- 1AEF/Systems Physiology, Heart Failure Research Center, Academic Medical Center at the University of Amsterdam, Amsterdam, Netherlands
- 2Vascular Medicine, Academic Medical Center at the University of Amsterdam, Amsterdam, Netherlands
Background: A sympathetic shift in heart rate variability (HRV) from high to lower frequencies may be an early signal of deterioration in a monitored patient. Most chronic heart failure (CHF) patients receive β-blockers. This tends to obscure HRV observation by increasing the fast variations. We tested which HRV parameters would still detect the change into a sympathetic state.
Methods and results: β-blocker (Carvedilol®) treated CHF patients underwent a protocol of 10 min supine rest, followed by 10 min active standing. CHF patients (NYHA Class II–IV) n = 15, 10m/5f, mean age 58.4 years (47–72); healthy controls n = 29, 18m/11f, mean age 62.9 years (49–78). Interbeat intervals (IBI) were extracted from the finger blood pressure wave (Nexfin®). Both linear and non-linear HRV analyses were applied that (1) might be able to differentiate patients from healthy controls under resting conditions and (2) detect the change into a sympathetic state in the present short recordings.
Linear: mean-IBI, SD-IBI, root mean square of successive differences (rMSSD), pIBI-50 (the proportion of intervals that differs by more than 50 ms from the previous), LF, HF, and LF/HF ratio.
Non-linear: Sample entropy (SampEn), Multiscale entropy (MSE), and derived: Multiscale variance (MSV) and Multiscale rMSSD (MSD). In the supine resting situation patients differed from controls by having higher HF and, consequently, lower LF/HF. In addition their longer range (τ = 6–10) MSE was lower as well. The sympathetic shift was, in controls, detected by mean-IBI, rMSSD, pIBI-50, and LF/HF, all going down; in CHF by mean-IBI, rMSSD, pIBI-50, and MSD (τ = 6–10) going down. MSD6–10 introduced here works as a band-pass filter favoring frequencies from 0.02 to 0.1 Hz.
Conclusions: In β-blocker treated CHF patients, traditional time domain analysis (mean-IBI, rMSSD, pIBI-50) and MSD6–10 provide the most useful information to detect a condition change.
Introduction
Heart rate variability (HRV) analysis has seen an increasing interest since the early work of B.McA. Sayers in the 1970's (Hyndman et al., 1971), picking up speed since the 1980's (Akselrod et al., 1981; Baselli et al., 1987; deBoer et al., 1987). However, these analysis techniques still have not made it to the bedside, probably due to the fact that equipment manufacturers do not offer standard solutions in ECG-monitors to provide intricate HRV-data. Only recently, some HRV-analysis methods have sneaked into the clinic by a “stealth” method, hidden in algorithms that give a generalized “alert value” to a patient condition, based on observation of a series of vital parameters (Clark et al., 2012; Lee et al., 2012; Morris et al., 2007).
In the present study we tried a clinical approach to a problem that has received little attention in biomedical literature. It has been fairly well-established that chronic heart failure patients (CHF) have different HRV patterns compared to matched healthy controls (Saul et al., 1988; Casolo et al., 1989). However, many of those patients will be on β-blocker therapy, which has a strong influence on both HR and HRV (Coumel et al., 1991; Pousset et al., 1996; Mortara et al., 2000; Kubo et al., 2005). Under these circumstances HRV has been shown to improve toward normal (Mortara et al., 2000; Lin et al., 2001), a fact that might mask an underlying developing disease status. We therefore tested which HRV-analysis technique might be able to give early warning when there is a “slipping of” in the sympathetic direction of the autonomic balance. To test this we used recordings in CHF patients who went from supine to upright, as a simple model to reduce vagal outflow to the heart and induce generalized sympathetic activation. For this study we had a set of 21 recordings in CHF-patients on Carvedilol® treatment that has been fully described earlier (Truijen et al., 2011). In view of the practical applicability in situations where a diagnosis should be available after a short period of recording only, we were wondering if the 10 min in supine posture followed by 10 min upright that we had were sufficient to answer two questions:
- Is there still a distinction health/disease when comparing the supine recordings in β-blocker treated CHF patients to those from matched, healthy controls?
- Can HRV-analysis demonstrate an intra-individual shift toward a more sympathetic state when comparing the upright to the supine recording, even in this group of β-blocker treated patients?
The first question is of importance for a quick triage of patients, the second to detect a deterioration of a patient's health before a “normal” alarm would sound.
We decided to test a number of obvious linear measures, from the time domain: mean-IBI (interbeat interval), SD-IBI (standard deviation), rMSSD (root mean square of successive differences of IBI's), pIBI-50 (proportion of pairs of successive IBI's that differ by more than 50 ms) and from the frequency domain: LF, HF and LF/HF (Low Frequency around 0.1 Hz, High Frequency, i.e., respiratory frequency, mostly around 0.25 Hz, and their quotient). In view of earlier studies where the use of short recordings for non-linear analysis has been analyzed (Batchinsky et al., 2009; Lake and Moorman, 2011; Leistedt et al., 2011; Turianikova et al., 2011), we decided to use SampEn [sample entropy (Richman and Moorman, 2000)] and Multiscale Entropy (MSE; Costa et al., 2002). The latter method computes entropy over progressively coarser grained versions of the original series. As a by-product we considered the variances (Multiscale variance or MSV) and multiscale root mean square of successive differences (MSD) of those newly constructed series as well.
