as originally described by Sokolov (1966). One implication of the current research is the hypothesis that brain lesions affecting the OS lead to deficient contextual adjustment, a pervasive behavioral disturbance which represents one of the hallmarks of executive dysfunctions (Kopp, 2012).
Conflict of Interest Statement
The authors declare that the research was conducted in the absence of any commercial or financial relationships that could be construed as a potential conflict of interest.
Acknowledgments
This work was supported by the Deutsche Forschungsgemeinschaft (DFG) through an “Open Access Publikationsfonds” to the Medical School Hannover.
Footnotes
1 ^Sokolov (1966) used the labels Kj and Ai rather than et and Sj, respectively.
References
Alexander, W. H., and Brown, J. W. (2010). Computational models of performance monitoring and cognitive control. Top. Cogn. Sci. 2, 658–677. doi: 10.1111/j.1756-8765.2010.01085.x
Alexander, W. H., and Brown, J. W. (2011). Medial prefrontal cortex as an action-outcome predictor. Nat. Neurosci. 14, 1338–1344. doi: 10.1038/nn.2921
Army Individual Test Battery. (1944). Manual of Directions and Scoring. Washington, DC: War Department, Adjutant General's Office.
Astle, D. E., Jackson, G. M., and Swainson, R. (2006). Dissociating neural indices of dynamic cognitive control in advance task-set preparation: An ERP study of task switching. Brain Res. 1125, 94–103. doi: 10.1016/j.brainres.2006.09.092
Astle, D. E., Jackson, G. M., and Swainson, R. (2008). Fractionating the cognitive control required to bring about a change in task: a dense-sensor event-related potential study. J. Cogn. Neurosci. 20, 255–267. doi: 10.1162/jocn.2008.20015
Bach, D. R., and Dolan, R. J. (2012). Knowing how much you don't know: a neural organization of uncertainty estimates. Nat. Rev. Neurosci. 13, 572–586.
Badre, D. (2008). Cognitive control, hierarchy, and the rostro-caudal organization of the frontal lobes. Trends Cogn. Sci. 12, 193–200. doi: 10.1016/j.tics.2008.02.004
Badre, D., Doll, B. B., Long, N. M., and Frank, M. J. (2012). Rostrolateral prefrontal cortex and individual differences in uncertainty-driven exploration. Neuron 73, 595–607. doi: 10.1016/j.neuron.2011.12.025
Badre, D., Hoffman, J., Cooney, J. W., and D'Esposito, M. (2009). Hierarchical cognitive control deficits following damage to the human frontal lobe. Nat. Neurosci. 12, 515–522. doi: 10.1038/nn.2277
Badre, D., Kayser, A. S., and D'Esposito, M. (2010). Frontal cortex and the discovery of abstract action rules. Neuron 66, 315–326. doi: 10.1016/j.neuron.2010.03.025
Barceló, F. (2003). The Madrid card sorting test (MCST): a task switching paradigm to study executive attention with event-related potentials. Brain Res. Protoc. 11, 27–37. doi: 10.1016/S1385-299X(03)00013-8
Barceló, F., Escera, C., Corral, M. J., and Periáñez, J. A. (2006). Task switching and novelty processing activate a common neural network for cognitive control. J. Cogn. Neurosci. 18, 1734–1748. doi: 10.1162/jocn.2006.18.10.1734
Barceló, F., and Knight, R. T. (2002). Both random and perseverative errors underlie WCST deficits in prefrontal patients. Neuropsychologia 40, 349–356. doi: 10.1016/S0028-3932(01)00110-5
Barceló, F., Periáñez, J. A., and Knight, R. T. (2002). Think differently: a brain orienting response to task novelty. Neuroreport 13, 1887–1892. doi: 10.1097/00001756-200210280-00011
Behrens, T. E., Woolrich, M. W., Walton, M. E., and Rushworth, M. F. (2007). Learning the value of information in an uncertain world. Nat. Neurosci. 10, 1214–1221. doi: 10.1038/nn1954
Berg, E. A. (1948). A simple objective technique for measuring flexibility in thinking. J. Gen. Psychol. 39, 15–22. doi: 10.1080/00221309.1948.9918159
Boorman, E. D., Behrens, T. E., Woolrich, M. W., and Rushworth, M. F. (2009). How green is the grass on the other side. Frontopolar cortex and the evidence in favor of alternative courses of action. Neuron 62, 733–743. doi: 10.1016/j.neuron.2009.05.014
