- Cognitive Science & Traffic Research Unit, Institute of Behavioural Sciences, University of Helsinki, Helsinki, Finland
A fundamental question in human brain plasticity is how sensory, motor, and cognitive functions adapt in the process of skill acquisition extended over a period of many years. Recently, there has emerged a growing interest in cognitive neuroscience on studying the functional and structural differences in the brains of elite athletes. Elite performance in sports, music, or the arts, allows us to observe sensorimotor and cognitive performance at the limits of human capability. In this mini-review, we look at driving expertise. The emerging brain imaging literature on the neural substrates of real and simulated driving is reviewed (for the first time), and used as the context for interpreting recent findings on the differences between racing drivers and non-athlete controls. Also the cognitive psychology and cognitive neuroscience of expertise are discussed.
Introduction
Elite performance in sports, music, or the arts allows us to observe human performance at the limits of the sensorimotor and cognitive capacity of the mind and brain. Recently, interest has emerged in cognitive neuroscience on the functional and structural adaptations in the brains of elite athletes that make possible their exceptional performance (Yarrow et al., 2009). Findings in specific contexts, such as sports, can also teach us more generally about how sensory, motor, and cognitive functions adapt during the process of extended skill acquisition. This may have applications in designing optimal sports coaching methods, but also potentially in overcoming learning disabilities, or in neurological rehabilitation.
Research in the cognitive psychology of expertise has shown that these feats of excellence are not just a reflection of superior innate sensorimotor ability or general intelligence. They are, instead, based on a wealth of domain-specific skills and knowledge, accumulated over years of sustained effort. It is generally agreed that to attain expertise it is necessary to engage in sufficient amount of diligent and well-designed deliberate practice (Ericsson et al., 1993). The oft-quoted “10 000 h rule” (Figure 1A) states that to attain professional-level expertise in many domains, 10 000 h of engagement in deliberate practice is necessary (but not necessarily sufficient; Hambrick et al., 2014; Lombardo and Deaner, 2014). This translates to engagement in a laborious process of self-improvement for 4 h/day, on average, over a period 10 years.
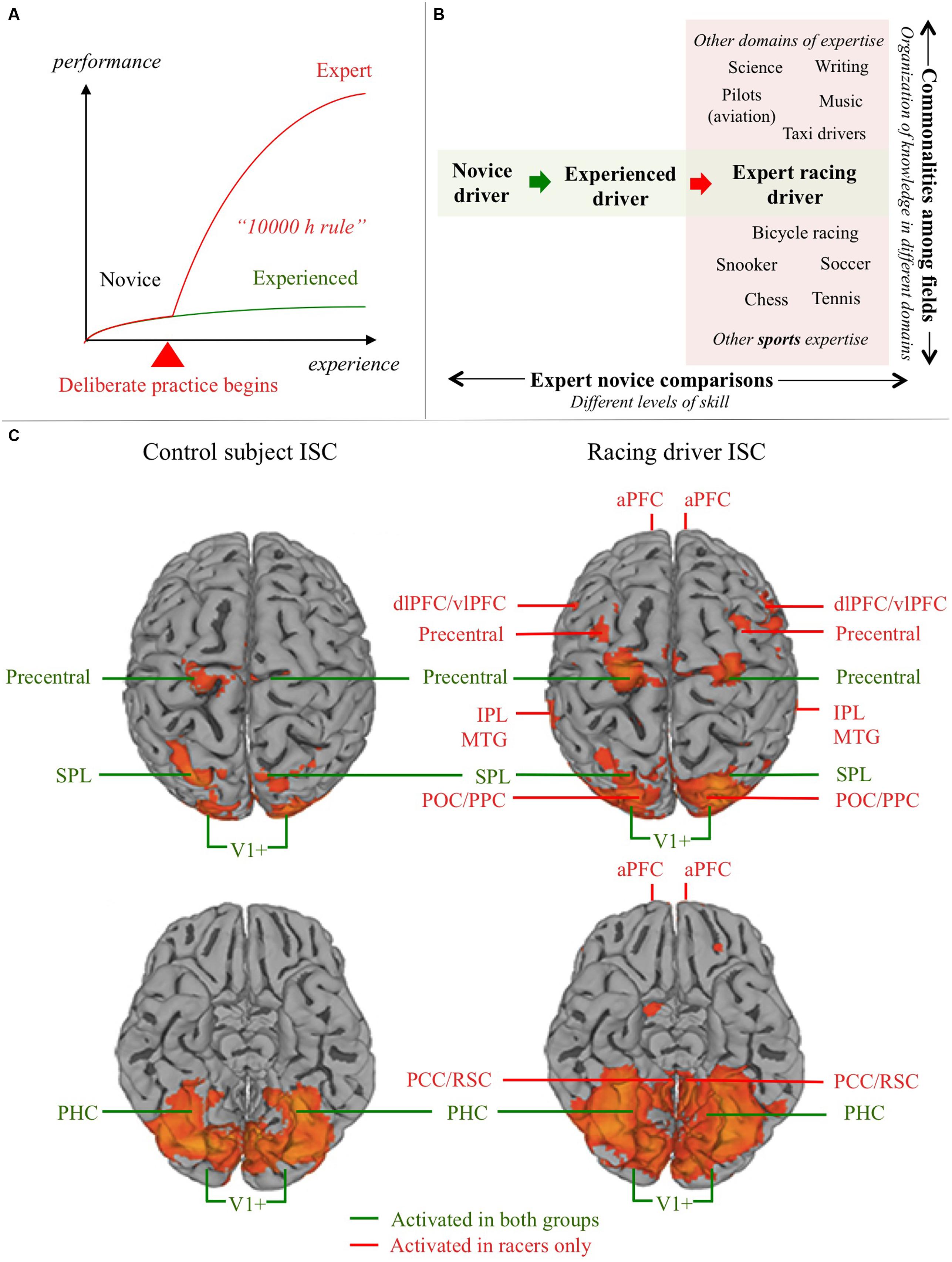
