- 1Aix Marseille Univ, INSERM, INRA, C2VN, Marseille, France
- 2Pôle Cardio-Vasculaire et Thoracique, Service des Explorations Fonctionnelles Respiratoires, AP-HM, Hôpital Nord, Marseille, France
- 3Service de Médecine et Santé au Travail, AP-HM, Hôpital de la Timone, Marseille, France
- 4Pôle Cardio-Vasculaire et Thoracique, Service de Cardiologie, AP-HM, Hôpital de la Timone, Marseille, France
- 5AltraBio, Lyon, France
Mental workload is known to alter cardiovascular function leading to increased cardiovascular risk. Nevertheless, there is no clear autonomic nervous system unbalance to be quantified during mental stress. We aimed to characterize the mental workload impact on the cardiovascular function with a focus on heart rate variability (HRV) non-linear indexes. A 1-h computerized switching task (letter recognition) was performed by 24 subjects while monitoring their performance (accuracy, response time), electrocardiogram and blood pressure waveform (finger volume clamp method). The HRV was evaluated from the beat-to-beat RR intervals (RRI) in time-, frequency-, and informational- domains, before (Control) and during the task. The task induced a significant mental workload (visual analog scale of fatigue from 27 ± 26 to 50 ± 31 mm, p < 0.001, and NASA-TLX score of 56 ± 17). The heart rate, blood pressure and baroreflex function were unchanged, whereas most of the HRV parameters markedly decreased. The maximum decrease occurred during the first 15 min of the task (P1), before starting to return to the baseline values reached at the end of the task (P4). The RRI dimension correlation (D2) decrease was the most significant (P1 vs. Control: 1.42 ± 0.85 vs. 2.21 ± 0.8, p < 0.001) and only D2 lasted until the task ended (P4 vs. Control: 1.96 ± 0.9 vs. 2.21 ± 0.9, p < 0.05). D2 was identified as the most robust cardiovascular variable impacted by the mental workload as determined by posterior predictive simulations (p = 0.9). The Spearman correlation matrix highlighted that D2 could be a marker of the generated frustration (R = –0.61, p < 0.01) induced by a mental task, as well as the myocardial oxygen consumption changes assessed by the double product (R = –0.53, p < 0.05). In conclusion, we showed that mental workload sharply lowered the non-linear RRI dynamics, particularly the RRI correlation dimension.
Introduction
Studies of work and its mental consequences raised the concept of mental workload that has been initially defined by Gopher and Donchin (1986): “Mental workload may be viewed as the difference between the capacities of the information processing system that are required for task performance to satisfy performance expectations and the capacity available at any given time.” According to Hart and Staveland (1988), the developer of the NASA Task Load Index (NASA-TLX), workload can be defined as “the cost incurred by a human operator to achieve a particular level of performance” (p. 2) that can have deleterious consequences on health.
The impact of mental workload on health has been widely investigated, particularly the links between mental workload and cardiovascular diseases. Job-strain (high job demands and/or low decision latitude) is found to raise the risk of coronary heart disease (Bosma et al., 1997; Kivimäki et al., 2002, 2012; Netterstrom et al., 2006), as well as hypertension (Markovitz, 1993; Schwartz et al., 1996). However, no absolute causal demonstration has emerged from the studies to date. High mental workload raises heart rate and blood pressure (Schnall et al., 1990, 1994; Wilson, 1992, 1993; Veltman and Gaillard, 1996; Hankins and Wilson, 1998; Wilson and O’Donnell, 1988; Hjortskov et al., 2004), two indexes of cardiovascular risk. Subtler cardiovascular modifications, also linked to an increased cardiovascular risk, have been described as a result of heart rate variability analysis (HRV). HRV is a highly sensitive marker of clinical status, especially of cardiac disease and autonomic neuropathy (Task Force of the European Society of Cardiology and the North American Society of Pacing and Electrophysiology, 1996). Furthermore, HRV has been successfully used to assess environmental or physiological conditions likely to modify sympathetic-parasympathetic balance even without any cardiac disease or autonomic neuropathy. HRV is lowered by mental effort and mental workload (Mulder and Mulder, 1981; Hjortskov et al., 2004; Wang et al., 2005) probably due to sympathetic activation and/or to parasympathetic withdrawal (Hatch et al., 1986; Grossman and Svebak, 1987; Grossman, 1992; Hjortskov et al., 2004; Collins et al., 2005; Wang et al., 2005; Taelman et al., 2011). According to more recent studies, mean RR interval (RRI) appears to be a most sensitive measure (Capa et al., 2008a,b; De Rivecourt et al., 2008; Henelius et al., 2009; Weippert et al., 2009) even if there is a strong inverse relationship (Hennessy et al., 2001; Sandercock et al., 2005; Fu et al., 2006; Paul-Labrador et al., 2006) between mental effort and HRV power. Few studies have searched for links between mental workload and baroreflex function while arterial baroreflex is a major determinant of the sympatho-vagal balance and of the neurally mediated HRV supported by the autonomic nervous system (ANS). Mental workload seems to reduce the baroreflex sensitivity (Mulder and Mulder, 1981) as exhibited in most cardiovascular diseases and identified as an independent and robust cardiovascular risk factor (Rovere et al., 1998).
But HRV analysis trough time- and frequency- domains mainly focus on sympatho-vagal balance assessment (even for baroreflex assessment) which does not completely and robustly describe the complex time behavior of heart rhythm. The lack of a clear ANS unbalance during mental stress, and the need of exploring different metrics (e.g., non-linear or multivariate) than standard time and frequency domain measures, have been reported recently (Vuksanović and Gal, 2007; Melillo et al., 2011; Widjaja et al., 2015; Faes et al., 2016). Non-linear analysis technics and metrics try to catch non-linear dynamics properties that characterize complex systems. Heart rate fluctuations (RRI time series) are the result of a complex process including a complex system that is the heart (Goldberger, 1996, 2002) inside the human body and several influencing environmental factors. These recent tools seem to be more sensitive and precise to describe cardiac and clinical status and to assess the associated prognosis in different diseases (Huikuri et al., 2008). Recent studies have demonstrated relationships between mental workload and mental fatigue and HRV. The first study (Mukherjee et al., 2011) highlighted the sensitivity and reliability of HRV time domain (mean RRI), frequency domain (high and low frequency powers), and non-linear indexes (Poincaré estimates and detrended fluctuation analysis indexes) to mental effort tasks in healthy seniors. The second study (Gergelyfi et al., 2015) investigated HRV, as well as electroencephalogram (EEG), eye blink and skin conductance variables during a mental fatigue induced task (Sudoku) in a protocol dedicated to discriminate between motivational state and mental fatigue. Both studies showed the interest, the sensitivity and the reliability of the non-linear Poincaré plot analysis. Recent non-linear metrics have been developed to characterize fractal time behavior, autosimilarity, or complexity of the time series. Among them, RRI dimension correlation (D2) is considered to be a marker of the cardiovascular system adaptability assessing its degree of freedom. As Tako-Tsubo syndrome is a brutal heart failure that is associated with rhythmic troubles and often follows a severe emotional stress (Sharkey et al., 2010), including extreme cases of overworking (Akashi et al., 2008), and as D2 has been shown to be the more precocious and sensitive cardiovascular parameter in the prediction of ventricular fibrillation occurrence in high-risk subjects (Skinner et al., 1993), we tested the hypothesis that D2 could be a monitoring index of the cardiovascular impact of mental workload intensity induced by a switching task.
In this study, we aimed to investigate cardiovascular function changes during a fatiguing mental task, focusing on HRV and particularly its non-linear properties, but also on spontaneous arterial baroreflex, a robust independent cardiovascular risk factor modulating sympatho-vagal balance, and on myocardial oxygen consumption that reflect cardiac metabolic constraint and cardiac stress. The main objective was to identify relevant cardiovascular indexes of mental workload and document their respective time course. We specifically targeted the correlation dimension (D2) of the RRI.
Materials and Methods
Subjects
Power analysis was performed to determine sample size. Sixteen number of pairs are necessary to achieve a power of 80% and a level of significance of 5% (two sided), for detecting an effect size of 0.8 between pairs. Accordingly, twenty-four healthy volunteers participated in the study. The subjects were orally checked by a medical doctor for cardiovascular or neurologic history, addiction, pregnancy and high level sport practicing and were not included (n = 1) accordingly. None of the subjects were taking medication. The subjects were instructed to refrain from exercising, drinking alcohol, coffee or tea, and sleep deprivation for at least 24 h before their participation. The study was approved by the Ethical Committee of the University of Aix-Marseille and was conducted in accordance with the Declaration of Helsinki. Written consent was provided by each subject. The subjects performed the study protocol as illustrated in Figure 1.
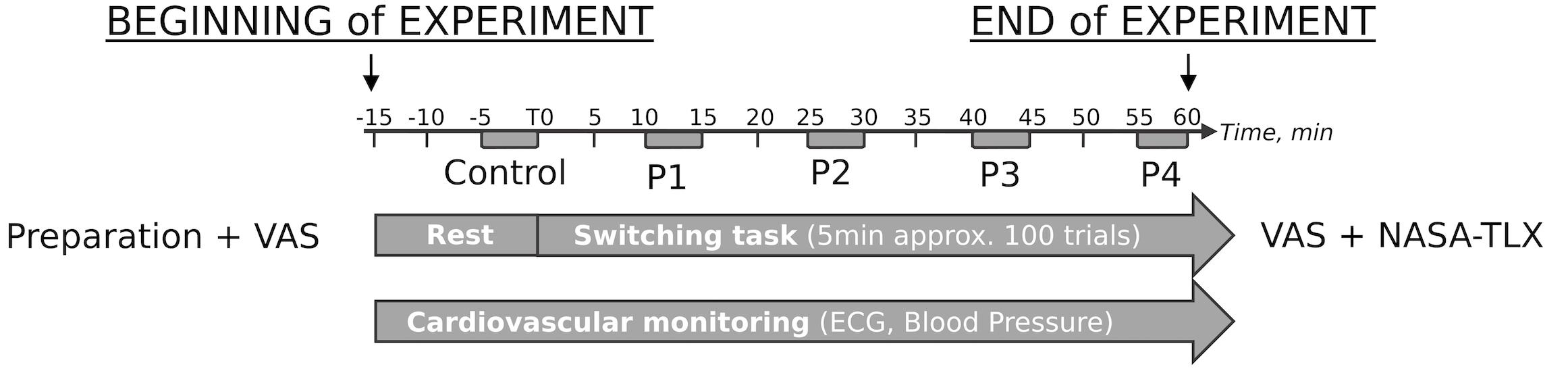
Figure 1. Protocol design. The protocol lasted approximately 1 h 20 min, including 15 min under resting conditions (calm, room temperature control, sitting position in front of a computer, no visual stimulus), of which the last 5 min were used as the Control conditions, and 60 min of the switching task on the computer. Before the task started the visual analog scale (VAS) was administered and VAS + NASA-TLX questionnaires were completed immediately after the task ended. During the task, the last 5 min of each temporal quartile (i.e., 25, 50, 75, and 100% of task duration, respectively) were used for cardiovascular function assessment and denoted by P1, P2, P3, and P4, respectively.
