- 1Department of Psychology I, Institute of Psychology, University of Würzburg, Würzburg, Germany
- 2Department of Mechanical Engineering, Curtin University, Perth, WA, Australia
- 3Department of Psychology and Speech Pathology, Curtin University, Perth, WA, Australia
- 4Neurosciences Unit, Health Department of Western Australia, Perth, WA, Australia
A repetitive movement practice by motor imagery (MI) can influence motor cortical excitability in the electroencephalogram (EEG). This study investigated if a realistic visualization in 3D of upper and lower limb movements can amplify motor related potentials during subsequent MI. We hypothesized that a richer sensory visualization might be more effective during instrumental conditioning, resulting in a more pronounced event related desynchronization (ERD) of the upper alpha band (10–12 Hz) over the sensorimotor cortices thereby potentially improving MI based brain-computer interface (BCI) protocols for motor rehabilitation. The results show a strong increase of the characteristic patterns of ERD of the upper alpha band components for left and right limb MI present over the sensorimotor areas in both visualization conditions. Overall, significant differences were observed as a function of visualization modality (VM; 2D vs. 3D). The largest upper alpha band power decrease was obtained during MI after a 3-dimensional visualization. In total in 12 out of 20 tasks the end-user of the 3D visualization group showed an enhanced upper alpha ERD relative to 2D VM group, with statistical significance in nine tasks.With a realistic visualization of the limb movements, we tried to increase motor cortex activation during subsequent MI. The feedback and the feedback environment should be inherently motivating and relevant for the learner and should have an appeal of novelty, real-world relevance or aesthetic value (Ryan and Deci, 2000; Merrill, 2007). Realistic visual feedback, consistent with the participant’s MI, might be helpful for accomplishing successful MI and the use of such feedback may assist in making BCI a more natural interface for MI based BCI rehabilitation.
Introduction
Over the past several years, advances in the analysis of electroencephalogram (EEG) signals and improved computing capabilities have enabled people with severe motor disabilities to use their own brain activity for communication and control of objects in their environment, thereby bypassing their impaired neuromuscular system (Kübler et al., 2001; Wolpaw et al., 2002; Allison et al., 2007; Perdikis et al., 2014). A new potential brain-computer interface (BCI) therapeutic approach that is generating substantial interest concerns the use of EEG-based BCI protocols to improve volitional motor control that has been impaired by trauma or disease by influencing processes underlying brain plasticity and thereby inducing recovery of motor control (Pichiorri et al., 2011). Repetitive movement practices by motor imagery (MI) can influence activity-dependent central nervous system (CNS) plasticity that underpins normal function. For example, it has recently been suggested that MI based BCI training can restore motor control in persons with hemiplegia due to stroke (Daly and Wolpaw, 2008; Broetz et al., 2010; Caria et al., 2011). Pichiorri et al. (2011) showed that training with MI led to a significant increase in motor cortical excitability in BCI naïve participants. The peak amplitude and volume of the motor evoked potentials recorded from a particular hand muscle were significantly higher only in those subjects who developed a MI strategy based on imagining their hand grasping in order to successfully control a computer cursor. Furthermore, functional analysis indicated that there was a change in the topology of active brain networks with practice of hand grasping MI. Rizzolatti et al. (2001) suggested that the capacity to associate the visual representation of an observed action with the motor representation of that action can lead to imitative learning. By inducing a better engagement of motor areas with respect to MI, it has therefore been suggested that BCI protocols are able to influence and guide neuroplasticity to promote recovery in affected brain regions to restore motor function after brain injury (Mulder, 2007; Cincotti et al., 2012).
Sensorimotor rhythms (SMRs) refer to localized sinusoidal frequencies in the upper alpha band (10–12 Hz; Pfurtscheller and Neuper, 2001), which can be recorded over primary somatosensory and motor cortical areas. SMR decreases or desynchronizes (event related desynchronization, ERD) by movement, observing the movement of others and by imagined self-movement (MI) in the contralateral sensorimotor areas (Schnitzler et al., 1997; Lotze et al., 1999; Neuper et al., 2005; Halder et al., 2011). MI is defined as the mental simulation of a kinesthetic movement without overt movements by muscular activity (Decety and Ingvar, 1990; Neuper et al., 2005, 2009). Signal processing algorithms, individual user’s characteristics, such as psychosocial and physiological parameters (e.g., fine motor skills) or brain structures, can predict performances for SMR-based BCIs (Blankertz et al., 2010; Halder et al., 2011; Hammer et al., 2012; Randolph, 2012). Besides these factors, feedback is a necessary feature for initial learning to modulate the sensorimotor rhythm (Wolpaw et al., 1991, 2002; McFarland et al., 1998). The end-user have to be properly trained to be able to successfully control their EEG signals, especially for the use of a BCI based on the recognition of mental imagery tasks (e.g., MI; Neuper and Pfurtscheller, 2001).
To learn modulating SMR power, usually unimodal visual feedback is provided: the end-user receives feedback by an extending bar or a moving cursor in one or two dimensions according to the classification results (Neuper and Pfurtscheller, 2010; Schreuder et al., 2010). This feedback can often be wrong, due to a poor performance in the calibration task, since first time end-users cannot be expected to perform the required mental tasks perfectly from the start (Lotte et al., 2013). The feedback and the feedback environment should be inherently motivating and relevant for the learner and should have an appeal of novelty, challenge, real-world relevance or aesthetic value (Ryan and Deci, 2000; Merrill, 2007). This supports the use of more engaging feedback environments, employing rather realistic and engaging feedback scenarios, which are closely related to the specific target application. A rich visual representation of the signal e.g., in the form of a 3-dimensional video game or Virtual reality (VR) environment may enhance the end user’s control of a SMR based-BCI (Pineda et al., 2003). Subjects learned to control levels of SMR activity and were able to control a SMR-based BCI (Pfurtscheller et al., 2006a; Friedmann et al., 2007) during motivationally engaging and a realistic, interactive task. On the basis of these and related findings, some researchers have proposed that realistic feedback is a powerful medium to improve BCI-presentation by creating immersive and motivating environments (Friedmann et al., 2007; Leeb et al., 2007; Ron-Angevin and Díaz-Estrella, 2009). This may also be expected to help the end-user getting used to richer and more complex environments, thus lowering the mismatch between the feedback provided during training and during real-world use (Lotte et al., 2013). For example one could expect that observing a realistic moving hand should have greater effect on the SMRs than watching an abstract feedback (Pfurtscheller et al., 2007).