FIGURE 1. Domain expertise and the neural substrates of driving. (A) Schematic depiction of the relationship between experience and expertise. Development of expertise differs qualitatively from mere experience: initiating and sustaining deliberate practice is something we are able to do in one or maybe a few domains in our lives. Most skills (such as normal driving) never develop into a form of expertise. 10 000 h of driving experience does not make you an expert, because performance quickly plateaus to a level of merely satisfactory automatic performance. Experts, in contrast, re-invest cognitive capacity (freed by automatization) to improve performance further. (B) Understanding the neural substrate of expert performance can be approached in two ways: (i) Commonalities can be looked for in expert brain structure and function in different fields, to seek for general markers of expertise (e.g., different sports, or a sport and fields of expertise more or less similar in terms of cognitive demands); (ii) Experts in a specific domain can be compared to novices, or (as here) experienced non-experts in the domain, in order to understand how domain expertise modifies the neural substrates of task performance. (C) Control subject (experienced non-expert) and expert (racing car driver) ISC activation in a semi-active driving task. Based on Bernardi et al., 2014, Figure 1; doi: 10.3389/fnhum.2014.00888, Copyright Bernardi et al. (2014). aPFC, anterior prefrontal cortex; dlPFC, dorsolateral prefrontal cortex; vlPFC, ventrolateral prefrontal cortex; IPL, inferior parietal lobule; SPL, superior parietal lobule; MTG, middle temporal gyrus; POC/PPC, parieto-occipital/posterior parietal cortex; PHC, parahippocampal cortex; PCC/RSC, posterior cingulate/retrosplenial cortex; V1+, occipital visual areas
If expertise is the product of domain-specific knowledge and skills, then it makes sense to ask whether expert performance is made possible by specific, localized neural circuits. And if it is acquired by many years of extensive domain-specific training, one can look for evidence of experience-dependent plasticity in these circuits.
Race driving is a particularly good domain of sports expertise to study, because physically accurate stimuli and motor tasks can be presented in a controlled environment (simulators). Also, the racing driver is tightly harnessed to the vehicle, essentially leaving only eye, neck, shoulder, elbow, wrist, and ankle free to move. And even the highest levels of expertise are exercised through a low-dimensional system of vehicle controls (steering, throttle, brakes).