Long Duration Switching Task Procedure
The subjects had to perform a task based on the task-switching paradigm during 1 h (Jersild, 1927). We used a custom version of alternating runs based on Rogers and Monsell (1995). Because we aimed to preserve the performance of our subjects on a long run, stimuli were displayed until the subject responded or 2 s. The aim of each trial was to identify accurately and as quickly as possible a letter randomly selected among 16 colored (Blue or Red) letters (AEOUGKMR) displayed on a 2∗2 grid on a computer screen (Peirce, 2008) with Psychopy (Open source, University of Nottingham, United Kingdom). The subjects had to identify both the color and nature (vowel or consonant) of the displayed letter. Each trial was constituted as follows: a 2∗2 grid pattern appeared immediately at the beginning of the trial, and after 500 ms, a colored letter appeared in one of the four boxes. When the letter appeared on the upper part of the 2∗2 grid pattern, the subjects had to push the right arrow keyboard key if the letter was red or the left arrow key if the letter was blue; when the letter appeared in the lower part, the subjects had to push the left arrow key if the letter was a vowel and the right arrow key if the letter was a consonant, regardless of the color. The letters were presented for a maximum 2 s, and the subjects had to press the adequate keyboard key within this time limit. The absence of a response was assimilated to and considered as a wrong response. Following the letter presentation, regardless of whether there was a response, a new trial was initiated after a random interval (200, 450 or 700 ms), while the 2∗2 grid pattern remained displayed. The task automatically stopped after 1 h or if the error rate outran 49% starting at 30 min of practice. The first 100 trials were not taken into account; however, they were used as training, and feedback was presented at the end of each trial. The performance feedback disappeared from the 101st trial and then the countdown began.
Measurements
Mental Workload
Objective assessment of mental workload was performed throughout the entire switching task, measuring the mental performance through the calculation of the switching costs for accuracy and response time (Rogers and Monsell, 1995). Four periods (P1, P2, P3, and P4, respectively) of 100 trials (approximately 5 min) were extracted from the 1-h duration task starting at the 10th, 25th, 40th and the 55th min, respectively. These periods were determined to be equally time spaced during the whole protocol duration, to coincide with the end of the temporal quartile of the protocol duration, and to be synchronic with the 5 min cardiovascular recordings necessary for HRV measurements. No performance feedback was provided during these periods.
The visual analog scale (VAS) for fatigue, drowsiness and anxiety was performed before and after the switching task to evaluate the impact experienced by subjects. The NASA-TLX rating scale was completed at the end of the experiment. The NASA TLX rates perceived workload in order to assess a task and consists in 6 subjective subscales rating within a 100-points different aspects of the task. As a multidimensional scale, the NASA-TLX is divided into 6 components with specific subscales as follows: mental demand, physical demand, temporal demand, performance, effort, and frustration (NASA Sc1, NASA Sc2, NASA Sc3, NASA Sc4, NASA Sc5, and NASA Sc6, respectively). As discussed by Hart (2006), we used the raw score of each of the subscales of the NASA-TLX (no pairwise comparisons, no weighting process, no addition), which are more stable and valid metrics.
Cardiovascular Function
Room experiment was quiet and its temperature kept constant. Subjects were asked to seat in the comfortable office chair installed in front of the personal computer provided for the experiment. Subjects were asked to relax with free breathing. All experiments started at 10 AM. Three lead electrocardiogram (ECG) and arterial pulse wave were non-invasively (surface thoracic ECG and digital photoplethysmography, respectively) acquired (CNAP Monitor 500, version 3.7.2., CNSystems Medizintechnik AG, Graz, Austria) during the 15 min before the task started (resting control conditions) and during the whole task. The data were then digitized and stored on a personal computer for further analysis. Five periods of 5 min were extracted and analyzed: 5 min before the end of the resting control conditions (Control) and 5 min starting from the 10th, 25th, 40th and 55th min of the task (P1, P2, P3, and P4, respectively). The P1 to P4 periods corresponded to the periods analyzed for the switching costs and performance assessment.
Cardiovascular Data Analysis
All cardiovascular data analysis was performed from the 5 min beat-to-beat time series constituted from inter-beat intervals from ECG R waves, i.e., the RRI and systolic/mean/diastolic blood pressure (SBP, MBP, and DBP, respectively) that were extracted from raw signals. Raw signals were firstly visually checked and corrected for artifacts (including ectopic beat management, i.e., detection, cancelation, and interpolation) when necessary. Non-evident non-stationarity, such as very slow drifting of the mean or sudden changes of the variance, was observed after linear detrending. No additional filtering techniques such as integration was used. Raw signals were sampled at 1,000 Hz and digitized with a 24-bit analog-to-digital converter. Concerning ECG, Pan and Tompkins real-time QRS detection algorithm was used to automatically detect R-waves and build the RRI series. Discrete original RRI series were resampled by cubic spline interpolation with a 4 Hz sampling rate to generate equidistantly sampled time series x(i), i = 1, 2,…, N.
The HRV was assessed according to the consensual standards (Task Force of the European Society of Cardiology and the North American Society of Pacing and Electrophysiology, 1996). We used Kubios software (Kubios HRV, 2.1, Biosignal Analysis and Medical Imaging Group, Kuopio, Finland).
Time-Domain Analysis of HRV
Several classical metrics were used as indexes of the total variability that arises from both random and periodic sources (Billman, 2011), including: the mean RRI and heart rate (HR), the standard deviation of the RRI (STD RRI) and HR (STD HR), the square root of the mean squared differences between adjacent normal RRI (RMSSD), the count of successive normal beat lengths that differed more than 50 ms (NN50), the percentage of successive normal beat lengths that differed more than 50 ms (pNN50), the RRI triangular index, and the triangular interpolation of the discrete distribution of the normal RRI (TINN).
Frequency-Domain Analysis of HRV
Different metrics were used to characterize the periodic oscillations of the studied time series using the estimated power spectrum by fast Fourier transform Welch’s periodogram technique. These metrics included the centroid frequency (cf expressed in Hz) and power (pw expressed in ms2) in 3 frequency bands of interest: high frequencies (HF, 0.15–0.4 Hz), low frequencies (LF, 0.04–0.15 Hz), and very low frequencies (VLF, 0.003–0.04 Hz). The total power (TP) and LHpw/HFpw ratio were also computed. The HF and LF powers were also expressed as the percentage of TP (HFperc and LFperc, respectively) and normalized units (HFnu and LFnu, respectively) to better reflect the sympatho-vagal components of the HRV and were defined as HFnu = HFpw/(TP-VLFpw) × 100 and LFnu = LFpw/(TP-VLFpw) × 100, respectively. These variables were used (Akselrod et al., 1981; Billman, 2011) as indexes of the total variability of the heart rate (TP), the modulation of the sinus node activity by the parasympathetic component of the ANS (HFnu), the modulation of the sinus node activity by both the parasympathetic and sympathetic components of the ANS (LFnu), the influence of the temperature, renin-angiotensin system and other humoral and hormonal factors on the heart rate (VLFpw), and the sympathetic/parasympathetic balance (LF/HF).
Informational Domain Analysis of HRV
Most informational domain metrics are known to catch and highlight non-linear properties of the studied time series. We plotted the RRI of rank n + 1 as a function of the RRI of rank n (lag 1 Poincaré plot) and calculated its SD1, SD2 and SD1/SD2 defined as the standard deviation of the instantaneous beat-to-beat RRI variability (minor axis of the fitted ellipse), the standard deviation of the continuous long-term RRI variability (major axis of the fitted ellipse) and the axis ratio, respectively (Hoshi et al., 2013). They were respectively used as non-linear indexes of (1) the rapid changes in the RRI and thus of the parasympathetic sinus node control (Mourot et al., 2004), (2) the effects of both parasympathetic and sympathetic components on the sinus node activity (De Vito et al., 2002), and (3) the relationship between these components, which is the ratio of the short interval variation to the long interval variation (Acharya et al., 2002). We also used other non-linear tools to characterize the RRI dynamics. Recurrence plot analysis quantification (Mean line length, Max line length, Recurrence rate (REC), Determinism (DET) and Shannon entropy) was performed with embedding dimension, lag, and threshold distance set to m = 10, τ = 1, and of the RRI time series analyzed, respectively. We computed detrended fluctuation analysis α1 and α2 coefficients with segment length set to n∈(4,16) and n∈(16,64), respectively. Approximate Entropy (ApEn) and Sample Entropy (SampEn) estimates of each RRI time series were computed with m (embedding dimension) and r (filtering level) set to 2 and 0.2 SD of the RRI time series analyzed, respectively.
