- 1Laboratory of Biophysics and Bioinformatics, Neuroscience Institute, Lithuanian University of Health Sciences, Kaunas, Lithuania
- 2Department of Informatics, Vytautas Magnus University, Kaunas, Lithuania
- 3Université Côte d'Azur, Centre National de la Recherche Scientifique (CNRS) UMR 7275, Institut de Pharmacologie Moléculaire et Cellulaire (IPMC), Valbonne, France
- 4Institute of Biophysics, National Research Council, Palermo, Italy
Synaptic plasticity is believed to be a key mechanism underlying learning and memory. We developed a phenomenological N-methyl-D-aspartate (NMDA) receptor-based voltage-dependent synaptic plasticity model for synaptic modifications at hippocampal CA3-CA1 synapses on a hippocampal CA1 pyramidal neuron. The model incorporates the GluN2A-NMDA and GluN2B-NMDA receptor subunit-based functions and accounts for the synaptic strength dependence on the postsynaptic NMDA receptor composition and functioning without explicitly modeling the NMDA receptor-mediated intracellular calcium, a local trigger of synaptic plasticity. We embedded the model into a two-compartmental model of a hippocampal CA1 pyramidal cell and validated it against experimental data of spike-timing-dependent synaptic plasticity (STDP), high and low-frequency stimulation. The developed model predicts altered learning rules in synapses formed on the apical dendrites of the detailed compartmental model of CA1 pyramidal neuron in the presence of the GluN2B-NMDA receptor hypofunction and can be used in hippocampal networks to model learning in health and disease.
1. Introduction
Long-term synaptic plasticity has been proposed to be the cellular substrate of learning and memory in the brain (Malenka and Nicoll, 1999; Malenka and Bear, 2004). In the hippocampal CA1 area, CA3 Schaffer collateral-CA1 pyramidal neuron synapses can undergo long-term potentiation (LTP) and long-term depression (LTD), triggered by high or low frequency presynaptic stimulation (Collingridge et al., 1983; Dudek and Bear, 1992; Mulkey and Malenka, 1992; Bliss and Collingridge, 1993; Goh and Manahan-Vaughan, 2013; Pousinha et al., 2017). Spike-timing-dependent synaptic plasticity (STDP) is a bidirectional form of synaptic modifications, induced by correlated pre- and postsynaptic neuronal activation, where precise timing of spikes is a major determinant of the direction and magnitude of synaptic modifications (Markram et al., 1997; Bi and Poo, 1998; Debanne et al., 1998; Feldman, 2012). The induction of LTP, LTD, and STDP in excitatory synapses at Schaffer collateral pathway requires activation of N-methyl-D-aspartate receptors (NMDARs) (Bliss and Collingridge, 1993, 2013; Collingridge and Bliss, 1995; Lüscher and Malenka, 2012; Volianskis et al., 2015). An NMDA-mediated rise in postsynaptic calcium triggers complex biochemical signaling pathways and translates into α-amino-3-hydroxy-5-methyl-4-isoxazole propionic acid receptors (AMPARs) insertion or removal (MacDermott et al., 1986; MacDonald et al., 2006; Lau et al., 2009) underlying LTP and LTD.
The function of NMDARs carries a profound effect on learning, memory, connectivity of neural networks in hippocampus, cognition, and psychiatric diseases (Buzsáki, 2002; Liu et al., 2004). NMDARs are composed of two GluN1 subunits and two GluN2 subunits, which may be of the GluN2A, GluN2B, GluN2C, and GluN2D type, and a pair of GluN3A and GluN3B subunits (Cull-Candy et al., 2001; Paoletti, 2011). NMDA GluN1/GluN2A channels exhibit faster kinetics than NMDA GluN1/GluN2B type channels (Cull-Candy et al., 2001). NMDA receptors in the hippocampus are composed mainly of GluN2A-NMDA and GluN2B-NMDA type subunits that are important for synaptic plasticity and normal memory functioning.
The GluN2B-NMDAR subunit plays a critical role in the induction of LTD and LTP. The deficits of GluN2B-NMDAR impaired LTP in hippocampal slices (Gardoni et al., 2009), and inhibition of this receptor subunit led to disruption or abolishment of synaptic plasticity in CA1 pyramidal neurons (Clayton et al., 2002; Berberich et al., 2005; Foster et al., 2010; Zamzow et al., 2013; France et al., 2017; Pousinha et al., 2017). Overexpression of the GluN2B-NMDAR subunit in the transgenic mice improved memory in cortex (Cui et al., 2011), while its blockade by the GluN2B-NMDAR subunit-specific antagonist, ifenprodil, disrupted fear memory (Zhao et al., 2005). Blocking GluN2B-NMDAR led the abolishment of LTP (Morishita et al., 2007; Andrade-Talavera et al., 2016; Pousinha et al., 2017) at hippocampal CA3-CA1 synapses.
GluN2B-NMDAR is strongly coupled with calcium-calmodulin-dependent protein kinase II (CaMKII), a key protein that induces downstream signaling cascades mediating LTP expression, learning, and memory. CaMKII leads to phosphorylation of synaptic proteins, increase in the number of active AMPARs or their single-channel conductance (Park et al., 2021; Yasuda et al., 2022). During LTP induction, Ca2+ influx through GluN2B-NMDAR directly activates CaMKII and leads to synapse strengthening (Shipton and Paulsen, 2014). Experimental data shows that disruption of GluN2B-NMDAR/CaMKII interactions downregulates CaMKII activation and autophosphorylation, prevents LTP in hippocampus, and impairs spatial learning in transgenic mice (Zhou et al., 2007). GluN2B-NMDA type of receptor is crucial for normal learning in hippocampus in vivo (Li et al., 2007). Moreover, GluN2B-NMDAR subunit is implicated in variety of psychiatric disorders like dementia, Alzheimer's disease (Liu et al., 2019), and Schizophrenia (Kocsis, 2012). GluN2B-NMDAR plays a critical role in synaptic plasticity and cognitive impairment in Alzheimer's disease (Parameshwaran et al., 2008; Pousinha et al., 2017, 2019).
In modeling studies of synaptic plasticity, the challenge is to integrate knowledge at molecular, synaptic, neuronal, and microcircuit levels, to understand the underlying LTP and LTD mechanisms in synapses and transfer the knowledge to large network simulations. Numerous computational studies of synaptic plasticity exist, and the models can be grouped into three main classes that employ phenomenological, optimal, and biophysical approach. Phenomenological models are abstract, and encode data and intuitions about synaptic plasticity taking into account spike timing (Gerstner et al., 1996; Kempter et al., 1999; Kistler and van Hemmen, 2000; Song and Abbott, 2000; Song et al., 2000). Optimal models use some optimality criterion to deduce the rules of synaptic modifications (Toyoizumi et al., 2005; Pfister et al., 2006). Biophysical models rely on biologically realistic descriptions of the electrophysiological and biochemical processes, usually are based on intracellular calcium dynamics, and involve detailed biochemical reactions to explain synaptic plasticity (Bhalla and Iyengar, 1999; Senn et al., 2001; Shouval et al., 2002; Badoual et al., 2006; Graupner and Brunel, 2007; Pi and Lisman, 2008; Clopath et al., 2010; Urbanczik and Senn, 2014; Migliore et al., 2015; Saudargiene et al., 2015; Sacramento et al., 2018; Ebner et al., 2019; Mäki-Marttunen et al., 2020; Chindemi et al., 2022).
In biophysical models a widely used approach is to investigate the molecular networks underlying synaptic plasticity such as CaMKII and protein phosphatase competition activated by NMDAR-mediated calcium (Graupner and Brunel, 2007; Pi and Lisman, 2008; Saudargiene et al., 2015). A well-known calcium control hypothesis states that low calcium levels in dendritic spine do not evoke any changes, intermediate calcium levels depress the synapse and high calcium transients potentiate the synapse (Shouval et al., 2002). Biophysical models, embedded into detailed compartmental models, account for the factors shaping synaptic plasticity—different membrane mechanisms of the dendritic tree, dendritic integration, morphological features, pattern of pre- and postsynaptic spiking (Poirazi and Papoutsi, 2020). The models include complex biochemical reactions of calcium induced kinase and phosphatase activation that underlie synaptic modifications (Bhalla and Iyengar, 1999; Graupner and Brunel, 2007; Saudargiene et al., 2015; Jędrzejewska-Szmek et al., 2017; Mäki-Marttunen et al., 2020), represent molecular cascades applying simplified functions, dependent on postsynaptic NMDAR-mediated intracellular calcium transients (Shouval et al., 2002; Graupner and Brunel, 2012; Standage et al., 2014; Chindemi et al., 2022), use formulation on the level of postsynaptic voltage (Clopath et al., 2010; Meissner-Bernard et al., 2020), utilize a kinetic model of synapse upregulation and downregulation mediated by NMDAR and based on the precise timing of pre and post spikes (Senn et al., 2001), or describe the weight change in a phenomenological way taking into account spike timing (Gerstner et al., 1996; Song and Abbott, 2000; Song et al., 2000). Phenomenological models are efficient, but lack biological realism; on the other hand, detailed models, sensitive to NMDAR functioning, are not easily applied in network simulations as they include many complex biochemical reactions, large parameter space, and are computationally expensive. The models that account for the NMDAR subunit properties and are suitable to analyze learning properties in networks are still lacking.
Different forms of LTP and LTD coexist that have different induction and expression mechanisms. In this study we focus on the GluN2B-NMDAR subunit effect on LTP induction in STDP, high and low frequency stimulation protocols. The aim of this work is to build a phenomenological NMDAR-based synaptic plasticity model that separates the influence of GluN2A-NMDAR and GluN2B-NMDAR subunits, and gain insight into the GluNR2B-NMDAR effect on synaptic modifications of hippocampal CA3-CA1 synapses. We modeled synaptic plasticity induced by a STDP protocol and high and low frequency stimulation, and explored the impact of GluN2B-NMDAR subunit properties on the modification of synaptic strength of the synapses spatially distributed on the apical dendrites of CA1 pyramidal neuron. The novelty of the work is the approach to include the influence of the specific NMDA receptor subunits on synaptic plasticity as the separate mediators of LTP and LTD. We assume that LTP is mainly mediated by GluN2B-NMDAR (Morishita et al., 2007; Andrade-Talavera et al., 2016; Pousinha et al., 2017), and LTD is triggered by other mechanisms, possibly including GluN2A-NMDAR. Experimental studies showed that GluN2A-NMDAR blockade prevented LTD induction in the CA1 region of hippocampal slices (Bartlett et al., 2007; Li et al., 2007). The study of Morishita et al. (2007) also suggested that GluN2A-NMDAR might be responsible for LTD as the application of the GluN2B-NMDAR antagonist ifenprodil did not prevent the induction of LTD by low frequency stimulation.
We analyzed the effect of GluN2B-NMDAR on the properties of learning at the synapses of hippocampal CA1 pyramidal neuron. The modeling results provide insights into the learning rules of hippocampal CA1 pyramidal neuron synapses in healthy and GluN2B-NMDAR hypofunction conditions.
