- 1Department of Clinical Psychology, School of Medical Psychology, Fourth Military Medical University, Xi'an, China
- 2Department of Radiology, Xijing Hospital, Fourth Military Medical University, Xi'an, China
- 3Department of Radiology, The Second Medical Center, Chinese PLA General Hospital, Beijing, China
- 4Department of Psychiatry, Xijing Hospital, Fourth Military Medical University, Xi'an, China
- 5Department of Anatomy and K. K. Leung Brain Research Centre, Fourth Military Medical University, Xi'an, China
Schizophrenia is a complex mental illness with genetic heterogeneity, which is often accompanied by alterations in brain structure and function. The neurobiological mechanism of schizophrenia associated with heredity remains unknown. Recently, the development of trans-scale and multi-omics methods that integrate gene and imaging information sheds new light on the nature of schizophrenia. In this article, we summarized the results of brain structural and functional changes related to the specific single-nucleotide polymorphisms (SNPs) in the past decade, and the SNPs were divided into non-coding regions and coding regions, respectively. It is hoped that the relationship between SNPs and cerebral alterations can be displayed more clearly and intuitively, so as to provide fresh approaches for the discovery of potential biomarkers and the development of clinical accurate individualized treatment decision-making.
Introduction
Schizophrenia is a common and complex multidimensional disease with high heredity, and genetic factors play an important role in its pathophysiological mechanism (McCutcheon et al., 2020). Understanding the genetic basis of schizophrenia is of great help to explore its pathogenesis.
In recent years, the application of trans-scale and multi-omics methods that combine gene and imaging in schizophrenia has promoted the elucidation of gene-related pathogenesis and the exploration of potential biomarkers (Reddaway et al., 2018). Trans-scale analysis is a research strategy for the joint analysis of information between different scales (van den Heuvel et al., 2019). From the macroscopic level, the human brain can be viewed as a complex network system made up of structural and functional connections between brain regions. And, from the microscopic perspective, the neurons containing dendrites and axons form a complex system of wiring which makes up the structural basis of our brain. Microscale level information, such as genetic, molecular, and cellular, can provide sufficient evidence for the construction of brain phenotypes and mechanisms of brain injury at the macroscale level (van den Heuvel et al., 2019). Multi-omics analysis is a research strategy of integrative analysis that integrates information between different disciplines. Many disciplines, including genomics, epigenomics, transcriptomics, proteomics, metabolomics, gut microbiomics, and connectomics, have made significant contributions to the study of the pathogenesis of schizophrenia (Guan et al., 2021). The multi-omics analysis strategy can provide a more comprehensive perspective on the exploration of schizophrenia pathogenesis.
With the evolvement of functional magnetic resonance imaging (fMRI), structural magnetic resonance imaging (sMRI), diffusion-weighted imaging (DWI), and other sequences, alterations of brain structure and function can be displayed more accurately on MRI. Brain microstructures, such as white matter myelination, can be measured indirectly in a non-invasive manner using magnetization transfer imaging (MTI) technique (Whitaker et al., 2016). MRI plays an increasingly important role in the study of brain phenotypes and differential diagnosis of mental disorders. Previous studies have confirmed that there are some alterations in brain structure and function in patients with schizophrenia (Brown and Thompson, 2010), which may be related to clinical symptoms of schizophrenia (Cui et al., 2017; Liu et al., 2019). Another study has found out that quantitative and specific functional connectivity (FC) biomarkers could be an effective radiomics features for individualized diagnosis for schizophrenia (Cui et al., 2018). The appearance of brain phenotypes on MRI may provide clues to the differential diagnosis of schizophrenia and bipolar disorder, which overlap in risk genes and clinical symptoms. While brain disturbances in patients with bipolar disorder are primarily located in the fronto-limbic subsystems, schizophrenia is characterized by disorders of the small world and rich club and the effects still present in the unaffected offspring (Perry et al., 2019). Therefore, MRI-based imaging study has gradually become the most common method to study mental disorders at macroscale, providing connectomics and radiomics approaches.
Sinlge-nucletode polymorphisms (SNPs) are the most common genetic variation at the genome level, which are caused by the change of a single base pair in the DNA sequence. SNPs are found randomly in the coding region or the non-coding region of the gene and produce corresponding effects by affecting gene expression, mRNA processing, and protein translation (Bush and Moore, 2012; Gurung and Prata, 2015; Roy et al., 2020). According to the research, SNPs account for a large part of the genetic variation associated with schizophrenia (Pardinas et al., 2018). Although recent genome-wide association studies (GWASs) have identified many SNPs loci associated with schizophrenia (Schizophrenia Working Group of the Psychiatric Genomics C., 2014), providing many possible genetic variation resources with biological functions for analyzing the pathogenesis of schizophrenia, the functions behind these mutations still need to be verified. Changes in genes at the molecular level may be the basis that SNPs affect the functional and structural connectivity of the brain, as well as the volume and density of gray matter and white matter.
