- 1Educational Neuroimaging Center, Faculty of Biomedical Engineering – Faculty of Education in Science and Technology, Technicon – Israel Institute of Technology, Haifa, Israel
- 2Reading and Literacy Discovery Center, Department of Pediatrics, University of Cincinnati College of Medicine, Cincinnati, OH, United States
- 3Division of General and Community Pediatrics, Department of Pediatrics, University of Cincinnati College of Medicine, Cincinnati, OH, United States
- 4Department of Environmental Health, University of Cincinnati College of Medicine, Cincinnati, OH, United States
- 5Imaging Research Center, Cincinnati Children’s Hospital Medical Center, Cincinnati, OH, United States
- 6Department of Radiology, University of Cincinnati College of Medicine, Cincinnati, OH, United States
Children with dyslexia exhibit slow and inaccurate reading, as well as problems in executive functions. Decreased signal activation in brain regions related to visual processing and executive functions has been observed with functional magnetic resonance imaging with reports of sex differences in brain patterns for visual processing regions. However, the underlying neurochemistry associated with deficits in executive functions for children with dyslexia has not been thoroughly evaluated. Reading ability and executive functions were assessed in fifty-three children [ages 8–12 years old, dyslexia (n = 24), and typical readers (n = 30)]. We employed short echo, single voxel, proton magnetic resonance spectroscopy to evaluate the perigenual anterior cingulate cortex (ACC). Pearson correlations were calculated between metabolite concentrations and measures of reading, processing speed, and executive function. Logistic regression models were used to determine the effects of brain metabolite concentrations, processing speed, and reading scores on dyslexia status. Differences by child’s sex were also examined. Compared to typical readers, higher global executive composite t-score is associated with greater odds for dyslexia (OR 1.14; 95% CI 1.05, 1.23); increased processing speed appears to be protective for dyslexia (OR 0.95; 95% 0.89–1.00). After adjustment for multiple comparisons, females with dyslexia showed strong and significant negative correlations between processing speed and myo-inositol (r = -0.55, p = 0.005) and choline (r = -0.54, p = 0.005) concentrations; effect modification by sex was confirmed in linear regression models (psex∗Cho = 0.0006) and (psex∗mI = 0.01). These associations were not observed for males or the group as a whole. These findings suggest that children with dyslexia share difficulty in one or more areas of executive function, specifically those related to response time. Also, metabolite changes in the ACC may be present in children with dyslexia, especially for females, and may hold value as possible markers for dyslexia.
Highlights
- Compared to typical readers, higher global executive composite t-score is associated with greater odds for dyslexia.
- Increased processing speed appears to be protective for dyslexia.
- Processing speed in females was negatively correlated with perigenual anterior cingulate concentrations of choline and myo-inositol.
Introduction
Reading Difficulties and Executive Functions
Reading difficulties (or dyslexia) are characterized by slow and inaccurate reading which continues into adulthood despite remedial intervention and exposure to the written language (International Dyslexia Association IDA, 2011). Specific challenges related to phonological and orthographical processing deficits, and more broadly the reading process, have been observed in individuals with dyslexia (Seidenberg and McClelland, 1989; Pugh et al., 2000). However, our accumulated data suggests that children with reading difficulties also demonstrate challenges in several higher order abilities, i.e., in executive functions (Horowitz-Kraus, 2014). More specifically, we found that children and adults with dyslexia demonstrate decreased error monitoring ability, as manifested using electroencephalographic (EEG)-event related potentials (ERP) amplitudes following a commission of an error (i.e., decreased error related negativity potential) compared to age matched typical readers (Horowitz-Kraus and Breznitz, 2008, 2013; Horowitz-Kraus, 2011, 2012). This alteration was not specific to reading materials but was also extended to tasks which do not contain verbal stimulation (i.e., the Wisconsin task) (Horowitz-Kraus, 2014). This ERP pattern, which is related to a mismatch between an actual and a desired response, is an evoked post-response and is related to a change in dopaminergic serge (Falkenstein et al., 1991, 2000). The error related negativity evoked from the anterior cingulate cortex (ACC) (Falkenstein et al., 2000) is part of the error detection system in the brain. The ACC is a critical part of the cingulo-opercular network which is related to a top-down monitoring process (Dosenbach et al., 2008). Support for the altered brain activation related to the ACC was found in our study showing a decreased functional connection within the cingulo-opercular network during reading in children with dyslexia and an increased functional connectivity following intervention that accompanied reading achievement (Horowitz-Kraus et al., 2015b). Since decreased ERPs are related to decreased neuronal firing, which in turn points at decreased signal activation on functional magnetic resonance imaging (fMRI), it is therefore not surprising that this network showed decreased functional connections with visual processing during a reading task in children with dyslexia, and altered functional connection during a Stroop task in this population (Levinson et al., 2018) emphasizing its critical role in monitoring during reading (Horowitz-Kraus et al., 2015a) as well as in the absence of a task (i.e., during rest) (Horowitz-Kraus et al., 2015b). Other ERP and fMRI studies revealed differences between individuals with dyslexia and typical readers, including manipulations aimed at improving reading through a specific triggering of executive functions, increased ERPs related to error monitoring (Horowitz-Kraus and Breznitz, 2014), and increased activation of the ACC (Horowitz-Kraus et al., 2014) and of functional connectivity of the cingulo-opercular network (Horowitz-Kraus et al., 2015b). These EEG and fMRI findings led us to examine the specific differences in metabolite concentrations within the ACC mediating reading ability.
Are There Neurochemical Characteristics for Reading Difficulties?
