Development and validation of radiomic signature for predicting overall survival in advanced stage cervical cancer
- 1Department of Radiation Oncology (Maastro), GROW - School for Oncology and Reproduction, Maastricht University Medical Centre+, Maastricht, Netherlands
- 2Department of Nuclear Medicine, Tata Memorial Hospital, Mumbai, India
- 3Homi Bhabha National Institute, BARC Training School Complex, Mumbai, India
- 4Department of Medical Oncology, Tata Memorial Hospital, Mumbai, India
- 5Department of Surgical Oncology, Tata Memorial Hospital, Mumbai, India
- 6Advance Center for Treatment, Research, Education in Cancer, Navi-Mumbai, India
Background: The role of artificial intelligence and radiomics in prediction model development in cancer has been increasing every passing day. Cervical cancer is the 4th most common cancer in women worldwide contributing to 6.5% of all cancer types. The treatment outcome of cervical cancer patients varies and individualized prediction of disease outcome is of paramount importance.
Purpose: The purpose of this study is to develop and validate the digital signature for 5-year overall survival prediction in cervical cancer using robust CT radiomic and clinical features.
Materials and Methods: Pretreatment clinical features and CT radiomic features of 68 patients, who were treated with chemoradiation therapy in our hospital were used in this study. Radiomic features were extracted using an in-house developed python script and pyradiomic package. Clinical features were selected by the recursive feature elimination technique. Whereas radiomic feature selection was performed using a multi-step process i.e., step-1: only robust radiomic features were selected based on our previous study, step-2: a hierarchical clustering was performed to eliminate feature redundancy, and final step-3: recursive feature elimination was performed to select the best features for prediction model development. Four machine algorithms i.e., Logistic regression (LR), Random Forest (RF), Support vector classifier (SVC), and Gradient boosting classifier (GBC) were used to develop 24 models (six models using each algorithm) using clinical, radiomic and combined features. Models were compared based on the prediction score in the internal validation.
Results: The average prediction accuracy was found to be 0.65 (95% CI: 0.60–0.70), 0.72 (95% CI: 0.63–0.81), and 0.77 (95% CI: 0.72–0.82) for clinical, radiomic, and combined models developed using four prediction algorithms respectively. The average prediction accuracy was found to be 0.69 (95% CI: 0.62–0.76), 0.79 (95% CI: 0.72–0.86), 0.71 (95% CI: 0.62–0.80), and 0.72 (95% CI: 0.66–0.78) for LR, RF, SVC and GBC models developed on three datasets respectively.
Conclusion: Our study shows the promising predictive performance of robust radiomic signature to predict 5-year overall survival in cervical cancer patients.
1. Introduction
Cancer is one of the most fatal diseases and is considered the second most lethal disease across the world (1). As per Global Cancer Statistics 2020 (GLOBOCAN 2020), cervical cancer is the 4th commonest cancer worldwide, 6th commonest cancer in developed countries and 2nd commonest cancer in developing countries in the female population (2, 3). The Cervical cancer-related mortality rate among women varies across the globe and there is a distinct difference in developed and developing countries (2–4). Breast and cervical cancer are the leading causes of cancer death in 103 and 42 countries, whereas lung cancer is the leading cause of cancer death in 28 countries (1–4). In developing countries, cervical cancer is the second leading cause of cancer-related death, whereas in developed countries it is the sixth leading cause (1–7). Cervical cancer management has been approached on two fronts i.e., prevention or early detection of cervical cancer by implementing screening programs and treatment of cervical cancer using evidence-based medicine (8–14). The incidence of cervical cancer in developed countries has reduced to half between 1972 and 2018 (8–10). The reason for the reduced incidence and mortality rate due to cervical cancer in developed countries can be attributed to the effective implementation of cervical cancer screening and HPV vaccination programs. Availability of several new technologies or advancements in existing technology like CT, PET/CT, ultrasound and MRI has led to early diagnosis and better staging of the disease leading to improvement in overall survival and quality of life index (11–14). The staging of cervical cancer is very complex and technically demanding. The staging system developed by the International Federation of Obstetrics and Gynecology (Fédération Internationale de Gynecologie et d’Obstetrique, or FIGO) is used for cervical cancer. Bhatla N. et.al. have published the recently revised FIGO staging of carcinoma of the cervix uteri to differentiate the various stages and substages of the disease (15). Improvement in diagnostic accuracy due to the implementation of newer technologies like PET/CT, MRI, and transvaginal ultrasound has improved cervical cancer staging and treatment in the last few years. As conventional treatment has a very low response rate of around 20–30 per cent that proves; one size fits for all principle usually doesn't work in cancer management (15–17). In the last few years, diagnostic modalities like immunohistochemistry (IHC), genetic profiling, tumor marker studies have established the fact that there are variations in disease in the same disease in different patients (18). Hence, cancer treatment is gradually shifting towards personalized treatment or tailored treatment and replacing conventional treatment (19). With the growing use of various computer-aided technologies in oncology in the last decade, these technologies have taken the forefront in cancer management worldwide (20). These technologies are being utilized for diagnosis, treatment planning, interim evaluation, and follow-up of the disease. In the last few years as the effort is being taken to provide personalized treatment to the patients, the ability of these technologies is being tested to predict treatment outcome, toxicity profile, and treatment selection for patients. Utilization of available technologies like machine learning, radiomics, genomics, etc. for enabling personalized treatment, especially those at high risk and are responding very poorly to standard treatment protocols, is of great interest for the clinicians (20). Such a technological-driven system has shown promising results in the selection or modification of treatment plans, to improve the treatment outcome (21–23). Major types of ML techniques include Decision Tree (DT), Support Vector Machine (SVM), Artificial Neural Networks (ANN), Naïve Bayesian Classifier (BC), Bayesian Network (BN), K-Nearest Neighbor (KNN) and Random Forest (RF) have been used for nearly three decades in cancer detection (21–25). In cancer prediction modelling, the main three predictive tasks are the prediction of cancer susceptibility, the prediction of cancer recurrence/metastasis, and the prediction of survival. Several such technology-driven prediction models have been developed, tested, and utilized in the last decade in screening programs and the treatment of cervical cancer (26–39). However, several prediction models have been developed using clinical and radiomics features predicting survival outcomes but the stability of radiomic features has been questioned by many researchers. In our earlier study, we have performed a detailed stability study of CT radiomic features and found around 100 robust radiomic features. In this study, we have tried to find the prediction capability of robust radiomic features with and without clinical features in predicting 5-year overall survival. This study is also the first of this kind from India.
