Automatic Sensory Predictions: A Review of Predictive Mechanisms in the Brain and Their Link to Conscious Processing
- 1Institute of Computer Science, University of Bern, Bern, Switzerland
- 2Helen Wills Neuroscience Institute, University of California, Berkeley, Berkeley, CA, United States
- 3Department of Psychology, University of California, Berkeley, Berkeley, CA, United States
- 4Sleep-Wake Epilepsy Center | NeuroTec, Department of Neurology, Inselspital, Bern University Hospital, University of Bern, Bern, Switzerland
The human brain has the astonishing capacity of integrating streams of sensory information from the environment and forming predictions about future events in an automatic way. Despite being initially developed for visual processing, the bulk of predictive coding research has subsequently focused on auditory processing, with the famous mismatch negativity signal as possibly the most studied signature of a surprise or prediction error (PE) signal. Auditory PEs are present during various consciousness states. Intriguingly, their presence and characteristics have been linked with residual levels of consciousness and return of awareness. In this review we first give an overview of the neural substrates of predictive processes in the auditory modality and their relation to consciousness. Then, we focus on different states of consciousness - wakefulness, sleep, anesthesia, coma, meditation, and hypnosis - and on what mysteries predictive processing has been able to disclose about brain functioning in such states. We review studies investigating how the neural signatures of auditory predictions are modulated by states of reduced or lacking consciousness. As a future outlook, we propose the combination of electrophysiological and computational techniques that will allow investigation of which facets of sensory predictive processes are maintained when consciousness fades away.
Introduction
Learning information from our environment and forming predictions about future events is a key skill for survival. Stimuli from the world around us contain repetitive rules and patterns, as for example music, or speech. Being able to form predictions about future events facilitates perception and increases chances of survival, as a deviation from an expected pattern can signal danger.
The human brain has the astonishing capacity to formulate predictions about future events, relying on internal models that generate automatic predictions (generative models) about the most plausible states of the environment given prior information. Neural predictions are generated not only in the case of active perception (SanMiguel et al., 2013), but also when conscious access to the environment is diminished, such as in sleep, anesthesia, or coma (Figure 1). The study of predictive processes has pervaded neuroscientific publications in the last three decades and painted a new view of the brain as a predictive organ (Dayan et al., 1995; Friston, 2005). Prediction provides explanation of phenomena at both the macro- and the micro-scales of brain functioning, including psychology (perception, cognition) and electrophysiology (neuronal processes). The study of predictive processes, which was first hinted to in the later 1800s (Lotze, 1852; Von Helmholtz, 1867) has been concretely formalized by statistics, information theory and machine learning. This review will focus on how sensory predictions have been used to probe different states of consciousness, and on what unknowns they have revealed about brain dynamics and functioning in these different states. By summarizing research done in both humans and animals, we examine the different components of the predictive network, and how these are modulated by conscious perception.
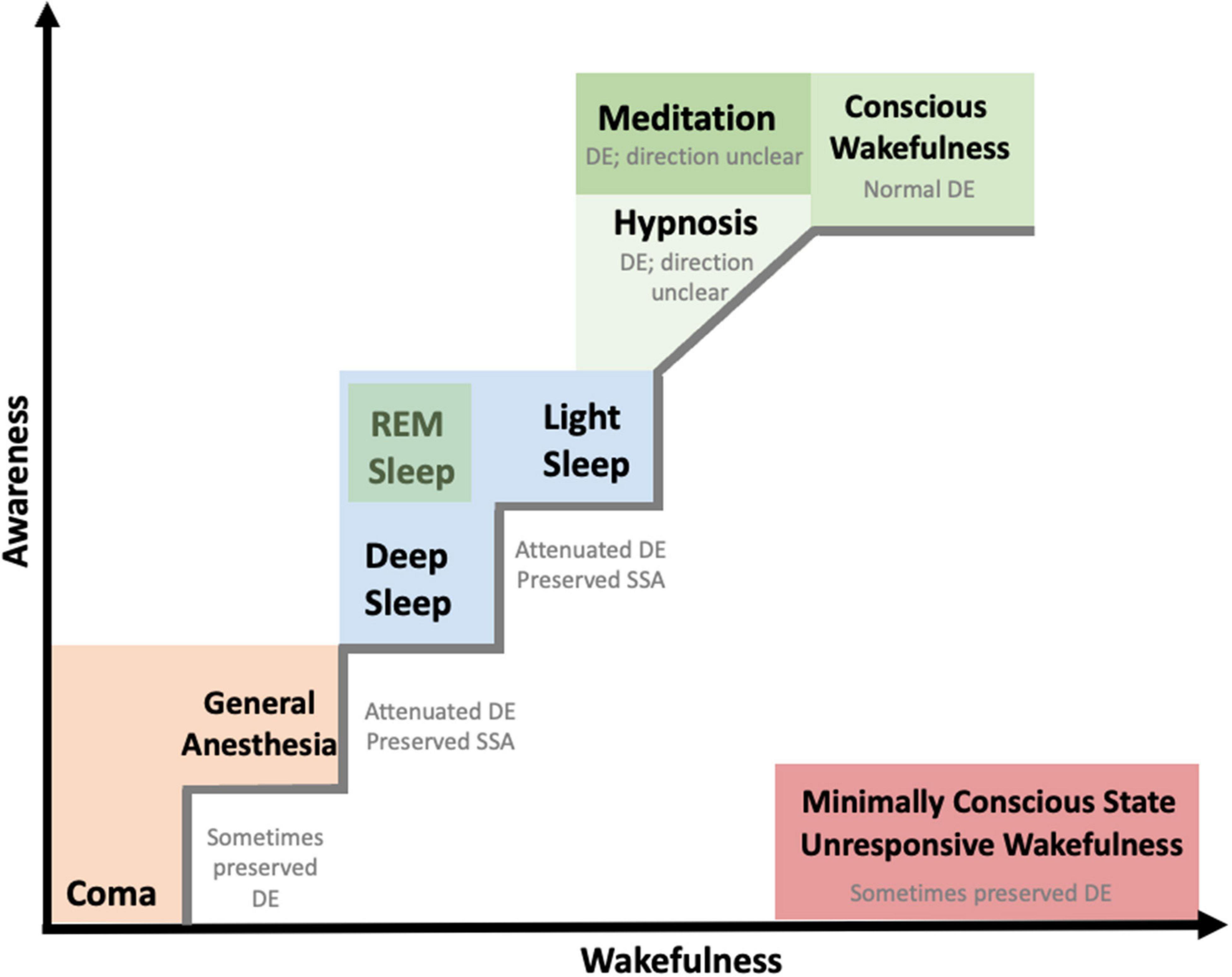
Figure 1. Manifestation of auditory predictive processes in different consciousness states. States of consciousness are placed according to the observed level of wakefulness and awareness, adapted from Laureys, 2005. The colors represent similarities or overarching groupings of states of consciousness. While there is a continuum from coma to conscious wakefulness, minimally conscious state and unresponsive wakefulness syndrome do not lie on the same continuum; neither do REM sleep, meditation, and hypnosis. The positioning of different consciousness states is following previous studies (e.g., Laureys, 2005) when possible and is an approximate estimate for consciousness states that were not originally included in previous studies (e.g., hypnosis, meditation). *DE: deviance effects, grouping together mainly effects observed at scalp EEG level. For more fine-grained information for each consciousness state we refer readers to Tables 1–4.
Introduction to Auditory Predictive Mechanisms
Paradigms for Studying Sensory Predictions and Consciousness
The most common sensory modality for studying predictive processes in the absence of consciousness is the auditory modality. Auditory stimulation is relatively straightforward to achieve, and it can reach the brain even in the absence of attention, or under conditions where eyes are closed, such as for example during sleep. The most famous paradigm for studying sensory predictions is the oddball paradigm (Näätänen and Alho, 1995; Garrido et al., 2009b), where a stream of identical repeated sounds (standards) is broken by an oddball, or a different sound (deviant), that is occasionally presented. In this paradigm, a regularity is built by repeating stimuli or sequences of stimuli. Prediction errors (PE) are signaled when deviations from the established regularity occur (Mumford, 1992; Rao and Ballard, 1999; Friston, 2005), by comparing neural responses to predicted (standard) versus observed (deviant) stimuli. Other experimental paradigms consist of the roving paradigm, where the oddball sound is followed by a stream of identical sounds, which at some point become standards, and are then followed by another stream of oddball sounds that turn into standards, with this pattern repeating (Garrido et al., 2009b); and the local-global paradigm (Bekinschtein et al., 2009), which is used to study local and global deviance effects. In the local-global paradigm, two forms of regularities are created – a local and a global one. These two types of regularities are built over single sounds (local), or groups of sounds (global). For local deviance, a standard sound is repeated a few times, followed by a deviant sound (e.g., aaaaB). This is similar to the deviance effect in a standard oddball paradigm. The global deviance effect is built by repeating this classic oddball structure, and then breaking this sequence by replacing the deviant in the third repetition with a standard (aaaaB aaaaB aaaaa).
Mechanisms Underlying Auditory Predictions
Stimulus Specific Adaptation and Deviance Detection
To formulate a prediction, first a regularity needs to be established, often through repetition. Repeating a given stimulus, for example a sound, results in a reduced response at the neural level, commonly referred to as stimulus specific adaptation (SSA) in animal research (Carbajal and Malmierca, 2018; Sikkens et al., 2019), and repetition suppression (RS) in human research (Rangarajan et al., 2020). SSA quantifies the change in the firing rate of a neuron when a certain tone is frequently or infrequently presented (Ulanovsky et al., 2004; Khouri and Nelken, 2015). The SSA was first recorded in the cortex of anesthetized cats (Condon and Weinberger, 1991), where small but precise reductions in the responses to standard, tones were demonstrated, appearing minutes after the first presentation of the standard, and lasting for an hour or more. Neurons along the auditory pathway and in frontal and subcortical areas (see section “Cortical and Subcortical Generators”) show progressively reduced responses to repetitive stimuli, possibly as a result of short-term plasticity (Carbajal and Malmierca, 2018). Interestingly, neurons along the auditory processing pathway can express SSA, which in mice include parts of the inferior colliculus (IC), the dorsal and medial divisions of the medial geniculate body (MGB) and parts of the auditory cortex (Carbajal and Malmierca, 2018). This pathway is thought to carry predictions and prediction error signals (Carbajal and Malmierca, 2018).
A second crucial component of formulating a prediction is being able to detect violations from an established regularity. A deviant event may result in an increased neuronal response compared to the response to regular events, a phenomenon referred to as Deviance Detection (DD; Sikkens et al., 2019). For DD to occur, the increased neural response to deviant stimuli needs to be stronger than the neural response to standard stimuli, over and above SSA. DD is considered a correlate of error signaling (Sikkens et al., 2019). Although SSA occurs at early latencies, generally within the first 80 ms after stimulus onset, DD occurs at later latencies, around 120–240 ms post-stimulus onset (Sikkens et al., 2019). Macroscopically, these two processes of SSA and DD are thought to be contributors to a human EEG signature of regularity detection, the MMN (Sikkens et al., 2019).
Mismatch Negativity
The Mismatch Negativity (MMN) signal was first discovered in the late 1970’s (Näätänen et al., 1978). The MMN manifests as a negative component of a difference wave peaking at about 100–250 milliseconds (ms) post-deviance onset, obtained by subtracting responses to standard stimuli from responses to deviant stimuli (Näätänen, 2003; Garrido et al., 2009b). MMN was originally thought to be elicited based on a previously created sensory memory trace (Näätänen, 2003), thus offering an observation window into the central auditory system and its functioning (Näätänen and Escera, 2000). This is known as the “trace-mismatch” explanation of MMN (Winkler, 2007), where MMN is seen as a signal of mismatch or surprise between a retrospective memory trace and the current input. Another interpretation of MMN is found in the adaptation hypothesis (May et al., 1999; Jääskeläinen et al., 2004). According to this hypothesis, cells tuned to standard sounds adapt, while cells tuned to more infrequent deviant sounds do not adapt and thus elicit higher responses (May et al., 1999). More recently, the MMN has been examined under the lens of predictive coding, which suggests that the MMN is a neural signature of a sensory prediction error signal, and that it represents an ‘error’ response that is elicited by deviant sounds (Garrido et al., 2009b). This view is supported by computational modeling studies, which have linked trial by trial changes in the MMN signal with the adjustment of an internal probabilistic model of the environment (Lieder et al., 2013). Under predictive processing, MMN is a signal of mismatch between sensory input and, contrary to the “trace-mismatch” hypothesis, a prospective and not retrospective sensory stimulus.
Interestingly, the MMN is described as a pre-attentive, automatic response, which can be elicited despite variations in states of wakefulness (Sculthorpe et al., 2009), such as during sleep or anesthesia, coma, or states of altered awareness, including hypnosis and meditation (Cahn and Polich, 2009; Chennu and Bekinschtein, 2012; Morlet and Fischer, 2014; Jamieson, 2016). In addition to extensive research in humans, MMN responses have also been recorded in cats (Csépe et al., 1987; Pincze et al., 2001), monkeys (Javitt et al., 1992, 1994), rabbits (Ruusuvirta et al., 1995, 1996a, b), guinea pigs (Kraus et al., 1994), and rats (Shiramatsu et al., 2013; Harms et al., 2014), via epidural EEG electrodes or cortical surface microelectrode arrays. Results are comparable, but not completely identical. For example, MMN responses in cats appear with shorter latencies due to the smaller size of cat cortex (Pincze et al., 2001). In summary, the MMN is an event-related potential (ERP) component that represents a scalp EEG signature of predictive processing, and is observed across species and states of consciousness.
P300
The P300 component is a positive deflection in the human ERP, with a peak latency at about 300 ms post-stimulus onset in response to a novel or task-relevant stimulus (Sutton et al., 1965). The P300 is usually elicited in an oddball paradigm when behavioral responses to deviants are demanded – thus, as a response to a target deviant stimulus (Picton, 1992). It has been proposed that the P300 reflects contextual updating (Donchin, 1981; Donchin and Coles, 1988) driven by attentional processes (Polich, 2007), namely updating of a stimulus or of task-related (working) memory and expectancies (Verleger, 1988). The P300 has two main subcomponents, the P3a and P3b, which have different topographies and functional implications. While the P3a is fronto-centrally distributed and appears as a response to novel and distracting stimuli, the P3b is maximal over parietal recording sites in response to conscious detection of target and novel stimuli (Squires et al., 1975; Polich, 2007).
Neural Circuits Underlying Auditory Predictions
Predictive neural traces manifest in multiple stages of sensory processing. The most prevalent view is that higher level regions in a processing hierarchy generate and propagate sensory predictions to lower level regions, which compare these predictions to the actual sensory input (Rao and Ballard, 1999; Friston, 2005). Predictions flow ‘down’ the processing stream from higher level areas to lower level areas, while the opposite is true for error signaling, meaning that errors are signaled ‘upward’ by lower level areas detecting a mismatch with the current prediction (Rao and Ballard, 1999; Bastos et al., 2012). Importantly, the signaling of predictions and errors is posited to underlie multiple stages of information processing, so that sensory processing would, at each processing level, have to resolve the correspondence between predictions and sensory input (Friston, 2005; Summerfield and Egner, 2009). For this reason, some argue that predictive coding theories go beyond the standard bottom-up and top-down dichotomy (Rauss and Pourtois, 2013), as higher levels do not only modulate activity at lower levels of processing, but have the chance to initiate such activity (Mumford, 1992), in addition to lower level stages of the hierarchy being able to generate predictions for higher-level error signals (Kok and de Lange, 2015). There are multiple models of predictive processing (e.g., Spratling, 2008a, b; Bastos et al., 2012), which deviate from standard models with regards to where the error units are situated (i.e., in middle and not superficial cortical layers), and how predictions flow (i.e., not only ‘downward’ through the processing stream, but also ‘upward’). Nevertheless, most models posit that error and predictive units have different laminar profiles (see Heilbron and Chait, 2018 for a detailed review).
Cortical and Subcortical Generators of Sensory Predictions
Sensory predictions are supported by distributed circuits in the brain, including sensory and prefrontal, but also subcortical regions, which may compute different variables related to predictions (Johnson et al., 2020). Predictive mechanisms are not only inherent properties of microcircuits in the brain, but also find expression through cortical connectivity (Johnson et al., 2020). Connected regions in the cortical hierarchy interact recurrently in a joint effort to find the world model that best explains the sensory inputs in the prediction units, and thereby reduce the activity of these units (Kok and de Lange, 2015).
In the auditory modality, magnetoencephalography (MEG) studies first showed that the MMN is generated in the auditory cortex (Hari et al., 1984). Later, using functional Magnetic Resonance Imaging (fMRI) and EEG, it was discovered that frontal regions are also involved in MMN generation (Alho, 1995; Opitz et al., 2002). Specifically, Opitz et al. (2002) used fMRI and EEG to study the temporal and frontal generators of the MMN and showed that responses to deviant stimuli of medium and large, but not small amplitude are found in the superior temporal gyrus (STG) bilaterally, and in the inferior frontal gyrus (IFG). Since then, these areas were often studied using EEG and fMRI combined with dynamic causal modeling (Garrido et al., 2007, 2008, 2009a; Boly, 2011; Chennu et al., 2016), and were also confirmed by multiunit activity (MUA) recordings (Nieto-Diego and Malmierca, 2016) and local field potential (LFP) measurements of SSA in rats (Imada et al., 2013). The neural correlates of the P300 component have been localized to multiple brain regions. The generators of the P3a include frontal cortical generators, the cingulate cortex, the supramarginal gyrus, and the hippocampus, while the generators of the scalp P3b include mainly temporo-parietal and frontal regions (Fonken et al., 2019).
Intracranial EEG (iEEG) recordings in humans have further advanced our understanding of the neural underpinnings of the predictive circuit (Johnson et al., 2020), by confirming the involvement of temporal and frontal regions in responding to deviant events (e.g., Rosburg et al., 2005). Additionally, Durschmid and colleagues showed that the temporal cortex detects deviations at the level of single stimuli, while prefrontal regions are sensitive to whether these deviations were predictable (Dürschmid et al., 2016), as well as to the likelihood of a deviant sound to occur (Dürschmid et al., 2019). Intracranial recordings have also implicated the posterior cingulate and parietal lobe (Halgren et al., 1995; Clarke et al., 1999), limbic structures such as the hippocampus, the amygdala (Halgren et al., 1980), and basal ganglia and thalamic circuits such as the caudate nucleus (Kropotov et al., 2000) and nucleus accumbens (Zaehle et al., 2013; Dürschmid et al., 2016) in supporting the auditory predictive network.