This mini-review looks at the neural substrates of driving, and the expertise involved in racing. Functional neuroimaging results on the neural substrates driving from the past 15 years are reviewed (the first systematic overview of this literature). This is used as a context to interpret recently observer differences in brain function between racing drivers and non-athlete controls by Bernardi et al. (2014). This approach is predicated on the idea of interpreting exceptional individuals’ brain function in comparison to experienced non-experts. (The other approach would be to seek “loci of excellence” that would be common in all sports or cognitively similar exceptional skills; Figure 1B). The thinking is that one should first analyze in detail the sensorimotor and cognitive task requirements of particular domains, and use this to guide the interpretation of the patterns of functional and structural plasticity produced by everyday learning and the rigorous demands of deliberate practice. In time, a more complete picture of what brain processes are specific to each task, and what general principles might be shared between expertise in different domains, will emerge.
Neural Substrates Of Normal Driving
An overview of the recent brain imaging literature on simulated and real driving in normal subjects is presented in Table 1. (Talairach coordinates of peak activation are collected in Supplementary Table S1, along with additional details of the experimental tasks and contrasts). The studies were selected on the basis that they should report brain activation in basic steering tasks (i.e., with no complex secondary tasks).
The most common finding is the activation of premotor frontal areas (BA6/8) and occipital visual areas (BA17/18/19), extending dorsally into medial temporal cortex and occipito-parietal areas (cuneus, precuneus), all the way to the posterior parietal cortex (PPC; BA7 and BA40 in SPL and IPL, respectively). The frontal activation extends to dorsolateral (BA9/46), anterior (BA10), and ventrolateral (BA 44/45) prefrontal cortices, and medial prefrontal and insular areas (BA47/13). Cingulate cortex activation is prevalent in the posterior cingulate/retrosplenial cortex, in the right hemisphere in particular. Within the ventral (occipito-temporal) system, activation is seen in the angular and fusiform gyri. Cerebellar activation at various sites has been observed in most studies, and some studies report activation in the basal ganglia and thalamus, but not all.
The general pattern is reasonably consistent, and the premotor and occipito-parietal activations in particular make sense, as driving is a visuomotor task where steering action needs to be adjusted to observed road geometry. The pattern of results is, however, by no means specific enough to amount to a detailed neurological understanding of the circuitry involved in driving. And the specific pattern of activation seems to depend on task, stimulus, and imaging methods in ways that are not yet well understood.
Racer vs. Control Comparison
Bernardi et al. (2014) used inter-subject correlation (ISC) to identify group level differences in brain activation between professional racing drivers (n = 11) and “naïve” controls (n = 11; Figure 1C). The advantage of reverse-correlation methods like ISC is that it reveals hemodynamic responses in multiple brain areas based on the synchrony of activation between subjects. It therefore does not require a rigid trial structure, repeating the same stimulus, or a priori identification of trigger events. This makes it particularly suitable for designs with naturalistic stimuli that present the subject with continuous streams stimulation, unlike more traditional block designs (Hasson et al., 2004, 2010; Kauppi et al., 2010).
The participants were shown in-car footage of an F1 car driving on official circuits, and were instructed to “imagine themselves driving the racing car”. Brain activity was measured using fMRI, and the BOLD signal time-series were analyzed to find areas that were reliably co-activated within each group (voxelwise Pearson’s correlation, significance testing by permutation test).
Significant activation was observed in both groups, bilaterally, in the visual cortex (V1+, BA 17/18), precentral cortex, posterior parietal cortex (superior parietal lobule, SPL), and parahippocampal cortex (PHC). The racer group showed, in addition, activation in dorsal visual stream (dorsal occipital/PPC), medial temporal gyrus (MTG), lateral prefrontal cortex, and the frontal pole (BA10), and posterior cingulate/retrosplenial cortex (PCC/RSC).
The groupwise activations were compared to reveal brain areas where activity was stimulus-modulated in the racer group significantly more than in the control group. This Racer > Control comparison showed activation in prefrontal cortex (middle frontal gyrus, bilaterally, Broca’s area in the inferior frontal gyrus of the left hemisphere, and right anterior prefrontal cortex), culmen of the right cerebellum, and further bilateral activations in MTG, PPC (supramarginal IPL), anterior cingulate (ACC), PCC/RSC, and caudate nucleus.