Lebel et al. (2016) observed using proton magnetic resonance spectroscopy (MRS) in typical preschool children that phonological processing ability was positively associated with glutamate (Glu), creatine (Cr), and myo-inositol (mI) concentrations in the pregenual anterior cingulate. As mI concentrations are thought to have a role as a marker of glial cells, the increased levels supported increased Glu neurotransmission and this metabolism provided a coupled relationship with phonological processing. However, most studies in the field of dyslexia focus on the role of phonological and orthographical routes (Shaywitz and Shaywitz, 2003a,b; Shaywitz et al., 2003). Pugh et al. (2014) characterized metabolite levels in children with dyslexia within the occipital cortex. This study employed English speaking, 6–10 year old typical readers and individuals with dyslexia and suggested a negative correlation between Cho and Glu concentrations with reading and phonological processing abilities (Pugh et al., 2014). The authors speculated that elevated Glu, a marker for hyperexcitability, may influence the coherence of neuronal networks involved in learning, which may also be the case for other pathologies. These researchers suggested that an unstable performance of children with reading difficulties is characterized with a moment to moment variance in performance and a lack of consistency (Pugh et al., 2014). This may provide a link to the monitoring challenges found in this population, which are not restricted to the reading domain. In a subsequent study, Del Tufo et al. (2018) found in children that cross-modal word matching mediates the relationship with increased Glu and increased Cho with poorer reading ability. Given that Cho levels represent membrane turnover as well as cellular and white matter density (Miller et al., 1996), elevated levels are in-turn related to increased connectivity or atypical myelination of the occipital cortex with additional regions in children with dyslexia (Pugh et al., 2014). Similarly, higher Cho levels in the angular gyrus are also associated with low reading scores in adults (Bruno et al., 2013). There it was suggested that Cho may be related to less efficient neuronal activity in this regions related to phonological processing and lower reading scores (Bruno et al., 2013). Bruno et al. (2013) also indicated that N-acetylaspartate (NAA) levels in the angular gyrus, a marker for intact neuronal ability, was related to a higher cognitive ability in adults (Jung et al., 2005). Del Tufo et al. (2018) also found that higher NAA predicted faster cross-modal matching reaction times in children. Increased NAA in the prefrontal cortex was also related to enhanced metabolic rates in these regions, probably due to differences in prefrontal maturation, as was observed in Asperger patients (Murphy et al., 2002). Higher NAA was previously related as a marker of viable neurons (Erickson et al., 2012) and there is conflicting evidence of positive and negative correlations (Mohamed et al., 2010) of NAA levels and cognitive control abilities. However, there is still a gap in knowledge as to the significance of metabolite concentrations in regions specifically related to executive functions in children with dyslexia.
Therefore, the goals of the current study were to: (1) determine whether metabolite concentrations in the ACC predict silent reading and sight word efficiency, processing speed or executive functions, (2) evaluate whether metabolite concentrations, reading ability, processing speed or executive functions vary in children with dyslexia compared to typical readers, and (3) determine if the associations vary by sex.
Materials and Methods
Participants
Participants were recruited from posted ads and through commercial advertisements. All participants gave their informed written assent and their parents provided informed written consents prior to inclusion in the study. This study was approved by the Cincinnati Children’s Hospital Medical Center Institutional Review Board.
Behavioral Measures
Baseline Reading Measures Used to Confirm Dyslexia Status
We confirmed the existence of reading difficulties using a battery of normative reading tests in English. Children with dyslexia were all diagnosed as having difficulty reading. Inclusion criteria for the dyslexia group were standard score of -1 and below or meeting the 25% or below cutoff in words reading, decoding and comprehension abilities. This battery included (a) words reading accuracy/orthography: the “Letter-Word” subset from the Woodcock and Johnson-III (WJ-III) battery (Woodcock and Johnson, 1989); (b) decoding: the “Word Attack” subset from the WJ-III (Woodcock and Johnson, 1989); and (c) reading comprehension subset from the WJ-III (Horowitz-Kraus et al., 2016). Participants in the typical readers group were age-matched students who volunteered for the study with fluent and accurate reading according to established normative levels for the WJ-III.
Reading Abilities
We further evaluated children’s reading abilities using the Tests of Word Reading Efficiency (TOWRE) to assess the participant’s ability to pronounce printed words (sight word efficiency) and the Tests of Silent Reading Efficiency and Comprehension (TORSEC) to assess the participant’s fluency ability.
Executive Functions and Cognitive Abilities
To assess executive functions and cognitive abilities, we used the Behavioral Rating Inventory of Executive Functions (BRIEF) (Gioia et al., 2002) parents questionnaire as well as the speed of processing tests from the Wechsler Intelligence Scale for Children, Fifth Edition (WISC-V) (Wechsler, 2014). The BRIEF questionnaire includes questions covering the child’s cognitive abilities (i.e., inhibition, organization, attention, monitoring, and emotional regulation). In the speed of processing subtests of the WISC-V, participants performed the symbol search and the coding sub-tests, both result in the Processing Speed Index (PSI) employed for the analyses.
Magnetic Resonance Measures
Acquisition Methods
Brain magnetic resonance imaging (MRI) and spectroscopy (MRS) were acquired using a Philips Achieva MR scanner operating at 3 Tesla (3T) and equipped with a 32-channel head coil. A three-dimensional (3D), high-resolution, isotropic, T1-weighted fast Fourier echo (FFE) anatomical imaging sequence was performed using 8.2 ms repetition time (TR), 3.7 ms echo time (TE), 1057 ms inversion time (TI), 8 degree flip angle, sensitivity encoding factor (SENSE) of 2, contiguous slices with a 1 mm thickness, and 1 × 1 mm voxel size. A single voxel, point resolved spectroscopy (PRESS) sequence was conducted using a 2000 ms TR, 30 ms TE, and 96 averages with water suppression along with an embedded unsuppressed water reference series of 16 averages. The 8 cubic centimeter single voxel was prescribed about the perigenual ACC within the medial frontal lobe localized from the 3D T1-FFE anatomical imaging sequence similar to the position described by Lebel et al. (2016) See Figure 1.