2. Material and method
2.1. Patient demographics
The study was approved by the institutional ethics committee as a retrospective study with a waiver of consent. In total 68 patients were included in this study and had ages ranging 45–72 years (median: 56 years), at the time of diagnosis. All patients were diagnosed with cervical cancer between 2005 and 2009 and treated with definitive chemoradiotherapy or concomitant chemo and radiation therapy were included in this study. External beam radiation therapy (EBRT) dose range between 43.2 and 60.4 Gy (median = 50 Gy) was considered as radiotherapy procedures. Disease staging was performed according to the International Federation of Gynecology and Obstetrics (FIGO) classification. The numbers of patients in various FIGO stages in this cohort of patients are provided in Table 1. The majority of the patients (85%) had squamous cell carcinoma and only a few patients (15%) had other histology. From diagnosis to the last follow-up, the meantime was 72 (range: 5–140) months. 48 of the 68 patients had survived more than five years, whereas 26 had survived less than five years. In our study, we have aimed to establish the correlation between radiomics/clinical features and overall survival. The initial characteristics of the study population are given in Table 1.
20 clinical, pathological and radiological features were extracted from electronic health records as approved by the hospital ethics committee and out of that 13 features were used for further processing. Pretreatment PET/CT scans were also downloaded from the PACS for radiomic extraction. 1,093 CT radiomics features were extracted from the CT series of PET/CT scans.
2.2. PET/CT imaging procedure
All of the baseline PET/CT scans were performed using Gemini TF16 or Gemini TF64 PET/CT scanners (Philips Medical Systems, Netherlands) (40). F-18 FDG radiopharmaceutical was administered to the patient as per institutional protocol i.e., 4–5 MBq/kg body weight after 6 h of fasting. Scans were performed between 60 min and 100 min after administration of the radiopharmaceutical.
Contrast-enhanced CT scans were performed after the injection of 60–80 ml of non-ionic contrast using the protocol mentioned in Supplementary Table S1. CT images were reconstructed using the Filtered back project (FBP) reconstruction algorithm.
2.3. Radiomic extraction
DICOM images of PET/CT scan were downloaded on Philips Intellispace Discovery (research-only build; Philips Medical System, Eindhoven, The Netherlands) from PACS. The tumor was contoured using 3D contouring software installed on Intellispace Discovery by a 15-year experienced medical physicist and checked & approved by a 30-year experienced nuclear medicine physician. The contours were saved as RTStructure by the name of GTV. Subsequently, the image and GTV were transferred to the research computer for radiomic extraction.
Images and GTV were converted into NRRD format using Plastmatch software (41). Thereafter following pre-processing steps were applied using an in-house developed python script and the Pyradiomics package (42) for radiomic extraction. Resampling: Images were resampled using a 2 × 2 × 2 mm cube isotropic voxel. Filtering and transformation of image: From original images three sets of filtered images were produced applying Laplacian of Gaussian (LoG) filters with 1, 2, and 3 mm sigma values. We also generated 8 sets of wavelet-transformed images using eight combinations of high-pass and low-pass wavelet filters (42–44).
A total of 1,093 radiomic features were extracted from 12 sets of images (1 set of original images, 3 sets of LoG images, and 8 sets of Wavelet Images) and corresponding GTVs (42).
2.4. Prediction algorithm used
The commonly used machine learning algorithms for classification problems i.e., Logistic regression (LR), Random Forest classifier (RF), Gradient boosting classifier (GBC), and Support vector classifier (SVC) were used for prediction model development (45–52).
2.5. Feature selection
The multi-step process was adopted for feature selection in this study. The following subsections describe the various methods adopted for feature selection. The steps utilized for feature selection are summarized in Figure 1.
2.5.1. Clinical features selection
Considering the completeness of data 13 clinical features were selected for further processing. Spearman correlation test was performed to find correlating features and reduce the redundancy among the features. The association of clinical features with outcome i.e., 5years overall survival (OS) was carried out using a t-test. Finally, recursive feature elimination (RFE) methods using logistic regression (RFE-LR) and random forest (RFE-RF) were applied to select two sets of features for prediction model development.
2.5.2. Radiomic feature selection
We opted for a two-step process to select the best radiomic features for OS prediction out of 1,093 radiomic features extracted from CT images. In the first step of feature selection, step-1: we included 121 stable radiomic features for the next step of feature selection based on our earlier radiomic stability study (53). In the second step of feature selection, step-2: we performed a Spearman correlation test to identify redundant features followed by step-3: recursive feature elimination (RFE) methods using logistic regression (RFE-LR) and random forest (RFE-RF) were applied to select two sets of features for the prediction model development.
2.5.3. Combined (clinical + radiomic) features selection
The top 7 clinical features and top 15 radiomic features which were identified in clinical and radiomic feature selection steps were used to select the best features for the combined model. Recursive features selection (RFE) methods using logistic regression (RFE-LR) and random forest (RFE-RF) were applied to select two sets of features for prediction model development.
Features selected using random forest model were used to develop models using random forest (RF) Support vector classifier (SVC) and Gradient Boosting and feature selected using logistic regression (LR) was used to develop logistic regression model.
2.6. Nested cross-validation
Nested cross-validation was performed on the entire dataset using 7 outer and 6 inner loops were used for tuning the hyperparameters of the models (54). Finally, a random train-test split (in 7:3 ratio) of data was performed and a prediction model was developed and validated.
2.7. Data balancing
After the train-test split, the training dataset was used to develop the prediction models with and without balancing the train data set for survival outcomes. Data balancing was performed by using minority oversampling. Validation was performed using the test data set without balancing the data.
2.8. Model development
A total of 24 prediction models were developed using the aforementioned four prediction algorithms, three data sets with and without balancing the train data sets (Supplementary Table S2 and Figure 2).
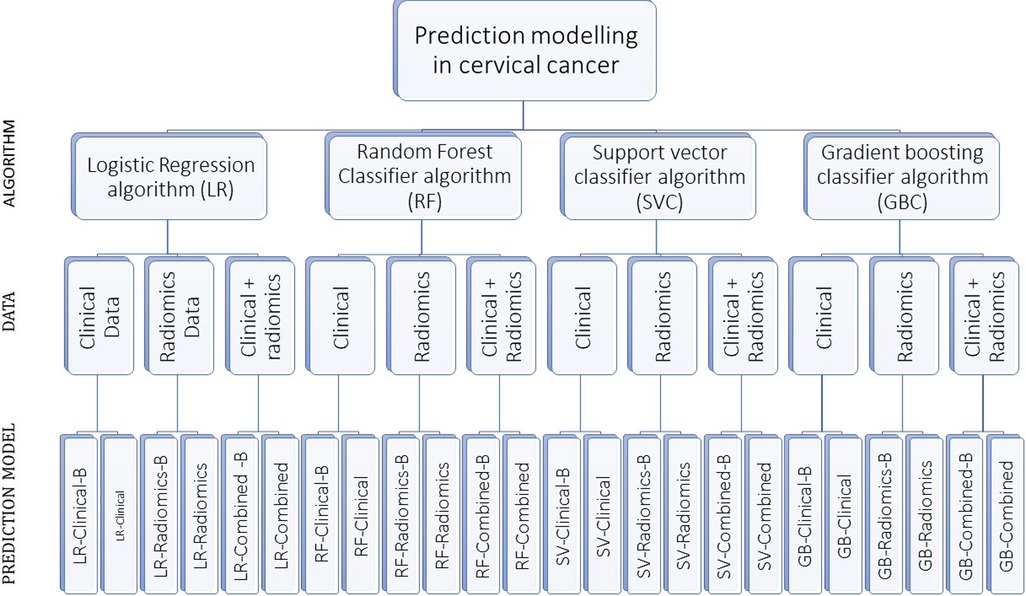
Figure 2. The figure shows our algorithm to develop 24 prediction models using various combinations. “-B” in the model’s name indicates the model developed using a balanced train data set.