A successful analysis method or combination of methods should be able to do the triage (Question 1) as well as detect the sympathetic shift (Question 2) within the limits of the 20 min recordings that were available.
Methods
Study Population
Patients
The patient recordings had been made in the study that has extensively been described in (Truijen et al., 2011). In short: 21 CHF patients (NY Heart Assoc. classification II–IV) participated in a study that was directed to discrimination of β-blocker sensitivity depending on the specific β2-receptor subtype that was present in the patient. In a double-blind cross-over design they received the non-selective β-blocker Carvedilol® (Eucardic, Roche, Mijdrecht, Netherlands) or the selective metoprolol succinate (Selokeen ZOC, AstraZeneca, Zoetermeer, Netherlands) as β-blocker for 6 weeks. Both drugs were titrated to equipotent dosages, additionally checked by resting heart rate. Since recordings made under Carvedilol showed fewer extrasystoles and other rhythm disturbances, we have restricted our study to the recordings made after 6 weeks on this drug. It should be mentioned here that Carvedilol is known to also have α1-blocking properties, without intrinsic sympathetic activity (Eggertsen et al., 1984).
Patients had given their written informed consent after study approval by the local Ethics committee. Due to problems with too frequent premature ventricular contractions and erroneous blood pressure tracings, 6 out of the original 21 patient recordings (Truijen et al., 2011) under Carvedilol had to be rejected. This left 15 CHF patients for the present study, 10/5 (male/female), age 58.4 ± 6.5 (mean ± SD), BMI 27.4 ± 6.0.
Healthy control subjects
Subjects had been recruited by advertisement and selected to match the patient group by gender, age and β2-receptor subtype. They were in good health, free of cardiovascular disease, non-smokers. After written, informed consent 34 subjects participated. Due to technical problems in the recordings and 2 cases of near-syncope in the stand-test, 5 out of the original 34 control recordings had to be rejected. This left 29 (18/11, m/f), age 62.9 ± 7.3 BMI 26.1 ± 4.2 for analysis. There are no significant differences in age and BMI distribution between healthy controls and CHF patients.
Measurements and Data Preprocessing
Continuous non-invasive blood pressure was measured from a finger by the volume-clamp technique. A Nexfin® (BMEYE, Amsterdam, Netherlands) hemodynamic monitor was used with instantaneous display of reconstructed upper arm blood pressure, heart rate, pulse contour derived cardiac output and systemic vascular resistance. This enabled proper monitoring during the stand test. To prevent hydrostatic errors the hand was held at heart level in both positions by a sling around the neck.
Patients and controls underwent a test protocol which included blood draws for clotting factors in the supine position as described earlier (Truijen et al., 2011). Then they rested for at least 20 min before actively standing up. They remained standing for another 10 min. From the Nexfin computed data we only analysed IBI values for the present study, measured to an accuracy of 5 ms (200 Hz sample rate of A/D conversion).
In view of dysrhythmias like PVC's, other rhythm disturbances and, occasionally, movement artifacts that were present in the IBI-recordings, they had to be pre-processed before analyses could be performed. We used a two-step spike-removal procedure. First we established the global mean value IBImean−glb of the whole set (supine or upright), and substituted any IBIi outside the range 80–120% of IBImean−glb by that value. Next, a 10-beat window would slide over the recording, replacing any newly added IBIj outside the 80–120% range around the IBImean−local by the value of the local mean. The first step deletes sharp spikes globally, making it easier for the second step which is required to preserve continuity of the time series.
Calculation of HRV Parameters
Linear methods
Since we derived heart periods from blood pressure recordings rather than from an ECG, we cannot call them NN-intervals (normal to normal) since, strictly spoken, we have no information on the origin of the heartbeat, whether it originates from the sinus node or from some other pacemaking site in the heart. Although all patients underwent a test-ECG just prior to the present recording, where normal sinus rhythm had been established, we will use the more general term “IBI” (interbeat interval) instead.
After data pre-processing as described above we calculated mean-IBI, SD-IBI, rMSSD, pIBI-50 (the proportion of intervals that differs by more than 50 ms from the previous) following the usual methods (Task Force of the European Society of Cardiology, and the North American Society of Pacing and Electrophysiology, 1996). We chose a period of at least 5 min stable recording for both the supine and upright periods. Of the upright recording a period of 2 min after the standing up maneuver was skipped, to allow for the first transient in blood pressure and heart rate to disappear. This left a period of maximally 8 min upright to be included in the computations.
For the frequency analysis we used the IBI data set without interpolation, putting the average interbeat interval as spacing between heart beats (deBoer et al., 1984). After removal of a linear trend and Hanning-windowing we applied a digital Fourier transform (Matlab®) rather than FFT. This method can be applied to an arbitrary number of data points without the need of zero-padding until a power of two has been reached. LF, HF, and LF/HF ratio were computed after integration of the spectral curve from 0.04 to 0.15 Hz for LF and from 0.15 to 0.4 Hz for HF. The values were reduced to normalized units by division by the total variance (Task Force, 1996).