However, because non-linear deterministic measures of heartbeats have recently been shown to be more sensitive than the stochastic indexes in detecting the autonomic changes related to mortality and because what the time-dependent non-linear metrics show as indicators of cardiac vulnerability to lethal arrhythmias are transient non-stationary shifts of dimension of the heartbeat dynamics to a low value, we ultimately evaluated the correlation dimension (D2) of the RRI times series. D2 quantified the time self-similarity of a signal, i.e., of the RRI time series. D2 can be considered as an estimation of the lower threshold of the number of degrees of freedom for the underlying system generating the observed data and is typically used as an index of the overall complexity of the system dynamics estimated from a time series (Sammer, 1998). D2 was computed as described by Grassberger and Procaccia (1983) with r = 15% from the standard deviation of the RRI. Accordingly, the first step is to construct the correlation integral (N,m,r) function. The correlation integral counts the fraction of pairs (Xi, Xj) whose distance is smaller than r and is defined as:
Xi and Xj represent phase-space trajectory points, N represents the total amount of phase-space points, and H represents the Heaviside step function, i.e., H(α) = 0 if α < 0 and H(α) = 1 if α ≥ 0. D2 is subsequently computed as:
Finally, the baroreflex function assessment consisted of evaluating the set-point (averaged RRI and averaged SBP over 5 min subsequently represented on an x-y plot of the SBP-RRI) and the sensitivity (BRS) of the spontaneous arterial baroreflex. The baroreflex sensitivity was calculated as the ratio of the standard deviation of the RRI by the standard deviation of the SBP that has been demonstrated to be a robust estimator of the spontaneous arterial baroreflex sensitivity when compared to 6 other established methods (Bernardi et al., 2010).
To assess the myocardial oxygen consumption, we calculated an indirect index using the double product (SAP × HR), also referred to as the systolic pressure-rate product (Nelson et al., 1974).
Statistics
Statistics were performed using R statistical software (R Core Team, 2018) with additional packages including lme4 (Bates et al., 2015), car (Fox and Weisberg, 2019), Multcomp (Hothorn et al., 2008). Normality of variables distribution was checked and all the variables used meet the assumptions for the analysis performed. All descriptive data are presented as the mean ± standard deviation. For each cardiovascular variable, five periods were determined: Control, before the task started, and the first, second, third, and fourth temporal quartiles of the task (i.e., from 0 to the 15th min, from the 15th to the 30th min, from the 30th to the 45th min, and from the 45th to the 60th min). For each period, the 5 last minutes of the data were analyzed and will be referred as the Control, P1, P2, P3, and P4, respectively.
To identify the cardiovascular parameters that were altered during mental workload, we performed the following steps. First, linear mixed models and effects were respectively performed and fitted on each measure (Bates et al., 2015), as well as calculated cardiovascular variables (42 cardiovascular variables generated 42 models, i.e., one model per cardiovascular variable) to highlight their modifications during the switching task. The formula for each mixed model was: Y ∼ Period + (1| subject), where Y is the cardiovascular variable to explain, Period is the categorical fixed effect term (Control and P1 to P4) and subject is the random effect term subject. The form (1| subject) indicates that the model will estimate a random effect intercept for subject. ANOVA tables for each cardiovascular variable were computed on each corresponding linear mixed model using the Wald F-test statistics with Kenward-Roger degree of freedom (Fox and Weisberg, 2019) and post hoc analysis were performed (Hothorn et al., 2008). Thus, a list of 42 F and corresponding p-values was obtained for Period, the fixed effect term. Second, Bonferroni correction was applied on the p-value list to control family-wise error rate. From the 42 p-values, only cardiovascular models that included cardiovascular variables with significant adjusted p-values were selected. Finally, we complementary computed posterior predictive simulation to “look for systematic discrepancies between real and simulated data” (Gelman and Hill, 2006) and used as a diagnostic tool to identify the best of our selected models. The posterior predictive p-value is a marker of model robustness (stability across simulations): the higher p-value, the higher model robustness.
To quantify statistical links between objective and subjective mental workload quantifiers and cardiovascular HRV parameters, we performed the following steps. First, linear mixed models were used for the task performance variables using the formula: Y ∼ Period + (1| subject), where Y is each of the task performance variables. Because task was not proposed during the Control period, by definition, the Period variable therefore has only 4 levels (P1, P2, P3, and P4). Linear mixed models were also computed to observe the difference between the VAS scores before and after the task. A final Spearman’s correlation matrix was performed with each regression Period coefficient of the cardiovascular models and the regression Period coefficients of the task performance models, VAS score changes and NASA-TLX subscales scores.
Results
Subject Characteristics
Twenty-four subjects were included in the study and performed the task. Nineteen subjects were analyzed (3 subjects were excluded for misunderstanding the task, 1 subject was excluded for lost data, and 1 subject was excluded for highly noisy data). None of the subjects reported mental or physical over activity (sport, work), mental or physical acute or chronic fatigue feelings, or daily medical treatment. Finally, no subjects reported sleep deprivation and drowsiness at the inclusion. The subjects were 25.58 ± 3.24 years old, and their weight, height and BMI were 64.61 ± 12.02 kg, 173.79 ± 8.72 cm, and 21.21 ± 2.27 kg/m2, respectively. Nine subjects were female, and nine subjects had regular sports activities (2–4 h per week). Cardiovascular risk factors included a family history of cardiovascular disease or a personal history of cardiovascular disease, including hypertension, angina, and acute coronary syndrome. Seven subjects had at least one cardiovascular risk factor (family history). All patients were also checked for sinus rhythm and a high rate of occurrence of ectopic atrial or ventricular beats.
Mental Workload
All subjects successfully performed the protocol designed as illustrated in Figure 1 until the end of the task. Switching cost kinetics throughout the protocol are summarized in Figure 2. As indicated, the switching cost on accuracy was impacted throughout the task [F(3,54) = 2.98, p = 0.039]. The zenith was observed at P3 (P3-P1, estimate = 0.052, z = 2.991, p = 0.017). No other post hoc difference was identified. The switching cost on the response time was also impacted [F(3,54) = 9.68, p < 0.001]. The nadir was observed at P4 (P4-P1, estimate = –74,996, z = 4.818, p < 0.001).
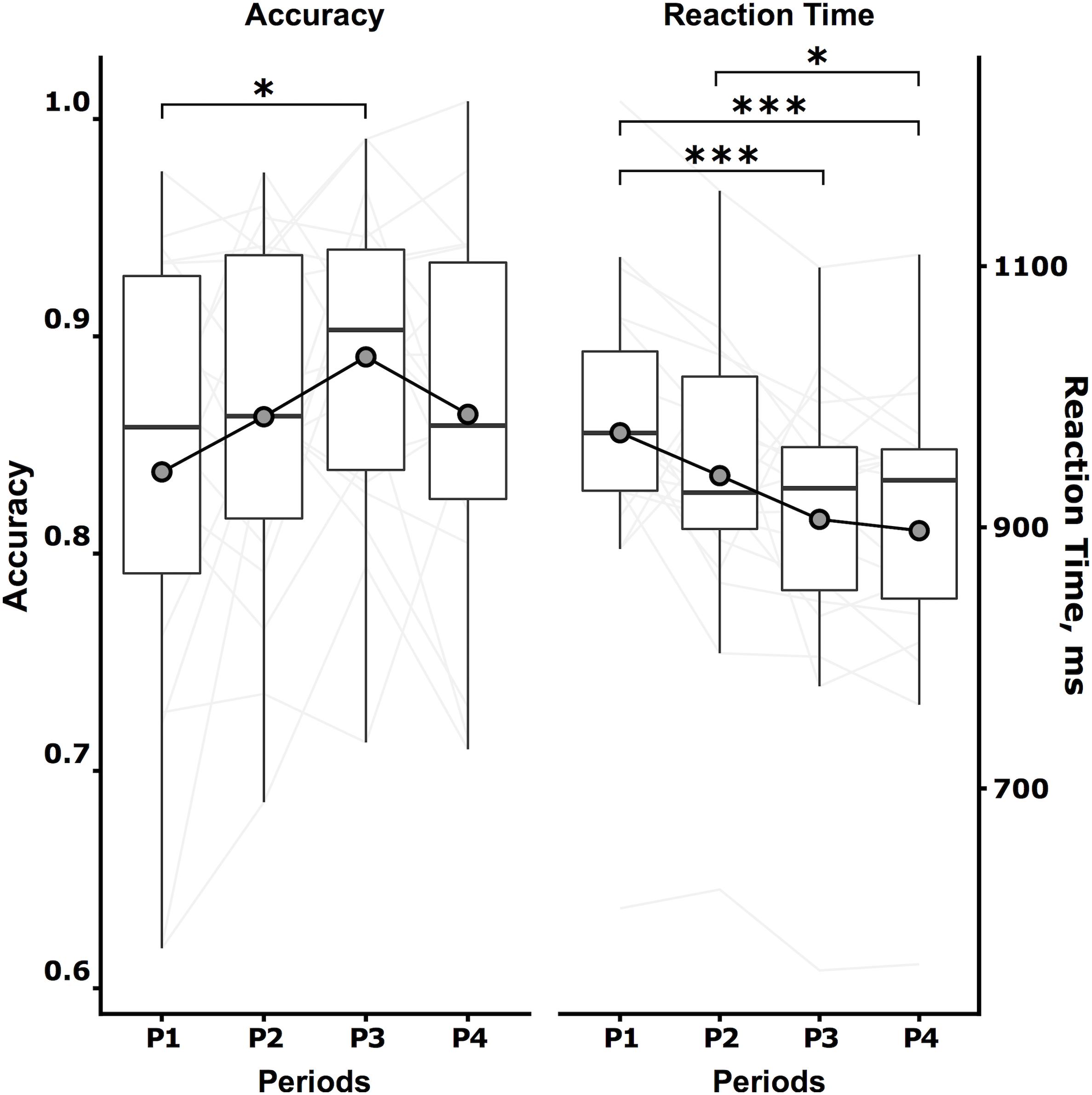
Figure 2. Switching cost kinetics. The switching cost kinetics, i.e., the Accuracy (left, no unit) and Reaction Time (right, milliseconds) during the switching task, are expressed by the boxplot summarizing the data of the 5 last minutes of the four temporal quartiles of the task duration (P1, P2, P3, and P4, respectively). Individuals are represented as light gray continuous lines, and mean data are represented as dark gray circles. Tukey post hoc test significance is represented by ∗p < 0.05 and ∗∗∗ p < 0.001.
