- 1Oxford Centre for Human Brain Activity, Wellcome Centre for Integrative Neuroimaging, Department of Psychiatry, University of Oxford, Oxford, United Kingdom
- 2FMRIB Centre, Wellcome Centre for Integrative Neuroimaging, Nuffield Department of Clinical Neurosciences, University of Oxford, Oxford, United Kingdom
- 3Translational Neuromodeling Unit, Institute for Biomedical Engineering, University of Zurich and ETH Zürich, Zurich, Switzerland
- 4Wellcome Centre for Human Neuroimaging, University College London, London, United Kingdom
- 5Max Planck Institute for Metabolism Research, Cologne, Germany
- 6Nuffield Department of Clinical Neurosciences, University of Oxford, Oxford, United Kingdom
Research on how humans perceive sensory inputs from their bodies (“interoception”) has been rapidly gaining momentum, with interest across a host of disciplines from physiology through to psychiatry. However, studying interoceptive processes is not without significant challenges, and many methods utilized to access internal states have been largely devoted to capturing and relating naturally occurring variations in interoceptive signals (such as heartbeats) to measures of how the brain processes these signals. An alternative procedure involves the controlled perturbation of specific interoceptive axes. This is challenging because it requires non-invasive interventions that can be repeated many times within a subject and that are potent but safe. Here we present an effective methodology for instigating these perturbations within the breathing domain. We describe a custom-built circuitry that is capable of delivering inspiratory resistive loads automatically and precisely. Importantly, our approach is compatible with magnetic resonance imaging (MRI) environments, allowing for the administration of complicated experimental designs in neuroimaging as increasingly required within developing fields such as computational psychiatry/psychosomatics. We describe the experimental setup for both the control and monitoring of the inspiratory resistive loads, and demonstrate its possible utilities within different study designs. This methodology represents an important step forward from the previously utilized, manually controlled resistive loading setups, which present significant experimental burdens with prolonged and/or complicated sequences of breathing stimuli.
Introduction
A fundamental aspect of complex beings is the sensation, perception, and control of the physical body. “Interoception” can be considered as the processing of sensory inputs from the body by the nervous system across both subconscious and conscious domains (Khalsa et al., 2017). However, surprisingly little is known about the dynamic interplay between the brain and body beyond homeostatic reflex control (Pezzulo et al., 2015; Stephan et al., 2016; Petzschner et al., 2017), and the burgeoning field of interoceptive research has its sights set on understanding brain–body axes for potential applicability to emotions, decision making, consciousness, and mental health (recently reviewed by Khalsa et al., 2017), to name a few. In this article, we propose a significant methodological advancement regarding the experimental requirements for investigating interoception in the respiratory domain.
In order to study the brain–body interface across interoception, we need to equip ourselves with tools for capturing (and potentially inducing) perturbations in interoceptive experiences. This may take the form of measuring and modeling natural fluctuations that occur within and across interoceptive domains, such as variations in heart rate (Pollatos et al., 2007; Pappens et al., 2014), breath-to-breath variability in respiration (Paulus, 2013; Vlemincx et al., 2013), or irregularities across the gastric cycle (Herbert et al., 2012). Alternatively, we can consider actively invoking changes in these systems in a controlled, timely and reversible manner. Such active disturbances are critical for testing hypotheses about the principles that govern interoception. For example, current theories postulate that interoception obeys the same hierarchical Bayesian principles as exteroception (Seth, 2013; Pezzulo et al., 2015; Stephan et al., 2016; Petzschner et al., 2017). A critical prediction of these theories concerns the occurrence of specific prediction error signals (interoceptive surprise), e.g., reflected by activity of the insula, in response to unexpected changes in sensory inputs from the body. Testing this prediction requires experimental perturbations that elicit controlled prediction errors in a trial-by-trial fashion. Active perturbations of bodily states are also important for clinical applications (Stewart et al., 2014; Berner et al., 2017), not least in computational psychiatry/psychosomatics where model-based assessment of interoceptive surprise in individual patients plays a central role in proposals for differential diagnostics (Stephan et al., 2016; Petzschner et al., 2017).
Methods to induce controlled perturbations of interoception have so far been trialed in the cardiac domain (via intra-venous administration of isoproterenol Hassanpour et al., 2016), with breathing (via inspiratory and/or expiratory resistance; Paulus et al., 2012; Hayen et al., 2015, 2017; Berner et al., 2017; Faull and Pattinson, 2017), with gastric and bladder disturbances (via inflation of inserted balloons; Wang et al., 2008), or via baroreceptor manipulation (Owens et al., 2018). While pharmacologically-induced cardiac alterations provide strong effects and appeal to the wide body of research on natural fluctuations in interoceptive heart-related signals (Critchley et al., 2013; Garfinkel et al., 2015, 2016a,b; Quadt et al., 2018; Petzschner et al., 2019), the time-related decays required for returning to baseline as well as the burden and risks associated with repeated pharmacological interventions within the same subject pose restrictions on widespread application of this method. Similarly, the use of both gastric/bladder balloons and baroreceptor manipulations face challenges with regard to patient comfort and acceptance.
Here, we pursue a different approach for perturbing interoception in a fashion that is controlled, safe, repeatable, and not too inconvenient for human participants. We focus on respiration, presenting an advanced circuitry for the automated administration of inspiratory resistances. This circuitry builds on a previously published magnetic resonance imaging (MRI)-compatible inspiratory resistance circuit (Faull et al., 2016, 2017; Faull and Pattinson, 2017), incorporating computer-controlled solenoid valves for timely commencement and elimination of resistance, and an electronically controlled, flow-mediated inspiratory valve device (POWERbreathe, IMT Technologies Ltd., Birmingham, United Kingdom) to allow for adjustable maximal resistances. While the previous methodology has been employed to measure the brain activity [via functional MRI (fMRI)] related to the conditioned anticipation and perception of inspiratory resistive loads, these advances significantly aid the development of complicated protocols required for a more detailed study of dynamic brain–body interactions (Paulus et al., 2019). These protocol requirements often call for accurately controlled, repeatable, and changeable administration of interoceptive perturbations, which are essential to amass the necessary statistical evidence for quantifying subject-specific processes involved in interoception such as dynamic predictions and learning (Rescorla et al., 1972).
Materials and Equipment
Previous Inspiratory Resistance Administration Circuitry
The previous circuitry that has been employed to manually administer inspiratory resistances within an MRI scanner (Faull et al., 2016, 2017; Faull and Pattinson, 2017) is outlined in Figure 1A. In this experimental setup, compressed and humidified medical air was delivered to the participant via a breathing system, whereby air flow was maintained at a rate to adequately allow free breathing and access to an available air reservoir (within a reservoir bag) of 2 L. When a (conditioned) visual cue appeared on the screen, the delivery of compressed air was manually halted via closure of the corresponding air flowmeters, allowing the reservoir bag to empty over the course of approximately 3–8 s (anticipation period), followed by the application of inspiratory resistance once the air reservoir was empty. Additionally, delivery of supplementary gas mixtures [oxygen (O2) and a carbon dioxide (CO2) mix of 10% CO2, 21% O2, balance nitrogen] was supplied to the participant, allowing for periods of manual gas mixture administration (during rest periods) to decorrelate changes in expired CO2 and O2 from periods of inspiratory resistance. For participant safety when using the system, a new anti-bacterial, anti-viral, single-use filter is used for each participant. As this single-use filter is located within the pathways of both inspiration and expiration through the circuit, the filter protects both the participant from the system and the system from the participant. Therefore, after each use the filter is disposed of and everything between the filter and participant (the scuba mouthpiece and one elbow connector) is disinfected.
