- 1Division of Nuclear Medicine, University Hospitals Leuven, Leuven, Belgium
- 2Department of Imaging and Pathology, KU Leuven, Leuven, Belgium
- 3Department of Biomedical Engineering, Yale University, New Haven, CT, United States
- 4Department of Radiology and Biomedical Imaging, Yale University, New Haven, CT, United States
- 5Department of Psychiatry, Yale University, New Haven, CT, United States
- 6Invicro LLC, New Haven, CT, United States
- 7Athinoula A. Martinos Center for Biomedical Imaging, Department of Radiology, Massachusetts General Hospital, Charlestown, MA, United States
- 8Harvard Medical School, Boston, MA, United States
Positron emission tomography (PET) neuroimaging in neuropsychiatry is a powerful tool for the quantification of molecular brain targets to characterize disease, assess disease subtype differences, evaluate short- and long-term effects of treatments, or even to measure neurotransmitter levels in healthy and psychiatric conditions. In this work, we present different methodological approaches (time-invariant models and models with time-varying terms) that have been used to measure dynamic changes in neurotransmitter levels induced by pharmacological or behavioral challenges in humans. The developments and potential use of hybrid PET/magnetic resonance imaging (MRI) for neurotransmission brain research will also be highlighted.
Introduction
Many transformative therapies for neurological and psychiatric disease states over the last decades have targeted neurotransmitter systems through serotonin, dopamine, or opioid receptors and transporters. Neurotransmitters play an important role in regulating brain activity at the molecular and neurochemical level and are centrally involved in many brain functions, including, for example, cognition, behavior, sleep, appetite, and mood. Endogenous and exogenous stimuli, including behaviorally relevant stimuli, mood changes, and pharmacological challenges, evoke widespread changes in neurotransmitter systems. These changes are important to understand neural function in health and disease. One of the most and extensively characterized neurotransmitter system is the mesocorticolimbic dopaminergic pathway. Dopamine, both the “pleasure” and “goal-directed movement” chemical neurotransmitter, has been the main central pathway target for the pharmacological effects of habit-forming drugs. For example, dynamic changes in the dopaminergic system are known to contribute to a wide range of behaviors including affect, reward, decision-making, and inhibitory control.
Using in vivo functional molecular positron emission tomography (PET) imaging, we are able to image and quantify with very high sensitivity and specificity the local concentration of a range of neuroreceptor targets in a non-invasive way. PET radiotracers for imaging of neurotransmission had been primarily focused on studying changes in endogenous levels of dopamine in the striatum (Finnema et al., 2015), mainly using 11C-raclopride, but has not yet been adequately extended to other neurotransmitter systems (Paterson et al., 2010; da Cunha-Bang et al., 2019). More recently, advances have been made in developing new antagonist radioligands with higher dopamine D2 receptor affinity, such as 11C-FLB457 or 18F-fallypride and agonist radioligands like 11C-PHNO. Together with developments in the methodology for measuring neurotransmitter dynamics extending to extrastriatal brain regions, this has provided an increased understanding of the role of synaptic dopamine in drug action, normal neuropsychology, pathophysiology of addiction, Parkinson’s disease, and schizophrenia. Efforts in measuring neurotransmitter dynamics have currently extended to other targets such as the serotonin (Gryglewski et al., 2014; Erritzoe et al., 2019), noradrenaline, γ-aminobutyric acid, glutamate, acetylcholine, and opioid peptides [see review (Sander and Hesse, 2017)].
Data analysis methods have been developed to detect and characterize endogenous neurotransmitter release during dynamic PET imaging with a displaceable radioligand, in response to pharmacological, behavioral, or cognitive interventions, through mechanisms of a “pure competition” radioligand-target displacement model. Changes in binding potential (BPND) represent receptors as static targets that are not dynamically regulated by processes like internalization within post-synaptic membranes [see Ginovart (2005) for a review of this topic]. Models that incorporate receptor internalization and its effect on PET quantification have been proposed in by integrating PET with functional magnetic resonance imaging (fMRI) studies [see section “Imaging Dynamic Neurotransmission Using Simultaneous PET and Functional MRI” and Sander et al. (2015)]. The most commonly used drug to induce dopamine change is amphetamine, exhibiting several well-documented effects on dopamine neurotransmission, including increased synthesis and release together with inhibited degradation and uptake.
Conventional PET methods to estimate the BPND are commonly based on kinetic models that assume that the system under investigation is at equilibrium. However, this assumption is intentionally violated in studies using pharmacological or behavioral stimuli to invoke transient dopamine release. When the assumption of a steady-state neurotransmitter level is violated, conventional analysis methods, which rely on time-invariant parameters (time-invariant model), may produce biased BPND estimates (Yoder et al., 2004; Sullivan et al., 2013). Therefore, methods to detect neurotransmitter release during an activation study have been developed allowing for a non-constant dopamine level during the scan (i.e., time-variant parameter models). For example, the linearized simplified reference tissue model or the linearized simplified reference region model (LSRTM or LSRRM) models dopamine release as an exponential decay that peaks instantaneously at the start of the stimulus (Alpert et al., 2003). A more flexible and innovative kinetic model to fully characterize endogenous neurotransmission, named the linear parametric neurotransmitter PET (lp-ntPET) model (Morris et al., 2005; Normandin et al., 2012; Wang et al., 2017), allows the dopamine curve to take on a variety of forms with a peak dopamine concentration to occur sometime after the start of the task. However, the performance of lp-ntPET remains suboptimal. It is sensitive to noise and limited in sensitivity and accuracy.
Additionally, dynamic changes in neurotransmission are also known to contribute to blood oxygenation level dependent (BOLD) and cerebral blood volume (CBV) changes (Mandeville et al., 2013). The advent of hybrid PET/MRI scanners paved the way for more comprehensive investigation of the relationship of simultaneous changes in neuroreceptor occupancy and hemodynamic parameters, therefore clarifying the contributions of specific neurotransmitter systems to dynamic changes in BOLD response (Sander et al., 2013).
In this article, we first summarize the basics of various PET methodological approaches to measure dynamic changes in endogenous neurotransmitter levels induced by pharmacological or behavioral challenges. Next, we discuss how the use of simultaneous PET and fMRI can provide complementary and new views on quantitative imaging of neurotransmission.