2.9. Model evaluation and selection
All the developed models were evaluated by plotting the area under the receiver operator curve (AUC) to graphically represent the association between the features and the outcome i.e., 5-year overall survival in the validation set. The best model was selected based on the performance score of each model in the validation set.
2.10. Statistical analysis
Statistical analyses were performed using R (v3.5.2, the R foundation for statistical computing, Vienna, Austria) or Python 3.9.0 software. Prediction model development and validation of model were performed using python 3.9.0 software.
3. Results
In total 68 patients were selected for this study who fulfilled the criteria of completeness of data sets. The details of data collection are provided in Figure 3.
3.1. Feature selection
3.1.1. Clinical
In total 13 clinical and radiological features were used for this study. Figure 4 shows the Spearman correlation among the features there are a few features which have a strong positive and are few which has a strong negative correlation. For example; surgery and R0 resection have a strong negative correlation with EBRT and Brachytherapy (r2 = −0.65 to −0.69) HPR adenocarcinoma has a very strong negative correlation (r2 = −1) with that of HPR squamous cell carcinoma probably they do not exist together and both cannot be used as they are redundant features. Whereas follow-up time in months has a strong positive correlation (r2 = 0.8) with that of new vital (recurrence) may be because increasing follow-up time increases the chance of recurrence. Surgery has a strong positive correlation (r2 = 0.88) with that of R0 resection probably both cooccur. Among strong correlating features, one feature each was selected for the next step of feature selection. Recursive feature elimination (RFE) was performed using logistic regression and random forest algorithms. Total 5 clinical features were found significant for each algorithm independently (Table 2 and Figure 6).
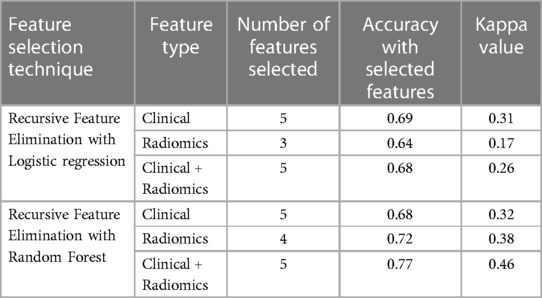
Table 2. The table shows the number of features selected, accuracy and kappa value for various combinations of data sets using multivariate recursive feature elimination with logistic regression and random forest.
3.1.2. Radiomics
121 stable radiomics features based on our earlier study were included in this study (53). Spearman correlation shows distinct 10 clusters (Figure 5) and these clusters were having positive or negative correlations. Based on these clusters and r2 value 15 radiomic features were selected to include in the next step of feature selection. Recursive feature elimination (RFE) was performed using logistic regression and random forest algorithms. In total 3 and 4 radiomic features were found to be significant for logistic regression and random forest algorithms respectively (Table 2 and Figure 6).
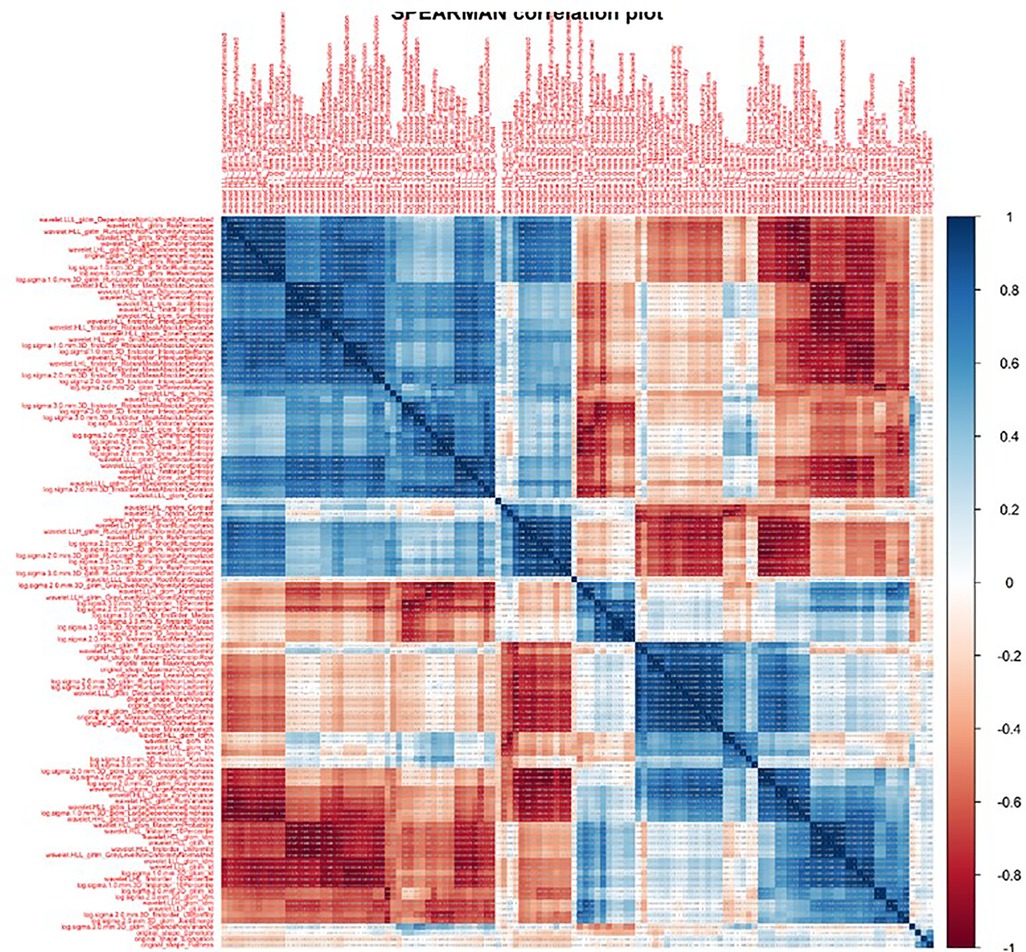
Figure 5. The figure shows the spearman correlation among the radiomic features showing clusters of features with positive and negative correlations.
