- 1Centre for Neurocognitive Research and Department of Psychology, University of Salzburg, Salzburg, Austria
- 2Neuroscience Institute and Centre for Neurocognitive Research, Christian-Doppler-Klinik, Paracelsus Medical University Salzburg, Salzburg, Austria
- 3Department of Psychiatry and Psychotherapy II, Christian-Doppler-Klinik, Paracelsus Medical University Salzburg, Salzburg, Austria
- 4Department of Addictive Behavior and Addiction Medicine, Central Institute of Mental Health, University of Heidelberg, Mannheim, Germany
Functional neuroimaging studies of pathological gambling (PG) demonstrate alterations in frontal and subcortical regions of the mesolimbic reward system. However, most investigations were performed using tasks involving reward processing or executive functions. Little is known about brain network abnormalities during task-free resting state in PG. In the present study, graph-theoretical methods were used to investigate network properties of resting state functional magnetic resonance imaging data in PG. We compared 19 patients with PG to 19 healthy controls (HCs) using the Graph Analysis Toolbox (GAT). None of the examined global metrics differed between groups. At the nodal level, pathological gambler showed a reduced clustering coefficient in the left paracingulate cortex and the left juxtapositional lobe (supplementary motor area, SMA), reduced local efficiency in the left SMA, as well as an increased node betweenness for the left and right paracingulate cortex and the left SMA. At an uncorrected threshold level, the node betweenness in the left inferior frontal gyrus was decreased and increased in the caudate. Additionally, increased functional connectivity between fronto-striatal regions and within frontal regions has also been found for the gambling patients. These findings suggest that regions associated with the reward system demonstrate reduced segregation but enhanced integration while regions associated with executive functions demonstrate reduced integration. The present study makes evident that PG is also associated with abnormalities in the topological network structure of the brain during rest. Since alterations in PG cannot be explained by direct effects of abused substances on the brain, these findings will be of relevance for understanding functional connectivity in other addictive disorders.
Introduction
Patients suffering from pathological gambling (PG) show persistent gambling behavior despite negative consequences resulting in a wide-range of psychosocial impairments (Goudriaan et al., 2004). PG is classified as an impulse control disorder in DSM-IV (American Psychiatric Association, 2000), but is increasingly conceptualized as a behavioral addiction with striking similarities to substance addictions such as withdrawal symptoms and signs of tolerance (Petry, 2007). Therefore, PG (besides being renamed as disordered gambling) has been reclassified under the chapter “Addiction and related disorders” (together with substance addictions) in DSM 5 (American Psychiatric Association, 2013; Petry et al., 2013).
Most functional neuroimaging studies in PG up to date have examined brain activity abnormalities using paradigms such as reward processing, reactivity to gambling related cues, learning, decision making, and executive functions (for reviews, see Potenza, 2008, 2013; van Holst et al., 2010). In line with brain imaging studies on substance addiction, activation abnormalities in regions of the mesolimbic reward system (mainly in orbitofrontal, medial and lateral prefrontal regions, and the ventral striatum) were consistently found in patients with PG (Cavedini et al., 2002; Potenza et al., 2003; Reuter et al., 2005; Tanabe et al., 2007; Balodis et al., 2012; Choi et al., 2012; Miedl et al., 2012; van Holst et al., 2012a; Hudgens-Haney et al., 2013; Limbrick-Oldfield et al., 2013).
Brain activation differences in fronto-striatal regions in PG have also been found in executive function tasks and been commonly interpreted as reflecting impairments in cognitive control and inhibitory functions (Potenza et al., 2003) which contribute to maladaptive decision making in PG, comparable to such impairments in substance addiction (Tanabe et al., 2007).
Recent interest in functional neuroimaging studies on neuropsychiatric disorders has focused on analyzing resting state functional connectivity (Fox and Greicius, 2010; van den Heuvel and Hulshoff Pol, 2010; Menon, 2011; Xia and He, 2011; Buckholtz and Meyer-Lindenberg, 2012; Yu et al., 2012). Compared to task based studies, resting state data is easier to obtain and does not have to deal with group differences in task performance and compliance. Resting state connectivity studies have revealed abnormalities in a wide range of neuropsychiatric disorders such as depression, schizophrenia, attention-deficit hyperactivity disorder (ADHD), and Alzheimer’s disease (for review, see Greicius, 2008).
Resting state functional magnetic resonance imaging (fMRI) data can also be used to analyze topological network properties of the brain using graph-theoretical approaches (He and Evans, 2010; Bullmore and Sporns, 2012). These approaches provide important information on the architecture of brain networks. Small-world networks are characterized by dense local interconnectivity and short path length linking individual network nodes in a short and efficient way (e.g., brain regions based on a parcellation atlas; Bullmore and Sporns, 2009). Short pathways between one node and any other node as well as a high density of connections between nearest neighbors are necessary for efficient segregation and functional integration (Salvador et al., 2005; Achard et al., 2006; Bassett and Bullmore, 2009). Network graphs are based on structural or functional data and quantify the structural and functional organization of the brain (Stam and Reijneveld, 2007).
Studies have shown that the small-world architecture and topological network properties of the brain exhibit abnormalities in neuropsychiatric disorders (e.g., He et al., 2009; Lynall et al., 2010; Zhang et al., 2011a; Cocchi et al., 2012; Cisler et al., 2013; for a review, see Xia and He, 2011). For example, patient with schizophrenia show lower cortical integration (lower amount of connections, longer path lengths, and lower clustering coefficients) in the frontal, parietal, and temporal pole (Liu et al., 2009). Zhang et al. (2011a) found global integration differences between HCs and patients with major depressive disorder and differences in nodal centrality for frontal areas, and regions of the default-mode network as well as for subcortical regions like the caudate. Furthermore, patients with obsessive-compulsive disorder (OCD) show altered functional connectivity and small worldness-properties (Zhang et al., 2011b). OCD patients demonstrate higher local clustering in the brain’s cognitive control network (posterior temporal regions and the cingulate cortex). Differences in brain topology are also reported for young adults with ADHD (Cocchi et al., 2012). Functional segregation of the orbitofrontal cortex in the intrinsic brain network is enhanced in ADHD which can be linked to attentional and perceptual control deficits. Both approaches demonstrate how network analyses of the brain identify alternations directly related to symptoms of the specific mental disorder.
To date, comparatively less is known about resting state functional connectivity in addictive disorders (for review, see Sutherland et al., 2012). For example, a resting state fMRI study in chronic heroin addicts found increased functional connectivity of mesolimbic pathways and decreased functional connectivity between frontal areas (Ma et al., 2010). Two studies using graph-theoretical approaches reported differences in global small-world properties and an increased degree in a number of medial frontal, frontal, and subcortical regions in chronic abstinent heroin addicts (Liu et al., 2009; Yuan et al., 2010). These studies suggest that topological network properties may provide important insights in functional brain abnormalities in addiction. However, both of these studies on small-world properties in addiction had a relatively small sample size (11 patients in Liu et al., 2009 and 12 patients in Yuan et al., 2010). Furthermore, in studies investigating substance addiction, results may also partly reflect the effects of the abused substance on brain structure and function (Clark and Limbrick-Oldfield, 2013).
To our knowledge, not one single study on resting brain connectivity and especially on topological network properties has been conducted in PG. Two recent reports of white matter microstructural abnormalities in PG suggest that brain connectivity and network organization may be affected in PG (Joutsa et al., 2011; Yip et al., 2013). Two studies in internet addiction report functional connectivity abnormalities (Ding et al., 2013; Hong et al., 2013). Ding et al. (2013) report differences between controls and internet addicts in functional connectivity between a part of the default mode network, that is, the posterior cingulate cortex (PCC), and regions in the cerebellum, the inferior parietal lobule, and the middle temporal gyrus. Hong et al. (2013) report decreased connectivity in internet addiction between a number of cortical and subcortical regions but no significant group differences in topological network properties. The authors point out that the low number of participants (11 addicted adolescents and 11 matched HCs) could be a reason for the absence of statistically significant differences in network properties.