Approaches for Measuring Endogenous Neurotransmitter Release: The Current Status
Time-Invariant Models
Traditional analyses of dynamic PET quantification of changes in neurotransmitter levels estimate the BPND, a static parameter that represents the potential for specific binding of the radioligand to specific enzymes in the brain, by fitting the dynamic data with compartmental or graphical (linearized) models. These models, including reference region models, such as the SRTM (Lammertsma and Hume, 1996), the Logan graphical reference method (Logan et al., 1990), and equilibrium analysis, assume that the system under investigation is in equilibrium condition. Another assumption is that the endogenous neurotransmitter and receptor concentration does not change during the course of the scan. Under these conditions, BPND is estimated from a reference tissue model considering the time activity curve of the reference region as an indirect input function to the kinetic model of the target region.
In these traditional studies, BPND is measured at rest and after a specific (cognitive or pharmacological) stimulus, during two separate PET sessions. The standard calculated endpoint to quantify neurotransmitter release is formulated as the fractional reduction in the radiotracer BPND following the stimulus (post-stimulus, BP) compared to the BPND at rest or baseline (pre-stimulus) (BP), Eq. (1):
A decrease in BPND is used as an index of neurotransmitter release induced by a stimulus. This approach has been used conventionally with 11C-raclopride, 18F-fallypride, 11C-(+)-PHNO, 11C-FLB457, 11C-CIMBI-36, and 11C-carfentanil PET, to quantify respective endogenous dopamine (Martinez et al., 2003; Montgomery et al., 2007; Wai et al., 2019; Zakiniaeiz et al., 2019; Whitton et al., 2020), serotonin (Erritzoe et al., 2019), and opioid release (Turton et al., 2018) before and after the administration of cannabis, nicotine, methylphenidate, and amphetamine.
However, traditional models that estimate BPND do not contain explicit functions to describe short-lived neurotransmitter responses. When the stimulus is applied during a single scanning session, inconsistency in the results [of smoking studies, for example, Brody et al. (2004, 2010)] could be attributed to limitations of the conventional models. As BPND estimates become sensitive to the amount of data used post-stimulus, poor fitting of the data can lead to biased estimates of BP demonstrated by Sullivan et al. (2013), as critically reviewed by Liu et al. (2019). Therefore, a more flexible time-varying kinetic model would be better configured than conventional time-invariant models to reliably and reproducibly capture transient responses.
Temporal Changes in Neurotransmitter Levels: Models With Time-Varying Terms
The first neurotransmitter competition kinetic model that was implemented to detect and characterize changes in ligand binding using only a single PET experiment is the linear extension of the reference region model (LSRTM or LSRRM), proposed by Alpert et al. (2003). LSRRM accounts for time-dependent changes in radiotracer binding, influx, and clearance induced by cognitive or drug effects in a single scan session, with the inclusion of both baseline and activation terms. LSRRM models neurotransmitter release as an exponential decay that peaks instantaneously at the start of the stimulus. It assumes that the physiologic steady state is not maintained throughout the paradigm but accounts for time-variation in the dissociation rate of ligand k2a = k2/[1 + BPND], where k2 is the tissue-to-plasma efflux constant in the tissue region. The dopamine–radioligand competition at the receptor site is reflected by a temporal change in apparent dissociation rate, which is accounted for by adding a time-dependent parameter γh(t) to a fixed k2a. The parameter γ represents the amplitude of the ligand displacement, hence the peak dopamine level. The function h(t) in Eq. (2) describes the rapid change after task onset and dissipation over time, where u(t) is the unit step function, while τ controls the rate at which activation effects die away and T indicates the timing of stimulus initiation:
An increased k2a reflects a decreased BPND for D2/D3 receptors, which in turn can be ascribed to an increased dopamine release and will result in a positive value of γ.
There have been promising results using a single 18F-fallypride injection protocol and the LSRRM to describe extrastriatal and striatal dopamine release induced by emotional processing (Badgaiyan et al., 2009), attention, reward, and stress task (Christian et al., 2006; Lataster et al., 2011; Ceccarini et al., 2012; Kasanova et al., 2017), and also during dopamine-releasing pharmacological challenges, such as intravenous alcohol administration (Leurquin-Sterk et al., 2018) and Δ9-THC (Kuepper et al., 2013), as can be seen in Figure 1.