Burgess, P. W., Dumontheil, I., and Gilbert, S. J. (2007). The gateway hypothesis of rostral prefrontal cortex (area 10) function. Trends Cogn. Sci. 11, 290–298. doi: 10.1016/j.tics.2007.05.004
Cunillera, T., Fuentemilla, L., Periañez, J., Marco-Pallarès, J., Krämer, U. M., Càmara, E., et al. (2012). Brain oscillatory activity associated with task switching and feedback processing. Cogn. Affect. Behav. Neurosci. 12, 16–33. doi: 10.3758/s13415-011-0075-5
Daw, N. D., Niv, Y., and Dayan, P. (2005). Uncertainty-based competition between prefrontal and dorsolateral striatal systems for behavioral control. Nat. Neurosci. 8, 1704–1711. doi: 10.1038/nn1560
Dayan, P., Hinton, G. E., Neal, R. M., and Zemel, R. S. (1995). The Helmholtz machine. Neural Comput. 7, 889–904. doi: 10.1162/neco.1995.7.5.889
Demakis, G. J. (2003). A meta-analytic review of the sensitivity of the Wisconsin Card Sorting Test to frontal and lateralized frontal brain damage. Neuropsychology 17, 255–264. doi: 10.1037/0894-4105.17.2.255
Donchin, E. (1981). Surprise! … Surprise. Psychophysiology 18, 493–513. doi: 10.1111/j.1469-8986.1981.tb01815.x
Dosenbach, N. U., Fair, D. A., Cohen, A. L., Schlaggar, B. L., and Petersen, S. E. (2008). A dual-networks architecture of top-down control. Trends Cogn. Sci. 12, 99–105. doi: 10.1016/j.tics.2008.01.001
Doya, K., Ishii, S., Pouget, A., and Rao, R. P. (Eds.). (2007). Bayesian brain: Probabilistic Approaches to Neural Coding. Cambridge, MA: MIT press.
Duncan, C. C., Barry, R. J., Connolly, J. F., Fischer, C., Michie, P. T., Näätänen, R., et al. (2009). Event-related potentials in clinical research: guidelines for eliciting, recording, and quantifying mismatch negativity, P300, and N400. Clin. Neurophysiol. 120, 1883–1908. doi: 10.1016/j.clinph.2009.07.045
Fleming, S. M., and Dolan, R. J. (2012). The neural basis of metacognitive ability. Philos Trans. R. Soc. Lond. B Biol. Sci. 367, 1338–1349. doi: 10.1098/rstb.2011.0417
Fleming, S. M., Huijgen, J., and Dolan, R. J. (2012). Prefrontal contributions to metacognition in perceptual decision making. J. Neurosci. 32, 6117–6125. doi: 10.1523/JNEUROSCI.6489-11.2012
Fleming, S. M., Weil, R. S., Nagy, Z., Dolan, R. J., and Rees, G. (2010). Relating introspective accuracy to individual differences in brain structure. Science 329, 1541–1543. doi: 10.1126/science.1191883
Forstmann, B. U., Brass, M., Koch, I., and von Cramon, D. (2005). Internally generated and directly cued task sets: an investigation with fMRI. Neuropsychologia 43, 943–952. doi: 10.1016/j.neuropsychologia.2004.08.008
Friedman, D., Cycowicz, Y. M., and Gaeta, H. (2001). The novelty P3: an event-related brain potential (ERP) sign of the brain's evaluation of novelty. Neurosci. Biobehav. Rev. 25, 355–373. doi: 10.1016/S0149-7634(01)00019-7
Friston, K. (2010). The free-energy principle: a unified brain theory. Nat. Rev. Neurosci. 11, 127–138. doi: 10.1038/nrn2787
Gajewski, P. D., and Falkenstein, M. (2011). Diversity of the P3 in the task-switching paradigm. Brain Res. 1411, 87–97. doi: 10.1016/j.brainres.2011.07.010
Gehring, W. J., and Knight, R. T. (2000). Prefrontal-cingulate interactions in action monitoring. Nat. Neurosci. 3, 516–520. doi: 10.1038/74899
Gilbert, S. J., and Shallice, T. (2002). Task switching: a PDP model. Cogn. Psychol. 44, 297–337. doi: 10.1006/cogp.2001.0770
Gläscher, J., Daw, N., Dayan, P., and O'Doherty, J. P. (2010). States versus rewards: dissociable neural prediction error signals underlying model-based and model-free reinforcement learning. Neuron 66, 585–595. doi: 10.1016/j.neuron.2010.04.016
Grant, D. A., and Berg, E. A. (1948). A behavioral analysis of degree of impairment and ease of shifting to new responses in a Weigl-type card sorting problem. J. Exp. Psychol. 39, 404–411. doi: 10.1037/h0059831
Heaton, S. K., Chelune, G. J., Talley, J. L., Kay, G. G., and Curtiss, G. (1993). Wisconsin Card Sorting Test Manual: Revised and Expanded. Odessa, FL: Psychological Assessment Resources.