In addition, Cacciaglia et al. (2015) used event-related fMRI during an oddball task and found evidence of involvement of human inferior colliculus (IC) and MGB of the thalamus (Cacciaglia et al., 2015), confirming previous similar results found using SSA in animals for the occurrence of infrequent speech-like stimuli (Kraus et al., 1994), as well as for sounds with different binaural phases (King et al., 1995). fMRI studies further involved the amygdala (Kropotov et al., 2000; Czisch et al., 2009; Blackford et al., 2010) and hippocampal (Blackford et al., 2010) structures in deviance detection. Subsequent single unit recordings, and fMRI implicated the IC (Pérez-González et al., 2005; Malmierca et al., 2009; Patel et al., 2012; Gao et al., 2014) and the MGB (Anderson et al., 2009; Antunes et al., 2010; Richardson et al., 2013) in SSA (see also, Duque et al., 2015 for an extensive review on subcortical structures implicated in SSA generation).
In summary, sensory predictions rely on a distributed network of brain regions, expressed in low-level sensory processing areas, cortico-thalamic circuits involving subcortical thalamic and basal ganglia structures together with the amygdala and hippocampus, as well as higher-level parietal and frontal areas. This complex distributed network involved in sensory processing and PE generation works in concert to allow learning of sensory regularities and the formation of predictions.
Attention
The role of attention in MMN generation was initially investigated in auditory tasks, where the ear to be attended was manipulated (Näätänen et al., 1993; Trejo et al., 1995; Alain and David, 1997). The debate was initiated when Näätänen proposed that the MMN was unaffected by manipulations of attention (see Näätänen, 1990 for a review). This view was challenged by research showing attentional effects on MMN (Woldorff and Hillyard, 1991; Szymanski et al., 1999; Auksztulewicz and Friston, 2015). There is now a plethora of studies showing that attention enhances the amplitude of MMN (Woldorff and Hillyard, 1991; Alain and David, 1997; Szymanski et al., 1999; Chennu et al., 2013; Auksztulewicz and Friston, 2015) and P300 (Chennu et al., 2013) responses. Electrophysiologically, manipulations of attention have been shown to predominantly affect the detection of oddball stimuli in prefrontal, but not temporal, regions, and to increase effects of oddball detection (Kam et al., 2020).
Later views suggested that the MMN response can be considered as a two-step process, composed of both standard formation and deviance detection (Sussman, 2007). The standard formation phase consists of auditory processes such as scene analysis and is susceptible to attentional effects. In contrast, the deviance detection phase, which depends on the standard formation phase, is independent of attentional manipulations. From a computational perspective, attention is thought to increase the precision of PEs, leading to more reliable estimates and a more accurate update of an environmental model (Auksztulewicz and Friston, 2015).
Although attention is not the focus of the present review, it can be argued that inattentive states represent states where sensory signals and predictions are elicited in an automatic way, as in unconscious states. We therefore mentioned these key findings in the field to emphasize that the brain not only produces predictions about the features of a signal (i.e., intensity, frequency, etc.), but also about the signal’s reliability or precision (i.e., how predictable is the signal). When signal reliability is low, such as in inattentive conditions, deviations are down-weighted; when it is high, deviations are amplified and prioritized for further processing (Heilbron and Chait, 2018). In this view, predictive processes and attentive processes are distinct, independent processes which can interact. The role of predictive mechanisms is making inferences about what causes the sensory input and how precise this input is, whereas attention optimizes the precision of this input and regulates the gain of feedforward PEs (Schröger et al., 2015).
Sensory Predictions in Reduced Consciousness States
Automatic sensory predictions manifest during wakefulness, but also when conscious access to the environment is lost, as will be subsequently reviewed. The interest for studying how neural responses are elicited during various awareness states first appeared when it was discovered that the MMN was evoked in the absence of attention (Näätänen, 1990), albeit with a much lower amplitude. MMN responses were even observed when subjects were engaged in other tasks, such as reading a book (Näätänen et al., 1993). Early studies recording MMN responses in animals anesthetized with barbiturates also confirmed MMN responses (Csépe et al., 1987; Javitt et al., 1992; Kraus et al., 1994). MMN responses were also observed during sleep in humans (Nielsen-Bohlman et al., 1991) and animals (Csépe et al., 1987). These studies indicated a great potential for studying auditory predictions in the absence of conscious access to the environment. Therefore, the value of the MMN response as a diagnostic tool for patients with disorders of consciousness (Chennu and Bekinschtein, 2012), or with psychiatric disorders (e.g., depersonalisation and derealisation) became evident (Lew et al., 2003; Kotchoubey et al., 2005; Wijnen et al., 2007).
Understanding the neural underpinnings that are associated with the emergence of conscious experience is of one of the main unresolved questions in neuroscience, with a first major challenge consisting in the clarification of the experimental definition of the term consciousness (Dehaene and Changeux, 2011). This is a fundamental challenge, due to the implications it brings for patients in coma, anesthesia, and those suffering from disorders of consciousness. Here, we adopt a widely used, non-exhaustive, functional definition of consciousness, which assesses conscious states by their expressed level of consciousness (wakefulness) on the one hand, and content of consciousness (awareness) on the other hand (Laureys, 2005; and Figure 1). This clinical definition of consciousness is also used to diagnose disorders of consciousness (see Giacino et al., 2014 for a review), characterized by a disrupted relationship between awareness and wakefulness (Gosseries et al., 2011), where observations of spontaneous and stimulus-evoked behaviors are used. Predictive processing was recently characterized as a “neural motif,” which is present in many computations in the brain (Aitchison and Lengyel, 2017), but how does it relate to our conscious wakefulness and awareness? In fact, auditory predictive coding is commonly used to assess residual brain functions in patients with disorders of consciousness, often through scalp EEG components that are considered as neural signatures of predictive processing (Chennu and Bekinschtein, 2012; Gosseries et al., 2014a).
In the next sections we will provide an overview of findings from the last 30 years studying the extent to which the neural markers of predictive processes are altered by reduced or absent consciousness. We will present findings from studies in sleep, anesthesia, disorders of consciousness, or altered states of consciousness, in humans and animals. In particular, we will focus on different neural signatures of auditory predictive processes, such as MMN and P3, or SSA, and we will review how these are modulated by the absence or reduction of consciousness. When possible, we will elaborate on neural mechanisms and circuits of auditory predictions, for example, in the case of studies using techniques with a high spatial resolution (e.g., iEEG or source localization techniques). In other cases, we will discuss findings based on neural markers of predictive processing at a more macroscopic level such as scalp EEG components and their possible clinical applications.
Sleep
Sleep represents a naturally occurring and rapidly reversible state of reduced consciousness (Campbell and Colrain, 2002). Sleep electrophysiology is altered with respect to wakefulness (Destexhe et al., 2007; Cox et al., 2014), but is well-characterized and relatively uniform across individuals (Steriade, 2006). In terms of the physiology of sleep, we distinguish paradoxical sleep or rapid eye-movement sleep (REM), and non-REM (NREM) sleep, which is further divided into three stages. NREM1 is the sleep onset period, NREM2 is light sleep, and NREM3 sleep is slow-wave-sleep. Different sleep stages have been associated with reduced consciousness or arousal (Goupil and Bekinschtein, 2012; Lendner et al., 2020).
Research in Humans
Several studies have investigated the neural correlates of MMN during sleep (Camman et al., 1987; Csépe et al., 1987; Näätänen and Lyytinen, 1994; Sallinen et al., 1994; Winter et al., 1995; Loewy et al., 1996; Atienza et al., 1997; Sallinen and Lyytinen, 1997; to name a few). After the wave of research in the 90’s, which employed standard intensity or duration oddball paradigms, the consensus was that MMN and P300 components appeared in REM sleep, but not in NREM2 (see e.g., Winter et al., 1995; Loewy et al., 1996, 2000; Cote, 2002; Colrain and Campbell, 2007; Sculthorpe et al., 2009). The main evoked potentials were K-complexes and late potentials that were functionally different from the classic deviance response (Wesensten and Badia, 1988; Nielsen-Bohlman et al., 1991; Van Sweden et al., 1994; Nordby et al., 1996). Nevertheless, some studies still indicated differential processing of auditory information even during deeper sleep stages (Nielsen-Bohlman et al., 1991; Winter et al., 1995). Laboratories therefore modified their paradigms in order to have more sensitive tests, and presented either rapidly succeeding stimuli (every 150 ms) (Sabri et al., 2003), or used “hyper-salient” stimuli (Chennu and Bekinschtein, 2012) – i.e., very rare, very deviant stimuli, as used for example by Loewy and colleagues, with low probability and 1000 Hz difference between the standard and the deviant stimuli. In some of these studies, MMN responses were elicited during NREM1 and NREM2 (Sabri et al., 2003; Sabri and Campbell, 2005), whereas in others they were only evoked during REM sleep (Loewy et al., 1996; see Table 1A for a summary).
A more recent study employed MEG and EEG recordings during sleep and used a local-global paradigm (Strauss et al., 2015). Results showed a disrupted global response in NREM2 sleep, associated with an absence of the P300 response together with a simultaneous absence of behavioral responses, despite retained local mismatch responses across all sleep stages (Strauss et al., 2015). Moreover, authors used an additional manipulation where expectation was induced by alternating different sounds (aBaBa and aBaBB sequences), instead of repeating the same stimulus (aaaaa). In this case, the differential response that was observed between predicted and unpredicted sequences during wakefulness vanished during NREM2 sleep. This was interpreted as evidence that predictive processing during sleep could be explained with an adaptation framework (through repetition of the same stimuli) and not by using prediction error (through repetitions of different stimuli) mechanisms.
Even when MMN responses are present during sleep, their characteristics (i.e., amplitude or latency) are typically attenuated with respect to awake conditions (Atienza et al., 2001). It is, however, unclear whether predictive processes during sleep are altered because the underlying predictive computations are fundamentally different compared to wakefulness, or because the sleep electrophysiology is modified (Sabri and Campbell, 2002). Apart from detecting deviant events, there is an ongoing debate whether new information can be learned during sleep, and if so, under which conditions (Andrillon et al., 2017). A large body of literature reports no evidence for learning new rules in deep NREM sleep, but more recent findings show that semantic associations can be learned if these are presented during peaks (i.e., “up” states) of slow-wave activity (Züst et al., 2019), which are characterized by similar conditions of cortical excitability as wakefulness (Destexhe et al., 2007; Cox et al., 2014). Moreover, other studies have shown that sleep facilitates encoding of previously learned generative models, improving sensory predictions (Lutz et al., 2018).
Research in Animals
Animal sleep research has investigated evoked responses in sensory systems (Hennevin et al., 2007). From a physiological viewpoint, two states of sleep are classically categorized in animals, paradoxical or REM sleep, and NREM sleep. Physiological studies in sleep further demonstrate preserved auditory processing (Edeline et al., 2000; Issa and Wang, 2008), with reported decreases in the amount and quality of information reaching the higher-level cortices (for an extensive review, see Coenen and Drinkenburg, 2002; see also Murata and Kameda, 1963). This reduction in information transmission is thought to be due to thalamic gating (McCormick and Bal, 1994), with sensory input to the cortex partially blocked at the thalamus (Hall and Borbely, 1970; Edeline et al., 2000). Pre-thalamic processing is thought to be mostly maintained (Steriade, 1991). Nevertheless, relevant stimuli can have some form of impact on the functional state of the sleeping animal, suggesting that the sleeping brain is still able to judge the meaningfulness of stimuli (Nielsen-Bohlman et al., 1991). Sophisticated paradigms suggest that simple forms of learning are also still possible, as for example in extinction (where a pre-conditioned association between two stimuli is erased) and pre-exposure (when animals are exposed to the to-be-conditioned stimulus before actual conditioning) experiments; and there is evidence that new associations can be formed with lower quality than the ones formed during waking (Coenen and Drinkenburg, 2002).
An early study in cats reported that the MMN can be elicited during all sleep stages (Csépe et al., 1987). Auditory evoked potentials were elicited by standard and deviant tones of different probabilities during wakefulness and sleep. A large MMN response was elicited by deviant tones, with MMN amplitude inversely proportional to deviants’ probability. MMN during slow-wave sleep exhibited longer latency and was only evoked by deviant tones at the lowest probabilities. Another more recent study in rats used an oddball paradigm and found comparable SSA responses across REM, NREM and wake cycles in the core auditory region, defined by the authors as the core auditory fields receiving input from the ventral division of the medial geniculate nucleus of the thalamus (Nir et al., 2015; Figure 2B; see also Table 1B, for a summary). This suggests that evoked activity in low-level sensory cortices during sleep is driven by external stimuli with little modulation by the vigilance state, and that the disconnection of cortical processing during sleep may occur at a later stage, thus corroborating the physiological findings described above.
Conclusion
In conclusion, the majority of sleep studies suggest that auditory predictive processing may be retained during sleep, in particular within core auditory areas (Nir et al., 2015). There is consensus that scalp EEG components related to predictive processes can manifest during REM sleep, with similar characteristics as during wakefulness. For NREM, the question of whether auditory predictions can occur remains actively debated. One key factor that will need to be taken into account in the design of new experiments and during data analysis is the complex and dynamic brain physiology of sleep.
Different sleep stages are characterized by multiple local disruptions (Drummond et al., 2004; Magnin et al., 2010), leading to qualitatively different epochs with differences in sensory processing (Hennevin et al., 2007). Additionally, different stages of sleep are not homogeneous, as they are characterized by tonic and phasic fluctuations of arousal, of the background EEG activity, and of neuromodulator release (Hobson et al., 2000). As a result, cortico-thalamic long-range connectivity is affected, while some basic cortico-cortical connectivity might be preserved, as for example in the default mode network (Koike et al., 2011).
These fluctuations in sleep physiology might explain the attenuated MMN responses measured during sleep, and might mirror the decreasing thalamic activity, by indicating an impaired bottom-up component of MMN elicitation (Atienza et al., 2002). The impaired top-down component might stem from prefrontal lobe deactivation during sleep (Atienza et al., 2002). The cortico-thalamic network during REM sleep seems to be characterized by general activations in thalamic and posterior areas including temporo-occipital cortices (Maquet et al., 1996; Braun et al., 1997; Maquet, 2000; Portas et al., 2000), while frontal area activity is reduced (Maquet, 2000; Portas et al., 2000). All these areas are deactivated during NREM sleep (Maquet, 2000). Alternatively, connectivity at a later stage of information processing has also been reported during sleep (Massimini et al., 2005; Horovitz et al., 2009; Tagliazucchi et al., 2013), with preserved activation of primary sensory cortices in both animals (Peña et al., 1999; Edeline et al., 2001; Issa and Wang, 2008) and humans (Portas et al., 2000; Czisch et al., 2002; Dang-Vu et al., 2011).
Future research investigating predictive processing in sleep is crucial, given the sparseness of the current literature. Auditory paradigms are particularly important for assessing brain processing during sleep, as well as associations between sleep disorders and generalized reduced cognitive performance (Pilcher and Huffcutt, 1996; Banks and Dinges, 2007), or impaired auditory processing (Raz et al., 2001; Key et al., 2009; Bortoletto et al., 2011; Liberalesso et al., 2012; Leite et al., 2017).
Anesthesia
Phenomenologically and behaviorally, anesthetic states can be described as a continuum ranging between mild sedation, “a pharmacologically induced, reversible state, characterized by dose-related impairment of cognitive functions, including attention and memory, but during which consciousness and awareness are maintained” (Stamatakis et al., 2010), to complete anesthesia, “a drug-induced loss of consciousness during which patients are not rousable, even by painful stimulation” (Anesthesiologists Task Force on Intraoperative Awareness, 2006).
Anesthetics have complex effects on neural activity, such as alterations in the activity of wide-spread cortico-thalamic networks (Rudolph and Antkowiak, 2004; Scheinin et al., 2021), and disruptions of cortico-thalamic connectivity (Guldenmund et al., 2017). Interestingly, general anesthesia and NREM sleep share similarities, such as slow oscillatory activity, a disruption of cortico-cortical connections (Massimini et al., 2005; Pal et al., 2016), and changes in non-oscillatory neural dynamics (Lendner et al., 2020). During anesthesia and NREM, thalamocortical hyperpolarized neurons are alternating between active and silent periods. By contrast, during wakefulness and REM sleep, the thalamocortical system is depolarized with awake-like low-voltage activity, and with tonic firing in neurons (Steriade et al., 2001). At high doses, general anesthesia during surgery can approximate brain stem death, where patients are unconscious, have inhibited brain stem reflexes, do not respond to nociceptive stimuli, and require cardiorespiratory and thermoregulatory support (Brown et al., 2010). These levels of anesthesia can be accompanied by isoelectric (i.e., almost a flat line) EEG activity (Brown et al., 2010).
In terms of cerebral metabolism, most anesthetics result in a general reduction in cortical brain activity, with certain regions, including cortical association areas, the thalamus, and the midbrain showing a higher decrease in cerebral metabolism (Heinke and Koelsch, 2005). In human studies, anesthesia is typically induced using either propofol (Plourde and Picton, 1991; Reinsel et al., 1995; Koelsch et al., 2006) or opioids (Plourde and Boylan, 1991). Propofol is an agonist at the GABA receptor and exerts a hypnotic and sedative effect through this mechanism (Rudolph and Antkowiak, 2004). Light propofol anesthesia, as administered in surgery, causes stage 2 sleep-like brain electrophysiological activity, with sleep and sleep-like spindles appearing during deep propofol anesthesia (Stamatakis et al., 2010; see Purdon et al., 2015, for a review). Opioids such as fentanyl are mostly used in cardiovascular surgery due to limited fluctuations in cardiovascular dynamics (Saidman et al., 1984). The EEG trace during opioid anesthesia is characterized by high amplitude slow delta waves (Wauquier et al., 1984). Opioids provide anesthesia, analgesia and unconsciousness after premedication with other anesthetic agents such as benzodiazepines (Sebel et al., 1981).
Research in Humans
Early human anesthesia studies did not compute the MMN response, but rather examined the P300 response, due to its suspected association with conscious awareness (Plourde and Boylan, 1991; Plourde and Picton, 1991; Reinsel et al., 1995). These studies report a decrease in amplitude of the P300 response with progressive sedation and abolishment when unconsciousness is reached (Plourde and Boylan, 1991; Plourde and Picton, 1991; Sneyd et al., 1994; Reinsel et al., 1995), accompanied by absent behavioral responses to deviant stimuli (Plourde and Picton, 1991).