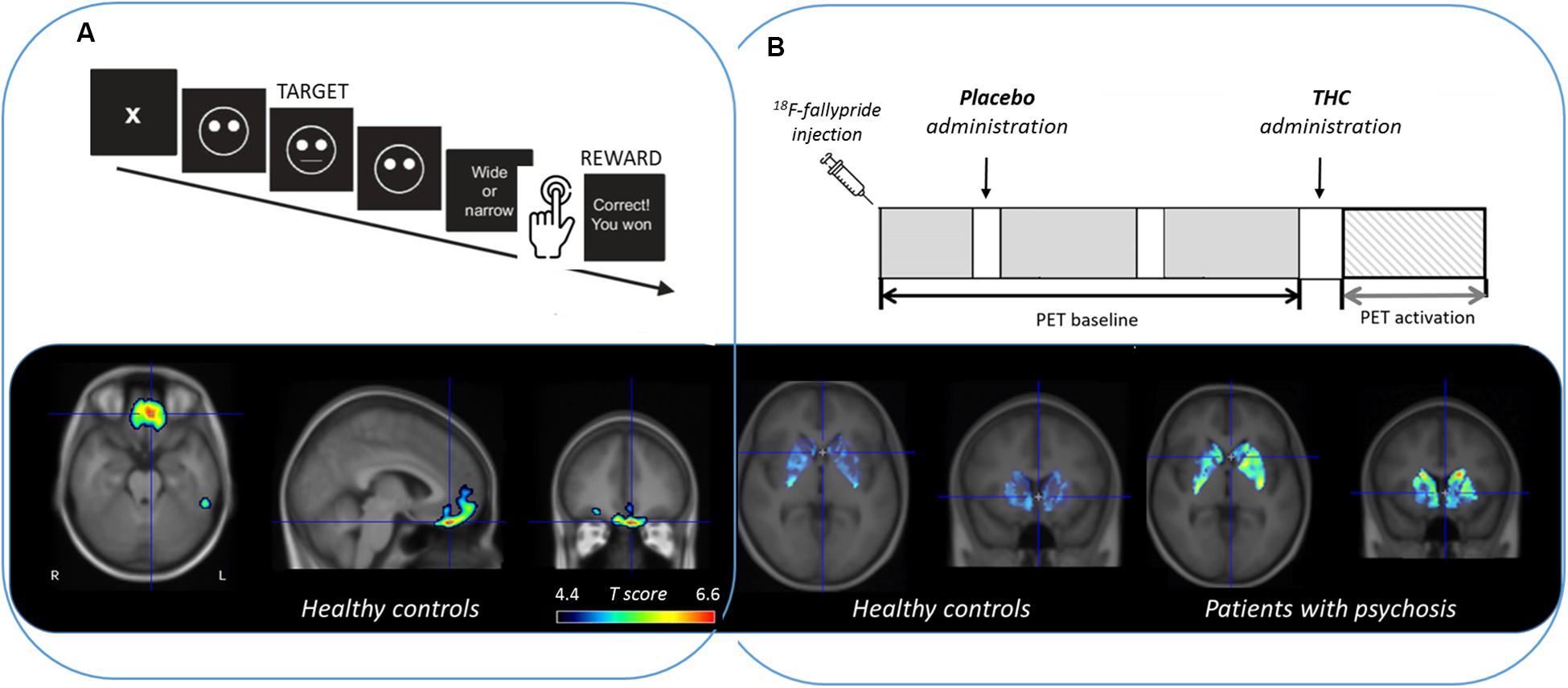
Figure 1. Extrastriatal and striatal dopamine release measured with 18F-fallypride PET and quantified with LSRRM (reported as a statistical parametric t map based on the significance of γ) during (A) a reward responsiveness learning task in healthy controls (Ceccarini et al., 2012) and (B) Δ9-THC (the main psychoactive ingredient of cannabis) administration in healthy controls compared to patients with psychosis (Kuepper et al., 2013).
However, LSRRM assumes that effects of the stimuli on endogenous neurotransmitter release are instantaneous, maximal at the start time of stimulation [equal to T, see Eq. (2)], and decay exponentially to baseline thereafter (at rate τ). When any of these assumptions are violated, the estimates of the model parameters could be biased and/or inaccurate.
More flexible kinetic approaches and associated resolution models have been proposed to resolve these limitations, such as the lp-ntPET model (Morris et al., 2005; Normandin et al., 2012; Kim et al., 2014). In order to estimate the temporal characteristics of a transient neurotransmitter component in PET data with less stringent assumptions than LSRRM, lp-ntPET employs gamma-variate basis functions spanning a wide range of feasible neurotransmitter shapes, times of onset, and duration.
Specifically, lp-ntPET is the union of the conventional multilinear reference tissue model (MRTM) (Ichise et al., 2003) and a time-varying term that describes the transient neurotransmitter term, Eq. (3):
The novelty and the flexibility of the lp-ntPET approach consists of the use of the, γ, gamma-variate functions hi(t), where γ describes the response magnitude, and the three implicit parameters (tD, tP, α) describe the time course of the response (tD, the start of the response or time delay; tP, the peak time; and α, the decay rate or sharpness), assuming values incremented over finite intervals. The efficiency of lp-ntPET makes it practical to perform a voxel-by-voxel analysis of the whole brain or for localized activation patterns. The model has been successfully applied to 11C-raclopride data to estimate the temporal dynamics of dopamine release in the mesolimbic circuit during smoking (Cosgrove et al., 2014; Kim et al., 2014). Recently, lp-ntPET has been applied in preclinical 11C-raclopride PET studies following amphetamine administration (Angelis et al., 2019).
To evaluate the validity of extending lp-ntPET to the cortex, Liu et al. (2018) compared the ability of the lp-ntPET model and LSRRM to detect and characterize cortical dopamine release induced by a stress task with simulated 18F-fallypride PET data. In 18F-fallypride PET studies that detect cortical DA release induced by a sustained behavioral stress challenge, simulations suggest that both LSSRM and MRTM methods produce comparable t-scores over a wide range of sharpness, α, and rise time for DA signals. However, LSRRM consistently outperformed MRTM in terms of fitting accuracy. This may be relevant if the study goal is to characterize the PET signal and/or to deduce the dopamine signal shape or duration. Further, LSRRM and other time-varying models may be the safer choice when the duration of the dopamine response and/or its shape are pertinent or unknown (Liu et al., 2018).
The significance of the responses estimated by lp-ntPET can be assessed using model selection criteria and statistical testing on γ, the estimated response magnitude, obtained by either t or F-test values comparing the goodness of fit of MRTM and lp-ntPET for each data set (Normandin et al., 2012). However, it has been reported that the t-score is not an indicator of goodness of fit but merely a measure of the magnitude of an estimated parameter relative to its variability (Liu et al., 2018). Additionally, the goodness of fit metrics depend critically on the number of parameters in the model and, together with the number of basis functions, have an impact on the false positive rate (FPR) (Liu and Morris, 2019). Given their findings, Liu and Morris (2019). proposed a set of modified goodness-of-fit metrics that adapt to the number of basis functions to maintain a stable FPR (Liu and Morris, 2020). Another recent Monte Carlo method proposed by Bevington et al. (2020) improves the detection sensitivity while preserving the cluster size threshold.
An optimization of the lp-ntPET displacement modeling method, called 2-step lp-ntPET, has been proposed by Mérida et al. (2018) where the model parameters are estimated in two steps, starting with the estimation of R1, k2, and k2a with MRTM followed by the estimation of the release parameters (tD, tP, α, and γ) (unpublished results). In this way, the kinetic parameters are estimated more accurately, independently from the magnitude of the endogenous neurotransmitter release, and the macroparameter, the displacement ratio, seems to allow better detection of neurotransmitter discharge.
Finally, recent developments regarding the investigation of neurotransmitter dynamics have been introduced. For instance, the assessment of temporal changes in dopamine release has been proposed to advance to a resolution of a few minutes using detailed modeling of dopamine dynamics (Lippert et al., 2019). Furthermore, the assessment of rapid changes in dopamine release and synthesis rates during cognitive performance have also been investigated by extending the technique of functional PET (fPET) imaging using 11C-raclopride (Zhang et al., 2019) and 18F-FDOPA (Hahn et al., 2019).
