- 1Centre for Mind/Brain Sciences, University of Trento, Trento, Italy
- 2Interuniversity Research Centre “Cognition, Language, and Deafness”, CIRCLeS, Catania, Italy
- 3Max Planck Institute for Psycholinguistics, Donders Institute for Brain, Cognition, and Behaviour, Radboud University, Nijmegen, Netherlands
In face-to-face communication, humans are faced with multiple layers of discontinuous multimodal signals, such as head, face, hand gestures, speech and non-speech sounds, which need to be interpreted as coherent and unified communicative actions. This implies a fundamental computational challenge: optimally binding only signals belonging to the same communicative action while segregating signals that are not connected by the communicative content. How do we achieve such an extraordinary feat, reliably, and efficiently? To address this question, we need to further move the study of human communication beyond speech-centred perspectives and promote a multimodal approach combined with interdisciplinary cooperation. Accordingly, we seek to reconcile two explanatory frameworks recently proposed in psycholinguistics and sensory neuroscience into a neurocognitive model of multimodal face-to-face communication. First, we introduce a psycholinguistic framework that characterises face-to-face communication at three parallel processing levels: multiplex signals, multimodal gestalts and multilevel predictions. Second, we consider the recent proposal of a lateral neural visual pathway specifically dedicated to the dynamic aspects of social perception and reconceive it from a multimodal perspective (“lateral processing pathway”). Third, we reconcile the two frameworks into a neurocognitive model that proposes how multiplex signals, multimodal gestalts, and multilevel predictions may be implemented along the lateral processing pathway. Finally, we advocate a multimodal and multidisciplinary research approach, combining state-of-the-art imaging techniques, computational modelling and artificial intelligence for future empirical testing of our model.
Introduction
In face-to-face communication, we encounter multiple layers of discontinuous multimodal signals: head, face, mouth movements, hand gestures, speech and non-speech sounds. This implies a fundamental computational challenge: optimally binding only signals belonging to the same communicative action while segregating unrelated signals (Noppeney, 2021). Within this challenge, the temporal misalignment of fast-changing signals across different sensory channels raises a central binding problem (Chen and Vroomen, 2013). Finally, each conversational partner is taxed by fast turn-taking dynamics (Levinson, 2016). Despite these critical constraints, we process multimodal communicative signals faster than speech alone (Holler et al., 2018; Drijvers and Holler, 2022). Crucially, we use non-verbal communicative signals to facilitate semantic understanding (Özyürek, 2014) and pragmatic inference (Holler, 2022). How do we achieve such an extraordinary feat?
To address this question, we need to move beyond the prominent speech-centred research perspective on the neurocognitive mechanisms of human communication. Building on previous calls for the need to study language in its multimodal manifestation and ecological context (Levinson and Holler, 2014; Vigliocco et al., 2014; Hasson et al., 2018; Perniss, 2018), the view we put forward here seeks to reconcile two explanatory frameworks recently proposed in psycholinguistics and sensory neuroscience. Specifically, we first highlight that verbal and non-verbal communicative signals are integrated to represent socially relevant acts (Levinson and Holler, 2014) through domain-general mechanisms of multimodal integration and prediction (Holler and Levinson, 2019). Accordingly, we then reconceive the neuroscientific evidence of a third visual pathway, specialised for dynamic aspects of social perception (Pitcher and Ungerleider, 2021), from a multimodal perspective. Finally, we propose that the resulting brain network implements the sensory processing gateway necessary toward successful multimodal processing and interpretation of face-to-face communicative signals.
Multimodal processing in face-to-face interactions: A possible computational framework
Holler and Levinson (2019) recently outlined the key computational principles that support fast and efficient multimodal processing in face-to-face communication, with the ultimate goal of interpreting communicative social actions (Figure 1A). First, domain-general mechanisms of multimodal integration (Stein, 2012; Noppeney, 2021) are hypothesised to be co-opted for detecting communicative signals. For example, faster processing of multimodal relative to unimodal communicative inputs mirrors multimodal facilitation outside the domain of communication in humans (Murray et al., 2001; Senkowski, 2005; Diederich et al., 2009) and animals (Gingras et al., 2009). Holler and Levinson (2019) proposed that multimodal interactions resting on statistical regularities among sensory inputs allow chunking the stream of concurrent dynamic inputs into multiplex signals at a perceptual, pre-semantic level. Further, the statistical regularities between multiplex signals and communicative meanings generate multimodal gestalts that bear semantic and pragmatic value, thus signalling a specific social action. For example, eyebrow frowns often accompany a raising voice pitch to signal the intention to ask a question (Nota et al., 2021). Mechanisms of Gestalt perception (Wagemans et al., 2012), social affordance (Gallagher, 2020), and relevance (Sperber and Wilson, 1995) may jointly contribute to the recognition of multimodal communicative gestalts (Trujillo and Holler, 2023). Finally, the recognition of a specific social action may trigger top-down multilevel predictions about how the message will unfold in time. For example, frowning and pointing at an object typically anticipates a question about that object, triggering top-down hierarchical predictions at multiple sensory levels (e.g., vocal sounds, bodily movements) and linguistic levels (e.g., words, sentential units). Multiplex signals, multimodal gestalts, and multilevel predictions are thought to interact in a continuous, dialectic process, leading to incremental unification while the message unfolds (Hagoort, 2005, 2019). Specifically, this supports a parallel processing framework whereby the beginning of the message simultaneously activates multiple potential interpretations (i.e., multimodal gestalts). As the message unfolds, concurrent bottom-up sensory processing and multilevel predictions iteratively refine each other toward a final gestalt solution (Trujillo and Holler, 2023). Such a parallel account accommodates evidence that processing of communicative social actions starts early (Redcay and Carlson, 2015), perhaps in parallel to semantic comprehension (Tomasello et al., 2022).