Later studies carried out in the 2000’s (Simpson et al., 2002; Yppärilä et al., 2002; Heinke et al., 2004; Koelsch et al., 2006) started to measure MMN responses alongside the P300 responses. These studies reported a dose-dependent incremental breakdown of MMN and P300 (Yppärilä et al., 2002; Heinke et al., 2004; Koelsch et al., 2006). As patients transition from wakefulness to anesthesia, AEPs tend to decrease in amplitude: Simpson et al. (2002) reported that N100 (thought to reflect the early processing of acoustic features of a stimulus; Näätänen and Picton, 1987) responses to auditory stimuli disappear when patients become unconscious, and MMN is no longer elicited right before consciousness is lost, at the point of highest propofol concentration at which patients are still responsive. Yppärilä et al. (2002) complemented these findings by showing that the amplitudes of AEPs including N100 and MMN gradually decrease, and latencies gradually increase as patients transition from light to deep sedation. Notably, a small subset of patients retains both MMN and P300 responses even in deep sedation (Yppärilä et al., 2002). Similar findings were reported by Heinke et al. (2004), who showed decreasing amplitudes and increasing latencies for MMN as propofol sedation progresses, and an abolishment of P300 responses in deeper sedation levels (Heinke et al., 2004).
Koelsch et al. (2006) measured MMN and P300 responses in healthy volunteers undergoing propofol sedation in a state of sedation shallower than surgical anesthesia, as participants were still arousable by loud and repeated utterances of their own name or by mild prodding. The authors noted reduced, but existent, MMN and P3a responses during propofol sedation, with a missing P3b response. With recovery from deep propofol sedation, MMN recovered quickly to wake levels, but not the P300 response. Lastly, Zhang et al. (2018) report that MMN is abolished during deep anesthesia. The authors used source localization techniques to investigate how the network underlying the MMN response during awake conditions is altered by anesthesia. Deviant stimuli during anesthesia induced less long-distance connections between frontal and temporal-parietal regions than in an awake state (Zhang et al., 2018).
More recent studies have employed the local-global paradigm (Shirazibeheshti et al., 2018; Witon et al., 2020) with high-density EEG or iEEG recordings (Nourski et al., 2018) to test this hypothesis directly. Specifically, Shirazibeheshti et al. (2018) measured high-density EEG during a local-global paradigm in wakefulness, propofol sedation, and recovery. During sedation, both local and global deviance responses were recorded, but their amplitude was reduced with respect to wakefulness. The authors observed an interaction between effects of local and global deviance, namely that effects of local deviance exacerbate effects of global deviance. Nevertheless, under anesthesia this interaction was reduced. The authors posited that the coincidence of local and global deviance had a facilitatory effect on global deviance responses, which was reduced when individuals were sedated. Witon et al. (2020) further examined the neural circuits of this effect and observed effects of sedation on local deviance responses during early (100–150 ms post-stimulus onset) and middle (250–350 ms) time periods, indicative of modulations of evoked power responses early in the processing pathway. The interaction between the local and global effects was significant in a late time window (400–600 ms). The authors found a locally mediated acceleration of global deviance responses (Witon et al., 2020) during sedation and recovery. The second important interaction – the local standard global deviant, representing the pure global deviance effect – was reduced in anesthesia compared to recovery. Here, deviance processing is thought to be instantiated by more higher-level than low-level predictions. Key findings during sedation included a reduction in amplitude of the responses, and a slowing of the responses to deviant stimuli, specifically in global deviance.
Nourski et al. (2018) examined the neural networks that are preserved for local and global deviance responses in iEEG recordings. High frequency activity responses, which correlate with local infragranular multi-unit activity and superficial dendritic potentials (Leszczynski et al., 2020), and intracranial auditory evoked potentials were recorded. Authors used vowels instead of pure tones in patients implanted in temporal and inferior frontal regions, as well as in the amygdala, under propofol sedation. This study reported retained local deviance effects under loss of consciousness in auditory regions, but not outside of these regions, indicating intact low-level sensory predictive processing independent of the state of consciousness (Figure 2). By contrast, local deviance responses in frontal regions began to reduce during initial sedation and vanished during anesthesia. Global deviance was completely abolished with anesthesia, and in some patients, it was abolished even during a sedated state in which they were still responsive (Nourski et al., 2018). The authors concluded that the presence of a global deviance effect is indicative of conscious processing, while its absence is not necessarily linked to loss of consciousness (see Table 2A for a summary).
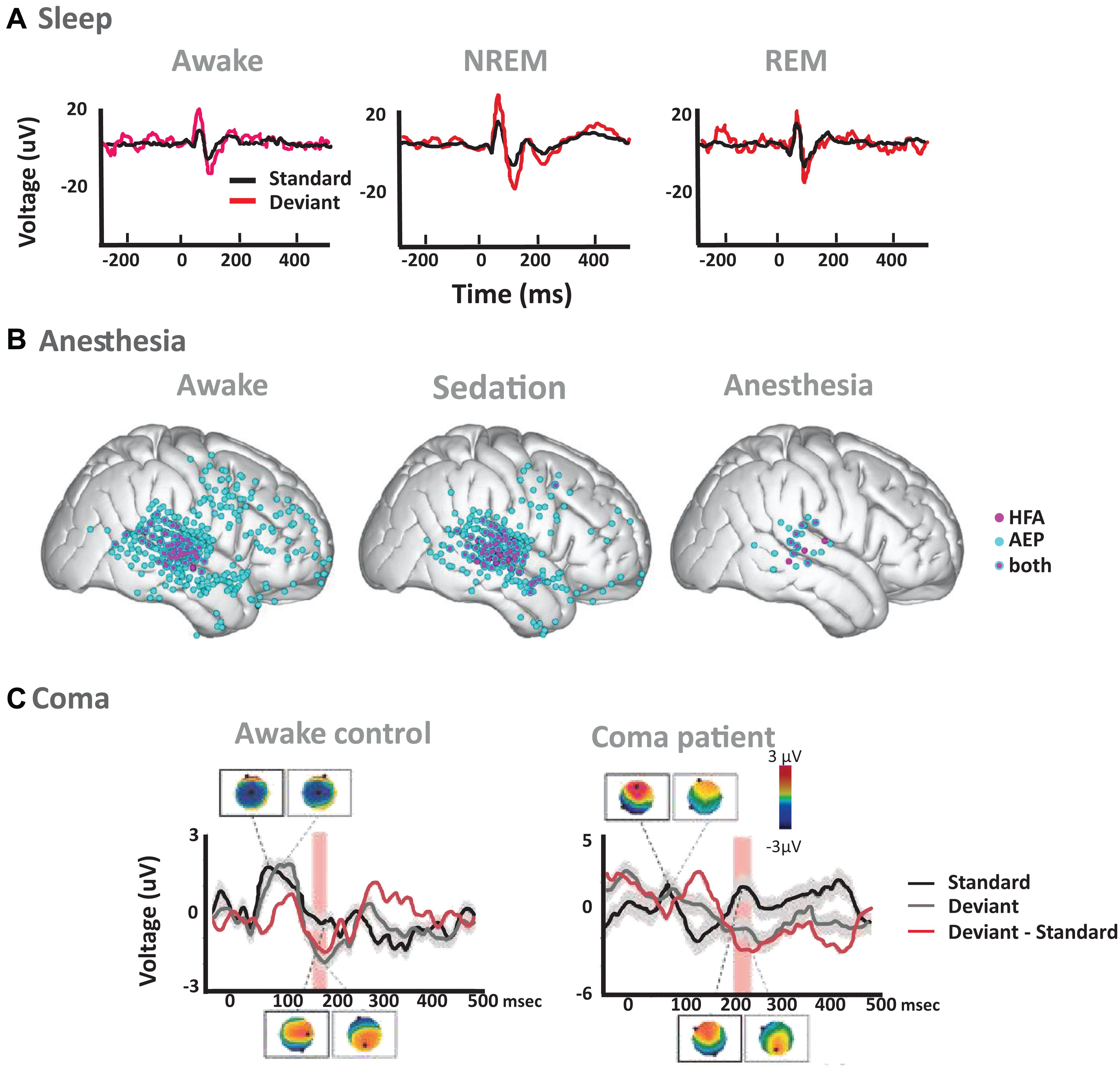
Figure 2. Examples of auditory predictive processes across states of reduced consciousness. (A) Auditory averaged ERP responses to standard (black) and deviant (red) tones during normal wakefulness (left), NREM sleep (middle) and REM sleep (right) from EEG recordings in rats. Figure adapted from Nir et al. (2015). EEG recordings showed weaker responses following standard compared to deviant tones in wakefulness, NREM and REM sleep, an effect that was additionally quantified by the authors as SSA in single unit activity of the primary auditory cortex (Nir et al., 2015). (B) Local Deviance effects in electrocorticography (ECoG) recordings of patients with epilepsy (Figure adapted from Nourski et al., 2018). Pink dots show electrodes with significant differences between responses to standard and deviant sounds in high frequency activity (HFA; 70–150 Hz); blue dots show electrodes with differences in evoked potentials (AEP); and pink and blue dots show electrodes with significant AEP and HFA effects. Local deviance was defined as significant increases in response to the deviant versus standard stimuli along a 0–800 ms post 5th stimulus window: (aaaaB – aaaaa) or (BBBBa – BBBBB). Stimuli were vowels /α/ and /i/, extracted from a female voice uttering the words h/α/d and h/i/d. Significant electrodes are shown for the awake state (left), for sedation (middle) and for the anesthesia state (right). (C) Auditory evoked potentials (AEP) and scalp topographies for an exemplar awake control and a coma patient, measured over frontal electrodes to standard (black) and duration deviant (gray) sounds, as well as the difference of the two responses (red; Figure adapted from Tzovara et al., 2013). The awake control shows a typical N100 response to auditory stimuli, manifesting as a central negativity in the AEP topography, and an MMN response starting around 170 ms post-stimuli onset. The exemplar patient shows differential responses between standard and deviant sounds at later latencies, after 220 ms post-stimuli onset. Red rectangles signify periods of significant difference in response to standard vs. deviant sounds.
Research in Animals
In animals, anesthesia is mostly induced using ketamine, urethane, or halothane (see Table 2B for summary). Anesthesia in general, whether with barbiturates or ketamine, seems to have more wide-spread effects in animals than in humans. Specifically, inhibition of auditory cortical units was reported 70 years ago (Thomas and Jenkner, 1952). Anesthetics are known to affect the entire central auditory pathway, from the dorsal cochlear nucleus (Young and Brownell, 1976) to core auditory regions (Gaese and Ostwald, 2001), such as the primary auditory cortex (A1). A1 neurons demonstrate reduced mean bandwidth in anesthesia than when animals are awake, with reductions up to threefold (Qin et al., 2003). In particular, ketamine anesthesia depth modulates not only average evoked responses but also response variability, which is highest under medium anesthesia, where ongoing cortical activity exhibits rhythmic bursting activity (Kisley and Gerstein, 1999). Importantly, this observed variability in shape and amplitude can be accounted for by the background ongoing activity, which speaks for transitions in thalamocortical excitability modulating these effects (Zurita et al., 1994). Specifically, stronger excitatory responses are observed in the thalamus after ketamine injection, despite decreasing overall cortical and thalamic firing rates (Kisley and Gerstein, 1999). Halothane, a gas anesthetic, shows a weaker suppressive effect on auditory-evoked responses (Johnson and Taylor, 1998; Moshitch et al., 2006), with responses found to sometimes resemble those in awake animals. Auditory working memory was found to be active for hundreds of ms after stimulus onset (Moshitch et al., 2006). Urethane causes moderate cardiovascular depression, with long duration of anesthesia (greater than 24 h), excellent anesthesia depth, and analgesia (Field et al., 1993). During urethane anesthesia auditory neurons show higher minimum thresholds, lower spontaneous firing rates, longer response latencies, and more frequent occurrence of tuning alterations, with stronger inhibition (Huang et al., 2013).
Because anesthesia facilitates experimental procedures, there are a multitude of deviance studies done in different species of anesthetized animals. Most of the studies have been carried out in rats (Ruusuvirta et al., 1998; Lazar and Metherate, 2003; Eriksson and Villa, 2005; Astikainen et al., 2006; Nakamura et al., 2011; Taaseh et al., 2011; Xu et al., 2014; Takahashi et al., 2015; Ahnaou et al., 2017; Parras et al., 2017; Rui et al., 2018; Cappotto et al., 2021), and mice (Ehrlichman et al., 2008; Chen et al., 2015; Duque and Malmierca, 2015; Duque et al., 2018; Lipponen et al., 2019), with a few studies in non-human primates (Uhrig et al., 2014), guinea pigs (Kraus et al., 1994; Christianson et al., 2014), gerbils (Bäuerle et al., 2011), and songbirds (Beckers and Gahr, 2012). These studies mainly report successful recordings of SSA or MMN-like responses in auditory cortices, especially under urethane anesthesia (Astikainen et al., 2006; Taaseh et al., 2011; Duque et al., 2015; Rui et al., 2018). Nevertheless, depending on the used anesthetic, higher-level deviance responses are attenuated or eliminated, despite retained low-level responses to deviant stimuli, as for example under ketamine anesthesia (Ehrlichman et al., 2008; Uhrig et al., 2016). Uhrig et al. (2016) anesthetized macaque monkeys with propofol and ketamine and presented a local-global auditory task during anesthesia. The authors observed no local deviance responses during light propofol sedation and deep anesthesia. By contrast, the global effect was preserved in core auditory areas bilaterally and the MGN, as well as in the anterior cingulate and prefrontal areas, albeit with diminished activations compared to wakefulness. During anesthesia, the global effect was reduced compared to wakefulness in all brain regions.
Thalamic SSA responses were recorded during ketamine anesthesia in gerbils (Bäuerle et al., 2011). In order to control for auditory cortical regulatory effects on subcortical regions, the authors pharmacologically inactivated cortical regions using muscimol, which preserves subcortical auditory processing. Interestingly, this led to a reduction of responses in the MGB of the thalamus of the anesthetized gerbil. The authors interpreted their findings as a demonstration that SSA in subcortical structures is mainly regulated by the descending corticofugal system, highlighting a more general function in information processing than just novelty detection. Finally, another interesting study in anesthetized zebra finches (Beckers and Gahr, 2012) used a switching oddball paradigm with naturalistic short-range contact zebra finch social calls, different to usual zebra finch background vocalizations. Birds were anesthetized with isoflurane gas, which produces behavioral and physiological effects through binding at multiple targets in the brain and central nervous system (binding to GABAa receptors and enhancing GABAergic inhibition; blocking glutamate release by binding to NMDA receptors), and shows similar effects on EEG as propofol (Purdon et al., 2015). Results indicate deviance processing in secondary, but not primary, cortices, suggesting that deviant events, more than just stimulating a larger part of a single sensory processing network, may activate a different network compared to standards, eliciting more widespread activity. It is worth noting that social calls are more complex than the pure tones generally used in the majority of oddball paradigms, and thus might recruit more complex predictive mechanisms.
Conclusion
Overall, studies in humans and animals suggest that auditory predictions are reduced but may still be present in conditions of sedation and anesthesia. Interestingly, scalp EEG components corresponding to auditory predictive processes like the MMN or P3a may be preserved in anesthesia but are altered with respect to wakefulness. The latencies of scalp level auditory and deviance components are longer, and their amplitudes decrease. Moreover, the processing of deviant events at a local level is spatially restricted as shown via iEEG and source localization studies (Nourski et al., 2018; Zhang et al., 2018). Global deviance effects seem to be further restricted or even absent as the depth of anesthesia progresses in humans (Nourski et al., 2018; Shirazibeheshti et al., 2018), although they may be preserved in core auditory areas, at least in non-human primates (Uhrig et al., 2016). Importantly, similar to sleep, SSA is preserved also in anesthesia. These findings suggest that predictive processes are maintained to some degree under anesthesia, although they involve limited brain regions and sub-networks as compared to wakefulness.
Disorders of Consciousness
One important application of auditory deviance paradigms has been the prognosis of patients with disorders of consciousness (DOC; Lew et al., 2003; Kotchoubey, 2005; Wijnen et al., 2007; Tzovara et al., 2013). DOCs are defined as a disrupted relationship between the two components clinically defining consciousness – wakefulness/arousal and awareness (Laureys, 2005). Coma is characterized by the absence of arousal and awareness. The vegetative state (VS) or unresponsive wakefulness syndrome (UWS; Laureys et al., 2010) is described by some degree of arousal in the absence of awareness, and the minimally conscious state (MCS) is characterized by preserved arousal with varying signs of awareness (Gosseries et al., 2011; Figure 1). In contrast, in the locked-in syndrome, often a consequence of brainstem damage, patients are fully aware and awake, but suffer from complete paralysis of all voluntary muscles except for vertical eye movements, as in amyotrophic lateral sclerosis (Bauer et al., 1979; Sharma, 2011). The famous American case of patient Terri Schiavo (see e.g., Perry et al., 2005) is a good example of the important and nuanced medical, ethical, religious, social, familial, philosophical, and political debates around retained awareness and prognosis in patients suffering from DOC.
About 50% of patients emerging from coma are expected to evolve into a MCS (Giacino et al., 2006), which is difficult to differentially diagnose from UWS because of intermittent signs of consciousness in MCS patients (Fins et al., 2007). Despite the immense societal importance, DOCs remain among the most poorly understood conditions of modern neurology (Boly et al., 2012). For many years, clinical and behavioral examinations were the leading approaches to diagnosing retained consciousness (Plum and Posner, 1982), but this approach has high rates of misdiagnosis (Laureys, 2005). Electrophysiology typically using ERPs is currently used in the majority of studies investigating patients with DOC (see Giacino et al., 2006; Owen and Coleman, 2007; Demertzi et al., 2008; Boly, 2011; Boly et al., 2012; Gosseries et al., 2014b), and is applied to the search for a “consciousness marker” to be used in diagnosis of DOC.
Auditory Predictions and Their Link to Coma Outcome
Despite the heterogeneity of coma aetiologies and types of brain injury, several studies suggest that some patients in a coma can detect environmental deviant events at a neural level (Laureys et al., 2004; see also Table 3A for a summary). For instance, scalp EEG components such as the MMN and P300 correlate with patients’ outcome (Fischer et al., 1999; Kane et al., 2000; Luauté et al., 2005; Daltrozzo et al., 2007). Studies undertaken in the 90s have shown that some, but not all, coma patients may have preserved N100 (thought to reflect the early processing of acoustic features of a stimulus; Näätänen and Picton, 1987) and MMN responses, indicative of intact auditory deviance processing (Fischer et al., 1999; Daltrozzo et al., 2007). Interestingly, the presence of a MMN response was more frequently observed in patients who later awoke from coma (Fischer et al., 2004; Naccache et al., 2005), suggesting that the MMN is a predictor of patients’ chances of awakening. This hypothesis was driven by the fact that non-survivors generally did not show a MMN response (Fischer et al., 2004). However, these studies were performed several weeks or months after coma onset (Fischer et al., 2004; Boly, 2011).