The subjective ratings of fatigue and drowsiness were increased by the switching task as shown in Table 1. The switching task led to a relevant mental workload according to the NASA-TLX subscales scores as shown in Table 2. The subjects experienced a true effort characterized by mental, temporal, performance, effort and frustration demands with a similar profile than induced by the “LeeMoray” supervision task (Lee and Moray, 1992).
Switching Task Effect on Cardiovascular Variables
Selection Step
The global evolution of all cardiovascular parameters during the task and compared to the Control is summarized in Table 3. The switching task led to significant (adjusted p-values) changes for 13 cardiovascular variables (family-wise error rate), as shown in Table 4, including descriptors of hemodynamic macro-variables, such as heart rate, blood pressure, and double product, as well as linear and non-linear descriptors of heart rate short-term, long-term, and global dynamics, such as spectral analysis parameters or RMSSD, SD1, SD2, and D2. The subsequent analysis shows that D2 had the highest posterior predictive p-value (0.9), nearly double of the mean blood pressure and product (0.465 and 0.457). Interestingly, the posterior predictive p-value analysis highlights that all other significantly modified variables by the task had very low posterior predictive p-values (<0.3) and extremely low for spectral analysis parameters, such as TP and LFpw.
Effects Throughout the Protocol
The kinetics of the hemodynamic macro-variables during the protocol are represented in Figure 3, and the kinetics of selected heart rate dynamic indexes are represented in Figure 4. According to the post hoc test results, two different behaviors have been shown as follows: first, the zenith at P1 and a nadir at the Control follow-up by a progressive restoration to the initial level (heart rate family) and second, a zenith was achieved at P1 and a nadir at P4 (blood pressure family).
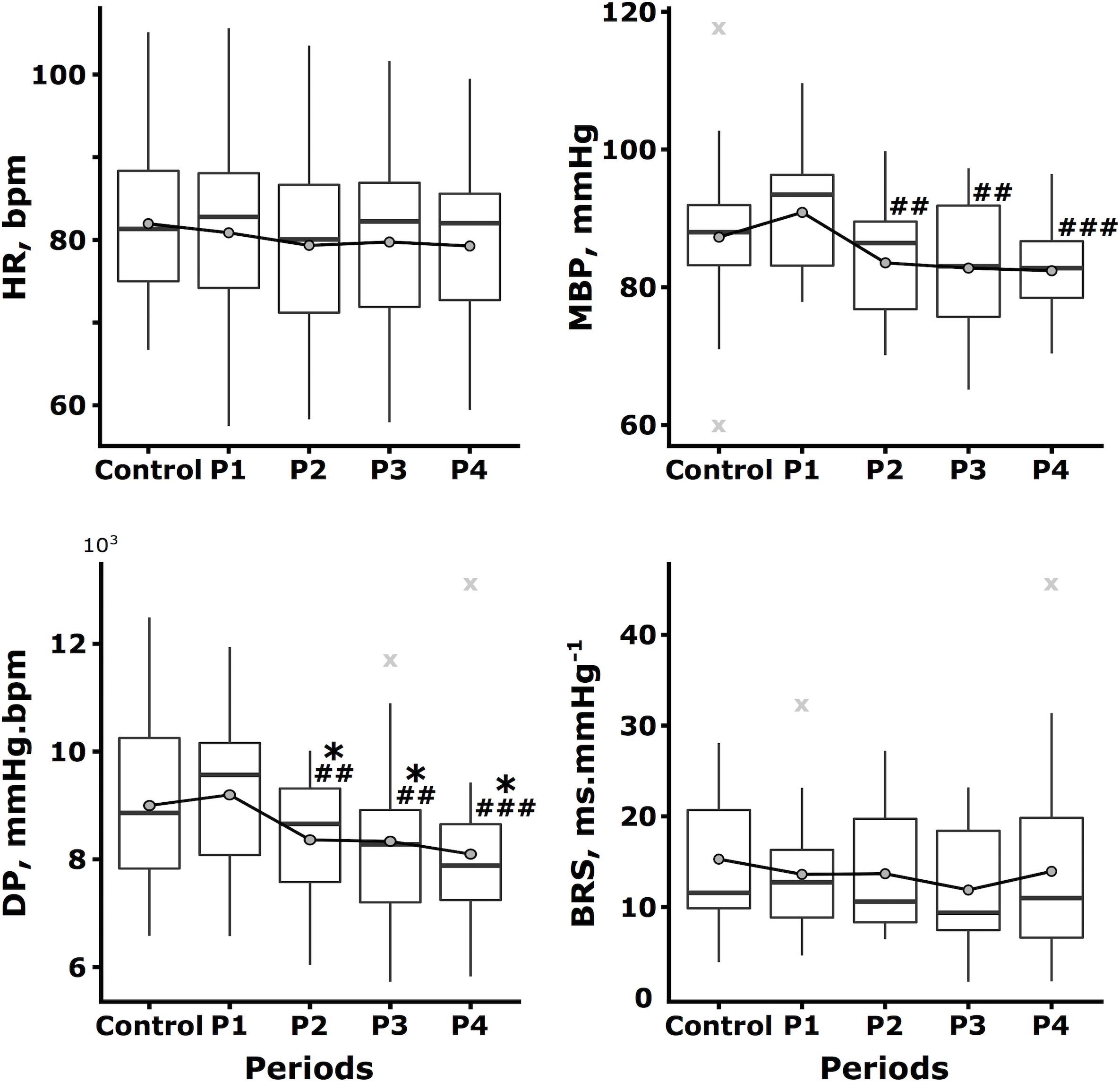
Figure 3. Kinetics of the hemodynamic macro-variables throughout the protocol. The heart rate (HR, bpm), mean blood pressure (MBP, mmHg), double product (DP, mmHg⋅bpm), and baroreflex sensitivity (BRS, ms⋅mmHg-1) are expressed by the boxplot represented throughout the protocol, i.e., during the Control condition and during the last 5 min of the first, second, third, and fourth temporal quartiles of the switching task (P1, P2, P3, and P4, respectively). Mean data are represented as dark gray circles and outliers (outlier identification by Tukey’s method) are represented by light gray crosses. Tukey post hoc test significance is represented by ∗p < 0.05 and ∗∗p < 0.01 compared to the Control, as well as ##p < 0.01 and ###p < 0.001 compared to P1.
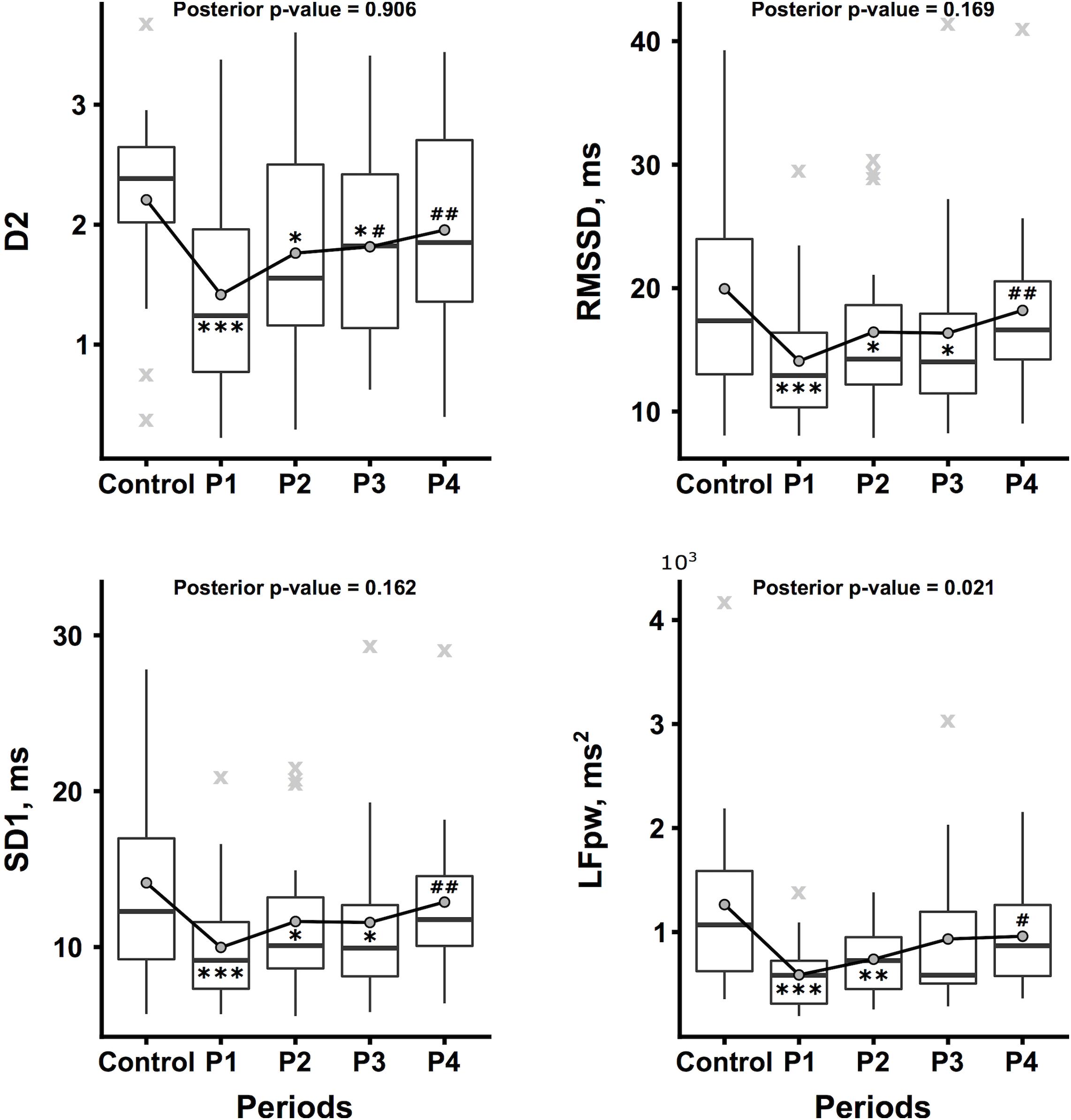
Figure 4. Kinetics of selected heart rate dynamic indexes throughout the protocol. The correlation dimension (D2, no unit), root mean square of successive differences (RMSSD, ms), standard deviation of the instantaneous beat-to-beat inter-beat interval variability (SD1, ms), and low frequency power (LFpw, ms2) are expressed by the boxplot represented throughout the protocol, i.e., during the Control condition and during the last 5 min of the first, second, third, and fourth temporal quartiles of the switching task (P1, P2, P3, and P4, respectively). Mean data are represented as dark gray circles and outliers (outlier identification by Tukey’s method) are represented by light gray crosses. Tukey post hoc test significance is represented by ∗p < 0.05, ∗∗p < 0.01, and ∗∗∗p < 0.001 compared to the Control, as well as #p < 0.05, ##p < 0.01, and ###p < 0.001 compared to P1.