The effects produced by SNPs represent information at the cellular, molecular, and other microscopic levels, whereas MRI can provide a macroscopic view of brain phenotypes. The approach of combining micro-level and macro-level information for research exemplifies the trans-scale research strategy. Methods that combine genetic information with imaging information fall under the umbrella of multi-omics technologies. On the one hand, the trans-scale and multi-omics analysis strategy that combines genomics, connectomics, and radiomics is instrumental to visualize the link between functional genetic variants associated with schizophrenia and the imaging phenotype. On the other hand, the trans-scale and multi-omics analysis strategy linking gene variation with brain structure and function have further contributed to the advanced studies of molecular biological mechanisms behind brain phenotypes and clinical manifestations of schizophrenia. The goal of trans-scale neuroscience of psychiatric illnesses is to deepen insights of the relationship between alterations at different scales (van den Heuvel et al., 2019). Therefore, an updated overview of trans-scale properties of schizophrenia based on multi-omics research strategies is needed.
The effect of risk genes on structural connectivity and FC during executive tasks was found in a systematic review summarized by Gurung and Prata in 2015 (Gurung and Prata, 2015). In this review, we have carefully categorized the types of brain phenotype alterations and the location of SNPs separately. For alterations in brain phenotype, we address both structural and functional aspects. Based on the location of the SNPs, we have divided them into non-coding regions and coding regions to describe, respectively. The purpose of this article is to summarize the studies on the relationship between specific SNPs loci in schizophrenia-related genes and cerebral alterations based on trans-scale and multi-omics strategies in the past decade, as well as demonstrate the advantages of trans-scale and multi-omics research strategy through the relationship between SNPs and alterations in brain phenotypes. The candidate genes are listed as follows, and the specific SNPs loci and their possible effects on trans-scale properties of schizophrenia will be discussed in detail (zinc finger protein 804A [ZNF804A], calcium voltage-gated channel subunit alpha 1C [CACNA1C], neurogranin [NRGN], cholinergic receptor, muscarinic 3 [CHRM3], oligodendrocyte lineage transcription factor 2 [OLTG2], D-amino acid oxidase activator [DAOA], D-amino acid oxidase [DAAO], Disrupted in Schizophrenia Gene 1 [DISC1], nitric oxide synthase 1 [NOS1], KIAA0319, N-Methyl D-Aspartate 1 [GRIN1], Glutamate receptor 2 [GRIA2], microRNA 137 [MIR137], metabotropic glutamate receptor 3 [GRM3], contactin-associated protein-like 2 [CNTNAP2], Neuregulin1 [NRG1], glutamate receptor delta 1 [GRID1], and cyclin M2 gene [CNNM2]) (Table 1).
MRI and SNPs in Schizophrenia
Brain Function and SNPs in Schizophrenia
Single-Nucleotide Polymorphisms of Non-Coding Region
In first-episode treatment-naive schizophrenia, FC network analysis has suggested significant effects of CHRM3 rs6800381 on the abnormal thalamo-orbital frontal cortex connectivity (Wang et al., 2016). Compared with C allele carriers, NRGN gene rs12807809 TT homozygotes in patients with schizophrenia have significantly lower hippocampus-seeded FC values in bilateral middle cingulate gyri and left anterior cingulate gyrus, suggesting that rs12807809 may be involved in the pathophysiological process of abnormal Papez circuit function (Zhang et al., 2019). The effects of GRIN1 rs11146020 are mainly reflected on the causality connections between the bilateral dorsolateral prefrontal cortex (DLPFC) (Cai et al., 2020b).
However, some SNP genotypes are not associated with changes of FC in patients with schizophrenia, demonstrating the uncertainty of genetic factors at the molecular and cellular levels. For example, left Broca superior/inferior parietal network and bilateral Wernicke-frontoparietal network are related to KIAA0319 SNPs (rs2038136, rs2038137) only in controls, respectively, but not in schizophrenia (Jamadar et al., 2013).
Single-Nucleotide Polymorphisms of Coding Region
Chen et al. identified that rs1344706 within intron 2 of the ZNF804A gene played a role in degree centrality in the precuneus, an important hub of the whole-brain network, in patients with schizophrenia (Chen et al., 2018). The investigation on the relationship between rs1059004 polymorphism which locates in the 3′-untranslated region (3′UTR) intronic region of the OLIG2 gene and the whole-brain FC in patients with first-episode schizophrenia reveals that the FC strength decreased both in patients with schizophrenia and healthy controls with risk A allele and there is at some level a positive relationship between FC strength and verbal fluency score in patients, suggesting that there are synergistic effects between rs1059004 polymorphism and brain connections (Cai et al., 2020a). Compared with C allele homozygote, patients with schizophrenia with risk A allele have significantly lower nodal efficiency in the right precuneus and left middle temporal pole (Lv et al., 2020). Using brain connectivity network properties, “AG” carriers of DAOA rs2391191 have higher connectivity density and larger global efficiency than “AA” carriers (Liu et al., 2014).