2. Methods
We developed a model of synaptic modifications based on the well-established phenomenological models (Clopath et al., 2010; Meissner-Bernard et al., 2020) and integrated the separated influence of postsynaptic NMDAR subunits GluN2A-NMDAR and GluN2B-NMDAR to account for the crucial effect of GluN2B-NMDAR in hippocampal synaptic plasticity. We utilized two computational models of CA1 pyramidal neuron: a modified two-compartmental Pinsky-Rinzel model (Pinsky and Rinzel, 1994; Ferguson and Campbell, 2009) to validate the extended synaptic plasticity model, and a multicompartmental model (Migliore et al., 2018) to study the influence of GluN2B-NMDAR properties on synaptic strength modifications at a cluster of CA3-CA1 synapses distributed randomly onto apical dendrites of CA1 neuron.
2.1. NMDAR-based voltage-dependent synaptic plasticity model
We extended a voltage-based model of synaptic plasticity (Clopath et al., 2010; Meissner-Bernard et al., 2020) by including the effect of postsynaptic NMDAR subunits GluN2A-NMDAR and GluN2B-NMDAR. The instantaneous weight change consists of two additive NMDAR-dependent LTD and LTP contributions, and , following Clopath et al. (2010) and Meissner-Bernard et al. (2020):
where wmax and wmin set the limits for synaptic weight w. The LTP component is expressed as the product of the NMDAR-dependent function ϕNMDA+(t) and a low-filtered membrane potential :
where A+ is the LTP amplitude parameter. Similarly, the LTD component is proportional to the product of the NMDAR-dependent function ϕNMDA−(t) and a low-filtered membrane potential :
where is a presynaptic activity variable, and A− is the LTD amplitude parameter.
The contribution of the postsynaptic GluN2A-NMDAR and GluN2B-NMDAR gated channel is captured by the newly introduced Hill function ϕNMDA[*](t), here [*] indicates the LTP and LTD components ([*] is [+] for LTP and [−] for LTD):
where is the filtered NMDAR conductance, Ka[*] is a value of , producing half activation of ϕNMDA[*](t), n[*] is the Hill coefficient, and θϕ[*] is a threshold of ϕNMDA[*](t) for LTP and LTD induction. Values of n[*], Ka[*], and θϕ[*] differ for the LTD and LTP contributions. Function ϕNMDA+(t) governs LTP induction and is caused by the filtered NMDAR conductance . Function ϕNMDA−(t) accounts for LTD, and is triggered by the filtered NMDAR conductance .
The moving threshold function θϕ[*] lowers ϕNMDA[*](t) activity and implements competition between LTP and LTD:
where τθϕ[*] is a time constant and bθϕ[*] is a scaling coefficient, and [*] denotes [−] for LTP and [+] for LTP components.
The moving threshold θϕ−(t) is increasing, if ϕNMDA+(t) is strongly activated and LTP is induced, thus vetoing LTD. Threshold θϕ+(t) may also increase if ϕNMDA−(t) accumulates, and leads to LTD.
The filtered NMDAR-dependent variables for LTP and LTD components are described:
where τNMDA[*] is a time constant, and gNMDA[*](t) is a conductance of postsynaptic NMDAR that incorporates both GluN2A-NMDAR and GluNB-NMDAR subunits with a different weighting coefficient k2B[*] for LTP and LTD components:
Following Morishita et al. (2007), Andrade-Talavera et al. (2016), and Pousinha et al. (2017), we assume that LTP is mainly governed by GluN2B-NMDAR subunit, and LTD is mediated by GluN2A-NMDAR (or other) subunit. We set the coefficient of GluN2B-NMDAR effect on LTP k2B+ = 0.8, and coefficient of GluN2B-NMDAR effect on LTD k2B− = 0.2.
GluN2B-NMDAR subunit has a slower inactivation time than GluN2A-NMDAR subunit. Kinetic parameters of forward and backward binding rates are adjusted (Cull-Candy et al., 2001).
Synaptic conductances of GluN2A-NMDAR and GluNB-NMDAR subunits are modeled following Destexhe et al. (1994):
where [GluN2†] denotes two types of NMDAR GluN2 subunits, GluN2A-NMDAR and GluN2B-NMDAR, Ron[GluN2†] and Roff[GluN2†] are the fraction of open and closed GluN2A-NMDAR and GluNB-NMDAR, ĝNMDA[GluN2†] is the maximal GluN2A-NMDAR and GluN2B-NMDAR conductances, and is a NMDAR gating function, dependent of extracellular magnesium concentration [Mg2+] and local membrane potential V(t):
Ron[GluN2†], Roff[GluN2†], and Rinf[GluN2†] of GluN2A-NMDAR and GluNB-NMDAR are equal:
and
where α[GluN2†] and β[GluN2†] are forward and backward binding rates of GluN2A-NMDAR and GluN2B-NMDAR, adjusted following (Cull-Candy et al., 2001).
Time constant τ[GluN2†] is defined:
Variables and are the functions of the filtered membrane potential V(t) at the synapse location, and contribute to the LTD and LTP components:
where τ[*] is a time constant and θ[*] is a threshold for the LTP and LTD components.
A presynaptic activity variable in Equation (3) is calculated as a low pass filter of the presynaptic spike train Σiδ(t−ti) with time constant τδ using .
Schematic representation of synaptic plasticity model is shown in Figure 1. The presynaptic activity triggers NMDAR synaptic conductance gNMDA(t), composed of GluN2A-NMDAR and GluN2B-NMDAR subunits, and induces variables ϕNMDA+(t) and ϕNMDA−(t). Once activated, the LTP variable ϕNMDA+(t) inhibits the LTD variable ϕNMDA−(t) preventing LTD induction, and vice versa—ϕNMDA−(t) may reduce the activity of ϕNMDA+(t). Postsynaptic activity is captured by a local membrane potential V(t) that is thresholded using the thresholds θ+ and θ−, and low-pass filtered resulting in and . The LTP component is obtained by multiplying V+(t) and ϕNMDA+(t), and the LTD component is a product of V−(t), ϕNMDA−(t), and . The weight change of the AMPAR strength w is composed of the scaled LTP and LTD parts and .
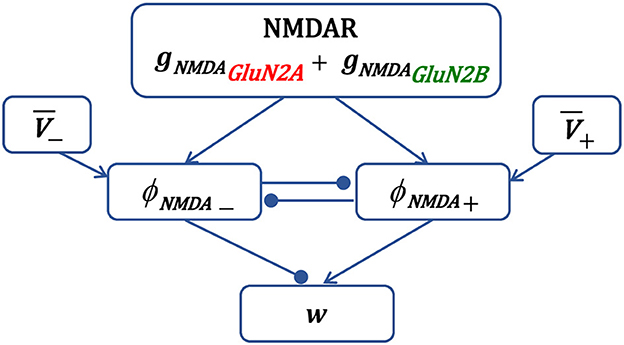
Figure 1. Schematic diagram of the main components of synaptic plasticity model. Presynaptic action potential activates NMDAR, induces the NMDAR conductance, composed of GluN2A-NMDAR and GluN2B-NMDAR subunits gNMDAGluN2A and gNMDAGluN2B, and triggers variables ϕNMDA+ and ϕNMDA− that account for the LTP and LTD contribution, respectively. The LTP variable ϕNMDA+, once activated, inhibits the LTD variable ϕNMDA− to prevent LTD, and vice versa. Postsynaptic local membrane potential V is low-pass filtered, and the resulting LTP and LTD variables and are multiplied by the corresponding NMDAR-dependent variables ϕNMDA+ and ϕNMDA− to form the LTP and LTD components of the weight w.
The novelty of the model is that it captures the separate specific influence of GluN2A-NMDAR and GluN2B-NMDAR subunits on LTP and LTD induction using the NMDAR subunit-dependent activation functions ϕNMDA−(t) and ϕNMDA+(t) (Equation 4).
The GluN2A-NMDAR and GluN2B-NMDAR subunit-dependent functions (Equations 4, 6, 7) shape the LTP and LTD components (Equations 2, 3). The filtered NMDAR synaptic conductance-dependent variables (Equation 6) can be interpreted as the intracellular Ca2+ concentration, and functions ϕNMDA[*](t) (Equation 4) reflect the activation of intracellular Ca2+-triggered second messenger cascades underlying synaptic plasticity induction. Specifically, ϕNMDA+(t) may represent phosphorylation of CaMKII, leading to LTP, and ϕNMDA−(t) may indicate dephosphorylation of protein phosphatase 2B (PP2B, calcineurin), responsible for LTD. The description of the signaling pathways is simplified and implemented in a phenomenological manner using the NMDAR-dependent functions. The model does not require the estimation of the intracellular calcium concentration at a postsynaptic site and relies on the NMDAR properties and local membrane potential.
The parameters of the synaptic plasticity model are given in Table 1. The parameters of the GluN2A-NMDAR and GluN2B-NMDAR synaptic conductances are presented in Table 2.
2.2. Computational models of CA1 pyramidal neuron
We employed two computational models of CA1 pyramidal neuron: a modified two-compartmental Pinsky-Rinzel model for synaptic plasticity model validation (Pinsky and Rinzel, 1994; Ferguson and Campbell, 2009) and a compartmental detailed model (Migliore et al., 2018) for analysis of GluN2B-NMDAR-dependent synaptic plasticity properties at CA3-CA1 synapses distributed on the apical dendrites of CA1 pyramidal neuron in the stratum radiatum (SR) region.
2.2.1. Two-compartmental model of CA1 pyramidal neuron
A two-compartmental Pinsky-Rinzel model consisted of a somatic and dendritic compartments connected by the coupling conductance (Pinsky and Rinzel, 1994; Ferguson and Campbell, 2009). The somatic compartment had five ionic current channels: inward Na+ current INa,s, outward delayed rectifier K+ current IKDR,s, inward Ca2+ current ICa,s, outward short-duration voltage and Ca2+- dependent K+ current IKCa,s, and outward long-duration Ca2+-dependent after hyperpolarization (AHP) K+ current IKAHP,s. The dendritic compartment had three ionic current channels: inward Ca2+ current ICa,d, outward short-duration voltage and Ca2+-dependent K+ current IKCa,d, outward long-duration Ca2+-dependent AHP potassium current IKAHP,d. Both compartments had leak current Ileak,s, Ileak,d. A single synapse containing AMPAR and GluN2A-NMDAR/GluN2B-NMDAR was formed on the dendritic compartment. The two-compartment model and synaptic currents are described in Supplementary material. The partial blockade of GluN2B-NMDAR-gated channel was simulated by reducing the conductance gNMDAGluN2B (Equation 7).
2.2.2. Multicompartmental model of CA1 pyramidal neuron
A multicompartmental model of a CA1 pyramidal cell oh140807_A0_idA (Migliore et al., 2018) consisting of 175 compartments was used, and it included 11 ionic current channels and a leak current. The ionic currents were the following: inward Na+ current INa; four types of K+ currents: outward delayed rectifier K+ current IKDR, transient A-type K+ current IKA, currents IKM, IKD; three types of inward Ca2+ currents: N-type current ICaN, L-type current ICaL, T-type current ICaT; two types of Ca2+-dependent K+ currents: outward short-duration voltage and Ca2+-dependent K+ current IKCa and ICagk current; and the non-specific Ih current. Ionic channels were uniformly distributed in all dendritic compartments except IKA and Ih, which increased with distance from the soma. Channels were described using a conventional Hodgkin-Huxley formalism, and peak conductances of each channel were optimized for soma, axon, basal, and apical dendrite compartments and validated against experimental data. Intracellular calcium concentration was described by a simple Ca2+ extrusion mechanism with a single exponential decay. The multicompartmental model and synaptic currents are described in Migliore et al. (2018).