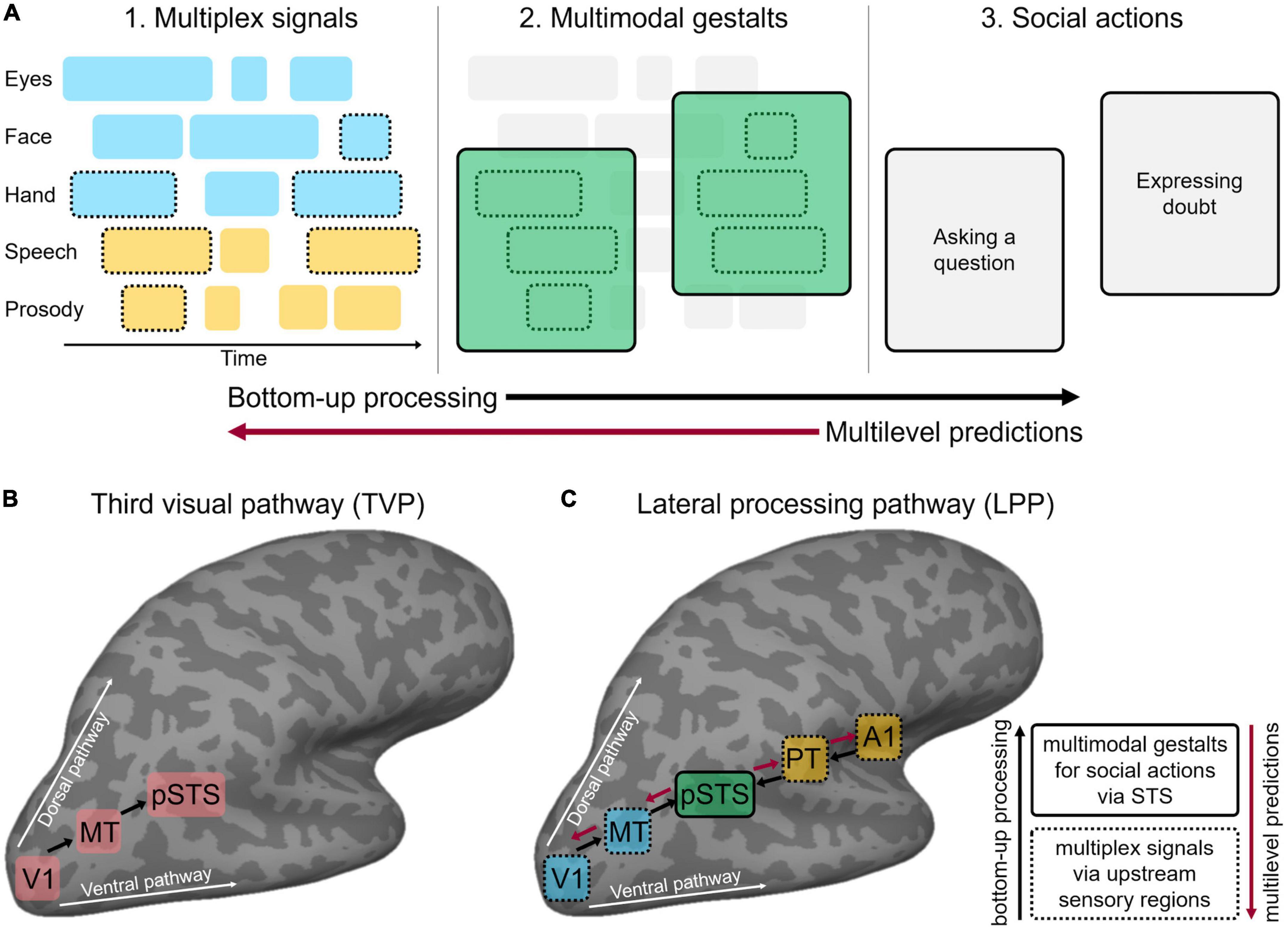
Figure 1. (A) Face-to-face communication comprises multiple layers of discontinuous multimodal signals emitted by different articulators (eyes, face, etc.) over time (visual in cyan, auditory in yellow). To enable effective communication, interlocutors must bind only signals belonging to the same communicative action while segregating tangential, unrelated signals that do not share the communicative content. Temporal statistical regularities allow chunking coherent communicative inputs into multiplex signals (dashed contours) at a perceptual, pre-semantic level (A1). Further, statistical regularities between multiplex signals and communicative meanings generate multimodal gestalts (solid contours) that bear semantic and pragmatic value (A2) and thus signal a specific communicative intention in conversation, i.e., social action (A3). Finally, social action recognition may trigger top-down multilevel predictions across hierarchically organised linguistic and perceptual levels. Following a parallel processing framework, concurrent bottom-up sensory processing (black arrow) and multilevel predictions (red arrow) iteratively refine each other. (B) Schematic representation of the third visual cortical pathway (TVP) specialised for the dynamic aspects of social perception, as proposed by Pitcher and Ungerleider (2021). The pathway originates in the primary visual cortex (V1) and dissociates from both the ventral and dorsal pathways by projecting into the posterior portion of the superior temporal sulcus (pSTS) via motion-selective areas (MT). (C) Schematic representation of the multimodal lateral processing pathway (LPP) implementing the sensory processing gateway toward successful face-to-face communication, as proposed in the present perspective. The LPP originates in early visual (V1) and auditory (A1) areas and dissociates from ventral and dorsal pathways by projecting to the mid-posterior portion of STS via animacy and motion-selective areas (here, MT and PT only are represented for clarity of visualisation). Black arrows indicate bottom-up processing along the LPP hierarchy from upstream regions (dashed contours) responsible for multiplex signals (panel A1) to portions of the pSTS (solid contours) that contribute toward the implementation of multimodal gestalts (panel A2). Red arrows indicate top-down multilevel predictions via pSTS to upstream visual and auditory areas (in cyan and yellow, respectively). Panel (A) is based on Holler and Levinson, 2019.
Supporting this framework, there is substantial psycholinguistic evidence for systematic associations between facial-bodily signals and social actions (Holler and Levinson, 2019; Nota et al., 2021). Moreover, the early emergence of these perceptual associations in infants (Cameron-Faulkner et al., 2015), as well as parallels in non-human primates (Rossano and Liebal, 2014), suggest they might be deeply rooted in the human onto- and phylogenesis.
Multimodal processing in face-to-face interactions: A possible neural framework
Accumulating evidence (Pitcher et al., 2014; Walbrin and Koldewyn, 2019; Landsiedel et al., 2022) suggests that dynamic visual aspects of social perception (e.g., face, hand and body movements across the visual field) cannot be easily accommodated within the classic dual-stream model for visual perception (Ungerleider and Mishkin, 1982). Accordingly, resting on both anatomical and functional evidence in humans and non-human primates, Pitcher and Ungerleider (2021) proposed the existence of a third visual processing pathway (Figure 1B) that projects on the lateral cortical surface from the early visual cortex into the mid-posterior superior temporal sulcus (pSTS) via motion-selective occipito-temporal areas (V5/hMT). Consistent evidence shows that pSTS preferentially responds to multiple types of dynamic social bodily inputs including eye, mouth, hands, and body movements (Allison et al., 2000; Hein and Knight, 2008; Deen et al., 2020). Importantly, both anterior hMT (Desimone and Ungerleider, 1986; Huk et al., 2002) and pSTS (Bruce et al., 1981; Pitcher et al., 2020; Finzi et al., 2021) respond to dynamic signals across both visual hemifields in human and non-human primates, in opposition to the contralateral field bias that characterises the ventral pathway (Finzi et al., 2021). Together, these functional properties are thought to support social interaction, which is an inherently dynamic process requiring the integration of sensory information across the entire visual field (Pitcher and Ungerleider, 2021).
Relevantly, Pitcher and Ungerleider (2021) note that the “proximity (to pSTS, a.n.) of brain areas computing multisensory information relevant to social interactions further dissociates the third pathway from the established role of the ventral and dorsal pathways.” We further elaborate on this by reconceiving the third visual pathway as a fundamental part of a larger multimodal neural system that implements fast analysis of multisensory communicative signals during face-to-face interactions. This pathway projects from early visual and auditory regions along the lateral brain surface and into the pSTS (lateral processing pathway; LPP). From this perspective, regions in the mid-posterior and lateral superior temporal gyrus, which are sensitive to auditory motion, animacy, sounds of moving bodies and dynamic aspects of human vocalisation (i.e., prosodic intonation), become candidate nodes of the auditory bank of LPP.
Analogously to the third visual pathway, evidence supporting the existence of a third lateral auditory cortical pathway, independent of dorsal/ventral pathways (Rauschecker, 1998; Rauschecker and Tian, 2000) and projecting via motion-sensitive regions into the posterior STS, comes from both tracer studies in macaques and in vivo white matter tractography in humans (see Table 1, connectivity profiles). These mid-posterior lateral areas showing anatomical connectivity with the pSTS also show motion-sensitive and voice-sensitive responses, suggesting functional selectivity for dynamic biologically-relevant information along this lateral auditory pathway (see Table 1, functional properties). Relevantly, functional interactions and direct anatomical connections have also been observed between auditory and visual motion-sensitive regions (see Table 1), suggesting a structural scaffolding for early convergence of multimodal information (Benetti and Collignon, 2022) within temporo-occipital regions of the LPP that might share the same computational goal: fast and reliable analysis of multimodal information relevant to social interactions.
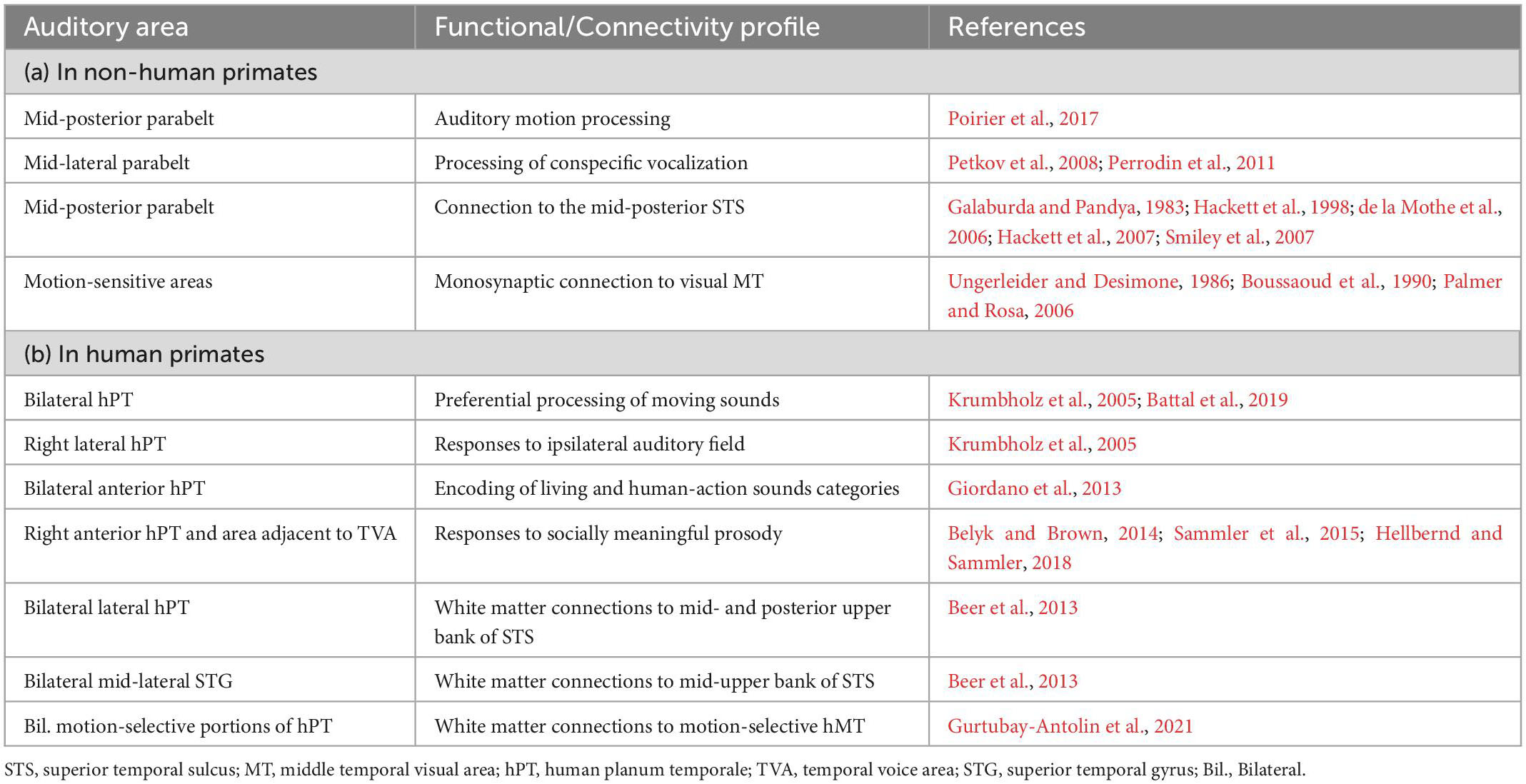
Table 1. Functional properties and structural connectivity profile of mid-posterior and lateral auditory areas in the superior temporal gyrus as described in (a) non-human and (b) human primates.