For the CACNA1C rs1006737, the risk allele carriers (AA/AG) show decreased connectivity between the left precentral gyrus/inferior frontal gyrus and superior temporal gyrus vs. non-risk allele homozygotes (GG) in schizophrenia, thus presenting abnormal verbal fluency (Tecelao et al., 2019). ZNF804A rs1344706 seems to play an important role in FC between the left hippocampus and right DLPFC, which may serve as the brain mechanism of rs1344706 in schizophrenia (Zhang et al., 2018). However, during a working memory task, seeded connectivity analysis of the homozygous control group of the risk allele (AA) demonstrate a disruption in right DLPFC–left hippocampal formation coupling when compared with the other genotype groups, but there is no effect of genotype in patients with schizophrenia (Rasetti et al., 2011). FC between the right inferior frontal gyrus and bilateral DLPFC is reduced in the risk allele carriers (the TT/TC group) of NOS1 gene rs3782206 in both Stroop task and resting state, suggesting a relevance of rs3782206 to cognitive functions and neural mechanisms at the inferior frontal gyrus (Zhang et al., 2015). Significant association is also detected between the right precuneus inferior frontal gyrus functional connection and the DISC1 rs821617 in patients with schizophrenia (Gong et al., 2014). For DAAO rs3918346 genotype, there are verbal fluency task-dependent changes of FC between the left precuneus and distributed networks including left and right precuneus, left putamen, right posterior cingulate gyrus, left caudate and right angular gyrus, and between the right posterior cingulate and right precuneus and left insula among patients with schizophrenia (Papagni et al., 2011). Similar to the effect of KIAA0319 SNPs (rs2038136,rs2038137) genotype, the influence of SNP rs4504469 located at the exon of KIAA0319 coding region on the left Broca upper/lower parietal network and bilateral Wernicke-frontalparietal network is reflected only in controls (Jamadar et al., 2013). In addition to investigating the influences of SNP rs11146020, which is located in the non-coding region of GRIN1, Cai et al. (2020b) found that SNP rs3813296 located in the intron region of GRIA2 also has certain effects on the causality connections which located on the descending pathway from DLPFC to the striatum and thalamus in patients with schizophrenia. In the meantime, the interaction effects of rs11146020 and rs3813296 on causality connectivity are mainly located in the upstream pathway from the bilateral pallidum to the right caudate and the bilateral DLPFC, and negatively correlated with the Mayer–Salovey–Caruso emotional intelligence test, managing emotions score.
Brain Structure and SNPs in Schizophrenia
Single-Nucleotide Polymorphisms of Non-Coding Region
As for structural connectivity and brain structure, MRI studies are helpful to explore biological clues about the genetic underpinnings of structural connectome deficits in schizophrenia (Voineskos, 2015). For the NRG1 rs35753505 genotype, fractional anisotropy in the anterior cingulum of patients with schizophrenia with the T allele is significantly lower than that of patients with schizophrenia with CC genotype and healthy controls with T allele (Wang et al., 2009). On the basis of previous studies, Nenadic et al. (2012) found that the SNP rs3814614 located in the GRID1 promoter region affected the gray matter density in the right medial cerebellum and a region of the medial parietal cortex. Moreover, the cerebellar cluster gray matter density of TT homozygous patients was the highest, CT heterozygote was the intermediate, and CC homozygote was the lowest, showing significant interaction effects of group × genotype (Nenadic et al., 2012). These findings contribute to our understanding of the mechanisms of the abnormal cortical–subcortical brain networks in schizophrenia with the involvement of the NRGN. Apart from the effect on FC, the NRGN rs12807809 genotype is also associated with the morphological and structural changes of the cerebral cortex. The frontal, parietal, and temporal cortices of patients with schizophrenia with TT genotype were extensively thinned, and there are also thalamic shape abnormalities in the regions involving pulvinar and medial dorsal nuclei (Thong et al., 2013). Furthermore, patients with schizophrenia carrying risk T allele have a smaller gray matter volume in the left anterior cingulate cortex, compared to non-risk C allele carriers (Ohi et al., 2012).
Single-Nucleotide Polymorphisms of Coding Region
The minor allele of rs7808623, located in the intronic region of GRM3 gene, is associated with higher white matter integrity in the anterior thalamic radiation and the corticospinal tract, as well as a series of tracts connecting the frontal cortex to the cerebellum (Mounce et al., 2014). This study indirectly mirrors the importance of GRM3 in maintaining white matter integrity. For MIR137 rs1625579 genotype, patients with schizophrenia with risk T allele homozygous genotype decreased fractional anisotropy values in both right orbitofrontal region and left striatum compared to G allele/A allele carriers (Kuswanto et al., 2015). There are some correlations between CNTNAP2, also known as Neurexin 4 (NRXN4), rs2710126 genotype and fractional anisotropy in the uncinate fasciculus (Clemm von Hohenberg et al., 2013). Despite the lack of association between ZNF804A rs1344706 and white matter integrity in schizophrenia (Wei et al., 2013), T allele carriers present higher white matter density in the left prefrontal lobe and bilateral hippocampi (Wei et al., 2012). Compared with non-risk A allele carriers, patients with schizophrenia with G/G genotype of risk variant rs7914558 which is located in intron1 of the CNNM2 have smaller gray matter volumes in the bilateral inferior frontal gyri, especially the orbital region (Ohi et al., 2013). Among the effects of GRIA2 gene rs3813296 on white matter (Cai et al., 2020b), the most significant effect is located on the bilateral superior corona radiata fibers. Compared with the TT genotype, patients with GT genotype have a significantly larger volume of the superior corona radiata, which leads to the dispersion of the connection strength between the left DLPFC and the right caudate (Cai et al., 2020b). This is seemingly the factor that patients with GT genotype have a decrease in connection strength between the two areas. All the above research results provide a possible mechanism underlying the association between cerebral abnormalities and schizophrenia at the level of genetic polymorphisms.