Correlation Between Psychometric and Cardiovascular Changes
A correlogram plot based on Spearman’s correlation matrix is shown in Figure 5. Only selected cardiovascular variables are shown. The cardiovascular variables were partially correlated with the NASA-TLX scores. In particular, DP was positively correlated with NASA Sc2, NASA Sc4, and NASA Sc6 (p = 0.03033, p = 0.0494, and p = 0.01544, respectively), and D2 was negatively correlated with DP (p = 0.02224) and NASA Sc6 (p = 0.005459).
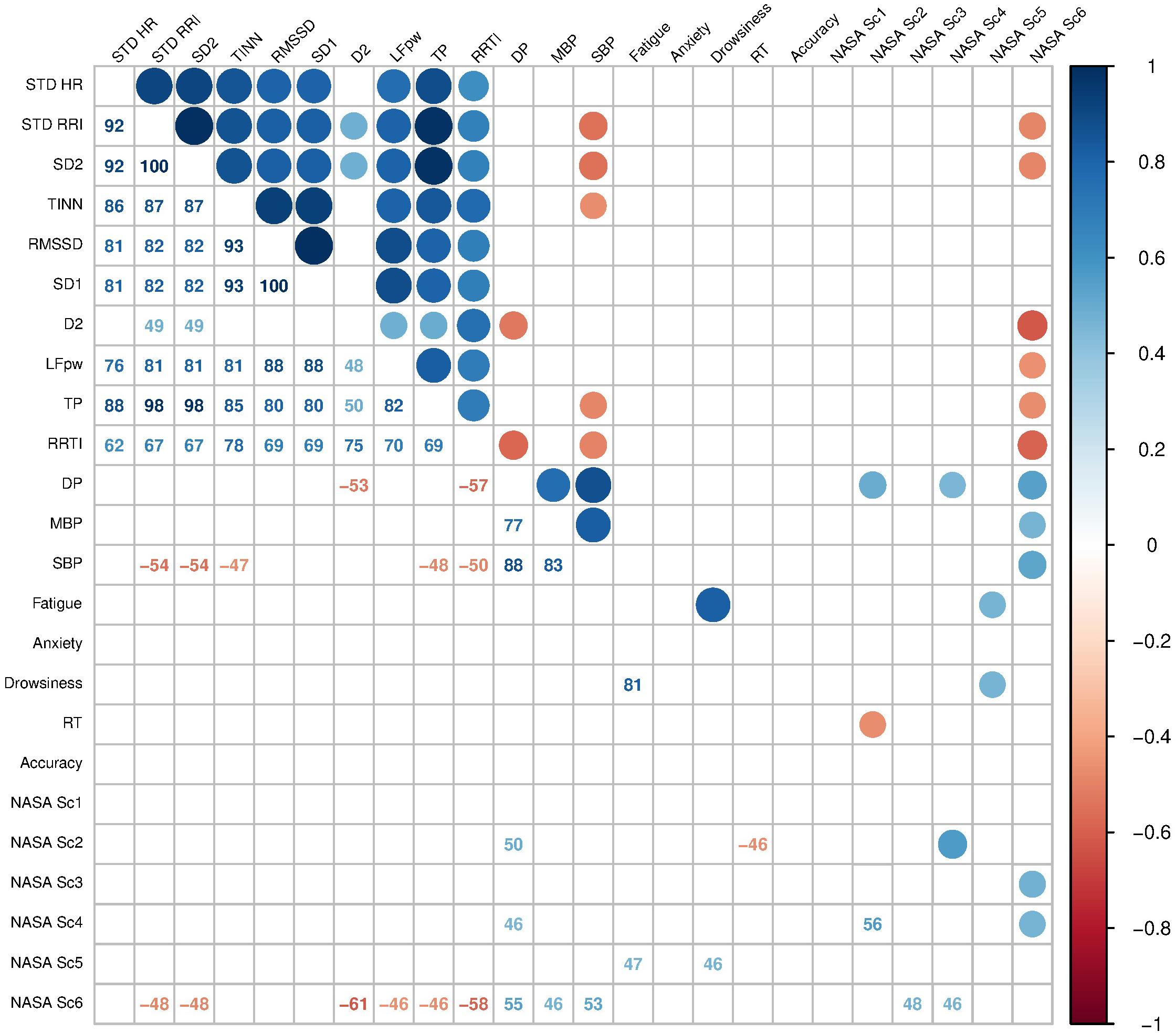
Figure 5. Cardiovascular variable and fatigue index correlations. Matrix correlation of cardiovascular variables and fatigue indexes. Spearman’s coefficient of correlation is expressed by the color scale (up-right) and values (down-left) reported. Selected interesting variables are represented to limit the high dimensional matrix. Fatigue, Anxiety and Drowsiness, visual analog scales of fatigue, anxiety, and drowsiness, respectively; STD HR, standard deviation of HR; STD RRI, standard deviation of RRI; SD2, standard deviation of the long term beat-to-beat inter-beat interval variability (semi-major axis length of Poincaré plot ellipse fitting); RRI TI, RRI triangular index; RMSSD, root mean square of successive differences; SD1, standard deviation of the instantaneous beat-to-beat inter-beat interval variability (semi-minor axis length of Poincaré plot ellipse fitting); D2, RRI correlation dimension; LFpw, low frequency power; TP, total spectral power; DP, double product (HR × SBP); MBP, mean blood pressure; DBP, diastolic blood pressure; SBP, systolic blood pressure; Reaction Time, reaction time to the switching task stimulus; Accuracy, accuracy of the switching task responses. NASA Sc1 to 6, NASA-TLX 1st to 6th subscale scores, respectively. Only significant correlations are shown (p < 0.05).
Discussion
Major Findings
We aimed to characterize the cardiovascular consequences of a calibrated mental workload and focused on the HRV responses. We also searched for a link between the magnitude of the mental workload and cardiovascular changes. A global decrease of the HRV was observed as assessed in time- as in frequency-domains, without any evident dominance of the para- or ortho-sympathetic components of the ANS. The sympatho-vagal balance that regulates HR was unchanged. The HRV decrease was maximum at the beginning of the task and then faded and even disappeared. D2, a non-linear index that does not reflect the neuromodulation of the sinus node activity by the sympatho-vagal balance but time self-similarity of the RRI time series, was decreased during the whole dual task in contrast to the other non-linear HRV parameters. D2 was the most relevant, significant, and robust HRV parameter modified by mental task and workload. D2 was negatively correlated with the NASA Sc6 component (frustration) and double product (myocardial oxygen consumption).
Mental Workload and HRV
Time and Frequency Domain HRV Indexes
Time domain and frequency domain HRV analyses have been widely used to investigate the cardiovascular consequences of mental work, as previously reported by Kramer (1990). Typically, mental workload leads to a decrease of the time domain measures, as well as a decrease of the LH and HF powers, while the LF/HF ratio increases. This supposes a predominant decrease of the parasympathetic activity or a predominant increase of the sympathetic activity (Hjortskov et al., 2004; Wang et al., 2005; Moses et al., 2007; Weippert et al., 2009; Trutschel et al., 2012). The cardiovascular response to mental workload we observed does not evidence a clear sympathetic activation while frequency domain analysis has been reported to be particularly recommended in these situations (Jorna, 1992). Two hypotheses can explain this finding: whether the subjects had a LF/HF ratio that was high prior to the beginning of the task, probably attesting an anxious anticipation phenomenon, or the task, which was repetitive, not performed in real-life but in a laboratory environment, and without a particular issue, could have limited the task-induced anxiety. Our baseline data support the first hypothesis, although the second hypothesis cannot be formally excluded.
Non-linear HRV Indexes
To our knowledge, non-linear HRV analysis methods have rarely been used in mental workload conditions. At most, a correlation between SD2 and cognitive performance indexes, i.e., vehicle lane-deviation in a night driving task and variation of the tracking error for a compensatory tracking task, has been found with r2 = 0.55 and r2 = 0.73, respectively (Trutschel et al., 2012). It is a positive correlation, which indicates that SD2 increases when performance decreases. We observed a decrease of SD1 and SD2 at the beginning of the task; however, these measures subsequently increased to reach their Control values at the end of the task. SD2 is typically considered as a non-linear index of both parasympathetic and sympathetic neuro-regulation of heart sinus activity.
Concerning D2, few studies are available. Both acute and chronic psychic stress have been shown to lower D2 (Schubert et al., 2009) while previously Sammer (1998) reported that D2 allowed to discriminate between a physical and mental task and that the Lyapunov exponent was related to the amount of the task. The values of D2 were lower in the physical task (approximately 3.7) but two main differences must be highlighted compared to our study. First, Sammer’s study used a short-term task (105s) that may induce a lower mental fatigue than in our task and second, the time series used were clearly too short (105s) to perform HRV spectral analysis according to standards, as well as to perform non-linear analysis. The non-linear analysis of a dynamic system is often limited with short and noisy time series (see “Limits” section).