More recent studies, performed in post-anoxic coma patients, have examined deviance processing in the acute coma phase, within the first 36 h of coma (Tzovara et al., 2013, 2016; Juan et al., 2016). In order to overcome the inherent difficulties associated with the detection of ERP components over single electrodes, these studies applied a multivariate decoding analysis (Tzovara et al., 2013) which models topographies of single-trial EEG activity. The model estimation was performed on a portion of the data (the training data set) and then used to decode the category of sounds (standard/deviant) in a separate portion of data. An above chance decoding performance implied a differential scalp EEG response to standard vs. deviant stimuli, focusing on the most discriminative time-windows within the trial. These studies have shown that during acute coma, even patients who do not survive show differential patterns of EEG activity in response to standard vs. deviant stimuli. Moreover, discrimination between standard and deviant sounds deteriorates from the first to the 2nd day of coma in non-survivors, while an improvement in auditory discrimination is observed for patients who later awake from coma (Tzovara et al., 2013, 2016).
More work in the acute coma phase using a local-global paradigm has shown that the global deviance effects, assessed via topographic patterns on scalp EEG, were preserved in 10 out of 24 patients (Tzovara et al., 2015). Moreover, while the global effect was not in itself predictive of the patient’s outcome, an improvement in decoding global standard vs. global deviant stimuli over the first 2 days of coma was informative of survival and return of consciousness (Tzovara et al., 2015).
The vast majority of deviance studies in coma target the auditory pathway, with the exception of one study comparing auditory and somatosensory stimuli, using the same oddball paradigm (Pfeiffer et al., 2018). Interestingly, this study found that discrimination between deviant and standard events at the EEG level is preserved in acute coma for both the auditory and somatosensory modalities. However, only the auditory modality was informative of coma outcome, with an improvement in auditory discrimination being indicative of survival. The specificity of deviance mechanisms for outcome prognosis is also highlighted by a study performed on the same type of patients, examining discrimination of naturalistic sounds, which, albeit preserved in some patients, was not informative of coma outcome (Cossy et al., 2014). Overall, these studies show that sensory deviance effects can be preserved in acute coma, suggesting a fundamental role for auditory predictions in relation to consciousness.
Auditory Predictions Differentiating Consciousness Levels
Unresponsive wakefulness syndrome is typically characterized by spared brainstem activity with widespread severe damage to gray and white matter in both cerebral hemispheres (Laureys et al., 2004). Although brainstem metabolism can be spared in UWS, preserving arousal and autonomic functions, several cortical regions, including prefrontal regions, parietotemporal and posterior parietal cortices, and the precuneus, are typically impaired (see Laureys et al., 2004 for a detailed review). Regarding patients, spared medial parietal cortex (precuneus) and adjacent posterior cingulate cortex metabolism seem to differentiate MCS from UWS (Laureys et al., 2004). Overall cortical metabolism is slightly higher in MCS than in UWS patients (Laureys, 2005).
Deviance effects are posited to correlate with retained consciousness in UWS and MCS patients (e.g., Wijnen et al., 2007; see Table 3B for a summary). While MMN and P300 can be recorded in both clinical groups, global deviance effects in active tasks (e.g., counting the number of deviant stimuli, but without behavioral responses) are only recorded in MCS, and thus are associated with the presence of residual levels of consciousness. A study using a passive and active oddball paradigm (i.e., where participants had to count the deviant stimulus) in MCS and UWS patients recorded MMN (between standard and deviant tones) and P300 (in response to the patients’ own name) responses in all but one patient (Risetti et al., 2013). Nevertheless, only in MCS did the P300 increase in amplitude during the active condition, corroborating the possible advantage of using this paradigm for probing awareness by bypassing the motor response. In a similar paradigm, Perrin et al. (2006) observed the P300 response to patients’ own name in 3 out of 5 UWS patients, and in all MCS patients, concluding that this ERP component is not specific enough to differentiate UWS ad MCS patients.
When regularities are established over groups of sounds, past studies have shown a link between global deviance effects in UWS patients and the presence of residual consciousness (Faugeras et al., 2011, 2012; King et al., 2013). Particularly, global deviance effects have been linked to conscious perception, mainly supported by the absence of evidence for a global deviance effect in UWS patients (Bekinschtein et al., 2009; Faugeras et al., 2012; King et al., 2013). Bekinschtein et al. (2009) measured local deviance effects in UWS/VS and MCS patients, but no global effects. King et al. (2013), observed a global effect in 14 % of UWS and 31 % of MCS patients. A more recent study reported that the presence of a global deviance effect in UWS patients is related to an eventual return of consciousness, while its absence is not informative of patients’ outcome (Perez et al., 2021). In particular, the majority of patients that showed a global effect eventually regained consciousness, while amongst patients that did not show a global effect some regained consciousness, and some did not, paralleling findings based on MMN (Fischer et al., 2004).
When investigated during recovery from UWS, the MMN was found to be an important predictor of recovery ability (Wijnen et al., 2007), as MMN amplitudes increased with recovery. Moreover, a sudden increase in amplitudes preceding overt external communication was interpreted as consolidation of the networks and mechanism supporting this ability. The study of functional connectivity supports this hypothesis (Boly et al., 2011). Boly et al. (2011) used a roving MMN paradigm in MCS and UWS patients and modeled cortical source activity using scalp EEG data to quantify backward and forward connections between temporal and frontal cortices during MMN generation. The authors found that compared to MCS and healthy controls, UWS patients had impaired connections from frontal to superior temporal cortex, but no impairments in connectivity within temporal cortical networks.
Conclusion
Taken together, studies in patients in a coma or with DOC show that scalp level EEG signatures of auditory predictive processes, including the MMN, may be preserved. An improvement of differential responses between standard and deviant stimuli over the 1st days of coma, or the presence of MMN responses in later coma stages, are frequently observed in patients that eventually regain consciousness.
Investigations of the neural circuits of predictive processes in patients with DOC remain sparse, and report that an impairment in predictive mechanisms may be accompanied by an impairment in backward connections from frontal to temporal cortical regions (Boly et al., 2011). One main challenge in studies with patients is pathological heterogeneity, for example relating to the cause of coma or DOC, to whether a focal lesion is present or not, or to the time of recording, as this may be followed by reconfigurations of brain networks supporting processing of environmental stimuli. Further studies of circuit level mechanisms are needed to better disentangle impaired and retained sensory predictive processes in patients with DOC, and link those to disease etiology and outcome.
Altered States of Consciousness
Altered states of consciousness were first defined in the late 60’s as “any mental state(s), induced by various physiological, psychological, or pharmacological maneuvers or agents. An altered state of consciousness can be recognized subjectively by the individual […] as representing a sufficient deviation in subjective experience or psychological functioning from certain general norms for that individual during alert, waking consciousness” (Ludwig, 1966). Despite the fact that all the above-mentioned states can be considered altered states of consciousness, we here focus on those states induced by hypnosis and meditation (see e.g., Vaitl et al., 2005, for a review) due to availability of research using MMN paradigms in these states.
The psychological mechanisms that hypnosis and meditation engage are distinct: while hypnotic suggestions are utilized to elicit changes in experience, meditation may be considered as a form of mental training that induces alterations in attention and self-regulation (Jamieson, 2016). A common feature of hypnosis and meditation is that both processes involve self-regulation, including attentional control and self-awareness. These involve sensory and frontal-parietal attentional systems that also support predictive processing (Tang et al., 2015; Jamieson, 2016). The human brain is hypothesized to use both perceptual and active inference to maximize the effectiveness of predictive processing: for perceptual inference internal models are adjusted to best fit perception using predictions that best explain the experienced sensory information, whereas active inference consists of performing actions that produce sensory input conforming to predictions (Martin and Pacherie, 2019). Perception in itself can be divided into exteroception (perception of the external world), proprioception (perception of one’s own motion and one’s body in space), and interoception (perception of one’s own homeostatically regulated physiological states) (Jamieson, 2016), all of which are used to generate predictive models of the world, our bodies and our mental states. As discussed below, the processes of perceptual and active inference are altered during both meditation and hypnosis through modified priors as well as through altered perception. Despite sparse research into the topic of auditory deviance processing in hypnosis and meditation, the few existing studies are worth discussing, due to insights they might offer into mechanisms of self-regulation.
Meditation
Meditation describes practices of self-regulation (Kabat-Zinn, 1982) and modulates the awareness component of consciousness (Brown and Ryan, 2004). Predictive processing during mindfulness meditation is thought to correspond to reductions in active inference and in the influence of priors (Pagnoni, 2019), as well as reduced stimulus salience weighting (Jamieson, 2016) – leading to reduced PEs, and thus reduced updating of expectancies, with parallel enhanced precision of proprioceptive and interoceptive predictions (Pagnoni, 2019). Collectively, these processes might lead to enhanced matching of interoceptive predictions and feedback (Jamieson, 2016), and thus to meta-awareness (Pagnoni, 2019).
Several ERP studies have investigated auditory oddball paradigms in mindfulness meditation (Cahn and Polich, 2009; Atchley et al., 2016; Biedermann et al., 2016; Fucci et al., 2018; see Table 4A for a summary). Cahn and colleagues compared a passive oddball task to a control thought period in expert meditators. They observed reduced amplitudes of the N1 and P2 components, representing early processing of acoustic features of a stimulus, and later P300 components to deviant tones and distractors (white noise), but not to standards (Cahn and Polich, 2009). Another study showed reductions in amplitudes of N1 and P2 components for all types of stimuli (standards, deviant, distractor), but not later P300, during mindfulness as compared to a tone detection task in expert and novice meditators versus controls (Atchley et al., 2016). A recent study in novice and expert meditators compared MMN responses during mindfulness meditation to a reading control condition (Biedermann et al., 2016). MMN amplitude was larger for both reading and meditation conditions in meditators as compared to controls. In novices, MMN responses were also increased during meditation as compared to reading. Taken together, these results indicate that mindfulness meditation might be associated with larger early sensory detection peaks for standard events, larger MMN responses and reduced P3a responses compared to normal wakefulness, which might be interpreted as greater environmental monitoring abilities, then applied to disengaging from distracting stimuli (supported by smaller early sensory detection peaks for distractors).
Hypnosis
Individuals who are susceptible to hypnosis are reported to experience changes in subjective awareness (Kihlstrom, 2005; Pekala, 2015). Hypnosis is thought to affect both active and perceptual inference, as well as perception, per se through attentional modulation (Jamieson, 2016, 2018; Martin and Pacherie, 2019). There are only a handful of studies investigating auditory predictive processes during hypnosis (Csépe et al., 1997; Kallio et al., 1999; Jamieson et al., 2005; Hiltunen et al., 2019; summarized in Table 4B). Perhaps the earliest systematic studies of this type were conducted by Gruzelier and colleagues (see Gruzelier, 1998, for a summary). In brief, medium-high hypnosis susceptible participants, but not low, showed decreased P300 to auditory oddballs and reduced MMN amplitudes during and following a hypnotic induction compared to pre-induction. By contrast, participants with low susceptibility showed an increase in MMN amplitudes following hypnotic induction. Measuring deviance responses in a passive oddball paradigm before the hypnotic induction and during neutral hypnosis (Kallio et al., 1999), as well as after the hypnosis in highly hypnotisable subjects (Jamieson et al., 2005; Hiltunen et al., 2019), and sometimes also using phonemes and participants with different levels of hypnotic suggestibility (Csépe et al., 1997), different studies demonstrate either increases or decreases of MMN amplitudes during hypnosis as compared to pre- or post-hypnosis. Another study found suppressed MMN amplitudes during hypnosis in highly hypnotisable subjects and no differences during waking between high, middle and low hypnotisable subjects (Csépe et al., 1997). While no changes were found in a recent study focusing on mean amplitude of ERP components from responses to standard and deviant sounds (Hiltunen et al., 2019), Jamieson et al. (2005) found increases in amplitude for MMN over frontal electrodes during hypnosis as compared to pre- and post-hypnosis in high suggestible participants (Jamieson et al., 2005). This trend was observed for these participants in temporal electrodes, too, but not for low suggestible participants, who showed linear increases in these electrodes from pre- to during to post-hypnosis. One possible interpretation for these results is that precision of deviance processing was enhanced, despite the engagement of attentional control with another active task.
Conclusion
As a general conclusion, it is hypothesized that both meditation and hypnosis modulate predictive processes manifesting through scalp EEG components. For meditation, the results are too sparse and heterogeneous to draw firm conclusions, highlighting the need for more research. To address these heterogeneous results, predictive processing theories offer testable hypotheses to assess these changes in awareness and subjective perception that are at the core of these states. Some of the seemingly inconsistent results in hypnosis and meditation emphasize the limitations of this literature: the focus on analysis of ERP components at single electrodes, the heterogeneity of instructions, high inter-individual variations, and the differences in statistical analyses and dependent variables, making it difficult to draw consistent conclusions. Future research can address these issues by focusing on replication studies using similar task instructions, and moving beyond analysis of single EEG electrodes, to measures that quantify the whole electrical field at the scalp level (see e.g., Michel and Murray, 2012).
Discussion and Future Outlook
A large body of literature has shown that sensory predictive signals manifest in the absence of consciousness. Here, we approached consciousness via states where consciousness is reduced or absent (sleep, anesthesia, disorders of consciousness), or altered (hypnosis, meditation). In the absence or alteration of consciousness, predictive processes can be preserved for predictions built over simple and long-lasting regularities. At the level of scalp EEG, evoked components associated with auditory predictions tend to have a reduced amplitude with decreasing levels of consciousness. At the level of generators, several studies suggest that the network underlying the generation of sensory predictions is restricted when conscious access and behavioral reactivity to the environment is lost. In the absence of consciousness, core auditory areas can preserve their capacities for generating deviance effects, while such effects in areas that are ‘higher’ in the sensory processing hierarchy (i.e., frontal areas) are abolished, likely as a result of disruption of connections from higher to lower regions.
However, as the generation of sensory predictions extends well beyond a two-node circuit of frontal-sensory areas, it remains an open question how each of the regions and the corresponding networks involved in sensory predictions is altered by the loss of consciousness. Importantly, the brain is a complex system, where mental states arise through the principle of emergence, and thus through an interaction of multiple functional, structural, and computational levels (Bassett and Gazzaniga, 2011). Within these computations, sensory predictive processes appear as a necessary, but not sufficient, condition for consciousness.
From an electrophysiological viewpoint, the loss of consciousness is accompanied by a plethora of changes in neural activity, such as the disruption of thalamo-cortical and cortico-cortical long-range connections, and changes in non-oscillatory components of the EEG (Magnin et al., 2010; Lendner et al., 2020, to name a few. These electrophysiological alterations may in turn affect circuit level mechanisms underlying predictions. Future studies should take into account these fundamental changes in neural activity when designing new experiments to study predictions in the absence of consciousness, and can choose to selectively stimulate specific states of neural activity, such as “up” or “down” sleep states.
In this review, we focused on neural signatures of predictive processes both at the neuronal level (e.g., SSA) and at the scalp EEG level (e.g., MMN or P300). The neural signals that can be recorded with scalp EEG have limited interpretation about the precise circuit or mechanisms underlying auditory predictions, because of the poor spatial resolution of EEG responses. Nevertheless, these scalp EEG components have strong clinical applications because of their relatively straightforward implementation (i.e., no invasive recordings are needed) that can facilitate their integration with other clinical measures to detect residual levels of consciousness.
From Electrophysiology to Computational Models
As the loss of consciousness engenders drastic changes to the predictive circuit, another important future question is how these changes affect the neural computations that lead to a predictive signal. Although theoretical modeling has been widely applied in the field of threat predictions (e.g., Tzovara et al., 2018), or reward learning (Abbott and Dayan, 2005), attempts to model sensory predictions are limited. This is important given the fact that scalp EEG responses associated with deviance processing such as the MMN are compound responses, reflecting multiple and complex processes from multiple brain regions and neural computations. Distinguishing which neural computations of deviance processing (e.g., adaptation, PEs, update of an internal model) are performed in different cortical and subcortical structures involved in the sensory predictive network is a crucial future necessity.
Previous studies have tested various theories of auditory PE generation, and have shown that trial-by-trial changes in deviance EEG responses are compatible with a Bayesian updating of a probabilistic model of the environment in the auditory (Lieder et al., 2013), somatosensory (Ostwald et al., 2012), and visual modalities (Stefanics et al., 2018). Modeling work has also supported claims that deviance effects reflect PE signals, weighted by the precision of predictions (Stefanics et al., 2018), with attention increasing the precision of PEs (Smout et al., 2019). Nevertheless, the MMN still remains opaque in terms of which computational components it represents, and which changes these components undergo when consciousness is lost.
A principled way to model PE signals comes from the field of reinforcement learning (see e.g., Hoy et al., 2021). When studying reward PEs, past studies have applied an axiomatic model developed in the field of economics to assess whether responses indeed reflect PEs (Caplin and Dean, 2008; Rutledge et al., 2010). Developed for testing dopamine-related hypotheses, namely whether the firing rate of midbrain dopamine neurons reflect PEs, these axioms represent necessary and sufficient conditions for a brain response to be considered a true PE signal. Given theoretical work drawing similarities between reward and sensory PEs (Gardner et al., 2018), future studies can investigate computational approaches to offer more objective means to disentangle complex constructs such as the MMN.
Regarding the ambiguity as to which computational components are altered when consciousness is lost, some first attempts to resolve this question have used ketamine, which was shown to diminish model quantities that correspond to PE signals related to higher order predictions, like transition probabilities (Weber et al., 2020). Another recent study examined how awareness and task-relevance affect the neural computations of the MMN component (Schlossmacher et al., 2021). When stimuli were task-irrelevant, both in unaware and aware conditions, the MMN was best explained by an adaptation model, whereas when stimuli were aware and task-relevant, the MMN was best explained by a precision-weighted prediction error. Interestingly, although the trial-by-trial N100 amplitude of the EEG response to repeated tones in UWS patients has been shown to change (Kotchoubey et al., 2006), indicative of cortical learning, to date there are no attempts to formally model such changes. Future studies will need to link the electrophysiological alterations that are observed in sensory predictions during sleep, coma or anesthesia to computational models, in order to obtain a mechanistic understanding of the neural computations underlying sensory predictions in the absence of consciousness.
An important future question is whether the presence or absence of consciousness can be linked to specific computations that result in the generation of prediction signals. It has been proposed that one of the main functions of consciousness is the generation of internal representations from incoming sensory input (Kanai et al., 2019) so that we can act meaningfully on this input (Hohwy, 2012). Under standard predictive theories, the influence of PEs depends on their precision (Auksztulewicz and Friston, 2015; Kanai et al., 2015), and, as explained previously, this is the effect of attentional selection. This means that ascending PEs with higher precision have more model-updating power than those with lower precision (Witon et al., 2020). Future studies can evaluate whether a similar computational role can be attributed to different states of consciousness and, in particular, according to their arousal and awareness contents.