Discussion
Based on the genetic variation data provided by GWAS research results, the current research takes the common SNPs in the whole genome as the objects to carry out association analysis at the overall level, and looks for the SNPs and susceptibility genes related to schizophrenia. The discovery of SNPs function increases the understanding of the association between functional genetic variation and imaging phenotypes related to schizophrenia from the gene level, and may also provide important clues about the anatomical heterogeneity of schizophrenia. Microscopic level alterations in gene function provide theoretical support for macroscopic level alterations in brain phenotype (van den Heuvel et al., 2019). The application of multi-omics method combining gene and MRI in schizophrenia intuitively reveals the possible pathogenesis related to genes from the perspective of brain structure and function changes (Figure 1). In particular, using genetic imaging strategies, especially based on the high-quality images presented by MRI techniques, to investigate the influence of genetic factors on brain phenotypes will help to study schizophrenia in a more integrated perspective. The key of the trans-scale and multi-omics research strategy is to synthesize the information of different scales and different disciplines, so as to provide the most comprehensive way to explain the nature of schizophrenia (Guan et al., 2021). A recent review systematically summarizes the application of multi-omics approaches in schizophrenia in terms of pathogenesis, disease typing, clinical grading, risk prediction, and precision interventions (Guan et al., 2021). Compared to the information provided by a single discipline, the combination of genetic data and imaging data can provide us with more comprehensive information. In other words, the trans-scale information provided by multi-omics methods can deepen the understanding of the pathophysiological mechanism of clinical symptoms of schizophrenia, and can provide new clew for the stratification of patients and high-risk groups and the development of more accurate risk and treatment response biomarkers. The analytical framework that combines clinical data from multi-view biclustering analysis with gene expression levels allows for the accurate identification of subtypes of schizophrenia (Yin et al., 2019). Protein interactome can be used to describe polygenic associations between antipsychotic drug targets and risk genes, and help to develop new targets for the treatment of negative symptoms and cognitive impairment in schizophrenia (Kauppi et al., 2018). Clinical transformation is one of the ultimate goals of all research. The heterogeneity of schizophrenia is indirectly reflected by the multiple imaging features exhibited by patients with schizophrenia. Different genotypes of patients also have different brain phenotypes, which show individualized characteristics in neuroimaging. This heterogeneity between different scales indirectly suggests that clinicians need to design more individualized and precise clinical decisions.
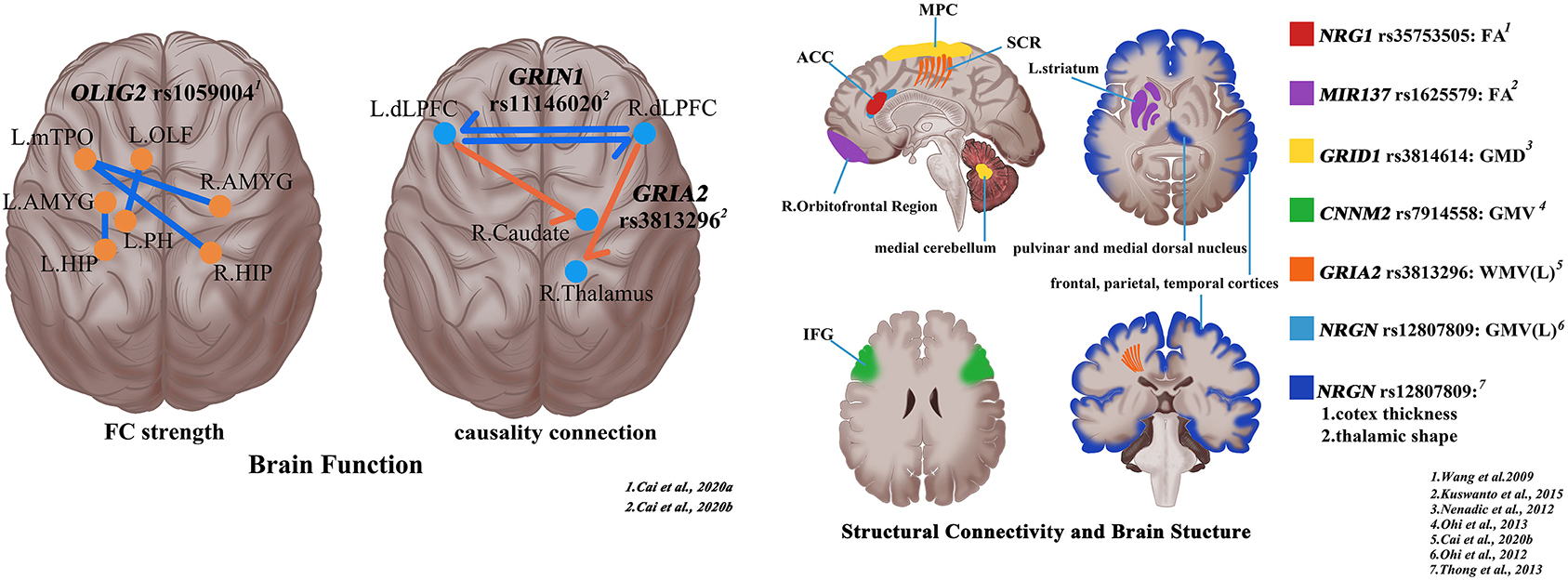
Figure 1. Shaping the trans-scale and multi-omics properties of schizophrenia via magnetic resonance imaging and single-nucleotide polymorphisms (SNPs). R, right; L, left; ACC, anterior cingulate cortex; MPC, medial parietal cortex; SCR, superior corona radiata; IFG, inferior frontal gyrus; FA, fractional anisotropy; GMD, gray matter density; GMV, Gray matter volume; WMV, white matter volume.