For the successful restoration of CNS function via the use of SMR-based BCI as a rehabilitation tool, interventions that optimally induce activity-dependent CNS plasticity must be developed. In the current context, the most effective kind of feedback visualization must be properly identified in order to enhance standard care approaches for the rehabilitation of motor function, which typically focus on interventions involving the upper and lower limbs (Mulder, 2007; Daly and Wolpaw, 2008). On this basis, the current study investigated if a 3-dimensional visualization of five different upper and lower limb movements could amplify motor cortex activation during subsequent MI and thereby give prospective support for the use of a SMR based BCI. The purpose of this study was therefore to identify possible advantages associated with the use of an enriched 3-dimensional movement visualization as opposed to the use of 2-dimensional modality. We hypothesize that this type of “realistic” and more sensorial rich visualization might be more effective during instrumental conditioning, in which the EEG signal classifier is fixed and unknown to the end-user, and this user has to find out how to control a cursor by modulating the brain activity in a specific way, resulting in more pronounced ERD of the SMR rhythm (10–12 Hz) over the sensorimotor cortices. Therefore we conducted a controlled study design with healthy volunteers to identify the reactivity of SMRs during MI after showing realistic 2D and 3D limb movement video presentation.
Materials and Methods
Participants
In total, 39 healthy SMR-BCI novices took part in the study which was approved by the Human Research Ethics Committee of the Office of Research and Development at Curtin University. Each participant was informed about the purpose of the study and signed informed consent prior to participation. Four of the participants were excluded from analysis due to noise in the data: three of them were moving too much during the experiment and for one it was not possible to attain impedances lower than 20 kΩ. Of the 35 participants whose data were included in the final analysis, 18 were women and the mean age of the sample was 26.56 years (SD 5.33, range 18–54). Two participants were left-handed. All participants had normal or corrected-to-normal vision.
Experimental Set-Up
Participants were seated in a comfortable chair directly in front of a True3Di 24″ SDM-240M Stereoscopic 3D Monitor wearing stereoscopic glasses. Each participant’s chin lay on a pre-assembled chin holder. Participants were instructed to sit in a relaxed posture with their eyes open and avoiding any eye and body movements. Using a within subjects design, all participants were instructed to watch attentively 18 randomized videos of different limb movements for the left and right body part that were presented on a stereoscopic screen. Videos were displayed in 2D and 3D (Figure 1), portraying the following movements of computer-generated models: rotation of the wrist, elbow, knees and ankle anteriorly and an arm flexion towards the spectator. The videos displayed the movements from the perspective of the participant to encourage the feeling that each participant was moving their own limbs. At the end of each video a 6 s recording phase started, with a blank screen being presented during this phase. During this recording period, participants were requested to replicate subsequently the just observed movement by MI. The task was to perform a kinesthetic rather than visual MI (Neuper et al., 2005). Instructions were important during this experiment, as the participants only received offline feedback. Participants were instructed to feel the just observed motion in their muscles and they should vividly remember a situation in which they performed a given movement before imagining it during the subsequent BCI use. This should activate their prior experience with the task they will imagine, which is expected to make the learning easier (Merrill, 2007). Data collection lasted 45 min, with participants performing three runs of 10 min each, with 5 min breaks between each run.
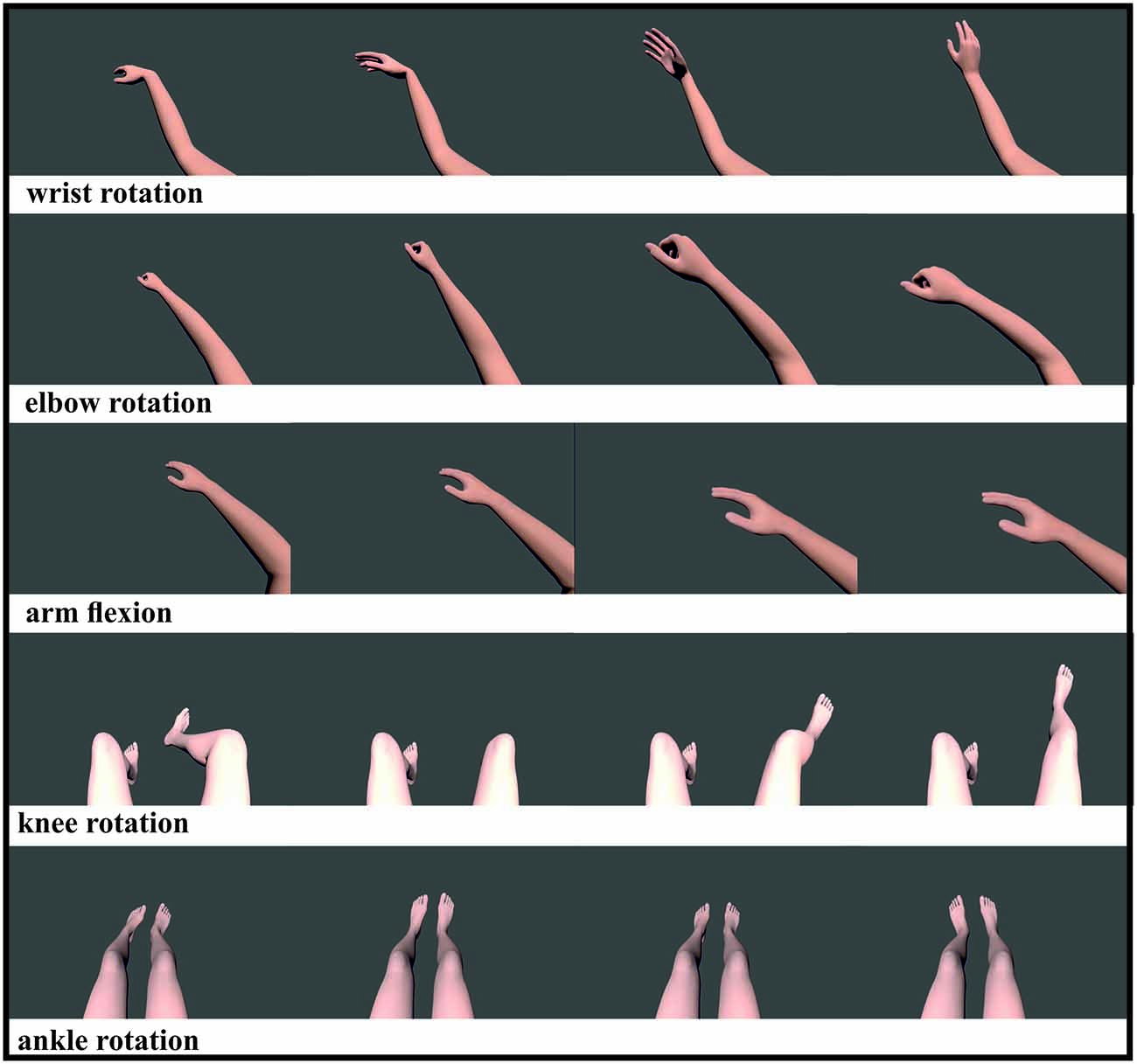
Figure 1. Visualization of five different limb movements: wrist movement, elbow rotation, arm flexion, knee and ankle rotation. All movements were shown for the left and right limb, except the ankle rotation which showed both feet rotating simultaneously. All videos were displayed randomized in 2D and 3D.
Data Acquisition