Conclusion
In this review, we summarized studies investigating sensory predictions and their modulations by the loss of consciousness. We reviewed studies of animal and human physiology, from the fields of sleep, anesthesia, disorders of consciousness, hypnosis and meditation. Predictive processes represent a key, cross-species mechanism of perception, that manifests in an automatic way, and is embedded in distributed neuronal circuits. Refining our understanding of the neural networks and computations that underly sensory predictions in the physiological absence of consciousness (i.e., sleep or anesthesia) can advance our understanding of its pathological loss, and lead to improved, theory-driven strategies for diagnosis and prognostication in patients with disorders of consciousness.
Author Contributions
RT, RK, and AT wrote sections of the manuscript. All authors read and approved the submitted version.
Funding
This work was supported by the Interfaculty Research Cooperation “Decoding Sleep: From Neurons to Health and Mind” of the University of Bern and the Swiss National Science Foundation #320030_188737, NINDS Grant R37NS21135, and NIMH CONTE Center P50MH109429.
Conflict of Interest
The authors declare that the research was conducted in the absence of any commercial or financial relationships that could be construed as a potential conflict of interest.
Publisher’s Note
All claims expressed in this article are solely those of the authors and do not necessarily represent those of their affiliated organizations, or those of the publisher, the editors and the reviewers. Any product that may be evaluated in this article, or claim that may be made by its manufacturer, is not guaranteed or endorsed by the publisher.
Acknowledgments
We hereby wish to gratefully thank Graham Jamieson (University of New England) for his very helpful comments on the section “Altered States of Consciousness.”
References
Abbott, L. F., and Dayan, P. (2005). Theoretical Neuroscience: Computational and Mathematical Modeling of Neural Systems. Cambridge: MIT press.
Ahnaou, A., Huysmans, H., Biermans, R., Manyakov, N. V., and Drinkenburg, W. (2017). Ketamine: differential neurophysiological dynamics in functional networks in the rat brain. Transl. Psych. 7, e1237–e1237. doi: 10.1038/tp.2017.198
Aitchison, L., and Lengyel, M. (2017). With or without you: predictive coding and bayesian inference in the brain. Curr. Opin. Neurobiol. 46, 219–227. doi: 10.1016/j.conb.2017.08.010
Alain, C., and David, L. W. (1997). Attention modulates auditory pattern memory as indexed by event-related brain potentials. Psychophysiology 34, 534–546. doi: 10.1111/j.1469-8986.1997.tb01740.x
Alho, K. (1995). Cerebral generators of mismatch negativity (MMN) and its magnetic counterpart (MMNM) elicited by sound changes. Ear Hearing 16, 38–51. doi: 10.1097/00003446-199502000-00004
Anderson, L. A., Christianson, G. B., and Linden, J. F. (2009). Stimulus-specific adaptation occurs in the auditory thalamus. J. Neurosci. 29, 7359–7363. doi: 10.1523/jneurosci.0793-09.2009
Andrillon, T., Pressnitzer, D., Léger, D., and Kouider, S. (2017). Formation and suppression of acoustic memories during human sleep. Nat. Commun. 8:179. doi: 10.1038/s41467-017-00071-z
Anesthesiologists Task Force on Intraoperative Awareness (2006). Practice advisory for intraoperative awareness and brain function monitoring: a report by the american society of anesthesiologists task force on intraoperative awareness. Anesthesiology 104, 847–864.
Antunes, F. M., Nelken, I., Covey, E., and Malmierca, M. S. (2010). Stimulus-specific adaptation in the auditory thalamus of the anesthetized rat. PLoS One 5:e14071. doi: 10.1371/journal.pone.0014071
Astikainen, P., Ruusuvirta, T., Wikgren, J., and Penttonen, M. (2006). Memory-based detection of rare sound feature combinations in anesthetized rats. Neuroreport 17, 1561–1564. doi: 10.1097/01.wnr.0000233097.13032.7d
Atchley, R., Klee, D., Memmott, T., Goodrich, E., Wahbeh, H., and Oken, B. (2016). Event-related potential correlates of mindfulness meditation competence. Neuroscience 320, 83–92. doi: 10.1016/j.neuroscience.2016.01.051
Atienza, M., Cantero, J. L., and Dominguez-Marin, E. (2002). Mismatch negativity (MMN): an objective measure of sensory memory and long-lasting memories during sleep. Int. J. Psychophysiol. 46, 215–225. doi: 10.1016/s0167-8760(02)00113-7
Atienza, M., Cantero, J. L., and Escera, C. (2001). Auditory information processing during human sleep as revealed by event-related brain potentials. Clin. Neurophysiol. 112, 2031–2045. doi: 10.1016/S1388-2457(01)00650-2
Atienza, M., Cantero, J. L., and Gómez, C. M. (1997). The mismatch negativity component reveals the sensory memory during rem sleep in humans. Neurosci. Lett. 237, 21–24. doi: 10.1016/s0304-3940(97)00798-2
Auksztulewicz, R., and Friston, K. (2015). Attentional enhancement of auditory mismatch responses: a DCM/MEG study. Cerebral Cortex 25, 4273–4283. doi: 10.1093/cercor/bhu323
Banks, S., and Dinges, D. F. (2007). Behavioral and physiological consequences of sleep restriction. J. Clin. Sleep Med. 3, 519–528. doi: 10.5664/jcsm.26918
Bassett, D. S., and Gazzaniga, M. S. (2011). Understanding complexity in the human brain. Trends Cogn. Sci. 15, 200–209. doi: 10.1016/j.tics.2011.03.006
Bastos, A. M., Usrey, W. M., Adams, R. A., Mangun, G. R., Fries, P., and Friston, K. J. (2012). Canonical microcircuits for predictive coding. Neuron 76, 695–711. doi: 10.1016/j.neuron.2012.10.038
Bauer, G., Gerstenbrand, F., and Rumpl, E. (1979). Varieties of the locked-in syndrome. J. Neurol. 221, 77–91. doi: 10.1007/bf00313105
Bäuerle, P., von, der Behrens, W., Kössl, M., and Gaese, B. H. (2011). Stimulus-specific adaptation in the gerbil primary auditory thalamus is the result of a fast frequency-specific habituation and is regulated by the corticofugal system. J. Neurosci. 31, 9708–9722. doi: 10.1523/jneurosci.5814-10.2011
Beckers, G. J. L., and Gahr, M. (2012). Large-scale synchronized activity during vocal deviance detection in the zebra finch auditory forebrain. J. Neurosci. 32, 10594–10608. doi: 10.1523/jneurosci.6045-11.2012
Bekinschtein, T. A., Dehaene, S., Rohaut, S., Tadel, F., Cohen, L., and Naccache, L. (2009). Neural signature of the conscious processing of auditory regularities. Proc. Natl. Acad. Sci. U.S.A 106, 1672–1677. doi: 10.1073/pnas.0809667106
Biedermann, B., De Lissa, P., Mahajan, Y., Polito, V., Badcock, N., Connors, M. H., et al. (2016). Meditation and auditory attention: an ERP study of meditators and non-meditators. Int. J. Psychophysiol. 109, 63–70. doi: 10.7287/peerj.preprints.2318
Blackford, J. U., Buckholtz, J. W., Avery, S. N., and Zald, D. H. (2010). A unique role for the human amygdala in novelty detection. Neuroimage 50, 1188–1193. doi: 10.1016/j.neuroimage.2009.12.083
Boly, M. (2011). Measuring the fading consciousness in the human brain. Curr. Opin. Neurol. 24, 394–400. doi: 10.1097/wco.0b013e328347da94
Boly, M., Garrido, M. I., Gosseries, O., Bruno, M. A., Boveroux, P., Schnakers, C., et al. (2011). Preserved feedforward but impaired top-down processes in the vegetative state. Science 332, 858–862. doi: 10.1126/science.1202043
Boly, M., Massimini, M., Garrido, M. I., Gosseries, O., Noirhomme, Q., Laureys, S., et al. (2012). Brain connectivity in disorders of consciousness. Brain Connectivity 2, 1–10. doi: 10.1089/brain.2011.0049
Bortoletto, M., Tona, G. D. M., Scozzari, S., Sarasso, S., and Stegagno, L. (2011). Effects of sleep deprivation on auditory change detection: a N1-mismatch negativity study. Int. J. Psychophysiol. 81, 312–316. doi: 10.1016/j.ijpsycho.2011.07.017
Braun, A. R., Balkin, T. J., Wesenten, N. J., Carson, R. E., Varga, M., Baldwin, P. I., et al. (1997). Regional cerebral blood flow throughout the sleep-wake cycle. An H2(15)O PET study. Brain 120, 1173–1197. doi: 10.1093/brain/120.7.1173
Brown, E. N., Lydic, R., and Schiff, N. D. (2010). General anesthesia, sleep, and coma. N. Engl. J. Med. 363, 2638–2650. doi: 10.1056/nejmra0808281
Brown, K. W., and Ryan, R. M. (2004). Perils and promise in defining and measuring mindfulness: observations from experience. Clin. Psychol. 11, 242–248. doi: 10.1093/clipsy.bph078
Cacciaglia, R., Escera, C., Slabu, L., Grimm, S., Sanjuán, A., Ventura-Campos, N., et al. (2015). Involvement of the human midbrain and thalamus in auditory deviance detection. Neuropsychologia 68, 51–58.
Cahn, B. R., and Polich, J. (2009). Meditation (vipassana) and the P3a event-related brain potential. Int. J. Psychophysiol. 72, 51–60. doi: 10.1016/j.ijpsycho.2008.03.013
Camman, R., Paavilainen, P., Alho, K., Näätänen, R., Reinikainen, K., and Sams, S. (1987). Disappearence of mismatch negativity during sleep. Int. J. Neurosci. 34, 154–155.
Campbell, K. B., and Colrain, I. M. (2002). Event-related potential measures of the inhibition of information processing: II. The sleep onset period. Int. J. Psychophysiol. 46, 197–214. doi: 10.1016/s0167-8760(02)00112-5
Caplin, A., and Dean, M. (2008). Axiomatic methods, dopamine and reward prediction error. Curr. Opin. Neurobiol. 18, 197–202. doi: 10.1016/j.conb.2008.07.007
Cappotto, D., Auksztulewicz, R., Kang, H., Poeppel, D., Melloni, L., and Schnupp, J. (2021). Decoding the content of auditory sensory memory across species. Cerebral Cortex. 31, 3226–3236. doi: 10.1093/cercor/bhab002
Carbajal, G. V., and Malmierca, M. S. (2018). The neuronal basis of predictive coding along the auditory pathway: from the subcortical roots to cortical deviance detection. Trends Hearing 22:2331216518784822. doi: 10.1177/2331216518784822
Chen, I.-W., Helmchen, F., and Lütcke, H. (2015). Specific early and late oddball-evoked responses in excitatory and inhibitory neurons of mouse auditory cortex. J. Neurosci. 35, 12560–12573. doi: 10.1523/jneurosci.2240-15.2015
Chennu, S., and Bekinschtein, T. A. (2012). Arousal modulates auditory attention and awareness: insights from sleep, sedation, and disorders of consciousness. Front. Psychol. 3:65. doi: 10.3389/fpsyg.2012.00065
Chennu, S., Noreika, V., Gueorguiev, D., Blenkmann, A., Kochen, S., Ibánez, A., et al. (2013). Expectation and attention in hierarchical auditory prediction. J. Neurosci. 33, 11194–11205. doi: 10.1523/jneurosci.0114-13.2013
Chennu, S., Noreika, V., Gueorguiev, D., Shtyrov, Y., Bekinschtein, T. A., and Henson, R. (2016). Silent expectations: dynamic causal modeling of cortical prediction and attention to sounds that weren’t. J. Neurosci. 36, 8305–8316. doi: 10.1523/jneurosci.1125-16.2016
Christianson, G. B., Chait, M., de Cheveigné, A., and Linden, J. F. (2014). Auditory evoked fields measured noninvasively with small-animal meg reveal rapid repetition suppression in the guinea pig. J. Neurophysiol. 112, 3053–3065. doi: 10.1152/jn.00189.2014
Clarke, J. M., Halgren, E., and Chauvel, P. (1999). Intracranial erps in humans during a lateralized visual oddball task: II. Temporal, parietal, and frontal recordings. Clin. Neurophysiol. 110, 1226–1244. doi: 10.1016/s1388-2457(99)00064-4
Coenen, A. M. L., and Drinkenburg, W. H. I. M. (2002). Animal models for information processing during sleep. Int. J. Psychophysiol. 46, 163–175. doi: 10.1016/s0167-8760(02)00110-1
Colrain, I. M., and Campbell, K. B. (2007). The use of evoked potentials in sleep research. Sleep Med. Rev. 11, 277–293. doi: 10.1016/j.smrv.2007.05.001
Condon, C. D., and Weinberger, N. M. (1991). Habituation produces frequency-specific plasticity of receptive fields in the auditory cortex. Behav. Neurosci. 105:416. doi: 10.1037/0735-7044.105.3.416
Cossy, N., Tzovara, A., Simonin, A., Rossetti, A. O., and De Lucia, M. (2014). Robust discrimination between eeg responses to categories of environmental sounds in early coma. Front. Psychol. 5:155. doi: 10.3389/fpsyg.2014.00155
Cote, K. A. (2002). Probing awareness during sleep with the auditory odd-ball paradigm. Int. J. Psychophysiol. 46, 227–241. doi: 10.1016/s0167-8760(02)00114-9
Cox, R., van Driel, J., de Boer, M., and Talamini, L. M. (2014). Slow oscillations during sleep coordinate interregional communication in cortical networks. J. Neurosci. 34, 16890–16901. doi: 10.1523/jneurosci.1953-14.2014
Csépe, V., Bányai, E. I., and Mády, K. (1997). Changes of phoneme contrast sensitivity in hypnosis: an Erp study. Int. J. Psychophysiol. 1:79. doi: 10.1016/s0167-8760(97)85567-5
Csépe, V., Karmos, G., and Molnar, M. (1987). Evoked potential correlates of stimulus deviance during wakefulness and sleep in cat—animal model of mismatch negativity. Electroencephalogr. Clin. Neurophysiol. 66, 571–578. doi: 10.1016/0013-4694(87)90103-9
Czisch, M., Wehrle, R., Stiegler, A., Peters, H., Andrade, K., Holsboer, F., et al. (2009). Acoustic oddball during NREM sleep: a combined EEG/fMRI study. PLoS One 4:e6749. doi: 10.1371/journal.pone.0006749
Czisch, M., Wetter, T. C., Kaufmann, C., Pollmächer, T., Holsboer, F., and Auer, D. P. (2002). Altered processing of acoustic stimuli during sleep: reduced auditory activation and visual deactivation detected by a combined fMRI/Eeg study. Neuroimage 16, 251–258. doi: 10.1006/nimg.2002.1071
Daltrozzo, J., Wioland, N., Mutschler, V., and Kotchoubey, B. (2007). Predicting coma and other low responsive patients outcome using event-related brain potentials: a meta-analysis. Clin. Neurophysiol. 118, 606–614. doi: 10.1016/j.clinph.2006.11.019
Dang-Vu, T. T., Bonjean, M., Schabus, M., Boly, M., Darsaud, A., Desseilles, M., et al. (2011). Interplay Between spontaneous and induced brain activity during human non-rapid eye movement sleep. Proc. Natl. Acad. Sci. U.S. A. 108, 15438–15443. doi: 10.1073/pnas.1112503108
Dayan, P., Hinton, G. E., Neal, R. N., and Zemel, R. S. (1995). The helmholtz machine. Neural Comput. 7, 889–904. doi: 10.1162/neco.1995.7.5.889
Dehaene, S., and Changeux, J. P. (2011). Experimental and theoretical approaches to conscious processing. Neuron 70, 200–227. doi: 10.1016/j.neuron.2011.03.018
Demertzi, A., Vanhaudenhuyse, A., Bruno, M.-A., Schnakers, C., Boly, M., Boveroux, P., et al. (2008). Is there anybody in there? detecting awareness in disorders of consciousness. Exp. Rev. Neurother. 8, 1719–1730. doi: 10.1586/14737175.8.11.1719
Destexhe, A., Hughes, S. W., Rudolph, M., and Crunelli, V. (2007). Are corticothalamic ‘up’ states fragments of wakefulness? Trends Neurosci. 30, 334–342. doi: 10.1016/j.tins.2007.04.006
Donchin, E. (1981). Surprise!? Surprise?. Psychophysiology 18, 493–513. doi: 10.1111/j.1469-8986.1981.tb01815.x
Donchin, E., and Coles, M. G. H. (1988). Is the p300 component a manifestation of context updating? Behav. Brain Sci. 11:357. doi: 10.1017/s0140525x00058027
Drummond, S. P. A., Smith, M. T., Orff, H. J., Chengazi, V., and Perlis, M. L. (2004). Functional imaging of the sleeping brain: review of findings and implications for the study of insomnia. Sleep Med. Rev. 8, 227–242. doi: 10.1016/j.smrv.2003.10.005