Non-linear methods: SampEn and MSE
The calculation of MSE has been fully described in (Costa et al., 2005). It is the sample entropy (SampEn) (Richman and Moorman, 2000) of consecutively coarser grained time series Y constructed from the original time series X:{x1,…, xi,…, xN} by a scale factor of τ.
The coarse-graining procedure is the first step to compute MSE, as well as MSV and multiscale successive differences (MSD). By taking τ consecutive values together, the original signal is progressively “smoothed” and more and more beat-to-beat “noise” is averaged out. This process is visualized in Figure 1 and formalized in formula 1:
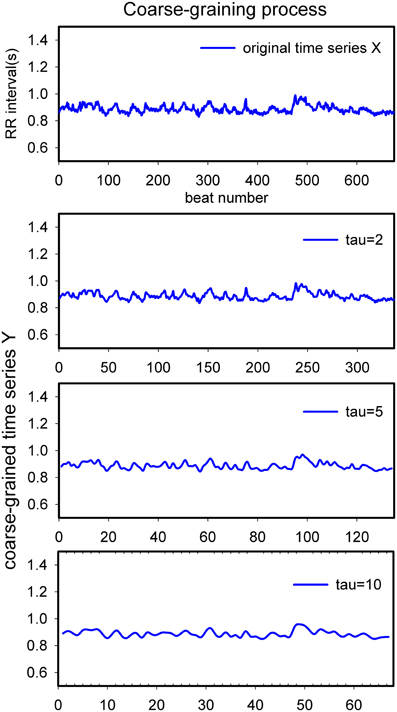
Figure 1. Schematic diagram of the process of coarse-graining. This is a representative ~10 min heart rate recording from a healthy volunteer in supine position. From top to bottom tau = 1, 2, 5, 10. X-scale: item number in the series; Y-scale: (averaged) duration of heart periods in seconds.
This describes a set of consecutively more coarse-grained time series, [y(τ)] from the series X, where τ is the scale factor. Next, the SampEn (Richman and Moorman, 2000) of each time series[y(τ)] is computed, resulting in MSE. SampEn is a measure of the probability that a sequence of m consecutive data points will not remain similar (within a given tolerance r) at the next point in the data set. A high SampEn value implies low regularity, i.e., few repetitions of the same pattern. Details on how to calculate SampEn can be found in references (Richman and Moorman, 2000; Costa et al., 2002; Xinnian et al., 2006).
In short, MSE aims to measure the complexity of the system. In a totally random sequence, SampEn will decrease to zero with increasing τ; in a sequence that has been generated by a system with some degree of complexity, like heart rate over time, it tends to find a stable non-zero value.
Linear extension: MSV and MSD
We also computed a side-product of the coarse-graining process, i.e., the (multiscale) variance (MSV) and multiscale rMSSD (MSD) of the newly constructed series Y(τ). We reasoned that these might show in a simple way the variability of heart rate at medium-scale time-intervals.
Statistics
All computations were done by use of SPSS®. After testing for normality, comparisons between groups were done by pairwise testing using Student's t-test or Mann–Whitney u-test where appropriate. For the change to a sympathetic state within one subject, we used the computed supine value to normalize the upright value. The resulting quotient upright/supine was then linearized by a log-transformation before statistical testing.
To compensate for the multiple comparisons we adapted the test magnitude alpha by applying the Bonferroni–Holm correction. This will lead to a value of alpha smaller than the 0.05 that we considered significant. The corrected alpha is mentioned in the tables along with the computed exact p-value. This allows the reader to judge the significance of observed changes, taking into account the type I/type II error as well as the biological significance of the change (Cabin and Mitchell, 2000; Nakagawa, 2004).
Results
Comparison CHF Patients vs. Healthy Controls
Table 1 gives an overview of the chosen 11 HRV parameters in patients and control subjects in the supine posture. It is remarkable that the mean supine heart rates, pIBI-50 and rMSSD in the two groups are equal despite the β-blockade in the patients. Total variability as expressed by SD-IBI is lower in CHF, although not statistically significant. HF as short term variability index is higher in CHF, but this may well be due to the β-blockade (Goldsmith et al., 1997; Witte and Clark, 2008). As a consequence LF/HF is significantly lower in CHF as well.
To further analyze the internal structure of the variability we computed, first, the MSE-curves for τ = 1–10, results shown in Figure 2A. At τ = 1 SampEn in CHF and controls are equal (Table 1). For values of τ above 3 the curve of the CHF-patients falls below that of the healthy controls. However, a large overlap exists. To emphasize the longer range interactions rather than the short-term variability (Ho et al., 2011) we integrated the values for τ from 6 to 10, resulting in the MSE6−10 number in Table 1. The variances of the coarse grained distributions for increasing τ are depicted by the MSV as shown in Figure 2B. Average MSV in CHF is lower than that in controls for all values of τ; we averaged the value for τ= 6–10 as MSV6−10 in Table 1. Except for τ = 1 the same holds true for the rMSSD of the coarse grained distributions, expressed as MSD in Figure 2C and averaged to one value from τ = 6 to 10 in Table 1. Both MSV6−10 and MSD6−10 are lower in CHF than in controls, however, in view of the wide distribution of the numbers, taking the Bonferroni–Holm corrected alpha and the magnitude of the difference into account, we do not consider these differences biologically significant. The same cannot be said for MSE6−10: although the number fails to meet the Bonferroni–Holm corrected alpha (p = 0.05/9 = 0.006), yet in view of the narrow distribution and the exact p-value of 0.015 we do consider this difference significant.