Toward a neurocognitive model of face-to-face communication
In the following section, we attempt to reconcile the psycholinguistic (Holler and Levinson, 2019) and sensory neuroscience (Pitcher and Ungerleider, 2021) frameworks, reviewed so far, toward a coherent neurocognitive model of multimodal face-to-face communication. Accordingly, we propose how key computational principles underlying the perception of multimodal social actions (multiplex signals, multimodal gestalts, and multilevel predictions) might be implemented along the LPP (Figure 1C).
Detecting multimodal co-occurrences: Multiplex signals via upstream sensory regions
Traditionally, it was thought that multimodal integration takes place in higher-order polysensory areas such as parietal or prefrontal cortices, after unimodal processing in early sensory regions (Ungerleider and Mishkin, 1982; Rauschecker and Tian, 2000); however, accumulating evidence over the past two decades shows clear cross-modal interactions between early sensory areas (Foxe and Schroeder, 2005; Ghazanfar and Schroeder, 2006; Kayser and Logothetis, 2007; Driver and Noesselt, 2008). In fact, several studies with humans (Foxe et al., 2000, 2002; Schürmann et al., 2006; Martuzzi et al., 2007; Besle et al., 2008; Lewis and Noppeney, 2010) and primates (Schroeder et al., 2001; Fu et al., 2003; Kayser et al., 2005, 2008; Lakatos et al., 2007) have proved driving or modulatory effects of cross-modal stimuli at the bottom of the sensory processing hierarchy. Beyond identifying multimodal interactions, such evidence also revealed their ubiquity across the (sub)cortical hierarchy and called for the need to further characterise the computational principles, neural properties and behavioural relevance of these interactions. One possibility is that they differ at different processing stages (i.e., multistage integration) along the (sub)cortical hierarchy (Calvert and Thesen, 2004; Noppeney et al., 2018; Noppeney, 2021).
Since visual bodily signals typically precede speech during natural face-to-face interactions (Nota et al., 2021), they may modulate the sound-induced activity in the auditory cortex by resetting the phase of ongoing oscillations (Biau et al., 2015; Mégevand et al., 2020; Pouw et al., 2021). In support of a temporally-sensitive mechanism, neurophysiological (Kayser et al., 2010; Atilgan et al., 2018), and fMRI studies (Lewis and Noppeney, 2010; Werner and Noppeney, 2011) have shown that audiovisual interactions in early auditory cortex and hPT depended on audiovisual temporal coincidence or coherence over time. Sensitivity to temporal co-occurrences is crucial to multiplex signals, which rest on temporal statistical regularities across sensory channels at a perceptual, pre-semantic level (Holler and Levinson, 2019). Therefore, it seems plausible that upstream sensory regions (e.g., visual and auditory cortices) interact in a temporally-sensitive fashion at corresponding processing stages (i.e., via multistage integration) to implement multiplex signals [see also Bizley et al. (2016)]. Specifically, it may be that primary visual and auditory cortices concur to support the automatic, salience-driven detection of multimodal co-occurrences, while secondary visual and auditory cortices along the LPP (hMT/EBA and hPT/TVA) concur to represent dynamic aspects of audiovisual bodily signals, mirroring results outside the realm of face-to-face communication (Lewis and Noppeney, 2010).
Recognizing communicative meanings: Multimodal gestalts via pSTS
As reviewed above, upstream visual and auditory sensory regions are structurally and functionally interconnected with pSTS. Crucially, this region represents a site of multimodal integration of social and non-social sensory information, as shown in neuroimaging and neurophysiological studies with humans (Beauchamp, 2005; Beauchamp et al., 2008; Werner and Noppeney, 2010a,b; Hirsch et al., 2018; Noah et al., 2020) and non-human primates (Ghazanfar et al., 2008; Froesel et al., 2021). While these studies employed non-linguistic but meaningful world categories such as animals, manipulable objects, and human actions, pSTS is also involved in the processing of communicative and meaningful audiovisual stimuli such as lip-speech (MacSweeney et al., 2000; Wright, 2003; Macaluso et al., 2004; van Atteveldt et al., 2004; Stevenson and James, 2009; Price, 2012; Venezia et al., 2017) and gesture-speech (Holle et al., 2008, 2010; Hubbard et al., 2009; Willems et al., 2016). Consistently, multimodal integration in pSTS may allow the creation of meaningful neural representations (Beauchamp et al., 2004; Noppeney et al., 2018), including those bearing semantic and pragmatic values for social communication (i.e., multimodal gestalts; Holler and Levinson, 2019). In particular, we propose that pSTS might concur toward such (multimodal) neural representations based on Bayesian Causal Inference principles (Körding et al., 2007; Shams and Beierholm, 2010; Noppeney, 2021), mirroring effects found along the dorsal audiovisual pathways for spatial localisation (Rohe and Noppeney, 2015, 2016; Aller and Noppeney, 2019; Ferrari and Noppeney, 2021).
Intriguingly, pSTS is positioned at the intersection of three brain systems respectively responsible for social perception, action observation, and theory of mind (Yang et al., 2015). As noticed by Pitcher and Ungerleider (2021), perceptual analysis of goal-directed actions in the pSTS likely influences activity in parietal and frontal systems that are responsible for action and intention recognition. As such, after receiving converging inputs from upstream sensory regions of the LPP, pSTS may represent the sensory processing gateway that feeds to higher-order networks for social action recognition during face-to-face communication. As a result, multiplex signals may be processed at the semantic and pragmatic levels, enabling the recognition of multimodal gestalts (Holler and Levinson, 2019).
Predicting how the conversation unfolds: Multilevel predictions along the cortical hierarchy
Increasing evidence shows that humans, among other species, build on their past experiences to construct predictive models of themselves and their sensory environment (de Lange et al., 2018). Accordingly, the brain can be conceived as a “prediction machine” (Clark, 2013) that attempts to match bottom-up sensory inputs with top-down expectations. Following hierarchical predictive coding (Rao and Ballard, 1999; Friston, 2005, 2010), any mismatch between expectation and actual input is signalled as a prediction error that propagates up the processing hierarchy to higher-level areas; vice versa, expected inputs are “explained away,” resulting in “expectation suppression” (Summerfield et al., 2008; Alink et al., 2010; Richter et al., 2018; Walsh et al., 2020). Importantly, expectation suppression reflects the neural tuning properties along a given processing hierarchy. For example, predictions about visual object and face identity are associated with expectation suppression respectively in object-selective regions (Meyer and Olson, 2011; Kaposvari et al., 2018; Richter et al., 2018; Ferrari et al., 2022; He et al., 2022) and face-selective regions (Summerfield et al., 2008; Amado et al., 2016; Schwiedrzik and Freiwald, 2017) along the ventral visual stream [for corresponding effects in the auditory domain, see e.g., Jaramillo and Zador (2011), Todorovic et al. (2011), Barascud et al. (2016), Heilbron and Chait (2018)].