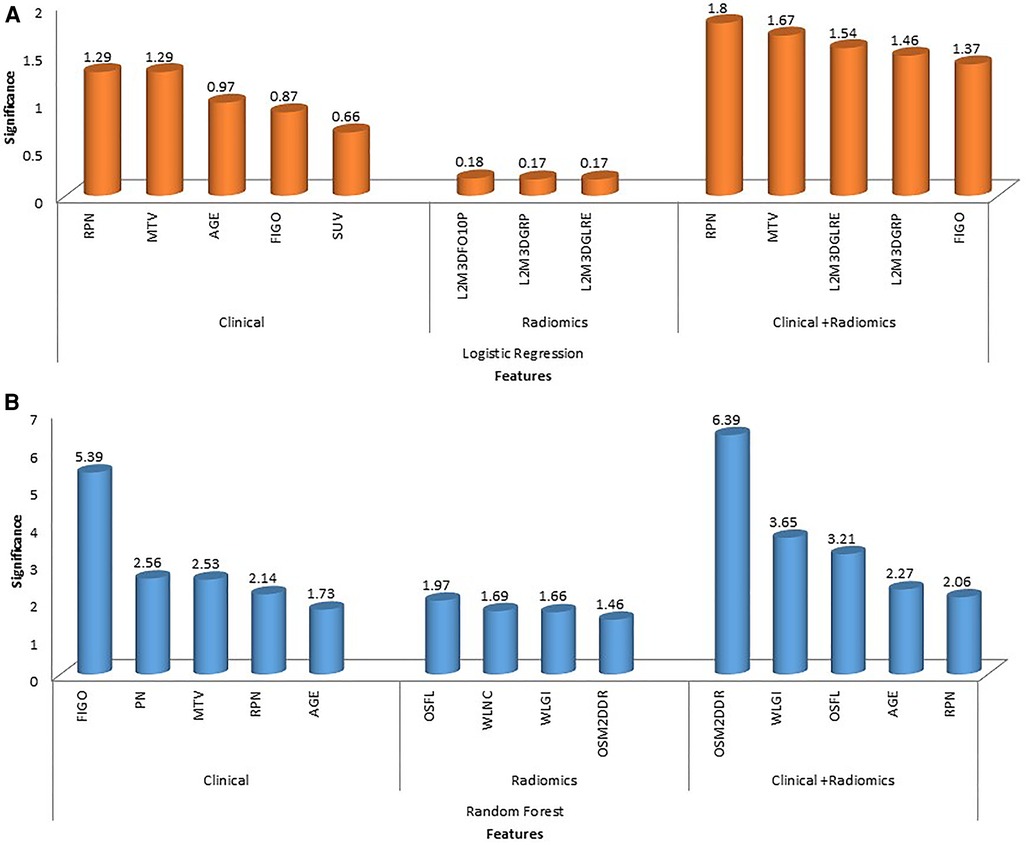
Figure 6. The figure shows feature importance in various combinations of algorithms and features. The first row shows feature importance using a logistic regression algorithm for clinical, radiomic and combined (clinical + radiomics) features (A); the second row shows feature importance using random forest algorithm for clinical, radiomic and combined (clinical + radiomics) features (B). (Abbreviations: OSFL, original_shape_flatness; WLNC, wavelet_LHL_ngtdm_contrast; WLGI, wavelet_LLL_glcm_Idn; OSM2DDR, original_shape_maximum2DDiameterRow; L3M3DFO10P, log-sigma-3-0-mm-3D_firstorder_10percentile; L2M3DGRP, log-sigma-2-0-mm-3D_glrlm_runPercentage; L2M3DGLFE, log-sigma-2-0-mm-3D_glrlm_longRunEmphasis).
3.1.3. Combined (clinical + radiomics)
Among clinical and radiomic features selected independently, the most significant mixed features were selected using recursive feature elimination with logistic regression and random forest algorithms. Total 5 clinical + radiomics features were found significant for each of the algorithms separately (Table 2 and Figure 6). The selected radiomic feature shows the distinct distribution of feature values in two groups of patients i.e., OS > 5 years and OS < 5 years. The box plots show the distribution of all the selected features in two groups of patients (Supplementary Figures S1–S9).
3.2. Model development and validation
Four algorithms i.e., Logistic regression (LR), Random Forest (RF), Support vector classifier (SVC) and gradient boost classifier (GBC) were used for prediction model development total 24 prediction models using four prediction algorithms for clinical, radiomics and combined features.
Nested cross-validation: Nested cross-validation was performed for all the prediction algorithms for tuning their hyperparameters. The prediction algorithms along with the best hyperparameters and validation scores are shown in Table 3.
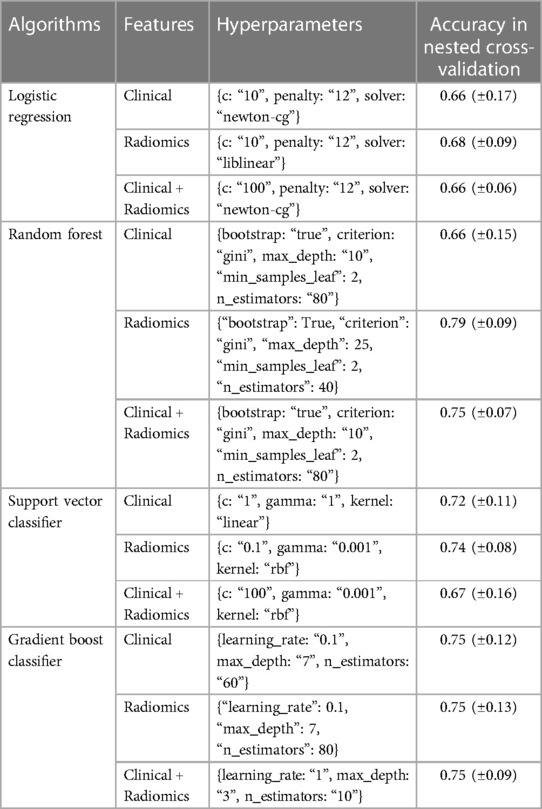
Table 3. This table shows the selected hyperparameters and nested cross-validation scores of various models.
All 24 models showed good prediction capability of 5-year overall survival. The average accuracy and AUC in validation sets across all the 24-prediction models were found to be 0.73 (95% CI: 0.66–0.80) and 0.60 (95% CI: 0.49–0.71) respectively. The detailed complete validation scores of all the models are shown in Table 4 and Figure 7.