An Integrative View And Theoretical Interpretations
Activation of visual and precentral cortices was present in both groups, consistent with the previous literature. Occipital/occipito-temporal activation makes sense, as the task is to watch a video. Precentral activation, as well as the activation in the PPC (SPL), in both groups may indicate motor preparation, motor imagery, and visuo-spatial attention, reflecting the subjects’ engagement (this was a semi-active task, see below).
Fronto-parietal (dlPFC, IPL) responses were observed only in the Racer group. However, dlPFC/IPL activation has been observed also in normal subjects, by Walter et al. (2001), Calhoun et al. (2002) and Li et al. (2012). The roles of these areas are not obvious – this activation may be related to motor planning, eye movement behavior (FEF, PEF), and/or attentional strategies, which could all be more systematic – and therefore synchronized at group level – in the racers. Spiers and Maguire (2006, 2007) observed IPL and dlPFC activation during action planning and route planning.
Dorsal visual stream (precuneus) and parietal activation was more widespread in the racers. Could this be taken as an indication of higher level of engagement and/or richer visual representations in the expert group? With this type of reasoning one must proceed with caution: one might expect from common sense that experts would bring more “brainpower” to bear on the task. But in fact, what one often finds is that increased proficiency in a task leads to reduced, “more focused”, brain activation. This phenomenon has been dubbed neural efficiency (Haier et al., 1992), a perhaps counterintuitive inverse relationship between cognitive performance and brain metabolic activity. The basis for this is not yet understood at the level of mechanistic explanation (Poldrack, 2015), but could be due to more selective activation (only the relevant circuits), or reduced “processing cost” (less activation for a given task in the circuits involved). For expert–control comparisons, this would seem to suggest that for the same task (within the domain of expertise), an expert’s brain should show less activation (and indeed, see Bernardi et al., 2013).
So, why the more widespread pattern of activation in the racer group? One reason may be the use of ISC, which measures inter-subject synchrony – not individual signal change/metabolic level. Another reason may be that while the stimulus and instruction were the same for the two groups, the performed tasks may still be different, in terms of cognitive processes engaged. In direct comparisons, it is necessary to use relatively simple tasks (so that even the non-expert can perform them qualitatively similarly to the expert). By contrast, with a fairly naturalistic task (such as semi-active engagement used here), the task and instruction underdetermine the strategy used. While neural efficiency involves reduced activation in expert performance in a given task, in complex naturalistic tasks the knowledge and skill of the subjects partly determines the task itself, in terms of cognitive processes engaged. Thus, neural efficiency is best assessed by restricting the task to relatively simple tasks – as opposed to investigating tasks that are more typical for the experts.
Now, “naïve” participants may have a large amount of driving experience (i.e., exposure to road environments similar enough to the racetrack to engage with the task). However, they are unlikely to have developed the kind of rich and extensive domain knowledge that underlies expert cognitive and sensorimotor performance. This in turn may make the “viewing task” qualitatively different for the expert group. Expertise in racing is a result of intense training at a high level of cognitive and physical demand, which can result in qualitatively different skill sets to be employed in the (racing car) driving task. Therefore, it is plausible that when the race drivers view the in-car footage, they are not merely engaged in mentally simulating steering in the direction of the bends – i.e., lower-level sensorimotor control routines sufficient for everyday driving – but bring to the task a number of not yet fully understood cognitive skills, required to “read” the visual information from road geometry and landmarks at racing speeds. And hence recruit more brain areas. The situation is analogous to the expert taxi–driver studies (Spiers and Maguire, 2007, 2008), where the simulated road environment is used to evoke the experts’ rich high-level knowledge schemata.
In this light, perhaps the most interesting results pertain to the pattern in the PCC/RSC (BA30) and PHC (BA36). Activation in the PHC – bilateral in both groups – is to be expected with stimuli depicting 3D scenes based on prior work on (non-driving) scene perception imaging studies (Aguirre et al., 1996; Epstein and Kanwisher, 1998). But whereas the PHC is thought to encode viewpoint-dependent scene information, the retrosplenial cortex has been implicated in viewpoint-independent integration of scene information – “piecing together” the scene from locally observed snapshots (Spiers and Maguire, 2006, 2007, observed RSC activation in relation to spontaneous navigation and action planning; see also Iaria et al., 2007; Park and Chun, 2009; Vann et al., 2009). That these areas show up in the Racer > Control contrast may give us a window into the specific ability to “read” a road scene for 3D information relevant to motor planning – an essential skill in race driving. Notably, RSC structural differences were predictive of real-world performance in the Racer group.