Most recently, functional striatal abnormalities have been developed as a new neuroimaging biomarker for the identification, prognosis, and subtyping of schizophrenia based on brain function (Li et al., 2020). Loci of striatal hyperactivity recapitulate the spatial distribution of dopaminergic function and the expression profiles of polygenic risk for schizophrenia. Furthermore, by applying a novel machine learning method, 413 genetic factors related to schizophrenia across 13 brain regions can be obviously identified (Huckins et al., 2019). The expression of schizophrenia-related genes is reflected in the whole neurodevelopmental process: some during specific stages of pregnancy, and others during adolescence or adulthood. Genetic influence on schizophrenia paves the way for the potential application of MRI in schizophrenia (Jiang et al., 2020). A network fusion-based approach has been applied to integrate three types of data, including genetic, epigenetic, and neuroimaging data, for the diagnosis and prediction of patients with schizophrenia (Su-Ping et al., 2016). For example, adolescents in a high-risk state can be screened by identified risk factors for schizophrenia, so as to predict and intervene at early stage in the future for adolescents who may suffer from schizophrenia. Future research will place more emphasis on integrated analysis of information across different dimensions supported by trans-scale and multi-omics technologies (van den Heuvel et al., 2019; Guan et al., 2021). This new trans-scale and multi-omics methods give us unprecedented power to understand the nature of schizophrenia.
But so far, this combination method has only played a hint and reference role in the pathogenesis of schizophrenia. Most of the studies involved in this article are hypothetical, only with the help of imaging methods to observe changes in brain structure and function in the presence of a specific SNPs. The researchers did not conduct animal experiments to confirm that the changes shown in the images were induced by the specific SNPs. This is a common problem in related research fields. At the same time, due to the complexity and genetic heterogeneity of schizophrenia, there are differences among regions, races, and populations. Therefore, experiments need to reduce the contingency and increase the universality of results. In addition, the sample size of most studies is relatively small, especially the studies on the effects of the relationship between genes and structure on the brain are based on healthy people. It is necessary to increase the sample size for the verification of the results. Furthermore, future research will place more emphasis on integrated analysis of information across different dimensions supported by trans-scale and multi-omics technologies. This is a great challenge to be faced in future research. It is also important to note that the use of antipsychotic drugs can lead to some alterations in the brain phenotype of patients with schizophrenia (Guo et al., 2019; Wang et al., 2019). Future studies need to be aware of whether or not patients with schizophrenia have been treated with antipsychotic medication when discussing changes in their brain phenotypes.
All in all, the new trans-scale and multi-omics methods give us unprecedented power to understand the nature of schizophrenia, which will promote the identification of biomarkers and risk prediction ability, provide help for the multigene strategy of heterogeneous dissection of schizophrenia, and further promote the implementation of individualized accurate diagnosis and treatment.
Author Contributions
Y-HZ, HY, and L-BC conceptualized the manuscript. S-WZ, XX, and L-BC wrote the first draft of the manuscript. All authors provided feedback and revised the manuscript.
Funding
This work was supported by the grant support of Fourth Military Medical University (2019CYJH) and the Project funded by the China Postdoctoral Science Foundation (2019TQ0130).
Conflict of Interest
The authors declare that the research was conducted in the absence of any commercial or financial relationships that could be construed as a potential conflict of interest.
Publisher's Note
All claims expressed in this article are solely those of the authors and do not necessarily represent those of their affiliated organizations, or those of the publisher, the editors and the reviewers. Any product that may be evaluated in this article, or claim that may be made by its manufacturer, is not guaranteed or endorsed by the publisher.
Acknowledgments
We are indebted to Xu-Sha Wu for her helpful suggestions and comments on the manuscript.