The EEG was recorded from 40 channels located over the sensorimotor cortex. The locations of the Ag/AgCl electrodes were based on the modified 10–20 system of the American Electroencephalographic Society (Sharbrough, 1991). Each channel was referenced to the left and grounded at the right mastoid. Impedances were kept below 5 kΩ via application of conductive gel. Data were collected via Neuroscan EEG equipment and signals were amplified using NuAmps amplifier. Data were sampled at 1000 Hz and bandpass filtered between 0.1–70 Hz with an additional notch filter applied to remove 50 Hz noise. A program algorithm was written to determine the presence of eye-blink artifacts; if identified, data from these periods were deleted. Data processing and storage were performed on a conventional laptop with an additional external monitor.
ERD/ERS Analyses
EEG signals were visually inspected and trials contaminated with muscle or eye movement activity were discarded. ERD/ERS (Energy Recovery System) calculation was undertaken by bandpass filtering of each trial, squaring of samples and subsequent averaging over trials and over sample points (Graimann et al., 2002). The ERD/ERS were expressed as proportional power decrease (ERD) or power increase (ERS) of the imagery period in the upper alpha frequency band (10–12 Hz) and were calculated relative to the baseline, in relation to a 1 s reference interval before the imagery period started. We generated topographical maps averaged for all participants for each task and visualization modality (VM). The resulting maps represent plots of significant ERD within the given frequency range of 10–12 Hz. Based on the results of the topographical maps, we computed the mean ERD/ERS in the alpha frequency band (10–12 Hz) with the traditional ERD/ERS method proposed by Pfurtscheller and Lopes da Silva (1999). For statistical analyses, we used the ERD/ERS values obtained from the right (C4) vs. left sensorimotor cortex (C3) temporally aggregated over the imagery period (1–6 s). In order to analyze the potential influence of the VM on the ERD/ERS patterns during task performance we performed a repeated measures ANOVA using the VM, task, electrode position (EP) and task side as within-subjects variables. The probability of a Type I error was maintained at 0.05.
Results
Figure 2 compares the topographical maps of the mean ERD values for the two VM groups, separately for the respective tasks (rotation of the wrist, elbow, knees and ankle in front and arm flexion towards the spectator) and pooled for both left and right MI in the upper alpha frequency band (10–12 Hz). In general, the results show a strong increase of the characteristic patterns of sensorimotor ERD of the upper alpha band components for left and right limb MI present over the sensorimotor areas in both visualization conditions. On basis of these findings EPs C3 and C4 were selected for further analyses, which is in accordance to other MI studies (Ron-Angevin and Díaz-Estrella, 2009; Neuper et al., 2009; Ono et al., 2013). A repeated measures ANOVA was performed on the ERD/ERS data using the VM (2 levels: 2D vs. 3D), task (5 levels: wrist movement, elbow rotation, arm flexion, knee and ankle rotation), EP (2 levels: C3 vs. C4) and task side (2 levels: left vs. right) as within-subjects variables, in order to analyze the potential influence of the VM on the ERD patterns during MI. In addition, we performed two 5 × 2 × 2 ANOVAs using the variables task, EP and task side as within-subjects variables for the two VM groups separately. Table 1 provides an overview of the significant ANOVA effects. Overall, significant differences were observed as a function of VM. This main effect is primarily due to the larger ERD during MI after 3D feedback. The significant main effect of Task indicates that ERD varied upon the different tasks. The averaged data for all upper limb (wrist rotation, elbow rotation, arm flexion) and lower limb MI tasks (knee rotation, ankle rotation) separated for the 2D and 3D condition were checked for normal distribution. Afterwards a post hoc paired sample t-test revealed significant smaller ERD values for lower limb MI tasks compared to upper limb MI tasks for the 2D (t(368) = 3.74, p = 0.041) and for the 3D (t(368) = 4.21, p = 0.0433) VM. A significant interaction between EP and task was found, which established the contralateral dominance of ERD. This analysis revealed significant interactions involving the factors VM, task, EP and task side (Table 1). Post hoc paired t-test comparison indicated that the largest upper alpha band power decrease during MI was obtained subsequent to the 3-dimensional visualization averaged or all tasks and both EPs (t(1007) = 3.126, p = 0.002).