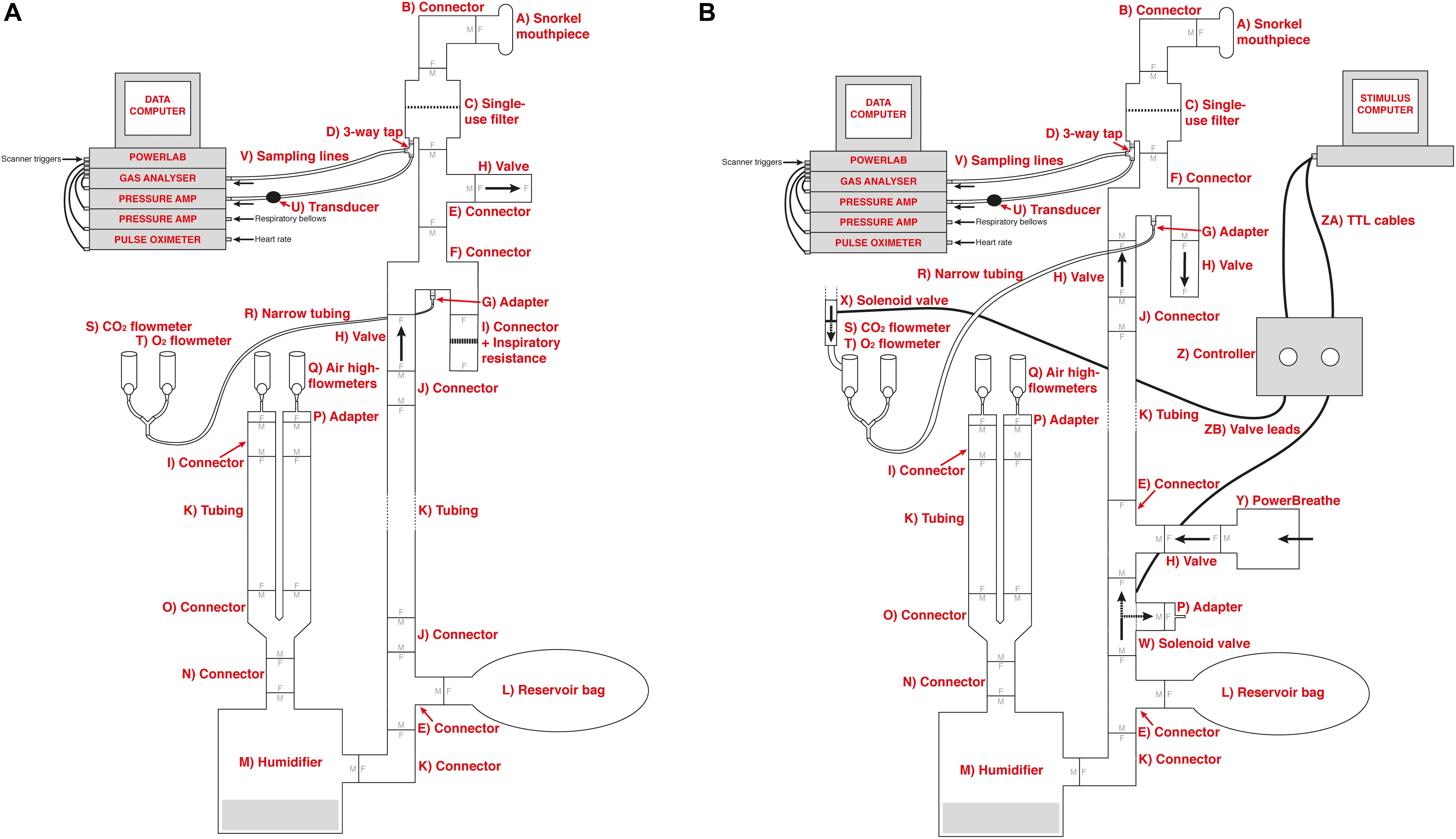
Figure 1. Schematic of the previously utilized inspiratory resistance circuit (Faull et al., 2016, 2017; Faull and Pattinson, 2017) (presented in (A)) and the new circuit design (B) that allows remote administrations of inspiratory resistance. In both systems, medical air is supplied to the subject, with a reservoir of 2 L. Excess flow and expiration escapes through a one-way valve (labeled H), close to the mouth to minimize rebreathing. A diving mouthpiece (labeled A) is connected to a bacterial and viral filter (labeled C), and sampling lines connect to a pressure transducer (labeled U) and amplifier (Pressure transducer indicator, PK Morgan Ltd., Kent, United Kingdom) for inspiratory pressure readings, and to a gas analyzer (via sampling line labeled V) (Gas Analyzer; ADInstruments Ltd., Oxford, United Kingdom) for respiratory gases. In (A), resistive inspiratory loading is induced by discontinuing the delivery of medical air (via the flowmeter and emptying of the reservoir bag), forcing the subject to draw air through the resistor (porous glass disc labeled I). In (B), resistive inspiratory loading is automatically achieved via the stimulus computer, whereby signals are sent through the parallel port to control valve 1 (labeled W) to redirect the supply of medical air to vent to the environment, forcing the subject to draw air through the POWERbreathe device (labeled Y). Periodically throughout scanning, small boluses of additional carbon dioxide (CO2) can be administered through manual control of the CO2 flowmeter (labeled S) in (A), or automatic control via valve 2 (labeled X) in (B), to raise the partial pressure of end-tidal CO2 (PETCO2) to match the PETCO2 rise induced by inspiratory loading periods. A final flowmeter (labeled T) is available for manual input of additional oxygen (O2) to the system. A full list of the labeled component parts can be found in the Supplementary Material.
Solenoid Valves
Within the new inspiratory resistance circuitry design (Figure 1B), two solenoid valves have been placed to allow for the automated and immediate application of inspiratory resistances, and administer small boluses of CO2 in a predetermined manner. Valve 1 (W in Figure 1B) is a wide-bore, direct-acting, 3/2-way solenoid valve (3/918-24/1002/R370-GN N.O. 24V DC, Buschjost Magnetventile GmbH & Co. KG, Germany) fitted with brass stem adaptors (MM052206N, John Guest Ltd.) to match the diameter of the air supply tubing, reducing the influence of any added baseline resistance to breathing. This valve is of the “normally open” type and will in its default state allow air to flow from the supply toward the mouthpiece. When actuated, the valve shuts off the port which supplies the mouthpiece and so initiates inspiratory loading. At the same time, the valve vents the medical air supply to the atmosphere to prevent overpressure. Valve 2 (X in Figure 1B) is a normally closed direct-acting 2/2-way valve (type 6013, Bürkert GmbH & Co. KG, Germany) that is inserted into the gas supply line for the CO2. This valve is closed by default, and when activated will open to allow the administration of the gas mixture for any adjustable length of time.
Solenoid Valve Control Box
The solenoid valves are operated via a custom-built control circuitry box (Figure 2), which can be interfaced with a presentation software program such as PsychToolBox (Borgo et al., 2012), or activated manually via a button press on the control box. The valve controller uses a 24 V DC 5 A power supply switched using optically coupled solid state (photoMOS) relays (AQZ102, Panasonic). Each output is connected to the supply via two parallel relays, one of which can be operated via a pushbutton, while the other one is energized directly from the TTL compatible control input. This allows standalone manual operation (without a PC connected) as well as manual override (i.e., manual operation with a PC connected), while ensuring the TTL inputs remain galvanically separated from the power supply and valve circuits. Kickback protection is achieved by the use of suitable solenoid valve leads (40881 Series, Murrelektronik).
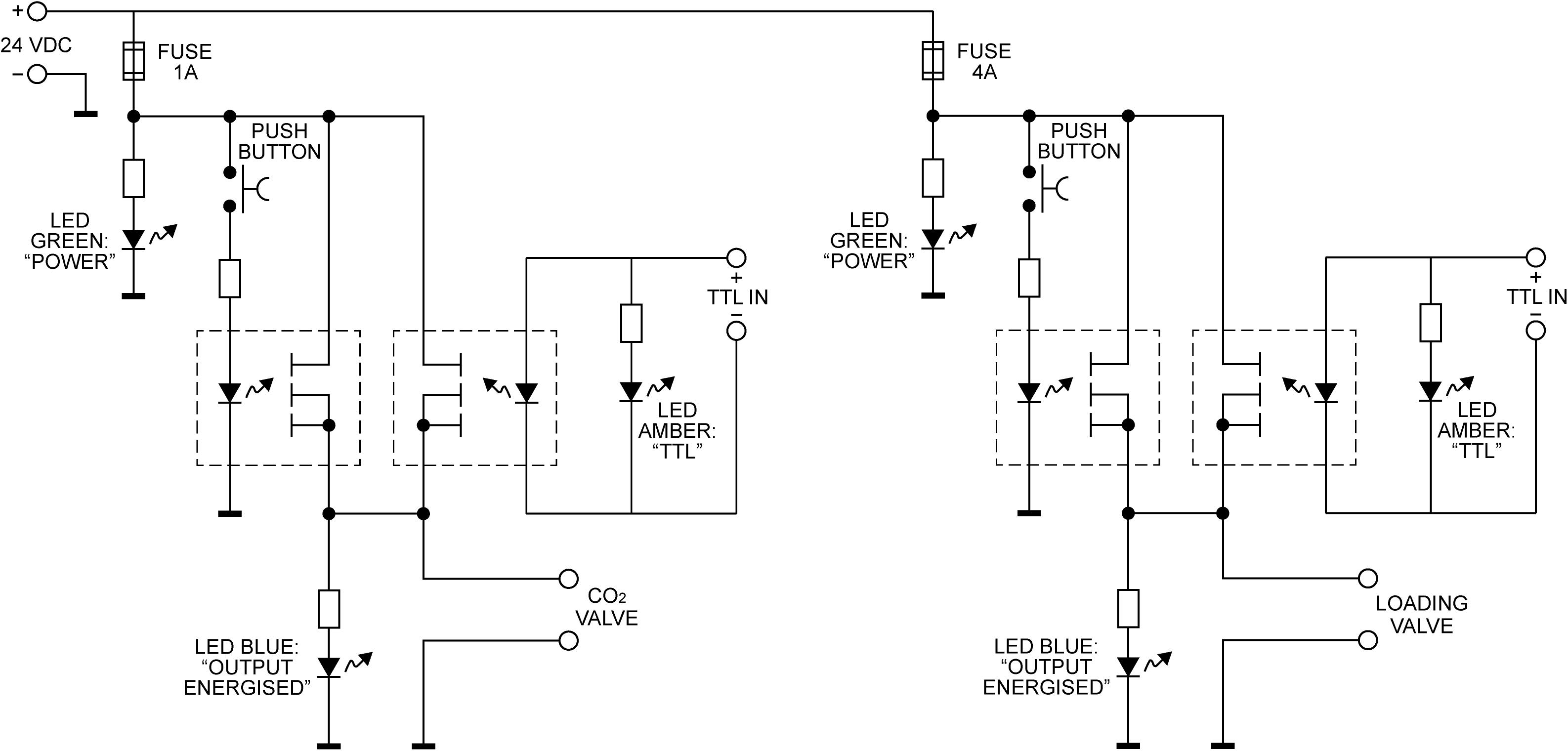
Figure 2. The valve controller circuit. Each of the two solenoid valves is switched using a pair of Panasonic AQZ102 optoFET relays connected in parallel, allowing them to be energized individually, either manually via the pushbutton, or from a TTL signal, while ensuring the TTL input remains galvanically separated from the control circuit. Power is supplied from a standard 24VDC power supply (Meanwell GST90A24-P1M, not shown). For additional protection from transients at the outputs, the valves are connected using cable assemblies with built in suppression (Murrelektronik 7000-40881-6360200, not shown).