The aim of the present study is to provide first evidence for alterations in topological network properties using resting state fMRI and gain further insights on the neural correlates of this disorder and addictive disorders in general.
Materials and Methods
Subjects
This study has been approved by the local ethics committee. Nineteen patients with PG and 19 age-matched HCs with no history of neurological or psychiatric disorders participated in this study. Written informed consent was provided by all participants. All patients were seeking treatment and have been recruited at the Pathological Gambling out-patient clinic at the Department of Psychiatry and Psychotherapy II. Control subjects were recruited via advertisements and mailings.
Behavioral Assessment
The German version of the short questionnaire on gambling behavior (Kurzfragebogen zum Glücksspielverhalten – KFG; Petry, 1996) and The South Oaks Gambling Screen (SOGS) by Lesieur and Blume (1987) were used to quantify gambling behavior. Furthermore, all participants completed the Alcohol Use Disorders Identification Test (AUDIT; Babor et al., 2006), the Fagerstrom Test for Nicotine Dependence (FTND; Fagerstrom, 1978), the Behavioral Inhibition Scale (BIS; Carver and White, 1994), and the Beck Depression Inventory (BDI; Beck et al., 1996).
fMRI Data Acquisition Preprocessing
Resting state fMRI was performed with a 3 Tesla Siemens Tim Trio MRI using a 32-channel head coil. All participants were asked to quietly rest in the scanner with their eyes closed and not to think of anything specific. Two-hundred and fifty T2*-weighted images were acquired (including six dummy scans which were discarded) with a gradient echo-planar imaging sequences with the following parameters: TR: 2.25 s; TE: 30 ms; flip angle: 78°; field of view (FOV): 192 mm × 192 mm; matrix size: 64 × 64; 36 slices; slice thickness: 3 mm; slice gap 0.3 mm; voxel size: 3 mm × 3 mm × 3 mm. Additionally, a high-resolution structural scan (sagittal T1-weighted MPRAGE sequence; TR: 2300 ms; TE: 2.91 ms; voxel size: 1 mm × 1 mm × 1.2 mm; slice thickness: 1.20 mm; FOV: 356 mm × 356 mm; 160 slices; flip angle: 9°) and fieldmaps were obtained from each participant.
Functional magnetic resonance imaging data were preprocessed using Statistical Parametrical Mapping (SPM 8, Wellcome Department of Imaging Neuroscience, London, UK1). The following procedures were included: realignment and unwarping to compensate for movement-related artifacts; slice timing correction; co-registration of the EPI scans to the skull-stripped T1-weighted structural scan; normalization to standard stereotaxic anatomical Montreal Neurological Institute (MNI) space; smoothing with 6 mm full-width at half-maximum (FWHM) Gaussian kernel; voxel size was resampled to isotropic 3 mm × 3 mm × 3 mm.
To address the problem of confounds due to small head motion which may influence resting state connectivity, we ensured that all data sets did not exhibit movements larger than 3 mm for translations or 3° for rotations. Movement parameters were compared between patients and HCs using two-tailed t-tests. There are no significant differences in any of the six movement parameters (all ts < 1, all ps > 0.3).
For further analyses, noise correction and filtering with a bandpass filter between 0.01 and 0.1 Hz was performed with the conn toolbox (Whitfield-Gabrieli and Nieto-Castanon, 2012). For noise correction all six movement parameters and the first derivative of the time-series were removed from the data by regression. For further noise reduction, noise signals were estimated from white matter and CSF signal and removed from the data with the CompCor method (Behzadi et al., 2007) as implemented in the conn toolbox. These noise removal steps have been shown to substantially reduce noise from non-neural sources and increase the sensitivity and reliability of functional connectivity analysis (Whitfield-Gabrieli and Nieto-Castanon, 2012). No global signal regression was performed as it may result in lower reproducibility of network metrics (see Telesford et al., 2013).
Network Construction
The Harvard–Oxford Atlas was used to extract the preprocessed fMRI data from 48 left and 48 right hemisphere cortical regions, as well as from seven left and seven right subcortical regions. Time-series of the low-frequency BOLD signal were extracted for each of the 110 regions and averaged over all voxels in each node. For each subject, the time-series of all 110 regions were correlated with each other to create an undirected and weighted correlation matrix using Pearson correlation. These steps were performed with the conn toolbox. In contrast to partial correlation, the Pearson correlation coefficient is gaining higher values of reproducibility (see Telesford et al., 2013). In this network, each region represents a node with the correlation coefficients of the time-series between the different regions defining the edges resulting in a 110 × 110 connectivity matrix.
Graph Analyses
Analyses of network properties were performed with the GAT2 (Hosseini et al., 2012), which uses routines of the Brain Connectivity Toolbox for network metrics calculation (Rubinov and Sporns, 2010).
Threshold Selection
To make groups comparable, we ensured that all graphs had the same number of edges by applying an individual threshold to each correlation matrix. This was done by calculating the ratio of the number of actual connections divided by the maximum number of all possible connections described as the so-called cost of the network (connection density). Since there is still no consensus of the best threshold to be chosen, a wide range of threshold values were applied in this study (0.11 ≤ T ≤ 0.55 with an increment of 0.02). To verify that the selection of the threshold range is not too wide which may produce disconnected nodes and networks without small worldness features on either ends of the range, we ensured that all subjects (a) had an averaged degree value of 2*log(N) with N = number of nodes and (b) showed network properties of small worldness with σ > 1.1 in all threshold values (Zhang et al., 2011a).
Network Metrics
For each threshold, the following global metrics were calculated: characteristic path length (L); the average of the clustering coefficient (C); global efficiency (Eglob); small worldness (σ); additionally, the following local metrics were calculated for each threshold: degree (k); local efficiency (Eloc); node betweenness (Nbc); clustering coefficient (C).
The degree describes the number of edges linking one node to the rest of the network and gives information on how functionally connected a network is. The clustering coefficient is a measure of degree to which nodes in a graph are forming a cluster. The characteristic path length describes the number of edges between one node and any other node in a network giving an overview of the effectiveness of information transfer. The global efficiency is inversely related to the characteristic path length. The local efficiency is computed on node neighborhoods and is related to the clustering coefficient reflecting the efficiency of parallel information transfer, robustness, and fault tolerance of a network. Compared to the clustering coefficient and the characteristic path length, measures of efficiency have the advantage of including disconnected nodes with a value of 0 while the former remove them from the analysis, and therefore, may falsify the results when disconnected nodes are present (Achard and Bullmore, 2007). The node betweenness is a measure of centrality and specifies the fraction of all shortest pathways in a network that contain a given node. The so-called small worldness is the ratio of the averaged and normalized clustering coefficient (γ) to the normalized characteristic path length (λ) and assesses the small-world properties of a network characterized by high clustering coefficient and a low characteristic path length. Small-worldness properties of a network are usually given when sigma (σ) is greater than 1.
All metrics were compared with the corresponding values obtained and averaged from 20 random networks with the same number of nodes, total edges, and degree distribution resulting in, for example, γ = C/Crand and λ = L/Lrand (Maslov and Sneppen, 2002).
Statistical Analyses
Group comparisons of the metrics were conducted with permutation tests implemented in the GAT toolbox using the area under the curve (AUC) calculated over the threshold for each metric (Bruno et al., 2012; Hosseini et al., 2012; Singh et al., 2013). All results were corrected for multiple comparisons using a false positive correction, p < 1/N (Alexander-Bloch et al., 2010). All p-values corrected for multiple comparisons have been transformed and are reported as pcor.