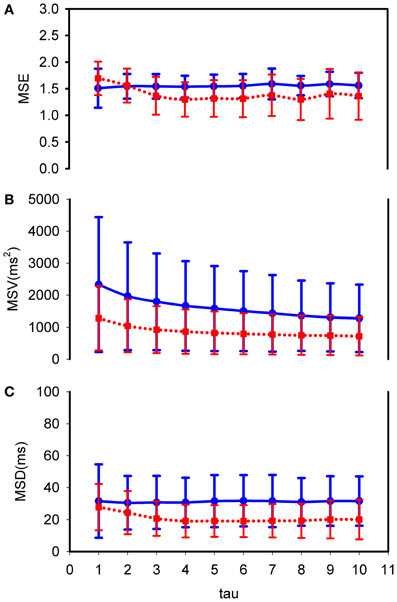
Figure 2. MSE, MSV, and MSD curves: comparison between control subjects and CHF patients in supine posture. (A) MSE; (B) MSV; (C) MSD. Fat (blue) line: control healthy subjects; dotted (red) line: CHF patients. Tau from 1 to 10. The curves represent mean values with ±1 standard deviation. CHF patients have lower MSE, MSV, and MSD than healthy subjects for tau above 2.
Sympathetic Shift in Control Subjects: Upright vs. Supine Posture
We computed the 11 parameters again for the upright condition, and expressed the upright value as fraction of the individual supine one. For statistical testing the values have been log-transformed, to obtain values which follow a normal distribution with mean = 0 if the supine and upright values are equal. The averages and p-values are shown in Table 2.
As to be expected, mean-IBI has significantly decreased, i.e., heart rate goes up on standing. All short-term variability measures go down as well: rMSSD, pIBI-50 and HF. The increase in LF did not reach statistical significance; LF/HF increased in line with the decreased HF. SampEn and the coarse grained measures, MSE6−10, MSV6−10, and MSD6−10 did not change.
Sympathetic Shift in CHF Patients: Upright vs. Supine Posture
Generally speaking, the sympathetic change in CHF-patients followed the same pattern as in healthy control subjects, without statistically significant differences between the two groups. However, there were a few notable within-group exceptions, as shown in Table 2.
In the patients mean-IBI decreased significantly, so did rMSSD, pIBI-50, but none of the frequency analysis measures. Of the non-linear and coarse-grained parameters only MSD6−10 decreased significantly, the other ones did not change appreciably.
Absolute Power Results from Fourier Analysis
Fourier analysis of HRV may be analyzed in many ways; we chose to look at the individual normalized powers of LF and HF and the LF/HF quotients. However, if the underlying (absolute) data are very much changed by the intervention (standing up in this case) these numbers may be misleading. Therefore, we checked the absolute powers and in addition those in the VLF band (very low frequency band: from the lowest observed frequency in the 5–10 min recording to 0.04 Hz). The results are given in Table 3. No significant changes in total power or power in the various bands with standing were detectable. These might have invalidated the above analyses.
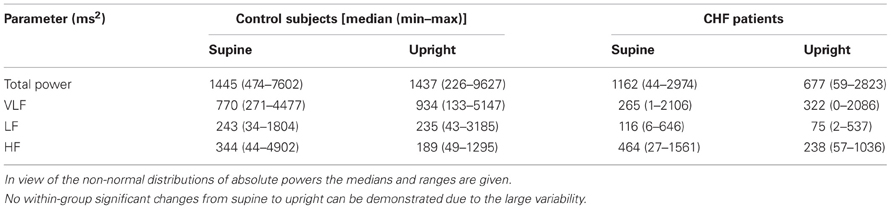
Table 3. Total power and power in the various bands: VLF, LF, HF; supine values compared to upright.
Discussion
The present computer-based post-hoc study tried to establish the numbers that could aid in fast diagnosis of a β-blocker treated patient's “slipping off” into a more sympathetic state. We chose a stand-test as model for this condition; no one can stand very well for 10 min without sympathetic system involvement in view of the induced drop in blood pressure at the level of the carotid sinuses and the relative hypovolemia that is observed by pressure sensitive receptors in the low-pressure area (atria, lungs) (Borst et al., 1982; Ten Harkel et al., 1993).
We reasoned that patients on a β-blocker, when remotely monitored or admitted to an intensive care unit for acute exacerbation of symptoms, might pose additional challenges to a monitoring system that would incorporate HRV-measures in an intelligent alarm. Beta-blockers have a tendency to increase short term HRV as well as total background variability as it may be observed in the low to very low frequency ranges (Goldsmith et al., 1997; Aronson and Burger, 2001; Bullinga et al., 2005). Moreover, the additional α1-blocking properties of Carvedilol may lead to less apparent blood pressure waves when sympathetic arousal takes place. This property has been pointed out as instrumental in not lowering HR as much as do other β-blockers (Stoschitzky et al., 2001), as compensation for the decreased systemic resistance that it provokes (Ferrua et al., 2005).