To interface with PsychToolBox (or other presentation programs), simple commands can be sent via the parallel port of the presentation computer and be fed directly into the control box. This feature allows for completely automated resistance administration, which could either be using a predetermined, randomized, or online-updating sequence of stimuli. Sample code for control via the parallel port is provided in the Supplementary Material.
Adjustable Maximal Resistance
To allow for an adjustable maximal inspiratory resistance, the porous glass disc previously used to evoke an inspiratory resistance (Figure 1A) has been replaced with an electronically controlled, flow-mediated resistance valve (POWERbreathe, IMT Technologies Ltd., Birmingham, United Kingdom). This device can be set to a maximal inspiratory pressure value between 3 and 200 cmH2O using a variable aperture to create a static resistance to inspiratory flow. When the pressure generated by the participant inspiring against the POWERbreathe valve exceeds this set maximal pressure value, the valve is released. In addition, pressure at the mouth is measured within the breathing system, allowing a direct recording of the inspiratory pressure generated voluntarily against the external resistance by the participant. However, if a measure of inspiratory resistance would be required, and additional spirometer flowhead (and associated differential transducer; ADInstruments Ltd., Oxford, United Kingdom) could be easily inserted into the system between the filter (labeled C in Figure 1) and connector leading to inspiratory and expiratory valves (labeled F in Figure 1).
Methods
Example Participant
One example participant gave written, informed consent to demonstrate the use of this equipment. Ethical approval granted by the Zurich Ethics Committee.
Resistance Administration
This circuit design provides a platform from which a number of inspiratory resistance protocols are compatible and possible. One of the simpler methodologies would be to employ an on/off binary protocol, where the maximal resistance on the POWERbreathe is set to a percentage of each participant’s maximum inspiratory pressure (which can be tested using a standardized maximal inspiratory pressure protocol on the POWERbreathe device). To demonstrate this, we conducted physiological recordings during repeated applications of an inspiratory resistance value of 55 cmH2O, which was determined by calculating 70% of the participant’s maximal inspiratory pressure of 79 cmH2O. The participant was instructed to maintain normal breathing depth and rate during the resistive loading periods.
Alternatively, graded levels of inspiratory resistance could be employed via a pre-determined protocol input to the POWERbreathe, although visual feedback may be a necessity here to ensure participants reach the desired inspiratory pressure in each trial. To demonstrate this protocol, we repeatedly activated valve 1 with 5 cmH2O graded increases in set pressure from 5 to 30 cmH2O.
Administration of Additional Gas Mixtures
To demonstrate the addition of specific gas mixtures into the system, we conducted physiological recordings during the administration of small boluses of elevated CO2. To achieve these boluses, valve 2 was repeatedly activated for a short (∼1 s) duration, whereby a gas mixture containing 20% CO2, 21% O2 and the balance nitrogen was released into the system with a maximal flow-rate of 2 L/min (controlled by the flowmeter labeled “S” in Figure 1B). Any approved gas mixture could be added into the system for any specified duration, and example code to control each of the valves automatically is provided in the Supplementary Material.
Results
Resistance Administration
In Figure 3, we demonstrate example physiological traces recorded during the repeated activation of valve 1, and subsequent inspiratory resistance applications for a set value (here 55 cmH2O). This protocol allows a very clear perceptual distinction between the presence and absence of an inspiratory resistance, and could then be programmed and automated to fulfill the requirements of the research question at hand. Furthermore, as the pressure generated on each trial results from the inspiratory effort produced by the participant, either visual biofeedback could be employed to ensure pressure consistency in each trial, or alternatively the natural variability in both inspiratory pressure and perceptual ratings across trials could be used to tease apart differences in physiological vs. perceptual brain activity (for example). This example additionally demonstrates the perturbations in expired end-tidal carbon dioxide (PETCO2) that immediately follow each resistance stimulus. The relationship between the inspiratory pressure generated in each stimulus and the remaining physiological measures is demonstrated in Figure 4, whereby no significant associations were observed between inspiratory pressure and breathing rate, depth, PETCO2 nor PETO2 for this example participant. The differences between the average changes in physiological variables during constant inspiratory resistive loading and baseline rest periods are presented in Table 1, whereby inspiratory resistance induced significant increases in PETCO2 and decreases in PETO2, with marginal decreases in both breathing rate and depth in this example participant.
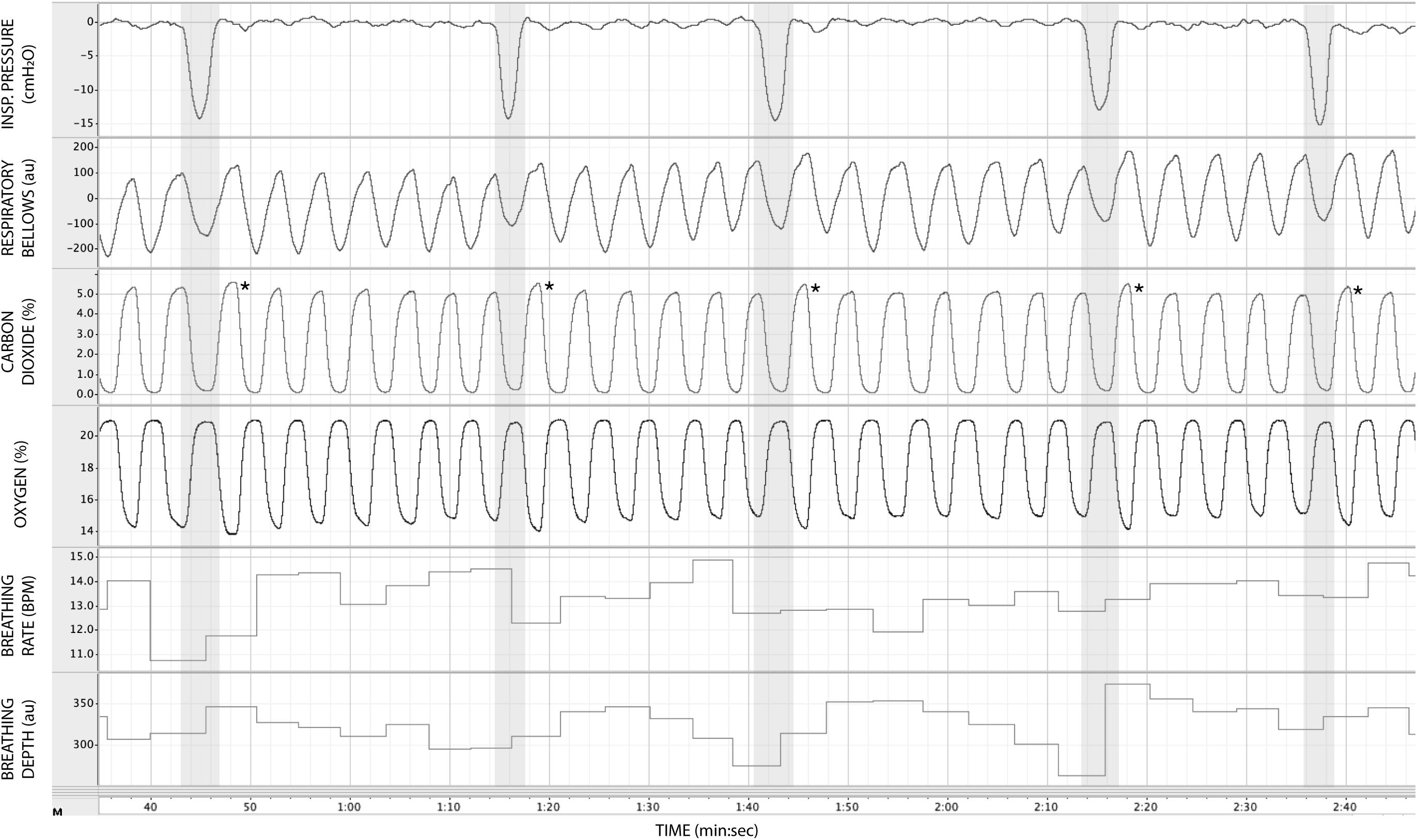
Figure 3. Physiological traces recorded during the application of five inspiratory resistance periods (gray areas), where the maximal inspiratory resistance was set at 55 cmH2O. Asterisks (*) denote an increase in expired pressure of end-tidal carbon dioxide (PETCO2—determined by the peak values at the end of each expiration in the CO2 trace) immediately following each inspiratory resistance period.