Since this study is an exploratory study and the first in PG using graph theoretical approaches to assess network properties in resting state data, we also report significant results with uncorrected p-values.
To examine possible alterations of functional connectivity strength between regions, the correlation values of all regions were compared between both groups to find significant differences in connectivity. Analyses of functional connectivity were performed with the conn toolbox and corrected for multiple comparisons using an FDR-threshold, p < 0.05.
Results
Sample Characteristics
Sample characteristics are shown in Table 1. No statistically significant group differences were found for sex ratio, years of education, or age. Furthermore, PG patients were comparable to HCs with respect to tobacco and alcohol consumption as assessed by the FTND and the AUDIT.
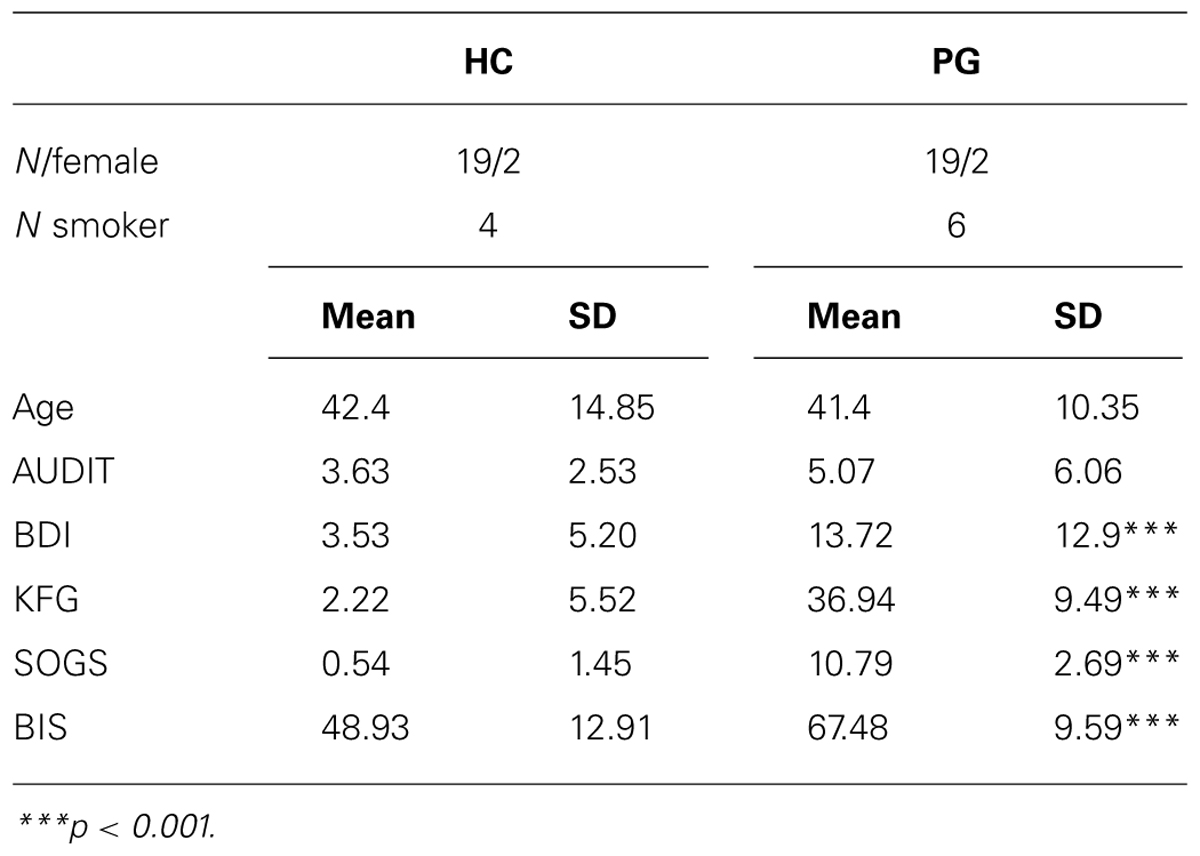
TABLE 1. Sample characteristics and group differences for healthy controls (HCs) and pathological gamblers (PG) in all questionnaires.
Large group differences were found in gambling behavior (KFG; SOGS). PG patients also demonstrated a larger number of depressive symptoms as measured by the BDI and higher impulsivity as measured by the BIS.
Global Metrics
Both groups showed small worldness properties with σ > 1 and there were no significant differences between groups (p = 0.845). Compared to random networks, both groups showed a higher averaged clustering coefficient (γ > 1) and similar values for the characteristic path length (λ ~ 1). None of the global metrics differed between patients and controls (Eglob: p = 0.646; λ: p = 0.797; γ: p = 0.817). Results for all global metrics are displayed in Figure 1.
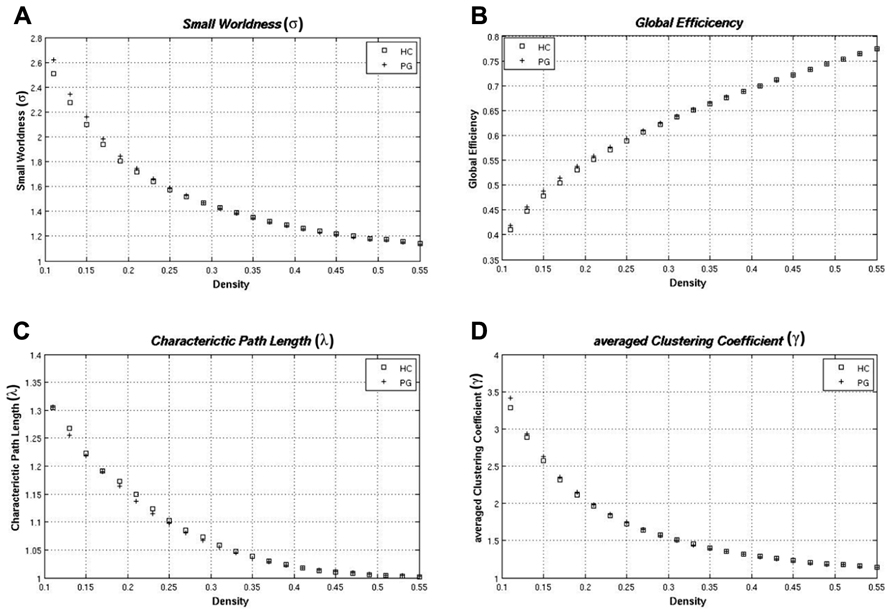
FIGURE 1. Global metrics for pathological gamblers (PG) and healthy controls (HC) in all density thresholds: (A) small worldness (σ); (B) global efficiency; (C) characteristic path length (λ); (D) averaged clustering coefficient (γ).
Nodal Metrics
At the corrected significance threshold, differences in nodal metrics were found in medial frontal regions. As can be seen in Figure 2, patients with PG demonstrated a decreased clustering coefficient for the left juxtapositional lobe (supplementary motor area, SMA; pcor = 0.038) and the left paracingulate gyrus (pcor = 0.044). Additionally, local efficiency for the left juxtapositional lobe (SMA) was decreased for PG patients (pcor = 0.022). Node betweenness was increased in the right paracingulate gyrus (pcor = 0.05) as well as in the left paracingulate gyrus (pcor = 0.011) in PG patients. Further differences in regional metrics at an uncorrected significance level are shown for exploratory purposes in Table 2.