Similarly, multilevel predictions during face-to-face interactions (Holler and Levinson, 2019) may be implemented via mechanisms of hierarchical predictive processing in neural pathways that are responsible for coding the relevant sensory information (e.g., vocal sounds, bodily movements) and linguistic information (e.g., words, sentential units, social actions). Increasing evidence shows signatures of hierarchical predictive processing during language comprehension in left-lateralized fronto-temporal regions of the language network (Blank and Davis, 2016; Sohoglu and Davis, 2016; Willems et al., 2016; Schmitt et al., 2021; Heilbron et al., 2022). Accordingly, predictive processing mechanisms may implement multimodal sensory predictions relevant to face-to-face interactions along the cortical hierarchy of the LPP. Initial evidence shows that hMT and pSTS activity is reduced in response to expected than unexpected visual actions (Koster-Hale and Saxe, 2013), such as human movements violating biomechanical predictions (Costantini et al., 2005; Saygin et al., 2012). Further, pSTS activity is reduced in response to actions that fit rather than violate the spatiotemporal structure of the environment (Koster-Hale and Saxe, 2013), such as shifting head and gaze toward rather than away an abrupt warning signal (Pelphrey et al., 2003). Interestingly, there is evidence of a functional dissociation between hMT and pSTS, with only the latter being sensitive to violations of action intentions (Pelphrey et al., 2004). Such dissociation is suggestive of a hierarchy of computations from sensory processing of dynamic inputs in hMT (at the level of multiplex signals) to semantic and pragmatic analysis in pSTS (at the level of multimodal gestalts), which may then be reflected in the respective expectation suppression profiles. Yet, it remains an open question whether and how multimodal (e.g., audiovisual) predictions arising from face-to-face interactions generate neural signatures of hierarchical predictive processing along the entire LPP, down to upstream sensory regions [for complementary evidence, see Lee and Noppeney (2014)]. Further, it is unknown whether and how higher-order expectations from language, action recognition and theory of mind networks may feed-back to pSTS (Yang et al., 2015) and thus travel down the LPP.
Discussion and conclusion
The current proposal leaves many aspects of the model un- or under-specified, including issues of hemispheric lateralization (Pitcher and Ungerleider, 2021) and the exact relationship between LPP and brain networks responsible for language (Hickok and Poeppel, 2000, 2007; Friederici, 2012; Hagoort, 2019), action recognition (Lingnau and Downing, 2015; Wurm and Caramazza, 2022), and theory of mind (Frith and Frith, 2006; Mar, 2011; Schaafsma et al., 2015). Future research must provide direct empirical evidence to support our framework, as well as refine and enrich it at the algorithmic and neural levels. To start, neuroimaging and neurostimulation techniques may characterise the functional and representational properties of the LPP as proposed here, as well as its degree of lateralization and interconnection with other brain networks (Thiebaut de Schotten and Forkel, 2022). Further, it will be crucial to combine these techniques with methodological approaches that enable human motion-tracking and near-to-optimal preservation of naturalistic, ecological contexts of face-to-face social interactions, such as virtual reality (Peeters, 2019). Complementarily, hyperscanning (Redcay and Schilbach, 2019; Hamilton, 2021) and multibrain stimulation techniques (Novembre and Iannetti, 2021) will be necessary to probe the functional relevance of the LPP during multimodal face-to-face processing across interacting brains. In parallel, the use of computational models (e.g., Bayesian Causal Inference) and neuroscientific-inspired artificial intelligence (i.e., convolutional or deep neural networks) could formalise the empirical evidence and test its role (e.g., necessity, sufficiency) for human behaviour (Hassabis et al., 2017) during face-to-face interactions. Last, but not least, it will be crucial to further embrace an interdisciplinary perspective in which psycholinguistics and neuroscientific frameworks would be reciprocally validated.
We conclude that the time is mature to accept the challenge we, among others before, advocated in this perspective and move beyond the speech-centred perspective dominating research on the neurocognitive mechanisms of human communication and language. We offer an original perspective bridging two recent propositions in psycholinguistics (Holler and Levinson, 2019) and sensory neuroscience (Pitcher and Ungerleider, 2021) into a neurocognitive model of multimodal face-to-face communication. Testing this framework represents a novel and promising endeavour for future research.
Author contributions
SB and AF contributed equally to the original conception of the perspective and wrote the first draft of the manuscript. FP contributed to further developing the preliminary conception. All authors contributed to manuscript revision, read, and approved the submitted version.
Funding
SB was supported by a “Starting Grant DM 737/21” from the University of Trento (R06). SB and FP were supported by a “Progetto di Rilevante Interesse Nazionale (PRIN)” from the Italian Ministry for Education, University and Research (MIUR-PRIN 2017 n.20177894ZH).
Acknowledgments
We would like to express our gratitude to Eugenio Parise for providing insightful comments on the preliminary version of this perspective.
Conflict of interest
The authors declare that the research was conducted in the absence of any commercial or financial relationships that could be construed as a potential conflict of interest.
The reviewer JT declared a shared parent affiliation with the author AF, and the handling editor declared a past collaboration with the author SB, at the time of review.
Publisher’s note
All claims expressed in this article are solely those of the authors and do not necessarily represent those of their affiliated organizations, or those of the publisher, the editors and the reviewers. Any product that may be evaluated in this article, or claim that may be made by its manufacturer, is not guaranteed or endorsed by the publisher.
References
Alink, A., Schwiedrzik, C. M., Kohler, A., Singer, W., and Muckli, L. (2010). Stimulus predictability reduces responses in primary visual cortex. J. Neurosci. 30, 2960–2966. doi: 10.1523/JNEUROSCI.3730-10.2010
Aller, M., and Noppeney, U. (2019). To integrate or not to integrate: Temporal dynamics of hierarchical Bayesian causal inference. PLoS Biol. 17:e3000210. doi: 10.1371/journal.pbio.3000210
Allison, T., Puce, A., and McCarthy, G. (2000). Social perception from visual cues: Role of the STS region. Trends Cogn. Sci. 4, 267–278. doi: 10.1016/S1364-6613(00)01501-1
Amado, C., Hermann, P., Kovács, P., Grotheer, M., Vidnyánszky, Z., and Kovács, G. (2016). The contribution of surprise to the prediction based modulation of fMRI responses. Neuropsychologia 84, 105–112. doi: 10.1016/j.neuropsychologia.2016.02.003
Atilgan, H., Town, S. M., Wood, K. C., Jones, G. P., Maddox, R. K., Lee, A. K. C., et al. (2018). Integration of visual information in auditory cortex promotes auditory scene analysis through multisensory binding. Neuron 97, 640–655.e4. doi: 10.1016/j.neuron.2017.12.034
Barascud, N., Pearce, M. T., Griffiths, T. D., Friston, K. J., and Chait, M. (2016). Brain responses in humans reveal ideal observer-like sensitivity to complex acoustic patterns. Proc. Natl. Acad. Sci. U.S.A. 113:E616–E625. doi: 10.1073/pnas.1508523113