References
Brown, G. G., and Thompson, W. K. (2010). Functional brain imaging in schizophrenia: selected results and methods. Curr. Top. Behav. Neurosci. 4, 181–214. doi: 10.1007/7854_2010_54
Bush, W. S., and Moore, J. H. (2012). Chapter 11: genome-wide association studies. PLoS Comput. Biol. 8:e1002822. doi: 10.1371/journal.pcbi.1002822
Cai, S., Lv, Y., Huang, K., Zhang, W., Kang, Y., Huang, L., et al. (2020a). Association of rs1059004 polymorphism in the OLIG2 locus with whole-brain functional connectivity in first-episode schizophrenia. Behav. Brain Res. 379:112392. doi: 10.1016/j.bbr.2019.112392
Cai, S., Lv, Y., Huang, K., Zhang, W., Wang, Q., Huang, L., et al. (2020b). Modulation on glutamic pathway of frontal-striatum-thalamus by rs11146020 and rs3813296 gene polymorphism in first-episode negative schizophrenia. Front. Neurosci. 14, 351. doi: 10.3389/fnins.2020.00351
Chen, X., Zhang, Z., Zhang, Q., Zhao, W., Zhai, J., Chen, M., et al. (2018). Effect of rs1344706 in the ZNF804A gene on the brain network. Neuroimage Clin. 17, 1000–1005. doi: 10.1016/j.nicl.2017.12.017
Clemm von Hohenberg, C., Wigand, M. C., Kubicki, M., Leicht, G., Giegling, I., Karch, S., et al. (2013). CNTNAP2 polymorphisms and structural brain connectivity: a diffusion-tensor imaging study. J. Psychiatr. Res. 47, 1349–1356. doi: 10.1016/j.jpsychires.2013.07.002
Cui, L. B., Liu, L., Guo, F., Chen, Y. C., Chen, G., Xi, M., et al. (2017). Disturbed brain activity in resting-state networks of patients with first-episode schizophrenia with auditory verbal hallucinations: a cross-sectional functional MR imaging study. Radiology 283, 810–819. doi: 10.1148/radiol.2016160938
Cui, L. B., Liu, L., Wang, H. N., Wang, L. X., Guo, F., Xi, Y. B., et al. (2018). Disease definition for schizophrenia by functional connectivity using radiomics strategy. Schizophr. Bull. 44, 1053–1059. doi: 10.1093/schbul/sby007
Gong, X., Lu, W., Kendrick, K. M., Pu, W., Wang, C., Jin, L., et al. (2014). A brain-wide association study of DISC1 genetic variants reveals a relationship with the structure and functional connectivity of the precuneus in schizophrenia. Hum. Brain Mapp. 35, 5414–5430. doi: 10.1002/hbm.22560
Guan, F., Ni, T., Zhu, W., Williams, L. K., Cui, L.-B., Li, M., et al. (2021). Integrative omics of schizophrenia: from genetic determinants to clinical classification and risk prediction. Mol. Psychiatry. doi: 10.1038/s41380-021-01201-2. [Epub ahead of print].
Guo, F., Zhu, Y.-Q., Li, C., Wang, X.-R., Wang, H.-N., Liu, W.-M., et al. (2019). Gray matter volume changes following antipsychotic therapy in first-episode schizophrenia patients: a longitudinal voxel-based morphometric study. J. Psychiatric Res. 116, 126–132. doi: 10.1016/j.jpsychires.2019.06.009
Gurung, R., and Prata, D. P. (2015). What is the impact of genome-wide supported risk variants for schizophrenia and bipolar disorder on brain structure and function? A systematic review. Psychol. Med. 45, 2461–2480. doi: 10.1017/S0033291715000537
Huckins, L. M., Dobbyn, A., Ruderfer, D. M., Hoffman, G., Wang, W., Pardinas, A. F., et al. (2019). Gene expression imputation across multiple brain regions provides insights into schizophrenia risk. Nat. Genet. 51, 659–674. doi: 10.1038/s41588-019-0364-4
Jamadar, S., Powers, N. R., Meda, S. A., Calhoun, V. D., Gelernter, J., Gruen, J. R., et al. (2013). Genetic influences of resting state fMRI activity in language-related brain regions in healthy controls and schizophrenia patients: a pilot study. Brain Imaging Behav. 7, 15–27. doi: 10.1007/s11682-012-9168-1
Jiang, J. B., Cao, Y., An, N. Y., Yang, Q., and Cui, L. B. (2020). Magnetic resonance imaging-based connectomics in first-episode schizophrenia: from preclinical study to clinical translation. Front. Psychiatry 11:565056. doi: 10.3389/fpsyt.2020.565056
Kauppi, K., Rosenthal, S. B., Lo, M.-T., Sanyal, N., Jiang, M., Abagyan, R., et al. (2018). Revisiting antipsychotic drug actions through gene networks associated with schizophrenia. Am. J. Psychiatry 175, 674–682. doi: 10.1176/appi.ajp.2017.17040410
Kuswanto, C. N., Sum, M. Y., Qiu, A., Sitoh, Y. Y., Liu, J., and Sim, K. (2015). The impact of genome wide supported microRNA-137 (MIR137) risk variants on frontal and striatal white matter integrity, neurocognitive functioning, and negative symptoms in schizophrenia. Am. J. Med. Genet. B. Neuropsychiatr. Genet. 168B, 317–326. doi: 10.1002/ajmg.b.32314