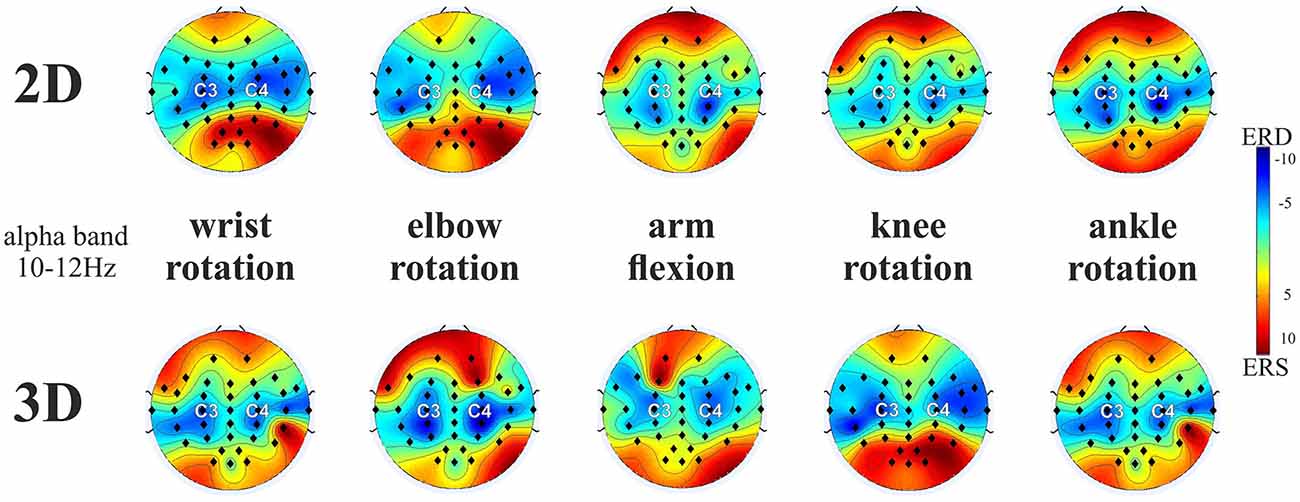
Figure 2. ERD/ERS patterns averaged over all end-users for the five motor imagery (MI) tasks (averaged across left and right limb movements) for 2D and 3D visualization modality (VM) in the upper alpha frequency band (10–12 Hz). Note: ERD is indicated in blue and ERS is indicated in red. The black dots represent the electrode positions (EPs).
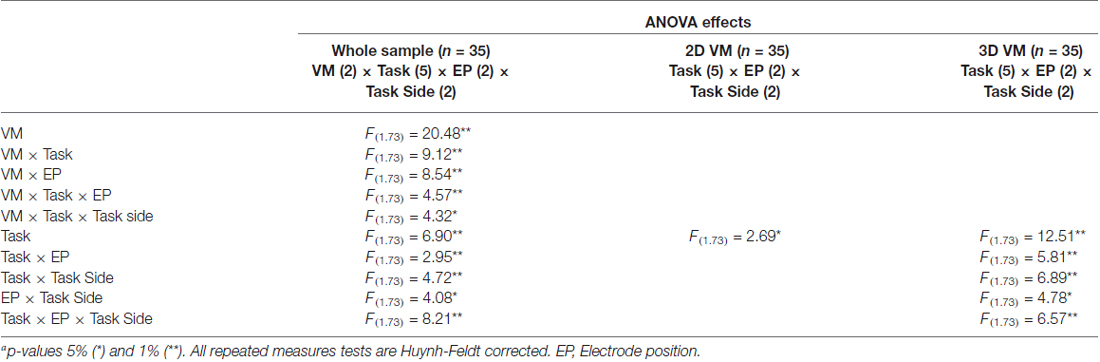
Table 1. Summary of significant F-valuesa for ERD/ERS analyses for the whole sample and separated for each visualization modality (VM).
Figure 3 presents a detailed overview of the mean ERD/ERS values with standard deviation and with t-test post hoc comparisons using a conservative significance level of 0.01, since we did not correct for multiple comparisons for the two visualization modalities (2D and 3D), separately for the different task, task side (left and right MI) and EP (C3 and C4). A difference between the visualization modalities can be seen in almost all tasks, depending on the EP and side of movement. In total in 12 out of 20 tasks the end-user of the 3D visualization group showed an enhanced upper alpha ERD relative to 2D VM group, with statistical significance (although not corrected for multiple comparisons) in nine tasks. The pattern of results suggests a generally higher ERD over the right (as compared to the left) sensorimotor region.