3.2.1. Logistic regression model
The average accuracy and AUC for logistic regression models across six models developed with various combinations were found to be 0.69 (95% CI: 0.62–0.76) and 0.60 (95% CI: 0.55–0.65) respectively. The AUC of all the logistic regression models is shown in Supplementary Figure S10. Radiomics [accuracy: 0.76 (LR-Radiomics-B); 0.71 (LR-Radiomics)] or combined prediction [accuracy: 0.71 (LR-Combined-B); 0.76 (LR-Combined)] model models had better prediction capabilities in comparison of clinical models [accuracy: 0.61 (LR-Clinical-B); 0.61 (LR-Clinical)] developed with logistic regression algorithm.
3.2.2. Random forest model
The average accuracy and AUC for random forest models were found to be 0.79 (95% CI: 0.72–0.86) and 0.73 (95% CI: 0.66–0.80) respectively. The AUC of all the Random Forest models is shown in Figure 7. Radiomics [accuracy: 0.86 (RF-Radiomics-B); 0.81 (RF-Radiomics)] or combined prediction [accuracy: 0.81 (RF-Combined-B); 0.81 (RF-Combined)] model models had better prediction capabilities in comparison of clinical models [accuracy: 0.67 (RF-Clinical-B); 0.76 (RF-Clinical)] developed with random forest algorithm.
3.2.3. Support vector classifier (SVC) model
The average accuracy and AUC for support vector models were found to be 0.71 (95% CI: 0.63–0.79) and 0.69 (95% CI: 0.51–0.87) respectively. The AUC of all the support vector classifier models is shown in Supplementary Figure S12. Radiomics [accuracy: 0.76 (SV-Radiomics-B); 0.71 (SV-Radiomics)] or combined prediction [accuracy: 0.76 (SV-Combined-B); 0.81 (SV-Combined)] model models had better prediction capabilities in comparison of clinical models [accuracy: 0.62 (SV-Clinical-B); 0.62 (SV-Clinical)] developed with support vector classifier algorithm.
3.2.4. Gradient boosting classifier (GBC) model
The average accuracy and AUC for gradient boosting models were found to be 0.72 (95% CI: 0.66–0.78) and 0.73 (95% CI: 0.68–0.78) respectively. The AUC of all the Gradient busting classifier models is shown in Supplementary Figure S13. Radiomics [accuracy: 0.76 (GB-Radiomics-B); 0.76 (GB-Radiomics)] or combined prediction [accuracy: 0.76 (GB-Combined-B); 0.76 (GB-Combined)] models had better prediction capabilities in comparison of clinical models [accuracy: 0.67 (GB-Clinical-B); 0.62 (GB-Clinical)] developed with gradient boosting algorithm.
3.3. Model selection
RF-Radiomics-B model had the best prediction accuracy (accuracy = 0.86; AUC = 0.82) among all 24 models developed. The average prediction accuracy for clinical, radiomic, and combined models were found to be 0.65 (95% CI: 0.60–0.70), 0.72 (95% CI: 0.63–0.81), and 0.77 (95% CI: 0.72–0.82) respectively. The average prediction accuracy for logistic regression, random forest, support vector classifier, and gradient boosting classifier models were found to be 0.69 (95% CI: 0.62–0.76), 0.79 (95% CI: 0.72–0.86), 0.71 (95% CI: 0.62–0.80), 0.72 (95% CI: 0.66–0.78) respectively.
4. Discussion
Our study shows the significance of radiomic features in generating statistical machine-learning models for disease outcomes like 5-year overall survival prediction in cervical cancer. With this study, we were able to identify the gap in the data archival system in our hospital related to medical image archives as well as other clinical data points as described in the result section. With this study, we were able to determine the most effective radiomic feature and their combination for the prediction of disease outcomes. A rigorous method of feature selection by applying various techniques has helped this study to select the most efficient features which can become a digital signature for the stated disease outcome. We tested various prediction algorithms with radiomics and clinical features separately and in combination. In multivariate analysis with random forest, radiomic features were found to be better associated with disease outcomes in our cohort. Our result was consistent with various other studies performed on cervical cancer outcome prediction. If we consider our study with other studies performed in this field our study design had similarities with others except, we tested several prediction algorithms to select the best fits for our cohort. Our finding is consistent with other studies similar studies performed earlier (12, 14, 15, 26–39, 55–66). Clinical features like age, presence or absence of retroperitoneal node, and peritoneal node FIGO stage at the time of diagnosis were also found to be prognostic markers in our study which was consistent with the published literature (12, 14, 15, 26–33, 57–60). In univariate and multivariate analysis clinical features i.e., Age, FIGO stage, absence and presence of retroperitoneal node and peritoneal node, imaging features i.e., SUV MTV found an association with 5year overall survival, which was consistent with other published literature (12, 14, 29, 33, 34, 57, 61, 66). Similarly in univariate and multivariate studies, radiomic features showed a significant association with 5-year OS which is also consistent with published literature (28, 29, 34, 66). As we had selected only stable radiomic features based on our earlier study (53) that shows the repeatable and reproducible radiomic features also show excellent prognostic and predictive value in cervical cancer. The effort of the radiomic community should be to identify the robust features and find out the predictive capabilities of those stable features in various disease groups for various prediction endpoints. Among various prediction models tested in our study, RF-Radiomics-B random forest model showed the best accuracy in nested cross-validation as well as the train-test final model outperformed all the prediction models used in our study. Whereas LR-Clinical-B and LR-Clinical logistic regression models show the lowest accuracy in predicting overall survival in this study. When we compared the performance score of prediction models with radiomic, clinical and combined models, again random forest and gradient boosting models were at the top.
The average accuracy of clinical models with all four prediction algorithms was less than that of radiomics and combined models which is similar to previously published work (26–30). The radiomic and combined model performance across all four prediction algorithms were found to be more or less similar. Our study also confirms the superiority of radiomic features over clinical features in predating overall survival in cervical cancer. Comparing the prediction algorithms, the random forest-based prediction models had better accuracy in comparison to the other three which affirms the findings of earlier published literature in cervical cancer (26, 27). We found little difference between the models developed using with or without balanced train sets maybe because of event rate in our study was adequately balanced and may not require data balancing as an additional step. The radiomic community has been concerned about the stability of radiomic features. The radiomic community is skeptical about stable radiomic features' ability to predict outcomes (67). This is probably the first study published on cancer prediction modelling using stable radiomic features independently or in combination with clinical features. In our study, we were able to show that radiomic features can be used for 5-year overall prediction in cervical cancer. This was also the first prediction modelling study to be conducted on cervical cancer patients in India. Other researchers in India will be motivated to conduct prediction modelling studies for evolving digital signatures of disease outcomes based on our study. Study type: single-center, small sample size, and no external or prospective validation are some of the limitations of this study. The future will involve repeating this study at our hospital with larger sample size, as well as initiating multicentric studies to develop a universally accepted model. It is the ultimate objective of this research to validate this model using prospective clinical trials and then implement decision support systems in clinics based on a validated predictive model with retrospective and prospective data.