Duque, D., and Malmierca, M. S. (2015). Stimulus-specific adaptation in the inferior colliculus of the mouse: anesthesia and spontaneous activity effects. Brain Struct. Funct. 220, 3385–3398. doi: 10.1007/s00429-014-0862-1
Duque, D., Ayala, Y. A., and Malmierca, M. S. (2015). Deviance detection in auditory subcortical structures: what can we learn from neurochemistry and neural connectivity? Cell Tissue Res. 361, 215–232. doi: 10.1007/s00441-015-2134-7
Duque, D., Pais, R., and Malmierca, M. S. (2018). Stimulus-specific adaptation in the anesthetized mouse revealed by brainstem auditory evoked potentials. Hearing Res. 370, 294–301. doi: 10.1016/j.heares.2018.08.011
Dürschmid, S., Reichert, C., Hinrichs, H., Heinze, H. J., Kirsch, H. E., Knight, R. T., et al. (2019). Direct evidence for prediction signals in frontal cortex independent of prediction error. Cerebral Cortex 29, 4530–4538. doi: 10.1093/cercor/bhy331
Dürschmid, S., Zaehle, T., Hinrichs, H., Heinze, H.-J., Voges, J., Garrido, M. I., et al. (2016). Sensory deviancy detection measured directly within the human nucleus accumbens. Cerebral Cortex 26, 1168–1175. doi: 10.1093/cercor/bhu304
Edeline, J. M., Manunta, Y., and Hennevin, E. (2000). Auditory thalamus neurons during sleep: changes in frequency selectivity, threshold, and receptive field size. J. Neurophysiol. 84, 934–952. doi: 10.1152/jn.2000.84.2.934
Edeline, J.-M., Dutrieux, G., Manunta, Y., and Hennevin, E. (2001). Diversity of receptive field changes in auditory cortex during natural sleep. Eur. J. Neurosci. 14, 1865–1880. doi: 10.1046/j.0953-816x.2001.01821.x
Ehrlichman, R. S., Maxwell, C. R., Majumdar, S., and Siegel, S. J. (2008). Deviance-elicited changes in event-related potentials are attenuated by ketamine in mice. J. Cogn. Neurosci. 20, 1403–1414. doi: 10.1162/jocn.2008.20097
Eriksson, J., and Villa, A. E. P. (2005). Event-related potentials in an auditory oddball situation in the rat. Biosystems 79, 207–212. doi: 10.1016/j.biosystems.2004.09.017
Faugeras, F., Rohaut, B., Weiss, N., Bekinschtein, T. A., Galanaud, D., Puybasset, L., et al. (2011). Probing consciousness with event-related potentials in the vegetative state. Neurology 77, 264–268. doi: 10.1212/wnl.0b013e3182217ee8
Faugeras, F., Rohaut, B., Weiss, N., Bekinschtein, T., Galanaud, D., Puybasset, L., et al. (2012). Event related potentials elicited by violations of auditory regularities in patients with impaired consciousness. Neuropsychologia 50, 403–418. doi: 10.1016/j.neuropsychologia.2011.12.015
Field, K. J., White, W. J., and Lang, M. C. (1993). Anaesthetic effects of chloral hydrate, pentobarbitone and urethane in adult male rats. Lab. Anim. 27, 258–269. doi: 10.1258/002367793780745471
Fins, J. J., Master, M. G., Gerber, L. M., and Giacino, J. T. (2007). The minimally conscious state: a diagnosis in search of an epidemiology. Archiv. Neurol. 64, 1400–1405. doi: 10.1001/archneur.64.10.1400
Fischer, C., Luaute, J., Adeleine, P., and Morlet, D. (2004). Predictive value of sensory and cognitive evoked potentials for awakening from coma. Neurology 63, 669–673. doi: 10.1212/01.wnl.0000134670.10384.e2
Fischer, C., Morlet, D., Bouchet, P., Luaute, J., Jourdan, C., and Salord, F. (1999). Mismatch negativity and late auditory evoked potentials in comatose patients. Clin. Neurophysiol. 110, 1601–1610. doi: 10.1016/s1388-2457(99)00131-5
Fonken, Y. M., Kam, J. W. Y., and Knight, R. T. (2019). A differential role for human hippocampus in novelty and contextual processing: implications for P300. Psychophysiology 57:400. doi: 10.1111/psyp.13400
Friston, K. (2005). A theory of cortical responses. Philosoph. Transact. Roy. Soc. B 360, 815–836. doi: 10.1098/rstb.2005.1622
Fucci, E., Abdoun, O., Caclin, A., Francis, A., Dunne, J. D., Ricard, M. R. J., et al. (2018). Differential Effects of non-dual and focused attention meditations on the formation of automatic perceptual habits in expert practitioners. Neuropsychologia 119, 92–100. doi: 10.1016/j.neuropsychologia.2018.07.025
Gaese, B. H., and Ostwald, J. (2001). Anesthesia changes frequency tuning of neurons in the rat primary auditory cortex. J. Neurophysiol. 86, 1062–1066. doi: 10.1152/jn.2001.86.2.1062
Gao, P. P., Zhang, J. W., Cheng, J. S., Zhou, I. Y., and Wu, E. X. (2014). The inferior colliculus is involved in deviant sound detection as revealed by bold fMRI. Neuroimage 91, 220–227. doi: 10.1016/j.neuroimage.2014.01.043
Gardner, M. P. H., Schoenbaum, G., and Gershman, S. J. (2018). Rethinking dopamine as generalized prediction error. Proc. Roy. Soc. B 285:20181645. doi: 10.1098/rspb.2018.1645
Garrido, M. I., Friston, K. J., Kiebel, S. J., Stephan, K. E., Baldeweg, T., and Kilner, J. M. (2008). The functional anatomy of the MMN: a DCM study of the roving paradigm. Neuroimage 42, 936–944. doi: 10.1016/j.neuroimage.2008.05.018
Garrido, M. I., Kilner, J. M., Kiebel, S. J., and Friston, K. J. (2009a). Dynamic causal modeling of the response to frequency deviants. J. Neurophysiol. 101, 2620–2631. doi: 10.1152/jn.90291.2008
Garrido, M. I., Kilner, J. M., Kiebel, S. J., Stephan, K. E., and Friston, K. J. (2007). Dynamic causal modelling of evoked potentials: a reproducibility study. Neuroimage 36, 571–580. doi: 10.1016/j.neuroimage.2007.03.014
Garrido, M. I., Kilner, J. M., Stephan, K. E., and Friston, K. J. (2009b). The mismatch negativity: a review of underlying mechanisms. Clin. Neurophysiol. 120, 453–463. doi: 10.1016/j.clinph.2008.11.029
Giacino, J. T., Fins, J. J., Laureys, S., and Schiff, N. D. (2014). Disorders of consciousness after acquired brain injury: the state of the science. Nat. Rev. Neurol. 10:99. doi: 10.1038/nrneurol.2013.279
Giacino, J. T., Hirsch, J., Schiff, N., and Laureys, S. (2006). Functional neuroimaging applications for assessment and rehabilitation planning in patients with disorders of consciousness. Archiv. Phys. Med. Rehabilitat. 87, 67–76. doi: 10.1016/j.apmr.2006.07.272
Gosseries, O., Di, H., Laureys, S., and Boly, M. (2014a). Measuring consciousness in severely damaged brains. Annu. Rev. Neurosci. 37, 457–478. doi: 10.1146/annurev-neuro-062012-170339
Gosseries, O., Vanhaudenhuyse, A., Bruno, M.-A., Demertzi, A., Schnakers, C., Boly, M. M., et al. (2011). Disorders of Consciousness: Coma, Vegetative and Minimally Conscious States. Netherland: Springer, 29–55. doi: 10.1007/978-3-642-18047-7_2
Gosseries, O., Zasler, N. D., and Laureys, S. (2014b). Recent advances in disorders of consciousness: focus on the diagnosis. Brain INJ. 28, 1141–1150. doi: 10.3109/02699052.2014.920522
Goupil, L., and Bekinschtein, T. (2012). Cognitive processing during the transition to sleep. Archiv. Ital. Biol. 150, 140–154. doi: 10.4449/aib.v150i2.1247
Gruzelier, J. (1998). A working model of the neurophysiology of hypnosis: a review of evidence. Contemp. Hypn. 15, 3–21. doi: 10.1002/ch.112
Guldenmund, P., Vanhaudenhuyse, A., Sanders, R. D., Sleigh, J., Bruno, M. A., Demertzi, A., et al. (2017). Brain functional connectivity differentiates dexmedetomidine from propofol and natural sleep. Br. J. Anaesth. 119, 674–684. doi: 10.1093/bja/aex257
Halgren, E., Patrick, B., Clarke, J. M., Heit, G., Liégeois, C., Chauvel, P., et al. (1995). Intracerebral potentials to rare target and distractor auditory and visual stimuli. I. superior temporal plane and parietal lobe. Electroencephalogr. Clin. Neurophysiol. 94, 191–220. doi: 10.1016/0013-4694(94)00259-n
Halgren, E., Squires, N. K., Wilson, C. L., Rohrbaugh, J. W., Babb, T. L., and Crandall, P. H. (1980). Endogenous potentials generated in the human hippocampal formation and amygdala by infrequent events. Science 210, 803–805. doi: 10.1126/science.7434000
Hall, R. D., and Borbely, A. A. (1970). Acoustically evoked potentials in the rat during sleep and waking. Exp. Brain Res. 11, 93–110. doi: 10.1007/bf00234203
Hari, R., Hämäläinen, H., Ilmoniemi, R., Kaukoranta, E., Reinikainen, K., Salminen, J., et al. (1984). Responses of the primary auditory cortex to pitch changes in a sequence of tone pips: neuromagnetic recordings in man. Neurosci. Lett. 50, 127–132. doi: 10.1016/0304-3940(84)90474-9
Harms, L., Fulham, W. R., Todd, J., Budd, T. W., Hunter, M., Meehan, C., et al. (2014). Mismatch negativity (mmn) in freely-moving rats with several experimental controls. PLoS One 9:e110892. doi: 10.1371/journal.pone.0110892
Heilbron, M., and Chait, M. (2018). Great expectations: is there evidence for predictive coding in auditory cortex? Neuroscience 389, 54–73. doi: 10.1016/j.neuroscience.2017.07.061
Heinke, W., and Koelsch, S. (2005). The effects of anesthetics on brain activity and cognitive function. Curr. Opin. Anesthesiol. 18, 625–631. doi: 10.1097/01.aco.0000189879.67092.12
Heinke, W., Kenntner, R., Gunter, T. C., Sammler, D., Olthoff, D., and Koelsch, S. (2004). Sequential effects of increasing propofol sedation on frontal and temporal cortices as indexed by auditory event-related potentials. J. Am. Soc. Anesthesiol. 100, 617–625. doi: 10.1097/00000542-200403000-00023
Hennevin, E., Huetz, C., and Edeline, J. M. (2007). Neural representations during sleep: from sensory processing to memory traces. Neurobiol. Learn. Mem. 87, 416–440. doi: 10.1016/j.nlm.2006.10.006
Hiltunen, S., Virta, M., Kallio, S., and Paavilainen, P. (2019). The effects of hypnosis and hypnotic suggestions on the mismatch negativity in highly hypnotizable subjects. Int. J. Clin. Exp. Hypn. 67, 192–216. doi: 10.1080/00207144.2019.1580966
Hobson, J. A., Pace-Schott, E. F., and Stickgold, R. (2000). Dreaming and the brain: toward a cognitive neuroscience of conscious states. Behav. Brain Sci. 23, 793–842. doi: 10.1017/s0140525x00003976
Hohwy, J. (2012). Attention and conscious perception in the hypothesis testing brain. Front. Psychol. 3:96. doi: 10.3389/fpsyg.2012.00096
Horovitz, S. G., Braun, A. R., Carr, W. S., Picchioni, D., Balkin, T. J., Fukunaga, M., et al. (2009). Decoupling of the brain’s default mode network during deep sleep. Proc. Natl. Acad. Sci. U.S. A. 106, 11376–11381. doi: 10.1073/pnas.0901435106
Hoy, C. W., Steiner, S. C., and Knight, R. T. (2021). Single-trial modeling separates multiple overlapping prediction errors during reward processing in human EEG. Commun. Biol. 4:910. doi: 10.1038/s42003-021-02426-1
Huang, L., Bai, L., Zhao, Y., and Xiao, Z. (2013). Comparison of tonal response properties of primary auditory cortex neurons of adult rats under urethane and ketamine anesthesia. J. South. Med. Univ. 33, 785–793. doi: 10.1016/j.heares.2016.06.012
Imada, A., Morris, A., and Wiest, M. C. (2013). Deviance detection by a P3-like response in rat posterior parietal cortex. Front. Integr. Neurosci. 6:127. doi: 10.3389/fnint.2012.00127
Issa, E. B., and Wang, X. (2008). Sensory responses during sleep in primate primary and secondary auditory cortex. J. Neurosci. 28, 14467–14480. doi: 10.1523/jneurosci.3086-08.2008
Jääskeläinen, I. P., Ahveninen, J., Bonmassar, G., Dale, A. M., Ilmoniemi, R. J., Levänen, S., et al. (2004). Human posterior auditory cortex gates novel sounds to consciousness. Proc. Natl. Acad. Sci. U.S.A. 101, 6809–6814. doi: 10.1073/pnas.0303760101
Jamieson, G. A. (2016). “A unified theory of hypnosis and meditation states: the interoceptive predictive coding approach,” in Hypnosis and meditation: Towards an integrative science of conscious planes, eds A. Raz and M. Lifshitz (Oxford: Oxford University Press), 313–342.
Jamieson, G. A. (2018). Expectancies of the future in hypnotic suggestions. Psychol. Consci. 5, 258–277. doi: 10.1037/cns0000170
Jamieson, G. A., Dwivedi, P., and Gruzelier, J. H. (2005). Changes in mismatch negativity across pre-hypnosis, hypnosis and post-hypnosis conditions distinguish high from low hypnotic susceptibility groups. Brain Res. Bull. 67, 298–303. doi: 10.1016/j.brainresbull.2005.06.033
Javitt, D. C., Schroeder, C. E., Steinschneider, M., Arezzo, J. C., and Vaughan, H. G. Jr. (1992). Demonstration of mismatch negativity in the monkey. Electroencephalogr. Clin. Neurophysiol. 83, 87–90. doi: 10.1016/0013-4694(92)90137-7
Javitt, D. C., Steinschneider, M., Schroeder, C. E., Vaughan, H. G. Jr., and Arezzo, J. C. (1994). Detection of stimulus deviance within primate primary auditory cortex: intracortical mechanisms of mismatch negativity (MMN) generation. Brain Res. 667, 192–200. doi: 10.1016/0006-8993(94)91496-6
Johnson, C. B., and Taylor, P. M. (1998). Comparison of the effects of halothane, isoflurane and methoxyflurane on the electroencephalogram of the horse. Br. J. Anaesth. 81, 748–753. doi: 10.1093/bja/81.5.748
Johnson, E., Kam, J., Tzovara, A., Robert, T., and Knight, R. T. (2020). Insights into human cognition from intracranial eeg: a review of audition, memory, internal cognition, and causality. J. Neural Engin. 17:051001. doi: 10.1088/1741-2552/abb7a5
Juan, E., De Lucia, M., Tzovara, A., Beaud, V., Oddo, M., Clarke, S., et al. (2016). Prediction of cognitive outcome based on the progression of auditory discrimination during coma. Resuscitation 106, 89–95. doi: 10.1016/j.resuscitation.2016.06.032
Kabat-Zinn, J. (1982). An outpatient program in behavioral medicine for chronic pain patients based on the practice of mindfulness meditation: theoretical considerations and preliminary results. Gener. Hosp. Psych. 4, 33–47. doi: 10.1016/0163-8343(82)90026-3
Kallio, S., Revonsuo, A., Lauerma, H., Hämäläinen, H., and Lang, H. (1999). The MMN amplitude increases in hypnosis: a case study. NeuroReport 10, 3579–3582. doi: 10.1097/00001756-199911260-00021
Kam, J. W. Y., Helfrich, R. F., Solbakk, A.-K., Endestad, T., Larsson, P. G., Lin, J. J., et al. (2020). Top- down attentional modulation in human frontal cortex: differential engagement during external and internal attention. Cerebral Cortex 31, 873–883. doi: 10.1093/cercor/bhaa262
Kanai, R., Chang, A., Yu, Y., Magrans, de Abril, I., Biehl, M., et al. (2019). Information generation as a functional basis of consciousness. Neurosci. Conscious. 2019:niz016. doi: 10.1093/nc/niz016
Kanai, R., Komura, Y., Shipp, S., and Friston, K. (2015). Cerebral hierarchies: predictive processing, precision and the pulvinar. Philosoph. Transact. Roy. Soc. B 370:20140169. doi: 10.1098/rstb.2014.0169
Kane, N. M., Butler, S. R., and Simpson, T. (2000). Coma outcome prediction using event-related potentials: P3 and mismatch negativity. Audiol. Neurotol. 5, 186–191. doi: 10.1159/000013879
Key, A. P. F., Molfese, D. L., O’Brien, L., and Gozal, D. (2009). Sleep-disordered breathing affects auditory processing in 5–7-year-old children: evidence from brain recordings. Devel. Neuropsychol. 34, 615–628. doi: 10.1080/87565640903133608
Khouri, L., and Nelken, I. (2015). Detecting the unexpected. Curr. Opin. Neurobiol. 35, 142–147. doi: 10.1016/j.conb.2015.08.003
Kihlstrom, J. F. (2005). Is hypnosis an altered state of consciousness or what? Contemp. Hypnosis 22, 34–38. doi: 10.1002/ch.20
King, C., McGee, T., Rubel, E. W., Nicol, T., and Kraus, N. (1995). Acoustic features and acoustic change are represented by different central pathways. Hearing Res. 85, 45–52.