Holroyd, C. B., and Coles, M. G. (2002). The neural basis of human error processing: reinforcement learning, dopamine, and the error-related negativity. Psychol. Rev. 109, 679–709. doi: 10.1037/0033-295X.109.4.679
Johnson, R. Jr., and Donchin, E. (1982). Sequential expectancies and decision making in a changing environment: an electrophysiological approach. Psychophysiology 19, 183–200. doi: 10.1111/j.1469-8986.1982.tb02545.x
Jost, K., Mayr, U., and Rösler, F. (2008). Is task switching nothing but cue priming. Evidence from ERPs. Cogn. Affect. Behav. Neurosci. 8, 74–84. doi: 10.3758/CABN.8.1.74
Karayanidis, F., Coltheart, M., Michie, P. T., and Murphy, K. (2003). Electrophysiological correlates of anticipatory and poststimulus components of task switching. Psychophysiology 40, 329–348. doi: 10.1111/1469-8986.00037
Karayanidis, F., Jamadar, S., Ruge, H., Phillips, N., Heathcote, A., and Forstmann, B. U. (2010). Advance preparation in task-switching: converging evidence from behavioral, brain activation, and model-based approaches. Front. Psychol. 1:25. doi: 10.3389/fpsyg.2010.00025
Karayanidis, F., Mansfield, E. L., Galloway, K. L., Smith, J. L., Provost, A., and Heathcote, A. (2009). Anticipatory reconfiguration elicited by fully and partially informative cues that validly predict a switch in task. Cogn. Affect. Behav. Neurosci. 9, 202–215. doi: 10.3758/CABN.9.2.202
Kepecs, A., and Mainen, Z. F. (2012). A computational framework for the study of confidence in humans and animals. Philos. Trans. R. Soc. Lond. B Biol. Sci. 367, 1322–1337. doi: 10.1098/rstb.2012.0037
Kieffaber, P. D., and Hetrick, W. P. (2005). Event-related potential correlates of task switching and switch costs. Psychophysiology 42, 56–71. doi: 10.1111/j.1469-8986.2005.00262.x
Kiesel, A., Steinhauser, M., Wendt, M., Falkenstein, M., Jost, K., Philipp, A. M., et al. (2010). Control and interference in task switching—a review. Psychol. Bull. 136, 849–874. doi: 10.1037/a0019842
Knill, D. C., and Pouget, A. (2004). The Bayesian brain: the role of uncertainty in neural coding and computation. Trends Neurosci. 27, 712–719. doi: 10.1016/j.tins.2004.10.007
Koechlin, E., Ody, C., and Kouneiher, F. (2003). The architecture of cognitive control in the human prefrontal cortex. Science 302, 1181–1185. doi: 10.1126/science.1088545
Kolossa, A., Fingscheidt, T., Wessel, K., and Kopp, B. (2013). A model-based approach to trial-by-trial P300 amplitude fluctuations. Front. Hum. Neurosci. 6:359. doi: 10.3389/fnhum.2012.00359
Kopp, B. (2008). “The P300 component of the event-related brain potential and Bayes' theorem,” in Cognitive Sciences at the Leading Edge, ed M. K. Sun (New York, NY: Nova Science Publishers), 87–96.