Battal, C., Rezk, M., Mattioni, S., Vadlamudi, J., and Collignon, O. (2019). Representation of auditory motion directions and sound source locations in the human planum temporale. J. Neurosci. 39, 2208–2220. doi: 10.1523/JNEUROSCI.2289-18.2018
Beauchamp, M. S. (2005). See me, hear me, touch me: Multisensory integration in lateral occipital-temporal cortex. Curr. Opin. Neurobiol. 15, 145–153. doi: 10.1016/j.conb.2005.03.011
Beauchamp, M. S., Argall, B. D., Bodurka, J., Duyn, J. H., and Martin, A. (2004). Unraveling multisensory integration: Patchy organization within human STS multisensory cortex. Nat. Neurosci. 7, 1190–1192. doi: 10.1038/nn1333
Beauchamp, M. S., Yasar, N. E., Frye, R. E., and Ro, T. (2008). Touch, sound and vision in human superior temporal sulcus. Neuroimage 41, 1011–1020. doi: 10.1016/j.neuroimage.2008.03.015
Beer, A. L., Plank, T., Meyer, G., and Greenlee, M. W. (2013). Combined diffusion-weighted and functional magnetic resonance imaging reveals a temporal-occipital network involved in auditory-visual object processing. Front. Integr. Neurosci. 7:5. doi: 10.3389/fnint.2013.00005
Belyk, M., and Brown, S. (2014). Perception of affective and linguistic prosody: An ALE meta-analysis of neuroimaging studies. Soc. Cogn. Affect. Neurosci. 9, 1395–1403. doi: 10.1093/scan/nst124
Benetti, S., and Collignon, O. (2022). Cross-modal integration and plasticity in the superior temporal cortex. Handb. Clin. Neurol. 187, 127–143. doi: 10.1016/B978-0-12-823493-8.00026-2
Besle, J., Fischer, C., Bidet-Caulet, A., Lecaignard, F., Bertrand, O., and Giard, M.-H. (2008). Visual activation and audiovisual interactions in the auditory cortex during speech perception: Intracranial recordings in humans. J. Neurosci. 28, 14301–14310. doi: 10.1523/JNEUROSCI.2875-08.2008
Biau, E., Torralba, M., Fuentemilla, L., de Diego Balaguer, R., and Soto-Faraco, S. (2015). Speaker’s hand gestures modulate speech perception through phase resetting of ongoing neural oscillations. Cortex 68, 76–85. doi: 10.1016/j.cortex.2014.11.018
Bizley, J. K., Maddox, R. K., and Lee, A. K. C. (2016). Defining auditory-visual objects: Behavioral tests and physiological mechanisms. Trends Neurosci. 39, 74–85. doi: 10.1016/j.tins.2015.12.007
Blank, H., and Davis, M. H. (2016). Prediction Errors but Not Sharpened Signals Simulate Multivoxel fMRI Patterns during Speech Perception. PLoS Biol. 14:e1002577. doi: 10.1371/journal.pbio.1002577
Boussaoud, D., Ungerleider, L. G., and Desimone, R. (1990). Pathways for motion analysis: Cortical connections of the medial superior temporal and fundus of the superior temporal visual areas in the macaque. J. Comp. Neurol. 296, 462–495. doi: 10.1002/CNE.902960311
Bruce, C., Desimone, R., and Gross, C. G. (1981). Visual properties of neurons in a polysensory area in superior temporal sulcus of the macaque. J. Neurophysiol. 46, 369–384. doi: 10.1152/jn.1981.46.2.369
Calvert, G. A., and Thesen, T. (2004). Multisensory integration: Methodological approaches and emerging principles in the human brain. J. Physiol. Paris 98, 191–205. doi: 10.1016/j.jphysparis.2004.03.018
Cameron-Faulkner, T., Theakston, A., Lieven, E., and Tomasello, M. (2015). The relationship between infant holdout and gives, and pointing. Infancy 20, 576–586. doi: 10.1111/infa.12085
Chen, L., and Vroomen, J. (2013). Intersensory binding across space and time: A tutorial review. Atten. Percept. Psychophys. 75, 790–811. doi: 10.3758/s13414-013-0475-4
Clark, A. (2013). Whatever next? predictive brains, situated agents, and the future of cognitive science. Behav. Brain Sci. 36, 181–204. doi: 10.1017/S0140525X12000477
Costantini, M., Galati, G., Ferretti, A., Caulo, M., Tartaro, A., Romani, G. L., et al. (2005). Neural systems underlying observation of humanly impossible movements: An FMRI study. Cereb. Cortex 15, 1761–1767. doi: 10.1093/cercor/bhi053
de la Mothe, L. A., Blumell, S., Kajikawa, Y., and Hackett, T. A. (2006). Cortical connections of the auditory cortex in marmoset monkeys: Core and medial belt regions. J. Comp. Neurol. 496, 27–71. doi: 10.1002/cne.20923
de Lange, F. P., Heilbron, M., and Kok, P. (2018). How do expectations shape perception? Trends Cogn. Sci. 22, 764–779. doi: 10.1016/j.tics.2018.06.002
Deen, B., Saxe, R., and Kanwisher, N. (2020). Processing communicative facial and vocal cues in the superior temporal sulcus. Neuroimage 221:117191. doi: 10.1016/J.NEUROIMAGE.2020.117191
Desimone, R., and Ungerleider, L. G. (1986). Multiple visual areas in the caudal superior temporal sulcus of the macaque. J. Comp. Neurol. 248, 164–189. doi: 10.1002/cne.902480203
Diederich, N. J., Fénelon, G., Stebbins, G., and Goetz, C. G. (2009). Hallucinations in Parkinson disease. Nat. Rev. Neurol. 5, 331–342.
Drijvers, L., and Holler, J. (2022). The multimodal facilitation effect in human communication. Psychon. Bull. Rev. [Epub ahead of print]. doi: 10.3758/S13423-022-02178-X
Driver, J., and Noesselt, T. (2008). Multisensory interplay reveals crossmodal influences on “sensory-specific” brain regions, neural responses, and judgments. Neuron 57, 11–23. doi: 10.1016/j.neuron.2007.12.013
Ferrari, A., and Noppeney, U. (2021). Attention controls multisensory perception via two distinct mechanisms at different levels of the cortical hierarchy. PLoS Biol. 19:e3001465. doi: 10.1371/journal.pbio.3001465
Ferrari, A., Richter, D., and de Lange, F. P. (2022). Updating contextual sensory expectations for adaptive behaviour. J. Neurosci. 42, 8855–8869. doi: 10.1523/JNEUROSCI.1107-22.2022
Finzi, D., Gomez, J., Nordt, M., Rezai, A. A., Poltoratski, S., and Grill-Spector, K. (2021). Differential spatial computations in ventral and lateral face-selective regions are scaffolded by structural connections. Nat. Commun. 12:2278. doi: 10.1038/s41467-021-22524-2
Foxe, J. J., Morocz, I. A., Murray, M. M., Higgins, B. A., Javitt, D. C., and Schroeder, C. E. (2000). Multisensory auditory-somatosensory interactions in early cortical processing revealed by high-density electrical mapping. Cogn. Brain Res. 10, 77–83. doi: 10.1016/S0926-6410(00)00024-0
Foxe, J. J., and Schroeder, C. E. (2005). The case for feedforward multisensory convergence during early cortical processing. Neuroreport 16, 419–423. doi: 10.1097/00001756-200504040-00001
Foxe, J. J., Wylie, G. R., Martinez, A., Schroeder, C. E., Javitt, D. C., Guilfoyle, D., et al. (2002). Auditory-somatosensory multisensory processing in auditory association cortex: An fMRI study. J. Neurophysiol. 88, 540–543. doi: 10.1152/jn.00694.2001
Friederici, A. D. (2012). The cortical language circuit: From auditory perception to sentence comprehension. Trends Cogn. Sci. 16, 262–268. doi: 10.1016/j.tics.2012.04.001
Friston, K. (2005). A theory of cortical responses. Philos. Trans. R. Soc. B 360, 815–836. doi: 10.1098/rstb.2005.1622
Friston, K. (2010). The free-energy principle: A unified brain theory? Nat. Rev. Neurosci. 11, 127–138. doi: 10.1038/nrn2787
Frith, C. D., and Frith, U. (2006). The neural basis of mentalizing. Neuron 50, 531–534. doi: 10.1016/j.neuron.2006.05.001
Froesel, M., Gacoin, M., Clavagnier, S., Hauser, M., Goudard, Q., and Hamed, S. (2021). Neural correlates of audio-visual integration of socially meaningful information in macaque monkeys. bioRxiv [Preprint]. doi: 10.1101/2021.05.02.442333
Fu, K. M. G., Johnston, T. A., Shah, A. S., Arnold, L., Smiley, J., Hackett, T. A., et al. (2003). Auditory cortical neurons respond to somatosensory stimulation. J. Neurosci. 23, 7510–7515.