Imaging Dynamic Neurotransmission Using Simultaneous PET and Functional MRI
With the current availability of scanners capable of simultaneous dynamic PET/MRI acquisitions, there is an increasing interest in measuring endogenous neurotransmitter release and time-varying measures of receptor occupancies in combination with dynamic neurovascular changes using fMRI techniques. The capability to combine multi-modal fMRI measures and neuroreceptor PET during activation paradigms provides an unprecedented opportunity to study neurotransmission dynamics through multiple lenses in the living brain. Indeed, as technical and methodological advances in simultaneous PET/MRI have matured (Ladefoged et al., 2017; Sari et al., 2017; Chen et al., 2018), we have seen an emergence of biological questions that favor simultaneous acquisitions of PET and MR signals (Cecchin et al., 2017; Streeter Barrett et al., 2019; Sander et al., 2020).
A revolution offered by PET/fMRI is the potential for resolving dynamic transitions in brain physiology, chemistry, and neurotransmission in space and time. Being able to acquire simultaneous functional measurements under the same physiological or pharmacological conditions not only reduces confounding factors, interscan, and intrasubject variability but also enables cross-validation of biological measurements. A key importance of simultaneously acquired PET/fMRI signals for imaging dynamic neurotransmission is the ability to link actions at receptors, such as ligand binding or adaptations, to changes in hemodynamics. Alterations in local hemodynamics (such as BOLD, local perfusion, or CBV) have been shown to occur in response to changes in neuronal activity and during neurotransmission (Logothetis et al., 2001; Jenkins, 2012), although the topic of neurovascular coupling is still an active research area. The opportunity to now study a new facet of neurotransmission—neurotransmitter or receptor changes and its relationship to fMRI signal—will further elucidate the nature and interpretation of fMRI. Conversely, being able to track vascular changes during PET receptor measurements can address important questions like the dependence of PET signals on radiotracer delivery. On the latter topic, it has been demonstrated that blood flow is not a confound during dynamic PET neuromodulation experiments that evaluate within-scan time-dependent challenges (Sander et al., 2019). The advent of simultaneous PET/MR scanners has paved the way for investigations that evaluate how dopamine receptor densities may organize functional cortical networks during working memory (Roffman et al., 2016) or delineate the role of opioid receptor availability while evaluating pain processing pathways (Karjalainen et al., 2017). Time-varying PET kinetic models will be a crucial component of a comprehensive investigation between functional brain organization, physiological and molecular processes within similar timescales.
Several PET/MRI studies have been performed that have investigated the effects of time-varying neurotransmitter modulations (using pharmacological challenges) on fMRI and receptor-specific PET signals simultaneously. Much of this line of work has focused on establishing contributions of the dopaminergic system on the fMRI response, demonstrating that the hemodynamic response can be directly linked to D2/D3 receptor occupancy through neurovascular coupling mechanisms (Sander et al., 2013). The relationship between hemodynamic changes (e.g., BOLD, CBV, or CBF) as measured with different fMRI techniques, receptor occupancy, and endogenous neurotransmitter has been described with a neurovascular coupling model (Sander et al., 2013, 2015). Considering specifically a ligand L (e.g., an administered drug) with efficacy εL that binds to D2 receptors and displaces dopamine (DA), a linear relationship between hemodynamic changes (ΔH) and receptor occupancy (θ) is expressed as:
where ND2 is a neurovascular coupling constant (determined experimentally), Bmax,D2 is the total concentration of D2 receptors, θL(t) is the time-varying occupancy of an exogenous ligand (if present), and ΔθDA(t) is the time-varying occupancy of dopamine. In a more general framework, the functional hemodynamic response ΔH can be expressed as the sum of receptor occupancies by different ligands, L, (endogenous and exogenous) and receptors, R, as:
This model incorporates the possibility of any number of receptors and neurotransmitters (or ligands) that may contribute to the hemodynamic response. Experimental evidence for this relationship has been demonstrated using D2/D3 antagonism and agonism (Figure 2), yet remains to be evaluated for multiple receptor systems working in parallel. The temporal correlation proposed in this model can be complicated by other biological parameters like receptor desensitization and internalization in vivo and requires further expansion of these model frameworks (Sander et al., 2015).
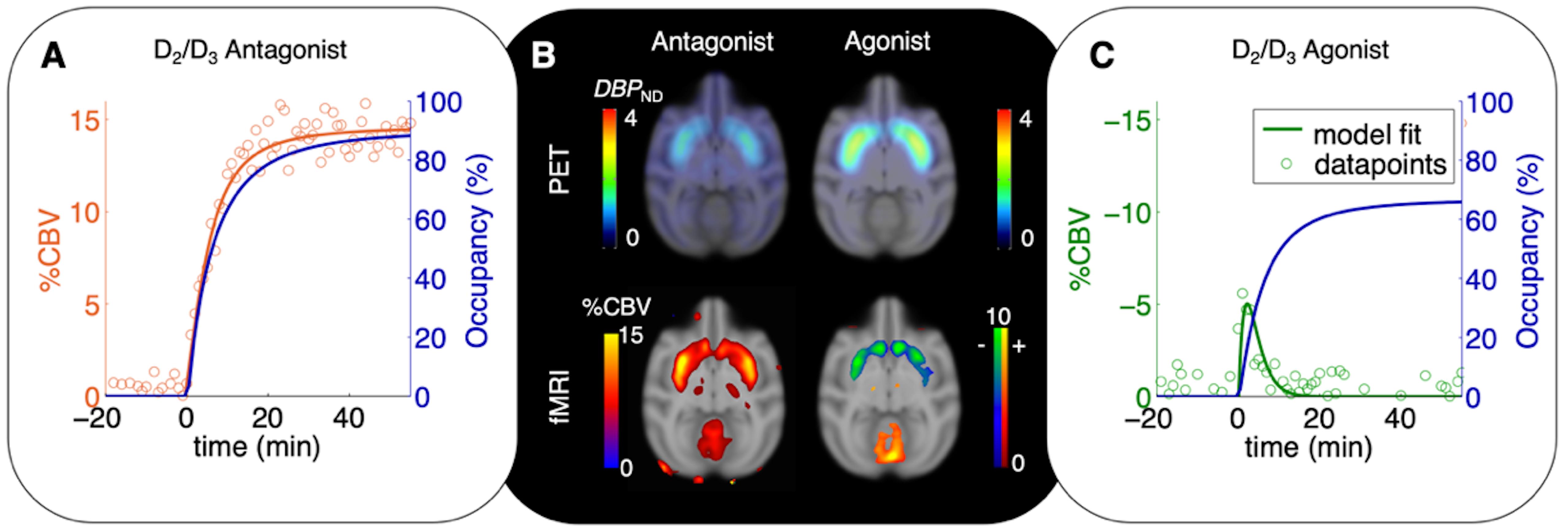
Figure 2. Time-varying occupancy (blue) and cerebral blood volume (CBV) responses [positive (orange) or negative (green) percent change] due to a pharmacological response from the D2/D3 antagonist prochlorperazine (A) and the D2/D3 agonist quinpirole (C) in non-human primates. Occupancy curves are derived from a time-varying specific binding term during kinetic modeling, and the CBV curves are fitted using the general linear model. (B) 11C-raclopride-PET binding potential maps (upper row) and CBV maps shown at peak value of the dynamic modeling term (Sander et al., 2015).