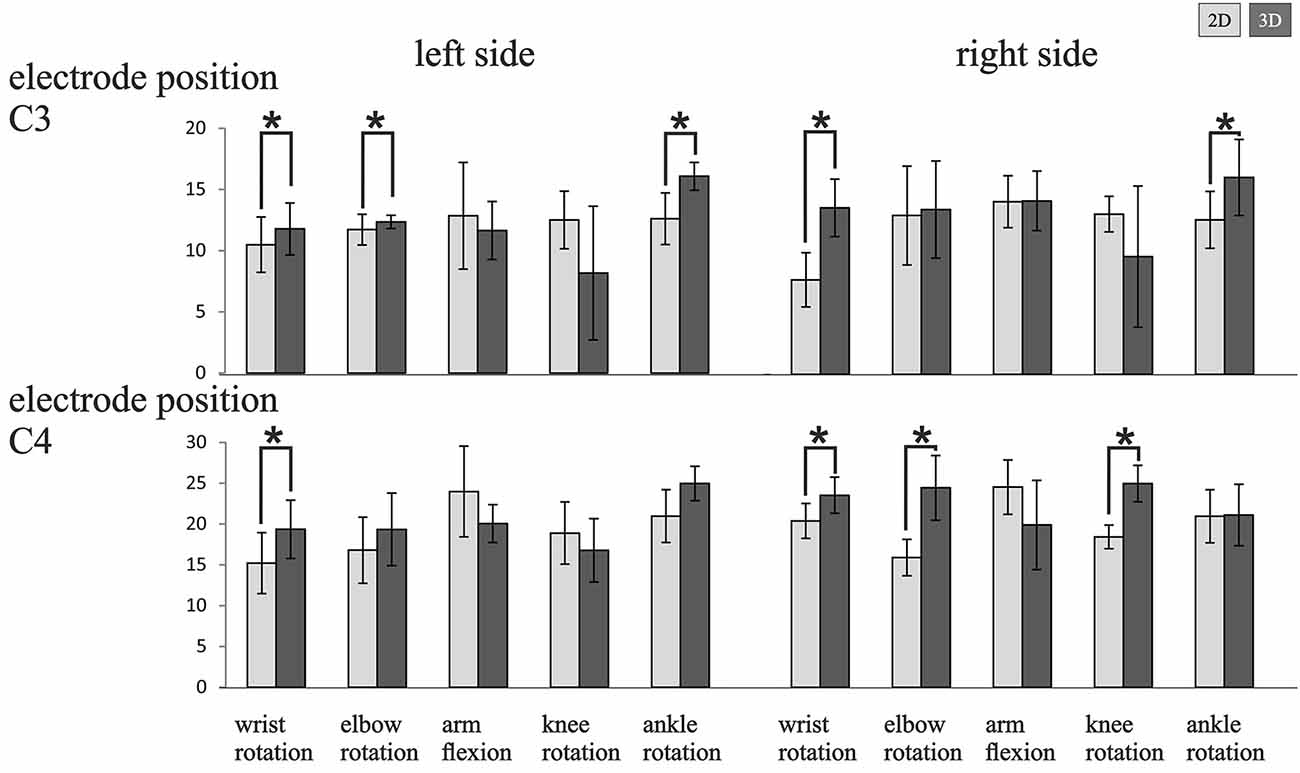
Figure 3. Mean ERD/ERS values (i.e., mean and standard deviation) obtained for the left (left panel) and right (right panel) limb MI side of the 10–12 Hz upper alpha frequency band for all subjects with the two visualization conditions (2D, light grey bar; 3D, dark grey bar) on EP C3 and C4. Significant differences between the visualization modalities are indicated (*p < 0.01).
Discussion
The present study was performed to investigate whether a 3-dimensional visualization of upper and lower limb movements can amplify motor cortex activation during a subsequent MI phase. Little is currently known about the impact of such a “realistic” VM. The SMR rhythm in humans can characteristically be found over the sensorimotor area with peaks around 10–12 Hz (Kuhlman, 1978; Hari et al., 1998; Pfurtscheller and Neuper, 2001). This frequency shows typical reactivity in association with MI (Pfurtscheller and Neuper, 1997; McFarland et al., 2000; Wolpaw et al., 2002; Blankertz et al., 2010). In the present study, we found a discernable decrease of the upper alpha rhythm (10–12 Hz) during imagery of limb movements over sensorimotor areas that significantly increased in the 3D visualization condition. The results showed in both VM conditions a more pronounced ERD for MI of the upper limbs compared to the lower limbs. We think this could be explained with the fewer difficulties that the SMR naïve participants have in imagining hand and arm movements. In daily life, we pay more attention to our movements of the upper limbs than conscious movements with the foot or knees and could explain the effect on motor cortex activation during MI.
The visualization of the different limb movements in a first person perspective was supposed to facilitate the task of performing MI. One potential limitation of the realistic video presentation was due to the fact that we used computerized limb models. We tried to create them as realistic as possible with skin color, texture and anatomical correct movement sequences. Especially for rehabilitation a computer animated version can give the advantage to adapt the limb to each individual user. Although a lot of effort was contributed in video programing still a visible difference exists compared to a video of a real limb movement. We refrained from using videos of taped limb motion, as this would not be an option for impaired patients. The main difficulty some people have especially those with limited motor function is to get a kinesthetic feeling (Neuper et al., 2005) of the movements and we wanted to support their MI by showing the specific motion beforehand. Previous work has suggested an important role for the perception of the body within a 3-dimensional environment (Slater et al., 1995). The body should be used naturally and should be anchored into the feedback for a successful ERD reproducibility. A possible explanation for this effect is the activation of the SMR which is in correspondence to the human mirror neuron system. This system matches action observation and execution and is capable of performing a simulation of just observed actions (Pineda, 2005; Neuper et al., 2009) and some researchers proposed a functional link between the observation of an action, the internal simulation, MI and the execution of the motor action (Grèzes and Decety, 2001; Neuper et al., 2005). The execution, imagination or observation of motor actions produces asynchronous firing in the mirror neurons and causes a suppression or desynchronization of the SMR-rhythm (Lopes da Silva, 2006). To exclude an overlaying effect of “motion observation” on the ERD in the alpha band (Muthukumaraswamy et al., 2004; Hammon et al., 2006; Perry and Bentin, 2009) we integrated a short pause between the videos and the MI phase, where the screen turned blank. How long the ERD of such a motion observation can last is not yet known. To be sure that the effects on the upper alpha band are only due to actual MI, we expanded the MI phase to 6 s. The current findings indicate that a 3-dimensional realistic presentation of movements to support a subsequent MI phase seems to be a suitable strategy to achieve locally restricted activation patterns for SMR-BCI use.
In a study by Friedmann et al. (2007), participants tried to control a SMR-based BCI in a CAVE system and showed that navigation was possible. Participants reported afterwards that they were more motivated in this kind of task compared to the training on a conventional visual monitor. They also reported that the interaction seemed more natural to them than traditional BCI. Virtual reality and 3D non-VR visualization are powerful tools with significant possibilities to improve BCI-feedback presentation (Pineda et al., 2003; Pfurtscheller et al., 2006b; Ron-Angevin and Díaz-Estrella, 2009). With this technology immersive and motivating environments can be created, which can positively influence a successful training (Leeb et al., 2007). A study by Gruzelier et al. (2010) could show that a SMR neurofeedback training in VR could enhance the artistic performance of actors more successfully than a training with a 2D feedback rendition. The efficacy of this training was attributed to the psychological engagement through the ecologically relevant learning context of the immersive VR technology.