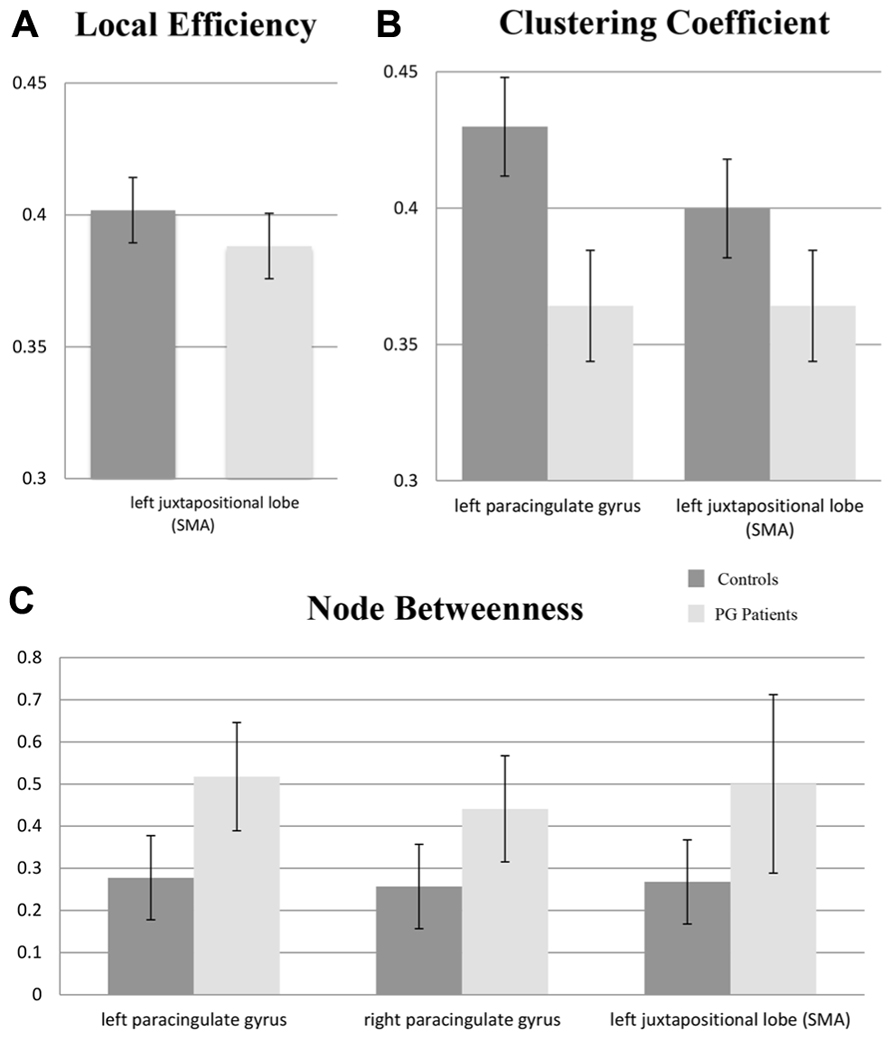
FIGURE 2. Mean area under the curve (AUC) values for nodal metrics in regions with statistically significant group differences (pcor < 0.05) between controls and patients with pathological gambling (PG): (A) local efficiency; (B) clustering coefficient; (C) node betweenness. Error bars reflect standard deviations; SMA: supplementary motor area.
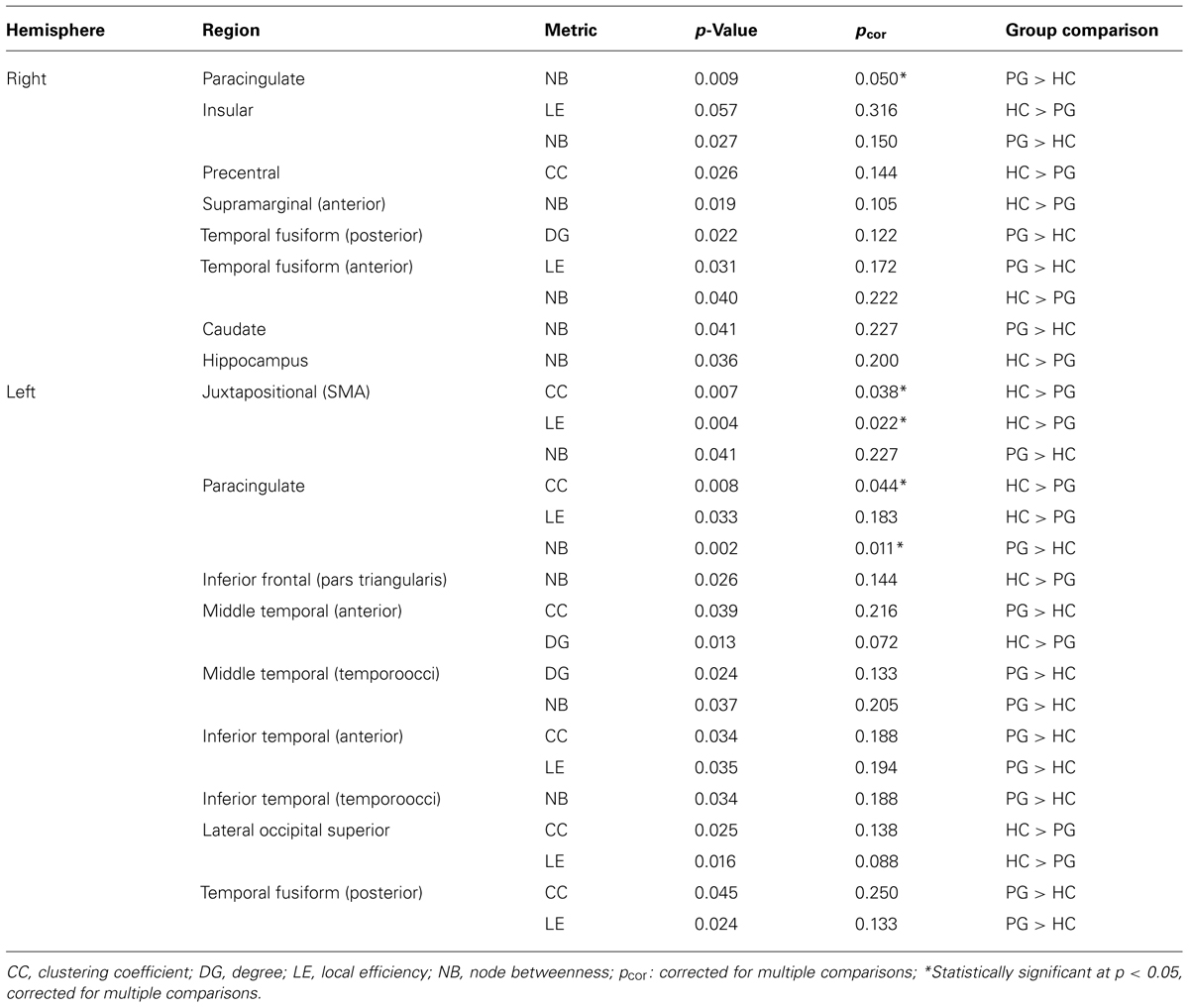
TABLE 2. Significant differences in all metrics using area under the curve (AUC) for pathological gamblers (PG) and healthy controls (HCs).
Functional Connectivity Analyses
Functional connectivity was increased in patients between frontal regions and between frontal and temporal regions (see Table 3). Furthermore, we found increased connectivity in patients between the left caudate and the right anterior cingulum as well as the left anterior cingulum. Additionally, the left amygdala with the left subcallosal cortex demonstrated weaker connectivity in patients than in controls.