The effects of a partial serotonin receptor agonist have also been evaluated using simultaneous PET/fMRI (Hansen et al., 2017), demonstrating that biphasic functional signals can be linked to serotonin receptor occupancies. As an example of stimulus-based simultaneous PET/fMRI studies, the opioid pain system has been examined using 11C-diprenorphine (Wey et al., 2014). In the thalamus, co-localized and positively correlated fMRI and PET signal changes suggest that opioid neurotransmission contributes to pain-induced fMRI changes.
The use of time-varying models to quantify dynamic receptor occupancy together with fMRI has been key to these types of studies. For the purpose of comparing time-dependent kinetic rate constants, outcome measures such as “dynamic binding potential” (DBP) have been proposed to signify the dynamic nature of the system (Sander et al., 2013). Since dynamic measurements with PET versus fMRI still operate on different time resolutions (minutes vs. seconds), the combination of time-varying outcome measures from PET kinetic models with fast-changing repetitive signals in fMRI can present a challenge for direct and equivalent comparisons in the temporal domain. As more studies are carried out in this area, careful multi-modal experimental design together with integrating multi-modal models will no doubt play a key role.
The exploration of dynamic neurotransmission with simultaneous PET/fMRI is still in its infancy. Within the field of neuropsychiatry, PET/fMRI can help evaluate whole-brain functional effects of antipsychotic drug treatments in relation to neurotransmitter or receptor changes (Selvaggi et al., 2019) and shed a light on distributed networks that these drugs modulate. Connecting findings from multi-modal outcomes in complex mental illness, e.g., in schizophrenia, can also serve to connect hyper- and hypo-neurotransmitter tone to cortical function during relevant cognitive tasks (Slifstein et al., 2015). These approaches extend the field beyond traditional hemodynamic-based functional imaging methods or pure pharmacological target evaluations.
Conclusion
Quantifying dynamic neurotransmission in the living brain with PET has provided insight into the molecular dynamics of the living brain. Both time-invariant and time-varying pharmacokinetic models have played an important role in the ability to accurately quantify neurotransmitter dynamics. In the age of multi-modal methods, simultaneous PET/fMRI can have a profound impact on our understanding of neuropsychiatric diseases, drawing connections between neurotransmitter imbalances to wide-spread changes in functional activation in diseases such as addiction, psychosis, and depression. Overall, models and methods for imaging neurotransmission, non-invasively, will play an important role in elucidating mechanisms underlying brain (dys)function in health and disease.
Author Contributions
JC and CS revised the literature and wrote the first draft of the manuscript. HL, KV, and EM revised it critically for important intellectual content. All authors contributed to the article and approved the submitted version.
Funding
JC is a postdoctoral fellow from Research Foundation Flanders (FWO/12R1619N). KV is a senior clinical research fellow from FWO. HL received support from Robert E. Apafel Fellowship. HL and EM received support from the National Institutes of Health (NIH) (Grant No. R01DA038709). CS received support from the NIH (Grant Nos. R00DA043629 and R01NS112295).
Conflict of Interest
EM is a half-time employee of the company Invicro LLC.
The remaining authors declare that the research was conducted in the absence of any commercial or financial relationships that could be construed as a potential conflict of interest.