We could show that the 3-dimensional visualization enhanced ERD in the upper alpha band in some but not in all MI tasks. Eleven tasks showed no significant differences in the mean ERD values however a high variance in this data can be found. A study by Neuper et al. (2009) compared the effects of abstract and realistic feedback on SMR BCI performance and could not find any significant differences between the two groups. One explanation for that was that feedback stimuli seem to become closely associated with the action goal during MI and therefore both feedback types were able to enhance the desired electrophysiological signals for individuals to perform accurately. This could also be true for our experiment. Most of the present studies compared “abstract” vs. “realistic” feedback (Neuper et al., 2009), presented activation maps during BCI training (Hwang et al., 2009) or game like feedback in VR (Scherer et al., 2008; Ron-Angevin and Díaz-Estrella, 2009; Zhao et al., 2009). Our study compared for the first time the actual effects of 2D and 3D visualization on MI during the same limb motion tasks: the video of the movements of the limbs were the same in both visualization conditions. We were aware that differences in the motor cortex activation may only be slightly detectable due to the similarity of the video presentations. In future studies, the influence of these two visualization modalities have to be further investigated as it is possible that the effect can be increased in an online setting where the end-user imagined movements affect the animated limb in real time. Following the herein presented results we can conclude that VM plays an important role in a SMR-controlled BCI. Providing end-users with a realistic 3-dimensional presentation of limb movements seems to help to get a concrete feeling of kinesthetic MI and exerts significant effects on motor cortex activation.
Conflict of Interest Statement
The authors declare that the research was conducted in the absence of any commercial or financial relationships that could be construed as a potential conflict of interest.
Acknowledgments
This work was funded by German Research Foundation (DFG) and the University of Wuerzburg in the funding programme Open Access Publishing, the Deutscher Akademischer Austauschdienst (DAAD), the Department of Mechanical Engineering at Curtin University (Perth/Western Australia) and partly supported by the Research Training Group of the GK Emotion (RTG 1253) and EU grant FP7-ICT-2011 “CONTRAST” project and the Graduate School of Life Sciences.
References
Allison, B. Z., Wolpaw, E. W., and Wolpaw, J. R. (2007). Brain-computer interface systems: progress and prospects. Expert Rev. Med. Devices 4, 463–474. doi: 10.1586/17434440.4.4.463
Blankertz, B., Sannelli, C., Halder, S., Hammer, E. M., Kübler, A., Müller, K. R., et al. (2010). Neurophysiological predictor of SMR-based BCI performance. Neuroimage 51, 1303–1309. doi: 10.1016/j.neuroimage.2010.03.022
Broetz, D., Braun, C., Weber, C., Soekadar, S. R., Caria, A., and Birbaumer, N. (2010). Combination of brain-computer interface training and goal-directed physical therapy in chronic stroke: a case report. Neurorehabil. Neural Repair 24, 674–679. doi: 10.1177/1545968310368683
Caria, A., Weber, C., Brötz, D., Ramos, A., Ticini, L. F., Gharabaghi, A., et al. (2011). Chronic stroke recovery after combined BCI training and physiotherapy: a case report. Psychophysiology 48, 578–582. doi: 10.1111/j.1469-8986.2010.01117.x
Cincotti, F., Pichiorri, F., Aricò, P., Leotta, F., de Vico Fallani, F., Millán Jdel, R., et al. (2012). EEG-based Brain-Computer Interface to support post-stroke motor rehabilitation of the upper limb. Conf. Proc. IEEE Eng. Med. Biol. Soc. 2012, 4112–4115. doi: 10.1109/EMBC.2012.6346871
Daly, J. J., and Wolpaw, J. R. (2008). Brain-computer interfaces in neurological rehabilitation. Lancet Neurol. 7, 1032–1043. doi: 10.1016/S1474-4422(08)70223-0
Decety, J., and Ingvar, D. H. (1990). Brain structures participating in mental simulation of motor behavior: a neuropsychological interpretation. Acta Psychol. (Amst) 73, 13–34. doi: 10.1016/0001-6918(90)90056-L
Friedmann, D., Leeb, R., Guger, C., Steed, A., Pfurtscheller, G., and Slater, M. (2007). Navigating virtual reality by thought: what is it like? Presence 16, 100–110. doi: 10.1162/pres.16.1.100
Graimann, B., Huggins, J. E., Levine, S. P., and Pfurtscheller, G. (2002). Visualization of significant ERD/ERS patterns in multichannel EEG and ECoG data. Clin. Neurophysiol. 113, 43–47. doi: 10.1016/s1388-2457(01)00697-6
Grèzes, J., and Decety, J. (2001). Functional anatomy of execution, mental simulation, observation and verb generation of actions: a meta-analysis. Hum. Brain Mapp. 12, 1–19. doi: 10.1002/1097-0193(200101)12:1<1::aid-hbm10>3.0.co;2-v
Gruzelier, J., Inoue, A., Smart, R., Steed, A., and Steffert, T. (2010). Acting performance and flow state enhanced with sensory-motor rhythm neurofeedback comparing ecologically valid immersive VR and training screen scenarios. Neurosci. Lett. 480, 112–116. doi: 10.1016/j.neulet.2010.06.019
Halder, S., Agorastos, D., Veit, R., Hammer, E. M., Lee, S., Varkuti, B., et al. (2011). Neural mechanisms of brain-computer interface control. Neuroimage 55, 1779–1790. doi: 10.1016/j.neuroimage.2011.01.021
Hammer, E. M., Halder, S., Blankertz, B., Sannelli, C., Dickhaus, T., Kleih, S., et al. (2012). Psychological predictors of SMR-BCI performance. Biol. Psychol. 89, 80–86. doi: 10.1016/j.biopsycho.2011.09.006
Hammon, P. S., Pineda, J. A., and de Sa, V. R. (2006). “Viewing motion animations during motor imagery: effects on EEG rhythms,” in Proceedings of the 3rd International Brain-Computer Interface Workshop and Training Course 2006, eds G. Müller-Putz, C. Brunner, R. Leeb, R. Scherer, A. Schlögl, S. Wriessnegger, et al. (Graz: University of Technology), 62–63.