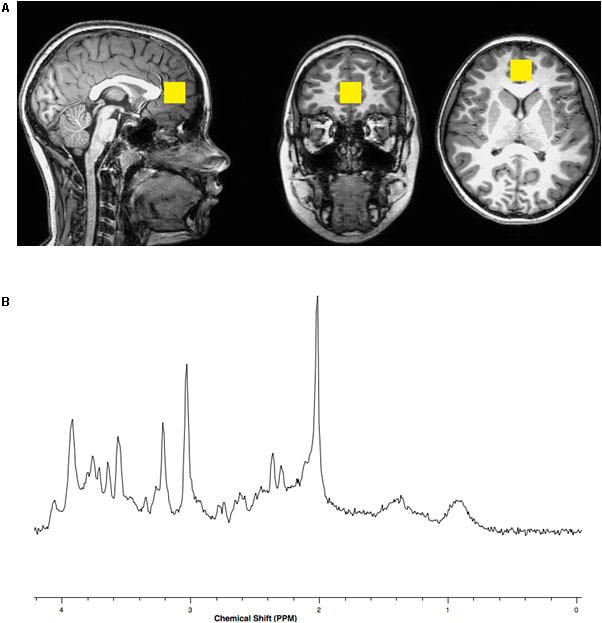
FIGURE 1. (A) Representative location within the anterior cingulate cortex for the 8 cubic centimeter (2 cm per side) spectroscopic voxel positioned on T1 weighted imaging slices centered in the sagittal, coronal and axial plane orientations. (B) Representative single voxel, short echo, magnetic resonance spectrum with N-acetylasparate appearing on the x-axis with a peak at a chemical shift value of 2 parts per million (PPM), glutamate at 2.3–2.4 PPM, creatine at 3.0 PPM, choline at 3.2 PPM and myo-inositol at 3.5 PPM.
Participants were acclimated and desensitized to condition them for comfort inside the scanner (see Byars et al., 2002 for details). Head motions were controlled using elastic straps that were attached to either side of the head-coil apparatus. An MRI-compatible audio/visual system (Avotec, SS3150/ SS7100) was used for the presentation of a movie during the session.
MRS Data Analysis
The raw spectroscopy data were imported into LCModel (Provencher, 1993) commercial software for quantitative processing. Metabolite and water reference levels were determined in institutional units. The raw metabolite levels were adjusted for the tissue contributions from gray matter, white matter and cerebrospinal fluid (CSF) using FSL (Woolrich et al., 2009) adjusted to the T1 and T2 relaxation decay rate of the corrected water concentration and corrected for literature reported T1 and T2 relaxation decay rates of the primary metabolites including NAA, Cho, Cr, and mI (Wansapura et al., 1999; Traber et al., 2004; Tal et al., 2012). However, pure Glu as well as the combined glutamate and glutamine (GLX) concentrations were unadjusted for metabolite T1 and T2 relaxation decay (Gussew et al., 2012). Reported values were concentrations in units of millimolar (mM).
Statistical Analyses
First, two sample t-tests were used to examine differences in age and IQ measures, reading and executive function measures as well as metabolite concentrations among children with dyslexia and typical readers.
Second, Pearson (r) correlations were calculated between individual metabolite concentrations and reading scales, processing speed, and global executive function. Correlation differences by dyslexia status and sex were examined. Correction for multiple comparisons was conducted by False Discovery Rate (FDR) estimation.
Third, significant correlations (FDR cutoff of 0.05) were then evaluated by linear regression adjusting for child’s sex and age at visit/test. Effect modification by sex was investigated by (1) including a two-way interaction term between metabolite concentration and child’s sex in the model, and (2) performing sex-stratified linear regression if the two-way interaction term was significant (p < 0.05). Effect sizes (β) represent the change in outcome for an interquartile range (IQR) increase in metabolite concentration.
Fourth, to determine if metabolite concentrations, processing speed, and global executive function predict dyslexia status, logistic regression models were implemented. For logistic models of metabolites, all metabolites were included in the same model after the investigation of multi-collinearity (based on correlations among metabolites).
Lastly, to confirm reading difficulty among children with dyslexia, we evaluated the association between silent reading and sight word efficiency and dyslexia status using the TOWRE and TOSREC. While it is likely these measures would be associated with dyslexia, it should be noted that these specific measures were not used to define the individuals in our study (see section 2.2.1). Logistic models were adjusted for child’s sex and age at visit/testing. Effect modification was evaluated by including a two-way interaction term between reading/behavior measure and child’s sex in the model and by stratification if interaction term was significant. Analyses were conducted with SAS 9.4 (SAS Institute Inc., Cary, NC, United States) and SPSS13 (IBM, Armonk, NY, United States).
Results
Participants
Twenty four children with dyslexia (mean age = 9.79 years, SD = 1.11, 8 females) and 30 typical readers (mean age = 10.73 years, SD = 1.07 year; 17 females) participated in the current study, all matched for age [t(51) = -1.77, non-significant (ns)]. All participants were within the normal range of non-verbal IQ (mean 101.45, SD = 10.26; children with dyslexia, mean 98.69, SD = 11.81 and typical readers mean 103.57, SD = 8.49) with [t(51) = -1.75, ns].
All participants were native English speakers, Caucasian, and with United States average socioeconomic status by income and education, as reported by the families using a socio-economic status questionnaire (Barratt, 2006) Participants were right-handed, displayed normal or corrected-to-normal vision in both eyes, and had normal hearing. None had a history of neurological or mood disorders, and individuals with reported history of attention difficulties were excluded.
Behavioral Measures
Overall, children with dyslexia demonstrated significant lower scores in all reading measures taken in the study: these readers showed lower phonological and orthographical abilities. Executive functions and speed of processing in children with dyslexia were significantly lower than typical readers. See Table 1.
Neurochemical-Behavior Correlations
Pearson correlation coefficient (r) values between mI, NAA, Cr, Cho, Glu, GLX, reading scales, processing speed, and global executive function were evaluated. There were no significant correlations observed among the group as a whole; however, there were differences observed by dyslexia status and sex (Table 2). Among children with dyslexia, there was a strong negative correlation between Cho and silent reading score (r = 0.51, p = 0.01); typical readers showed a strong negative correlation between mI and processing speed (r = -0.42, p = 0.02). After correction for multiple comparisons, no significant differences were found between group metabolite concentrations in children with dyslexia and typical readers.