In our study, D2 was the most impacted cardiovascular parameter in terms of significance as well as kinetics. Concerning significance, the exact physiology that underlies D2 is not established; however, it is supported by the complex systems approach. Time- and frequency- domain measures are considered to reflect the ANS influence on the cardiovascular system, unlike D2. D2 expresses the rate of visitation of areas in the multidimensional space spanned by the data. In our study, the lowest mean value of D2 was at 1.42 ± 0.85 and the mean value of D2 at the end of the task was 1.96 ± 0.9. These values are lower than experiment control D2 values (2.21 ± 0.8), but also than our internal reference values from a similar but distinct 25 healthy subjects group (3.21 ± 1.14) and finally than literature data. In the Skinner’s study (Skinner et al., 1993), only 3 of 25 normal subjects had values below 2.0 and the mean value of the ventricular tachycardia group was 1.3 ± 1.7. Thus, our values could be considered pathologic or at least non-physiological. This result is particularly important as it is established that a decreased D2 can be predictive of the onset of severe arrhythmias in the population of patients at high risk of sudden death. D2 has been shown to be precocious and independent from the other cardiovascular parameter arrhythmic risk factor markers, including time-domain and spectral analysis indexes of the HRV (Skinner et al., 1993). As typical cardiovascular risk factors are not always found to be heralding of sudden cardiac death (Myerburg and Spooner, 2001), the clinical interest of D2 as a complex heart rate dynamic index might be confirmed in a healthy population. In our study, in which the subjects did not exhibit cardiovascular risk factors except tobacco smoking for some subjects, we showed that the heart rate dynamic complexity (cardiac adaptability) was altered according to the mental workload. We could propose the possibility of considering D2 as a monitoring parameter for the cardiovascular impact on the mental workload. Concerning kinetics, we note that D2, as an index of the overall complexity of RRI time series that reflect HR adaptability, reached its minimum value during P1, i.e., at the beginning of the task. This initial drop of D2 could be linked with the learning solicitation that is maximum at the beginning of the task. This hypothesis is supported by our others results. Mental performance as assessed by objective metrics seems to improve throughout the task supporting a clear learning effect: Accuracy increases while Reaction Time decreases. Simultaneously, double product, that is an indirect index of myocardial oxygen consumption, i.e., of cardiac metabolic stress, increases at the very beginning of the task (P1) while it significantly lowers at P2, P3 and P4. This initial burst in cardiac solicitation (significant with paired t-test) could be explained as a side-effect of mental strain since DP is correlated to NASA Sc2, Sc4, and Sc6 and fatigue is clear at the end of the task. On the contrary, the delayed decrease in DP is synchronous of the Accuracy increase as the Reaction improvement that could be explained as a benefic effect of learning, the experimental condition being less wounding as drowsiness is highly expressed at the end of the task also. Tasks that do not continuously involve working memory can induce a high mental workload also; however, subjects will be able to reduce their effort during the task without causing an unacceptable drop of performance (Mulder, 1992). In our study, working memory is not involved, except probably at the very beginning of the task to memorize the rules. But the effect of such learning on cardiovascular parameters has been reported by others also (Taelman et al., 2011). The authors studied the heart rate and HRV under a physical task, the same task was then added with a mental task, during 6 min. Spectral analysis of the HRV indicated a decrease in the variability expressed by the time domain parameters and a decrease in the power of all spectral components with the addition of the mental task. This effect was transient and lasted only 3 min. Moreover, the alterations observed in a first session of the double task are less important than the alterations observed in the next session of this task, which further confirms the hypothesis of adaptation. This phenomenon was studied by McEwen and Stellar through the concept of homeostasis: they explain the mechanisms of adaptability in humans to unknown stressful situations (McEwen and Stellar, 1993). Finally, we showed that D2 is negatively correlated to DP, the highest DP, the lowest D2. We can then hypothesize that D2 is a sensitive marker of cardiac metabolic strain modifications induced by the mental workload unlike usual HRV indexes that should be tested in further studies. The usual parameters describing the HRV (from time- and frequency- domain analyses) and D2 are not always linked and correlated. Linear approaches loose a part of their sensitivity when a non-stationary phenomenon occurs, and as the breathing pattern is known to be altered and variable during mental workload (Veltman and Gaillard, 1996; Taelman et al., 2011), we can consider that the neuro-regulation of the HR was not stationary. In our protocol, subjects maintained a free-breathing pattern during the whole task, with the respiratory sinus arrhythmia being possibly not regular nor stationary.
Limits
Sample Size and Population Characteristics
Even if sample size calculation (n = 16) led to the theoretical adequate number of subjects to be included (N = 24) to have a statistical power of 0.8, only 19 subjects’ data were used. Twelve to twenty subjects are usually relevant in HRV physiology studies, especially when powerful stimuli are used (orthostatism, hypoxia, hypercapnia, or exercise) to disrupt the HR regulation system. However, because of HRV inter-individual as intra-individual high variability and because of the weaker stimulus used here that is mental workload, all results need to be interpreted with caution. Moreover, our work concerned healthy subjects, aged from 20 to 35 years old. They are supposed to have a normal cardiovascular function with a normal HRV. This recruiting bias makes an extrapolation of the results to the general population difficult and even more to the cardiovascular-risked population. Further studies seem necessary to replicate and strengthen our results.
Length of the Analyze Time Series
On one hand, the length of RRI time series used to perform time- and frequency- domains HRV analysis is usually set to 5 min (Task Force of the European Society of Cardiology and the North American Society of Pacing and Electrophysiology, 1996). The value of the scaling exponent is mostly defined by the dynamics of the short-time variability. Therefore, it can be used as a certain measure of short-time variability of the signal but records length has to be comparable. On the other hand, and oppositely, exploring the dimensionality of the space spanned by the data, particularly when using D2, requires long time series to be reliably computed (Säkki et al., 2004). Time series are supposed to include 10D2 data points, i.e., around 10.000 data points should be used. Using only and approximately 400 data points per time series, we can’t consider that D2 we computed represent robustly and reliably the whole concept and the underlying properties defined by the correlation dimension. But decidedly, the computation we performed (that of D2, i.e., the correlation sum) on 400 data points time series led to a metrics that was statistically characterized as the most sensitive, reliable, and stable marker. Accordingly, we then highlight that the interpretation of the time behavior of the RRI time series and HR dynamics should be made with caution: The D2 changes we measured during the task are not necessarily the results of the changes in the scaling behavior of the heart rate dynamics. Specific studies are now needed to test this hypothesis.
Baroreflex Assessment
We found no change in the spontaneous arterial baroreflex sensitivity. We used a simple methodological approach, the ratio between the standard deviations of RRI and SBP, that intercepted the median of standard BRS methods equally or better than any other method, indicating that this index can be used as a reliable method for measuring BRS (Bernardi et al., 2010). Nevertheless, this approach could be not appropriate to really disentangle true baroreflex effects from non-baroreflex or respiration-mediated influences. Further research is needed with appropriate and specific tools targeting specifically baroreflex assessment through causality concept (Porta et al., 2000; Nollo et al., 2005; Faes et al., 2013) before definitively excluding effects on arterial baroreflex.
Habituation
Our task’s characteristics and particularly its monotony, as well as the instructions’ constancy might have enabled a habituation phenomenon. Thus, mental workload mainly lies in the attention required during a long time and thereby the struggle against fatigue and drowsiness, substantially more than the cognitive load, which is preponderant in the beginning of the task only. The physical task elicited in our study may have been lower than in other tasks closer to the real practice, and it may contribute to a non-activation of the sympathetic system, as attested by the unchanged sympatho-vagal balance and the non-elevation of heart rate and blood pressure. The design of the ideal study would be a compromise between the duration of the task and the habituation aroused, i.e., a more complex task, for example, that involves the working memory, and/or a longer task duration.
Conclusion, Contributions and Prospects
We investigated the changes of HRV non-linear indexes during a switching task to search for markers of the cardiovascular consequences of mental work and workload. We showed that the RRI correlation dimension (D2), a non-linear marker of the degree of freedom of the cardiovascular system, was the most sensible and impacted metric by the mental task and workload and was negatively correlated with frustration and cardiac oxygen consumption. Nevertheless, additional research is required to confirm these initial results on the usefulness of D2 as a marker of the impact of mental workload on cardiovascular function.
Ethics Statement
This study was carried out in accordance with the recommendations of the Ethical Committee of the University of Aix-Marseille with written informed consent from all subjects. All subjects gave written informed consent in accordance with the Declaration of Helsinki. The protocol was approved by the Ethical Committee of the University of Aix-Marseille.
Author Contributions
SD conceptualized and designed the study, performed the HRV analysis and exploratory data processing, analyzed the results, wrote the manuscript, and led the research. GC designed the study, developed and wrote the switching task procedure, performed extensive data analysis, analyzed the results and wrote the manuscript. AD managed the clinical data acquirement, conducted elementary statistics, and wrote the manuscript. J-CD intensively discussed the results and highly commented the revised manuscript.
Funding
This work, which is part of the APPRISE/HIT project, was performed with the support of the A∗MIDEX project (n° ANR-11-IDEX-0001-02) funded by the “Investissements d’Avenir” program of the French Government, which is managed by the French National Research Agency (ANR).
Conflict of Interest Statement
GC was employed by the company AltraBio.
The remaining authors declare that the research was conducted in the absence of any commercial or financial relationships that could be construed as a potential conflict of interest.
Acknowledgments
The authors sincerely thank Dr. Doris Flotzinger, Clinical Research Coordinator at CNSystems Medizintechnik AG Reininghausstrasse 13/8020 Graz/Austria, for help in providing the CNSystems data acquirement platform.