Galaburda, A. M., and Pandya, D. N. (1983). The intrinsic architectonic and connectional organization of the superior temporal region of the rhesus monkey. J. Comp. Neurol. 221, 169–184. doi: 10.1002/CNE.902210206
Gallagher, S. (ed.) (2020). “Direct Social Perception,” in Action and Interaction, (Oxford: Oxford University Press), 121–154. doi: 10.1093/oso/9780198846345.003.0007
Ghazanfar, A., and Schroeder, C. (2006). Is neocortex essentially multisensory? Trends Cogn. Sci. 10, 278–285. doi: 10.1016/j.tics.2006.04.008
Ghazanfar, A. A., Chandrasekaran, C., and Logothetis, N. K. (2008). Interactions between the superior temporal sulcus and auditory cortex mediate dynamic face/voice integration in rhesus monkeys. J. Neurosci. 28, 4457–4469. doi: 10.1523/JNEUROSCI.0541-08.2008
Gingras, G., Rowland, B. A., and Stein, B. E. (2009). The differing impact of multisensory and unisensory integration on behavior. J. Neurosci. 29, 4897–4902. doi: 10.1523/JNEUROSCI.4120-08.2009
Giordano, B. L., McAdams, S., Zatorre, R. J., Kriegeskorte, N., and Belin, P. (2013). Abstract encoding of auditory objects in cortical activity patterns. Cereb. Cortex 23, 2025–2037. doi: 10.1093/CERCOR/BHS162
Gurtubay-Antolin, A., Battal, C., Maffei, C., Rezk, M., Mattioni, S., Jovicich, J., et al. (2021). Direct structural connections between auditory and visual motion-selective regions in humans. J. Neurosci. 41, 2393–2405. doi: 10.1523/jneurosci.1552-20.2021
Hackett, T. A., Smiley, J. F., Ulbert, I., Karmos, G., Lakatos, P., De La Mothe, L. A., et al. (2007). Sources of somatosensory input to the caudal belt areas of auditory cortex. Perception 36, 1419–1430. doi: 10.1068/p5841
Hackett, T. A., Stepniewska, I., and Kaas, J. H. (1998). Subdivisions of auditory cortex and ipsilateral cortical connections of the parabelt auditory cortex in macaque monkeys. J. Comp. Neurol. 394, 475-495. doi: 10.1002/(SICI)1096-9861(19980518)394:4
Hagoort, P. (2005). On broca, brain, and binding: A new framework. Trends Cogn. Sci. 9, 416–423. doi: 10.1016/j.tics.2005.07.004
Hagoort, P. (2019). The neurobiology of language beyond single-word processing. Science 366, 55–58. doi: 10.1126/science.aax0289
Hamilton, A. F. C. (2021). Hyperscanning: Beyond the Hype. Neuron 109, 404–407. doi: 10.1016/j.neuron.2020.11.008
Hassabis, D., Kumaran, D., Summerfield, C., and Botvinick, M. (2017). Neuroscience-Inspired Artificial Intelligence. Neuron 95, 245–258. doi: 10.1016/j.neuron.2017.06.011
Hasson, U., Egidi, G., Marelli, M., and Willems, R. M. (2018). Grounding the neurobiology of language in first principles: The necessity of non-language-centric explanations for language comprehension. Cognition 180, 135–157. doi: 10.1016/J.COGNITION.2018.06.018
He, T., Richter, D., Wang, Z., and de Lange, F. P. (2022). Spatial and temporal context jointly modulate the sensory response within the ventral visual stream. J. Cogn. Neurosci. 34, 332–347. doi: 10.1162/jocn_a_01792
Heilbron, M., Armeni, K., Schoffelen, J. M., Hagoort, P., and de Lange, F. P. (2022). A hierarchy of linguistic predictions during natural language comprehension. Proc. Natl. Acad. Sci. U.S.A. 119:e2201968119. doi: 10.1073/pnas.2201968119
Heilbron, M., and Chait, M. (2018). Great expectations: Is there evidence for predictive coding in auditory cortex? Neuroscience 389, 54–73. doi: 10.1016/j.neuroscience.2017.07.061
Hein, G., and Knight, R. T. (2008). Superior temporal sulcus - it’s my area: Or is it? J. Cogn. Neurosci. 20, 2125–2136. doi: 10.1162/jocn.2008.20148
Hellbernd, N., and Sammler, D. (2018). Neural bases of social communicative intentions in speech. Soc. Cogn. Affect. Neurosci. 13, 604–615. doi: 10.1093/SCAN/NSY034
Hickok, G., and Poeppel, D. (2000). Towards a functional neuroanatomy of speech perception. Trends Cogn. Sci. 4, 131–138. doi: 10.1016/S1364-6613(00)01463-7
Hickok, G., and Poeppel, D. (2007). The cortical organization of speech processing. Nat. Rev. Neurosci. 8, 393–402. doi: 10.1038/nrn2113
Hirsch, J., Noah, J. A., Zhang, X., Dravida, S., and Ono, Y. (2018). A cross-brain neural mechanism for human-to-human verbal communication. Soc. Cogn. Affect. Neurosci. 13, 907–920. doi: 10.1093/scan/nsy070
Holle, H., Gunter, T. C., Rüschemeyer, S. A., Hennenlotter, A., and Iacoboni, M. (2008). Neural correlates of the processing of co-speech gestures. Neuroimage 39, 2010–2024. doi: 10.1016/j.neuroimage.2007.10.055
Holle, H., Obleser, J., Rueschemeyer, S. A., and Gunter, T. C. (2010). Integration of iconic gestures and speech in left superior temporal areas boosts speech comprehension under adverse listening conditions. Neuroimage 49, 875–884. doi: 10.1016/j.neuroimage.2009.08.058
Holler, J. (2022). Visual bodily signals as core devices for coordinating minds in interaction. Philos. Trans. R. Soc. B 377:20210094. doi: 10.1098/rstb.2021.0094
Holler, J., Kendrick, K. H., and Levinson, S. C. (2018). Processing language in face-to-face conversation: Questions with gestures get faster responses. Psychon. Bull. Rev. 25, 1900–1908. doi: 10.3758/s13423-017-1363-z
Holler, J., and Levinson, S. C. (2019). Multimodal Language Processing in Human Communication. Trends Cogn. Sci. 23, 639–652. doi: 10.1016/j.tics.2019.05.006
Hubbard, A. L., Wilson, S. M., Callan, D. E., and Dapretto, M. (2009). Giving speech a hand: Gesture modulates activity in auditory cortex during speech perception. Hum. Brain Mapp. 30, 1028–1037. doi: 10.1002/hbm.20565
Huk, A. C., Dougherty, R. F., and Heeger, D. J. (2002). Retinotopy and functional subdivision of human areas MT and MST. J. Neurosci. 22, 7195–7205. doi: 10.1523/jneurosci.22-16-07195.2002
Jaramillo, S., and Zador, A. M. (2011). The auditory cortex mediates the perceptual effects of acoustic temporal expectation. Nat. Neurosci. 14, 246–253. doi: 10.1038/nn.2688
Kaposvari, P., Kumar, S., and Vogels, R. (2018). Statistical learning signals in macaque inferior temporal cortex. Cereb. Cortex 28, 250–266. doi: 10.1093/cercor/bhw374
Kayser, C., and Logothetis, N. K. (2007). Do early sensory cortices integrate cross-modal information? Brain Struct. Funct. 212, 121–132. doi: 10.1007/s00429-007-0154-0
Kayser, C., Logothetis, N. K., and Panzeri, S. (2010). Visual enhancement of the information representation in auditory cortex. Curr. Biol. 20, 19–24. doi: 10.1016/j.cub.2009.10.068
Kayser, C., Petkov, C. I., Augath, M., and Logothetis, N. K. (2005). Integration of touch and sound in auditory cortex. Neuron 48, 373–384. doi: 10.1016/j.neuron.2005.09.018
Kayser, C., Petkov, C. I., and Logothetis, N. K. (2008). Visual modulation of neurons in auditory cortex. Cereb. Cortex 18, 1560–1574. doi: 10.1093/cercor/bhm187
Körding, K. P., Beierholm, U., Ma, W. J., Quartz, S., Tenenbaum, J. B., and Shams, L. (2007). Causal inference in multisensory perception. PLoS One 2:e943. doi: 10.1371/journal.pone.0000943
Koster-Hale, J., and Saxe, R. (2013). Theory of mind: A neural prediction problem. Neuron 79, 836–848. doi: 10.1016/j.neuron.2013.08.020
Krumbholz, K., Schönwiesner, M., Von Cramon, D. Y., Rübsamen, R., Shah, N. J., Zilles, K., et al. (2005). Representation of interaural temporal information from left and right auditory space in the human planum temporale and inferior parietal lobe. Cereb. Cortex 15, 317–324. doi: 10.1093/CERCOR/BHH133
Lakatos, P., Chen, C. M., O’Connell, M. N., Mills, A., and Schroeder, C. E. (2007). Neuronal oscillations and multisensory interaction in primary auditory cortex. Neuron 53, 279–292. doi: 10.1016/j.neuron.2006.12.011
Landsiedel, J., Daughters, K., Downing, P. E., and Koldewyn, K. (2022). The role of motion in the neural representation of social interactions in the posterior temporal cortex. Neuroimage 262, 119533. doi: 10.1016/j.neuroimage.2022.119533
Lee, H., and Noppeney, U. (2014). Temporal prediction errors in visual and auditory cortices. Curr. Biol. 24:R309–R310. doi: 10.1016/j.cub.2014.02.007
Levinson, S. C. (2016). Turn-taking in human communication – origins and implications for language processing. Trends Cogn. Sci. 20, 6–14. doi: 10.1016/J.TICS.2015.10.010
Levinson, S. C., and Holler, J. (2014). The origin of human multi-modal communication. Philos. Trans. R. Soc. B 369:20130302. doi: 10.1098/rstb.2013.0302
Lewis, R., and Noppeney, U. (2010). Audiovisual synchrony improves motion discrimination via enhanced connectivity between early visual and auditory areas. J. Neurosci. 30, 12329–12339. doi: 10.1523/JNEUROSCI.5745-09.2010