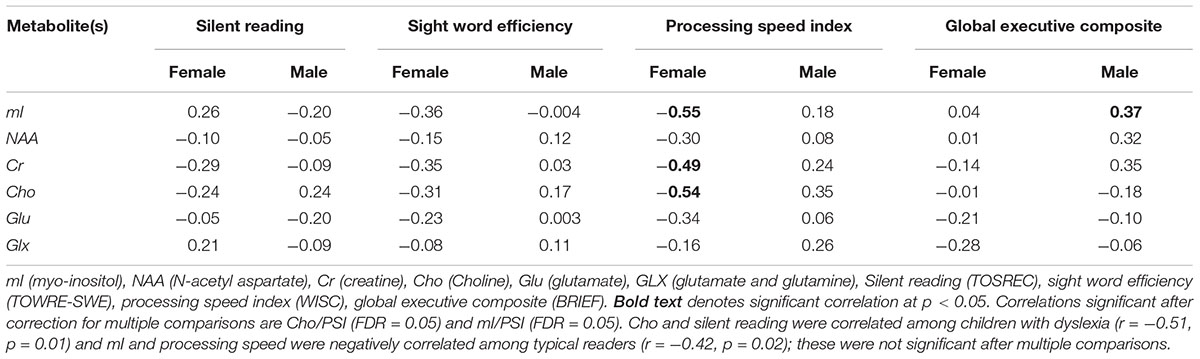
TABLE 2. Pearson correlation among metabolites and reading scales, processing speed, and global executive function by sex.
Females showed strong and significant negative correlations between processing speed and mI (r = -0.55, p = 0.005), Cr (r = -0.49, p = 0.01), Cho (r = -0.54, p = 0.005) levels. See Table 2. After correction for multiple comparisons, the correlations between PSI, mI, and Cho remained significant (FDRs = 0.05). Among males, there was a positive correlation between global executive function and mI (r = 0.35, p = 0.05); this did not hold up after correction for multiple comparisons (FDR = 0.49).
Effect of Neurochemical Levels on Processing Speed
The overall effect of Cho and mI on processing speed was not significant; however there was indication of effect modification by child’s sex. Figure 2 shows the effect of (a) Cho (pinteraction = 0.0006) and (b) mI (pinteraction = 0.01) on predicted mean scores for processing speed by child’s sex. Higher levels of Cho (β -8.10; 95% CI -12.73, -3.45) and mI (β -5.22; 95%CI -8.22, -2.22) are associated with decreased processing speed among females but not males (Table 3).
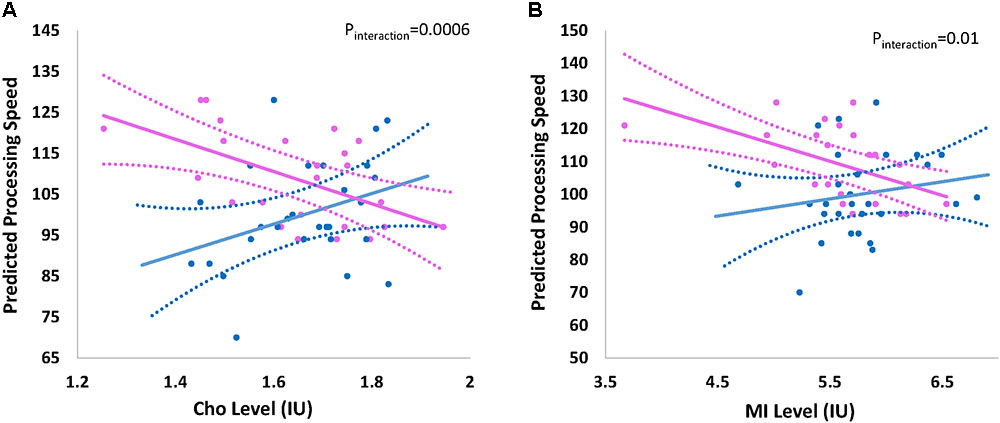
FIGURE 2. Effect of (A) choline and (B) myo-inositol on processing speed by sex. Graph represents the effect of (A) choline (Cho) and (B) myo-inositol (mI) on the predicted processing speed of participants by child’s sex Sex-stratified regression models are adjusted for age at visit/testing. Blue and pink indicates the effect for males and females, respectively. Dashed lines represent 95% confidence intervals. Actual individual level data are overlaid for males (blue circles) and females (pink circles). Interaction p-value represents the p-value for the formal two-way interaction between metabolite level and child’s sex in the linear regression model.
Effect of Global Executive Function, Processing Speed, Reading Measures, and Metabolites on Dyslexia Status
Children with a higher global executive composite t-score are more likely to have dyslexia (OR 1.14; 95% CI 1.05, 1.23) suggesting some difficulty in one or more areas of executive function is present among children with dyslexia compared to typical readers (Table 4). We also observed a marginally significant (p = 0.049) association between increased processing speed and protective odds for dyslexia (OR 0.95; 95% 0.89–1.00) compared to typical readers. Metabolite concentrations did not predict dyslexia status. Metabolite concentrations were moderately to significantly correlated (r ranging from 0.22 to 0.59, r = 0.80 for GLX/Glu correlations) and given the strong correlation between GLX and Glu, a sensitivity analysis was conducted to determine if removal of one of these metabolites impacted the results; the findings remained insignificant. As expected, higher scores on silent reading and sight word efficiency are observed among typical readers as evident by the ORs less than 1 (Table 4). There was no evidence of effect modification by child’s sex (all interaction p-values > 0.20).