Posterior parietal cortex and PFC are also activated in the Racers group. The RSC is closely anatomically and functionally connected to posterior parietal areas (involved in egocentric frame of reference transformations; Andersen et al., 1993; Pouget et al., 2002; McGuire and Sabes, 2009; Crawford et al., 2011), to the hippocampal complex (involved in construction of allocentric cognitive maps; O’Keefe and Nadel, 1978), and the prefrontal cortex (involved in planning and monitoring of complex actions, Ramnani and Owen, 2004). An intriguing possibility – though at this point speculative – is that this pattern reflects higher-level of cognitive task-organization in the experts. The vlPFC (Broca’s area) and dlPFC/aPFC have been implicated complex hierarchical organization in language and music (Patel, 2003), and the organization of hierarchical goals (Fuster, 2001; Koechlin et al., 2003; Petrides, 2005; Koechlin and Hyafil, 2007; Badre, 2008; Botvinick, 2008). Could the prefrontal–parietal–retrosplenial–hippocampal network be involved in processing a hierarchical representation of the driving line in terms of (loco)motor subgoals? This would mean that while the virtual driving task would be essentially path following for the controls, for the experts the planning of driving line would closely resemble a chunking process found to underlie pattern recognition, memory, and decision-making in many domains of skill and expertise (Cooper and Shallice, 2000; Gobet et al., 2001).
Open Issues And Future Directions
One essential factor that is not yet well understood is how the task and the instruction given may affect brain responses. Almost all the studies reviewed are “simulated driving” tasks (except the Jeong et al., 2006 PET study). The subject observes or controls a virtual car in a game environment. Such tasks may be active (controlling steering and/or speed of simulated ego-vehicle) or semi-active (actively imagining one is driving the ego-vehicle, but no overt movements). These contrast with “passive simulated driving” (viewing an in-car movie from real or simulated driving, as if one were the passenger rather than the driver). Which brain areas are activated by “simulated driving” can then depend on the contrast: in (semi)active tasks the active condition can be contrasted with passive viewing of the moving road scene (or a control stimulus), whereas in passive tasks the passive condition can be “driving”, compared to a non-driving control task. Thus, “driving” can be sometimes active > control, sometimes passive > control, but sometimes it can be active > passive.
Another factor is eye movement. Many of the areas activated in driving are also areas implicated in the control of saccadic and pursuit eye movements (Munoz, 2002; Krauzlis, 2004; Pierrot-Deseilligny et al., 2004), and coordinated and timely information pick-up is essential in many domains of skill. Differences in eye movement patterns caused by differences in the task, display, or subject skill may underpin some of the fronto-parietal activity differences (frontal and parietal eye-fields, FEF, and PEF, respectively). Differences in the way the gaze samples visual information create different retinal inputs, which can in turn produce differences in cortical processing downstream.
In expert vs. experienced non-expert comparisons, it would also be desirable to control the relevant background experience of the controls in more detail. Only sports experience was controlled by Bernardi et al. (2014), not prior exposure to the stimuli (in-car footage is commonly broadcast in televised Formula One events, and virtual models of the tracks can be driven in commercial games). The familiarity of the circuits to the racers is thus confounded with racing-specific skill. Recognition of familiar landmarks will elicit navigational memory in experts, but if some “naïve” controls are familiar with some circuits (through watching TV or playing videogames) this could mask group differences.
Racing game experience is probably especially relevant. If some “naïve” controls have played on virtual versions of the tracks, they may have developed some of the cognitive strategies of the professional racers. Comparison between truly naïve controls (but experienced drivers), experienced computer gamers, and real racers would make for an interesting research question in itself. This would control for familiarity and cognitive skills that can be learned in simulators, and might pinpoint specific adaptations in real racing, and begin to tease apart the component skills in “driving” and “racing”.
Of course, non-specific demographic control variables are important, too. Biological and socio-economical heterogeneity in the control group, and matching for ethnicity, socio-economic background, and body mass index are important to consider. But more to the point, by judicious use of controls, it may in fact be possible to better isolate the component skills. This means analysing “expertise” in terms of component capacities, not treating it as a monolithic state variable (and also clearly differentiating it from mere experience without deliberate practice).