Li, A., Zalesky, A., Yue, W., Howes, O., Yan, H., Liu, Y., et al. (2020). A neuroimaging biomarker for striatal dysfunction in schizophrenia. Nat. Med. 26, 558–565. doi: 10.1038/s41591-020-0793-8
Liu, A., Chen, X., Wang, Z. J., Xu, Q., Appel-Cresswell, S., and McKeown, M. J. (2014). A genetically informed, group FMRI connectivity modeling approach: application to schizophrenia. IEEE Trans. Biomed. Eng. 61, 946–956. doi: 10.1109/TBME.2013.2294151
Liu, L., Cui, L. B., Xi, Y. B., Wang, X. R., Liu, Y. C., Xu, Z. L., et al. (2019). Association between connectivity of hippocampal sub-regions and auditory verbal hallucinations in schizophrenia. Front. Neurosci. 13:424. doi: 10.3389/fnins.2019.00424
Lv, Y., Wu, S., Lin, Y., Wang, X., Wang, J., Cai, S., et al. (2020). Association of rs1059004 polymorphism in the OLIG2 locus with functional brain network in first-episode negative schizophrenia. Psychiatry Res. Neuroimaging 303:111130. doi: 10.1016/j.pscychresns.2020.111130
McCutcheon, R. A., Reis Marques, T., and Howes, O. D. (2020). Schizophrenia-an overview. JAMA Psychiatry 77, 201–210. doi: 10.1001/jamapsychiatry.2019.3360
Mounce, J., Luo, L., Caprihan, A., Liu, J., Perrone-Bizzozero, N. I., and Calhoun, V. D. (2014). Association of GRM3 polymorphism with white matter integrity in schizophrenia. Schizophr. Res. 155, 8–14. doi: 10.1016/j.schres.2014.03.003
Nenadic, I., Maitra, R., Scherpiet, S., Gaser, C., Schultz, C. C., Schachtzabel, C., et al. (2012). Glutamate receptor delta 1 (GRID1) genetic variation and brain structure in schizophrenia. J. Psychiatr. Res. 46, 1531–1539. doi: 10.1016/j.jpsychires.2012.08.026
Ohi, K., Hashimoto, R., Yamamori, H., Yasuda, Y., Fujimoto, M., Umeda-Yano, S., et al. (2013). The impact of the genome-wide supported variant in the cyclin M2 gene on gray matter morphology in schizophrenia. Behav. Brain Funct. 9:40. doi: 10.1186/1744-9081-9-40
Ohi, K., Hashimoto, R., Yasuda, Y., Nemoto, K., Ohnishi, T., Fukumoto, M., et al. (2012). Impact of the genome wide supported NRGN gene on anterior cingulate morphology in schizophrenia. PLoS ONE 7:e29780. doi: 10.1371/journal.pone.0029780
Papagni, S. A., Mechelli, A., Prata, D. P., Kambeitz, J., Fu, C. H., Picchioni, M., et al. (2011). Differential effects of DAAO on regional activation and functional connectivity in schizophrenia, bipolar disorder and controls. Neuroimage 56, 2283–2291. doi: 10.1016/j.neuroimage.2011.03.037
Pardinas, A. F., Holmans, P., Pocklington, A. J., Escott-Price, V., Ripke, S., Carrera, N., et al. (2018). Common schizophrenia alleles are enriched in mutation-intolerant genes and in regions under strong background selection. Nat. Genet. 50, 381–389. doi: 10.1038/s41588-018-0059-2
Perry, A., Roberts, G., Mitchell, P. B., and Breakspear, M. (2019). Connectomics of bipolar disorder: a critical review, and evidence for dynamic instabilities within interoceptive networks. Mol. Psychiatry 24, 1296–1318. doi: 10.1038/s41380-018-0267-2
Rasetti, R., Sambataro, F., Chen, Q., Callicott, J. H., Mattay, V. S., and Weinberger, D. R. (2011). Altered cortical network dynamics: a potential intermediate phenotype for schizophrenia and association with ZNF804A. Arch. Gen. Psychiatry 68, 1207–1217. doi: 10.1001/archgenpsychiatry.2011.103
Reddaway, J. T., Doherty, J. L., Lancaster, T., Linden, D., Walters, J. T., and Hall, J. (2018). Genomic and imaging biomarkers in schizophrenia. Curr. Top. Behav. Neurosci. 40, 325–352. doi: 10.1007/7854_2018_52
Roy, J., Anand, K., Mohapatra, S., Nayak, R., Chattopadhyay, T., and Mallick, B. (2020). Single nucleotide polymorphisms in piRNA-pathway genes: an insight into genetic determinants of human diseases. Mol. Genet. Genomics 295, 1–12. doi: 10.1007/s00438-019-01612-5
Schizophrenia Working Group of the Psychiatric Genomics C. (2014). Biological insights from 108 schizophrenia-associated genetic loci. Nature 511, 421–427. doi: 10.1038/nature13595
Su-Ping, D., Dongdong, L., Calhoun, V. D., and Yu-Ping, W. (2016). “Predicting schizophrenia by fusing networks from SNPs, DNA methylation and fMRI data,” in 2016 38th Annual International Conference of the IEEE Engineering in Medicine and Biology Society (EMBC) (Orlando, FL: IEEE), 1447–1450.