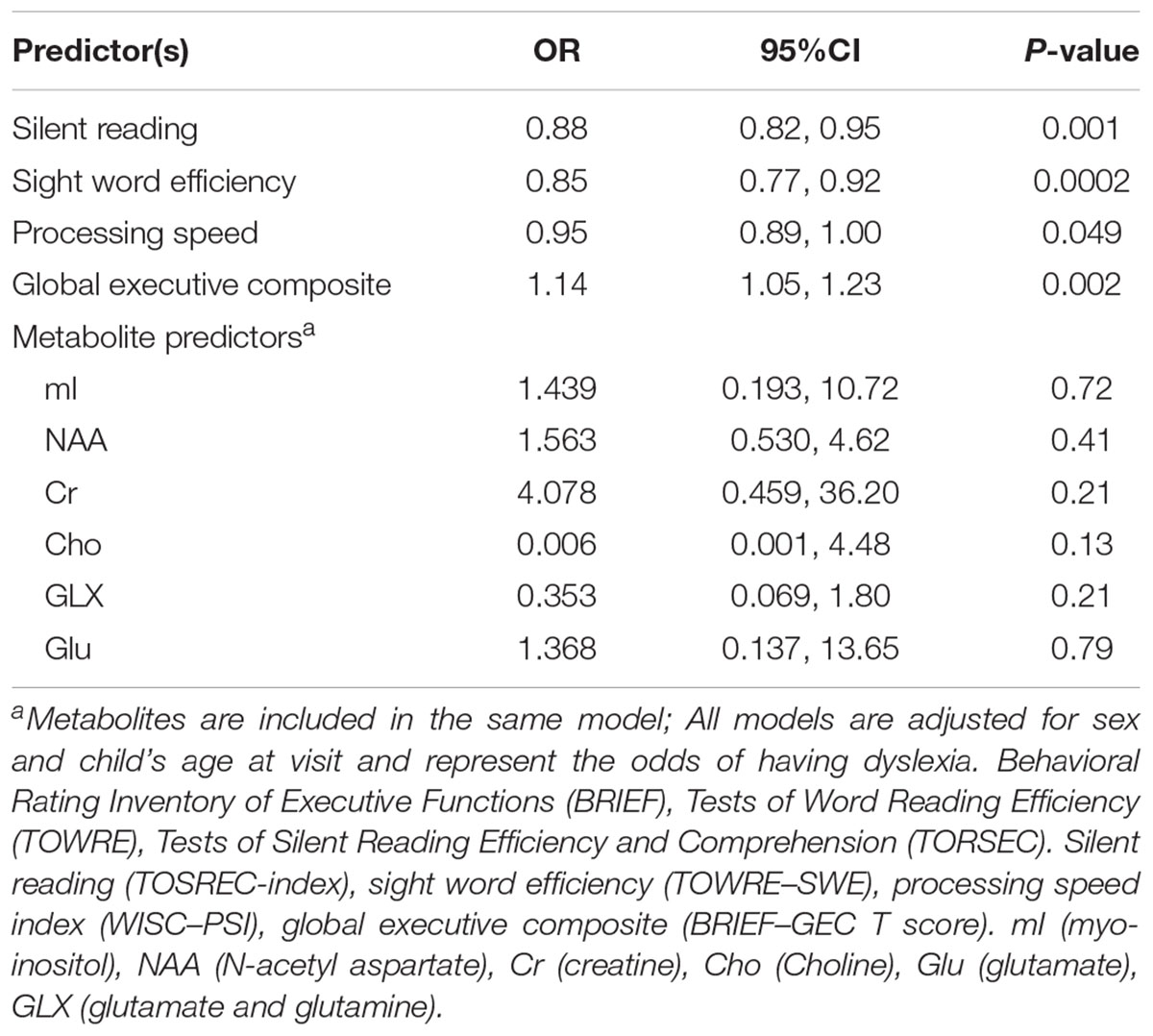
TABLE 4. Relationship between reading scales, processing speed, global executive function, and metabolite levels on dyslexia status.
Discussion
Over the past decade, multiple studies have implicated difficulties in executive functions for individuals with dyslexia. However, there is still a gap in knowledge as to the neurochemical characteristics related to reading difficulties in neural circuits associated with challenges in executive functions for this population. The current study aimed to characterize the metabolite concentrations related to reading ability in typical readers and in children with dyslexia, specifically focusing on the ACC. As hypothesized, lower reading ability was associated with high Cho levels, however, it was negatively associated with processing speed in females with dyslexia. The current study also demonstrated a negative relationship between processing speed and mI concentration. To our knowledge, this is among the first pediatric studies to look at MRS measures in the ACC and to report higher levels of Cho in the brain of females with dyslexia. Also, the study confirmed children, both boys and girls, with dyslexia demonstrated lower reading ability and executive functions scores compared to typical readers.
The study did not observe relationships of the cognitive and reading measures with other metabolites, such as NAA, Glu, or GLX, nor did it observe metabolite changes in males. In this study, metabolite concentrations were determined with corrections for metabolite and water relaxation along with contributions from different tissue types contributing to the voxel. Other studies report relative metabolite levels to one another, usually to creatine, and fail to account for differences in tissue contributions, which may explain observed differences. The voxel placement within the pregenual ACC in the current study matches the location described by Lebel et al. (2016) however, it differs from that of other studies of individuals with dyslexia focused on the occipital lobe including lingual gyrus, calcarine sulcus, and cuneus.
High Cho and Reading Difficulty
Previous studies have pointed at high Cho levels related to reading levels in the occipital lobe (Pugh et al., 2014; Del Tufo et al., 2018) in the cerebellum (Laycock et al., 2008) and the left temporoparietal region (Bruno et al., 2013). The authors suggested that these high Cho levels in individuals with reading difficulties for reading-related regions reflect high membrane turnover, cellular density, and white matter density. These results are in line with evidence of impaired myelination in this population in white matter tracts passing the temporoparietal regions and the occipital lobe (Yeatman et al., 2012; Wandell and Yeatman, 2013). Our study is believed to be among the first to reveal metabolite changes in regions that were not traditionally included as part of the classical reading circuitry. However, in recent years there are additional models pointing at the critical role of executive functions-related neural circuits in reading, including the ACC (Horowitz-Kraus et al., 2013, 2018; Horowitz-Kraus and Hutton, 2015). Our results provide additional evidence for the involvement of neural circuits related to executive functions in children with dyslexia extending the high Cho findings also to the ACC. The relationship to alterations in myelination (especially in the genu which passes though the ACC) should also be examined. As the ACC in a major hub in the cingulo-opercular network, (Dosenbach et al., 2008) it would be interesting to measure Cho levels in other sub-regions of the ACC (as outlined in) (de la Vega et al., 2016) and other brain regions that are related to the fronto-parietal network and the fast monitoring of cognitive processes also impaired in children with dyslexia.