A cluster of 50 AMPARs and GluN2A/GluN2B-NMDARs containing synapses, distributed randomly on the apical dendrites of the neuron in the SR region at 140 μm from the soma with a synaptic density of 0.8 synapses/μm of dendrite (Gasparini et al., 2004; Bezaire et al., 2016) was formed to model synaptic modifications.
The ratio of AMPAR/NMDAR gated channel currents was replicated using the experimental protocol used in (Pousinha et al., 2017). The voltage was clamped at -65 mV for AMPAR gated channel current estimation, and at +40 mV for NMDAR gated channel assessment. The peak AMPAR current was compared with the NMDAR current 60 ms after the onset of stimulus. The maximal conductances of the AMPAR and NMDAR-gated channels were set to ensure this ratio to be equal to 4 as in Pousinha et al. (2017). The partial blockade of GluN2B-NMDAR-gated channel was simulated by lowering the conductance gNMDAGluN2B (Equation 7).
2.3. Stimulation protocols for synaptic plasticity induction at CA3-CA1 synapses
Synapses were stimulated using the activation patterns applied in the following electrophysiological studies of synaptic plasticity:
• STDP induction protocol (Wittenberg and Wang, 2006; Inglebert et al., 2020). Presynaptic input was paired with a doublet of postsynaptic action potentials 60 times at 5 Hz; 5 times at 5 Hz frequency; and 30 times at 1 Hz. Temporal difference ΔT was measured between pre- and a second postsynaptic spike. In addition, a presynaptic spike was paired with a single postsynaptic action potential 60 times at 5 Hz. Temporal difference ΔT was measured between a pre- and a postsynaptic spike (Wittenberg and Wang, 2006). Pairing frequency was increased from 1 to 50 Hz for spike pairs with a temporal difference ΔT = 10 ms between a pre- and a single postsynaptic spike. The number of postsynaptic spikes was varied from one to four with a temporal difference ΔT = 10 ms between pre- and the first postsynaptic spike (Inglebert et al., 2020). The spike pairings were repeated 30 times. Somatic action potential was induced by current pulse injection into the soma.
• Frequency-dependent synaptic plasticity induction protocol (Pousinha et al., 2017). Presynaptic input was stimulated at 100 Hz for 1 s (LTP protocol) or at 1 Hz for 100 s (LTD protocol). To estimate the change in the excitatory postsynaptic potential (EPSP), a presynaptic stimulus was delivered before and after the conditioning stimulation, and the resulting ratio between the maximal values of the resulting EPSPs was calculated.
Simulations were performed in the Python and NEURON simulation environment (version 8.0.0) (Hines and Carnevale, 1997). All model files in Python are available for public download under the ModelDB section of the Senselab database, accession number 267680 (https://senselab.med.yale.edu/ModelDB/).
3. Results
3.1. Validation of synaptic plasticity model
The developed synaptic plasticity model was validated against experimental data (Wittenberg and Wang, 2006; Pousinha et al., 2017; Inglebert et al., 2020).
We used a two-compartment neuron model with a single synapse on its dendrite, and applied the STDP induction protocol by pairing presynaptic activity with a doublet of postsynaptic action potentials (Wittenberg and Wang, 2006) (Figure 2). For pre-post stimulation protocol and ΔT = 10 ms, the presynaptic activation precedes a second action potential (Figure 2A1, blue triangle and black line, respectively) and results in opening of GluN2A-NMDAR gNMDAGluN2A (red line) and GluN2B-NMDAR gNMDAGluN2B (green line) (Figure 2A2); filtered NMDAR-dependent variables (red) and (green) for LTD and LTP induction (Figure 2A3) favor activation of the LTP function ϕNMDA+ (Figure 2A4, green line). The product of ϕNMDA+ and forms and leads to the increased weight w (Figure 2A5). For post-pre stimulation protocol and ΔT = −10 ms, the presynaptic activation follows a second somatic action potential (Figure 2B1, blue triangle and black line, respectively), NMDAR activation is weaker (Figure 2B2), failing to sufficiently activate ϕNMDA+, but strong enough to induce ϕNMDA− (Figure 2B4, green and red lines, respectively). Functions ϕNMDA−, , and combine into and result in the decreased weight w (Figure 2B5).
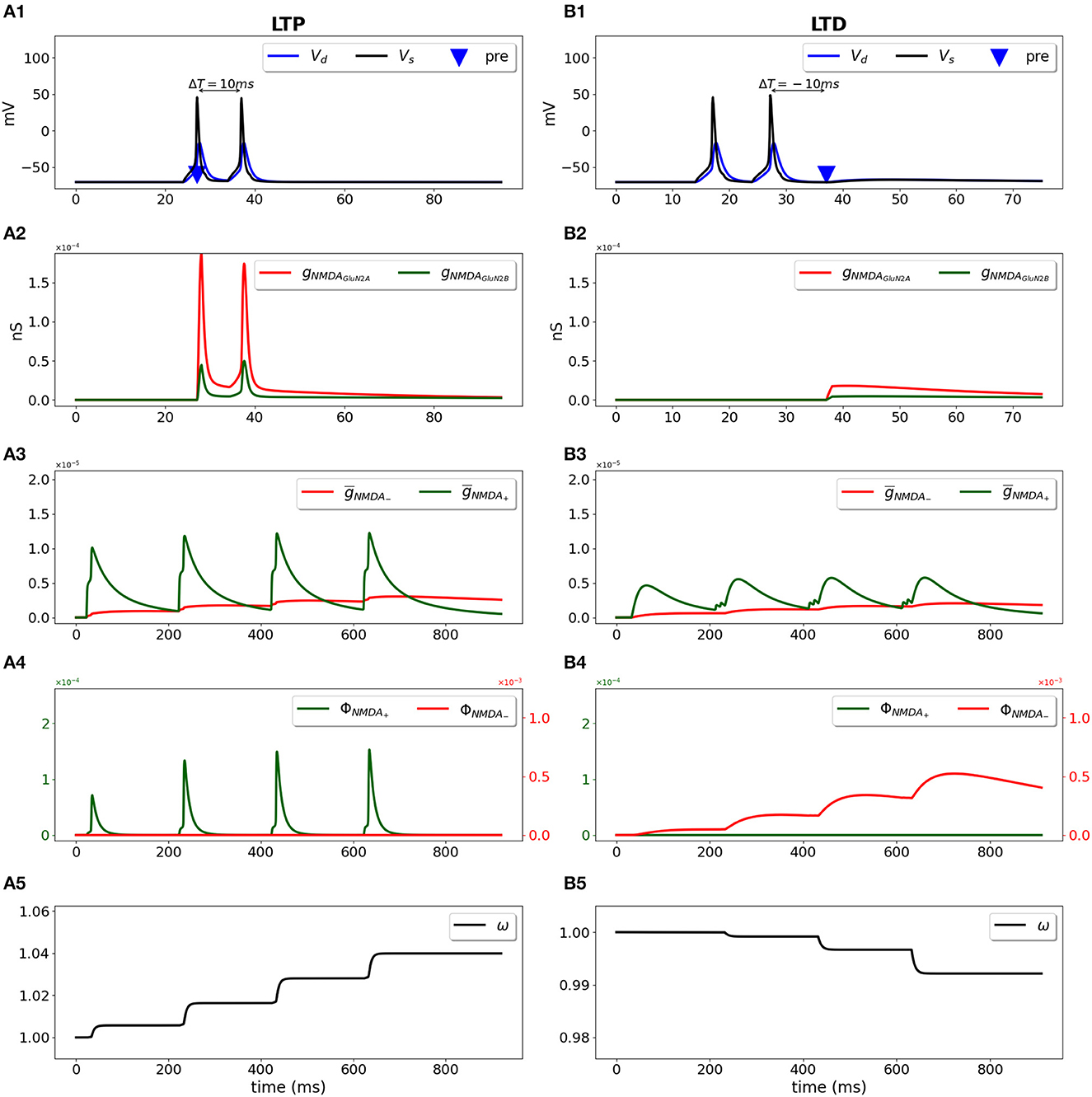
Figure 2. Synaptic weight change for the STDP induction protocol using a two-compartment model of CA1 pyramidal neuron. Presynaptic input was paired with a doublet of postsynaptic action potentials with the temporal difference between pre- and a second postsynaptic activity ΔT = 10 ms (A1–A5) and ΔT = -10 ms (B1–B5). (A1, B1) Membrane potential in soma Vs (black line) and dendrite Vd (blue line); presynaptic input is indicated by a blue triangle; (A2, B2) GluN2A-NMDAR and GluN2B-NMDAR conductances gNMDAGluN2A (red line) and gNMDAGluN2B (green line); (A3, B3) filtered NMDAR-dependent variables (red) and (green) for LTD and LTP; (A4, B4) NMDAR-dependent functions ϕNMDA− (red line) and ϕNMDA+ (green line) for the LTD and LTP components; (A5, B5) synaptic weight w.
The STDP curve of the weight change Δw induced by pairing the presynaptic input with a doublet of postsynaptic action potential 60 times at 5 Hz frequency with temporal difference ΔT ∈ [-100; 100 ms] is presented in Figure 3A. For the positive ΔT window from 0 ms up to 40 ms a synapse undergoes LTP, and LTD is obtained for anti-causal pairings and causal pairings within the ΔT interval [40; 100 ms]. Shorter stimulation of five pairings at 5 Hz results in a potentiation-only plasticity rule (Figure 3B) as the duration is not sufficient for the accumulation of the LTD mechanisms activity (ϕNMDA− in our model). Pairings at low frequency of 1 Hz results in LTD only (Figure 3C). A single postsynaptic action potential paired with a presynaptic action potential 60 times at 5 Hz evokes LTD, as the activation of LTP variable is too weak (ϕNMDA+ in our model, Figure 3D). The modeled STDP weight modifications replicate the experimental data (Wittenberg and Wang, 2006).
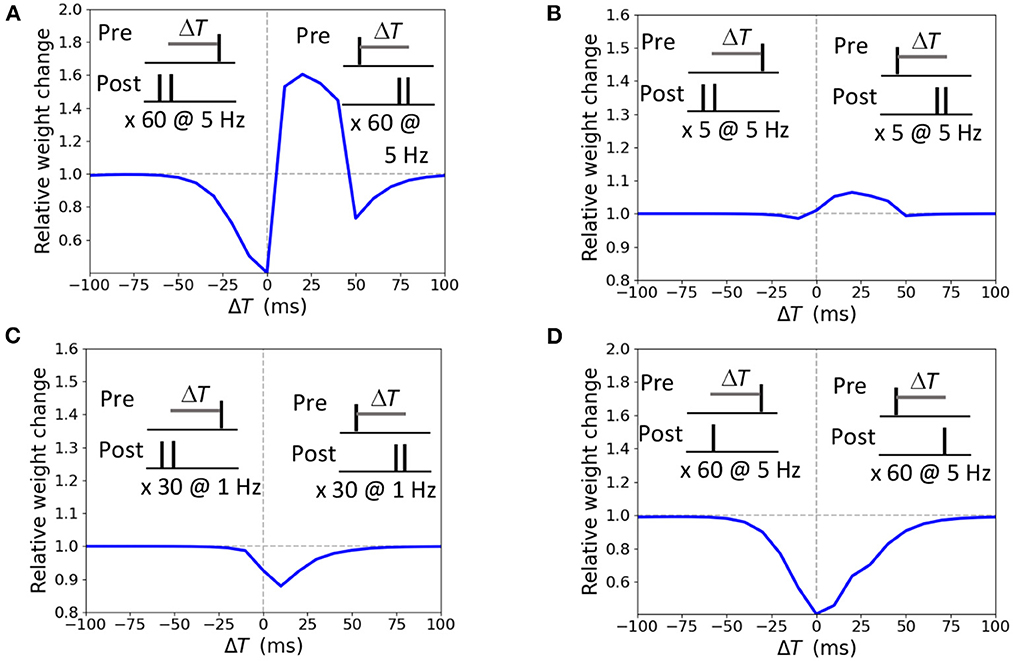
Figure 3. Synaptic modifications induced by pairing a presynaptic action potential with a doublet (A–C) or a single postsynaptic action potential (D). Temporal difference ΔT is measured between the presynaptic and a second postsynaptic action potential (A–C) or a single postsynaptic action potential (D). (A) 60 pairings at 5 Hz lead to LTP and two LTD windows; (B) 5 pairings at 5 Hz induce LTP; (C) 30 pairings at 1 Hz triggers LTD; (D) 60 pairings at 5 Hz results in LTD.