Kopp, B. (2012). A simple hypothesis of executive function. Front. Hum. Neurosci. 6:159. doi: 10.3389/fnhum.2012.00159
Kopp, B., Moschner, C., and Wessel, K. (2005). Ereigniskorrelierte Potenziale und kognitive Flexibilität. [Event-related brain potentials and cognitive flexibility.] Klin. Neurophysiol. 36, 75–85. doi: 10.1055/s-2005-866868
Kopp, B., Tabeling, S., Moschner, C., and Wessel, K. (2006). Fractionating the neural mechanisms of cognitive control. J. Cogn. Neurosci. 18, 949–965. doi: 10.1162/jocn.2006.18.6.949
Kopp, B., and Wessel, K. (2011). Dissociating proactively and retroactively cued task switching: a route towards neuropsychological analyses of cognitive control. Neuropsychol. Trends 10, 55–70.
Logan, G. D., and Bundesen, C. (2003). Clever homunculus: is there an endogenous act of control in the explicit task-cuing procedure. J. Exp. Psychol. Hum. Percept. Perform. 29, 575–599. doi: 10.1037/0096-1523.29.3.575
Mayr, U., Kuhns, D., and Rieter, M. (2013). Eye movements reveal dynamics of task control. J. Exp. Psychol. Gen. 142, 489–509. doi: 10.1037/a0029353
Milner, B. (1963). Effects of different brain lesions on card sorting: the role of the frontal lobes. Arch. Neurol. 9, 90–100. doi: 10.1001/archneur.1963.00460070100010
Monsell, S. (2003). Task switching. Trends Cogn. Sci. 7, 134–140. doi: 10.1016/S1364-6613(03)00028-7
Monsell, S., and Mizon, G. A. (2006). Can the task-cuing paradigm measure an endogenous task-set reconfiguration process. J. Exp. Psychol. Hum. Percept. Perform. 32, 493–516. doi: 10.1037/0096-1523.32.3.493
Nelson, H. E. (1976). A modified card sorting test sensitive to frontal lobe defects. Cortex 12, 313–324. doi: 10.1016/S0010-9452(76)80035-4
Nelson, T. O., and Narens, L. (1990). “Metamemory: A theoretical framework and some new findings,” in The Psychology of Learning and Motivation, Vol. 26, ed G. H. Bower (New York, NY: Academic Press), 125–173.
Nessler, D., Friedman, D., and Johnson, R. (2012). A new account of the effect of probability on task switching: ERP evidence following the manipulation of switch probability, cue informativeness and predictability. Biol. Psychol. 91, 245–262. doi: 10.1016/j.biopsycho.2012.07.005
Nicholson, R., Karayanidis, F., Bumak, E., Poboka, D., and Michie, P. T. (2006). ERPs dissociate the effects of switching task sets and task cues. Brain Res. 1095, 107–123. doi: 10.1016/j.brainres.2006.04.016
Nicholson, R., Karayanidis, F., Poboka, D., Heathcote, A., and Michie, P. T. (2005). Electrophysiological correlates of anticipatory task-switching processes. Psychophysiology 42, 540–554.