References
Alpert, N. M., Badgaiyan, R. D., Livni, E., and Fischman, A. J. (2003). A novel method for noninvasive detection of neuromodulatory changes in specific neurotransmitter systems. Neuroimage 19, 1049–1060. doi: 10.1016/S1053-8119(03)00186-1
Angelis, G. I., Gillam, J. E., Ryder, W. J., Fulton, R. R., and Meikle, S. R. (2019). Direct estimation of voxel-wise neurotransmitter response maps from dynamic PET data. IEEE Trans. Med. Imaging 38, 1371–1383. doi: 10.1109/TMI.2018.2883756
Badgaiyan, R. D., Fischman, A. J., and Alpert, N. M. (2009). Dopamine release during human emotional processing. Neuroimage 47, 2041–2045. doi: 10.1016/j.neuroimage.2009.06.008
Bevington, C. W., Cheng, J.-C. K., Klyuzhin, I. S., Cherkasova, M. V., Winstanley, C. A., and Sossi, V. (2020). A monte carlo approach for improving transient dopamine release detection sensitivity. J. Cereb. Blood Flow Metab. 12:271678X20905613. doi: 10.1177/0271678X20905613
Brody, A. L., London, E. D., Olmstead, R. E., Allen-Martinez, Z., Shulenberger, S., Costello, M. R., et al. (2010). Smoking-induced change in intrasynaptic dopamine concentration: effect of treatment for tobacco dependence. Psychiatry Res. 183, 218–224. doi: 10.1016/j.pscychresns.2009.06.004
Brody, A. L., Olmstead, R. E., London, E. D., Farahi, J., Meyer, J. H., Grossman, P., et al. (2004). Smoking-induced ventral striatum dopamine release. Am. J. Psychiatry 161, 1211–1218. doi: 10.1176/appi.ajp.161.7.1211
Ceccarini, J., Vrieze, E., Koole, M., Muylle, T., Bormans, G., Claes, S., et al. (2012). Optimized in vivo detection of dopamine release using18F- fallypride PET. J. Nucl. Med. 53, 1565–1572. doi: 10.2967/jnumed.111.099416
Cecchin, D., Palombit, A., Castellaro, M., Silvestri, E., Bui, F., Barthel, H., et al. (2017). Brain PET and functional MRI: why simultaneously using hybrid PET/MR systems? Q. J. Nucl. Med. Mol. Imaging 61, 345–359. doi: 10.23736/S1824-4785.17.03008-4
Chen, K. T., Salcedo, S., Chonde, D. B., Izquierdo-Garcia, D., Levine, M. A., Price, J. C., et al. (2018). MR-assisted PET motion correction in simultaneous PET/MRI studies of dementia subjects. J. Magn. Reson. Imaging 48, 1288–1296. doi: 10.1002/jmri.26000
Christian, B. T., Lehrer, D. S., Shi, B., Narayanan, T. K., Strohmeyer, P. S., Buchsbaum, M. S., et al. (2006). Measuring dopamine neuromodulation in the thalamus: using [F-18]fallypride PET to study dopamine release during a spatial attention task. Neuroimage 31, 139–152. doi: 10.1016/j.neuroimage.2005.11.052
Cosgrove, K. P., Wang, S., Kim, S. J., McGovern, E., Nabulsi, N., Gao, H., et al. (2014). Sex differences in the brain’s dopamine signature of cigarette smoking. J. Neurosci. 34, 16851–16855. doi: 10.1523/JNEUROSCI.3661-14.2014
da Cunha-Bang, S., Ettrup, A., Mc Mahon, B., Skibsted, A. P., Schain, M., Lehel, S., et al. (2019). Measuring endogenous changes in serotonergic neurotransmission with [11 C]Cimbi-36 positron emission tomography in humans. Transl. Psychiatry 9:134. doi: 10.1038/s41398-019-0468-8
Erritzoe, D., Ashok, A. H., Searle, G. E., Colasanti, A., Turton, S., Lewis, Y., et al. (2019). Serotonin release measured in the human brain: a PET study with [11C]CIMBI-36 and d-amphetamine challenge. Neuropsychopharmacology 45, 804–810. doi: 10.1038/s41386-019-0567-5
Finnema, S. J., Scheinin, M., Shahid, M., Lehto, J., Borroni, E., Bang-Andersen, B., et al. (2015). Application of cross-species PET imaging to assess neurotransmitter release in brain. Psychopharmacology 232, 4129–4157. doi: 10.1007/s00213-015-3938-6
Ginovart, N. (2005). Imaging the dopamine system with in vivo [11C]raclopride displacement studies: understanding the true mechanism. Mol. Imaging Biol. 7, 45–52. doi: 10.1007/s11307-005-0932-0
Gryglewski, G., Lanzenberger, R., Kranz, G. S., and Cumming, P. (2014). Meta-analysis of molecular imaging of serotonin transporters in major depression. J. Cereb. Blood Flow Metab. 34, 1096–1103. doi: 10.1038/jcbfm.2014.82
Hahn, A., Reed, M. B., Pichler, V., Michenthaler, P., Rischka, L., Godbersen, G. M., et al. (2019). Sex differences in monetary reward and punishment processing are associated with fast dynamics of dopamine synthesis. bioRxiv [Preprint]. doi: 10.1101/2019.12.23.886812
Hansen, H. D., Mandeville, J. B., Sander, C. Y., Hooker, J. M., Catana, C., Rosen, B. R., et al. (2017). Functional characterization of 5-HT1B receptor drugs in nonhuman primates using simultaneous PET-MR. J. Neurosci. 37, 10671–10678. doi: 10.1523/JNEUROSCI.1971-17.2017
Ichise, M., Liow, J. S., Lu, J. Q., Takano, A., Model, K., Toyama, H., et al. (2003). Linearized reference tissue parametric imaging methods: application to [11C]DASB positron emission tomography studies of the serotonin transporter in human brain. J. Cereb. Blood Flow Metab. 23, 1096–1112. doi: 10.1097/01.WCB.0000085441.37552.CA
Jenkins, B. G. (2012). Pharmacologic magnetic resonance imaging (phMRI): imaging drug action in the brain. Neuroimage 62, 1072–1085. doi: 10.1016/j.neuroimage.2012.03.075
Karjalainen, T., Karlsson, H. K., Lahnakoski, J. M., Glerean, E., Nuutila, P., Jääskeläinen, I. P., et al. (2017). Dissociable roles of cerebral μ-opioid and type 2 dopamine receptors in vicarious pain: a combined PET-fMRI study. Cereb. Cortex 27, 4257–4266. doi: 10.1093/cercor/bhx129
Kasanova, Z., Ceccarini, J., Frank, M. J., Amelsvoort, T. V., Booij, J., Heinzel, A., et al. (2017). Striatal dopaminergic modulation of reinforcement learning predicts reward—oriented behavior in daily life. Biol. Psychol. 127, 1–9. doi: 10.1016/j.biopsycho.2017.04.014
Kim, S. J., Sullivan, J. M., Wang, S., Cosgrove, K. P., and Morris, E. D. (2014). Voxelwise lp-ntPET for detecting localized, transient dopamine release of unknown timing: sensitivity analysis and application to cigarette smoking in the pet scanner. Hum. Brain Mapp. 35, 4876–4891. doi: 10.1002/hbm.22519
Kuepper, R., Ceccarini, J., Lataster, J., Van Os, J., and Van Kroonenburgh, M. (2013). Delta-9-tetrahydrocannabinol-induced dopamine release as a function of psychosis risk: 18 F-Fallypride positron emission tomography study. PLoS One 8:70378. doi: 10.1371/journal.pone.0070378
Ladefoged, C. N., Law, I., Anazodo, U., St Lawrence, K., Izquierdo-Garcia, D., Catana, C., et al. (2017). A multi-centre evaluation of eleven clinically feasible brain PET/MRI attenuation correction techniques using a large cohort of patients. Neuroimage 147, 346–359. doi: 10.1016/j.neuroimage.2016.12.010
Lammertsma, A. A., and Hume, S. P. (1996). Simplified reference tissue model for PET receptor studies. Neuroimage 4, 153–158. doi: 10.1006/nimg.1996.0066
Lataster, J., Collip, D., Ceccarini, J., Haas, D., Booij, L., van Os, J., et al. (2011). Psychosocial stress is associated with in vivo dopamine release in human ventromedial prefrontal cortex: a positron emission tomography study using [18F]fallypride. Neuroimage 58, 1081–1089. doi: 10.1016/j.neuroimage.2011.07.030
Leurquin-Sterk, G., Ceccarini, J., Crunelle, C. L., Weerasekera, A., de Laat, B., Himmelreich, U., et al. (2018). Cerebral dopaminergic and glutamatergic transmission relate to different subjective responses of acute alcohol intake: an in vivo multimodal imaging study. Addict. Biol. 23, 931–944. doi: 10.1111/adb.12542
Lippert, R. N., Cremer, A. L., Edwin Thanarajah, S., Korn, C., Jahans-Price, T., Burgeno, L. M., et al. (2019). Time-dependent assessment of stimulus-evoked regional dopamine release. Nat. Commun 10:336. doi: 10.1038/s41467-018-08143-4
Liu, H., Ceccarini, J., de Laat, B., Lataster, J., Myin-Germeys, I., and Morris, E. D. (2018). A comparison of kinetic models for PET imaging of cortical dopamine release induced by a task. J. Nucl. Med. 59(Suppl. 1):502.