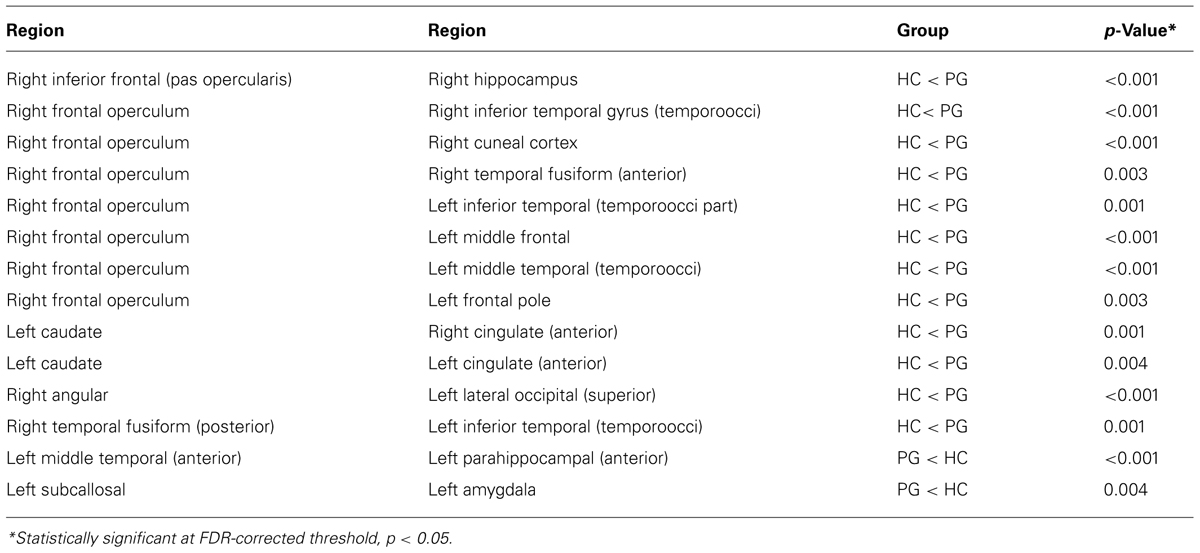
TABLE 3. Significant differences between pathological gamblers (PG) and healthy controls (HC) in functional connectivity.
Discussion
In this exploratory study, we investigated the functional network properties of patients with PG during the resting state using a graph-theoretical approach. While several studies could demonstrate functional abnormalities in PG during tasks associated with gambling, executive functions, and reward processing (Reuter et al., 2005; Tanabe et al., 2007; Balodis et al., 2012; Choi et al., 2012; Miedl et al., 2012; van Holst et al., 2012a; Hudgens-Haney et al., 2013; Limbrick-Oldfield et al., 2013; for a review, see Potenza, 2013), we are the first to show that patients with a behavioral addiction such as PG exhibit alterations in the topology of resting state networks in regions associated with reward processing and self-regulation.
Network properties at the global level showed no differences between patients and HCs. Global efficiency of information transfer and fault tolerance, for example, were similarly high in both groups. This is in line with a previous graph-theoretical study investigating the global topology of subjects suffering from internet addiction (Hong et al., 2013).
In contrast to global network properties, we found significant differences between healthy subjects and patients in network properties at the nodal level. Corrected for multiple comparisons, only medial frontal regions were affected in patients with PG. The SMA and the paracingulate cortex both showed a reduced clustering coefficient and impaired local efficiency of information transfer and fault tolerance. Furthermore, the contribution to the number of shortest paths was increased in both regions suggesting that these regions seem to adopt a more central position in the network than in healthy subjects. Note that the results for local efficiency in the paracingulate cortex and for betweenness centrality in the SMA are only tendencies, since they are not significant at a corrected level. These findings indicate that in medial frontal regions the balance between integration and segregation seem to be altered.
Medial frontal regions like the paracingulate cortex are associated with reward processing (Knutson et al., 2001; van den Bos et al., 2007; Fujiwara et al., 2009). Dysfunctions in reward processing are typical findings of previous investigations in PG (Reuter et al., 2005; Clark and Limbrick-Oldfield, 2013). The cingulate cortex is also important for gambling situations especially for specific processes of gambling (Campbell-Meiklejohn et al., 2008) like loss-chasing and quitting gambling.
Another frontal region which was found to be affected in PG is the SMA. The SMA demonstrated the same pattern of impairments as the paracingulate cortex with decreased clustering and efficiency of local information transfer but an increase in betweenness centrality.
The SMA is associated with motor execution and vigilance performance (Hinds et al., 2013) but is also involved in error detection and reward expectancy (McClure et al., 2004). Thus, the findings of this study demonstrating alterations in integration and segregation of medial frontal regions may underlie specific behavioral difficulties patients with PG exhibit.
Since this was an exploratory study, we also want to discuss findings which do not exceed the threshold selected to correct for multiple comparisons.
We found a reduced fraction of path length in the left inferior frontal gyrus which also contributes to the general findings of impairments in frontal regions in gambling and addiction. A previous study showed that PG patients exhibit alterations in inferior frontal activity during gambling cue presentation (Crockford et al., 2005). The inferior frontal gyrus has been associated with executive control and response inhibition (Hampshire et al., 2010). Interestingly, while medial frontal regions showed an increase in betweenness centrality, in lateral frontal regions, this metric was decreased. This pattern may support previous findings demonstrating deficits in self-regulation and working memory in PG (Forbush et al., 2008), but enhanced involvement of the reward system.
Additionally, we further found alterations in subcortical regions at an uncorrected threshold level. The right caudate plays a more central role as a main hub for integration of information compared to HCs while the hippocampus is less involved. Again, this points out the enhanced involvement of the reward system in PG. The caudate is part of the striatum which is an important part of the mesolimbic reward system. The alterations found in network properties of the hippocampus, are in line with deficits in heroin addicts identified in a previous study (Liu et al., 2009).
This pattern of impaired topology in regions which were previously associated with the executive control network and the reward system (Potenza et al., 2003; Reuter et al., 2005; Tanabe et al., 2007; Limbrick-Oldfield et al., 2013) is complemented by our findings of increased functional connectivity of fronto-striatal circuits and between frontal regions. Note that almost all differences in connectivity in which patients with PG exhibit higher functional connectivity than controls affect regions associated with the reward system. This is in line with previous studies finding alterations in functional connectivity between medial frontal and subcortical regions in addiction (Ma et al., 2010).
One previous study investigating network properties in behavioral addiction was performed in subjects with internet addiction (Hong et al., 2013). This study did not identify any alterations in the network topology in addicts. However, the authors emphasize that the non-significant results may be due to the small sample size. Additionally, two studies in heroin addiction also focused on graph-theoretical methods to investigate network properties (Liu et al., 2009; Yuan et al., 2010). They report dysfunction in several frontal regions including the cingulate cortex and the SMA, and subcortical regions including the striatum and the hippocampus. Our findings endorse the association of addictive behavior with alterations in functional connectivity and network topology during resting state in these specific frontal and striatal regions. This finding is of high relevance since previous investigations showing abnormalities in brain topology focused on addiction involving substance abuse. Thus, conclusions drawn from these studies are confounded by the neurotoxic effects of the abused substances (Clark and Limbrick-Oldfield, 2013). With this study, we confirm that abnormalities in network properties can also be found in behavioral addiction and therefore cannot solely be explained by effects of drugs on brain connectivity.