Differences in mI Between Children With Dyslexia and Typical Readers
myo-inositol is a carbocyclic sugar molecule localized to glial cells. There are several known roles for mI in the brain. It is primarily considered a glial cell marker as it’s concentration increases with glial cell based neoplasms and gliosis. However, it also functions as an osmolyte such that during periods of osmotic stress, as balance is maintained via mI transport across plasma membranes. However, in the context of the current study with Cho findings, there is support for the role of mI as a key precursor of phosphoinositides and phospholipids, cell membranes and myelin structures. The composition of these structures within the brain networks could directly influence reading abilities.
Sex-Specific Findings
We were able to explore sex-based differences as eight of twenty-four children with dyslexia and seventeen of thirty typical readers in our study were females. Sex differences are known in many disorders, including ADHD, and dyslexia, with greater male frequency with the diagnosis (Shaywitz and Shaywitz, 2003a,b, 2008). Multiple genetic, and non-inherited factors have been posited to explain this observation. The sex hormones, estrogen and progesterone, demonstrate cognitive and neuroprotective effects (Brann et al., 2007; Dumitriu et al., 2010).
Arnett et al. (2017) concluded that the higher prevalence in males with reading difficulties can be explained by slower and more variable processing speed along with worse inhibitory control. Geschwind and Levitsky (1968) first reported structural differences and asymmetry in persons with dyslexia upon post-mortem brain examinations. Non-invasive neuroimaging investigations of brain structure have provided further insight into the neurobiological basis for sex-specific differences. Evans et al., found gray matter volume differences in adults and children, male and female with developmental dyslexia, specifically in the occipital lobe for girls (Evans et al., 2014).
Study’s Limitations
The results of this study should be considered in light of the following limitations. First, the sample size of this study is relatively small. Second, the perigenual placement of the MRS voxel within the ACC limited the ability to relate to executive functions of the ACC assigned to more posterior aspects, such as cognitive control and conflict monitoring. Third, technical differences in the MRS acquisition and quantitation of metabolite levels, as previously discussed, can influence the results. Also, the relationship between BOLD and functional connectivity and our findings should be validated in a future study using a joint MRS-fMRI model combining the results of these two methodologies.
Conclusion
The current study’s findings pinpoint metabolic differences related to the medial frontal lobe in females with dyslexia and typical readers. Further investigations are necessary to explore the metabolism and function of the ACC and how they influence reading abilities.
Author Contributions
All authors contributed to data analysis and manuscript writing.
Funding
This study was supported by the National Institute of Child Health and Human Development (R01 HD086011; PI: TH–K), the Cincinnati Children’s Hospital Medical Center Board of Trustees Award. Funding from the National Institute of Environmental Health Sciences for KB: P30 ES006096, R00 ES024116 and for KC: R01 ES027224, R01 ES026446.
Conflict of Interest Statement
The authors declare that the research was conducted in the absence of any commercial or financial relationships that could be construed as a potential conflict of interest.
References
Arnett, A. B., Pennington, B. F., Peterson, R. L., Willcutt, E. G., DeFries, J. C., and Olson, R. K. (2017). Explaining the sex difference in dyslexia. J. Child Psychol. Psychiatry 58, 719–727. doi: 10.1111/jcpp.12691
Barratt, W. (2006). Barratt Simplified Measure of Social Status (BSMSS). Terre Haute, IN: Indiana State University.
Brann, D. W., Dhandapani, K., Wakade, C., Mahesh, V. B., and Khan, M. M. (2007). Neurotrophic and neuroprotective actions of estrogen: basic mechanisms and clinical implications. Steroids 72, 381–405. doi: 10.1016/j.steroids.2007.02.003
Bruno, J. L., Lu, Z. L., and Manis, F. R. (2013). Phonological processing is uniquely associated with neuro-metabolic concentration. Neuroimage 67, 175–181. doi: 10.1016/j.neuroimage.2012.10.092
Byars, A. W., Holland, S. K., Strawsburg, R. H., Bommer, W., Dunn, R. S., Schmithorst, V. J., et al. (2002). Practical aspects of conducting large-scale functional magnetic resonance imaging studies in children. J. Child Neurol. 17, 885–890. doi: 10.1177/08830738020170122201
de la Vega, A., Chang, J., Banich, M. T., Wager, T. D., and Yarkoni, T. (2016). Large-scale meta-analysis of human medial frontal cortex reveals tripartite functional organization. J. Neurosci. 36, 6553–6562. doi: 10.1523/JNEUROSCI.4402-15.2016
Del Tufo, S. N., Frost, S. J., Hoeft, F., Cutting, L. E., Molfese, P. J., Mason, G. F., et al. (2018). Neurochemistry predicts convergence of written and spoken language: a proton magnetic resonance spectroscopy study of cross-modal language integration. Front. Psychol. 9:1507. doi: 10.3389/fpsyg.2018.01507