Liu, H., and Morris, E. D. (2019). Controlling the false positive rate for lp-ntPET: a correction to goodness of fit metrics for “‘effective”’ number of parameters. J. Nucl. Med. 60(Suppl. 1):580.
Liu, H., Zakiniaeiz, Y., Cosgrove, K. P., and Morris, E. D. (2019). Toward whole-brain dopamine movies: a critical review of PET imaging of dopamine transmission in the striatum and cortex. Brain Imaging Behav. 13, 314–322. doi: 10.1007/s11682-017-9779-7
Liu, H., and Morris, E. D. (2020). Model comparison metrics require adaptive correction if parameters are discretized: proof-of-concept applied to transient signals in dynamic PET. IEEE Trans. Med. Imaging doi: 10.1109/TMI.2020.2969425
Logan, J., Fowler, J. S., Volkow, N. D., Wolf, A. P., Dewey, S. L., Schlyer, D. J., et al. (1990). Graphical analysis of reversible radioligand binding from time-activity measurements applied to [N-11C-methyl]-(-)-cocaine PET studies in human subjects. J. Cereb. Blood Flow Metab. 10, 740–747. doi: 10.1038/jcbfm.1990.127
Logothetis, N. K., Pauls, J., Augath, M., Trinath, T., and Oeltermann, A. (2001). Neurophysiological investigation of the basis of the fMRI signal. Nature 412, 150–157. doi: 10.1038/35084005
Mandeville, J. B., Sander, C. Y. M., Jenkins, B. G., Hooker, J. M., Catana, C., Vanduffel, W., et al. (2013). A receptor-based model for dopamine-induced fMRI signal. Neuroimage 75, 46–57. doi: 10.1016/J.NEUROIMAGE.2013.02.036
Martinez, D., Slifstein, M., Broft, A., Mawlawi, O., Hwang, D.-R., Huang, Y., et al. (2003). Imaging human mesolimbic dopamine transmission with positron emission tomography. Part II: amphetamine-induced dopamine release in the functional subdivisions of the striatum. J. Cereb. Blood Flow Metab. 23, 285–300. doi: 10.1097/01.WCB.0000048520.34839.1A
Mérida, I., Olivier, F., Hammers, A., Redouté, J., Reilhac, A., Costes, N., et al. (2018). “Kinetic modelling for endogenous neurotransmitter discharge characterization using pet imaging: optimization of lp-ntPET,” in The XII International Symposium of Functional Neuroreceptor Mapping of the Living Brain (NRM18) (London).
Montgomery, A. J., Asselin, M.-C., Farde, L., and Grasby, P. M. (2007). Measurement of methylphenidate-induced change in extrastriatal dopamine concentration using [11 C]FLB 457 PET. J. Cereb. Blood Flow Metab. 27, 369–377. doi: 10.1038/sj.jcbfm.9600339
Morris, E. D., Yoder, K. K., Wang, C., Normandin, M. D., Zheng, Q.-H., Mock, B., et al. (2005). ntPET: a new application of PET imaging for characterizing the kinetics of endogenous neurotransmitter release. Mol. Imaging 4, 473–489.