We investigated the weight change dependence on frequency of postsynaptic pairings and a number of postsynaptic spikes. When a presynaptic action potential was paired with a single postsynaptic action potential at ΔT = 10 ms, the synapse underwent LTD for very low repetition frequencies and switched to LTP for the increasing frequency above 5 Hz (Figure 4A). A single postsynaptic spike, paired with the input activity at ΔT = 10 ms, induced LTD, while two, three, and four postsynaptic spikes led to LTP (Figure 4B). The results qualitatively reproduces the experimental observations on LTP recovery with increasing pairing frequency and postsynaptic spike number (Inglebert et al., 2020).
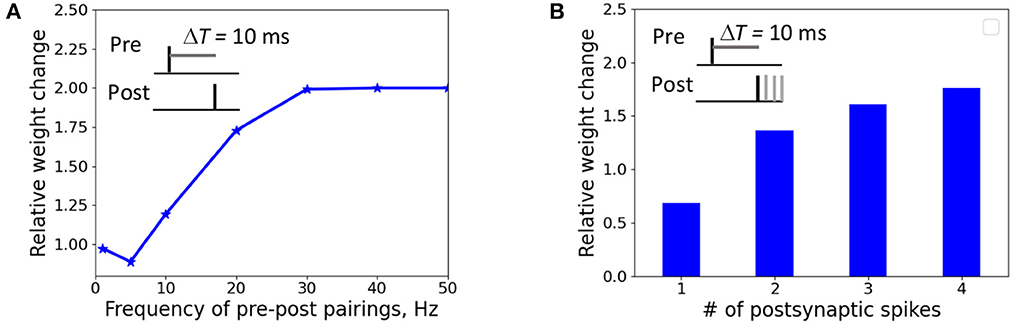
Figure 4. Weight change dependence on frequency of postsynaptic pairings (A) and a number of postsynaptic spikes (B). (A) A presynaptic action potential was paired with a single postsynaptic action potential 20 times at ΔT = 10 ms. (A) A presynaptic action potential was paired with a one to four postsynaptic action potentials 30 times at 5 Hz at ΔT = 10 ms.
Next, we applied the frequency-dependent stimulation protocol using the same two-compartment model of CA1 pyramidal neuron with a single synapse (Figure 5). In subthreshold regime (Figures 5A1–A5), stimulation of presynaptic input at 100 Hz resulted in opening of Glu2NA-NMDAR and Glu2NB-NMDAR channels (Figure 5A2), activation of ϕNMDA+ and inhibition of ϕNMDA− (Figures 5A3, A4, green and red lines respectively), and increase in weight w (Figure 5A5). In suprathreshold regime (Figures 5B1–B5), the same protocol led to the generation of somatic action potentials (Figure 5B1, black line), high activity of NMDAR channels, strong increase in ϕNMDA+, and inhibition of ϕNMDA− (Figure 5B4) causing strong LTP (Figure 5B5). Low frequency stimulation at 1 Hz only slightly opened Glu2NA-NMDAR and Glu2NB-NMDAR channels (Figure 5C2) that was sufficient to activate ϕNMDA−, but not ϕNMDA+ (Figure 5C4, red line) and induce LTD (Figure 5C5). The results indicate that the model is suitable to account for the synaptic changes using frequency dependent LTD and LTP protocols in subthreshold and suprathreshold regimes.
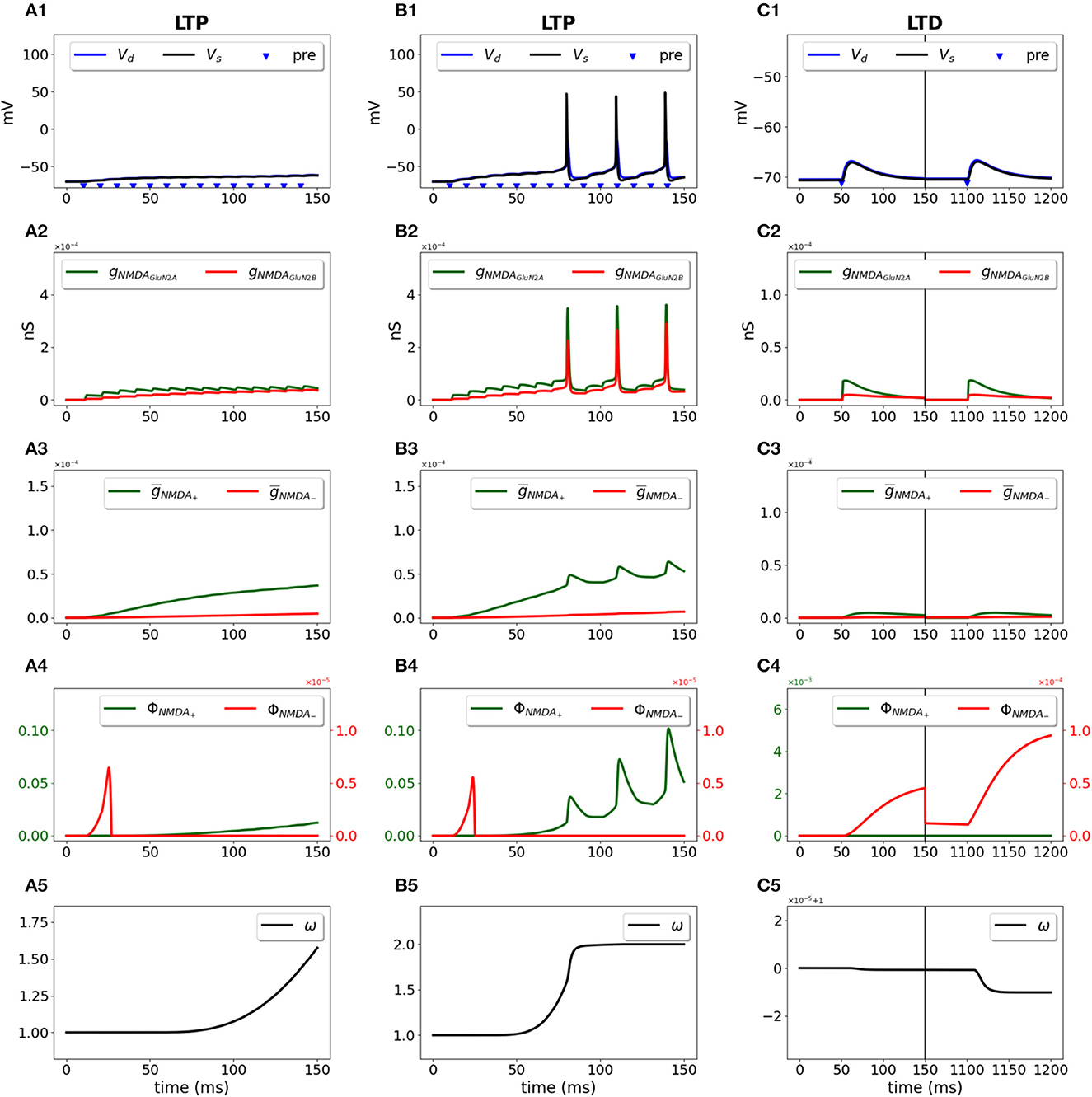
Figure 5. Synaptic weight change for the frequency-dependent subthreshold LTP (A1–A5), suprathreshold LTP (B1–B5), and LTD (C1–C5) protocols using a two-compartment model of CA1 pyramidal neuron. Presynaptic input was stimulated at 100 Hz for 1 s (LTP protocol) or at 1 Hz for 100 s (LTD protocol). (A1–C1) Membrane potential in soma Vs (black line) and dendrite Vd (blue line); presynaptic activity is indicated by blue triangles; (A2–C2) GluN2A-NMDAR and GluN2B-NMDAR conductances gNMDAGluN2A (red line) and gNMDAGluN2B (green line); (A3–C3) filtered NMDAR-dependent variables (red) and (green) for LTD and LTP components; (A4–C4) NMDAR-dependent functions ϕNMDA+ (green line) and ϕNMDA− (red line) for the LTP and LTD components; (A5–C5) Synaptic weight w.
The model validation analysis showed that the NMDAR-dependent synaptic plasticity model was capable of reproducing the experimental STDP weight change curves for different frequencies and postsynaptic patterns, high-frequency, and low-frequency stimulation protocols.
3.2. Synaptic plasticity depends on GluN2B-NMDAR properties in a synapse cluster on a CA1 pyramidal neuron
We employed the developed model of synaptic plasticity and a compartmental detailed model of a CA1 pyramidal neuron (Migliore et al., 2018) to analyze the dependence of synaptic modifications on the GluN2B-NMDAR functioning using STDP and frequency-dependent stimulation protocols. We modeled weight modifications at clustered synapses on the apical branches of CA1 pyramidal neuron and measured EPSP change in soma before and after the stimulation protocol. Experimental data and computational modeling studies suggest that synapses tend to form tightly-packed groups or clusters on the dendrites of neurons [for review see Kastellakis and Poirazi (2019) and Miry et al. (2021)]. Such nearly-synchronous activated inputs carry similar information onto the same dendrite and enable emerging of memory engrams. Thus, we formed a cluster of 50 effectively activated AMPAR and GluN2A-NMDAR/GluN2B-NMDAR containing synapses on the apical dendrites of CA1 pyramidal neuron.
During stimulation, each synapse on the dendritic branch developed its weight depending on the NMDAR-gated synaptic conductance function (Equation 4) that sensed local depolarization and presynaptic glutamate release. Figure 6 shows the evolvement of 50 synaptic weights in a cluster during LTP and LTD stimulation protocols.