Hari, R., Forss, N., Avikainen, S., Kirveskari, E., Salenius, S., and Rizzolatti, G. (1998). Activation of human primary motor cortex during action observation: a neuromagnetic study. Proc. Natl. Acad. Sci. U S A 95, 15061–15065. doi: 10.1073/pnas.95.25.15061
Hwang, H. J., Kiwoon, K., and Im, C. H. (2009). Neurofeedback-based motor imagery training for brain-computer interface (BCI). J. Neurosci. Methods 179, 150–156. doi: 10.1016/j.jneumeth.2009.01.015
Kübler, A., Kotchoubey, B., Kaiser, J., Wolpaw, J. R., and Birbaumer, N. (2001). Brain-computer communication: unlocking the locked in. Psychol. Bull. 127, 358–375. doi: 10.1037/0033-2909.127.3.358
Kuhlman, W. N. (1978). Functional topography of the human Mu rhythm. Electroencephalogr. Clin. Neurophysiol. 44, 83–93. doi: 10.1016/0013-4694(78)90107-4
Leeb, R., Friedman, D., Müller-Putz, G. R., Scherer, R., Slater, M., and Pfurtscheller, G. (2007). Self-paced (asynchronous) BCI control of a wheelchair in virtual environments: a case study with a tetraplegic. Comput. Intell. Neurosci. 79642. doi: 10.1155/2007/79642
Lopes da Silva, F. H. (2006). Event-related neural activities: what about phase? Prog. Brain Res. 159, 3–17. doi: 10.1016/s0079-6123(06)59001-6
Lotte, F., Larrue, F., and Mühl, C. (2013). Flaws in current human training protocols for spontaneous brain-computer interfaces: lessons learned from instructional design. Front. Hum. Neurosci. 7:568. doi: 10.3389/fnhum.2013.00568
Lotze, M., Montoya, P., Erb, M., Hülsmann, E., Flor, H., Klose, U., et al. (1999). Activation of cortical and cerebellar motor areas during executed and imagined hand movements: an fmri study. J. Cogn. Neurosci. 11, 491–501. doi: 10.1162/089892999563553
McFarland, D. J., McCane, L. M., and Wolpaw, J. R. (1998). EEG-based communication and control: short-term role of feedback. IEEE Trans. Rehabil. Eng. 6, 7–11. doi: 10.1109/86.662615
McFarland, D. J., Miner, L. A., Vaughan, T. M., and Wolpaw, J. R. (2000). Mu and beta rhythm topographies during motor imagery and actual movements. Brain Topogr. 12, 177–186. doi: 10.1023/A:1023437823106
Merrill, M. D. (2007). A task-centered instructional strategy. J. Res. Technol. Educ. 40, 5–22. doi: 10.1080/15391523.2007.10782493
Mulder, T. (2007). Motor imagery and action observation: cognitive tools for rehabilitation. J. Neural Transm. 114, 1265–1278. doi: 10.1007/s00702-007-0763-z
Muthukumaraswamy, S. D., Johnson, B. W., and McNair, N. A. (2004). Mu rhythm modulation during observation of an object-directed grasp. Brain Res. Cogn. Brain Res. 19, 195–201. doi: 10.1016/j.cogbrainres.2003.12.001
Neuper, C., and Pfurtscheller, G. (2001). Event-related dynamics of cortical rhythms: frequency-specific features and functional correlates. Int. J. Psychophysiol. 43, 41–58. doi: 10.1016/s0167-8760(01)00178-7
Neuper, C., and Pfurtscheller, G. (2010). “Neurofeedback trainingfor BCI control,” in Brain-Computer Interfaces, eds B. Graimann, G. Pfurtscheller, and B. Allison (London: Springer), 65–78.
Neuper, C., Scherer, R., Reiner, M., and Pfurtscheller, G. (2005). Imagery of motor actions: differential effects of kinesthetic and visual-motor mode of imagery in single-trial EEG. Brain Res. Cogn. Brain Res. 25, 668–677. doi: 10.1016/j.cogbrainres.2005.08.014
Neuper, C., Scherer, R., Wriessnegger, S., and Pfurtscheller, G. (2009). Motor imagery and action observation: modulation of sensorimotor brain rhythms during mental control of a brain-computer interface. Clin. Neurophysiol. 120, 239–247. doi: 10.1016/j.clinph.2008.11.015
Ono, T., Kimura, A., and Ushiba, J. (2013). Daily training with realistic visual feedback improves reproducibility of event-related desynchronisation following hand motor imagery. Clin. Neurophysiol. 124, 1779–1786. doi: 10.1016/j.clinph.2013.03.006
Perdikis, S., Leeb, R., Ramsay, A., Tavella, M., Desideri, L., Hoogerwerf, E. J., et al. (2014). Clinical evaluation of brain tree, a motor imagery hybrid BCI speller. J. Neural Eng. 11:036003. doi: 10.1088/1741-2560/11/3/036003
Perry, A., and Bentin, S. (2009). Mirror activity in the human brain while observing hand movements: a comparison between EEG desynchronization in the μ-range and previous fMRI results. Brain Res. 1282, 126–132. doi: 10.1016/j.brainres.2009.05.059
Pfurtscheller, G., Brunner, C., Schlögl, A., and Lopes da Silva, F. H. (2006a). Mu rhythm (de)synchronization and EEG single-trial classification of different motor imagery tasks. Neuroimage 31, 153–159. doi: 10.1016/j.neuroimage.2005.12.003
Pfurtscheller, G., Leeb, R., Keinrath, C., Friedman, D., Neuper, C., Guger, C., et al. (2006b). Walking from thought. Brain Res. 1071, 145–152. doi: 10.1016/j.brainres.2005.11.083
Pfurtscheller, G., and Lopes da Silva, F. H. (1999). Event-related EEG/MEG synchronization and desynchronization: basic principles. Clin. Neurophysiol. 110, 1842–1857. doi: 10.1016/s1388-2457(99)00141-8