Lingnau, A., and Downing, P. E. (2015). The lateral occipitotemporal cortex in action. Trends Cogn. Sci. 19, 268–277. doi: 10.1016/j.tics.2015.03.006
Macaluso, E., George, N., Dolan, R., Spence, C., and Driver, J. (2004). Spatial and temporal factors during processing of audiovisual speech: A PET study. Neuroimage 21, 725–732. doi: 10.1016/j.neuroimage.2003.09.049
MacSweeney, M., Amaro, E., Calvert, G. A., Campbell, R., David, A. S., McGuire, P., et al. (2000). Silent speechreading in the absence of scanner noise: An event-related fMRI study. Neuroreport 11:1729. doi: 10.1097/00001756-200006050-00026
Mar, R. A. (2011). The neural bases of social cognition and story comprehension. Annu. Rev. Psychol. 62, 103–134. doi: 10.1146/annurev-psych-120709-145406
Martuzzi, R., Murray, M. M., Michel, C. M., Thiran, J. P., Maeder, P. P., Clarke, S., et al. (2007). Multisensory interactions within human primary cortices revealed by BOLD dynamics. Cereb. Cortex 17, 1672–1679. doi: 10.1093/cercor/bhl077
Mégevand, P., Mercier, M. R., Groppe, D. M., Golumbic, E. Z., Mesgarani, N., Beauchamp, M. S., et al. (2020). Crossmodal phase reset and evoked responses provide complementary mechanisms for the influence of visual speech in auditory cortex. J. Neurosci. 40, 8530–8542. doi: 10.1523/JNEUROSCI.0555-20.2020
Meyer, T., and Olson, C. R. (2011). Statistical learning of visual transitions in monkey inferotemporal cortex. Proc. Natl. Acad. Sci. U.S.A. 108, 19401–19406. doi: 10.1073/pnas.1112895108
Murray, M. M., Foxe, J. J., Higgins, B. A., Javitt, D. C., and Schroeder, C. E. (2001). Visuo-spatial neural response interactions in early cortical processing during a simple reaction time task: A high-density electrical mapping study. Neuropsychologia 39, 828–844. doi: 10.1016/S0028-3932(01)00004-5
Noah, J. A., Zhang, X., Dravida, S., Ono, Y., Naples, A., McPartland, J. C., et al. (2020). Real-time eye-to-eye contact is associated with cross-brain neural coupling in angular gyrus. Front. Hum. Neurosci. 14:19. doi: 10.3389/fnhum.2020.00019
Noppeney, U. (2021). Perceptual inference, learning, and attention in a multisensory world. Annu. Rev. Neurosci. 44, 449–473. doi: 10.1146/annurev-neuro-100120-085519
Noppeney, U., Jones, S. A., Rohe, T., and Ferrari, A. (2018). See what you hear-How the brain forms representations across the senses. Neuroforum 24, 237–246. doi: 10.1515/nf-2017-A066
Nota, N., Trujillo, J. P., and Holler, J. (2021). Facial signals and social actions in multimodal face-to-face interaction. Brain Sci. 11:1017. doi: 10.3390/brainsci11081017
Novembre, G., and Iannetti, G. D. (2021). Hyperscanning alone cannot prove causality. Multibrain stimulation can. Trends Cogn. Sci. 25, 96–99. doi: 10.1016/j.tics.2020.11.003
Özyürek, A. (2014). Hearing and seeing meaning in speech and gesture: Insights from brain and behaviour. Philos. Trans. R. Soc. B 369:20130296. doi: 10.1098/rstb.2013.0296
Palmer, S. M., and Rosa, M. G. P. (2006). Quantitative analysis of the corticocortical projections to the middle temporal area in the marmoset monkey: Evolutionary and functional implications. Cereb. Cortex 16, 1361–1375. doi: 10.1093/cercor/bhj078
Peeters, D. (2019). Virtual reality: A game-changing method for the language sciences. Psychon. Bull. Rev. 26, 894–900. doi: 10.3758/s13423-019-01571-3
Pelphrey, K. A., Mitchell, T. V., McKeown, M. J., Goldstein, J., Allison, T., and McCarthy, G. (2003). Brain activity evoked by the perception of human walking: Controlling for meaningful coherent motion. J. Neurosci. 23, 6819–6825. doi: 10.1523/JNEUROSCI.23-17-06819.2003
Pelphrey, K. A., Morris, J. P., and McCarthy, G. (2004). Grasping the intentions of others: The perceived intentionality of an action influences activity in the superior temporal sulcus during social perception. J. Cogn. Neurosci. 16, 1706–1716. doi: 10.1162/0898929042947900
Perniss, P. (2018). Why we should study multimodal language. Front. Psychol. 9:1109. doi: 10.3389/FPSYG.2018.01109/BIBTEX
Perrodin, C., Kayser, C., Logothetis, N. K., and Petkov, C. I. (2011). Voice cells in the primate temporal lobe. Curr. Biol. 21, 1408–1415. doi: 10.1016/j.cub.2011.07.028
Petkov, C. I., Kayser, C., Steudel, T., Whittingstall, K., Augath, M., and Logothetis, N. K. (2008). A voice region in the monkey brain. Nat. Neurosci. 11, 367–374.
Pitcher, D., Duchaine, B., and Walsh, V. (2014). Combined TMS and fMRI reveal dissociable cortical pathways for dynamic and static face perception. Curr. Biol. 24, 2066–2070. doi: 10.1016/j.cub.2014.07.060
Pitcher, D., Pilkington, A., Rauth, L., Baker, C., Kravitz, D. J., and Ungerleider, L. G. (2020). The human posterior superior temporal sulcus samples visual space differently from other face-selective regions. Cereb. Cortex 30, 778–785. doi: 10.1093/cercor/bhz125
Pitcher, D., and Ungerleider, L. G. (2021). Evidence for a third visual pathway specialized for social perception. Trends Cogn. Sci. 25, 100–110. doi: 10.1016/j.tics.2020.11.006
Poirier, C., Baumann, S., Dheerendra, P., Joly, O., Hunter, D., Balezeau, F., et al. (2017). Auditory motion-specific mechanisms in the primate brain. PLoS Biol. 15:e2001379. doi: 10.1371/JOURNAL.PBIO.2001379
Pouw, W., Proksch, S., Drijvers, L., Gamba, M., Holler, J., Kello, C., et al. (2021). Multilevel rhythms in multimodal communication. Philos. Trans. R. Soc. B 376: 20200334. doi: 10.31219/OSF.IO/PSMHN
Price, C. J. (2012). A review and synthesis of the first 20years of PET and fMRI studies of heard speech, spoken language and reading. Neuroimage 62, 816–847. doi: 10.1016/j.neuroimage.2012.04.062
Rao, R. P. N., and Ballard, D. H. (1999). Predictive coding in the visual cortex: A functional interpretation of some extra-classical receptive-field effects. Nat. Neurosci. 2, 79–87. doi: 10.1038/4580
Rauschecker, J. P. (1998). Cortical processing of complex sounds. Curr. Opin. Neurobiol. 8, 516–521. doi: 10.1016/S0959-4388(98)80040-8
Rauschecker, J. P., and Tian, B. (2000). Mechanisms and streams for processing of “what” and “where” in auditory cortex. Proc. Natl. Acad. Sci. U.S.A. 97, 11800–11806. doi: 10.1073/pnas.97.22.11800
Redcay, E., and Carlson, T. A. (2015). Rapid neural discrimination of communicative gestures. Soc. Cogn. Affect. Neurosci. 10, 545–551. doi: 10.1093/scan/nsu089
Redcay, E., and Schilbach, L. (2019). Using second-person neuroscience to elucidate the mechanisms of social interaction. Nat. Rev. Neurosci. 20, 495–505. doi: 10.1038/s41583-019-0179-4
Richter, D., Ekman, M., and de Lange, F. P. (2018). Suppressed sensory response to predictable object stimuli throughout the ventral visual stream. J. Neurosci. 38, 7452–7461. doi: 10.1523/JNEUROSCI.3421-17.2018
Rohe, T., and Noppeney, U. (2015). Cortical hierarchies perform bayesian causal inference in multisensory perception. PLoS Biol. 13:e1002073. doi: 10.1371/journal.pbio.1002073
Rohe, T., and Noppeney, U. (2016). Distinct computational principles govern multisensory integration in primary sensory and association cortices. Curr. Biol. 26, 509–514. doi: 10.1016/j.cub.2015.12.056
Rossano, F., and Liebal, K. (2014). “Requests and offers,” in orangutans and human infants,” in Requesting in social interaction, ed. P. Drew (Amsterdam: Benjamins), 335–364. doi: 10.1075/slsi.26.13ros
Sammler, D., Grosbras, M. H., Anwander, A., Bestelmeyer, P. E. G., and Belin, P. (2015). Dorsal and ventral pathways for prosody. Curr. Biol. 25, 3079–3085. doi: 10.1016/J.CUB.2015.10.009
Saygin, A. P., Chaminade, T., Ishiguro, H., Driver, J., and Frith, C. (2012). The thing that should not be: Predictive coding and the uncanny valley in perceiving human and humanoid robot actions. Soc. Cogn. Affect. Neurosci. 7, 413–422. doi: 10.1093/scan/nsr025
Schaafsma, S. M., Pfaff, D. W., Spunt, R. P., and Adolphs, R. (2015). Deconstructing and reconstructing theory of mind. Trends Cogn. Sci. 19, 65–72. doi: 10.1016/j.tics.2014.11.007
Schmitt, L. M., Erb, J., Tune, S., Rysop, A. U., Hartwigsen, G., and Obleser, J. (2021). Predicting speech from a cortical hierarchy of event-based time scales. Sci. Adv. 7:eabi6070. doi: 10.1126/sciadv.abi6070
Schroeder, C. E., Lindsley, R. W., Specht, C., Marcovici, A., Smiley, J. F., and Javitt, D. C. (2001). Somatosensory input to auditory association cortex in the macaque monkey. J. Neurophysiol. 85, 1322–1327.