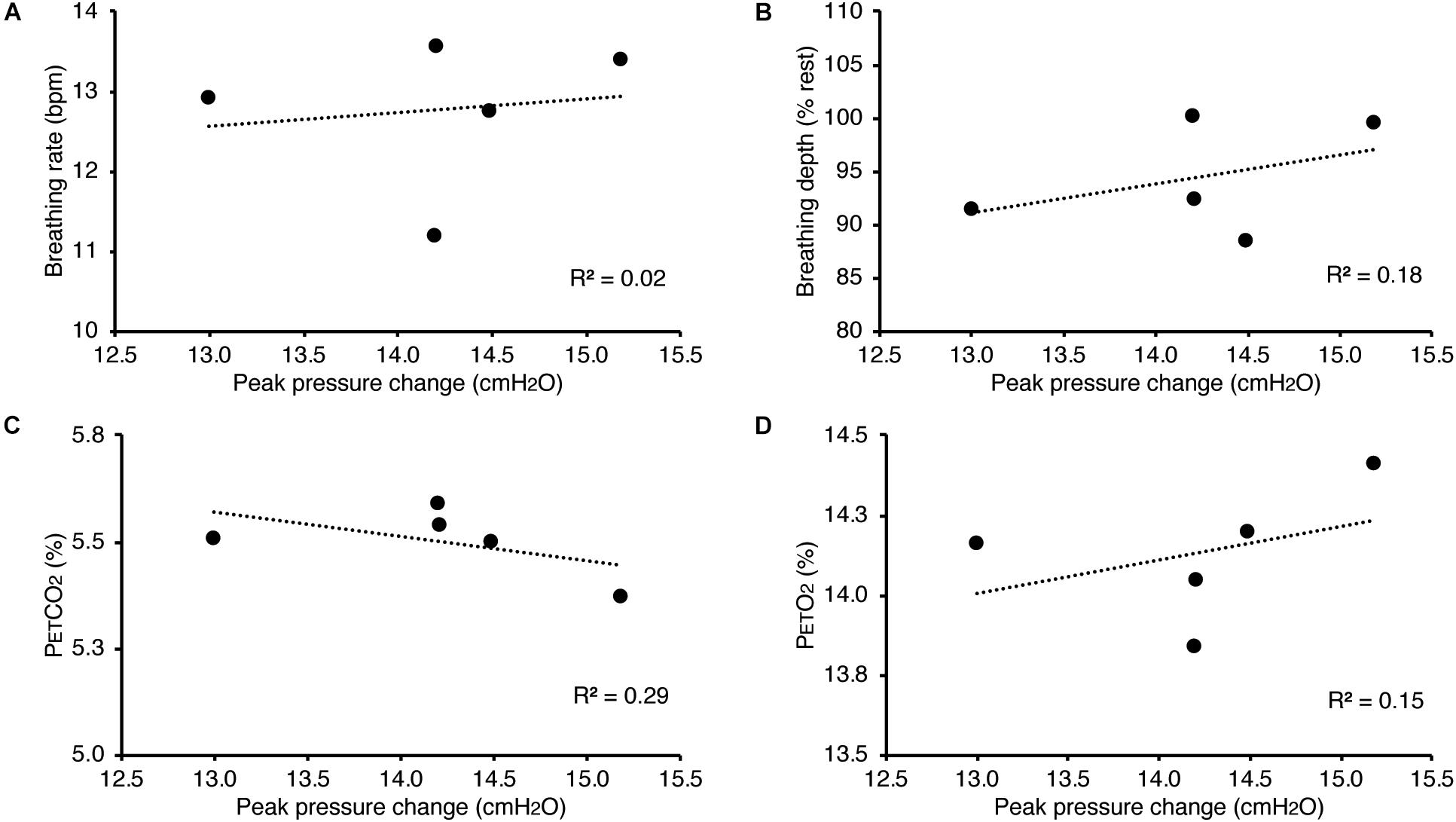
Figure 4. Relationship between the inspiratory pressures generated (from Figure 3) against a consistent inspiratory resistive load and the additional physiological variables measured. No significant relationships were found between the fluctuations in inspiratory pressure and breathing rate (A), breathing depth (measured as a change from resting values, B), pressure of end-tidal carbon dioxide (PETCO2, C), and pressure of end-tidal oxygen (PETO2, D).
In Figure 5, we present example results from a graded increase in inspiratory resistance across stimuli. Overcoming these resistances requires a matched graded increase in inspiratory muscular effort, and for greater resistances, this may be achieved by employing deeper and/or longer breaths. This strategy is exemplified in Figure 5, whereby the distention on the respiratory bellows increases with larger resistances, demonstrating an increase in the inspiratory depth required to overcome the set resistance and a matching compensatory decrease in breathing rate. The relationship between the increase in inspiratory pressure generated against these resistances and the remaining physiological measures is demonstrated in Figure 6 and Table 2, whereby significant associations were observed between inspiratory pressure and breathing rate, depth, PETCO2, and PETO2 in this example participant.
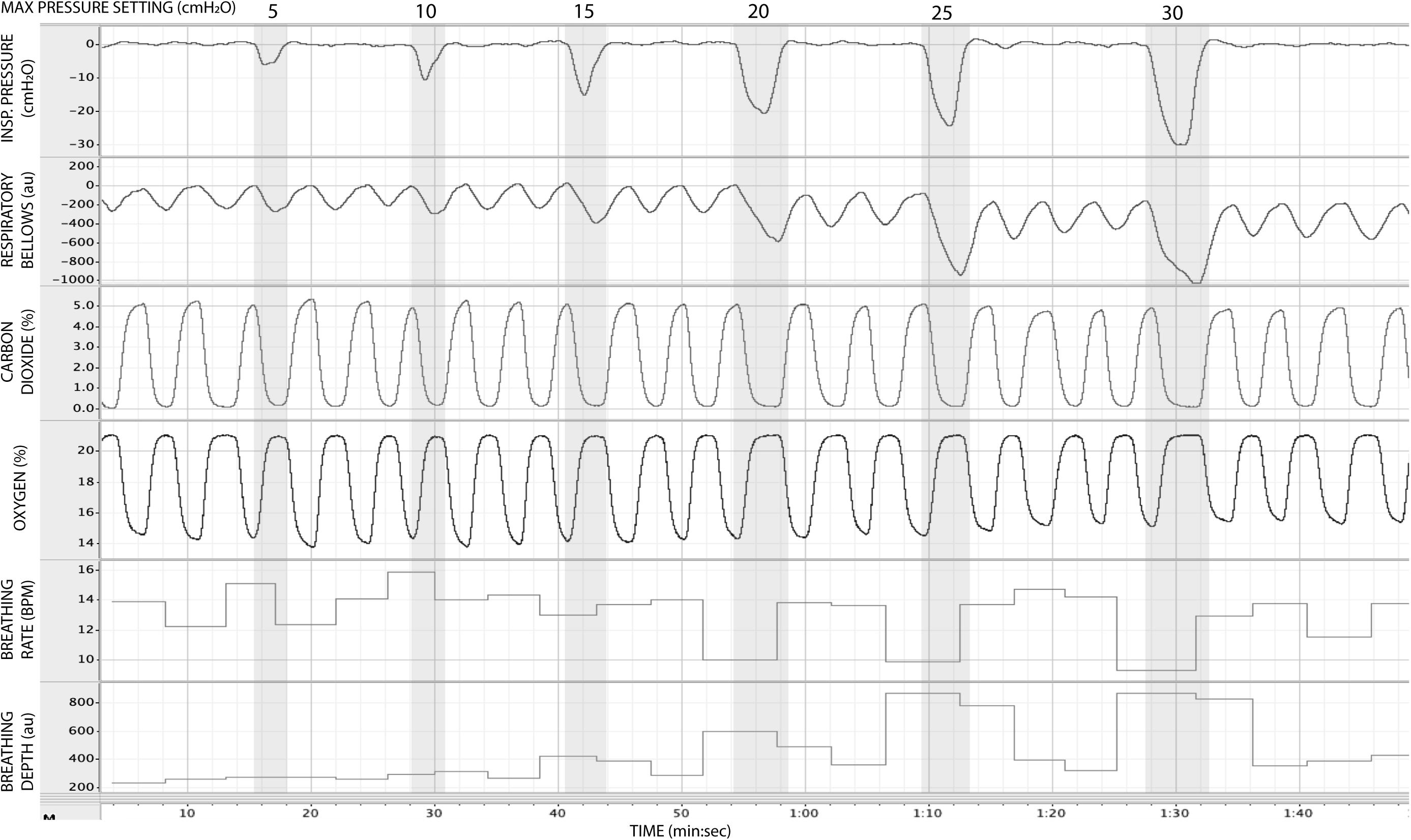
Figure 5. Physiological traces recorded during the application of five inspiratory resistance periods (gray areas), where the level of inspiratory resistance was graded at intervals of 5 cmH2O between 5 and 30 cmH2O.