References
Acharya, R. U., Lim, C. M., and Joseph, P. (2002). Heart rate variability analysis using correlation dimension and detrended fluctuation analysis. ITBM-RBM 23, 333–339. doi: 10.1016/s1297-9562(02)90002-1
Akashi, Y. J., Goldstein, D. S., Barbaro, G., and Ueyama, T. (2008). Takotsubo cardiomyopathy: a new form of acute, reversible heart failure. Circulation 118, 2754–2762. doi: 10.1161/circulationaha.108.767012
Akselrod, S., Gordon, D., Ubel, F. A., Shannon, D. C., Berger, A. C., and Cohen, R. J. (1981). Power spectrum analysis of heart rate fluctuation: a quantitative probe of beat-to-beat cardiovascular control. Science 213, 220–222. doi: 10.1126/science.6166045
Bates, D., Mächler, M., Bolker, B., and Walker, S. (2015). Fitting linear mixed-effects models using lme4. J. Stat. Softw. 67, 1–48.
Bernardi, L., De Barbieri, G., Rosengård-Bärlund, M., Mäkinen, V.-P., Porta, C., and Groop, P.-H. (2010). New method to measure and improve consistency of baroreflex sensitivity values. Clin. Auton. Res. 20, 353–361. doi: 10.1007/s10286-010-0079-1
Billman, G. E. (2011). Heart rate variability? A historical perspective. Front. Physiol. 2:86. doi: 10.3389/fphys.2011.00086
Bosma, H., Marmot, M. G., Hemingway, H., Nicholson, A. C., Brunner, E., and Stansfeld, S. A. (1997). Low job control and risk of coronary heart disease in Whitehall II (prospective cohort) study. BMJ 314, 558–565.
Capa, R. L., Audiffren, M., and Ragot, S. (2008a). The effects of achievement motivation, task difficulty, and goal difficulty on physiological, behavioral, and subjective effort. Psychophysiology 45, 859–868. doi: 10.1111/j.1469-8986.2008.00675.x
Capa, R. L., Audiffren, M., and Ragot, S. (2008b). The interactive effect of achievement motivation and task difficulty on mental effort. Int. J. Psychophysiol. 70, 144–150. doi: 10.1016/j.ijpsycho.2008.06.007
Collins, S. M., Karasek, R. A., and Costas, K. (2005). Job strain and autonomic indices of cardiovascular disease risk. Am. J. Ind. Med. 48, 182–193. doi: 10.1002/ajim.20204
De Rivecourt, M., Kuperus, M. N., Post, W. J., and Mulder, L. J. M. (2008). Cardiovascular and eye activity measures as indices for momentary changes in mental effort during simulated flight. Ergonomics 51, 1295–1319. doi: 10.1080/00140130802120267
De Vito, G., Galloway, S. D. R., Nimmo, M. A., Maas, P., and McMurray, J. J. V. (2002). Effects of central sympathetic inhibition on heart rate variability during steady-state exercise in healthy humans. Clin. Physiol. Funct. Imaging 22, 32–38. doi: 10.1046/j.1365-2281.2002.00395.x
Faes, L., Nollo, G., and Porta, A. (2013). Mechanisms of causal interaction between short-term RR interval and systolic arterial pressure oscillations during orthostatic challenge. J. Appl. Physiol. 114, 1657–1667. doi: 10.1152/japplphysiol.01172.2012
Faes, L., Porta, A., Nollo, G., and Javorka, M. (2016). Information decomposition in multivariate systems: definitions, implementation and application to cardiovascular networks. Entropy 19:5. doi: 10.1103/PhysRevE.92.062829
Fox, J., and Weisberg, S. (2019). An R Companion to Applied Regression, 2nd Edn. Thousand Oaks, CA: Sage Publications, Inc. Available at: http://socserv.socsci.mcmaster.ca/jfox/Books/Companion
Fu, C.-H., Yang, C. C. H., Lin, C.-L., and Kuo, T. B. J. (2006). Effects of long-term vegetarian diets on cardiovascular autonomic functions in healthy postmenopausal women. Am. J. Cardiol. 97, 380–383. doi: 10.1016/j.amjcard.2005.08.057
Gelman, A., and Hill, J. (2006). Data Analysis Using Regression and Multilevel/Hierarchical Models. Cambridge: Cambridge University Press.
Gergelyfi, M., Jacob, B., Olivier, E., and Zénon, A. (2015). Dissociation between mental fatigue and motivational state during prolonged mental activity. Front. Behav. Neurosci. 9:176. doi: 10.3389/fnbeh.2015.00176
Goldberger, A. L. (1996). Non-linear dynamics for clinicians: chaos theory, fractals, and complexity at the bedside. Lancet 347, 1312–1314. doi: 10.1016/s0140-6736(96)90948-4
Goldberger, A. L. (2002). Chronic fatigue syndrome and hidden happenings of the heartbeat. Clin. Auton. Res. 12, 228–230. doi: 10.1007/s10286-002-0054-6
Gopher, D., and Donchin, E. (1986). “Workload: an examination of the concept,” in Handbook of Perception and Human Performance, Vol. 2: Cognitive Processes and Performance, eds K. R. Boff, L. Kaufman, and J. P. Thomas (Oxford: John Wiley & Sons), 1–49.
Grassberger, P., and Procaccia, I. (1983). Characterization of strange attractors. Phys. Rev. Lett. 50, 346–349.
Grossman, P. (1992). Respiratory and cardiac rhythms as windows to central and autonomic biobehavioral regulation: selection of window frames, keeping the panes clean and viewing the neural topography. Biol. Psychol. 34, 131–161. doi: 10.1016/0301-0511(92)90013-k
Grossman, P., and Svebak, S. (1987). Respiratory sinus arrhythmia as an index of parasympathetic cardiac control during active coping. Psychophysiology 24, 228–235. doi: 10.1111/j.1469-8986.1987.tb00284.x
Hankins, T. C., and Wilson, G. F. (1998). A comparison of heart rate, eye activity, EEG and subjective measures of pilot mental workload during flight. Aviat. Space Environ. Med. 69, 360–367.
Hart, S. G. (2006). Nasa-task load index (NASA-TLX); 20 years later. Proc. Hum. Factors Ergon. Soc. Ann. Meet. 50, 904–908. doi: 10.1177/154193120605000909
Hart, S. G., and Staveland, L. E. (1988). “Development of NASA-TLX (Task Load Index): results of empirical and theoretical research,” in Advances in Psychology, eds P. A. Hancock and N. Meshkati (Amsterdam: Elsevier), 139–183. doi: 10.1016/s0166-4115(08)62386-9
Hatch, J. P., Klatt, K., Porges, S. W., Schroeder-Jasheway, L., and Supik, J. D. (1986). The relation between rhythmic cardiovascular variability and reactivity to orthostatic, cognitive, and cold pressor stress. Psychophysiology 23, 48–56. doi: 10.1111/j.1469-8986.1986.tb00592.x
Henelius, A., Hirvonen, K., Holm, A., Korpela, J., and Muller, K. (2009). Mental workload classification using heart rate metrics. Conf. Proc. IEEE Eng. Med. Biol. Soc. 2009, 1836–1839. doi: 10.1109/IEMBS.2009.5332602
Hennessy, M. J., Tighe, M. G., Binnie, C. D., and Nashef, L. (2001). Sudden withdrawal of carbamazepine increases cardiac sympathetic activity in sleep. Neurology 57, 1650–1654. doi: 10.1212/wnl.57.9.1650
Hjortskov, N., Rissén, D., Blangsted, A. K., Fallentin, N., Lundberg, U., and Søgaard, K. (2004). The effect of mental stress on heart rate variability and blood pressure during computer work. Eur. J. Appl. Physiol. 92, 84–89. doi: 10.1007/s00421-004-1055-z
Hoshi, R. A., Pastre, C. M., Vanderlei, L. C. M., and Godoy, M. F. (2013). Poincaré plot indexes of heart rate variability: relationships with other nonlinear variables. Auton. Neurosci. 177, 271–274. doi: 10.1016/j.autneu.2013.05.004
Hothorn, T., Bretz, F., and Westfall, P. (2008). Simultaneous inference in general parametric models. Biom. J. 50, 346–363. doi: 10.1002/bimj.200810425
Huikuri, H. V., Raatikainen, M. J. P., Moerch-Joergensen, R., Hartikainen, J., Virtanen, V., Boland, J., et al. (2008). Prediction of fatal or near-fatal cardiac arrhythmia events in patients with depressed left ventricular function after an acute myocardial infarction. Eur. Heart J. 30, 689–698. doi: 10.1093/eurheartj/ehn537
Jorna, P. G. (1992). Spectral analysis of heart rate and psychological state: a review of its validity as a workload index. Biol. Psychol. 34, 237–257. doi: 10.1016/0301-0511(92)90017-o
Kivimäki, M., Leino-Arjas, P., Luukkonen, R., Riihimäki, H., Vahtera, J., and Kirjonen, J. (2002). Work stress and risk of cardiovascular mortality: prospective cohort study of industrial employees. BMJ 325:857. doi: 10.1136/bmj.325.7369.857
Kivimäki, M., Nyberg, S. T., Batty, G. D., Fransson, E. I., Heikkilä, K., Alfredsson, L., et al. (2012). Job strain as a risk factor for coronary heart disease: a collaborative meta-analysis of individual participant data. Lancet 380, 1491–1497. doi: 10.1016/S0140-6736(12)60994-5
Kramer, A. F. (1990). Physiological Metrics of Mental Workload: A Review of Recent Progress (Interim No. NPRDC-TN-90-23). San Diego, CA: Navy Personnel Research and Development Center.
Lee, J. D., and Moray, N. (1992). Trust, control strategies and allocation of function inhuman-machine systems. Ergonomics 35, 1243–1270. doi: 10.1080/00140139208967392
Markovitz, J. H. (1993). Psychological predictors of hypertension in the framingham study: is there tension in hypertension? JAMA 270, 2439–2443. doi: 10.1001/jama.270.20.2439
McEwen, B. S., and Stellar, E. (1993). Stress and the individual. Mechanisms leading to disease. Arch. Intern. Med. 153, 2093–2101. doi: 10.1001/archinte.153.18.2093
Melillo, P., Bracale, M., and Pecchia, L. (2011). Nonlinear Heart Rate Variability features for real-life stress detection. Case study: students under stress due to university examination. Biomed. Eng. Online 10:96. doi: 10.1186/1475-925X-10-96
Moses, Z. B., Luecken, L. J., and Eason, J. C. (2007). “Measuring task-related changes in heart rate variability,” in Proceedings of the 29th Annual International Conference of the IEEE Engineering in Medicine and Biology Society, (Lyon: IEEE), 644–647.