Nieuwenhuis, S., De Geus, E. J., and Aston-Jones, G. (2011). The anatomical and functional relationship between the P3 and autonomic components of the orienting response. Psychophysiology 48, 162–175. doi: 10.1111/j.1469-8986.2010.01057.x
Nyhus, E., and Barceló, F. (2009). The Wisconsin Card Sorting Test and the cognitive assessment of prefrontal executive functions: a critical update. Brain Cogn. 71, 437–451. doi: 10.1016/j.bandc.2009.03.005
Pearson, J., and Platt, M. L. (2012). Dynamic decision making in the brain. Nat. Neurosci. 15, 341–342. doi: 10.1038/nn.3049
Penny, W. (2012). Bayesian models of brain and behaviour. ISRN Biomath. 2012:785791. doi: 10.5402/2012/785791
Periáñez, J. A., and Barceló, F. (2009). Updating sensory versus task representations during task-switching: Insights from cognitive brain potentials in humans. Neuropsychologia 47, 1160–1172. doi: 10.1016/j.neuropsychologia.2009.01.014
Petersen, S. E., and Posner, M. I. (2012). The attention system of the human brain: 20 years after. Annu. Rev. Neurosci. 35, 73–89. doi: 10.1146/annurev-neuro-062111-150525
Picton, T. W., Bentin, S., Berg, P., Donchin, E., Hillyard, S. A., Johnson, et al. (2000). Guidelines for using human event-related potentials to study cognition: recording standards and publication criteria. Psychophysiology 37, 127–152. doi: 10.1111/1469-8986.3720127
Polich, J. (2007). Updating P300: an integrative theory of P3a and P3b. Clin. Neurophysiol. 118, 2128–2148. doi: 10.1016/j.clinph.2007.04.019
Rabbitt, P. M. A. (1966). Errors and error-correction in choice-response tasks. J. Exp. Psychol. 71, 264–272. doi: 10.1037/h0022853
Ramnani, N., Elliott, R., Athwal, B. S., and Passingham, R. E. (2004). Prediction error for free monetary reward in the human prefrontal cortex. Neuroimage 23, 777–786. doi: 10.1016/j.neuroimage.2004.07.028
Ridderinkhof, K. R., Ullsperger, M., Crone, E. A., and Nieuwenhuis, S. (2004). The role of the medial frontal cortex in cognitive control. Science 306, 443–447. doi: 10.1126/science.1100301
Rushworth, M. F., Buckley, M. J., Gough, P. M., Alexander, I. H., Kyriazis, D., McDonald, K. R., et al. (2005). Attentional selection and action selection in the ventral and orbital prefrontal cortex. J. Neurosci. 25, 11628–11636. doi: 10.1523/JNEUROSCI.2765-05.2005
Rushworth, M. F., Hadland, K. A., Paus, T., and Sipila, P. K. (2002). Role of the human medial frontal cortex in task switching: a combined fMRI and TMS study. J. Neurophysiol. 87, 2577–2592.
Sakai, K. (2008). Task set and prefrontal cortex. Annu. Rev. Neurosci. 31, 219–245. doi: 10.1146/annurev.neuro.31.060407.125642
San Martín, R. (2012). Event-related potential studies of outcome processing and feedback-guided learning. Front. Hum. Neurosci. 6:304. doi: 10.3389/fnhum.2012.00304
Schneider, D. W., and Logan, G. D. (2007). Task switching versus cue switching: using transition cuing to disentangle sequential effects in task-switching performance. J. Exp. Psychol. Learn. 33, 370–378. doi: 10.1037/0278-7393.33.2.370
Shallice, T., Stuss, D. T., Picton, T. W., Alexander, M. P., and Gillingham, S. (2008). Mapping task switching in frontal cortex through neuropsychological group studies. Front. Neurosci. 2, 79–85. doi: 10.3389/neuro.01.013.2008
Shannon, C. E., and Weaver, W. (1948). The Mathematical Theory of Communication. Urbana, IL: University of Illinois Press.
Shimamura, A. P. (2008). “A Neurocognitive Approach to Metacognitive Monitoring and Control,” in Handbook of Memory and Metacognition, eds J. Dunlosky and R. Bjork (Mahwah, NJ: Psychology Press), 373–390.
Simons, J. S., Schölvinck, M. L., Gilbert, S. J., Frith, C. D., and Burgess, P. W. (2006). Differential components of prospective memory. Evidence from fMRI. Neuropsychologia 44, 1388–1397. doi: 10.1016/j.neuropsychologia.2006.01.005
Sokolov, Y. N. (1966). “Orienting reflex as information regulator,” in Psychological Research in the U.S.S.R., eds A. N. Leontiev, A. R. Luria, and A. A. Smirnov (Moscow: Progress Publishers), 334–360.