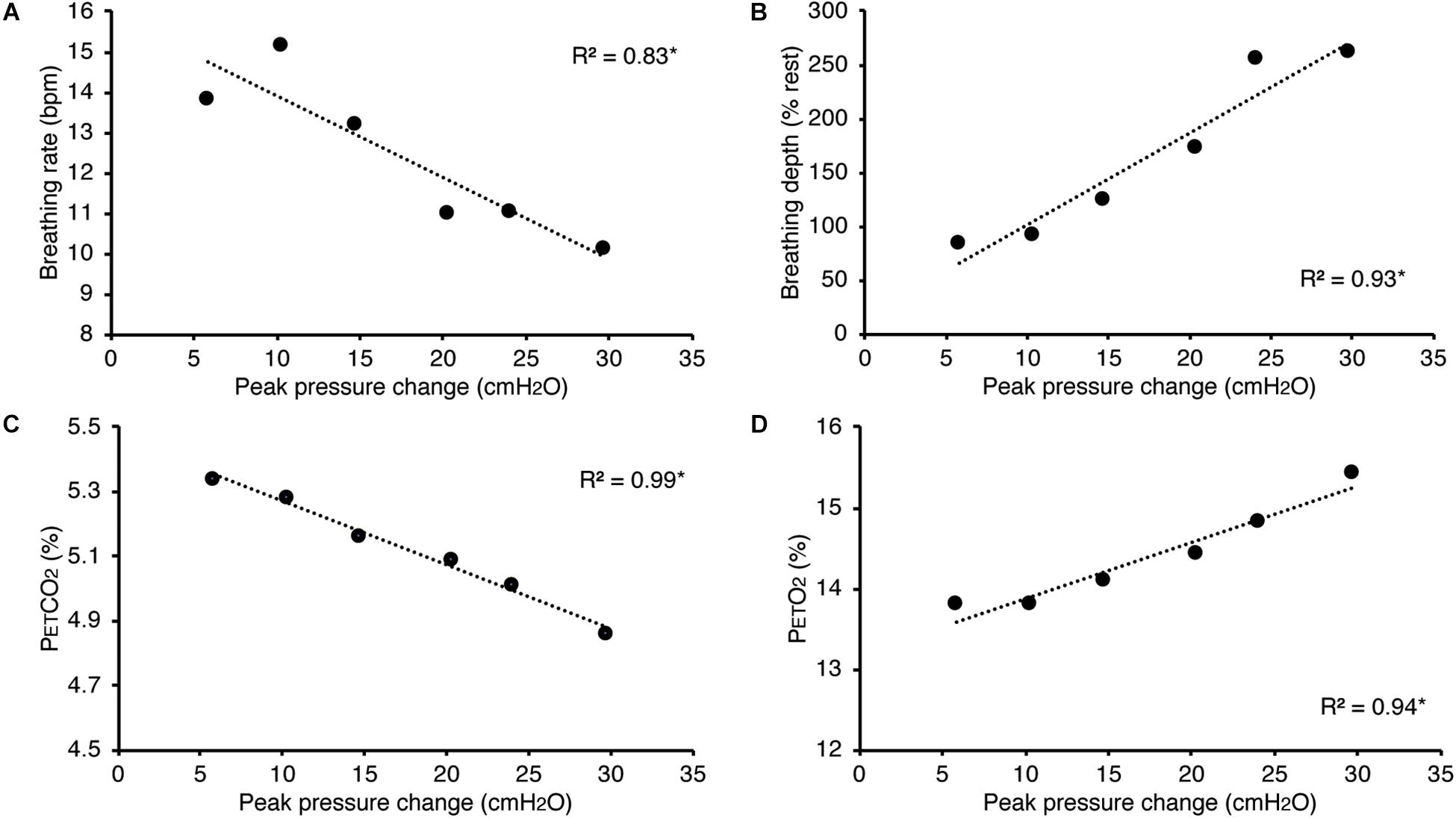
Figure 6. Relationship between the inspiratory pressures generated (from Figure 5) against a graded inspiratory resistive load and the additional physiological variables measured. Significant relationships (denoted by *) were found between graded inspiratory pressure and breathing rate (A), breathing depth (measured as a change from resting values, B), pressure of end-tidal carbon dioxide (PETCO2, C), and pressure of end-tidal oxygen (PETO2, D).
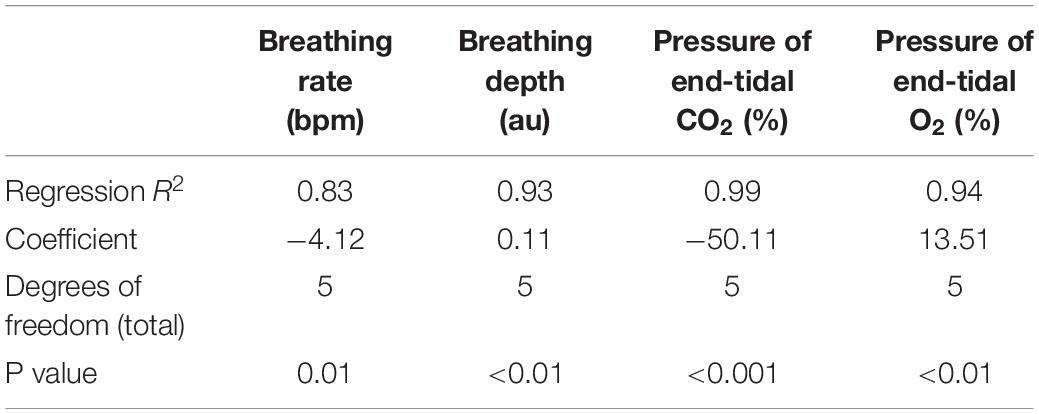
Table 2. Regression relationships between the inspiratory pressures generated (from Figures 5, 6) when inspiring against a graded inspiratory resistive load (X) and the additional physiological variables (Y) measured (included values were averaged across each inspiratory loading period).
Administration of Additional Gas Mixtures
In Figure 7, we demonstrate the physiological traces recorded during repeated activation of valve 2, here allowing the administration of a gas mixture containing elevated levels of CO2. Importantly, the end-tidal values of expired CO2 (the peak value at the end of expiration, highlighted in Figure 7) can be seen to rise and then recede over the course of 2–3 breaths, which could be extended by specifying a longer duration of administration of the gas mixture. It is also observed that the CO2 value between breaths does not return to baseline, as the partial pressure of the CO2 within the gas mixture has been temporarily increased. Minimal effects from this administration are observed in expired O2, as well as breathing rate and depth (results presented in Table 3).