There are no available standards for a uniform application of graph theories at present (Bullmore and Sporns, 2009). One methodological limitation when investigating network topology with a graph-theoretical approach, for example, is the choice of thresholds. There are several possibilities to select the threshold and no golden standard has been defined yet. When comparing groups it should be ensured that each network has the same number of edges. However, the problem with a global threshold is that it may lead to disconnected graphs. Comparing network properties of one graph with the other is problematic if the one is connected at a given node and the other is disconnected. To address this problem, we ensured that the averaged degree is above the selected threshold and all subjects show small-world properties. Furthermore, we also investigated the global and local efficiency in addition to the clustering coefficient and the characteristic path length. These metrics have some methodological advantages when dealing with disconnectedness (Achard and Bullmore, 2007).
Another limitation is the wide range of thresholds selected. Depending on the range, results differ between studies and make comparison of findings and their interpretation difficult. However, we have implemented strategies which have been successfully applied in previous studies using graph-theoretical approaches (e.g., Zhang et al., 2011a; Bruno et al., 2012). Since this is a first exploratory study in PG using graph-theoretical analyses of resting state fMRI data, further research must be conducted to confirm these results.
Moreover, Zalesky et al. (2012) has shown that the type of randomization (topology randomization, correlation matrix randomization, or time-series randomization) influences the normalization process of the metrics. For a low density of around 7% the authors identified a discrepancy of approximately 60% when applying topology randomization compared to correlation matrix randomization to estimate the normalized clustering coefficient. In addition, using correlation matrix randomization to normalize characteristic path length may lead to longer path lengths due to the randomization of hub nodes since the degree distribution is not preserved. These limitations affect especially low density thresholds around 7% and are evident when looking at absolute small-world properties of the networks in each group. However, they are less essential for the comparison of network metrics between groups which is the focus of this study.
This first study in PG using graph-theoretical approaches to investigate network properties demonstrates that alterations of regions associated with the reward system and executive functions are not only present in task-related activity but also during rest. Alterations are reflected in a decrease in segregation and an increase of information integration in specific regions of the reward system.
This may contribute to the ongoing discussion whether PG is characterized by a hyper- or a hypoactive reward system (Hommer et al., 2011; van Holst et al., 2012b). Furthermore, our results suggest deficits of integration in regions associated with executive functions. These alterations may provide further explanation for several symptoms and previous findings in PG (for a review see Goudriaan et al., 2004; van Holst et al., 2010)
Conflict of Interest Statement
The authors declare that the research was conducted in the absence of any commercial or financial relationships that could be construed as a potential conflict of interest.
Acknowledgments
Melanie Tschernegg was supported by the Doctoral Collage “Imaging the Mind” of the Austrian Science Foundation (FWF-W1233). Further support was provided by grants of the Austrian Science Foundation (FWF P-23916-B18) and the Scientific Funds of the Paracelsus Medical University (E-10/12/062-KRO) to Martin Kronbichler. We want to thank Eva Reiter for her help with data acquisition.
Footnotes
References
Achard, S., and Bullmore, E. (2007). Efficiency and cost of economical brain functional networks. PLoS Comput. Biol. 3:e17. doi: 10.1371/journal.pcbi.0030017
Achard, S., Salvador, R., Whitcher, B., Suckling, J., and Bullmore, E. (2006). A resilient, low-frequency, small-world human brain functional network with highly connected association cortical hubs. J. Neurosci. 26, 63–72. doi: 10.1523/JNEUROSCI.3874-05.2006
Alexander-Bloch, A. F., Gogtay, N., Meunier, D., Birn, R., Clasen, L., Lalonde, F., et al. (2010). Disrupted modularity and local connectivity of brain functional networks in childhood-onset schizophrenia. Front. Syst. Neurosci. 4:147. doi: 10.3389/fnsys.2010.00147.
American Psychiatric Association. (2000). Diagnostic Criteria from DSM-IV-TR. Washington, DC: American Psychiatric Association.
American Psychiatric Association. (2013). Diagnostic Criteria from DSM-V. Washington, DC: American Psychiatric Association.
Babor, T. F., Higgins-Biddle, J. C., Dauser, D., Burleson, J. A., Zarkin, G. A., and Bray, J. (2006). Brief interventions for at-risk drinking: patient outcomes and cost-effectiveness in managed care organizations. Alcohol Alcohol. 41, 624–631. doi: 10.1093/alcalc/agl078
Balodis, I. M., Kober, H., Worhunsky, P. D., Stevens, M. C., Pearlson, G. D., and Potenza, M. N. (2012). Diminished frontostriatal activity during processing of monetary rewards and losses in pathological gambling. Biol. Psychiatry 71, 749–757. doi: 10.1016/j.biopsych.2012.01.006
Bassett, D. S., and Bullmore, E. T. (2009). Human brain networks in health and disease. Curr. Opin. Neurol. 22, 340–347. doi: 10.1097/WCO.0b013e32832d93dd
Beck, A. T., Steer, R. A., Ball, R., and Ranieri, W. (1996). Comparison of Beck Depression Inventories-IA and -II in psychiatric outpatients. J. Pers. Assess. 67, 588–597. doi: 10.1207/s15327752jpa6703_13
Behzadi, Y., Restom, K., Liau, J., and Liu, T. T. (2007). A component based noise correction method (CompCor) for BOLD and perfusion based fMRI. Neuroimage 37, 90–101. doi: 10.1016/j.neuroimage.2007.04.042
Bruno, J., Hosseini, S. M., and Kesler, S. (2012). Altered resting state functional brain network topology in chemotherapy-treated breast cancer survivors. Neurobiol. Dis. 48, 329–338. doi: 10.1016/j.nbd.2012.07.009
Buckholtz, J. W., and Meyer-Lindenberg, A. (2012). Psychopathology and the human connectome: toward a transdiagnostic model of risk for mental illness. Neuron 74, 990–1004. doi: 10.1016/j.neuron.2012.06.002
Bullmore, E., and Sporns, O. (2009). Complex brain networks: graph theoretical analysis of structural and functional systems. Nat. Rev. Neurosci. 10, 186–198. doi: 10.1038/nrn2575
Bullmore, E., and Sporns, O. (2012). The economy of brain network organization. Nat. Rev. Neurosci. 13, 336–349.
Campbell-Meiklejohn, D. K., Woolrich, M. W., Passingham, R. E., and Rogers, R. D. (2008). Knowing when to stop: the brain mechanisms of chasing losses. Biol. Psychiatry 63, 293–300. doi: 10.1016/j.biopsych.2007.05.014
Carver, C. S., and White, T. L. (1994). Behavioral-inhibition, behavioral activation, and affective responses to impending reward and punishment – the BIS BAS scales. J. Pers. Soc. Psychol. 67, 319–333. doi: 10.1037/0022-3514.67.2.319
Cavedini, P., Riboldi, G., Keller, R., D’Annucci, A., and Bellodi, L. (2002). Frontal lobe dysfunction in pathological gambling patients. Biol. Psychiatry 51, 334–341. doi: 10.1016/S0006-3223(01)01227-6
Choi, J. S., Shin, Y. C., Jung, W. H., Jang, J. H., Kang, D. H., Choi, C. H., et al. (2012). Altered brain activity during reward anticipation in pathological gambling and obsessive-compulsive disorder. PLoS ONE 7:e45938. doi: 10.1371/journal.pone.0045938
Cisler, J. M., Elton, A., Kennedy, A. P., Young, J., Smitherman, S., Andrew James, G., et al. (2013). Altered functional connectivity of the insular cortex across prefrontal networks in cocaine addiction. Psychiatry Res. 213, 39–46. doi: 10.1016/j.pscychresns.2013.02.007
Clark L., and Limbrick-Oldfield, E. H. (2013). Disordered gambling: a behavioral addiction. Curr. Opin. Neurobiol. 23, 655–659. doi: 10.1016/j.conb.2013.01.004
Cocchi, L., Bramati, I. E., Zalesky, A., Furukawa, E., Fontenelle, L. F., Moll, J., et al. (2012). Altered functional brain connectivity in a non-clinical sample of young adults with attention-deficit/hyperactivity disorder. J. Neurosci. 32, 17753–17761. doi: 10.1523/JNEUROSCI.3272-12.2012
Crockford, D. N., Goodyear, B., Edwards, J., Quickfall, J., and el-Guebaly, N. (2005). Cue-induced brain activity in pathological gamblers. Biol. Psychiatry 58, 787–795. doi: 10.1016/j.biopsych.2005.04.037
Ding, W. N., Sun, J. H., Sun, Y. W., Zhou, Y., Li, L., Xu, J. R., et al. (2013). Altered default network resting-state functional connectivity in adolescents with Internet gaming addiction. PLoS ONE 8:e59902. doi: 10.1371/journal.pone.0059902
Fagerstrom, K. O. (1978). Measuring degree of physical dependence to tobacco smoking with reference to individualization of treatment. Addict. Behav. 3, 235–241. doi: 10.1016/0306-4603(78)90024-2
Forbush, K. T., Shaw, M., Graeber, M. A., Hovick, L., Meyer, V. J., Moser, D. J., et al. (2008). Neuropsychological characteristics and personality traits in pathological gambling. CNS Spectr. 13, 306–315.