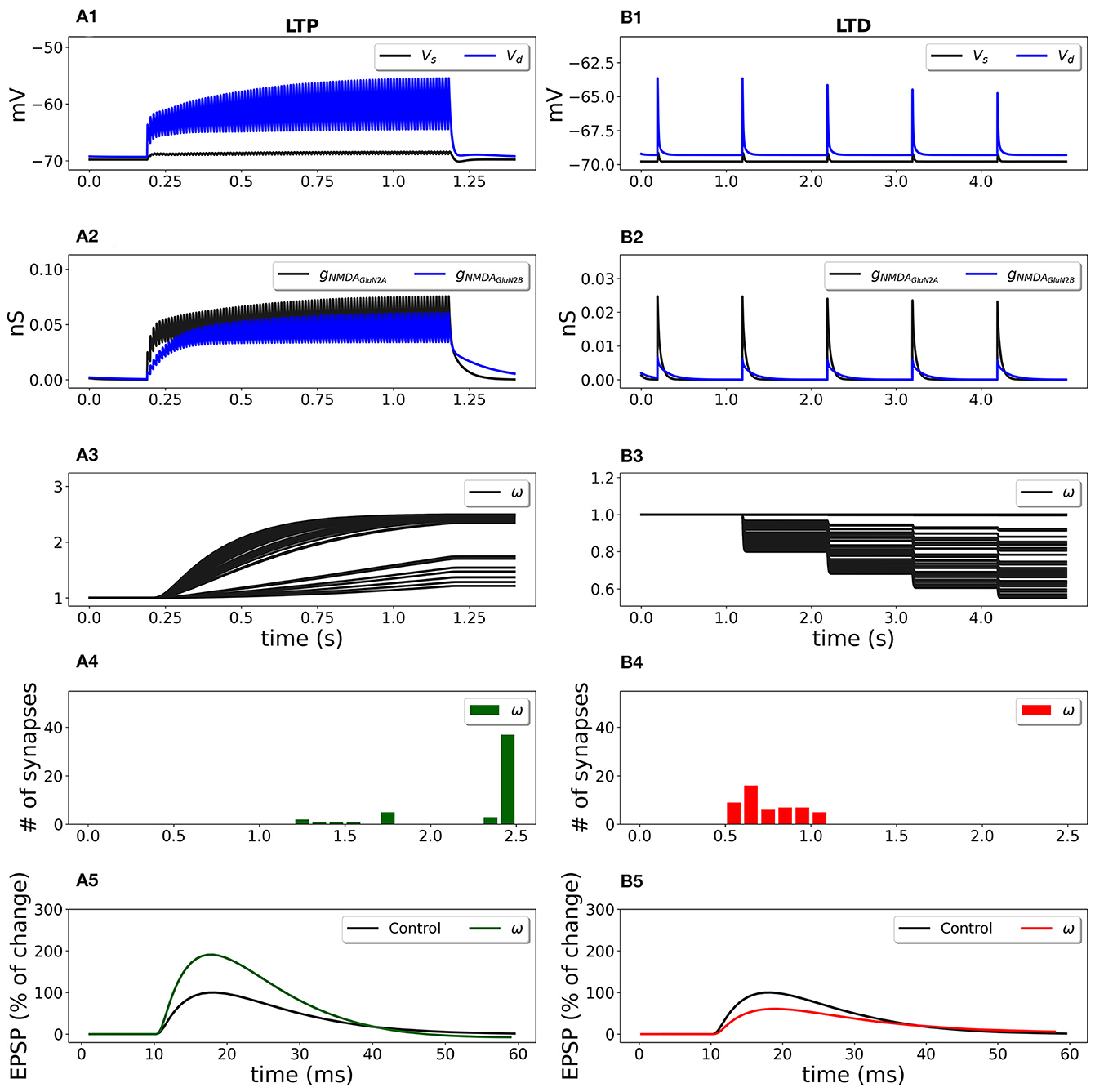
Figure 6. Synaptic weight change for the frequency-dependent LTP (A1–A5) and LTD (B1–B5) protocols using a multicompartmental model of CA1 pyramidal neuron. Fifty synapses were randomly distributed on the SR apical dendritic branches with the density of 0.8 synapses/μm of dendrite 140 μm from soma and stimulated at 100 Hz for 1 s (LTP protocol) and at 1 Hz for 100 s (LTD protocol). (A1, B1) Membrane potential in soma Vs (black line) and membrane potential Vd in dendrite (blue line) at a randomly selected synapse location; (A2, B2) GluN2A-NMDAR and GluN2B-NMDAR conductances gNMDAGluN2A (black line) and gNMDAGluN2B (blue line); (A3, B3) synaptic weights w of 50 synapses; (A4, B4) distribution of final synaptic weights w; (A5) normalized somatic EPSP before (black line) and after (green line) the synaptic plasticity induction protocol; LTP was induced with the EPSP change of 190%; (B5) normalized somatic EPSP before (black line) and after (red line) the synaptic plasticity induction protocol; LTD was triggered with the EPSP change of 61%.
High frequency presynaptic stimulation at 100 Hz for 1 s depolarized membrane potential (Figure 6A1; blue line—membrane potential Vd at a location of a randomly chosen synapse; black line—membrane potential in soma Vs) and activated GluN2A-NMDAR and GluN2B-NMDAR channels gNMDAGluN2A and gNMDAGluN2B (Figure 6A2, black and blue lines, respectively) that led to the increase of synaptic weights (Figure 6A3). The histograms of the synaptic weights (Figure 6A4) shows that the weights distributed in the interval from 1 up to the predefined maximum value wmax = 2.5. Some synapses were only slightly potentiated due to the low local membrane potential and weakly activated NMDAR. The EPSP increased by 190% if compared to the EPSP before the conditioning stimulation (Figure 6A5, green line vs. black line). Low-frequency stimulation at 1 Hz for 100 s induced a small membrane depolarization at the synapse location (Figure 6B1, red line), weak NMDAR activation (Figure 6B2), and resulted in the weakening of synaptic strength of all synapses (Figures 6B3, B4). After the stimulation, the somatic EPSP decreased to 61% (Figure 6B5).
The synaptic plasticity model embedded into a detailed model of a CA1 pyramidal cell qualitatively reproduced the experimental results. Experimental data showed that 500 pulses at 1 Hz induced 57% LTD and 100 pulses at 100 Hz led to 191% LTP in hippocampal CA1 pyramidal neurons in rats (Pousinha et al., 2017).
We investigated the influence of the partial and full blockade of GluN2B-NMDAR on synaptic plasticity outcome using frequency-dependent stimulation and STDP protocols (Figure 7). First, presynaptic input was stimulated at 100 Hz for 1 s (LTP protocol), and the normalized EPSP to the pre-LTP baseline value was estimated. The blockade of the GluN2B-NMDAR synaptic conductance, leaving 0.3 fraction its active baseline value, resulted in the decrease of LTP from 190% to 164%, while the full blockade of the GluN2B-NMDAR led to LTD, the decrease to 90% of somatic EPSP. The impairment of GluN2B-NMDAR did not affect LTD leaving it to 63% (Figure 7A). The model of synaptic plasticity qualitatively reproduced experimental data of GluN2B-NMDAR inhibitor ifenprodil effect on LTP (Pousinha et al., 2017). It was shown that ifenprodil dose-dependently inhibited LTP, evoked by high frequency stimulation in CA3-CA1 synapses. The maximal inhibition efficacy was observed with the increasing ifenprodil concentration of 5 μm that converted 190% LTP to 75% LTD, but it did not affect LTD. The results show that the synaptic plasticity model can capture the influence of GluN2B-NMDAR properties at a single synapse by decreasing the synaptic weight changes in response to the impaired GluN2B-NMDAR functioning, and quantitatively follows the experimental data (Pousinha et al., 2017).
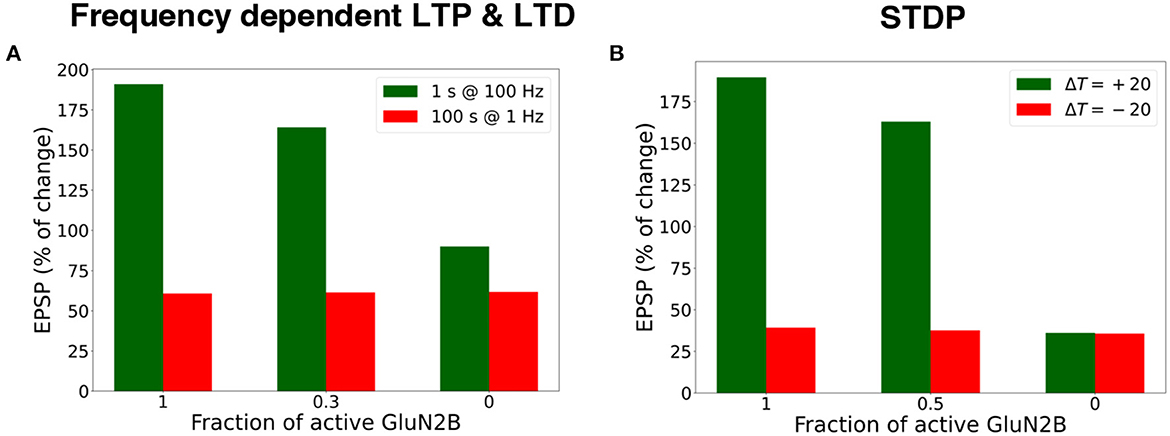
Figure 7. Blockade of GluN2B-NMDAR prevents LTP for high-frequency and STDP stimulation protocols. (A) Presynaptic input was stimulated at 100 Hz for 1 s (LTP protocol, green bars) and at 1 Hz for 100 s (LTD protocol, red bars). LTP was impaired, and LTD remained intact. (B) Presynaptic activity was paired with a doublet of postsynaptic action potentials 60 times at 5 Hz frequency with a temporal difference ΔT = +20 ms (LTP protocol, green bars) and ΔT = -20 ms (LTD protocol, red bars). LTP was abolished, and LTD was preserved.
Next, we applied the STDP stimulation protocol with the temporal difference ΔT = ± 20 ms between a presynaptic activity and a second postsynaptic spike (Figure 7B). GluN2B-NMDAR blockade led to the LTP switch into LTD for pre-post pairings and left LTD intact.
The results illustrate that synaptic plasticity is strongly affected by Glu2NB-NMDAR subunit properties. Hypofunction of GluN2B-NMDAR abolishes LTP induction and does not affect LTD for STDP protocol. For high frequency stimulation, LTP switches to LTD, and leaves LTD intact for low frequency stimulation. The results quantitatively align well with the experimental evidence on Glu2NB-NMDAR importance in shaping LTP at hippocampal synapse (Morishita et al., 2007; Andrade-Talavera et al., 2016; Pousinha et al., 2017). GluN2B-NMDAR may act as an additional modulatory mechanism of synaptic plasticity, and further experimental and computational studies are needed to understand the importance of the NMDAR subunit composition, and its effect on synaptic plasticity.
4. Discussion
We developed an NMDAR subunit-dependent voltage-based synaptic plasticity model of synaptic weight modifications at hippocampal CA3-CA1 synapses. We extended the computational model of STDP (Clopath et al., 2010; Meissner-Bernard et al., 2020) by simultaneously incorporating the GluN2A-NMDAR and GluN2B-NMDAR components to account for the specific functions of NMDAR subunits in synaptic learning. The model of synaptic plasticity was validated against the experimental data (Wittenberg and Wang, 2006; Pousinha et al., 2017; Inglebert et al., 2020) and reproduced STDP and frequency dependent LTP and LTD. Furthermore, the results show that this synaptic plasticity model is able to account for the impairment of LTP in GluN2B-NMDAR hypofunction conditions as in experimental studies (Pousinha et al., 2017), demonstrating that synaptic plasticity depends on GluN2B-NMDAR properties, and dysfunction of GluN2B-NMDAR leads to LTP impairment and its transformation to LTD.