Conclusion
We have discussed the expert racer brain activation in relation to known neural substrates of normal driving, and in the contexts of the cognitive psychology of expertise, and the representation of navigational space and complex motor action. The rationale is that the brain substrates of expert driving should first be understood in relation to normal driving (and tasks with similar cognitive components, even if they are not related to driving/sports). Comparison of the similarities and differences in the underpinnings of excellence in different sports can then proceed on a more secure footing.
The more widespread pattern of activation in racers (when semi-actively watching competitive driving from an egocentric perspective) could be due to more homogeneous synchronization of cognitive (and/or oculomotor) processes in the racer group. It may also be that with the development of cognitive expertise in racing, “the driving task” (i.e., the set of skills and cognitive operations involved) may have qualitatively changed. Whereas for a naïve participant steering a series of bends may effectively be reduced to a simple path-following visuomotor routine (even more so observing a video of a car steering a series of bends), to the expert with detailed survey knowledge of the track and a deep understanding of cornering techniques (cued by landmarks), many additional cognitive operations may be performed. This way, the pattern of activation itself can perhaps give us clues about the organization of the cognitive operations involved in racing.
Funding
Open Access cost for this publication were covered by a personal post-doctoral research grant to the author (Finnish Cultural Foundation, grant no. 00150514). The funders had no role in decision to publish, or preparation of the manuscript.
Conflict of Interest Statement
The author declares that the research was conducted in the absence of any commercial or financial relationships that could be construed as a potential conflict of interest.
Supplementary Material
The Supplementary Material for this article can be found online at: http://journal.frontiersin.org/article/10.3389/fnhum.2015.00635
TABLE S1 | Talairach coordinates from the studies in Table 1, as well as information on the contrasts and additional results for passive conditions.
References
Aguirre, G. K., Detre, J. A., Alsop, D. C., and D’Esposito, M. (1996). The parahippocampus subserves topographical learning in man. Cereb. Cortex 6, 823–829. doi: 10.1093/cercor/6.6.823
Andersen, R. A., Snyder, L. H., Li, C. S., and Stricanne, B. (1993). Coordinate transformations in the representation of spatial information. Curr. Opin. Neurobiol. 3, 171–176. doi: 10.1016/0959-4388(93)90206-E
Badre, D. (2008). Cognitive control, hierarchy, and the rostro-caudal organization of the frontal lobes. Trends Cogn. Sci. 12, 193–200.
Bernardi, G., Cecchetti, L., Handjaras, G., Sani, L., Gaglianese, A., Ceccarelli, R., et al. (2014). It’s not all in your car: functional and structural correlates of exceptional driving skills in professional racers. Front. Hum. Neurosci. 8:888. doi: 10.3389/fnhum.2014.00888
Bernardi, G., Ricciardi, E., Sani, L., Gaglianese, A., Papasogli, A., Ceccarelli, R., et al. (2013). How skill expertise shapes the brain functional architecture: an fMRI study of visuo-spatial and motor processing in professional racing-car and naïve drivers. PLoS ONE 8:e77764. doi: 10.1371/journal.pone.0077764
Botvinick, M. M. (2008). Hierarchical models of behavior and prefrontal function. Trends Cogn. Sci. 12, 201–208. doi: 10.1016/j.tics.2008.02.009
Calhoun, V. D., Pekar, J. J., McGinty, V. B., Adali, T., Watson, T. D., and Pearlson, G. D. (2002). Different activation dynamics in multiple neural systems during simulated driving. Hum. Brain Mapp. 16, 158–167. doi: 10.1002/hbm.10032