Tecelao, D., Mendes, A., Martins, D., Fu, C., Chaddock, C. A., Picchioni, M. M., et al. (2019). The effect of psychosis associated CACNA1C, and its epistasis with ZNF804A, on brain function. Genes Brain Behav. 18:e12510. doi: 10.1111/gbb.12510
Thong, J. Y., Qiu, A., Sum, M. Y., Kuswanto, C. N., Tuan, T. A., Donohoe, G., et al. (2013). Effects of the neurogranin variant rs12807809 on thalamocortical morphology in schizophrenia. PLoS ONE 8:e85603. doi: 10.1371/journal.pone.0085603
van den Heuvel, M. P., Scholtens, L. H., and Kahn, R. S. (2019). Multiscale neuroscience of psychiatric disorders. Biol. Psychiatry 86, 512–522. doi: 10.1016/j.biopsych.2019.05.015
Voineskos, A. N. (2015). Genetic underpinnings of white matter 'connectivity': heritability, risk, and heterogeneity in schizophrenia. Schizophr. Res. 161, 50–60. doi: 10.1016/j.schres.2014.03.034
Wang, F., Jiang, T., Sun, Z., Teng, S. L., Luo, X., Zhu, Z., et al. (2009). Neuregulin 1 genetic variation and anterior cingulum integrity in patients with schizophrenia and healthy controls. J. Psychiatry Neurosci. 34, 181–186. Available online at: http://jpn.ca/vol34-issue3/34-3-181/
Wang, L.-X., Guo, F., Zhu, Y.-Q., Wang, H.-N., Liu, W.-M., Li, C., et al. (2019). Effect of second-generation antipsychotics on brain network topology in first-episode schizophrenia: a longitudinal rs-fMRI study. Schizophrenia Res. 208, 160–166. doi: 10.1016/j.schres.2019.03.015
Wang, Q., Cheng, W., Li, M., Ren, H., Hu, X., Deng, W., et al. (2016). The CHRM3 gene is implicated in abnormal thalamo-orbital frontal cortex functional connectivity in first-episode treatment-naive patients with schizophrenia. Psychol. Med. 46, 1523–1534. doi: 10.1017/S0033291716000167
Wei, Q., Kang, Z., Diao, F., Guidon, A., Wu, X., Zheng, L., et al. (2013). No association of ZNF804A rs1344706 with white matter integrity in schizophrenia: a tract-based spatial statistics study. Neurosci. Lett. 532, 64–69. doi: 10.1016/j.neulet.2012.10.062
Wei, Q., Kang, Z., Diao, F., Shan, B., Li, L., Zheng, L., et al. (2012). Association of the ZNF804A gene polymorphism rs1344706 with white matter density changes in Chinese schizophrenia. Prog. Neuropsychopharmacol. Biol. Psychiatry 36, 122–127. doi: 10.1016/j.pnpbp.2011.08.021
Whitaker, K. J., Vértes, P. E., Romero-Garcia, R., Váša, F., Moutoussis, M., Prabhu, G., et al. (2016). Adolescence is associated with genomically patterned consolidation of the hubs of the human brain connectome. Proc. Natl. Acad. Sci. U. S. A. 113, 9105–9110. doi: 10.1073/pnas.1601745113
Yin, L., Chau, C. K. L., Sham, P.-C., and So, H.-C. (2019). Integrating clinical data and imputed transcriptome from GWAS to uncover complex disease subtypes: applications in psychiatry and cardiology. Am. J. Hum. Genet. 105, 1193–1212. doi: 10.1016/j.ajhg.2019.10.012
Zhang, Y., Gong, X., Yin, Z., Cui, L., Yang, J., Wang, P., et al. (2019). Association between NRGN gene polymorphism and resting-state hippocampal functional connectivity in schizophrenia. BMC Psychiatry 19:108. doi: 10.1186/s12888-019-2088-5
Zhang, Y., Yan, H., Liao, J., Yu, H., Jiang, S., Liu, Q., et al. (2018). ZNF804A Variation may affect hippocampal-prefrontal resting-state functional connectivity in schizophrenic and healthy individuals. Neurosci. Bull. 34, 507–516. doi: 10.1007/s12264-018-0221-y
Keywords: schizophrenia, magnetic resonance imaging, gene, trans-scale, single-nucleotide polymorphism
Citation: Zhao S-W, Xu X, Wang X-Y, Yan T-C, Cao Y, Yan Q-H, Chen K, Jin Y-C, Zhang Y-H, Yin H and Cui L-B (2021) Shaping the Trans-Scale Properties of Schizophrenia via Cerebral Alterations on Magnetic Resonance Imaging and Single-Nucleotide Polymorphisms of Coding and Non-Coding Regions. Front. Hum. Neurosci. 15:720239. doi: 10.3389/fnhum.2021.720239
Received: 04 June 2021; Accepted: 05 August 2021;
Published: 09 September 2021.
Edited by:
Mingrui Xia, Beijing Normal University, ChinaReviewed by:
Zhijiang Wang, Peking University Sixth Hospital, ChinaSuping Cai, Xidian University, China
Copyright © 2021 Zhao, Xu, Wang, Yan, Cao, Yan, Chen, Jin, Zhang, Yin and Cui. This is an open-access article distributed under the terms of the Creative Commons Attribution License (CC BY). The use, distribution or reproduction in other forums is permitted, provided the original author(s) and the copyright owner(s) are credited and that the original publication in this journal is cited, in accordance with accepted academic practice. No use, distribution or reproduction is permitted which does not comply with these terms.
*Correspondence: Ya-Hong Zhang, MTM1NzIyODE5ODZAMTI2LmNvbQ==; Hong Yin, eWluaG9uZ0BmbW11LmVkdS5jbg==; Long-Biao Cui, bGJjdWlAZm1tdS5lZHUuY24=
†These authors have contributed equally to this work