In line with our initial suppositions we found that in the supine resting state the CHF-patients differed from the healthy control subjects by showing equal HR with almost equal SD-IBI, but significantly higher HF variability, therefore lower LF/HF ratio. Furthermore, the patients had slightly lower values for MSE6−10, MSV6−10, and MSD6−10. In our view this is mirroring the increased beat-to-beat variability due to the β-blocker together with a slightly increased sympathetic activation. When going to the upright posture the control subjects displayed most of the expected changes: increased HR, decreased rMSSD, pIBI-50, HF, increased LF/HF, but not an increased LF, probably due to the large variance in this measure. MSE6−10, MSD6−10, or MSV6−10 did not record a change. When it came to the CHF-patients in upright posture the parameters that did show up as useful were HR (increased), rMSSD, pIBI-50 and MSD6−10 (decreased). None of the other parameters would indicate a shift into a sympathetic state. Our results in the healthy control group tally well with those of Turianikova et al. (2011) who recently published a comparable orthostasis study. Exception is our lack of results for MSE6−10; in comparison we would have expected a definite increase. However, we studied subjects around 63 years of age, the earlier study had subjects around 20 years of age.
A few notes should be made; the most important one being that almost all HRV is vagally mediated: both the fast beat-to-beat changes and the slower waves that may be riding on underlying blood pressure variations. As long as heart rate is in the vagal range, for humans below (120−0.6× age) (Karemaker et al., 1989), most variations in HR will, as first guess, mainly come from changes in vagal activity. That is not to say that the sympathetics play no role in HRV, their contribution can be found both in the underlying blood pressure variability and the longer-range variations in heart rate. These slower variations, to be observed over the course of minutes to hours, may influence both HR and BP at the same time, via central and peripheral mechanisms. In analysis techniques that aim at this “system complexity” it has become established that stable estimates may only be found when thousands of heart beats are incorporated, an order of magnitude requiring at least some 4 h observation. This is a requirement that is impractical for straightforward clinical monitoring. Although 4 h of data may become available in any patient on the monitor, a deterioration of condition should be signaled earlier than after 4 h.
In recent years the literature on HRV analysis methods has been reviewed for various areas of application. Rajendra Acharya et al. (2006) gave a more or less complete overview of methodologies that are applied, from time domain to frequency domain to non-linear analysis methods. Generally speaking, the main disadvantages of the latter are the large number of data required and the sensitivity to baseline shift and noise of some of the methods. In 2007 Maestri et al. (2007) reviewed the use of non-linear indices of HRV for CHF patients. Many were highly correlated to classical linear indices; only two (families of) analysis methods gave independent prognostic information, i.e., empirical mode decomposition and symbolic dynamics. We considered these not practical for our purpose. Again in 2009 Buccelletti et al. (2009) noted that techniques like power law (fractal) analysis or detrended fluctuation analysis were less practical in the prognosis of myocardial infarction patients than entropy directed methods in view of the number of required heart beats. In the present study we have, therefore, restricted our analysis to SampEn and MSE, being the most promising ones for our application. We looked at scale factors 6–10 for MSE, supported by a recent study by Ho et al. (2011) who had noticed that in CHF patients on β-blockade values of τ = 6 and up were insensitive to this therapy when used as predictors of mortality.
In short, the most reliable HRV parameters to early detect a patient's “slipping off” into a sympathetic state are those that indicate so-called vagal withdrawal, i.e., the disappearance of short-term variability and the loss of longer term “jumpiness” as shown by the decreased MSD6−10. These changes will occur even before heart rate goes up into a definite sympathetic region, where all vagal efferent traffic is silenced. The newer parameters like SampEn or MSE have no use here, at least not in the present group of patients who use β-blockers. A study by Batchinsky et al. (2009) has shown that SampEn can make a difference for triage in emergency care, even when only short recordings are available. Interestingly, the newly introduced parameter MSD6−10 seems to do a good job as well, detecting both the sympathetic shift and the difference between healthy controls and CHF-patients. This computes the “jumpiness” or rMSSD of coarse grained averages over 6–10 adjacent beats. This is not a non-linear parameter like MSE or SampEn, but one that is derived from the intermediate coarse grained series constructed for the computation of MSE. In that same vein we computed the variances of these series, which showed some promise in the controls-CHF comparison of Table 1, but failed to show a sympathetic shift (in Table 2).
rMSSD has peculiar properties, acting as a high-pass filter to the original heart rate signal. It has been proven (Berntson et al., 2005) that “classical” rMSSD captures the same frequency range as the HF band in frequency analysis does, roughly between 0.2 and 0.45 Hz. However, it is biased by the prevailing heart rate and is sensitive to lower frequencies as well. By extending the algorithm to progressively more coarse grained series of heart periods we have, with MSD6−10, constructed a combination of a low-pass filter (the coarse-graining process, cf. Figure 1) followed by a high-pass filter. Building on the earlier study into the rMSSD filter properties one may extrapolate that the number represented by MSD6−10 will favor frequencies between 0.02 and 0.07 Hz (i.e., 0.2/10 and 0.45/6 as 3dB points), thus mainly spanning the LF-band and slightly lower, as illustrated in Figure 3, the result of a simulation like in the Berntson study. It should be noted that these filter characteristics are dependent on the prevailing heart rate. In the present simulation, as in our study, we assumed an average heart rate of 60/min, 1 s intervals. This problem of scaling by heart rate is one that is omnipresent in MSE-studies, although very seldom mentioned.