5. Conclusion
We have demonstrated in our study that robust radiomic features are predictive of 5-year overall survival for cervical cancer patients. According to this study, random forest prediction algorithms can predict better than other algorithms. The model's predictive ability is slightly improved by using data balancing. Although radiomic features are superior to clinical features in terms of prediction abilities, they are most effective when combined with clinical features. Overall, this study suggests the importance of radiomics and artificial intelligence in implementing decision-support systems in the management of cervical cancer.
Data availability statement
The raw data supporting the conclusions of this article will be made available by the authors, without undue reservation.
Ethics statement
The studies involving human participants were reviewed and approved by IEC-I Tata Memorial Hospital, Parel, Mumbai. Written informed consent for participation was not required for this study in accordance with the national legislation and the institutional requirements.
Author contributions
All the authors have contributed to the research and manuscript writing.
Funding
This work was supported by Indo-Dutch NWO research grant BIONIC (grant no 629.002.205, Recipient: A. Dekker) and Ministry of Electronics and Information Technology (MietY) (IN) (grant no 13(2)-2015-CC-BT, Recipient: V. Rangarajan)
Conflict of interest
The authors declare that the research was conducted in the absence of any commercial or financial relationships that could be construed as a potential conflict of interest.
Publisher's note
All claims expressed in this article are solely those of the authors and do not necessarily represent those of their affiliated organizations, or those of the publisher, the editors and the reviewers. Any product that may be evaluated in this article, or claim that may be made by its manufacturer, is not guaranteed or endorsed by the publisher.
Supplementary material
The Supplementary Material for this article can be found online at: https://www.frontiersin.org/articles/10.3389/fnume.2023.1138552/full#supplementary-material.
References
1. Nagai H, Kim YH. Cancer prevention from the perspective of global cancer burden patterns. J Thorac Dis. (2017) 9(3):448–51. doi: 10.21037/jtd.2017.02.75
2. Sung H, Ferlay J, Siegel RL, Laversanne M, Soerjomataram I, Jemal A, et al. Global cancer statistics 2020: GLOBOCAN estimates of incidence and mortality worldwide for 36 cancers in 185 countries. CA Cancer J Clin. (2021) 71(3):209–49. doi: 10.3322/caac.21660
3. Bray F, Ferlay J, Soerjomataram I, Siegel RL, Torre LA, Jemal A. Global cancer statistics 2018: GLOBOCAN estimates of incidence and mortality worldwide for 36 cancers in 185 countries. CA Cancer J Clin. (2018) 68(6):394–424. doi: 10.3322/caac.21492
4. Bruni L, Albero G, Serrano B, Mena M, Gómez D, Muñoz J, et al. ICO/IARC information centre on HPV and cancer (HPV information centre). Human papillomavirus and related diseases in India. Summary Report 17 June 2019. https://hpvcentre.net/statistics/reports/IND.pdf (Accessed 2018).
5. Siegel R, Naishadham D, Jemal A. Cancer statistics, 2013. CA-Cancer J Clin. (2013) 63(1):11–30. doi: 10.3322/caac.21166
6. Cuzick J, Bergeron C, von Knebel DM, Gravitt P, Jeronimo J, Lorincz AT. Newtechnologies and procedures for cervical cancer screening. Vaccine. (2012) 30(Suppl 5):F107–16. doi: 10.1016/j.vaccine.2012.05.088
8. Catarino R, Petignat P, Dongui G, Vassilakos P. Cervical cancer screening in developing countries at a crossroad: emerging technologies and policy choices. World J Clin Oncol. (2015) 6(6):281–90. doi: 10.5306/wjco.v6.i6.281
9. Pramesh CS, Badwe RA, Bhoo-Pathy N., Booth CM, Chinnaswamy G, Dare AJ, et al. Priorities for cancer research in low- and middle-income countries: a global perspective. Nat Med. (2022) 28:649–57. doi: 10.1038/s41591-022-01738-x
10. Sankaranarayanan R. Screening for cancer in low- and middle-income countries. Ann Glob Health. (2014) 80(5):412–7. ISSN 2214-9996, doi: 10.1016/j.aogh.2014.09.014
11. American Cancer Society. Cancer facts & figures 2018. Atlanta: American Cancer Society (2018). Available at: https://www.cancer.org/content/dam/cancer-org/research/cancer-facts-and-statistics/annual-cancer-facts-and-figures/2018/cancer-facts-and-figures-2018.pdf (Accessed November 23, 2022].
12. Choi J, Kim HJ, Jeong YH, Lee JH, Cho A, Yun M, et al. The role of (18) F-FDG PET/CT in assessing therapy response in cervix cancer after concurrent chemoradiation therapy. Nucl Med Mol Imaging. (2014) 48(2):130–6. doi: 10.1007/s13139-013-0248-y
13. Guimaraes MD, Schuch A, Hochhegger B, Gross JL, Chojniak R, Marchiori E. Functional magnetic resonance imaging in oncology: state of the art. Radiol Bras. (2014) 47(2):101–11. doi: 10.1590/S0100-39842014000200013
14. Czernin J, Allen-Auerbach M, Nathanson D, Herrmann K. PET/CT in oncology: current status and perspectives. Curr Radiol Rep. (2013) 1(3):177–90. doi: 10.1007/s40134-013-0016-x
15. Bhatla N, Berek JS, CuelloFredes M, Denny LA, Grenman S, Karunaratne K, et al. Revised FIGO staging for carcinoma of the cervix uteri. Int J GynecolObstet. (2019) 145:129–35. doi: 10.1002/ijgo.12749
16. Rose PG, Bundy BN, Watkins EB, Thigpen JT, Deppe G, Maiman MA, et al. Concurrent cisplatin-based radiotherapy and chemotherapy for locally advanced cervical cancer. N EnglJ Med. (1999) 340:1144–53. doi: 10.1056/NEJM199904153401502
17. Thomas GM. Improved treatment for cervical cancer–concurrent chemotherapy and radiotherapy. N Engl J Med. (1999) 340:1198–200. doi: 10.1056/NEJM199904153401509
18. Kori M, Yalcin Arga K. Potential biomarkers and therapeutic targets in cervical cancer: insights from the meta-analysis of transcriptomics data within network biomedicine perspective. PLoS One. (2018) 13(7):e0200717. Published 2018 July 18. doi: 10.1371/journal.pone.0200717
19. Cohen AC, Roane BM, Leath CA 3rd. Novel therapeutics for recurrent cervical cancer: moving towards personalized therapy. Drugs. (2020) 80(3):217–27. doi: 10.1007/s40265-019-01249-z
20. Hamamoto R, Suvarna K, Yamada M, Kobayashi K, Shinkai N, Miyake M, et al. Application of artificial intelligence technology in oncology: towards the establishment of precision medicine. Cancers. (2020) 12(12):3532. Published 2020 November 26. doi: 10.3390/cancers12123532
21. Machine Learning in MATLAB. Available at: https://in.mathworks.com/help/stats/machine-learning-in-matlab.html (Accessed September 23, 2022).