The developed model can be interpreted as a phenomenological model, standing at the intersection with the class of biophysical models of synaptic plasticity. The model captures the influence of the GluN2B-NMDAR subunit on synaptic modifications. Usually, the calcium-based models of synaptic plasticity do not distinguish between the NMDAR subunits as mediators of calcium influx. Our approach enriches the model with the new features of specific NMDAR effect on synaptic plasticity.
Our model uses the functions of the NMDAR subunit dynamics that in an abstract form accounts for the CaMKII and phosphatase activation, does not require to model dendritic spines and estimate intracellular calcium concentration, a main trigger of synaptic plasticity. The formalism proposed captures synapse-specific mechanisms that define synaptic plasticity—the local non-linear activation of NMDAR, its subunit composition and functioning.
We chose the voltage-based approach as the voltage traces are conventionally recorded in the experimental setups, well-described by the mathematical formalism, enabling the model to be usable for future network level simulations. The level of modeling may also be more detailed and rely on intracellular calcium dynamics, as in e.g., Shouval et al. (2002) and Graupner and Brunel (2012), while focusing on the NMDAR subunit effects on LTP and LTD induction.
We explored the impact of the NMDAR properties on the somatic EPSP changes using a biologically realistic multicompartmental CA1 pyramidal neuron and taking into account the influence of the spatial distribution of the synapses. The results indicate that GluN2B-NMDAR regulates the amount of synaptic strength on the dendritic tree and the resulting EPSP changes in soma. The hypofunction of GluN2B-NMDAR leads to the impairment of LTP and gradual switch to LTD. The study extends the experimental observations of Pousinha et al. (2017) and predicts the pattern of the GluN2B-NMDAR functioning-mediated synaptic plasticity measured as changes in somatic EPSP for specific synapse cluster for STDP induction protocol. In detailed biophysical modeling studies, long-term synaptic plasticity depends on intracellular calcium influx, but the sources of calcium is usually not taken into consideration. Here, we discuss the importance of considering the mediating role of Glu2NB-NMDAR to study LTP in STDP and frequency dependent synaptic plasticity. As NMDARs can undergo activity-dependent long-term plasticity (Hunt and Castillo, 2012), this work shows the importance to consider the state of NMDARs, not only in the modeling studies of learning and memory, but also in physiological experiments. The model offers a possibility to include GluN2B-NMDAR effective contribution to the synaptic weight modifications. The incorporation of the separated influence of the GluN2A-NMDAR and GluN2B-NMDAR functioning makes the model a candidate to explore learning in the diseased brain, as the Glu2NB-NMDAR normal functioning is crucial for healthy CA3-CA1 synapses, and its dysfunction is observed in cognitive deficits in neurological diseases (Kocsis, 2012; Pousinha et al., 2017, 2019; Adell, 2020).
The model of synaptic plasticity is principally based on the critical role of postsynaptic NMDAR in LTP and LTD induction in adult CA3-CA1 synapses. GluN2A-NMDAR and GluN2B-NMDAR subunits mediate some forms of LTP and LTD at CA3-CA1 synapses (Paoletti et al., 2013). Experimental evidence suggests that GluN2B-NMDAR subunits are critical for LTP, but not necessary for LTD (Weitlauf et al., 2005; Bartlett et al., 2007). GluN2A-NMDAR blockade prevented LTD induction in the CA1 region of hippocampal slices (Bartlett et al., 2007; Li et al., 2007). However, other studies found that loss of GluN2B-NMDAR prevented LTD (Brigman et al., 2010), and GluN2A-NMDAR is not necessary for LTD (Gerkin et al., 2007; Li et al., 2007; Ge et al., 2010). Studies on GluN2 subunit composition for LTD have been inconsistent and conflicting, likely due to the problematic GluN2 subunit-selective pharmacology (Neyton and Paoletti, 2006; Shipton and Paulsen, 2014; Wong and Gray, 2018; Franchini et al., 2020). Sometimes seemingly the contradicting experimental data of synaptic plasticity outcomes depend on the developmental stage of the animal, extracellular or intracellular solution compositions, and other variables linked to the different experimental settings. In general, it is hypothesized that the GluN2A-to-GluN2B ratio defines the magnitude and sign of frequency-induced synaptic plasticity and shifts the LTP and LTD threshold. Higher GluN2A-to-GluN2B ratio requires stronger stimulation to induce LTP, confirming the critical role of GluN2B in LTP (Paoletti et al., 2013).
Recent experimental evidence shows that synaptic plasticity has different induction mechanisms depending on the NMDAR position (pre- or post-synaptic) and subunit composition, developmental stage of animal, or experimental settings including the type of protocol used. For example, presynaptic NMDARs at the CA3-CA1 synapse can mediate a pre-synaptic form of STDP LTD (t-LTD) in young mice (P13-021) (Andrade-Talavera et al., 2016). This t-LTD is lost in adult synapses when applying the same post-pre protocol and even converts LTP in adult animals not requiring NMDARs anymore (Pérez-Rodríguez et al., 2019; Falcón-Moya et al., 2020). Such diversity in mechanisms of synapse plasticity, even within one type of synapse, shows the limitation of the synaptic plasticity model proposed and indicates the need to extend the study by including other mechanisms such as the involvement of presynaptic NMDAR, group I metabotropic glutamate receptor (mGluR), astrocytic signaling.
The limitation of this study is the phenomenological nature of the synaptic plasticity model that relies on the NMDAR subunit-dependent synaptic plasticity functions, but does not include detailed molecular pathways of the possible LTP and LTD induction mechanisms such as protein kinase A (PKA), CaMKII, protein phosphatase 2A and 2B (PP2A, PP2B) activation and competition. The potential direction of synaptic plasticity studies is the extension of the detailed biophysical models to account for the influence of the postsynaptic NMDAR subunit effects on the biochemical pathways of CaMKII, PKA, PP2A/2B activation in LTP and LTD induction. On the other hand, the model allows reducing the complexity of the description of the molecular network underlying LTP and LTD. We were also confronted with sometimes seemingly contradicting experimental data of synaptic outcomes that probably depend on the developmental stage of the animal, extracellular or intracellular solution compositions, and other variables linked to the different experimental settings. For example, pre-post pairings of synaptic activity lead to LTD for 5 Hz stimulation (Wittenberg and Wang, 2006), but to LTP in Inglebert et al. (2020), and it might be explained by the extracellular calcium concentration (Inglebert et al., 2020).
The model could in future studies be extended to include more complexity and account not only for postsynaptic Glu2NA NMDAR and Glu2NB-NMDAR, but also for the influence of mGluR activation, endocannabinoind, astrocytic signaling, presynaptic Glu2NC/D-NMDAR mediating effect on synaptic plasticity (Andrade-Talavera et al., 2016), adenosine (Pérez-Rodríguez et al., 2019), or other possible postsynaptic and non-postsynaptic mediators.
Current trend in neuroscience is shifting the focus toward studying the relationships between different levels and scales of brain organization to better understand the nervous systems and, ultimately, human behavior. Strong emphasis in the field is to connect these different levels and scales by multiscale techniques, to allow better exploration of the information flow between the cellular-, molecular-, and network/circuit-level phenomena, and the cognitive processes and behavior. Therefore, the presented model of synaptic plasticity that enables linking synapse-specific NMDAR function to the cell and network behavior is an important step toward understanding learning in hippocampal networks.
Data availability statement
The original contributions presented in the study are included in the article/Supplementary material, further inquiries can be directed to the corresponding author.
Author contributions
AS, MM, JD, and HM planned the research. AS and JD performed the numerical simulations and wrote the manuscript. All authors have read and approved the final manuscript.
Funding
This research was funded by the Research Council of (Lithuania), Agence Nationale de la Recherche (France) (Flagship ERA-NET Joint Transnational Call JTC 2019 in synergy with the Human Brain Project, No. S-FLAG-ERA-20-1/2020-PRO-28), the EU Horizon 2020 Framework Program for Research and Innovation (Specific Grant 945539, Human Brain Project SGA3), Fenix computing and storage resources was provided under Specific Grant Agreement No. 800858 (Human Brain Project ICEI), and a grant from the Swiss National Supercomputing Centre (CSCS) under project ID ich011. MM also acknowledges a contribution from the Italian National Recovery and Resilience Plan (NRRP), M4C2 and funded by the European Union - NextGenerationEU (Project IR0000011, CUP B51E22000150006, EBRAINS-Italy.
Conflict of interest
The authors declare that the research was conducted in the absence of any commercial or financial relationships that could be construed as a potential conflict of interest.
Publisher's note
All claims expressed in this article are solely those of the authors and do not necessarily represent those of their affiliated organizations, or those of the publisher, the editors and the reviewers. Any product that may be evaluated in this article, or claim that may be made by its manufacturer, is not guaranteed or endorsed by the publisher.
