- 1Max Planck Institute for Dynamics and Self-Organization, Göttingen, Germany
- 2Institute for the Dynamics of Complex Systems, Georg-August-Universität Göttingen, Göttingen, Germany
- 3Department of Cognitive Neurology, University Medical Center Göttingen, Göttingen, Germany
- 4Department of Neurology, University Medical Center Göttingen, Göttingen, Germany
- 5Institute of Pharmacology and Toxicology, University Medical Center Göttingen, Göttingen, Germany
- 6German Center for Cardiovascular Research (DZHK), Partner Site Göttingen, Göttingen, Germany
- 7German Primate Center, Leibniz Institute for Primate Research, Göttingen, Germany
In this study, ordinal pattern analysis and classical frequency-based EEG analysis methods are used to differentiate between EEGs of different age groups as well as individuals. As characteristic features, functional connectivity as well as single-channel measures in both the time and frequency domain are considered. We compare the separation power of each feature set after nonlinear dimensionality reduction using t-distributed stochastic neighbor embedding and demonstrate that ordinal pattern-based measures yield results comparable to frequency-based measures applied to preprocessed data, and outperform them if applied to raw data. Our analysis yields no significant differences in performance between single-channel features and functional connectivity features regarding the question of age group separation.
1. Introduction
The study of physiological networks is of great interest in biomedical sciences. Especially functional brain networks, extracted from MRI- or EEG-recordings, are a frequent subject of studies. We obtain functional networks from EEG recordings and compare these networks to features of single EEG channels in their function as biomarkers.
Neurobiological changes in healthy and pathological aging and their electrophysiological correlates (EEG) are still a hot topic in the neuroscience community, particularly since the incidence and prevalence of mild cognitive impairment and dementia increase alongside life expectancy (Ricci, 2019). Although some consensus has been reached regarding some electrophysiological correlates of aging, such as the reduction of occipital alpha power (Babiloni et al., 2006) and a shifting of the individual alpha peak towards lower frequencies in elderly subjects (Scally et al., 2018), an electrophysiological marker with which we can confidently discriminate between young and elderly individuals is yet to be established.
Thus, the study of age group differences in EEG recordings has been of interest for several decades and has been addressed by many authors over the years. While some considered differences in single-channel (SC) measures (Waschke et al., 2017), others used functional connectivity (FC) (McIntosh et al., 2013) or a combination of multiple feature groups (Al Zoubi et al., 2018). All methods successfully extracted significant differences between two age groups or significant correlations between the measure of choice and age. This is why we wanted to directly compare the discriminating power of FC and SC features in this study.
In recent years, studies aiming not only at differentiating between age groups but also between individuals based on features extracted from EEG recordings have become available (Rocca et al., 2014; Demuru and Fraschini, 2020; Suetani and Kitajo, 2020; Wilaiprasitporn et al., 2020). In all mentioned works, the features of choice were based in the frequency domain.
A problem for commonly used features extracted from EEG signals is the observation that in most cases, extensive preprocessing of the data is required and done, which has recently been shown to possibly lead to different results (Robbins et al., 2020). Thus, a method of feature extraction where the amount of preprocessing can be reduced would be desirable. Therefore, we used ordinal pattern (OP) statistics (Bandt and Pompe, 2002) for characterizing our data which has been shown to be a robust method for analysing physiological time series (Keller et al., 2007a, 2014; Parlitz et al., 2012; Amigó et al., 2015; Unakafov, 2015). For example, OP analysis has been used to separate healthy subjects from patients suffering from congestive heart failure (Parlitz et al., 2012) or to differentiate between different experimental conditions in EEG recordings (Unakafov, 2015; Quintero-Quiroz et al., 2018).
The discriminating power of OP distributions of single channels is compared to FC measures given by the mutual information (MI) based on OP distributions. These time domain features are compared to spectral features given by power spectral densities (PSDs) of single channels and coherence characterizing interrelations of pairs of channels. As a benchmark task we aim at separating individuals and age groups based on different sets of features extracted from EEG recordings. To illustrate and quantify the separation the high dimensional features (feature vectors) are mapped to a two-dimensional plane using the nonlinear dimensionality reduction algorithm t-SNE (van der Maaten and Hinton, 2008).