Mourot, L., Bouhaddi, M., Perrey, S., Cappelle, S., Henriet, M.-T., Wolf, J.-P., et al. (2004). Decrease in heart rate variability with overtraining: assessment by the poincare plot analysis. Clin. Physiol. Funct. Imaging 24, 10–18. doi: 10.1046/j.1475-0961.2003.00523.x
Mukherjee, S., Yadav, R., Yung, I., Zajdel, D. P., and Oken, B. S. (2011). Sensitivity to mental effort and test-retest reliability of heart rate variability measures in healthy seniors. Clin. Neurophysiol. 22, 2059–2066. doi: 10.1016/j.clinph.2011.02.032
Mulder, G., and Mulder, L. J. M. (1981). Information processing and cardiovascular control. Psychophysiology 18, 392–402. doi: 10.1111/j.1469-8986.1981.tb02470.x
Mulder, L. J. (1992). Measurement and analysis methods of heart rate and respiration for use in applied environments. Biol. Psychol. 34, 205–236. doi: 10.1016/0301-0511(92)90016-n
Myerburg, R. J., and Spooner, P. M. (2001). Opportunities for sudden death prevention: directions for new clinical and basic research. Cardiovasc. Res. 50, 177–185. doi: 10.1016/s0008-6363(01)00253-x
Nelson, R. R., Gobel, F. L., Jorgensen, C. R., Wang, K., Wang, Y., and Taylor, H. L. (1974). Hemodynamic predictors of myocardial oxygen consumption during static and dynamic exercise. Circulation 50, 1179–1189. doi: 10.1161/01.cir.50.6.1179
Netterstrom, B., Kristensen, T. S., and Sjol, A. (2006). Psychological job demands increase the risk of ischaemic heart disease: a 14-year cohort study of employed Danish men. Eur. J. Cardiovasc. Prev. Rehabil. 13, 414–420. doi: 10.1097/01.hjr.0000201512.05720.87
Task Force of the European Society of Cardiology and the North American Society of Pacing and Electrophysiology (1996). Heart rate variability. Standards of measurement, physiological interpretation, and clinical use. Eur. Heart J. 17, 354–381. doi: 10.1093/oxfordjournals.eurheartj.a014868
Nollo, G., Faes, L., Porta, A., Antolini, R., and Ravelli, F. (2005). Exploring directionality in spontaneous heart period and systolic pressure variability interactions in humans: implications in the evaluation of baroreflex gain. Am. J. Physiol. Heart Circ. Physiol. 288, H1777–H1785.
Paul-Labrador, M., Polk, D., Dwyer, J. H., Velasquez, I., Nidich, S., Rainforth, M., et al. (2006). Effects of a randomized controlled trial of transcendental meditation on components of the metabolic syndrome in subjects with coronary heart disease. Arch. Intern. Med. 166, 1218–1224.
Peirce, J. W. (2008). Generating stimuli for neuroscience using PsychoPy. Front. Neuroinform. 2:10. doi: 10.3389/neuro.11.010.2008
Porta, A., Baselli, G., Rimoldi, O., Malliani, A., and Pagani, M. (2000). Assessing baroreflex gain from spontaneous variability in conscious dogs: role of causality and respiration. Am. J. Physiol. Heart Circ. Physiol. 279, H2558–H2567.
R Core Team (2018). R: A language and environment for statistical computing. R Foundation for Statistical Computing. Available at: https://www.R-project.org/ (accessed April 26, 2019).
Rogers, R. D., and Monsell, S. (1995). Costs of a predictible switch between simple cognitive tasks. J. Exp. Psychol. Gen. 124, 207–231. doi: 10.1037//0096-3445.124.2.207
Rovere, M. T. L., Bigger, J. T., Marcus, F. I., Mortara, A., and Schwartz, P. J. (1998). Baroreflex sensitivity and heart-rate variability in prediction of total cardiac mortality after myocardial infarction. Lancet 351, 478–484. doi: 10.1016/s0140-6736(97)11144-8
Säkki, M., Kalda, J., Vainu, M., and Laan, M. (2004). What does measure the scaling exponent of the correlation sum in the case of human heart rate? Chaos 14, 138–144. doi: 10.1063/1.1636151
Sammer, G. (1998). Heart period variability and respiratory changes associated with physical and mental load: non-linear analysis. Ergonomics 41, 746–755. doi: 10.1080/001401398186892
Sandercock, G. R. H., Bromley, P. D., and Brodie, D. A. (2005). The reliability of short-term measurements of heart rate variability. Int. J. Cardiol. 103, 238–247. doi: 10.1016/j.ijcard.2004.09.013
Schnall, P. L., Landsbergis, P. A., and Baker, D. (1994). Job strain and cardiovascular disease. Ann. Rev. Public Health 15, 381–411. doi: 10.1146/annurev.publhealth.15.1.381
Schnall, P. L., Pieper, C., Schwartz, J. E., Karasek, R. A., Schlussel, Y., Devereux, R. B., et al. (1990). The relationship between “job strain,” workplace diastolic blood pressure, and left ventricular mass index. Results of a case-control study. JAMA 263, 1929–1935. doi: 10.1001/jama.263.14.1929
Schubert, C., Lambertz, M., Nelesen, R. A., Bardwell, W., Choi, J.-B., and Dimsdale, J. E. (2009). Effects of stress on heart rate complexity—A comparison between short-term and chronic stress. Biol. Psychol. 80, 325–332. doi: 10.1016/j.biopsycho.2008.11.005
Schwartz, J. E., Pickering, T. G., and Landsbergis, P. A. (1996). Work-related stress and blood pressure: current theoretical models and considerations from a behavioral medicine perspective. J. Occup. Health Psychol. 1, 287–310. doi: 10.1037/1076-8998.1.3.287
Sharkey, S. W., Windenburg, D. C., Lesser, J. R., Maron, M. S., Hauser, R. G., Lesser, J. N., et al. (2010). Natural history and expansive clinical profile of stress (tako-tsubo) cardiomyopathy. J. Am. Coll. Cardiol. 55, 333–341. doi: 10.1016/j.jacc.2009.08.057
Skinner, J. E., Pratt, C. M., and Vybiral, T. (1993). A reduction in the correlation dimension of heartbeat intervals precedes imminent ventricular fibrillation in human subjects. Am. Heart J. 125, 731–743. doi: 10.1016/0002-8703(93)90165-6
Taelman, J., Vandeput, S., Vlemincx, E., Spaepen, A., and Van Huffel, S. (2011). Instantaneous changes in heart rate regulation due to mental load in simulated office work. Eur. J. Appl. Physiol. 111, 1497–1505. doi: 10.1007/s00421-010-1776-0
Trutschel, U., Golz, M., Heinze, C., Sommer, D., Sirois, B., and Edwards, D. (2012). Heart rate measures reflect the interaction of low mental workload and fatigue during driving simulation in Proceedings of the 4th International Conference on Automotive User Interfaces and Interactive Vehicular Applications - AutomotiveUI ’12, Portsmouth, NH: ACM Press.
Veltman, J. A., and Gaillard, A. W. (1996). Physiological indices of workload in a simulated flight task. Biol. Psychol. 42, 323–342. doi: 10.1016/0301-0511(95)05165-1
Vuksanović, V., and Gal, V. (2007). Heart rate variability in mental stress aloud. Med. Eng. Phys. 29, 344–349. doi: 10.1016/j.medengphy.2006.05.011
Wang, Z., Yang, L., and Ding, J. (2005). [Application of heart rate variability in evaluation of mental workload]. Zhonghua Lao Dong Wei Sheng Zhi Ye Bing Za Zhi 23, 182–184.
Weippert, M., Thielmann, B., Stoll, R., Pfister, E. A., and Böckelmann, I. (2009). Sympatho-vagale balance und kardiale reaktionen bei standardisierten psychomentalen belastungen / sympatho-vagal balance and cardiac response to mental challenge. Biomed. Eng. 54, 197–203. doi: 10.1515/bmt.2009.023
Widjaja, D., Montalto, A., Vlemincx, E., Marinazzo, D., Van Huffel, S., and Faes, L. (2015). Cardiorespiratory information dynamics during mental arithmetic and sustained attention. PLoS One 10:e0129112. doi: 10.1371/journal.pone.0129112
Wilson, G. F. (1992). Applied use of cardiac and respiration measures: practical considerations and precautions. Biol. Psychol. 34, 163–178. doi: 10.1016/0301-0511(92)90014-l
Wilson, G. F. (1993). Air-to-ground training missions: a psychophysiological workload analysis. Ergonomics 36, 1071–1087. doi: 10.1080/00140139308967979
Keywords: mental workload, heart rate variability, non-linear dynamics, correlation dimension, cardiovascular function, arrhythmia
Citation: Delliaux S, Delaforge A, Deharo J-C and Chaumet G (2019) Mental Workload Alters Heart Rate Variability, Lowering Non-linear Dynamics. Front. Physiol. 10:565. doi: 10.3389/fphys.2019.00565
Received: 06 September 2018; Accepted: 24 April 2019;
Published: 14 May 2019.
Edited by:
T. Alexander Quinn, Dalhousie University, CanadaReviewed by:
Lincoln M. Tracy, Monash University, AustraliaLuca Faes, University of Palermo, Italy
Nele A. J. De Witte, Thomas More Kempen, Belgium
Copyright © 2019 Delliaux, Delaforge, Deharo and Chaumet. This is an open-access article distributed under the terms of the Creative Commons Attribution License (CC BY). The use, distribution or reproduction in other forums is permitted, provided the original author(s) and the copyright owner(s) are credited and that the original publication in this journal is cited, in accordance with accepted academic practice. No use, distribution or reproduction is permitted which does not comply with these terms.
*Correspondence: Stéphane Delliaux, c3RlcGhhbmUuZGVsbGlhdXhAdW5pdi1hbXUuZnI=