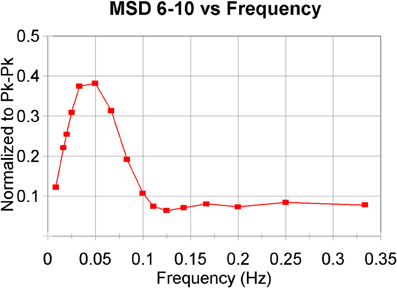
Figure 3. Band-pass filter characteristics of MSD6–10. The algorithm has been applied to model-generated beat-series with additional noise. A simplified model of baroreflex control has been used as in deBoer et al., 1987 to generate the intervals. Average heart period around 1000 ms. A modulating “respiratory” frequency was forced with periods from 3 to 120 s. The values for MDS6–10 have been normalized to the peak–peak amplitudes of the forced oscillations (Berntson et al., 2005).
In Conclusion
For patients on β-blockers only the gradual disappearance of short-term variability, as measured by traditional methods rMSSD and pIBI-50, proved a reliable indicator of a shift to a sympathetic state. The newly introduced MSD6−10—jumpiness in coarse grained beat series—shows some promise here as well. HRV analysis cannot work in clinical monitoring without taking HR-active medication into account; without β-blockade more parameters might be useful, notably SampEn and frequency analysis may carry useful information for the clinician. The best application for these measures is probably their use in intelligent monitoring, where the clinician is not bothered with the numbers and their intricacies, but just with the condition changes that are shown by analysis of HRV along with other vital parameters.
Limitations
This study was conducted in a small number of relatively healthy CHF-patients: they had been stable on their medication before entering the study. The circumstances for patients admitted to the ICU for an acute cardiac condition may be quite different. Therefore, this study should be extended to real-life ICU-recordings and to larger groups of patients before definite conclusions about the usefulness of the present HRV-measures may be reached.
In a standard ICU-setting one would turn to the ECG-monitor for more accurate heart period detection than was possible here. The use of a 200 Hz sampled BP-recording limits the accuracy to ~5 ms, whereas normally at least 1 ms should be obtainable. Therefore, some measures that came out as rather insensitive now, like SampEn or MSE for low values of τ might perform better then. For “classical” MSE the present recordings were too short anyway, reason why we limited the τ (coarse graining parameter) to 10 rather than going to 20. At τ = 10 we have in a 10 min recording about 60 points for the coarse grained series. Since our τ-MSE curves reached stable levels for the data sets that we used, we considered this choice appropriate.
Disclosures
None of the authors has any relevant disclosures to make. Ms. Zhang, PhD is the recipient of a post-doc training grant from the Department of Physiology.
Conflict of Interest Statement
The authors declare that the research was conducted in the absence of any commercial or financial relationships that could be construed as a potential conflict of interest.
Abbreviations
BMI, body mass index; CHF, chronic heart failure; HF, high frequency; HRV, heart rate variability; IBI, interbeat interval; ICU, Intensive Care Unit; LF, low frequency; MSD, multiscale root mean square of successive differences; MSE, Multi-Scale Entropy; MSV, multiscale variance; PVC, premature ventricular contraction; rMSSD, root mean square of successive differences of IBI's; pIBI-50, proportion of pairs of successive IBI's that differed by more than 50ms; SampEn, sample entropy; SD-IBI, standard deviation of interbeat intervals; VLF, very low frequency.
References
Akselrod, S., Gordon, D., Ubel, F. A., Shannon, D. C., Berger, A. C., and Cohen, R. J. (1981). Power spectrum analysis of heart rate fluctuation: a quantitative probe of beat-to-beat cardiovascular control. Science 213, 220–222.
Aronson, D., and Burger, A. J. (2001). Effect of beta-blockade on heart rate variability in decompensated heart failure. Int. J. Cardiol. 79, 31–39.
Baselli, G., Cerutti, S., Civardi, S., Lombardi, F., Malliani, A., Merri, M., et al. (1987). Heart rate variability signal processing: a quantitative approach as an aid to diagnosis in cardiovascular pathologies. Int. J. Biomed. Comput. 20, 51–70.
Batchinsky, A. I., Salinas, J., Kuusela, T., Necsoiu, C., Jones, J., and Cancio, L. C. (2009). Rapid prediction of trauma patient survival by analysis of heart rate complexity: impact of reducing data set size. Shock 32, 565–571.
Berntson, G. G., Lozano, D. L., and Chen, Y. (2005). Filter properties of root mean square successive difference (RMSSD) for heart rate. Psychophysiology 42, 246–252.