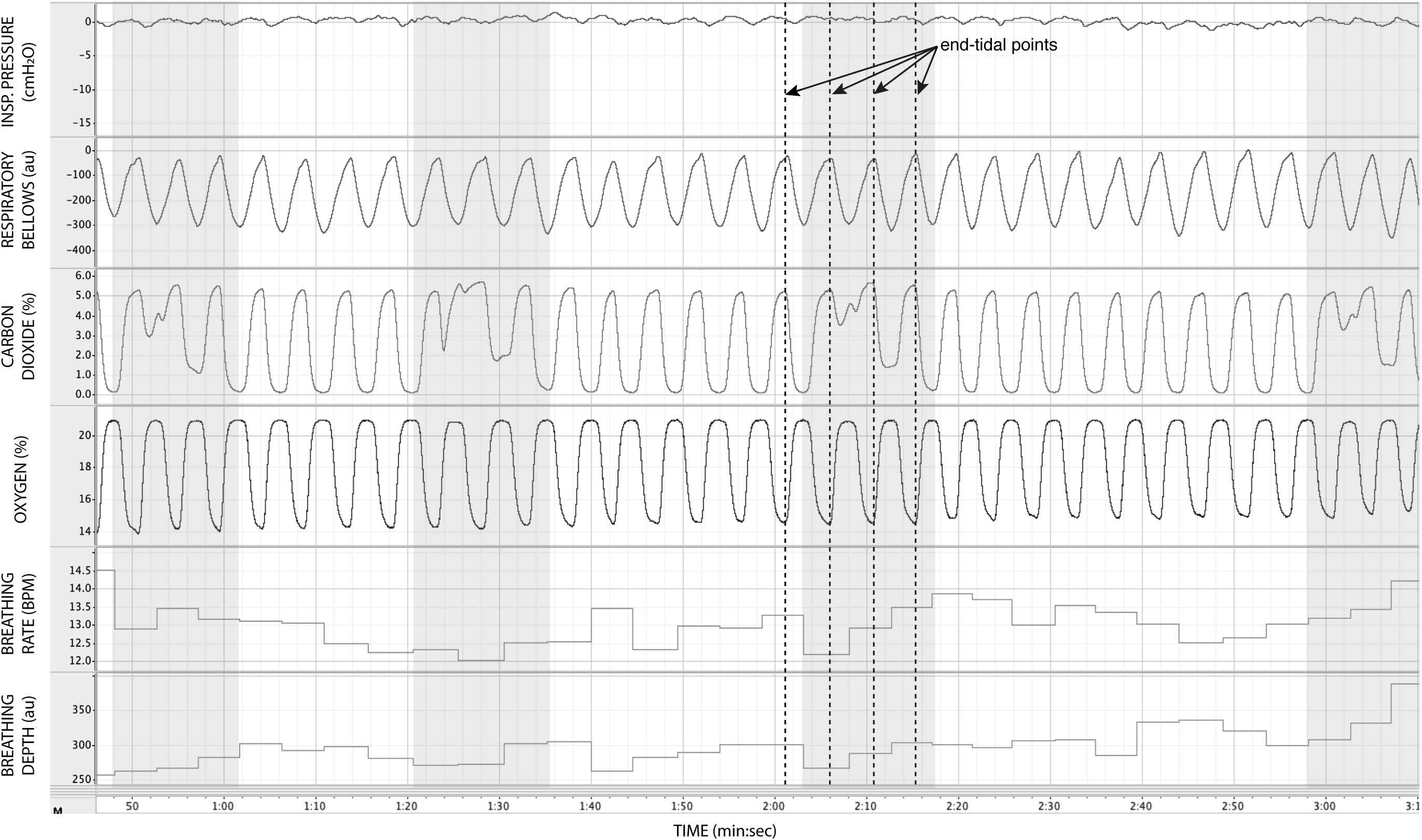
Figure 7. Physiological traces recorded during the application of four boluses of a carbon dioxide mixture (gray areas: 20% CO2, 21% O2, and the balance nitrogen) added to the medical air supply. Dotted lines represent example end-expiratory points, from which end-tidal CO2 and O2 values would be taken.
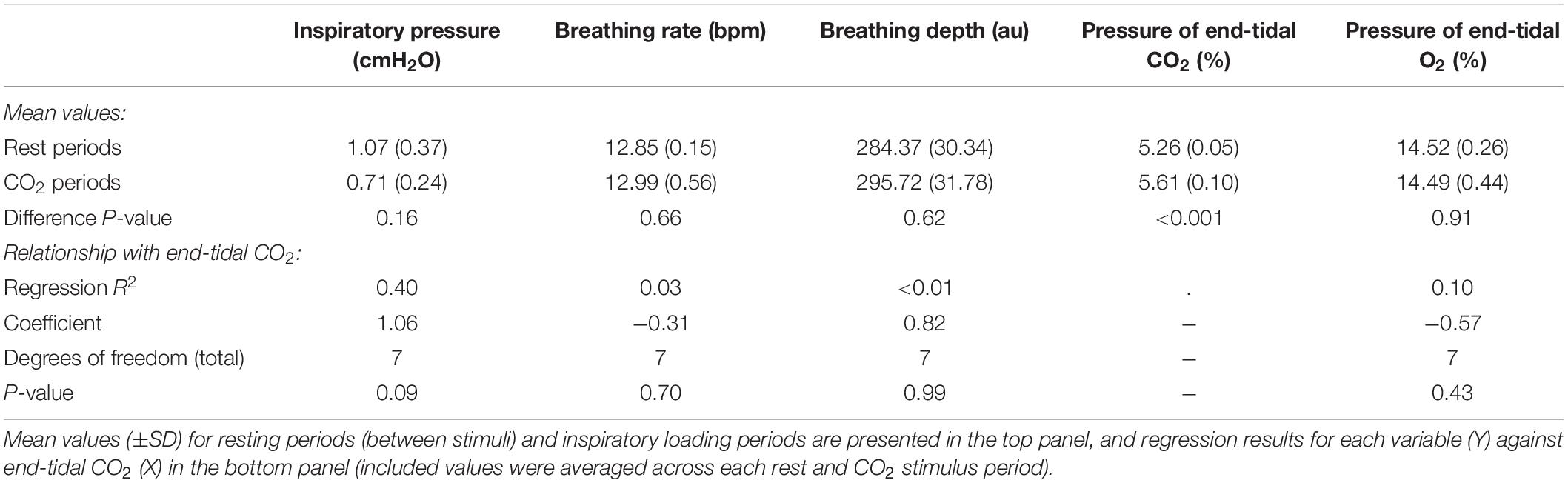
Table 3. Effect of adding small boluses of carbon dioxide (CO2) into the breathing circuit on the physiological variables measured.
Discussion
In this report, we have presented a custom-designed circuitry to administer automated, remote, timely, and repeated inspiratory resistances suitable for an MR environment. In this design, we have included the use of solenoid valves to control the administration of both inspiratory resistance and boluses of additional gas mixtures, which can be triggered either from a stimulus computer or by a manual button press on a custom-built circuitry box. This design represents a significant improvement from previously published methodologies (Faull et al., 2016, 2017; Faull and Pattinson, 2017), whereby inspiratory resistances were administered via manual closing of flowmeters and emptying of reservoir bags, both of which present substantial obstacles as the necessity of more complicated experimental designs beckons. As outlined in Section “Introduction,” methodologies such as the one described in this paper represent an essential step in our progression toward understanding the links between brain and body, and the importance of interoceptive processes across a wide range of neuroscientific domains.
The circuit design presented here has been specifically tailored to cater for the demands of an MRI environment. In this setting, where all equipment within the scanner room is required to be non-magnetic, the solenoid valves, electrical circuitry, gas flow control, and inspiratory resistance device can all be kept within the scanner control room, with flexible lengths of tubing allowing for the remote delivery of air supply and gas mixtures to scanners of variable distances from a control room port. All equipment within the scanner room is made of plastic or non-ferromagnetic materials, and both participants and the circuit are protected via a disposable, single-use bacterial and viral filter. Additionally, the sampling of gas and inspiratory pressures is fed back via sampling lines to monitoring devices that are also housed within the control room. To account for any gas sampling time-delays caused by the length of sampling line required, delays can simply be measured and the temporal shift incorporated into the analysis of any recorded physiological traces.
The advances made in this circuit design allow for implementation of a wide number of experimental procedures and inspiratory resistance protocols. While simple, binary protocols (such as the presence or absence of a noticeable inspiratory resistance) are one option, graded resistances are also possible with the use of the POWERbreathe device. Notably, the resistance to breathing set by the POWERbreathe does not necessarily determine the inspiratory pressure generated by the participant (recorded at the mouth), but rather a maximal pressure against which they inspire. As only initial ideas, we would postulate that this experimental setup could be utilized in one of three ways, namely: (1) using graded inspiratory resistances (within a limited range), where the participant is instructed to inspire using enough force to overcome the maximal pressure set on each trial (ensuring the external POWERbreathe inspiratory pressures are reached), (2) using a static (low or moderate) inspiratory pressure at the POWERbreathe, with participant instructions in the same manner as option 1 to ensure consistent pressures are generated at each trial, or (3) using a static, insurmountable pressure setting on the POWERbreathe, and simply measuring the natural variability that is inherent in the (voluntarily generated) inspiratory pressure readings (as demonstrated in Figure 3).