Cooper, R., and Shallice, T. (2000). Contention scheduling and the control of routine activities. Cogn. Neuropsychol. 17, 297–338. doi: 10.1080/026432900380427
Crawford, J. D., Henriques, D. Y., and Medendorp, W. P. (2011). Three-dimensional transformations for goal-directed action. Annu. Rev. Neurosci. 34, 309–331. doi: 10.1146/annurev-neuro-061010-113749
Epstein, R., and Kanwisher, N. (1998). A cortical representation of the local visual environment. Nature 392, 598–601. doi: 10.1038/33402
Ericsson, K. A., Krampe, R. T., and Tesch-Römer, C. (1993). The role of deliberate practice in the acquisition of expert performance. Psychol. Rev. 100, 363–406. doi: 10.1037/0033-295X.100.3.363
Fuster, J. M. (2001). The prefrontal cortex–an update: time is of the essence. Neuron 30, 319–333. doi: 10.1016/S0896-6273(01)00285-9
Gobet, F., Lane, P. C., Croker, S., Cheng, P. C., Jones, G., Oliver, I., et al. (2001). Chunking mechanisms in human learning. Trends Cogn. Sci. 5, 236–243. doi: 10.1016/S1364-6613(00)01662-4
Haier, R. J., Siegel, B., Tang, C., Abel, L., and Buchsbaum, M. S. (1992). Intelligence and changes in regional cerebral glucose metabolic rate following learning (Special Issue: Biology and Intelligence). Intelligence 16, 415–426. doi: 10.1016/0160-2896(92)90018-M
Hambrick, D. Z., Oswald, F. L., Altmann, E. M., Meinz, E. J., Gobet, F., and Campitelli, G. (2014). Deliberate practice: is that all it takes to become an expert? Intelligence 45, 34–45. doi: 10.1016/j.intell.2013.04.001
Hasson, U., Malach, R., and Heeger, D. J. (2010). Reliability of cortical activity during natural stimulation. Trends Cogn. Sci. 14, 40–48. doi: 10.1016/j.tics.2009.10.011
Hasson, U., Nir, Y., Levy, I., Fuhrmann, G., and Malach, R. (2004). Intersubject synchronization of cortical activity during natural vision. Science 303, 1634–1640. doi: 10.1126/science.1089506
Horikawa, E., Okamura, N., Tashiro, M., Sakurada, Y., Maruyama, M., Arai, H., et al. (2005). The neural correlates of driving performance identified using positron emission tomography. Brain Cogn. 58, 166–171. doi: 10.1016/j.bandc.2004.10.002
Iaria, G., Chen, J. K., Guariglia, C., Ptito, A., and Petrides, M. (2007). Retrosplenial and hippocampal brain regions in human navigation: complementary functional contributions to the formation and use of cognitive maps. Eur. J. Neurosci. 25, 890–899. doi: 10.1111/j.1460-9568.2007.05371.x
Jeong, M., Tashiro, M., Laxsmi, N. S., Yamaguchi, E., Horikawa, M., Miyake, S., et al. (2006). Functional brain mapping of actual car-driving using [18F]FDG-PET. Ann. Nuclear Med. 20, 623–628. doi: 10.1007/BF02984660
Kan, K., Schweizer, T. A., Tam, F., and Graham, S. J. (2013). Methodology for functional MRI of simulated driving. Med. Phys. 40, 012301. doi: 10.1118/1.4769107
Kauppi, J. P., Jaaskelainen, I. P., Sams, M., and Tohka, J. (2010). Inter-subject correlation of brain hemodynamic responses during watching a movie: localization in space and frequency. Front. Neuroinform. 4:5. doi: 10.3389/fninf.2010.00005
Koechlin, E., and Hyafil, A. (2007). Anterior prefrontal function and the limits of human decision-making. Science 318, 594–598. doi: 10.1126/science.1142995
Koechlin, E., Ody, C., and Kouneiher, F. (2003). The architecture of cognitive control in the human prefrontal cortex. Science 302, 1181–1185. doi: 10.1126/science.1088545
Krauzlis, R. J. (2004). Recasting the smooth pursuit eye movement system. J. Neurophysiol. 91, 591–603. doi: 10.1152/jn.00801.2003
Li, Y. O., Eichele, T., Calhoun, V. D., and Adali, T. (2012). Group study of simulated driving fMRI data by multiset canonical correlation analysis. J. Signal Process. Syst. 68, 31–48. doi: 10.1007/s11265-010-0572-8
Lombardo, M. P., and Deaner, R. O. (2014). You can’t teach speed: sprinters falsify the deliberate practice model of expertise. PeerJ. 2:e445.