Dosenbach, N. U., Fair, D. A., Cohen, A. L., Schlaggar, B. L., and Petersen, S. E. (2008). A dual-networks architecture of top-down control. Trends Cogn. Sci. 12, 99–105. doi: 10.1016/j.tics.2008.01.001
Dumitriu, D., Rapp, P., McEwen, B., and Morrison, J. (2010). Estrogen and the aging brain: an elixir for the weary cortical network. Ann. N. Y. Acad. Sci. 1204, 104–112. doi: 10.1111/j.1749-6632.2010.05529.x
Erickson, K. L., Weinstein, A. M., Sutton, B. P., Prakash, R. S., Voss, M. W., Chaddock, L., et al. (2012). Beyond vascularization: aerobic fitness is associated with N-acetylaspartate and working memory. Brain Behav. 2, 32–41. doi: 10.1002/brb3.30
Evans, T. M., Flowers, D. L., Napoliello, E. M., and Eden, G. F. (2014). Sex-specific gray matter volume differences in females with developmental dyslexia. Brain Struct. Funct. 219, 1041–1054. doi: 10.1007/s00429-013-0552-4
Falkenstein, M., Hohnsbein, J., Hoormann, J., and Blanke, L. (1991). Effects of crossmodal divided attention on late ERP components. II. Error processing in choice reaction tasks. Electroencephalogr. Clin. Neurophysiol. 78, 447–455. doi: 10.1016/0013-4694(91)90062-9
Falkenstein, M., Hoormann, J., Christ, S., and Hohnsbein, J. (2000). ERP components on reaction errors and their functional significance: a tutorial. Biol. Psychol. 51, 87–107. doi: 10.1016/S0301-0511(99)00031-9
Geschwind, N., and Levitsky, W. (1968). Human brain: left-right asymmetries in temporal speech region. Science 161, 186–187. doi: 10.1126/science.161.3837.186
Gioia, G. A., Isquith, P. K., Retzlaff, P. D., and Espy, K. A. (2002). Confirmatory factor analysis of the behavior rating inventory of executive function (BRIEF) in a clinical sample. Child Neuropsychol. 8, 249–257. doi: 10.1076/chin.8.4.249.13513
Gussew, A., Erdtel, M., Hiepe, P., Rzanny, R., and Reichenbach, J. R. (2012). Absolute quantitation of brain metabolites with respect to heterogeneous tissue compositions in (1)H-MR spectroscopic volumes. MAGMA 25, 321–333. doi: 10.1007/s10334-012-0305-z
Horowitz-Kraus, T. (2011). Does development affect the error-related negativity of impaired and skilled readers? An ERP study. Dev. Neuropsychol. 36, 914–932. doi: 10.1080/87565641.2011.606415
Horowitz-Kraus, T. (2012). “The error detection mechanism among dyslexic and skilled readers: characterization and plasticity,” in Reading, Writing, Mathematics and the Developing Brain: Listening to Many Voices, Vol. 6, eds Z. Breznitz, O. Rubinsten, V. J. Molfese, and D. L. Molfese (Dordrecht: Springer), 113–130.
Horowitz-Kraus, T. (2014). Pinpointing the deficit in executive functions in adolescents with dyslexia performing the Wisconsin card sorting test: an ERP study. J. Learn. Disabil. 47, 208–223. doi: 10.1177/0022219412453084
Horowitz-Kraus, T., and Breznitz, Z. (2008). An error-detection mechanism in reading among dyslexic and regular readers–an ERP study. Clin. Neurophysiol. 119, 2238–2246. doi: 10.1016/j.clinph.2008.06.009
Horowitz-Kraus, T., and Breznitz, Z. (2013). Compensated dyslexics have a more efficient error detection system than noncompensated dyslexics. J. Child Neurol. 28, 1266–1276. doi: 10.1177/0883073812460917
Horowitz-Kraus, T., and Breznitz, Z. (2014). Can reading rate acceleration improve error monitoring and cognitive abilities underlying reading in adolescents with reading difficulties and in typical readers? Brain Res. 1544, 1–14. doi: 10.1016/j.brainres.2013.11.027
Horowitz-Kraus, T., DiFrancesco, M., Kay, B., Wang, Y., and Holland, S. K. (2015a). Increased functional connectivity of specific brain networks after reading training in dyslexic children. Clin. Neuroimage 8, 619–630. doi: 10.1016/j.nicl.2015.06.010
Horowitz-Kraus, T., Toro-Serey, C., and DiFrancesco, M. (2015b). Increased resting-state functional connectivity in the cingulo-opercular cognitive-control network after intervention in children with reading difficulties. PLoS One 10:e0133762. doi: 10.1371/journal.pone.0133762
Horowitz-Kraus, T., and Hutton, J. S. (2015). From emergent literacy to reading: how learning to read changes a child’s brain. Acta Paediatr. 104, 648–656. doi: 10.1111/apa.13018
Horowitz-Kraus, T., Hutton, J. S., Phelan, K., and Holland, S. K. (2018). Maternal reading fluency is positively associated with greater functional connectivity between the child’s future reading network and regions related to executive functions and language processing in preschool-age children. Brain Cogn. 121, 17–23. doi: 10.1016/j.bandc.2018.01.003
Horowitz-Kraus, T., Kiefer, A., DiCesare, C., and Dorrmann, D. (2016). Eye-Movements and Decreased Connectivity in Cognitive-Control Regions During Rest in Children. Geneva: Organization of Human Brain Mapping.
Horowitz-Kraus, T., Vannest, J. J., and Holland, S. K. (2013). Overlapping neural circuitry for narrative comprehension and proficient reading in children and adolescents. Neuropsychologia 51, 2651–2662. doi: 10.1016/j.neuropsychologia.2013.09.002
Horowitz-Kraus, T., Vannest, J. J., Kadis, D., Cicchino, N., Wang, Y. Y., and Holland, S. K. (2014). Reading acceleration training changes brain circuitry in children with reading difficulties. Brain Behav. 4, 886–902. doi: 10.1002/brb3.281
IDA (2011). Definition of Dyslexia- Based in the Initial Definition of the Research Committee of the Orton Dyslexia Society, Former Name of the IDA, Done in 1994. Baltimore, MD: International Dyslexia Association.