Schürmann, M., Caetano, G., Hlushchuk, Y., Jousmäki, V., and Hari, R. (2006). Touch activates human auditory cortex. Neuroimage 30, 1325–1331. doi: 10.1016/j.neuroimage.2005.11.020
Schwiedrzik, C. M., and Freiwald, W. A. (2017). High-level prediction signals in a low-level area of the macaque face-processing hierarchy. Neuron 96, 89–97.e4. doi: 10.1016/j.neuron.2017.09.007
Senkowski, D. (2005). Oscillatory beta activity predicts response speed during a multisensory audiovisual reaction time task: A high-density electrical mapping study. Cereb. Cortex 16, 1556–1565. doi: 10.1093/cercor/bhj091
Shams, L., and Beierholm, U. R. (2010). Causal inference in perception. Trends Cogn. Sci. 14, 425–432. doi: 10.1016/j.tics.2010.07.001
Smiley, J. F., Hackett, T. A., Ulbert, I., Karmas, G., Lakatos, P., Javitt, D. C., et al. (2007). Multisensory convergence in auditory cortex, I. Cortical connections of the caudal superior temporal plane in macaque monkeys. J. Comp. Neurol. 502, 894–923. doi: 10.1002/CNE.21325
Sohoglu, E., and Davis, M. H. (2016). Perceptual learning of degraded speech by minimizing prediction error. Proc. Natl. Acad. Sci. U.S.A. 113:E1747–E1756. doi: 10.1073/pnas.1523266113
Sperber, D., and Wilson, D. (1995). Relevance: Communication and cognition, 2nd Edn. Hoboken, NJ: Blackwell Publishing.
Stein, B. E. (2012). The new handbook of multisensory processing. Cambridge, MA: The MIT Press. doi: 10.7551/mitpress/8466.003.0001
Stevenson, R. A., and James, T. W. (2009). Audiovisual integration in human superior temporal sulcus: Inverse effectiveness and the neural processing of speech and object recognition. Neuroimage 44, 1210–1223. doi: 10.1016/j.neuroimage.2008.09.034
Summerfield, C., Trittschuh, E. H., Monti, J. M., Mesulam, M. M., and Egner, T. (2008). Neural repetition suppression reflects fulfilled perceptual expectations. Nat. Neurosci. 11, 1004–1006. doi: 10.1038/nn.2163
Thiebaut de Schotten, M., and Forkel, S. J. (2022). The emergent properties of the connected brain. Science 378, 505–510. doi: 10.1126/science.abq2591
Todorovic, A., van Ede, F., Maris, E., and de Lange, F. P. (2011). Prior expectation mediates neural adaptation to repeated sounds in the auditory cortex: An MEG study. J. Neurosci. 31, 9118–9123. doi: 10.1523/JNEUROSCI.1425-11.2011
Tomasello, R., Grisoni, L., Boux, I., Sammler, D., and Pulvermüller, F. (2022). Instantaneous neural processing of communicative functions conveyed by speech prosody. Cereb. Cortex 32, 4885–4901. doi: 10.1093/cercor/bhab522
Trujillo, J. P., and Holler, J. (2023). Interactionally embedded gestalt principles of multimodal human communication. Perspect. Psychol. Sci. doi: 10.1177/17456916221141422
Ungerleider, L. G., and Desimone, R. (1986). Cortical connections of visual area MT in the macaque. J. Comp. Neurol. 248, 190–222. doi: 10.1002/CNE.902480204
Ungerleider, L. G., and Mishkin, M. (1982). “Two cortical visual systems,” in Analysis of visual behavior, eds D. Ingle, M. Goodale, and R. Mansfield (Cambridge: MIT Press).
van Atteveldt, N., Formisano, E., Goebel, R., and Blomert, L. (2004). Integration of letters and speech sounds in the human brain. Neuron 43, 271–282. doi: 10.1016/j.neuron.2004.06.025
Venezia, J. H., Vaden, K. I., Rong, F., Maddox, D., Saberi, K., and Hickok, G. (2017). Auditory, visual and audiovisual speech processing streams in superior temporal sulcus. Front. Hum. Neurosci. 11:174. doi: 10.3389/fnhum.2017.00174
Vigliocco, G., Perniss, P., and Vinson, D. (2014). Language as a multimodal phenomenon: Implications for language learning, processing and evolution. Philos. Trans. R. Soc. B 369:20130292. doi: 10.1098/RSTB.2013.0292
Wagemans, J., Elder, J. H., Kubovy, M., Palmer, S. E., Peterson, M. A., Singh, M., et al. (2012). A century of Gestalt psychology in visual perception: I. Perceptual grouping and figure-ground organization. Psychol. Bull. 138, 1172–1217. doi: 10.1037/a0029333
Walbrin, J., and Koldewyn, K. (2019). Dyadic interaction processing in the posterior temporal cortex. Neuroimage 198, 296–302. doi: 10.1016/j.neuroimage.2019.05.027
Walsh, K. S., McGovern, D. P., Clark, A., and O’Connell, R. G. (2020). Evaluating the neurophysiological evidence for predictive processing as a model of perception. Ann. N. Y. Acad. Sci. 1464, 242–268. doi: 10.1111/nyas.14321
Werner, S., and Noppeney, U. (2010b). Superadditive responses in superior temporal sulcus predict audiovisual benefits in object categorization. Cereb. Cortex 20, 1829–1842. doi: 10.1093/cercor/bhp248
Werner, S., and Noppeney, U. (2010a). Distinct functional contributions of primary sensory and association areas to audiovisual integration in object categorization. J. Neurosci. 30, 2662–2675. doi: 10.1523/JNEUROSCI.5091-09.2010
Werner, S., and Noppeney, U. (2011). The contributions of transient and sustained response codes to audiovisual integration. Cereb. Cortex 21, 920–931. doi: 10.1093/cercor/bhq161
Willems, R. M., Frank, S. L., Nijhof, A. D., Hagoort, P., and van den Bosch, A. (2016). Prediction during natural language comprehension. Cereb. Cortex 26, 2506–2516. doi: 10.1093/cercor/bhv075
Wright, T. M. (2003). Polysensory interactions along lateral temporal regions evoked by audiovisual speech. Cereb. Cortex 13, 1034–1043. doi: 10.1093/cercor/13.10.1034
Wurm, M. F., and Caramazza, A. (2022). Two ‘what’ pathways for action and object recognition. Trends Cogn. Sci. 26, 103–116. doi: 10.1016/j.tics.2021.10.003
Keywords: multimodal communication, face-to-face interactions, social actions, lateral cortical processing pathway, psycholinguistics, sensory neuroscience
Citation: Benetti S, Ferrari A and Pavani F (2023) Multimodal processing in face-to-face interactions: A bridging link between psycholinguistics and sensory neuroscience. Front. Hum. Neurosci. 17:1108354. doi: 10.3389/fnhum.2023.1108354
Received: 25 November 2022; Accepted: 11 January 2023;
Published: 02 February 2023.
Edited by:
Elena Nava, University of Milano-Bicocca, ItalyReviewed by:
James P. Trujillo, Radboud University, NetherlandsHuriye Atilgan, University of Oxford, United Kingdom
Jun-ichiro Watanabe, Hitachi, Japan
Copyright © 2023 Benetti, Ferrari and Pavani. This is an open-access article distributed under the terms of the Creative Commons Attribution License (CC BY). The use, distribution or reproduction in other forums is permitted, provided the original author(s) and the copyright owner(s) are credited and that the original publication in this journal is cited, in accordance with accepted academic practice. No use, distribution or reproduction is permitted which does not comply with these terms.
*Correspondence: Stefania Benetti, c3RlZmFuaWEuYmVuZXR0aUB1bml0bi5pdA==
†These authors have contributed equally to this work and share first authorship