Soon, C. S., Brass, M., Heinze, H. J., and Haynes, J. D. (2008). Unconscious determinants of free decisions in the human brain. Nat. Neurosci. 11, 543–545. doi: 10.1038/nn.2112
Squires, K. C., Wickens, C., Squires, N. K., and Donchin, E. (1976). The effect of stimulus sequence on the waveform of the cortical event-related potential. Science 193, 1142–1146. doi: 10.1126/science.959831
Summerfield, C., Behrens, T. E., and Koechlin, E. (2011). Perceptual classification in a rapidly changing environment. Neuron 71, 725–736. doi: 10.1016/j.neuron.2011.06.022
Sutton, R. S., and Barto, A. G. (1998). Reinforcement Learning: An Introduction. Cambridge, MA: MIT press.
Swainson, R., Jackson, S. R., and Jackson, G. M. (2006). Using advance information in dynamic cognitive control: an ERP study of task-switching. Brain Res. 1105, 61–72. doi: 10.1016/j.brainres.2006.02.027
Travers, S., and West, R. (2008). Neural correlates of cue retrieval, task set reconfiguration, and rule mapping in the explicit cue task switching paradigm. Psychophysiology 45, 588–601. doi: 10.1111/j.1469-8986.2008.00658.x
Tsujimoto, S., Genovesio, A., and Wise, S. P. (2010). Evaluating self-generated decisions in frontal pole cortex of monkeys. Nat. Neurosci. 13, 120–126. doi: 10.1038/nn.2453
Tsujimoto, S., Genovesio, A., and Wise, S. P. (2011). Frontal pole cortex: encoding ends at the end of the endbrain. Trends Cogn. Sci. 15, 169–176. doi: 10.1016/j.tics.2011.02.001
Ullsperger, M., von Cramon, D. Y., and Müller, N. G. (2002). Interactions of focal cortical lesions with error processing: evidence from event-related brain potentials. Neuropsychology 16, 548–561. doi: 10.1037/0894-4105.16.4.548
Velden, M. (1974). An empirical test of Sokolov's entropy model of the orienting response. Psychophysiology 11, 682–691. doi: 10.1111/j.1469-8986.1974.tb01137.x
Vilares, I., and Körding, K. (2011). Bayesian models: the structure of the world, uncertainty, behavior, and the brain. Ann. N.Y. Acad. Sci. 1224, 22–39. doi: 10.1111/j.1749-6632.2011.05965.x
Wessel, J. R., Danielmeier, C., Morton, J. B., and Ullsperger, M. (2012). Surprise and error: common neuronal architecture for the processing of errors and novelty. J. Neurosci. 32, 7528–7537. doi: 10.1523/JNEUROSCI.6352-11.2012
West, R., Langley, M. M., and Bailey, K. (2011). Signaling a switch: neural correlates of task switching guided by task cues and transition cues. Psychophysiology 48, 612–623. doi: 10.1111/j.1469-8986.2010.01123.x
Wylie, G. R., Murray, M. M., Javitt, D. C., and Foxe, J. J. (2009). Distinct neurophysiological mechanisms mediate mixing costs and switch costs. J. Cogn. Neurosci. 21, 105–118. doi: 10.1162/jocn.2009.21009
Keywords: event-related potentials, P3b, P3a, task-switching, uncertainty, surprise, entropy, orienting response
Citation: Kopp B and Lange F (2013) Electrophysiological indicators of surprise and entropy in dynamic task-switching environments. Front. Hum. Neurosci. 7:300. doi: 10.3389/fnhum.2013.00300
Received: 06 December 2012; Accepted: 05 June 2013;
Published online: 03 July 2013.
Edited by:
John J. Foxe, Albert Einstein College of Medicine, USAReviewed by:
Francisco Barcelo, University of Illes Balears, SpainFrini Karayanidis, University of Newcastle, Australia
Copyright © 2013 Kopp and Lange. This is an open-access article distributed under the terms of the Creative Commons Attribution License, which permits use, distribution and reproduction in other forums, provided the original authors and source are credited and subject to any copyright notices concerning any third-party graphics etc.
*Correspondence: Bruno Kopp, Department of Neurology, Hannover Medical School, Carl-Neuberg-Str. 1, 30625 Hannover, Germany e-mail: kopp.bruno@mh-hannover.de