Fox, M. D., and Greicius, M. (2010). Clinical applications of resting state functional connectivity. Front. Syst. Neurosci. 4:19. doi: 10.3389/fnsys.2010.00019
Fujiwara, J., Tobler, P. N., Taira, M., Iijima, T., and Tsutsui, K. (2009). Segregated and integrated coding of reward and punishment in the cingulate cortex. J. Neurophysiol. 101, 3284–3293. doi: 10.1152/jn.90909.2008
Goudriaan, A. E., Oosterlaan, J., de Beurs, E., and Van den Brink, W. (2004). Pathological gambling: a comprehensive review of biobehavioral findings. Neurosci. Biobehav. Rev. 28, 123–141. doi: 10.1016/j.neubiorev.2004.03.001
Greicius, M. (2008). Resting-state functional connectivity in neuropsychiatric disorders. Curr. Opin. Neurol. 21, 424–430. doi: 10.1097/WCO.0b013e328306f2c5
Hampshire, A., Chamberlain, S. R., Monti, M. M., Duncan, J., and Owen, A. M. (2010). The role of the right inferior frontal gyrus: inhibition and attentional control. Neuroimage 50, 1313–1319. doi: 10.1016/j.neuroimage.2009.12.109
He, Y., Chen, Z., Gong, G., and Evans, A. (2009). Neuronal networks in Alzheimer’s disease. Neuroscientist 15, 333–350. doi: 10.1177/1073858409334423
He, Y., and Evans, A. (2010). Graph theoretical modeling of brain connectivity. Curr. Opin. Neurol. 23, 341–350.
Hinds, O., Thompson, T. W., Ghosh, S., Yoo, J. J., Whitfield-Gabrieli, S., Triantafyllou, C., et al. (2013). Roles of default-mode network and supplementary motor area in human vigilance performance: evidence from real-time fMRI. J. Neurophysiol. 109, 1250–1258. doi: 10.1152/jn.00533.2011
Hommer, D. W., Bjork, J. M., and Gilman, J. M. (2011). Imaging brain response to reward in addictive disorders. Ann. N.Y. Acad. Sci. 1216, 50–61. doi: 10.1111/j.1749-6632.2010.05898.x
Hong, S. B., Zalesky, A., Cocchi, L., Fornito, A., Choi, E. J., Kim, H. H., et al. (2013). Decreased functional brain connectivity in adolescents with internet addiction. PLoS ONE 8:e57831. doi: 10.1371/journal.pone.0057831
Hosseini, S. M., Hoeft, F., and Kesler, S. R. (2012). GAT: a graph-theoretical analysis toolbox for analyzing between-group differences in large-scale structural and functional brain networks. PLoS ONE 7:e40709. doi: 10.1371/journal.pone.0040709
Hudgens-Haney, M. E., Hamm, J. P., Goodie, A. S., Krusemark, E. A., McDowell, J. E., and Clementz, B. A. (2013). Neural correlates of the impact of control on decision making in pathological gambling. Biol. Psychol. 92, 365–372. doi: 10.1016/j.biopsycho.2012.11.015
Joutsa, J., Saunavaara, J., Parkkola, R., Niemela, S., and Kaasinen, V. (2011). Extensive abnormality of brain white matter integrity in pathological gambling. Psychiatry Res. 194, 340–346. doi: 10.1016/j.pscychresns.2011.08.001
Knutson, B., Fong, G. W., Adams, C. M., Varner, J. L., and Hommer, D. (2001). Dissociation of reward anticipation and outcome with event-related fMRI. Neuroreport 12, 3683–3687. doi: 10.1097/00001756-200112040-00016
Lesieur, H. R., and Blume, S. B. (1987). The South Oaks Gambling Screen (SOGS) – a new instrument for the identification of pathological gamblers. Am. J. Psychiatry 144, 1184–1188.
Limbrick-Oldfield, E. H., van Holst, R. J., and Clark, L. (2013). Fronto-striatal dysregulation in drug addiction and pathological gambling: consistent inconsistencies? Neuroimage (Amst.) 2, 385–393. doi: 10.1016/j.nicl.2013.02.005
Liu, J., Liang, J., Qin, W., Tian, J., Yuan, K., Bai, L., et al. (2009). Dysfunctional connectivity patterns in chronic heroin users: an fMRI study. Neurosci. Lett. 460, 72–77. doi: 10.1016/j.neulet.2009.05.038
Lynall, M. E., Bassett, D. S., Kerwin, R., McKenna, P. J., Kitzbichler, M., Muller, U., et al. (2010). Functional connectivity and brain networks in schizophrenia. J. Neurosci. 30, 9477–9487. doi: 10.1523/JNEUROSCI.0333-10.2010
Ma, N., Liu, Y., Li, N., Wang, C. X., Zhang, H., Jiang, X. F., et al. (2010). Addiction related alteration in resting-state brain connectivity. Neuroimage 49, 738–744. doi: 10.1016/j.neuroimage.2009.08.037
Maslov, S., and Sneppen, K. (2002). Specificity and stability in topology of protein networks. Science 296, 910–913. doi: 10.1126/science.1065103
McClure, S. M., York, M. K., and Montague, P. R. (2004). The neural substrates of reward processing in humans: the modern role of FMRI. Neuroscientist 10, 260–268. doi: 10.1177/1073858404263526
Menon, V. (2011). Large-scale brain networks and psychopathology: a unifying triple network model. Trends Cogn. Sci. 15, 483–506. doi: 10.1016/j.tics.2011.08.003
Miedl, S. F., Peters, J., and Buchel, C. (2012). Altered neural reward representations in pathological gamblers revealed by delay and probability discounting. Arch. Gen. Psychiatry 69, 177–186. doi: 10.1001/archgenpsychiatry.2011.1552
Petry, N. M. (2007). Gambling and substance use disorders: current status and future directions. Am. J. Addict. 16, 1–9. doi: 10.1080/10550490601077668
Petry, N. M., Blanco, C., Stinchfield, R., and Volberg, R. (2013). An empirical evaluation of proposed changes for gambling diagnosis in the DSM-5. Addiction 108, 575–581. doi: 10.1111/j.1360-0443.2012.04087.x
Potenza, M. N. (2008). Review. The neurobiology of pathological gambling and drug addiction: an overview and new findings. Philos. Trans. R. Soc. Lond. B Biol. Sci. 363, 3181–3189. doi: 10.1098/rstb.2008.0100
Potenza, M. N. (2013). Neurobiology of gambling behaviors. Curr. Opin. Neurobiol. 23, 660–667. doi: 10.1016/j.conb.2013.03.004
Potenza, M. N., Leung, H. C., Blumberg, H. P., Peterson, B. S., Fulbright, R. K., Lacadie, C. M., et al. (2003). An fMRI stroop task study of ventromedial prefrontal cortical function in pathological gamblers. Am. J. Psychiatry 160, 1990–1994. doi: 10.1176/appi.ajp.160.11.1990
Reuter, J., Raedler, T., Rose, M., Hand, I., Glascher, J., and Buchel, C. (2005). Pathological gambling is linked to reduced activation of the mesolimbic reward system. Nat. Neurosci. 8, 147–148. doi: 10.1038/nn1378
Rubinov, M., and Sporns, O. (2010). Complex network measures of brain connectivity: uses and interpretations. Neuroimage 52, 1059–1069. doi: 10.1016/j.neuroimage.2009.10.003
Salvador, R., Suckling, J., Coleman, M. R., Pickard, J. D., Menon, D., and Bullmore, E. (2005). Neurophysiological architecture of functional magnetic resonance images of human brain. Cereb. Cortex 15, 1332–1342. doi: 10.1093/cercor/bhi016
Singh, M. K., Kesler, S. R., Hosseini, S. M. H., Kelley, R. G., Amatyaa, D., Hamilton, J. P., et al. (2013). Anomalous gray matter structural networks in major depressive disorder. Biol. Psychiatry. doi: 10.1016/j.biopsych.2013.03.005 [Epub ahead of print].