Different experimental options would need to be carefully considered in light of the specific study aim and also the potential confounding variables that each may entail. As can be seen from the example physiological traces provided in Figure 6, employing graded resistances almost perfectly induces corresponding changes in breathing rate and depth (to overcome the resistance), and expired PETCO2 and PETO2 in this instance. However, the relationship between these variables will be specific to the protocol used and the voluntary breathing actions taken by the participants. Therefore, the statistical analyses presented here are intended as a guide for how these could be assessed in different experimental scenarios, rather than a reference. While the system has methods for removing brain signal associated with pressure of expired gases (discussed below), the changes in breathing rate and depth may induce correlated head motion and associated image artifacts (if used in conjunction with brain imaging measures). Although established methods exist to remove physiological noise from brain imaging data (Brooks et al., 2013) (such as physiological noise modeling (PNM) in FSL; Brooks et al., 2008, and PhysIO for SPM; Kasper et al., 2017), this regression may partially remove neural signals of interest. While option 2 would allow a more constant change in corresponding physiology across trials, the latter option 3 has the potential advantage of decorrelating physiological changes from the perception of resistance to inspiration. As the interoceptive signal induced by this design will be composed of both the strength of the external resistance (i.e., how difficult it feels to inhale), as well as the motor recruitment response employed to overcome or inspire against that resistance, the dynamic nature of the latter may let us decorrelate the physiological artifacts from the static perception of the inspiratory resistance. In this scenario, regressing out the physiological artifacts (such as breathing rate and depth, head motion, etc.) would still leave the brain signal associated with the difficulty of inspiring against the resistance. Furthermore, the variability within the physiological signals could be compared to additional measures such as subjective perceptual ratings of work, effort, and/or anxiety across trials, allowing us to better understand which aspects of the voluntary motoric response may be associated with different aspects of interoceptive perception. However, one final note is that, due to the voluntary input into breathing circuitry, it is also possible that participants may choose to hold their breath and miss the interoceptive stimulus entirely. This scenario can be detected by both the inspiratory pressure and breathing belt traces, and we highly recommend collecting a rating of breathing difficulty (or similar) following each stimulus for confirmation of this event. These stimuli could then be accounted for in any analysis of behavioral and/or brain imaging data.
The design of this circuit also provides a means to tackle one of the key issues when utilizing breathing-related tasks in an fMRI protocol: Namely, the disturbances in blood gas concentrations and their subsequent effects on the blood oxygen level-dependent (BOLD) signal that is the fundament of most functional imaging sequences. The partial pressure of CO2 (PCO2) in the blood is a potent vasodilator, and tasks that induce changes in blood gases via altering natural breathing rhythms can thus either wash-out BOLD signal (via increases in PCO2) or induce global gray-matter vasoconstriction (via decreases in PCO2) that may interact non-linearly with the neurally induced BOLD response (Chang and Glover, 2009). Additionally, changes in the partial pressure of oxygen (PO2) in the blood also play a vasodilatory role; however, the magnitude of this response is considerably smaller than that induced by PCO2 (Chang and Glover, 2009). In this circuit design, we are first able to remotely measure the changes in expired CO2 and O2, whereby the end-tidal values are assumed to be indicative of alveolar gas (which closely parallels arterial carbon dioxide levels under normal ventilation-perfusion matching; Phan et al., 1987). Second, solenoid-valve controlled boluses of additional CO2 can also be delivered whenever required, for example, in some rest periods of the protocol, which allows the changes in expired gas to be decorrelated from the breathing task (Faull et al., 2015). However, it should be noted that maintenance of stable end-tidal gas measurements via end-tidal forcing (Wise et al., 2007) has not currently been incorporated into this system, although this could be integrated in place of the binary solenoid CO2 valve if necessary.
Conclusion
Here we have presented a circuitry that has been designed to automatically administer periods of inspiratory resistance in a remote environment (such as an MRI scanner), allowing the potential instigation of more complex paradigms that probe the relationship between brain and body. However, while breathing represents a somewhat more easily accessible avenue in which to study interoception, the changes in blood gas pressures that often result from breathing tasks cannot be overlooked. Therefore, in this design, we have incorporated methods for both recording and decorrelating fluctuations in the pressure of expired CO2 and O2, as a representation of fluctuations in arterial gas pressures. Lastly, the use of an electronically controlled inspiratory resistance device allows for specification of a ceiling of maximal inspiratory resistances, although careful use of biofeedback may be necessary to ensure graded levels of pressure are reached by the participant on each trial if required. We hope that this circuit design may aid the more nuanced study of brain–body interactions and interoception in the respiratory domain.
Data Availability Statement
The datasets generated for this study are available on request to the corresponding author.
Ethics Statement
The studies involving human participants were reviewed and approved by Kanton Zürich Kantonale Ethikkommission. The patients/participants provided their written informed consent to participate in this study.
Author Contributions
SR designed and built the automated valve system and contributed to the drafting and editing of the manuscript. KS oversaw the design and production of all methodological equipment and contributed to the drafting and editing of the manuscript. OH contributed to the design and production of all methodological equipment and contributed to the drafting and editing of the manuscript.
Funding
KS was supported by the René and Susanne Braginsky Foundation and the University of Zurich. OH (née Faull) is a Marie Skłodowska-Curie Postdoctoral Fellow, supported by the European Union’s Horizon 2020 Research and Innovation Program under Grant Agreement No. 793580.
Conflict of Interest
The authors declare that the research was conducted in the absence of any commercial or financial relationships that could be construed as a potential conflict of interest.
Acknowledgments
The authors would like to acknowledge Kyle Pattinson and the wider Wellcome Centre for Integrative Neuroimaging (Oxford) for their methodological expertise regarding the initial circuitry used, and for their support of SR. This manuscript has been released as a pre-print at BioRxiv.org (https://www.biorxiv.org/content/10.1101/2019.12.09.869511v1) (Rieger et al.). The authors would also like to thank Heidi Brunner for all her work organizing the acquisition of the components of the breathing system described in this manuscript.
Supplementary Material
The Supplementary Material for this article can be found online at: https://www.frontiersin.org/articles/10.3389/fnhum.2020.00161/full#supplementary-material
References
Berner, L. A., Simmons, A. N., Wierenga, C. E., Bischoff-Grethe, A., Paulus, M. P., Bailer, U. F., et al. (2017). Altered interoceptive activation before, during, and after aversive breathing load in women remitted from anorexia nervosa. Psychol. Med. 48, 142–154. doi: 10.1017/S0033291717001635
Borgo, M., Soranzo, A., and Grassi, M. (2012). “Psychtoolbox: sound, keyboard and mouse,” in MATLAB for Psychologists (New York, NY: Springer), 249–273.