22. Obermeyer Z, Emanuel EJ. Predicting the future—big data, machine learning, and clinical medicine. N Engl J Med. (2016) 375:1216–9. doi: 10.1056/NEJMp1606181
23. Raghupathi W, Raghupathi V. Big data analytics in healthcare: promise and potential. Health Inf Sci Syst. (2014) 2(1):3. doi: doi: 10.1186/2047-2501-2-3
24. Chartrand G, Cheng PM, Vorontsov E, Drozdzal M, Turcotte S, Pal CJ, et al. Deep learning : a primer for radiologists. RadioGraphics. (2017) 7(7):2113–31. doi: 10.1148/rg.2017170077
25. Erickson BJ, Korfiatis P, Akkus P, Kline TL. Machine learning for medical imaging. RadioGraphics. (2017) 37:505–15. doi: 10.1148/rg.2017160130
26. Tian X, Sun C, Liu Z, Li W, Duan H, Wang L, et al. Prediction of response to preoperative neoadjuvant chemotherapy in locally advanced cervical cancer using multicenter CT-based radiomic analysis. Front Oncol. (2020) 10:77. doi: 10.3389/fonc.2020.00077
27. Fang J, Zhang B, Wang S, Jin Y, Wang F, Ding Y, et al. Association of MRI-derived radiomic biomarker with disease-free survival in patients with early-stage cervical cancer. Theranostics. (2020) 10(5):2284–92. doi: 10.7150/thno.37429
28. Wang T, Gao T, Yang J, Yan X, Wang Y, Zhou X, et al. Preoperative prediction of pelvic lymph nodes metastasis in early-stage cervical cancer using radiomics nomogram developed based on T2-weighted MRI and diffusion-weighted imaging. Eur J Radiol. (2019) 114:128–35. doi: 10.1016/j.ejrad.2019.01.003
29. Lucia F, Visvikis D, Vallières M, Desseroit MC, Miranda O, Robin P, et al. External validation of a combined PET and MRI radiomics model for prediction of recurrence in cervical cancer patients treated with chemoradiotherapy. Eur J Nucl Med Mol Imaging. (2019) 46(4):864–77. doi: 10.1007/s00259-018-4231-9
30. Altazi BA, Fernandez DC, Zhang GG, Hawkins S, Naqvi SM, Kim Y, et al. Investigating multi-radiomic models for enhancing prediction power of cervical cancer treatment outcomes. Phys Med. (2018) 46:180–8. doi: 10.1016/j.ejmp.2017.10.009
31. Chen J, Chen H, Zhong Z, Wang Z, Hrycushko B, Zhou L, et al. Investigating rectal toxicity associated dosimetric features with deformable accumulated rectal surface dose maps for cervical cancer radiotherapy. Radiat Oncol. (2018) 13(1):125. doi: 10.1186/s13014-018-1068-0
32. Rose PG, Java J, Whitney CW, Stehman FB, Lanciano R, Thomas GM, et al. Nomograms predicting progression-free survival, overall survival, and pelvic recurrence in locally advanced cervical cancer developed from an analysis of identifiable prognostic factors in patients from NRG oncology/gynecologic oncology group randomized trials of chemoradiotherapy. J Clin Oncol. (2015) 33(19):2136–42. doi: 10.1200/JCO.2014.57.7122
33. Reuzé S, Orlhac F, Chargari C, Nioche C, Limkin E, Riet F, et al. Prediction of cervical cancer recurrence using textural features extracted from 18F-FDG PET images acquired with different scanners. Oncotarget. (2017) 8(26):43169–79. doi: 10.18632/oncotarget.17856
34. Lucia F, Visvikis D, Desseroit MC, Miranda O, Malhaire JP, Robin P, et al. Prediction of outcome using pretreatment 18F-FDG PET/CT and MRI radiomics in locally advanced cervical cancer treated with chemoradiotherapy. Eur J Nucl Med Mol Imaging. (2018) 45(5):768–86. doi: 10.1007/s00259-017-3898-7
35. Shim SH, Kim DY, Lee SJ, Kim SN, Kang SB, Lee SW, et al. Prediction model for para-aortic lymph node metastasis in patients with locally advanced cervical cancer. Gynecol Oncol. (2017) 144(1):40–5. doi: 10.1016/j.ygyno.2016.11.011
36. Kong TW, Kim J, Son JH, Kang SW, Paek J, Chun M, et al. Preoperative nomogram for prediction of microscopic parametrial infiltration in patients with FIGO stage IB cervical cancer treated with radical hysterectomy. Gynecol Oncol. (2016) 142(1):109–14. doi: 10.1016/j.ygyno.2016.05.010
37. Kim DY, Shim SH, Kim SO, Lee SW, Park JY, Suh DS, et al. Preoperative nomogram for the identification of lymph node metastasis in early cervical cancer. Br J Cancer. (2014) 110(1):34–41. doi: 10.1038/bjc.2013.718
38. Kumar S, Rana ML, Verma K, Singh N, Sharma AK, Maria AK, et al. PrediQt-Cx: post treatment health related quality of life prediction model for cervical cancer patients. PLoS One. (2014) 9(2):e89851. doi: 10.1371/journal.pone.0089851
39. Kang S, Nam BH, Park JY, Seo SS, Ryu SY, Kim JW, et al. Risk assessment tool for distant recurrence after platinum-based concurrent chemoradiation in patients with locally advanced cervical cancer: a Korean gynecologic oncology group study. J Clin Oncol. (2012) 30(19):2369–74. doi: 10.1200/JCO.2011.37.5923
40. Jha AK, Mithun S, Singh AM, Purandare NC, Shah S, Agrawal A, et al. 18-Month Performance assessment of gemini TF 16 PET/CT system in a high-volume department. J Nucl Med Technol. (2016) 44(1):36–41. doi: 10.2967/jnmt.115.168492
41. Plastimatch documentation. https://plastimatch.org/contents.html (Accessed September 23, 2022).
42. Pyradiomics. https://pyradiomics.readthedocs.io/en/latest/index.html (Accessed September 23, 2022).
43. Griethuysen JJM, Fedorov A, Parmar C, Hosny A, Aucoin N, Narayan V, et al. Computational radiomics system to decode the radiographic phenotype. Cancer Res. (2017) 77(21):e104–7. doi: 10.1158/0008-5472.CAN-17-0339
44. Zwanenburg A, Leger S, Vallières M, Löck S. Image biomarker standardisation initiative - feature definitions (2016). In eprint arXiv:1612.07003 [cs.CV].