Borst, C., Wieling, W., van Brederode, J. F., Hond, A., de Rijk, L. G., and Dunning, A. J. (1982). Mechanisms of initial heart rate response to postural change. Am. J. Physiol. 243, H676–H681.
Buccelletti, F., Gilardi, E., Scaini, E., Galiuto, L., Persiani, R., Biondi, A., et al. (2009). Heart rate variability and myocardial infarction: systematic literature review and metanalysis. Eur. Rev. Med. Pharmacol. Sci. 13, 299–307.
Bullinga, J. R., Alharethi, R., Schram, M. S., Bristow, M. R., and Gilbert, E. M. (2005). Changes in heart rate variability are correlated to hemodynamic improvement with chronic carvedilol therapy in heart failure. J. Card. Fail. 11, 693–699.
Cabin, R. J., and Mitchell, R. J. (2000). To Bonferroni or not to Bonferroni: when and how are the questions. Bull. Ecol. Soc. Am. 81, 246–248.
Casolo, G., Balli, E., Taddei, T., Amuhasi, J., and Gori, C. (1989). Decreased spontaneous heart rate variability in congestive heart failure. Am. J. Cardiol. 64, 1162–1167.
Clark, M. T., Rusin, C. G., Hudson, J. L., Lee, H., Delos, J. B., Guin, L. E., et al. (2012). Breath-by-breath analysis of cardiorespiratory interaction for quantifying developmental maturity in premature infants. J. Appl. Physiol. 112, 859–867.
Costa, M., Goldberger, A. L., and Peng, C. K. (2002). Multiscale entropy analysis of complex physiologic time series. Phys. Rev. Lett. 89, 068102.
Costa, M., Goldberger, A. L., and Peng, C. K. (2005). Multiscale entropy analysis of biological signals. Phys. Rev. E 71, 021906.
Coumel, P., Hermida, J. S., Wennerblöm, B., Leenhardt, A., Maison-Blanche, P., and Cauchemez, B. (1991). Heart rate variability in left ventricular hypertrophy and heart failure, and the effects of beta-blockade. A non-spectral analysis of heart rate variability in the frequency domain and in the time domain. Eur. Heart J. 12, 412–422.
deBoer, R. W., Karemaker, J. M., and Strackee, J. (1984). Comparing spectra of a series of point events particularly for heart rate variability data. IEEE Trans. Biomed. Eng. 31, 384–387.
deBoer, R. W., Karemaker, J. M., and Strackee, J. (1987). Hemodynamic fluctuations and baroreflex sensitivity in humans: a beat-to-beat model. Am. J. Physiol. 253, H680–H689.
Eggertsen, R., Andrén, L., Sivertsson, R., and Hansson, L. (1984). Acute haemodynamic effects of carvedilol (BM 14190), a new combined beta-adrenoceptor blocker and precapillary vasodilating agent, in hypertensive patients. Eur. J. Clin. Pharmacol. 27, 19–22.
Ferrua, S., Bobbio, M., Catalano, E., Grassi, G., Massobrio, N., Pinach, S., et al. (2005). Does carvedilol impair insulin sensitivity in heart failure patients without diabetes? J. Card. Fail. 11, 590–594.
Goldsmith, R. L., Bigger, J. T., Bloomfield, D. M., Krum, H., Steinman, R. C., Sackner-Bernstein, J., et al. (1997). Long-term carvedilol therapy increases para-sympathetic nervous system activity in chronic congestive heart failure. Am. J. Cardiol. 80, 1101–1104.
Ho, Y. L., Lin, C., Lin, Y. H., and Lo, M. T. (2011). The prognostic value of non-linear analysis of heart rate variability in patients with congestive heart failure–A pilot study of multiscale entropy. PLoS ONE 6:e18699. doi: 10.1371/journal.pone.0018699
Hyndman, B. W., Kitney, R. I., and Sayers, B. McA. (1971). Spontaneous rhythms in physiological control systems. Nature 233, 339–341.
Karemaker, J. M., Wieling, W., and Dunning, A. J. (1989). “Aging and the baroreflex,” in Hypertension in The Elderly, eds A. Amery and J. Staessen (Amsterdam: Elsevier Science Publishers, B.V. Biomedical Division), 24–38.
Kubo, T., Parker, J. D., Azevedo, E. R., Atchison, D. J., Newton, G. E., Picton, P., et al. (2005). Vagal heart rate responses to chronic beta-blockade in human heart failure relate to cardiac norepinephrine spillover. Eur. J. Heart Fail. 7, 878–881.
Lake, D. E., and Moorman, J. R. (2011). Accurate estimation of entropy in very short physiological time series: the problem of atrial fibrillation detection in implanted ventricular devices. Am. J. Physiol. 300, H319–H325.
Lee, H., Rusin, C. G., Lake, D. E., Clark, M. T., Guin, L., Smoot, T. J., et al. (2012). A new algorithm for detecting central apnea in neonates. Physiol. Meas. 33, 1–17.