Supplementary material
The Supplementary Material for this article can be found online at: https://www.frontiersin.org/articles/10.3389/fnsyn.2023.1113957/full#supplementary-material
References
Adell, A. (2020). Brain NMDA receptors in schizophrenia and depression. Biomolecules 10:947. doi: 10.3390/biom10060947
Andrade-Talavera, Y., Duque-Feria, P., Paulsen, O., and Rodríguez-Moreno, A. (2016). Presynaptic spike timing-dependent long-term depression in the mouse hippocampus. Cereb. Cortex 26, 3637–3654. doi: 10.1093/cercor/bhw172
Badoual, M., Zou, Q., Davison, A., Lilith, M., Bal, T., Frégnac, Y., et al. (2006). Biophysical and phenomenological models of multiple spike interactions in spike-timing dependent plasticity. Int. J. Neural Syst. 16, 79–97. doi: 10.1142/S0129065706000524
Bartlett, T. E., Bannister, N. J., Collett, V. J., Dargan, S. L., Massey, P. V., Bortolotto, Z. A., et al. (2007). Differential roles of NR2A and NR2B-containing NMDA receptors in LTP and LTD in the CA1 region of two-week old rat hippocampus. Neuropharmacology 52, 60–70. doi: 10.1016/j.neuropharm.2006.07.013
Berberich, S., Punnakkal, P., Jensen, V., Pawlak, V., Seeburg, P. H., Hvalby, Ø., et al. (2005). Lack of NMDA receptor subtype selectivity for hippocampal long-term potentiation. J. Neurosci. 25, 6907–6910. doi: 10.1523/JNEUROSCI.1905-05.2005
Bezaire, M. J., Raikov, I., Burk, K., Vyas, D., and Soltesz, I. (2016). Interneuronal mechanisms of hippocampal theta oscillations in a full-scale model of the rodent CA1 circuit. Elife 5:e18566. doi: 10.7554/eLife.18566
Bhalla, U. S., and Iyengar, R. (1999). Emergent properties of networks of biological signaling pathways. Science 283, 381–387. doi: 10.1126/science.283.5400.381
Bi, G.-Q., and Poo, M.-M. (1998). Synaptic modifications in cultured hippocampal neurons: dependence on spike timing, synaptic strength, and postsynaptic cell type. J. Neurosci. 18, 10464–10472. doi: 10.1523/JNEUROSCI.18-24-10464.1998
Bliss, T. V., and Collingridge, G. L. (2013). Expression of NMDA receptor-dependent LTP in the hippocampus: bridging the divide. Mol. Brain 6, 5. doi: 10.1186/1756-6606-6-5
Bliss, T. V. P., and Collingridge, G. L. (1993). A synaptic model of memory: long-term potentiation in the hippocampus. Nature 361, 31–39. doi: 10.1038/361031a0
Brigman, J. L., Wright, T., Talani, G., Prasad-Mulcare, S., Jinde, S., Seabold, G. K., et al. (2010). Loss of GluN2B-containing NMDA receptors in CA1 hippocampus and cortex impairs long-term depression, reduces dendritic spine density, and disrupts learning. J. Neurosci. 30, 4590–4600. doi: 10.1523/JNEUROSCI.0640-10.2010
Buzsáki, G. (2002). Theta oscillations in the hippocampus. Neuron 33, 325–340. doi: 10.1016/S0896-6273(02)00586-X
Chindemi, G., Abdellah, M., Amsalem, O., Benavides-Piccione, R., Delattre, V., Doron, M., et al. (2022). A calcium-based plasticity model for predicting long-term potentiation and depression in the neocortex. Nat. Commun. 13, 3038. doi: 10.1038/s41467-022-30214-w
Clayton, D. A., Mesches, M. H., Alvarez, E., Bickford, P. C., and Browning, M. D. (2002). A hippocampal NR2B deficit can mimic age-related changes in long-term potentiation and spatial learning in the Fischer 344 rat. J. Neurosci. 22, 3628–3637. doi: 10.1523/JNEUROSCI.22-09-03628.2002
Clopath, C., Büsing, L., Vasilaki, E., and Gerstner, W. (2010). Connectivity reflects coding: a model of voltage-based STDP with homeostasis. Nat. Neurosci. 13, 344–352. doi: 10.1038/nn.2479
Collingridge, G. L., and Bliss, T. V. P. (1995). Memories of NMDA receptors and LTP. Trends Neurosci. 18, 54–56. doi: 10.1093/acprof:oso/9780192625021.001.0001
Collingridge, G. L., Kehl, S. J., and McLennan, H. (1983). Excitatory amino acids in synaptic transmission in the Schaffer collateral-commissural pathway of the rat hippocampus. J. Physiol. 334, 33–46. doi: 10.1113/jphysiol.1983.sp014478
Cui, Y., Jin, J., Zhang, X., Xu, H., Yang, L., Du, D., et al. (2011). Forebrain NR2B overexpression facilitating the prefrontal cortex long-term potentiation and enhancing working memory function in mice. PLoS ONE 6:e20312. doi: 10.1371/journal.pone.0020312
Cull-Candy, S., Brickley, S., and Farrant, M. (2001). NMDA receptor subunits: diversity, development and disease. Curr. Opin. Neurobiol. 11, 327–335. doi: 10.1016/S0959-4388(00)00215-4
Debanne, D., Gähwiler, B. H., and Thompson, S. M. (1998). Long-term synaptic plasticity between pairs of individual CA3 pyramidal cells in rat hippocampal slice cultures. J. Physiol. 507(Pt 1), 237–247. doi: 10.1111/j.1469-7793.1998.237bu.x
Destexhe, A., Mainen, Z. F., and Sejnowski, T. J. (1994). Synthesis of models for excitable membranes, synaptic transmission and neuromodulation using a common kinetic formalism. J. Comput. Neurosci. 1, 195–230. doi: 10.1007/BF00961734
Dudek, S. M., and Bear, M. F. (1992). Homosynaptic long-term depression in area CA1 of hippocampus and effects of N-methyl-D-aspartate receptor blockade. Proc. Natl. Acad. Sci. U.S.A. 89, 4363–4367. doi: 10.1073/pnas.89.10.4363
Ebner, C., Clopath, C., Jedlicka, P., and Cuntz, H. (2019). Unifying long-term plasticity rules for excitatory synapses by modeling dendrites of cortical pyramidal neurons. Cell Rep. 29, 4295.e6–4307.e6. doi: 10.1016/j.celrep.2019.11.068
Falcón-Moya, R., Pérez-Rodríguez, M., Prius-Mengual, J., Andrade-Talavera, Y., Arroyo-García, L. E., Pérez-Artés, R., et al. (2020). Astrocyte-mediated switch in spike timing-dependent plasticity during hippocampal development. Nat. Commun. 11, 4388. doi: 10.1038/s41467-020-18024-4
Feldman, D. E. (2012). The spike-timing dependence of plasticity. Neuron 75, 556–571. doi: 10.1016/j.neuron.2012.08.001
Ferguson, K., and Campbell, S. (2009). A two compartment model of a CA1 pyramidal neuron. Canad. Appl. Math. Quart. 17, 293–307.
Foster, K. A., McLaughlin, N., Edbauer, D., Phillips, M., Bolton, A., Constantine-Paton, M., et al. (2010). Distinct roles of NR2A and NR2B cytoplasmic tails in long-term potentiation. J. Neurosci. 30, 2676–2685. doi: 10.1523/JNEUROSCI.4022-09.2010
France, G., Fernández-Fernández, D., Burnell, E. S., Irvine, M. W., Monaghan, D. T., Jane, D. E., et al. (2017). Multiple roles of GluN2B-containing NMDA receptors in synaptic plasticity in juvenile hippocampus. Neuropharmacology 112(Pt A), 76–83. doi: 10.1016/j.neuropharm.2016.08.010
Franchini, L., Carrano, N., Di Luca, M., and Gardoni, F. (2020). Synaptic GluN2A-containing NMDA receptors: from physiology to pathological synaptic plasticity. Int. J. Mol. Sci. 21, 1538. doi: 10.3390/ijms21041538
Gardoni, F., Mauceri, D., Malinverno, M., Polli, F., Costa, C., Tozzi, A., et al. (2009). Decreased NR2B subunit synaptic levels cause impaired long-term potentiation but not long-term depression. J. Neurosci. 29, 669–677. doi: 10.1523/JNEUROSCI.3921-08.2009
Gasparini, S., Migliore, M., and Magee, J. C. (2004). On the initiation and propagation of dendritic spikes in CA1 pyramidal neurons. J. Neurosci. 24, 11046–11056. doi: 10.1523/JNEUROSCI.2520-04.2004
Ge, Y., Dong, Z., Bagot, R. C., Howland, J. G., Phillips, A. G., Wong, T. P., et al. (2010). Hippocampal long-term depression is required for the consolidation of spatial memory. Proc. Natl. Acad. Sci. U.S.A. 107, 16697–16702. doi: 10.1073/pnas.1008200107
Gerkin, R. C., Lau, P.-M., Nauen, D. W., Wang, Y. T., and Bi, G.-Q. (2007). Modular competition driven by NMDA receptor subtypes in spike-timing-dependent plasticity. J. Neurophysiol. 97, 2851–2862. doi: 10.1152/jn.00860.2006
Gerstner, W., Kempter, R., van Hemmen, J. L., and Wagner, H. (1996). A neuronal learning rule for sub-millisecond temporal coding. Nature 383, 76–78. doi: 10.1038/383076a0
Goh, J. J., and Manahan-Vaughan, D. (2013). Synaptic depression in the CA1 region of freely behaving mice is highly dependent on afferent stimulation parameters. Front. Integr. Neurosci. 7:1. doi: 10.3389/fnint.2013.00001
Graupner, M., and Brunel, N. (2007). STDP in a bistable synapse model based on CaMKII and associated signaling pathways. PLOS Computat. Biol. 3:e221. doi: 10.1371/journal.pcbi.0030221
Graupner, M., and Brunel, N. (2012). Calcium-based plasticity model explains sensitivity of synaptic changes to spike pattern, rate, and dendritic location. Proc. Nat. Acad. Sci. U.S.A. 109, 3991–3996. doi: 10.1073/pnas.1109359109
Hines, M. L., and Carnevale, N. T. (1997). The NEURON simulation environment. Neural Comput. 9, 1179–1209. doi: 10.1162/neco.1997.9.6.1179
Hunt, D. L., and Castillo, P. E. (2012). Synaptic plasticity of NMDA receptors: mechanisms and functional implications. Curr. Opin. Neurobiol. 22, 496–508. doi: 10.1016/j.conb.2012.01.007
Inglebert, Y., Aljadeff, J., Brunel, N., and Debanne, D. (2020). Synaptic plasticity rules with physiological calcium levels. Proc. Natl. Acad. Sci. U.S.A. 117, 33639–33648. doi: 10.1073/pnas.2013663117
Jędrzejewska-Szmek, J., Damodaran, S., Dorman, D. B., and Blackwell, K. T. (2017). Calcium dynamics predict direction of synaptic plasticity in striatal spiny projection neurons. Eur. J. Neurosci. 45, 1044–1056. doi: 10.1111/ejn.13287
Kastellakis, G., and Poirazi, P. (2019). Synaptic Clustering and Memory Formation. Front. Mol. Neurosci. 12:300. doi: 10.3389/fnmol.2019.00300
Kempter, R., Gerstner, W., and van Hemmen, J. L. (1999). Hebbian learning and spiking neurons. Phys. Rev. E 59, 4498–4514. doi: 10.1103/PhysRevE.59.4498
Kistler, W., and van Hemmen, L. (2000). Modeling synaptic plasticity in conjunction with the timing of pre- and postsynaptic action potentials. Neural Comput. 12, 385–405. doi: 10.1162/089976600300015844
Kocsis, B. (2012). Differential role of NR2A and NR2B subunits in N-methyl-D-aspartate receptor antagonist-induced aberrant cortical gamma oscillations. Biol. Psychiatry 71, 987–995. doi: 10.1016/j.biopsych.2011.10.002
Lau, B., Cole, S. R., and Gange, S. J. (2009). Competing risk regression models for epidemiologic data. Am. J. Epidemiol. 170, 244–256. doi: 10.1093/aje/kwp107
Li, R., Huang, F.-S., Abbas, A.-K., and Wigström, H. (2007). Role of NMDA receptor subtypes in different forms of NMDA-dependent synaptic plasticity. BMC Neurosci. 8, 55. doi: 10.1186/1471-2202-8-55
Liu, J., Chang, L., Song, Y., Li, H., and Wu, Y. (2019). The role of NMDA receptors in Alzheimer's disease. . Front. Neurosci. 13:43. doi: 10.3389/fnins.2019.00043
Liu, L., Wong, T. P., Pozza, M. F., Lingenhoehl, K., Wang, Y., Sheng, M., et al. (2004). Role of NMDA receptor subtypes in governing the direction of hippocampal synaptic plasticity. Science 304, 1021–1024. doi: 10.1126/science.1096615