Mader, M., Bresges, A., Topal, R., Busse, A., Forsting, M., and Gizewskia, E. R. (2009). Simulated car driving in fMRI—Cerebral activation patterns driving an unfamiliar and a familiar route. Neurosci. Lett. 464, 222–227. doi: 10.1016/j.neulet.2009.08.056
McGuire, L. M., and Sabes, P. N. (2009). Sensory transformations and the use of multiple reference frames for reach planning. Nat. Neurosci. 12, 1056–1061. doi: 10.1038/nn.2357
Munoz, D. P. (2002). Commentary: saccadic eye movements: overview of neural circuitry. Prog. Brain Res. 140, 89–96. doi: 10.1016/S0079-6123(02)40044-1
O’Keefe, J., and Nadel, L. (1978). The Hippocampus as a Spatial Map. Oxford: Oxford University Press.
Park, S., and Chun, M. M. (2009). Different roles of the parahippocampal place area (PPA) and retrosplenial cortex (RSC) in panoramic scene perception. Neuroimage 47, 1747–1756. doi: 10.1016/j.neuroimage.2009.04.058
Patel, A. D. (2003). Language, music, syntax and the brain. Nat. Neurosci. 6, 674–681. doi: 10.1038/nn1082
Petrides, M. (2005). Lateral prefrontal cortex: architectonic and functional organization. Philos. Trans. R. Soc. Lond. B Biol. Sci. 360, 781–795. doi: 10.1098/rstb.2005.1631
Pierrot-Deseilligny, C., Miles, D., and Müri, R. M. (2004). Eye movement control by the cerebral cortex. Curr. Opin. Neurol. 17, 17–25. doi: 10.1097/00019052-200402000-00005
Poldrack, R. A. (2015). Is “efficiency” a useful concept in cognitive neuroscience? Dev. Cogn. Neurosci. 11, 12–17. doi: 10.1016/j.dcn.2014.06.001
Pouget, A., Deneve, S., and Duhamel, J. R. (2002). A computational perspective on the neural basis of multisensory spatial representations. Nat. Rev. Neurosci. 3, 741–747.
Ramnani, N., and Owen, A. M. (2004). Anterior prefrontal cortex: insights into function from anatomy and neuroimaging. Nat. Rev. Neurosci. 5, 184–194. doi: 10.1038/nrn1343
Spiers, H. J., and Maguire, E. A. (2006). Thoughts, behaviour, and brain dynamics during navigation in the real world. Neuroimage 31, 1826–1840. doi: 10.1016/j.neuroimage.2006.01.037
Spiers, H. J., and Maguire, E. A. (2007). Neural substrates of driving behaviour. Neuroimage 36, 245–255. doi: 10.1016/j.neuroimage.2007.02.032
Spiers, H. J., and Maguire, E. A. (2008). The dynamic nature of cognition during wayfinding. J. Env. Psychol. 28, 232–249. doi: 10.1016/j.jenvp.2008.02.006
Vann, S. D., Aggleton, J. P., and Maguire, E. A. (2009). What does the retrosplenial cortex do? Nat. Rev. Neurosci. 10, 792–802. doi: 10.1038/nrn2733
Walter, H., Vetter, S. C., Grothe, J. O., Wunderlich, A. P., Hahn, S., and Spitzer, M. (2001). The neural correlates of driving. Neuroreport 12, 1763–1767. doi: 10.1097/00001756-200106130-00049
Keywords: expertise, driving, race driving, simulators, brain imaging, fMRI
Citation: Lappi O (2015) The Racer’s Brain – How Domain Expertise is Reflected in the Neural Substrates of Driving. Front. Hum. Neurosci. 9:635. doi: 10.3389/fnhum.2015.00635
Received: 02 August 2015; Accepted: 06 November 2015;
Published: 24 November 2015.
Edited by:
Rachael D. Seidler, University of Michigan, USAReviewed by:
Tao Liu, Sun Yat-Sen University, ChinaPietro Pietrini, Azienda Ospedaliero Universitaria Pisana, Italy
Copyright © 2015 Lappi. This is an open-access article distributed under the terms of the Creative Commons Attribution License (CC BY). The use, distribution or reproduction in other forums is permitted, provided the original author(s) or licensor are credited and that the original publication in this journal is cited, in accordance with accepted academic practice. No use, distribution or reproduction is permitted which does not comply with these terms.
*Correspondence: Otto Lappi, b3R0by5sYXBwaUBoZWxzaW5raS5maQ==