2. Materials
2.1. Data Set
The data set analyzed in this study was on a set of recordings from 45 participants, divided into two different age groups, who participated in an image recognition task. We will refer to this data set as the Image Recognition data set.
Twenty-two young (12 female, mean age: 24.8 years ± 3.9 SD) and 23 elderly (11 female, mean age: 62.4 years ± 7.2 SD) healthy subjects were included. All subjects had normal or corrected-to-normal vision, normal contrast sensitivity and no color vision weakness/blindness. None of the participants had a history of neurological or psychiatric diseases. Normal visual acuity, contrast sensitivity and color vision were corroborated with the Snellen chart (Snellen, 1862), the Mars Letter Contrast Sensitivity test (Arditi, 2005), and a version of the Stilling, Hertel, and Velhagen color panels test (Broschmann and Kuchenbecker, 2011), respectively. To select the hand with which participants would answer the tests, handedness was assessed using the Edinburgh Handedness Inventory (Oldfield, 1971). Additionally, subjects were screened for cognitive impairment and depression using the Mini-Mental State Examination (MMSE) (Folstein et al., 1975) and the Beck Depression Inventory II (BDI-II) (Beck et al., 1996). A score of ≤ 24 points in the MMSE and/or a score of ≥9 points in the BDI-II were considered exclusion criteria.
The subjects participated in a modified version of the image recognition task described in Miloserdov et al. (2020). Subjects were shown images from three different categories: cars, faces and scrambled images. The images were shown on two different contrast levels, high (100% contrast) and low (10% contrast), giving six different conditions in total. The subjects were asked to categorize each image they were shown. Each condition was repeated a total of 80 times, resulting in a total of 480 trials.
2.2. Measurement and Preprocessing of EEG Data
The EEG data was recorded at a sampling rate of 1,000 Hz using a 64-channel Brain Products system elastic cap. The cap includes a reference electrode located at FCz. The FieldTrip toolbox for Matlab (Oostenveld et al., 2010) was used for data preprocessing. Continuous EEG data was segmented into 1,500 ms long epochs (either 1,500 ms prestimulus or 1,500 ms poststimulus). What we will refer to as “raw” data was analyzed without going through any additional preprocessing steps.
What we will further refer to as the “preprocessed” data went through the following additional steps. An offline 0.1 Hz–220 Hz band-pass filter (butterworth, hamming window) and a 50 Hz notch filter were applied. Jumps and clips were automatically detected using a amplitude z-value cutoff of 20 in the case of jumps and a time threshold of 0.02 s for clips. The data points identified as clips or jumps were then linearly interpolated. Muscle artifacts were detected automatically by first applying a band-pass filter of 120 Hz–140 Hz and selecting an amplitude z-value threshold of 5. The trials marked as having muscle artifacts were afterwards visually inspected and rejected. Blink artifacts were corrected for using Independent Component Analysis (ICA). Data was re-referenced to the common average, i.e., the average across all EEG channels is subtracted from the EEG signal of each individual channel.
3. Methods
The aim of this study was to compare the results from classical frequency-based neuroscientific features as coherence and power spectra to features extracted on the basis of symbolic dynamics and information theoretical measures. From each domain, we took one single-channel measure and one functional connectivity measure that takes into account the relationships between different areas in the brain.
3.1. Functional Connectivity in EEG Recordings
Functional connectivity (FC) quantifies the temporal statistical dependence between signals in different brain regions (Sakkalis, 2011) and there exists an abundance of different measures that are used in the neuroscientific community. Generally, FC can be measured in time domain or frequency domain. In both domains, linear and non-linear measures exist. In this study, the non-linear time domain based measure mutual information (MI, Cover and Thomas, 1991) is compared to one of the most popular measures in EEG analysis, the frequency domain-based linear coherence (Bastos and Schoffelen, 2016). These two measures will be introduced in the following.