Stam, C. J., and Reijneveld, J. C. (2007). Graph theoretical analysis of complex networks in the brain. Nonlinear Biomed. Phys. 1, 3. doi: 10.1186/1753-4631-1-3
Sutherland, M. T., McHugh, M. J., Pariyadath, V., and Stein, E. A. (2012). Resting state functional connectivity in addiction: lessons learned and a road ahead. Neuroimage 62, 2281–2295. doi: 10.1016/j.neuroimage.2012.01.117
Tanabe, J., Thompson, L., Claus, E., Dalwani, M., Hutchison, K., and Banich, M. T. (2007). Prefrontal cortex activity is reduced in gambling and nongambling substance users during decision-making. Hum. Brain Mapp. 28, 1276–1286. doi: 10.1002/hbm.20344
Telesford, Q. K., Burdette, J. H., and Laurienti, P. J. (2013). An exploration of graph metric reproducibility in complex brain networks. Front. Neurosci. 7:67. doi: 10.3389/fnins.2013.00067
van den Bos, W., McClure, S. M., Harris, L. T., Fiske, S. T., and Cohen, J. D. (2007). Dissociating affective evaluation and social cognitive processes in the ventral medial prefrontal cortex. Cogn. Affect. Behav. Neurosci. 7, 337–346. doi: 10.3758/CABN.7.4.337
van den Heuvel, M. P., and Hulshoff Pol, H. E. (2010). Exploring the brain network: a review on resting-state fMRI functional connectivity. Eur. Neuropsychopharmacol. 20, 519–534. doi: 10.1016/j.euroneuro.2010.03.008
van Holst, R. J., van den Brink, W., Veltman, D. J., and Goudriaan, A. E. (2010). Brain imaging studies in pathological gambling. Curr. Psychiatry Rep. 12, 418–425. doi: 10.1007/s11920-010-0141-7
van Holst, R. J., van Holstein, M., van den Brink, W., Veltman, D. J., and Goudriaan, A. E. (2012a). Response inhibition during cue reactivity in problem gamblers: an fMRI study. PLoS ONE 7:e30909. doi: 10.1371/journal.pone.0030909
van Holst, R. J., Veltman, D. J., van den Brink, W., and Goudriaan, A. E. (2012b). Right on cue? Striatal reactivity in problem gamblers. Biol. Psychiatry 72, e23–e24. doi: 10.1016/j.biopsych.2012.06.017
Whitfield-Gabrieli, S., and Nieto-Castanon, A. (2012). Conn: a functional connectivity toolbox for correlated and anticorrelated brain networks. Brain Connect. 2, 125–141. doi: 10.1089/brain.2012.0073
Xia, M., and He, Y. (2011). Magnetic resonance imaging and graph theoretical analysis of complex brain networks in neuropsychiatric disorders. Brain Connect. 1, 349–365. doi: 10.1089/brain.2011.0062
Yip, S. W., Lacadie, C., Xu, J., Worhunsky, P. D., Fulbright, R. K., Constable, R. T., et al. (2013). Reduced genual corpus callosal white matter integrity in pathological gambling and its relationship to alcohol abuse or dependence. World J. Biol. Psychiatry 14, 129–138. doi: 10.3109/15622975.2011.568068
Yu, Q., Allen, E. A., Sui J., Arbabshirani, M. R., Pearlson G., and Calhoun, V. D. (2012). Brain connectivity networks in schizophrenia underlying resting state functional magnetic resonance imaging. Curr. Top. Med. Chem. 12, 2415–2425. doi: 10.2174/156802612805289890
Yuan, K., Qin, W., Liu, J., Guo, Q., Dong, M., Sun, J., et al. (2010). Altered small-world brain functional networks and duration of heroin use in male abstinent heroin-dependent individuals. Neurosci. Lett. 477, 37–42. doi: 10.1016/j.neulet.2010.04.032
Zalesky, A., Fornito, A., and Bullmore, E. (2012). On the use of correlation as a measure of network connectivity. Neuroimage 60, 2096–2106. doi: 10.1016/j.neuroimage.2012.02.001
Zhang, J., Wang, J., Wu, Q., Kuang, W., Huang, X., He, Y., et al. (2011a). Disrupted brain connectivity networks in drug-naive, first-episode major depressive disorder. Biol. Psychiatry 70, 334–342. doi: 10.1016/j.biopsych.2011.05.018
Keywords: fMRI, graph theory, network, connectivity, pathological gambling, reward, behavioral addiction, small world
Citation: Tschernegg M, Crone JS, Eigenberger T, Schwartenbeck P, Fauth-Bühler M, Lemènager T, Mann K, Thon N, Wurst FM and Kronbichler M (2013) Abnormalities of functional brain networks in pathological gambling: a graph-theoretical approach. Front. Hum. Neurosci. 7:625. doi: 10.3389/fnhum.2013.00625
Received: 05 June 2013; Accepted: 10 September 2013;
Published online: 27 September 2013.
Edited by:
Yong He, Beijing Normal University, ChinaReviewed by:
Qingbao Yu, The Mind Research Network, USAXia Liang, National Institute on Drug Abuse, USA
Copyright © 2013 Tschernegg, Crone, Eigenberger, Schwartenbeck, Fauth-Bühler, Lemènager, Mann, Thon, Wurst and Kronbichler. This is an open-access article distributed under the terms of the Creative Commons Attribution License (CC BY). The use, distribution or reproduction in other forums is permitted, provided the original author(s) or licensor are credited and that the original publication in this journal is cited, in accordance with accepted academic practice. No use, distribution or reproduction is permitted which does not comply with these terms.
*Correspondence: Martin Kronbichler, Centre for Neurocognitive Research and Department of Psychology, University of Salzburg, Hellbrunnerstrasse 34, 5020 Salzburg, Austria e-mail:bWFydGluLmtyb25iaWNobGVyQHNiZy5hYy5hdA==
†Melanie Tschernegg and Julia S. Crone, as well as Friedrich M. Wurst and Martin Kronbichler have contributed equally to this work. Melanie Tschernegg and Julia S. Crone are joint first authors, and Friedrich M. Wurst and Martin Kronbichler are joint last authors.