Leistedt, S. J. J., Linkowski, P., Lanquart, J. P., Mietus, J. E., Davis, R. B., Goldberger, A. L., et al. (2011). Decreased neuroautonomic complexity in men during an acute major depressive episode: analysis of heart rate dynamics. Transl. Psychiatry 1:e27. doi: 10.1038/tp.2011.23
Lin, L. Y., Lin, J. L., Du, C. C., Lai, L. P., Tseng, Y. Z., and Huang, S. K. (2001). Reversal of deteriorated fractal behavior of heart rate variability by beta-blocker therapy in patients with advanced congestive heart failure. J. Cardiovasc. Electrophysiol. 12, 26.
Maestri, R., Pinna, G. D., Accardo, A., Allegrini, P., Balocchi, R., D'Addio, G., et al. (2007). Nonlinear indices of heart rate variability in chronic heart failure patients: redundancy and comparative clinical value. J. Cardiovasc. Electrophysiol. 18, 425–433.
Morris, J. A. Jr., Norris, P. R., Waitman, L. R., Ozdas, A., Guillamondegui, O. D., and Jenkins, J. M. (2007). Adrenal insufficiency, heart rate variability, and complex biologic systems: a study of 1,871 critically ill trauma patients. J. Am. Coll. Surg. 204, 885–892.
Mortara, A., La Rovere, M. T., Pinna, G. D., Maestri, R., Capomolla, S., and Cobelli, F. (2000). Nonselective beta-adrenergic blocking agent, carvedilol, improves arterial baroflex gain and heart rate variability in patients with stable chronic heart failure. J. Am. Coll. Cardiol. 36, 1612–1618.
Nakagawa, S. (2004). A farewell to Bonferroni: the problems of low statistical power and publication bias. Behav. Ecol. 15, 1044–1045.
Pousset, F., Copie, X., Lechat, P., Jaillon, P., Boissel, J. P., Hetzel, M., et al. (1996). Effects of bisoprolol on heart rate variability in heart failure. Am. J. Cardiol. 77, 612.
Rajendra Acharya, U., Paul Joseph, K., Kannathal, N., Lim, C. M., and Suri, J. S. (2006). Heart rate variability: a review. Med. Biol. Eng. Comput. 44, 1031–1051.
Richman, J. S., and Moorman, J. R. (2000). Physiological time-series analysis using approximate entropy and sample entropy. Am. J. Physiol. 278, H2039–H2049.
Saul, J. P., Arai, Y., Berger, R. D., Lilly, L. S., Colucci, W. S., and Cohen, R. J. (1988). Assessment of autonomic regulation in chronic congestive heart failure by heart rate spectral analysis. Am. J. Cardiol. 61, 1292–1299.
Stoschitzky, K., Koshucharova, G., Zweiker, R., Maier, R., Watzinger, N., Fruhwald, F. M., et al. (2001). Differing beta-blocking effects of carvedilol and metoprolol. Eur. J. Heart Fail. 3, 343–349.
Task Force of the European Society of Cardiology, the North American Society of Pacing and Electrophysiology. (1996). Heart rate variability: standards of measurement, physiological interpretation and clinical use. Circulation 93, 1043–1065.
Ten Harkel, A. D. J., van Lieshout, J. J., Karemaker, J. M., and Wieling, W. (1993). Differences in circulatory control in normal subjects who faint and who do not faint during orthostatic stress. Clin. Auton. Res. 3, 117–124.
Truijen, J., de Peuter, O. R., Kim, Y. S., van den Bogaard, B., Kok, W. E., Kamphuisen, P. W., et al. (2011). Beta2-adrenergic receptor genotype influences the effect of nonselective vs. selective beta-blockade on baroreflex function in chronic heart failure. Int. J. Cardiol. 153, 230–232.
Turianikova, Z., Javorka, K., Baumert, M., Calkovska, A., and Javorka, M. (2011). The effect of orthostatic stress on multiscale entropy of heart rate and blood pressure. Physiol. Meas. 32, 1425.
Witte, K. K., and Clark, A. L. (2008). Carvedilol in the treatment of elderly patients with chronic heart failure. Clin. Interv. Aging 3, 55–70.
Keywords: intensive care, home monitoring, heart rate variability, time domain analysis, frequency domain analysis, entropy
Citation: Zhang Y, de Peuter OR, Kamphuisen PW and Karemaker JM (2013) Search for HRV-parameters that detect a sympathetic shift in heart failure patients on β-blocker treatment. Front. Physiol. 4:81. doi: 10.3389/fphys.2013.00081
Received: 20 January 2013; Accepted: 26 March 2013;
Published online: 16 April 2013.
Edited by:
Zbigniew R. Struzik, The University of Tokyo, JapanReviewed by:
Junichiro Hayano, Nagoya City University Graduate School of Medical Sciences, JapanDirk Hoyer, Jena University Hospital, Germany
Copyright © 2013 Zhang, de Peuter, Kamphuisen and Karemaker. This is an open-access article distributed under the terms of the Creative Commons Attribution License, which permits use, distribution and reproduction in other forums, provided the original authors and source are credited and subject to any copyright notices concerning any third-party graphics etc.
*Correspondence: John M. Karemaker, AEF/Systems Physiology, Heart Failure Research Center, Academic Medical Center at the University of Amsterdam, Meibergdreef 9, Rm. M01-216, 1105AZ Amsterdam, Netherlands. e-mail:ai5tLmthcmVtYWtlckBhbWMudXZhLm5s