45. Nick TG, Campbell KM. Logistic regression. Methods Mol Biol. (2007) 404:273–301. doi: 10.1007/978-1-59745-530-5_14
46. Stoltzfus JC. Logistic regression: a brief primer. Acad Emerg Med. (2011) 18(10):1099–104. doi: 10.1111/j.1553-2712.2011.01185.x
49. Nedaie A, Najafi AA. Support vector machine with dirichlet feature mapping. Neural Netw. (2018) 98:87–101. doi: 10.1016/j.neunet.2017.11.006
50. Chao CF, Horng MH. The construction of support vector machine classifier using the firefly algorithm. ComputIntellNeurosci. (2015) 2015:212719. doi: 10.1155/2015/212719
51. Natekin A, Knoll A. Gradient boosting machines, a tutorial. Front Neurorobot. (2013) 7:21. Published 2013 December 4. doi: 10.3389/fnbot.2013.00021
52. Friedman J. Greedy boosting approximation: a gradient boosting machine. Ann. Stat. (2001) 29:1189–232. doi: 10.1214/aos/1013203451
53. Jha AK, Mithun S, Jaiswar V, Sherkhane UB, Purandare NC, Prabhash K, et al. Repeatability and reproducibility study of radiomic features on a phantom and human cohort. Sci Rep. (2021) 11(1):2055. Published 2021 January 21. doi: 10.1038/s41598-021-81526-8
54. Parvandeh S, Yeh HW, Paulus MP, McKinney BA. Consensus features nested cross-validation. Bioinformatics. (2020) 36(10):3093–8. doi: 10.1093/bioinformatics/btaa046
55. Yang J, Tian G, Pan Z, Zhao F, Feng X, Liu Q, et al. Nomograms for predicting the survival rate for cervical cancer patients who undergo radiation therapy: a SEER analysis. Future Oncol. (2019) 15(26):3033–45. doi: 10.2217/fon-2019-0029
56. Marchetti C, De Felice F, Di Pinto A, Romito A, Musella A, Palaia I, et al. Survival nomograms after curative neoadjuvant chemotherapy and radical surgery for stage IB2-IIIB cervical cancer. Cancer Res Treat. (2018) 50(3):768–76. doi: 10.4143/crt.2017.141
57. Lee WK, Chong GO, Jeong SY, Lee HJ, Park SH, Ryu JM, et al. Prognosis-predicting model based on 18F fluorodeoxyglucose PET metabolic parameters in locally advanced cervical cancer patients treated with concurrent chemoradiotherapy: multi-center retrospective study. J Clin Med. (2020) 9(2):427. doi: 10.3390/jcm9020427
58. Zheng RR, Huang XW, Liu WY, Lin RR, Zheng FY, Lin F. Nomogram predicting overall survival in operable cervical cancer patients. Int J Gynecol Cancer. (2017) 27(5):987–93. doi: 10.1097/IGC.0000000000000987
59. Shim SH, Lee SW, Park JY, Kim YS, Kim DY, Kim JH, et al. Risk assessment model for overall survival in patients with locally advanced cervical cancer treated with definitive concurrent chemoradiotherapy. Gynecol Oncol. (2013) 128(1):54–9. doi: 10.1016/j.ygyno.2012.09.033
60. Zhou H, Li X, Zhang Y, Jia Y, Hu T, Yang R, et al. Establishing a nomogram for stage IA-IIB cervical cancer patients after complete resection. Asian Pac J Cancer Prev. (2015) 16(9):3773–7. doi: 10.7314/APJCP.2015.16.9.3773
61. Kidd EA, El Naqa I, Siegel BA, Dehdashti F, Grigsby PW. FDG-PET-based prognostic nomograms for locally advanced cervical cancer. Gynecol Oncol. (2012) 127(1):136–40. doi: 10.1016/j.ygyno.2012.06.027
62. Seo Y, Yoo SY, Kim MS, Yang KM, Yoo HJ, Kim JH, et al. Nomogram prediction of overall survival after curative irradiation for uterine cervical cancer. Int J Radiat Oncol Biol Phys. (2011) 79(3):782–7. doi: 10.1016/j.ijrobp.2009.11.054
63. Biewenga P, van der Velden J, Mol BW, Stalpers LJ, Schilthuis MS, van der Steeg JW, et al. Prognostic model for survival in patients with early stage cervical cancer. Cancer. (2011) 117(4):768–76. doi: 10.1002/cncr.25658
64. Tseng JY, Yen MS, Twu NF, Lai CR, Horng HC, Tseng CC, et al. Prognostic nomogram for overall survival in stage IIB-IVA cervical cancer patients treated with concurrent chemoradiotherapy. Am J Obstet Gynecol. (2010) 202(2):174.e1–7. doi: 10.1016/j.ajog.2009.09.028
65. Polterauer S, Grimm C, Hofstetter G, Concin N, Natter C, Sturdza A, et al. Nomogram prediction for overall survival of patients diagnosed with cervical cancer. Br J Cancer. (2012) 107(6):918–24. doi: 10.1038/bjc.2012.340
66. Lee HJ, Han S, Kim YS, Nam JH, Kim HJ, Kim JW, et al. Individualized prediction of overall survival after postoperative radiation therapy in patients with early-stage cervical cancer: a Korean radiation oncology group study (KROG 13-03). Int J Radiat Oncol Biol Phys. (2013) 87(4):659–64. doi: 10.1016/j.ijrobp.2013.07.020
Keywords: cervical cancer, FIGO, prediction model, radiomics, machine learning
Citation: Jha AK, Mithun S, Sherkhane UB, Jaiswar V, Shah S, Purandare N, Prabhash K, Maheshwari A, Gupta S, Wee L, Rangarajan V and Dekker A (2023) Development and validation of radiomic signature for predicting overall survival in advanced stage cervical cancer. Front. Nucl. Med. 3:1138552. doi: 10.3389/fnume.2023.1138552
Received: 5 January 2023; Accepted: 3 May 2023;
Published: 17 May 2023.
Edited by:
Stefano Trebeschi, The Netherlands Cancer Institute (NKI), NetherlandsReviewed by:
Sean Benson, The Netherlands Cancer Institute (NKI), NetherlandsMarjaneh Taghavi, Wageningen University and Research, Netherlands
© 2023 Jha, Mithun, Sherkhane, Jaiswar, Shah, Purandare, Prabhash, Maheshwari, Gupta, Wee, Rangarajan and Dekker. This is an open-access article distributed under the terms of the Creative Commons Attribution License (CC BY). The use, distribution or reproduction in other forums is permitted, provided the original author(s) and the copyright owner(s) are credited and that the original publication in this journal is cited, in accordance with accepted academic practice. No use, distribution or reproduction is permitted which does not comply with these terms.
*Correspondence: Ashish Kumar Jha ashish.kumar.jha.77@gmail.com, a.jha@maastrichtuniversity.nl
†These authors share senior authorship