King, J.-R., Faugeras, F., Gramfort, A., Schurger, A., El Karoui, I., Sitt, J. D., et al. (2013). Single-trial decoding of auditory novelty responses facilitates the detection of residual consciousness. Neuroimage 83, 726–738. doi: 10.1016/j.neuroimage.2013.07.013
Kisley, M. A., and Gerstein, G. L. (1999). Trial-to-trial variability and state-dependent modulation of auditory-evoked responses in cortex. J. Neurosci. 19, 10451–10460. doi: 10.1016/0378-5955(95)00028-3
Koelsch, S., Heinke, W., Sammler, D., and Olthoff, D. (2006). Auditory processing during deep propofol sedation and recovery from unconsciousness. Clin. Neurophysiol. 117, 1746–1759. doi: 10.1016/j.clinph.2006.05.009
Koike, T., Kan, S., Misaki, M., and Miyauchi, S. (2011). Connectivity pattern changes in default-mode network with deep non-REM and REM sleep. Neurosci. Res. 69, 322–330. doi: 10.1016/j.neures.2010.12.018
Kok, P., and de Lange, F. P. (2015). Predictive coding in sensory cortex. Introduct. Model Based Cogn. Neurosci. 18, 221–244. doi: 10.1007/978-1-4939-2236-9_11
Kotchoubey, B. (2005). Event-related potential measures of consciousness: two equations with three unknowns. Progr. Brain Res. 150, 427–444. doi: 10.1016/s0079-6123(05)50030-x
Kotchoubey, B., Jetter, U., Lang, S., Semmler, A., Mezger, G., Schmalohr, D., et al. (2006). Evidence of cortical learning in vegetative state. J. Neurol. 253, 1374–1376. doi: 10.1007/s00415-006-0221-0
Kotchoubey, B., Lang, S., Mezger, G., Schmalohr, D., Schneck, M., Semmler, A., et al. (2005). Information processing in severe disorders of consciousness: vegetative state and minimally conscious state. Clin. Neurophysiol. 116, 2441–2453. doi: 10.1016/j.clinph.2005.03.028
Kraus, N., McGee, T., Littman, T., Nicol, T., and King, C. (1994). Nonprimary auditory thalamic representation of acoustic change. J. Neurophysiol. 72, 1270–1277. doi: 10.1152/jn.1994.72.3.1270
Kropotov, J. D., Alho, K., Näätänen, R., Ponomarev, V. A., Kropotova, O. V., Anichkov, A. D., et al. (2000). Human auditory-cortex mechanisms of preattentive sound discrimination. Neurosci. Lett. 280, 87–90. doi: 10.1016/s0304-3940(00)00765-5
Laureys, S. (2005). The neural correlate of (un) awareness: lessons from the vegetative state. Trends Cogn. Sci. 9, 556–559. doi: 10.1016/j.tics.2005.10.010
Laureys, S., Celesia, G. G., Cohadon, F., Lavrijsen, J., León-Carrión, J., Sannita, W. G., et al. (2010). Unresponsive wakefulness syndrome: a new name for the vegetative state or apallic syndrome. BMC Medicine 8:68. doi: 10.1186/1741-7015-8-68
Laureys, S., Owen, A. M., and Schiff, N. D. (2004). Brain function in coma, vegetative state, and related disorders. Lancet Neurol. 3, 537–546. doi: 10.1016/s1474-4422(04)00852-x
Lazar, R., and Metherate, R. (2003). Spectral interactions, but no mismatch negativity, in auditory cortex of anesthetized rat. Hearing Res. 181, 51–56. doi: 10.1016/s0378-5955(03)00166-7
Leite, F. A. C., da Silva, F. F., Pradella-Hallinan, M., Xavier, S. D., Miranda, M. C., and Pereira, L. D. (2017). Auditory behavior and auditory temporal resolution in children with sleep-disordered breathing. Sleep Med. 34, 90–95. doi: 10.1016/j.sleep.2017.03.009
Lendner, J. D., Helfrich, R. F., Mander, B. A., Romundstad, L., Lin, J. J., Walker, et al. (2020). An electrophysiological marker of arousal level in humans. eLife 9:e55092. doi: 10.7554/eLife.55092
Leszczynski, M., Barczak, A., Kajikawa, Y., Ulbert, I., Falchier, A., Tal, I., et al. (2020). Dissociation of broadband high-frequency activity and neuronal firing in the neocortex. Scient. Adv. 6:eabb0977. doi: 10.1101/531368
Lew, H. L., Dikmen, S., Slimp, J., Temkin, N., Lee, E. H., Newell, D., et al. (2003). Use of somatosensory-evoked potentials and cognitive event-related potentials in predicting outcomes of patients with severe traumatic brain injury. Am. J. Phys. Med. Rehabil. 82, 53–61. doi: 10.1097/00002060-200301000-00009
Liberalesso, P. B. N., D’Andrea, K. F. K., Cordeiro, M. L., Zeigelboim, B. S., Marques, J. M., and Jurkiewicz, A. L. (2012). Effects of sleep deprivation on central auditory processing. BMC Neuroscience 13:83. doi: 10.1186/1471-2202-13-83
Lieder, F., Daunizeau, J., Garrido, M. I., Friston, K. J., and Stephan, K. E. (2013). Modelling trial-by-trial changes in the mismatch negativity. PLoS Comput. Biol. 9:e1002911. doi: 10.1371/journal.pcbi.1002911
Lipponen, A., Kurkela, J. L. O., Kyläheiko, I., Hölttä, S., Ruusuvirta, T., Hämäläinen, J. A., et al. (2019). Auditory-evoked potentials to changes in sound duration in urethane-anaesthetized mice. Eur. J. Neurosci. 50, 1911–1919. doi: 10.1111/ejn.14359
Loewy, D. H., Campbell, K. B., and Bastien, C. (1996). The mismatch negativity to frequency deviant stimuli during natural sleep. Electroencephalogr. Clin. Neurophysiol. 98, 493–501. doi: 10.1016/0013-4694(96)95553-4
Loewy, D. H., Campbell, K. B., de Lugt, D. R., Elton, M., and Kok, A. (2000). The mismatch negativity during natural sleep: intensity deviants. Clin. Neurophysiol. 111, 863–872.
Luauté, J., Fischer, C., Adeleine, P., Morlet, D., Tell, L., and Boisson, D. (2005). Late auditory and event-related potentials can be useful to predict good functional outcome after coma. Archiv. Phys. Med. Rehabil. 86, 917–923. doi: 10.1016/j.apmr.2004.08.011
Ludwig, A. M. (1966). Altered states of consciousness. Archiv. Gener. Psych. 15, 225–234. doi: 10.1001/archpsyc.1966.01730150001001
Lutz, N. D., Wolf, I., Hübner, S., Born, J., and Rauss, K. (2018). Sleep strengthens predictive sequence coding. J. Neurosci. 38, 8989–9000. doi: 10.1523/jneurosci.1352-18.2018
Magnin, M., Rey, M., Bastuji, H., Guillemant, P., Mauguière, F., and Garcia-Larrea, L. (2010). Thalamic deactivation at sleep onset precedes that of the cerebral cortex in humans. Proc. Natl. Acad. Sci. 107, 3829–3833. doi: 10.1073/pnas.0909710107
Malmierca, M. S., Cristaudo, S., Pérez-González, D., and Covey, E. (2009). Stimulus-specific adaptation in the inferior colliculus of the anesthetized rat. J. Neurosci. 29, 5483–5493. doi: 10.1523/jneurosci.4153-08.2009
Maquet, P. (2000). Functional neuroimaging of normal human sleep by positron emission tomography. J. Sleep Res. 9, 207–232. doi: 10.1046/j.1365-2869.2000.00214.x
Maquet, P., Péters, J.-M., Aerts, J., Delfiore, G., Degueldre, C., Luxen, A., et al. (1996). Functional neuroanatomy of human rapid-eye-movement sleep and dreaming. Nature 383, 163–166. doi: 10.1038/383163a0
Martin, J.-R., and Pacherie, E. (2019). Alterations of agency in hypnosis: a new predictive coding model. Psychol. Rev. 126:133. doi: 10.1037/rev0000134
Massimini, M., Ferrarelli, F., Huber, R., Esser, S. K., Singh, H., and Tononi, G. (2005). Breakdown of cortical effective connectivity during sleep. Science 309, 2228–2232. doi: 10.1126/science.1117256
May, P., Tiitinen, H., Ilmoniemi, R. J., Nyman, G., Taylor, J. G., and Näätänen, R. (1999). Frequency change detection in human auditory cortex. J. Comput. Neurosci. 6, 99–120. doi: 10.1023/a:1008896417606
McCormick, D. A., and Bal, T. (1994). Sensory gating mechanisms of the thalamus. Curr. Opin. Neurobiol. 4, 550–556. doi: 10.1016/0959-4388(94)90056-6
Michel, C. M., and Murray, M. M. (2012). Towards the utilization of eeg as a brain imaging tool. NeuroImage 61, 371–385. doi: 10.1016/j.neuroimage.2011.12.039
Morlet, D., and Fischer, C. (2014). MMN and novelty P3 in coma and other altered states of consciousness: a review. Brain Topogr. 27, 467–479. doi: 10.1007/s10548-013-0335-5
Moshitch, D., Las, L., Ulanovsky, N., Bar-Yosef, O., and Nelken, I. (2006). Responses of neurons in primary auditory cortex (A1) to pure tones in the halothane-anesthetized cat. J. Neurophysiol. 95, 3756–3769. doi: 10.1152/jn.00822.2005
Mumford, D. (1992). On the computational architecture of the neocortex. Biol. Cybernet. 66, 241–251. doi: 10.1007/bf00198477
Murata, K., and Kameda, K. (1963). The activity of single cortical neurones of unrestrained cats during sleep and wakefulness. Archiv. Ital. Biol. 156, 127–136. doi: 10.12871/00039829201834
Näätänen, R. (1990). The role of attention in auditory information processing as revealed by event-related potentials and other brain measures of cognitive function. Behav. Brain Sci. 13, 201–233. doi: 10.1017/s0140525x00078407
Näätänen, R. (2003). Mismatch negativity: clinical research and possible applications. Int. J. Psychophysiol. 48, 179–188. doi: 10.1016/s0167-8760(03)00053-9
Näätänen, R., and Alho, K. (1995). Mismatch negativity – a unique measure of sensory processing in audition. Int. J. Neurosci. 80, 317–337. doi: 10.3109/00207459508986107
Näätänen, R., and Escera, C. (2000). Mismatch negativity: clinical and other applications. Audiol. Neurotol. 5, 105–110. doi: 10.1159/000013874
Näätänen, R., and Lyytinen, H. (1994). Mismatch negativity in sleep. Sleep Onset 20, 339–349. doi: 10.1037/10166-020
Näätänen, R., and Picton, T. (1987). The N1 wave of the human electric and magnetic response to sound: a review and an analysis of the component structure. Psychophysiology 24, 375–425. doi: 10.1111/j.1469-8986.1987.tb00311.x
Näätänen, R., Gaillard, A. W. K., and Mäntysalo, S. (1978). Early selective-attention effect on evoked potential reinterpreted. Acta Psychol. 42, 313–329. doi: 10.1016/0001-6918(78)90006-9
Näätänen, R., Paavilainen, P., Titinen, H., Jiang, D., and Alho, K. (1993). Attention and mismatch negativity. Psychophysiology 30, 436–450.
Naccache, L., Puybasset, L., Gaillard, R., Serve, E., and Willer, J.-C. (2005). Auditory mismatch negativity is a good predictor of awakening in comatose patients: a fast and reliable procedure. Clin. Neurophysiol. 116, 988–989. doi: 10.1016/j.clinph.2004.10.009
Nakamura, T., Michie, P. T., Fulham, W. R., Todd, J., Budd, T. W., Schall, U., et al. (2011). Epidural auditory event-related potentials in the rat to frequency and duration deviants: evidence of mismatch negativity? Front. Psychol. 2:367. doi: 10.3389/fpsyg.2011.00367
Nielsen-Bohlman, L., Knight, R. T., Woods, D. L., and Woodward, K. (1991). Differential auditory processing continues during sleep. Electroencephalogr. Clin. Neurophysiol. 79, 281–290. doi: 10.1016/0013-4694(91)90124-m
Nieto-Diego, J., and Malmierca, M. S. (2016). Topographic distribution of stimulus-specific adaptation across auditory cortical fields in the anesthetized rat. PLoS Biol. 14:e1002397. doi: 10.1371/journal.pbio.1002397
Nir, Y., Vyazovskiy, V. V., Cirelli, C., Banks, M. I., and Tononi, G. (2015). Auditory responses and stimulus-specific adaptation in rat auditory cortex are preserved across NREM and REM sleep. Cerebral Cortex 25, 1362–1378. doi: 10.1093/cercor/bht328
Nordby, H., Hugdahl, K., Stickgold, R., Bronnick, S. K., and Hobson, J. A. (1996). Event-related potentials (ERPS) to deviant auditory stimuli during sleep and waking. Neuroreport 7, 1082–6. doi: 10.1097/00001756-199604100-00026
Nourski, K. V., Steinschneider, M., Rhone, A. E., Kawasaki, H., Howard, M. A., and Banks, M. I. (2018). Auditory predictive coding across awareness states under anesthesia: an intracranial electrophysiology study. J. Neurosci. 38, 8441–8452. doi: 10.1523/jneurosci.0967-18.2018
Opitz, B., Rinne, T., Mecklinger, A., Von Cramon, D. Y., and Schröger, E. (2002). Differential contribution of frontal and temporal cortices to auditory change detection: fMRI and ERP results. Neuroimage 15, 167–174. doi: 10.1006/nimg.2001.0970
Ostwald, D., Spitzer, B., Guggenmos, M., Schmidt, T. T., Kiebel, S. J., and Blankenburg, F. (2012). Evidence for neural encoding of bayesian surprise in human somatosensation. NeuroImage 62, 177–188. doi: 10.1016/j.neuroimage.2012.04.050
Owen, A. M., and Coleman, M. R. (2007). Functional MRI in disorders of consciousness: advantages and limitations. Curr. Opin. Neurol. 20, 632–637. doi: 10.1097/wco.0b013e3282f15669
Pagnoni, G. (2019). The contemplative exercise through the lenses of predictive processing: a promising approach. Progr. Brain Res. 22, 299–322. doi: 10.1016/bs.pbr.2018.10.022
Pal, D., Silverstein, B. H., Lee, H., and Mashour, G. A. (2016). Neural correlates of wakefulness, sleep, and general anesthesia. Anesthesiology 125, 929–942.
Parras, G. G., Nieto-Diego, J., Carbajal, G. V., Valdés-Baizabal, C., Escera, C., and Malmierca, M. S. (2017). Neurons along the auditory pathway exhibit a hierarchical organization of prediction error. Nat. Commun. 8, 1–17. doi: 10.1038/s41467-017-02038-6
Patel, C. R., Redhead, C., Cervi, A. L., and Zhang, H. (2012). Neural sensitivity to novel sounds in the rat’s dorsal cortex of the inferior colliculus as revealed by evoked local field potentials. Hearing Res. 286, 41–54. doi: 10.1016/j.heares.2012.02.007
Pekala, R. J. (2015). Hypnosis as a ‘state of consciousness’: how quantifying the mind can help us better understand hypnosis. Am. J. Clin. Hypn. 57, 402–424. doi: 10.1080/00029157.2015.1011480
Peña, J. L., Pérez-Perera, L., Bouvier, M., and Velluti, R. A. (1999). Sleep and wakefulness modulation of the neuronal firing in the auditory cortex of the guinea pig. Brain Res. 816, 463–470. doi: 10.1016/s0006-8993(98)01194-9
Perez, P., Valente, M., Hermann, B., Sitt, J., Faugeras, F., Demeret, S., et al. (2021). Auditory event-related “global effect” predicts recovery of overt consciousness. Front. Neurol. 11:233. doi: 10.3389/fneur.2020.588233
Pérez-González, D., Malmierca, M. S., and Covey, E. (2005). Novelty detector neurons in the mammalian auditory midbrain. Eur. J. Neurosci. 22, 2879–2885. doi: 10.1111/j.1460-9568.2005.04472.x
Perrin, F., Schnakers, C., Schabus, M., Degueldre, C., Goldman, S., Brédart, S., et al. (2006). Brain response to one’s own name in vegetative state, minimally conscious state, and locked-in syndrome. Arch. Neurol. 63, 562–569. doi: 10.1001/archneur.63.4.562
Perry, J. E., Churchill, L. R., and Kirshner, H. S. (2005). The terri schiavo case: legal, ethical, and medical perspectives. Anna. Int. Med. 143:744. doi: 10.7326/0003-4819-143-10-200511150-00012
Pfeiffer, C., Nguissi, N. A. N., Chytiris, M., Bidlingmeyer, P., Haenggi, M., Kurmann, R., et al. (2018). Somatosensory and auditory deviance detection for outcome prediction during postanoxic coma. Anna. Clin. Transl. Neurol. 5, 1016–1024. doi: 10.1002/acn3.600
Picton, T. W. (1992). The P300 wave of the human event-related potential. J. Clin. Neurophysiol. 9, 456–479. doi: 10.1097/00004691-199210000-00002
Pilcher, J. J., and Huffcutt, A. I. (1996). Effects of sleep deprivation on performance: a meta-analysis. Sleep 19, 318–326. doi: 10.1093/sleep/19.4.318
Pincze, Z., Lakatos, P., Rajkai, C., Ulbert, I., and Karmos, G. (2001). Separation of mismatch negativity and the N1 wave in the auditory cortex of the cat: a topographic study. Clin. Neurophysiol. 112, 778–784. doi: 10.1016/s1388-2457(01)00509-0
Plourde, G., and Boylan, J. F. (1991). The long-latency auditory evoked potential as a measure of the level of consciousness during sufentanil anesthesia. J. Cardioth. Vasc. Anesth. 5, 577–583. doi: 10.1016/1053-0770(91)90010-q
Plourde, G., and Picton, T. W. (1991). Long-latency auditory evoked potentials during general anesthesia: N1 and P3 components. Anesthes. Anal. 72, 342–350. doi: 10.1213/00000539-199103000-00011
Plum, F., and Posner, J. B. (1982). The Diagnosis of Stupor and Coma, Vol. 19. Oxford, USA: Oxford University Press.