Normandin, M. D., Schiffer, W. K., and Morris, E. D. (2012). A linear model for estimation of neurotransmitter response profiles from dynamic PET data. Neuroimage 59, 2689–2699. doi: 10.1016/j.neuroimage.2011.07.002
Paterson, L. M., Tyacke, R. J., Nutt, D. J., and Knudsen, G. M. (2010). Measuring endogenous 5-HT release by emission tomography: promises and pitfalls. J. Cereb. Blood Flow Metab. 30, 1682–1706. doi: 10.1038/jcbfm.2010.104
Roffman, J. L., Tanner, A. S., Eryilmaz, H., Rodriguez-Thompson, A., Silverstein, N. J., Ho, N. F., et al. (2016). Dopamine D1 signaling organizes network dynamics underlying working memory. Sci. Adv. 2:e1501672. doi: 10.1126/sciadv.1501672
Sander, C. Y., Hansen, H. D., and Wey, H.-Y. (2020). Advances in simultaneous PET/MR for imaging neuroreceptor function. J. Cereb. Blood Flow Metab. 40, 1148–1166. doi: 10.1177/0271678X20910038
Sander, C. Y., and Hesse, S. (2017). News and views on in-vivo imaging of neurotransmission using PET and MRI. Q. J. Nucl. Med. Mol. Imaging 61, 414–428. doi: 10.23736/S1824-4785.17.03019-9
Sander, C. Y., Hooker, J. M., Catana, C., Normandin, M. D., Alpert, N. M., Knudsen, G. M., et al. (2013). Neurovascular coupling to D2/D3 dopamine receptor occupancy using simultaneous PET/functional MRI. Proc. Natl. Acad. Sci. U.S.A. 110, 11169–11174. doi: 10.1073/pnas.1220512110
Sander, C. Y., Hooker, J. M., Catana, C., Rosen, B. R., and Mandeville, J. B. (2015). Imaging agonist-induced D2/D3 receptor desensitization and internalization in vivo with PET/fMRI. Neuropsychopharmacology 41, 1427–1436. doi: 10.1038/npp.2015.296
Sander, C. Y., Mandeville, J. B., Wey, H. Y., Catana, C., Hooker, J. M., and Rosen, B. R. (2019). Effects of flow changes on radiotracer binding: simultaneous measurement of neuroreceptor binding and cerebral blood flow modulation. J. Cereb. Blood Flow Metab. 39, 131–146. doi: 10.1177/0271678X17725418
Sari, H., Erlandsson, K., Law, I., Larsson, H. B., Ourselin, S., Arridge, S., et al. (2017). Estimation of an image derived input function with MR-defined carotid arteries in FDG-PET human studies using a novel partial volume correction method. J. Cereb. Blood Flow Metab. 37, 1398–1409. doi: 10.1177/0271678X16656197
Selvaggi, P., Hawkins, P. C. T., Dipasquale, O., Rizzo, G., Bertolino, A., Dukart, J., et al. (2019). Increased cerebral blood flow after single dose of antipsychotics in healthy volunteers depends on dopamine D2 receptor density profiles. Neuroimage 188, 774–784. doi: 10.1016/j.neuroimage.2018.12.028
Slifstein, M., van de Giessen, E., Van Snellenberg, J., Thompson, J. L., Narendran, R., Gil, R., et al. (2015). Deficits in prefrontal cortical and extrastriatal dopamine release in schizophrenia: a positron emission tomographic functional magnetic resonance imaging study. JAMA Psychiatry 72, 316–324. doi: 10.1001/jamapsychiatry.2014.2414
Streeter Barrett, F., Shi, K., Minhas, D. S., Juhasz, C., Zhu, X., and Zhu, Y. (2019). MRI-Driven PET image optimization for neurological applications. Front. Neurosci. 13:782. doi: 10.3389/fnins.2019.00782
Sullivan, J. M., Kim, S. J., Cosgrove, K. P., and Morris, E. D. (2013). Limitations of SRTM, Logan graphical method, and equilibrium analysis for measuring transient dopamine release with [(11)C]raclopride PET. Am. J. Nucl. Med. Mol. Imaging 3, 247–260.
Turton, S., Myers, J. F. M., Mick, I., Colasanti, A., Venkataraman, A., Durant, C., et al. (2018). Blunted endogenous opioid release following an oral dexamphetamine challenge in abstinent alcohol-dependent individuals. Mol. Psychiatry 25, 1–10. doi: 10.1038/s41380-018-0107-4
Wai, J. M., Grassetti, A., Slifstein, M., Matuskey, D., Nabulsi, N., Ropchan, J., et al. (2019). Binge alcohol use is not associated with alterations in striatal dopamine receptor binding or dopamine release. Drug Alcohol. Depend 205:107627. doi: 10.1016/j.drugalcdep.2019.107627
Wang, S., Kim, S., Cosgrove, K. P., and Morris, E. D. (2017). A framework for designing dynamic lp-ntPET studies to maximize the sensitivity to transient neurotransmitter responses to drugs: application to dopamine and smoking. Neuroimage 146, 701–714. doi: 10.1016/j.neuroimage.2016.10.019
Wey, H.-Y., Catana, C., Hooker, J. M., Dougherty, D. D., Knudsen, G. M., Wang, D. J. J., et al. (2014). Simultaneous fMRI-PET of the opioidergic pain system in human brain. Neuroimage 102(Pt 2), 275–282. doi: 10.1016/j.neuroimage.2014.07.058
Whitton, A. E., Reinen, J. M., Slifstein, M., Ang, Y.-S., McGrath, P. J., Iosifescu, D. V., et al. (2020). Baseline reward processing and ventrostriatal dopamine function are associated with pramipexole response in depression. Brain 143, 701–710. doi: 10.1093/brain/awaa002
Yoder, K. K., Wang, C., and Morris, E. D. (2004). Change in binding potential as a quantitative index of neurotransmitter release is highly sensitive to relative timing and kinetics of the tracer and the endogenous ligand. J. Nucl. Med. 45, 903–911.
Zakiniaeiz, Y., Hillmer, A. T., Matuskey, D., Nabulsi, N., Ropchan, J., Mazure, C. M., et al. (2019). Sex differences in amphetamine-induced dopamine release in the dorsolateral prefrontal cortex of tobacco smokers. Neuropsychopharmacology 44, 2205–2211. doi: 10.1038/s41386-019-0456-y
Keywords: brain imaging quantification, neurotransmitter release, kinetic modeling, dopamine, PET/fMRI
Citation: Ceccarini J, Liu H, Van Laere K, Morris ED and Sander CY (2020) Methods for Quantifying Neurotransmitter Dynamics in the Living Brain With PET Imaging. Front. Physiol. 11:792. doi: 10.3389/fphys.2020.00792
Received: 14 April 2020; Accepted: 15 June 2020;
Published: 21 July 2020.
Edited by:
Ivo Rausch, Medical University of Vienna, AustriaReviewed by:
Daniele De Paula Faria, University of São Paulo, BrazilAndreas Hahn, Medical University of Vienna, Austria
Copyright © 2020 Ceccarini, Liu, Van Laere, Morris and Sander. This is an open-access article distributed under the terms of the Creative Commons Attribution License (CC BY). The use, distribution or reproduction in other forums is permitted, provided the original author(s) and the copyright owner(s) are credited and that the original publication in this journal is cited, in accordance with accepted academic practice. No use, distribution or reproduction is permitted which does not comply with these terms.
*Correspondence: Jenny Ceccarini, amVubnkuY2VjY2FyaW5pQHV6bGV1dmVuLmJl