Lüscher, C., and Malenka, R. C. (2012). NMDA receptor-dependent long-term potentiation and long-term depression (LTP/LTD). Cold Spring Harb. Perspect. Biol. 4, a005710. doi: 10.1101/cshperspect.a005710
MacDermott, A. B., Mayer, M. L., Westbrook, G. L., Smith, S. J., and Barker, J. L. (1986). NMDA-receptor activation increases cytoplasmic calcium concentration in cultured spinal cord neurones. Nature 321, 519–522. doi: 10.1038/321519a0
MacDonald, S. W. S., Nyberg, L., and Bäckman, L. (2006). Intra-individual variability in behavior: links to brain structure, neurotransmission and neuronal activity. Trends Neurosci. 29, 474–480. doi: 10.1016/j.tins.2006.06.011
Mäki-Marttunen, T., Iannella, N., Edwards, A. G., Einevoll, G. T., and Blackwell, K. T. (2020). A unified computational model for cortical post-synaptic plasticity. eLife 9, e55714. doi: 10.7554/eLife.55714.sa2
Malenka, R. C., and Bear, M. F. (2004). LTP and LTD: an embarrassment of riches. Neuron 44, 5–21. doi: 10.1016/j.neuron.2004.09.012
Malenka, R. C., and Nicoll, R. A. (1999). Long-term potentiation–a decade of progress? Science 285, 1870–1874. doi: 10.1126/science.285.5435.1870
Markram, H., Lübke, J., Frotscher, M., Roth, A., and Sakmann, B. (1997). Physiology and anatomy of synaptic connections between thick tufted pyramidal neurones in the developing rat neocortex. J. Physiol. 500(Pt 2), 409–440. doi: 10.1113/jphysiol.1997.sp022031
Meissner-Bernard, C., Tsai, M. C., Logiaco, L., and Gerstner, W. (2020). Dendritic voltage recordings explain paradoxical synaptic plasticity: a modeling study. Front. Synaptic Neurosci. 12:585539. doi: 10.3389/fnsyn.2020.585539
Migliore, M., De Simone, G., and Migliore, R. (2015). Effect of the initial synaptic state on the probability to induce long-term potentiation and depression. Biophys. J. 108, 1038–1046. doi: 10.1016/j.bpj.2014.12.048
Migliore, R., Lupascu, C. A., Bologna, L. L., Romani, A., Courcol, J.-D., Antonel, S., et al. (2018). The physiological variability of channel density in hippocampal CA1 pyramidal cells and interneurons explored using a unified data-driven modeling workflow. PLOS Comput. Biol. 14:e1006423. doi: 10.1371/journal.pcbi.1006423
Miry, O., Li, J., and Chen, L. (2021). The quest for the hippocampal memory engram: from theories to experimental evidence. Front. Behav. Neurosci. 14:632019. doi: 10.3389/fnbeh.2020.632019
Morishita, W., Lu, W., Smith, G. B., Nicoll, R. A., Bear, M. F., and Malenka, R. C. (2007). Activation of NR2B-containing NMDA receptors is not required for NMDA receptor-dependent long-term depression. Neuropharmacology 52, 71–76. doi: 10.1016/j.neuropharm.2006.07.005
Mulkey, R. M., and Malenka, R. C. (1992). Mechanisms underlying induction of homosynaptic long-term depression in area CA1 of the hippocampus. Neuron 9, 967–975. doi: 10.1016/0896-6273(92)90248-c
Neyton, J., and Paoletti, P. (2006). Relating NMDA receptor function to receptor subunit composition: Limitations of the pharmacological approach. J. Neurosci. 26, 1331–1333. doi: 10.1523/JNEUROSCI.5242-05.2006
Paoletti, P. (2011). Molecular basis of NMDA receptor functional diversity. Eur. J. Neurosci. 33, 1351–1365. doi: 10.1111/j.1460-9568.2011.07628.x
Paoletti, P., Bellone, C., and Zhou, Q. (2013). NMDA receptor subunit diversity: impact on receptor properties, synaptic plasticity and disease. Nat. Rev. Neurosci. 14, 383–400. doi: 10.1038/nrn3504
Parameshwaran, K., Dhanasekaran, M., and Suppiramaniam, V. (2008). Amyloid beta peptides and glutamatergic synaptic dysregulation. Exp. Neurol. 210, 7–13. doi: 10.1016/j.expneurol.2007.10.008
Park, P., Georgiou, J., Sanderson, T. M., Ko, K.-H., Kang, H., Kim, J.-I., et al. (2021). PKA drives an increase in AMPA receptor unitary conductance during LTP in the hippocampus. Nat. Commun. 12, 413. doi: 10.1038/s41467-020-20523-3
Pérez-Rodríguez, M., Arroyo-García, L. E., Prius-Mengual, J., Andrade-Talavera, Y., Armengol, J. A., Pérez-Villegas, E. M., et al. (2019). Adenosine receptor-mediated developmental loss of spike timing-dependent depression in the hippocampus. Cereb. Cortex 29, 3266–3281. doi: 10.1093/cercor/bhy194
Pfister, J.-P., Toyoizumi, T., Barber, D., and Gerstner, W. (2006). Optimal spike-timing-dependent plasticity for precise action potential firing in supervised learning. Neural Comput. 18, 1318–1348. doi: 10.1162/neco.2006.18.6.1318
Pi, H. J., and Lisman, J. E. (2008). Coupled phosphatase and kinase switches produce the tristability required for long-term potentiation and long-term depression. J. Neurosci. 28, 13132–13138. doi: 10.1523/JNEUROSCI.2348-08.2008
Pinsky, P. F., and Rinzel, J. (1994). Intrinsic and network rhythmogenesis in a reduced Traub model for CA3 neurons. J. Comput. Neurosci. 1, 39–60. doi: 10.1007/BF00962717
Poirazi, P., and Papoutsi, A. (2020). Illuminating dendritic function with computational models. Nat. Rev. Neurosci. 21, 303–321. doi: 10.1038/s41583-020-0301-7
Pousinha, P. A., Mouska, X., Bianchi, D., Temido-Ferreira, M., Rajão-Saraiva, J., Gomes, R., et al. (2019). The amyloid precursor protein C-terminal domain alters CA1 neuron firing, modifying hippocampus oscillations and impairing spatial memory encoding. Cell Rep. 29, 317.e5–331.e5. doi: 10.1016/j.celrep.2019.08.103
Pousinha, P. A., Mouska, X., Raymond, E. F., Gwizdek, C., Dhib, G., Poupon, G., et al. (2017). Physiological and pathophysiological control of synaptic GluN2B-NMDA receptors by the C-terminal domain of amyloid precursor protein. eLife 6, e25659. doi: 10.7554/eLife.25659.015
Sacramento, J., Costa, R. P., Bengio, Y., and Senn, W. (2018). “Dendritic cortical microcircuits approximate the backpropagation algorithm,” in Proceedings of the 32nd International Conference on Neural Information Processing Systems, NIPS'18 (Red Hook, NY: Curran Associates Inc.), 8735–8746.
Saudargiene, A., Cobb, S., and Graham, B. P. (2015). A computational study on plasticity during theta cycles at Schaffer collateral synapses on CA1 pyramidal cells in the hippocampus. Hippocampus 25, 208–218. doi: 10.1002/hipo.22365
Senn, W., Markram, H., and Tsodyks, M. (2001). An algorithm for modifying neurotransmitter release probability based on pre- and post-synaptic spike timing. Neural Comput. 13, 35–67. doi: 10.1162/089976601300014628
Shipton, O. A., and Paulsen, O. (2014). GluN2A and GluN2B subunit-containing NMDA receptors in hippocampal plasticity. Philos. Trans. R. Soc. Lond. B Biol. Sci. 369, 20130163. doi: 10.1098/rstb.2013.0163
Shouval, H. Z., Bear, M. F., and Cooper, L. N. (2002). A unified model of NMDA receptor-dependent bidirectional synaptic plasticity. Proc. Natl. Acad. Sci. U.S.A. 99, 10831–10836. doi: 10.1073/pnas.152343099
Song, S., and Abbott, L. (2000). Temporally asymmetric Hebbian learning and neuronal response variability. Neurocomputing 32–33, 523–528. doi: 10.1016/S0925-2312(00)00208-3
Song, S., Miller, K. D., and Abbott, L. F. (2000). Competitive Hebbian learning through spike-timing-dependent synaptic plasticity. Nat. Neurosci. 3, 919–926. doi: 10.1038/78829
Standage, D., Trappenberg, T., and Blohm, G. (2014). Calcium-dependent calcium decay explains STDP in a dynamic model of hippocampal synapses. PLoS ONE 9:e86248. doi: 10.1371/journal.pone.0086248
Toyoizumi, T., Pfister, J.-P., Aihara, K., and Gerstner, W. (2005). Generalized Bienenstock–Cooper–Munro rule for spiking neurons that maximizes information transmission. Proc. Nat. Acad. Sci. U.S.A. 102, 5239–5244. doi: 10.1073/pnas.0500495102
Urbanczik, R., and Senn, W. (2014). Learning by the dendritic prediction of somatic spiking. Neuron 81, 521–528. doi: 10.1016/j.neuron.2013.11.030
Volianskis, A., France, G., Jensen, M. S., Bortolotto, Z. A., Jane, D. E., and Collingridge, G. L. (2015). Long-term potentiation and the role of N-methyl-d-aspartate receptors. Brain Res. 1621, 5–16. doi: 10.1016/j.brainres.2015.01.016
Weitlauf, C., Honse, Y., Auberson, Y. P., Mishina, M., Lovinger, D. M., and Winder, D. G. (2005). Activation of NR2A-containing NMDA receptors is not obligatory for NMDA receptor-dependent long-term potentiation. J. Neurosci. 25, 8386–8390. doi: 10.1523/JNEUROSCI.2388-05.2005
Wittenberg, G. M., and Wang, S. S.-H. (2006). Malleability of spike-timing-dependent plasticity at the CA3-CA1 synapse. J. Neurosci. 26, 6610–6617. doi: 10.1523/JNEUROSCI.5388-05.2006
Wong, J. M., and Gray, J. A. (2018). Long-term depression is independent of GluN2 subunit composition. J. Neurosci. 38, 4462–4470. doi: 10.1523/JNEUROSCI.0394-18.2018
Yasuda, R., Hayashi, Y., and Hell, J. W. (2022). CaMKII: a central molecular organizer of synaptic plasticity, learning and memory. Nat. Rev. Neurosci. 23, 666–682. doi: 10.1038/s41583-022-00624-2
Zamzow, D. R., Elias, V., Shumaker, M., Larson, C., and Magnusson, K. R. (2013). An increase in the association of GluN2B containing NMDA receptors with membrane scaffolding proteins was related to memory declines during aging. J. Neurosci. 33, 12300–12305. doi: 10.1523/JNEUROSCI.0312-13.2013
Zhao, M.-G., Toyoda, H., Lee, Y.-S., Wu, L.-J., Ko, S. W., Zhang, X.-H., et al. (2005). Roles of NMDA NR2B subtype receptor in prefrontal long-term potentiation and contextual fear memory. Neuron 47, 859–872. doi: 10.1016/j.neuron.2005.08.014
Keywords: synaptic plasticity, NMDA receptor, GluN2B-NMDA receptor subunit, CA1 pyramidal neuron, hippocampus
Citation: Dainauskas JJ, Marie H, Migliore M and Saudargiene A (2023) GluN2B-NMDAR subunit contribution on synaptic plasticity: A phenomenological model for CA3-CA1 synapses. Front. Synaptic Neurosci. 15:1113957. doi: 10.3389/fnsyn.2023.1113957
Received: 01 December 2022; Accepted: 13 February 2023;
Published: 15 March 2023.
Edited by:
Alfredo Kirkwood, Johns Hopkins University, United StatesReviewed by:
Harel Z. Shouval, University of Texas Health Science Center at Houston, United StatesMikel Pérez-Rodríguez, MRC Laboratory of Molecular Biology (LMB), United Kingdom
Copyright © 2023 Dainauskas, Marie, Migliore and Saudargiene. This is an open-access article distributed under the terms of the Creative Commons Attribution License (CC BY). The use, distribution or reproduction in other forums is permitted, provided the original author(s) and the copyright owner(s) are credited and that the original publication in this journal is cited, in accordance with accepted academic practice. No use, distribution or reproduction is permitted which does not comply with these terms.
*Correspondence: Ausra Saudargiene, YXVzcmEuc2F1ZGFyZ2llbmVAbHNtdW5pLmx0