Polich, J. (2007). Updating P300: an integrative theory of P3a and P3b. Clin. Neurophysiol. 118, 2128–2148. doi: 10.1016/j.clinph.2007.04.019
Portas, C. M., Krakow, K., Allen, P., Josephs, O., Armony, J. L., and Frith, C. D. (2000). Auditory processing across the sleep-wake cycle: simultaneous EEG and fMRI monitoring in humans. Neuron 28, 991–999. doi: 10.1016/s0896-6273(00)00169-0
Purdon, P. L., Sampson, A., Pavone, K. J., and Brown, E. N. (2015). Clinical electroencephalography for anesthesiologists: Part I: background and basic signatures. Anesthesiology 123, 937–960. doi: 10.1097/aln.0000000000000841
Qin, L., Kitama, T., Chimoto, S., Sakayori, S., and Sato, Y. (2003). Time course of tonal frequency-response-area of primary auditory cortex neurons in alert cats. Neurosci. Res. 46, 145–152. doi: 10.1016/s0168-0102(03)00034-8
Rangarajan, V., Jacques, C., Knight, R. T., Weiner, K. S., and Grill-Spector, K. (2020). Diverse temporal dynamics of repetition suppression revealed by intracranial recordings in the human ventral temporal cortex. Cerebral Cortex 30, 5988–6003. doi: 10.1093/cercor/bhaa173
Rao, R. P. N., and Ballard, D. H. (1999). Predictive coding in the visual cortex: a functional interpretation of some extra-classical receptive-field effects. Nat. Neurosci. 2, 79–87. doi: 10.1038/4580
Rauss, K., and Pourtois, G. (2013). What is bottom-up and what is top-down in predictive coding? Front. Psychol. 4:276. doi: 10.3389/fpsyg.2013.00276
Raz, A., Deouell, L. Y., and Bentin, S. (2001). Is pre-attentive processing compromised by prolonged wakefulness? effects of total sleep deprivation on the mismatch negativity. Psychophysiology 38, 787–795. doi: 10.1111/1469-8986.3850787
Reinsel, R. A., Veselis, R. A., Wronski, M., and Marino, P. (1995). The P300 event-related potential during propofol sedation: a possible marker for amnesia? Br. J. Anaesth. 74, 674–680. doi: 10.1093/bja/74.6.674
Richardson, B. D., Hancock, K. E., and Caspary, D. M. (2013). Stimulus-specific adaptation in auditory thalamus of young and aged awake rats. J. Neurophysiol. 110, 1892–1902. doi: 10.1152/jn.00403.2013
Risetti, M., Formisano, R., Toppi, J., Quitadamo, L. R., Bianchi, L., Astolfi, L., et al. (2013). On ERPs detection in disorders of consciousness rehabilitation. Front. Hum. Neurosci. 7:775. doi: 10.3389/fnhum.2013.00775
Rosburg, T., Trautner, P., Dietl, T., Korzyukov, O. A., Boutros, N. N., Schaller, C., et al. (2005). Subdural recordings of the mismatch negativity (MMN) in patients with focal epilepsy. Brain 128, 819–828. doi: 10.1093/brain/awh442
Rudolph, U., and Antkowiak, B. (2004). Molecular and neuronal substrates for general anaesthetics. Nat. Rev. Neurosci. 5, 709–720. doi: 10.1038/nrn1496
Rui, Y.-Y., He, J., Zhai, Y.-Y., Sun, Z.-H., and Yu, X. (2018). Frequency-dependent stimulus-specific adaptation and regularity sensitivity in the rat auditory thalamus. Neuroscience 392, 13–24. doi: 10.1016/j.neuroscience.2018.09.015
Rutledge, R. B. M., Caplin, D. A., and Glimcher, P. W. (2010). Testing the reward prediction error hypothesis with an axiomatic model. J. Neurosci. 30, 13525–13536. doi: 10.1523/jneurosci.1747-10.2010
Ruusuvirta, T., Korhonen, T., Arikoski, J., and Kivirikko, J. (1996a). ERPs to pitch changes: a result of reduced responses to standard tones in rabbits. Neuroreport 7, 413–416. doi: 10.1097/00001756-199601310-00009
Ruusuvirta, T., Korhonen, T., Arikoski, J., and Kivirikko, J. (1996b). Multiple-unit responses to pitch changes in rabbits. Neuroreport 7, 1266–1268. doi: 10.1097/00001756-199605170-00009
Ruusuvirta, T., Korhonen, T., Penttonen, M., and Arikoski, J. (1995). Hippocampal evoked potentials to pitch deviances in an auditory oddball situation in the rabbit: no human mismatch-like dependence on standard stimuli. Neurosci. Lett. 185, 123–126. doi: 10.1016/0304-3940(94)11240-j
Ruusuvirta, T., Penttonen, M., and Korhonen, T. (1998). Auditory cortical event-related potentials to pitch deviances in rats. Neurosci. Lett. 248, 45–48. doi: 10.1016/s0304-3940(98)00330-9
Sabri, M., and Campbell, K. B. (2002). The effects of digital filtering on mismatch negativity in wakefulness and slow-wave sleep. J. Sleep Res. 11, 123–127. doi: 10.1046/j.1365-2869.2002.00292.x
Sabri, M., and Campbell, K. B. (2005). Is the failure to detect stimulus deviance during sleep due to a rapid fading of sensory memory or a degradation of stimulus encoding? J. Sleep Res. 14, 113–122. doi: 10.1111/j.1365-2869.2005.00446.x
Sabri, M., Labelle, S., Gosselin, A., and Campbell, K. B. (2003). Effects of sleep onset on the mismatch negativity (MMN) to frequency deviants using a rapid rate of presentation. Cogn. Brain Res. 17, 164–176. doi: 10.1016/s0926-6410(03)00090-9
Saidman, L. J., Bovill, J. G., Sebel, P. S., and Stanley, T. H. (1984). Opioid analgesics in anesthesia: with special reference to their use in cardiovascular anesthesia. J. Am. Soc. Anesthesiol. 61, 731–755. doi: 10.1097/00000542-198412000-00018
Sallinen, M., and Lyytinen, H. (1997). Mismatch negativity during objective and subjective sleepiness. Psychophysiology 34, 694–702. doi: 10.1111/j.1469-8986.1997.tb02144.x
Sallinen, M., Kaartinen, J., and Lyytinen, H. (1994). Is the appearance of mismatch negativity during stage 2 sleep related to the elicitation of K-complex? Electroencephalogr. Clin. Neurophysiol. 91, 140–148. doi: 10.1016/0013-4694(94)90035-3
SanMiguel, I., Widmann, A., Bendixen, A., Trujillo-Barreto, N., and Schroger, E. (2013). Hearing silences: human auditory processing relies on preactivation of sound-specific brain activity patterns. J. Neurosci. 33, 8633–8639. doi: 10.1523/jneurosci.5821-12.2013
Scheinin, A., Kantonen, O., Alkire, M., Långsjö, J., Kallionpää, R. E., Kaisti, K., et al. (2021). Foundations of human consciousness: imaging the twilight zone. J. Neurosci. 41, 1769–1778. doi: 10.1523/jneurosci.0775-20.2020
Schlossmacher, I., Lucka, F., Bruchmann, M., and Straube, T. (2021). Effects of awareness and task relevance on neurocomputational models of mismatch negativity generation. bioRxiv [preprint] doi: 10.1101/2021.02.03.429421
Schröger, E., Marzecová, A., and SanMiguel, I. (2015). Attention and prediction in human audition: a lesson from cognitive psychophysiology. Eur. J. Neurosci. 41, 641–664. doi: 10.1111/ejn.12816
Sculthorpe, L. D., Ouellet, D. R., and Campbell, K. B. (2009). MMN elicitation during natural sleep to violations of an auditory pattern. Brain Res. 1290, 52–62. doi: 10.1016/j.brainres.2009.06.013
Sebel, P. S., Bovill, J. G., Wauquier, A., and Rog, P. (1981). Effects of high-dose fentanyl anesthesia on the electroencephalogram. J. Am. Soc. Anesthesiol. 55, 203–211. doi: 10.1097/00000542-198109000-00004
Sharma, R. (2011). Oculomotor dysfunction in amyotrophic lateral sclerosis. Archiv. Neurol. 68:857. doi: 10.1001/archneurol.2011.130
Shiramatsu, T. I., Kanzaki, R., and Takahashi, H. (2013). Cortical mapping of mismatch negativity with deviance detection property in rat. PLoS One 8:e82663. doi: 10.1371/journal.pone.0082663
Shirazibeheshti, A., Cooke, J., Chennu, S., Adapa, R., Menon, D. K., Hojjatoleslami, S. A., et al. (2018). Placing meta-stable states of consciousness within the predictive coding hierarchy: the deceleration of the accelerated prediction error. Conscious. Cogn. 63, 123–142. doi: 10.1016/j.concog.2018.06.010
Sikkens, T., Bosman, C. A., and Olcese, U. (2019). The role of top-down modulation in shaping sensory processing across brain states: implications for consciousness. Front. Syst. Neurosci. 13:31. doi: 10.3389/fnsys.2019.00031
Simpson, T. P., Manara, A. R., Kane, N. M., Barton, R. L., Rowlands, C. A., and Butler, S. R. (2002). Effect of propofol anaesthesia on the event-related potential mismatch negativity and the auditory-evoked potential N1. Br. J. Anaesth. 89, 382–388. doi: 10.1093/bja/89.3.382
Smout, C. A., Tang, M. F., Garrido, M. I., and Mattingley, J. B. (2019). Attention promotes the neural encoding of prediction errors. PLoS Biol. 17:e2006812. doi: 10.1371/journal.pbio.2006812
Sneyd, J. R., Samra, S. K., Davidson, B., Kishimoto, T., Kadoya, C., and Domino, E. F. (1994). Electrophysiologic effects of propofol sedation. Anesth. Anal. 79, 1151–1158. doi: 10.1213/00000539-199412000-00022
Spratling, M. W. (2008a). Predictive coding as a model of biased competition in visual attention. Vision Res. 48, 1391–1408. doi: 10.1016/j.visres.2008.03.009
Spratling, M. W. (2008b). Reconciling predictive coding and biased competition models of cortical function. Front. Comput. Neurosci. 2:4. doi: 10.3389/neuro.10.004.2008
Squires, N. K., Squires, K. C., and Hillyard, S. A. (1975). Two varieties of long-latency positive waves evoked by unpredictable auditory stimuli in man. Electroencephalogr. Clin. Neurophysiol. 38, 387–401. doi: 10.1016/0013-4694(75)90263-1
Stamatakis, E. A., Adapa, R. M., Absalom, A. R., and Menon, D. K. (2010). Changes in resting neural connectivity during propofol sedation. PLoS One 5:e14224. doi: 10.1371/journal.pone.0014224
Stefanics, G., Heinzle, J., Horváth, A. A., and Stephan, K. E. (2018). Visual mismatch and predictive coding: a computational single-trial ERP study. J. Neurosci. 38, 4020–4030. doi: 10.1523/jneurosci.3365-17.2018
Steriade, M. (1991). Alertness, quiet sleep, dreaming. Norm. Altered Stat. Funct. 8, 279–357. doi: 10.1007/978-1-4615-6622-9_8
Steriade, M. (2006). Grouping of brain rhythms in corticothalamic systems. Neuroscience 137, 1087–1106. doi: 10.1016/j.neuroscience.2005.10.029
Steriade, M., Timofeev, I., and Grenier, F. (2001). Natural waking and sleep states: a view from inside neocortical neurons. J. Neurophysiol. 85, 1969–1985. doi: 10.1152/jn.2001.85.5.1969
Strauss, M., Sitt, J. D., King, J.-R., Elbaz, M., Azizi, L., Buiatti, M., et al. (2015). Disruption of hierarchical predictive coding during sleep. Proc. Natl. Acad. Sci. U.S.A. 112, E1353–E1362. doi: 10.1073/pnas.1501026112
Summerfield, C., and Egner, T. (2009). Expectation (and attention) in visual cognition. Trends Cogn. Sci. 13, 403–409. doi: 10.1016/j.tics.2009.06.003
Sussman, E. S. (2007). A new view on the MMN and attention debate. J. Psychophysiol. 21:164. doi: 10.1027/0269-8803.21.34.164
Sutton, S., Braren, M., Zubin, J., and John, E. R. (1965). Evoked-potential correlates of stimulus uncertainty. Science 150, 1187–1188. doi: 10.1126/science.150.3700.1187
Szymanski, M. D., Yund, E. W., and Woods, D. L. (1999). Phonemes, intensity and attention: differential effects on the mismatch negativity (MMN). J. Acoust. Soc. Am. 106, 3492–3505. doi: 10.1121/1.428202
Taaseh, N., Yaron, A., and Nelken, I. (2011). Stimulus-specific adaptation and deviance detection in the rat auditory cortex. PLoS One 6:e23369. doi: 10.1371/journal.pone.0023369
Tagliazucchi, E., von Wegner, F., Morzelewski, A., Brodbeck, V., Jahnke, K., and Laufs, H. (2013). Breakdown of long-range temporal dependence in default mode and attention networks during deep sleep. Proc. Natl. Acad. Sci. U.S.A. 110, 15419–15424. doi: 10.1073/pnas.1312848110
Takahashi, H., Tokushige, H., Shiramatsu, T. I, Noda, T., and Kanzaki, R. (2015). Covariation of pupillary and auditory cortical activity in rats under isoflurane anesthesia. Neuroscience 300, 29–38. doi: 10.1016/j.neuroscience.2015.05.004
Tang, Y.-Y., Hölzel, B. K., and Posner, M. I. (2015). The neuroscience of mindfulness meditation. Nat. Rev. Neurosci. 16, 213–225. doi: 10.1038/nrn3916
Thomas, L. B., and Jenkner, F. L. (1952). The effects of asphyxia and certain drugs on the activity of single cortical cells. Transact. Am. Neurol. Assoc. 56, 47–51. doi: 10.1002/9780470751466.ch23
Trejo, L. J., David, L. R. J., and Kramer, A. (1995). Attentional modulation of the mismatch negativity elicited by frequency differences between binaurally presented tone bursts. Psychophysiology 32, 319–328. doi: 10.1111/j.1469-8986.1995.tb01214.x
Tzovara, A., Korn, C. W., and Bach, D. R. (2018). Human pavlovian fear conditioning conforms to probabilistic learning. PLoS Comput. Biol. 14:e1006243. doi: 10.1371/journal.pcbi.1006243
Tzovara, A., Rossetti, A. O., Juan, E., Suys, T., Viceic, D., Rusca, M., et al. (2016). Prediction of awakening from hypothermic postanoxic coma based on auditory discrimination. Anna. f Neurol. 79, 748–757. doi: 10.1002/ana.24622
Tzovara, A., Rossetti, A. O., Spierer, L., Grivel, J., Murray, M. M., Oddo, M., et al. (2013). Progression of auditory discrimination based on neural decoding predicts awakening from coma. Brain 136, 81–89. doi: 10.1093/brain/aws264
Tzovara, A., Simonin, A., Oddo, M., Rossetti, A. O., and De Lucia, M. (2015). Neural detection of complex sound sequences in the absence of consciousness. Brain 138, 1160–1166. doi: 10.1093/brain/awv041
Uhrig, L., Dehaene, S., and Jarraya, B. (2014). A hierarchy of responses to auditory regularities in the macaque brain. J. Neurosci. 34, 1127–1132. doi: 10.1523/jneurosci.3165-13.2014
Uhrig, L., Janssen, D., Dehaene, S., and Jarraya, B. (2016). Cerebral responses to local and global auditory novelty under general anesthesia. Neuroimage 141, 326–340. doi: 10.1016/j.neuroimage.2016.08.004
Ulanovsky, N., Las, L., Farkas, D., and Nelken, I. (2004). Multiple time scales of adaptation in auditory cortex neurons. J. f Neurosci. 24, 10440–10453. doi: 10.1523/jneurosci.1905-04.2004
Vaitl, D., Birbaumer, N., Gruzelier, J., Jamieson, G. A., Kotchoubey, B., Kübler, et al. (2005). Psychobiology of altered states of consciousness. Psychol. Bull. 131:98. doi: 10.1037/0033-2909.131.1.98
Van Sweden, B., Van Dijk, J. G., and Caekebeke, J. F. V. (1994). Auditory information processing in sleep: late cortical potentials in an oddball paradigm. Neuropsychobiology 29, 152–156. doi: 10.1159/000119078
Verleger, R. (1988). Event-related potentials and cognition: a critique of the context updating hypothesis and an alternative interpretation of P3. Behav. Brain Sci. 11:343. doi: 10.1017/s0140525x00058015
Von Helmholtz, H. (1867). Handbuch Der Physiologischen Optik: Mit 213 in Den Text Eingedruckten Holzschnitten Und 11 Tafeln, Vol. 9. Leipzig: Leopold Voss.
Wauquier, A., Bovill, J. G., and Sebel, P. S. (1984). Electroencephalographic effects of fentanyl-, sufentanil-and alfentanil anaesthesia in man. Neuropsychobiology 11, 203–206. doi: 10.1159/000118078
Weber, L. A., Diaconescu, A. O., Mathys, C., Schmidt, A., Kometer, M., Vollenweider, F., et al. (2020). Ketamine affects prediction errors about statistical regularities: a computational single-trial analysis of the mismatch negativity. J. Neurosci. 40, 5658–5668. doi: 10.1523/jneurosci.3069-19.2020
Wesensten, N. J., and Badia, P. (1988). The P300 component in sleep. Physiol. Behav. 44, 215–220. doi: 10.1016/0031-9384(88)90141-2
Wijnen, V. J. M., Van Boxtel, G. J. M., Eilander, H. J., and De Gelder, B. (2007). Mismatch negativity predicts recovery from the vegetative state. Clin. Neurophysiol. 118, 597–605. doi: 10.1016/j.clinph.2006.11.020
Winkler, I. (2007). Interpreting the mismatch negativity. J. Psychophysiol. 21, 147–163. doi: 10.1027/0269-8803.21.34.147
Winter, O., Kok, A., Kenernans, J. L., and Elton, M. (1995). Auditory event-related potentials to deviant stimuli during drowsiness and stage 2 sleep. Electroencephalogr. Clin. Neurophysiol. 96, 398–412. doi: 10.1016/0168-5597(95)00030-v
Witon, A., Shirazibehehsti, A., Cooke, J., Aviles, A., Adapa, R., Menon, D. K., et al. (2020). Sedation modulates frontotemporal predictive coding circuits and the double surprise acceleration effect. Cerebral Cortex 30, 5204–5217. doi: 10.1093/cercor/bhaa071
Woldorff, M. G., and Hillyard, S. A. (1991). Modulation of early auditory processing during selective listening to rapidly presented tones. Electroencephalogr. Clin. Neurophysiol. 79, 170–191. doi: 10.1016/0013-4694(91)90136-r
Xu, X., Yu, X., He, J., and Nelken, I. (2014). Across-ear stimulus-specific adaptation in the auditory cortex. Front. Neural Circuits 8:89. doi: 10.3389/fncir.2014.00089
Young, E. D., and Brownell, W. E. (1976). Responses to tones and noise of single cells in dorsal cochlear nucleus of unanesthetized cats. J. Neurophysiol. 39, 282–300. doi: 10.1152/jn.1976.39.2.282
Yppärilä, H., Karhu, J., Westerén-Punnonen, S., Musialowicz, T., and Partanen, J. (2002). Evidence of auditory processing during postoperative propofol sedation. Clin. Neurophysiol. 113, 1357–1364. doi: 10.1016/s1388-2457(02)00158-x
Zaehle, T., Bauch, E. M., Hinrichs, H., Schmitt, F. C., Voges, J., Heinze, H.-J., et al. (2013). Nucleus accumbens activity dissociates different forms of salience: evidence from human intracranial recordings. J. Neurosci. 33, 8764–8771. doi: 10.1523/jneurosci.5276-12.2013
Zhang, Y., Yan, F., Wang, L., Wang, Y., Wang, C., Wang, Q., et al. (2018). Cortical areas associated with mismatch negativity: a connectivity study using propofol anesthesia. Front. Hum. Neurosci. 2:392. doi: 10.3389/fnhum.2018.00392
Zurita, P., Villa, A. E. P., De Ribaupierre, Y., De Ribaupierre, F., and Rouiller, E. M. (1994). Changes of single unit activity in the cat’s auditory thalamus and cortex associated to different anesthetic conditions. Neurosci. Res. 19, 303–316. doi: 10.1016/0168-0102(94)90043-4
Keywords: prediction error, mismatch negativity, coma, sleep, anesthesia, P300
Citation: Tivadar RI, Knight RT and Tzovara A (2021) Automatic Sensory Predictions: A Review of Predictive Mechanisms in the Brain and Their Link to Conscious Processing. Front. Hum. Neurosci. 15:702520. doi: 10.3389/fnhum.2021.702520
Received: 29 April 2021; Accepted: 12 July 2021;
Published: 18 August 2021.
Edited by:
Ryszard Auksztulewicz, City University of Hong Kong, SAR ChinaReviewed by:
Umberto Olcese, University of Amsterdam, NetherlandsMartina G. Vilas, Max Planck Institute for Empirical Aesthetics, Max Planck Society (MPG), Germany
Copyright © 2021 Tivadar, Knight and Tzovara. This is an open-access article distributed under the terms of the Creative Commons Attribution License (CC BY). The use, distribution or reproduction in other forums is permitted, provided the original author(s) and the copyright owner(s) are credited and that the original publication in this journal is cited, in accordance with accepted academic practice. No use, distribution or reproduction is permitted which does not comply with these terms.
*Correspondence: Athina Tzovara, Athina.tz@gmail.com