Pfurtscheller, G., and Neuper, C. (1997). Motor imagery activates primary sensorimotor area in humans. Neurosci. Lett. 239, 65–68. doi: 10.1016/S0304-3940(97)00889-6
Pfurtscheller, G., and Neuper, C. (2001). Motor imagery and direct brain-computer communication. Proc. IEEE 89, 1123–1134. doi: 10.1109/5.939829
Pfurtscheller, G., Scherer, R., Leeb, R., Keinrath, C., Neuper, C., Lee, F., et al. (2007). Viewing moving objects in virtual reality can change the dynamics of sensorimotor EEG rhythms. Presence 16, 111–118. doi: 10.1162/pres.16.1.111
Pichiorri, F., De Vico Fallani, F., Cincotti, F., Babiloni, F., Molinari, M., Kleih, S. C., et al. (2011). Sensorimotor rhythm-based brain-computer interface training: the impact on motor cortical responsiveness. J. Neural Eng. 8:025020. doi: 10.1088/1741-2560/8/2/025020
Pineda, J. A. (2005). The functional significance of mu rhythms: translating seeing and hearing into doing. Brain Res. Brain Res. Rev. 50, 57–68. doi: 10.1016/j.brainresrev.2005.04.005
Pineda, J. A., Silverman, D. S., Vankov, A., and Hestenes, J. (2003). Learning to control brain rhythms: making a brain-computer interface possible. IEEE Trans. Neural Syst. Rehabil. Eng. 11, 181–184. doi: 10.1109/tnsre.2003.814445
Randolph, A. B. (2012). “Not all created equal: individual-technology fit of brain-computer interfaces,” in 45th Hawaii International Conference on System Science (HICSS) (Maui, HI), 572–578.
Rizzolatti, G., Fogassi, L., and Gallese, V. (2001). Neurophysiological mechanisms underlying the understanding and imitation of action. Nat. Rev. Neurosci. 2, 661–670. doi: 10.1038/35090060
Ron-Angevin, R., and Díaz-Estrella, A. (2009). Brain-computer interfaces: changes in performance using virtual reality techniques. Neurosci. Lett. 449, 123–127. doi: 10.1016/j.neulet.2008.10.099
Ryan, R. M., and Deci, E. L. (2000). Self-determination theory and the facilitation of intrinsic motivation, social development and well-being. Am. Psychol. 55, 68–78. doi: 10.1037/0003-066x.55.1.68
Scherer, R., Lee, F., Schlogl, A., Leeb, R., Bischof, H., and Pfurtscheller, G. (2008). Toward self-paced brain-computer communication: navigation through virtual worlds. IEEE Trans. Biomed. Eng. 55, 675–682. doi: 10.1109/tbme.2007.903709
Schnitzler, A., Salenius, S., Salmelin, R., Jousmäki, V., and Hari, R. (1997). Involvement of primary motor cortex in motor imagery: a neuromagnetic study. Neuroimage 6, 201–208. doi: 10.1006/nimg.1997.0286
Schreuder, M., Blankertz, B., and Tangermann, M. (2010). A new auditory multi-class brain-computer interface paradigm: spatial hearing as an informative cue. PLoS One 5:e9813. doi: 10.1371/journal.pone.0009813
Sharbrough, F. (1991). American electroencephalographic society guidelines for standard electrode position nomenclature. J. Clin. Neurophysiol. 8, 200–202. doi: 10.1097/00004691-199104000-00007
Slater, M., Usoh, M., and Steed, A. (1995). Taking steps: the influence of a walking metaphor on presence in virtual reality. ACM Trans. Comput. Hum. Interact. 2, 201–219. doi: 10.1145/210079.210084
Wolpaw, J. R., Birbaumer, N., McFarland, D. J., Pfurtscheller, G., and Vaughan, T. M. (2002). Brain-computer interfaces for communication and control. J. Clin. Neurophysiol. 113, 767–791. doi: 10.1016/S1388-2457(02)00057-3
Wolpaw, J. R., McFarland, D. J., Neat, G. W., and Forneris, C. A. (1991). An EEG-based brain-computer interface for cursor control. Electroencephalogr. Clin. Neurophysiol. 78, 252–259. doi: 10.1016/0013-4694(91)90040-b
Keywords: brain-computer interfaces, EEG, motor cortex activation, 3-dimensional visualization
Citation: Sollfrank T, Hart D, Goodsell R, Foster J and Tan T (2015) 3D visualization of movements can amplify motor cortex activation during subsequent motor imagery. Front. Hum. Neurosci. 9:463. doi: 10.3389/fnhum.2015.00463
Received: 19 December 2014; Accepted: 06 August 2015;
Published: 20 August 2015.
Edited by:
Hauke R. Heekeren, Freie Universität Berlin, GermanyReviewed by:
Alissa Fourkas, National Institutes of Health, USAJakub Limanowski, Freie Universität Berlin, Germany
Copyright © 2015 Sollfrank, Hart, Goodsell, Foster and Tan. This is an open-access article distributed under the terms of the Creative Commons Attribution License (CC BY). The use, distribution and reproduction in other forums is permitted, provided the original author(s) or licensor are credited and that the original publication in this journal is cited, in accordance with accepted academic practice. No use, distribution or reproduction is permitted which does not comply with these terms.
*Correspondence: Teresa Sollfrank, Department of Psychology I, Institute of Psychology, University of Würzburg, Marcusstrasse 9-11, 97070 Würzburg, Germany,dGVyZXNhLnNvbGxmcmFua0B1bmktd3VlcnpidXJnLmRl