Jung, R. E., Haier, R. J., Yeo, R. A., Rowland, L. M., Petropoulos, H., Levine, A. S., et al. (2005). Sex differences in N-acetylaspartate correlates of general intelligence: an 1H-MRS study of normal human brain. Neuroimage Clin. 26, 965–972. doi: 10.1016/j.neuroimage.2005.02.039
Laycock, R., Crewther, C. D., and Crewther, S. G. (2008). The advantage in being magnocellular: a few more remarks on attention and the magnocellular system. Neurosci. Biobehav. Rev. 32, 1409–1415. doi: 10.1016/j.neubiorev.2008.04.008
Lebel, C., MacMaster, F. P., and Dewey, D. (2016). Brain metabolite levels and language abilities in preschool children. Brain Behav. 6:e00547. doi: 10.1002/brb3.623
Levinson, O., Hershey, A., Farah, R., and Horowitz-Kraus, T. (2018). Altered functional connectivity of the executive-functions network during a Stroop task in children with reading difficulties. Brain Connect. 8, 516–525. doi: 10.1089/brain.2018.0595
Miller, B. L., Chang, L., Booth, R., Ernst, T., Cornford, M., Nikas, D., et al. (1996). In vivo 1H MRS choline: correlation with in vitro chemistry/histology. Life Sci. 58, 1929–1935. doi: 10.1016/0024-3205(96)00182-8
Mohamed, M. A., Barkera, P. B., Skolasky, R. L., Selnesd, O. A., Moxleyd, R. T., Pompera, M. G., et al. (2010). Brain metabolism and cognitive impairment in HIV infection: a 3-T magnetic resonance spectroscopy study. Magn. Reson. Imaging 28, 1251–1257. doi: 10.1016/j.mri.2010.06.007
Murphy, D. G. M., Critchley, H. D., and Schmitz, N. (2002). Asperger syndrome a proton magnetic resonance spectroscopy study of brain. Arch. Gen. Psychiatry 59, 885–891. doi: 10.1001/archpsyc.59.10.885
Provencher, S. W. (1993). Estimation of metabolite concentrations from localized in vivo proton NMR spectra. Magn. Reson. Med. 30, 672–679. doi: 10.1002/mrm.1910300604
Pugh, K. R., Frost, S. J., Rothman, D. L., Hoeft, F., Del Tufo, S. N., Mason, G. F., et al. (2014). Glutamate and choline levels predict individual differences in reading ability in emergent readers. J. Neurosci. 34, 4082–4089. doi: 10.1523/JNEUROSCI.3907-13.2014
Pugh, K. R., Mencl, W. E., Jenner, A. R., Katz, L., Frost, S. J., Lee, J. R., et al. (2000). Functional neuroimaging studies of reading and reading disability (developmental dyslexia). Ment. Retard. Dev. Disabil. Res. Rev. 6, 207–213. doi: 10.1002/1098-2779(2000)6:3<207::AID-MRDD8>3.0.CO;2-P
Seidenberg, M. S., and McClelland, J. L. (1989). A distributed, developmental model of word recognition and naming. Psychol. Rev. 96, 523–568. doi: 10.1037/0033-295X.96.4.523
Shaywitz, S. E., and Shaywitz, B. A. (2003a). Dyslexia (specific reading disability). Pediatr. Rev. 24, 147–153. doi: 10.1542/pir.24-5-147
Shaywitz, S. E., and Shaywitz, B. A. (2003b). The science of reading and dyslexia. J. AAPOS 7, 158–166. doi: 10.1016/S1091-8531(03)00002-8
Shaywitz, S. E., and Shaywitz, B. A. (2008). Paying attention to reading: the neurobiology of reading and dyslexia. Dev. Psychopathol. 20, 1329–1349. doi: 10.1017/S0954579408000631
Shaywitz, S. E., Shaywitz, B. A., Fulbright, R. K., Skudlarski, P., Mencl, W. E., Constable, R. T., et al. (2003). Neural systems for compensation and persistence: young adult outcome of childhood reading disability. Biol. Psychiatry 54, 25–33. doi: 10.1016/S0006-3223(02)01836-X
Tal, A., Kirov, I. I., Grossman, R. I., and Gonen, O. (2012). The role of gray and white matter segmentation in quantitative proton MR spectroscopic imaging. NMR Biomed. 25, 1392–1400. doi: 10.1002/nbm.2812
Traber, F., Block, W., Lamerichs, R., Gieseke, J., and Schild, H. H. (2004). 1H metabolite relaxation times at 3.0 tesla: measurements of T1 and T2 values in normal brain and determination of regional differences in transverse relaxation. J. Magn. Reson. Imaging 19, 537–545. doi: 10.1002/jmri.20053
Wandell, B. A., and Yeatman, J. D. (2013). Biological development of reading circuits. Curr. Opin. Neurobiol. 23, 261–268. doi: 10.1016/j.conb.2012.12.005
Wansapura, J. P., Holland, S. K., Dunn, R. S., and Ball, W. S. Jr. (1999). NMR relaxation times in the human brain at 3.0 tesla. J. Magn. Reson. Imaging 9, 531–538. doi: 10.1002/(SICI)1522-2586(199904)9:4<531::AID-JMRI4>3.0.CO;2-L
Wechsler, D. (2014). Wechsler Intelligence Scale for Children, 5th Edn. San Antonio, TX: The Psychological Corporation.
Woodcock, R. W., and Johnson, M. B. (1989). Woodcock-Johnson Psycho-Educational Battery-Revised (WJ-R). Allen, TX: Developmental Learning Materials Teaching Resources.
Woolrich, M. W., Jbabdi, S., Patenaude, B., Chappell, M., Makni, S., Behrens, T., et al. (2009). Bayesian analysis of neuroimaging data in FSL. Neuroimage 45, S173–S186. doi: 10.1016/j.neuroimage.2008.10.055
Keywords: dyslexia, MRI, spectroscopy, reading, executive functions
Citation: Horowitz-Kraus T, Brunst KJ and Cecil KM (2018) Children With Dyslexia and Typical Readers: Sex-Based Choline Differences Revealed Using Proton Magnetic Resonance Spectroscopy Acquired Within Anterior Cingulate Cortex. Front. Hum. Neurosci. 12:466. doi: 10.3389/fnhum.2018.00466
Received: 25 July 2018; Accepted: 05 November 2018;
Published: 23 November 2018.
Edited by:
Guadalupe Dávila, Universidad de Málaga, SpainReviewed by:
Cyril R. Pernet, The University of Edinburgh, United KingdomMarcus Heldmann, University of Lübeck, Germany
Copyright © 2018 Horowitz-Kraus, Brunst and Cecil. This is an open-access article distributed under the terms of the Creative Commons Attribution License (CC BY). The use, distribution or reproduction in other forums is permitted, provided the original author(s) and the copyright owner(s) are credited and that the original publication in this journal is cited, in accordance with accepted academic practice. No use, distribution or reproduction is permitted which does not comply with these terms.
*Correspondence: Tzipi Horowitz-Kraus, VHppcGkuS3JhdXNAdGVjaG5pb24uYWMuaWw=