Brooks, J. C. W., Beckmann, C. F., Miller, K. L., Wise, R. G., Porro, C. A., Tracey, I., et al. (2008). Physiological noise modelling for spinal functional magnetic resonance imaging studies. Neuroimage 39, 680–692. doi: 10.1016/j.neuroimage.2007.09.018
Brooks, J. C. W., Faull, O. K., Pattinson, K. T. S., and Jenkinson, M. (2013). Physiological noise in brainstem FMRI. Front. Hum. Neurosci. 7:623. doi: 10.3389/fnhum.2013.00623
Chang, C., and Glover, G. H. (2009). Relationship between respiration, end-tidal CO2, and BOLD signals in resting-state fMRI. Neuroimage 47, 1381–1393. doi: 10.1016/j.neuroimage.2009.04.048
Critchley, H. D., Eccles, J., and Garfinkel, S. N. (2013). Interaction between cognition, emotion, and the autonomic nervous system. Handb. Clin. Neurol. 117, 59–77. doi: 10.1016/b978-0-444-53491-0.00006-7
Faull, O. K., Cox, P. J., and Pattinson, K. T. S. (2017). Cortical processing of breathlessness in the athletic brain. bioRxiv [preprint]. doi: 10.1101/174052
Faull, O. K., Jenkinson, M., Clare, S., and Pattinson, K. T. S. (2015). Functional subdivision of the human periaqueductal grey in respiratory control using 7 tesla fMRI. Neuroimage 113, 356–364. doi: 10.1016/j.neuroimage.2015.02.026
Faull, O. K., Jenkinson, M., Ezra, M., and Pattinson, K. T. S. (2016). Conditioned respiratory threat in the subdivisions of the human periaqueductal gray. elife 5:e12047. doi: 10.7554/eLife.12047
Faull, O. K., and Pattinson, K. T. (2017). The cortical connectivity of the periaqueductal gray and the conditioned response to the threat of breathlessness. eLife 6:95. doi: 10.7554/eLife.21749
Garfinkel, S. N., Manassei, M. F., Hamilton-Fletcher, G., In den Bosch, Y., Critchley, H. D., and Engels, M. (2016a). Interoceptive dimensions across cardiac and respiratory axes. Philos. Trans. R. Soc. Lond., B, Biol. Sci. 371:14. doi: 10.1098/rstb.2016.0014
Garfinkel, S. N., Seth, A. K., Barrett, A. B., Suzuki, K., and Critchley, H. D. (2015). Knowing your own heart: distinguishing interoceptive accuracy from interoceptive awareness. Biol. Psychol. 104, 65–74. doi: 10.1016/j.biopsycho.2014.11.004
Garfinkel, S. N., Tiley, C., O’Keeffe, S., Harrison, N. A., Seth, A. K., and Critchley, H. D. (2016b). Discrepancies between dimensions of interoception in autism: implications for emotion and anxiety. Biol. Psychol. 114, 117–126. doi: 10.1016/j.biopsycho.2015.12.003
Hassanpour, M. S., Yan, L., Wang, D. J. J., Lapidus, R. C., Arevian, A. C., Simmons, W. K., et al. (2016). How the heart speaks to the brain: neural activity during cardiorespiratory interoceptive stimulation. Philos. Trans. R. Soc. B Biol. Sci. 371, 20160017–20160018. doi: 10.1098/rstb.2016.0017
Hayen, A., Herigstad, M., Wiech, K., and Pattinson, K. T. (2015). Subjective evaluation of experimental dyspnoea: effects of isocapnia and repeated exposure. Resp. Physiol. Neurobiol. 208, 21–28. doi: 10.1016/j.resp.2014.12.019
Hayen, A., Wanigasekera, V., Faull, O. K., Campbell, S. F., Garry, P. S., Raby, S. J. M., et al. (2017). Opioid suppression of conditioned anticipatory brain responses to breathlessness. Neuroimage 150, 383–394. doi: 10.1016/j.neuroimage.2017.01.005
Herbert, B. M., Muth, E. R., Pollatos, O., and Herbert, C. (2012). Interoception across modalities: on the relationship between cardiac awareness and the sensitivity for gastric functions. PLoS One 7:e36646. doi: 10.1371/journal.pone.0036646
Kasper, L., Bollmann, S., Diaconescu, A. O., Hutton, C., Heinzle, J., Iglesias, S., et al. (2017). The PhysIO toolbox for modeling physiological noise in fMRI data. J. Neurosci. Methods 276, 56–72. doi: 10.1016/j.jneumeth.2016.10.019
Khalsa, S. S., Adolphs, R., Cameron, O. G., Critchley, H. D., Davenport, P. W., Feinstein, J. S., et al. (2017). Interoception and mental health: a roadmap. Biol. Psychiatry Cogn. Neurosci. Neuroimaging 3, 501–513. doi: 10.1016/j.bpsc.2017.12.004
Owens, A. P., Friston, K. J., Low, D. A., Mathias, C. J., and Critchley, H. D. (2018). Investigating the relationship between cardiac interoception and autonomic cardiac control using a predictive coding framework. Autonom. Neurosci. Basic Clin. 210, 65–71. doi: 10.1016/j.autneu.2018.01.001
Pappens, M., Schroijen, M., Sütterlin, S., Smets, E., Van den Bergh, O., Thayer, J. F., et al. (2014). Resting heart rate variability predicts safety learning and fear extinction in an interoceptive fear conditioning paradigm. PLoS One 9:e105054. doi: 10.1371/journal.pone.0105054
Paulus, M. P. (2013). The breathing conundrum - Interoceptive sensitivity and anxiety. Depress. Anxiety 30, 315–320. doi: 10.1002/da.22076
Paulus, M. P., Feinstein, J. S., and Khalsa, S. S. (2019). An active inference approach to interoceptive psychopathology. Annu. Rev. Clin. Psychol. 15, 97–122. doi: 10.1146/annurev-clinpsy-050718-095617
Paulus, M. P., Flagan, T., Simmons, A. N., Gillis, K., Kotturi, S., Thom, N., et al. (2012). Subjecting elite athletes to inspiratory breathing load reveals behavioral and neural signatures of optimal performers in extreme environments. PLoS One 7:e29394. doi: 10.1371/journal.pone.0029394
Petzschner, F. H., Weber, L. A., Wellstein, K. V., Paolini, G., Do, C. T., and Stephan, K. E. (2019). Focus of attention modulates the heartbeat evoked potential. Neuroimage 186, 595–606. doi: 10.1016/j.neuroimage.2018.11.037
Petzschner, F. H., Weber, L. A. E., Gard, T., and Stephan, K. E. (2017). Computational psychosomatics and computational psychiatry: toward a joint framework for differential diagnosis. Biol. Psychiatry 82, 421–430. doi: 10.1016/j.biopsych.2017.05.012
Pezzulo, G., Rigoli, F., and Friston, K. (2015). Active Inference, homeostatic regulation and adaptive behavioural control. Prog. Neurobiol. 134, 17–35. doi: 10.1016/j.pneurobio.2015.09.001
Phan, C. Q., Tremper, K. K., Lee, S. E., and Barker, S. J. (1987). Noninvasive monitoring of carbon dioxide: a comparison of the partial pressure of transcutaneous and end-tidal carbon dioxide with the partial pressure of arterial carbon dioxide. J. Clin. Monit. Comput. 3, 149–154. doi: 10.1007/bf01695936
Pollatos, O., Schandry, R., Auer, D. P., and Kaufmann, C. (2007). Brain structures mediating cardiovascular arousal and interoceptive awareness. Brain Res. 1141, 178–187. doi: 10.1016/j.brainres.2007.01.026
Quadt, L., Critchley, H. D., and Garfinkel, S. N. (2018). The neurobiology of interoception in health and disease. Ann. N.Y. Acad. Sci. 101, 6333–6317. doi: 10.1111/nyas.13915
Rescorla, R. A., Wagner, A. R., Black, A. H., and Prokasy, W. F. (1972). Classical Conditioning II: Current Research and Theory. New York, NY: Appleton-Century-Crofts.
Seth, A. K. (2013). Interoceptive inference, emotion, and the embodied self. Trends Cogn. Sci. 17, 565–573. doi: 10.1016/j.tics.2013.09.007
Stephan, K. E., Manjaly, Z. M., Mathys, C. D., Weber, L. A. E., Paliwal, S., Gard, T., et al. (2016). Allostatic self-efficacy: a metacognitive theory of dyshomeostasis-induced fatigue and depression. Front. Hum. Neurosci. 10:550. doi: 10.3389/fnhum.2016.00550
Stewart, J. L., May, A. C., Poppa, T., Davenport, P. W., Tapert, S. F., and Paulus, M. P. (2014). You are the danger: attenuated insula response in methamphetamine users during aversive interoceptive decision-making. Drug Alcohol. Depend. 142, 110–119. doi: 10.1016/j.drugalcdep.2014.06.003
Vlemincx, E., Abelson, J. L., Lehrer, P. M., Davenport, P. W., Van Diest, I., and Van den Bergh, O. (2013). Respiratory variability and sighing: a psychophysiological reset model. Biol. Psychol. 93, 24–32. doi: 10.1016/j.biopsycho.2012.12.001
Wang, G.-J., Tomasi, D., Backus, W., Wang, R., Telang, F., Geliebter, A., et al. (2008). Gastric distention activates satiety circuitry in the human brain. Neuroimage 39, 1824–1831. doi: 10.1016/j.neuroimage.2007.11.008
Keywords: interoception, breathing, perception, inspiratory resistance, MRI
Citation: Rieger SW, Stephan KE and Harrison OK (2020) Remote, Automated, and MRI-Compatible Administration of Interoceptive Inspiratory Resistive Loading. Front. Hum. Neurosci. 14:161. doi: 10.3389/fnhum.2020.00161
Received: 18 January 2020; Accepted: 14 April 2020;
Published: 12 May 2020.
Edited by:
Daniel S. Margulies, Centre National de la Recherche Scientifique (CNRS), FranceReviewed by:
Ian Kleckner, University of Rochester, United StatesTim Rohe, University Hospital Tübingen, Germany
Copyright © 2020 Rieger, Stephan and Harrison. This is an open-access article distributed under the terms of the Creative Commons Attribution License (CC BY). The use, distribution or reproduction in other forums is permitted, provided the original author(s) and the copyright owner(s) are credited and that the original publication in this journal is cited, in accordance with accepted academic practice. No use, distribution or reproduction is permitted which does not comply with these terms.
*Correspondence: Olivia K. Harrison, ZmF1bGxAYmlvbWVkLmVlLmV0aHouY2g=