3.1.1. Ordinal Pattern Statistics and Mutual Information
Ordinal patterns (OPs) are a symbolic approach to time series analysis that was originally introduced by Bandt and Pompe (2002). Since then, OP based methods have successfully been used in the analyses of biomedical data (Keller et al., 2007b; Amigó et al., 2010, 2015; Parlitz et al., 2012; Graff et al., 2013; Kulp et al., 2016; McCullough et al., 2017) and specifically EEG recordings (Keller et al., 2007a, 2014; Ouyang et al., 2010; Schinkel et al., 2012, 2017; O'Hora et al., 2013; Rummel et al., 2013; Shalbaf et al., 2015; Unakafov, 2015; Cui et al., 2016; Quintero-Quiroz et al., 2018). Statistics based on ordinal pattern have been shown to be robust to noise (Parlitz et al., 2012; Quintero-Quiroz et al., 2015) and can be used to define advanced concepts for quantifying information flow (Staniek and Lehnertz, 2008; Amigó et al., 2016) or to derive transition networks in state space from observed time series (McCullough et al., 2015; Zhang et al., 2017).
In ordinal pattern statistics, the order relations between values of a time series are considered rather than the values themselves. An ordinal pattern for a given length w and lag l describes the order relations between w points of a time series, each separated by l − 1 points. For a length w, there are w! possible different patterns, that can each be assigned a unique permutation index as illustrated for w = 4 in Figure 1. The permutation index characterizes the permutation π that is needed to get from a sample xt, xt+l, …, x(w−1)(t+l) of the time series to a sample xπ(t), xπ(t+l), …, xπ((w−1)(t+l)) that is ordered ascendingly according to the amplitude of the sample in the time series.
An important parameter is the lag l which can be used to address different time scales as illustrated in Figure 2.
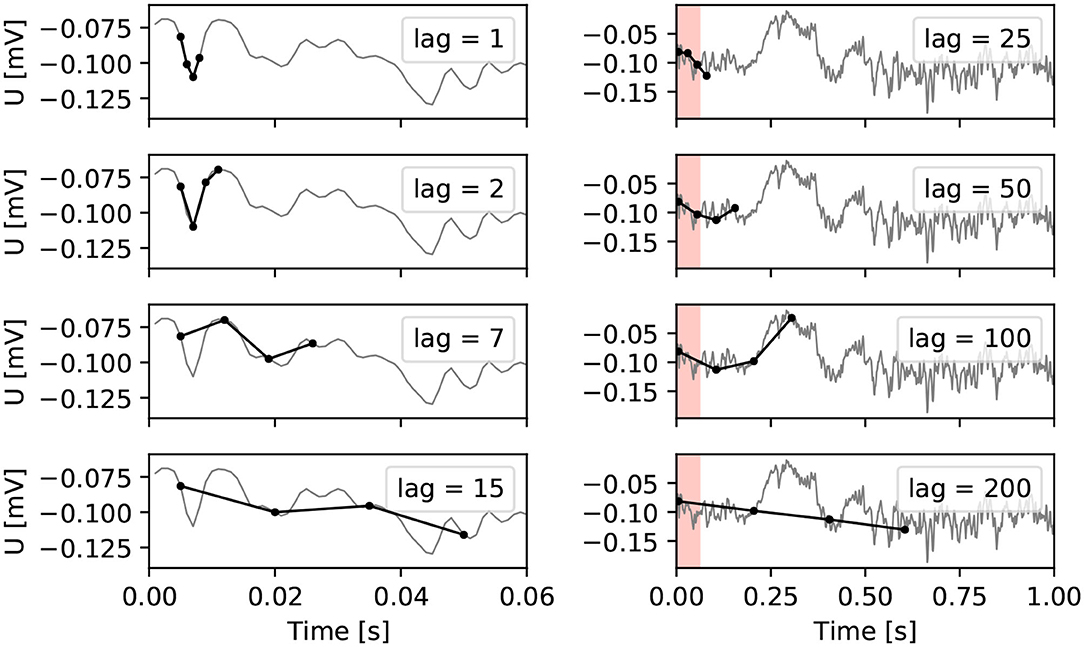
Figure 2. Illustration of ordinal patterns on different time scales in raw EEG data with sampling rate 1,000 Hz. The colored interval in the right column covers the same time span (0.06 s) as the entire window in the left column.
Ordinal patterns are easy and inexpensive to compute and have been shown to be robust to noise (Bandt and Pompe, 2002; Parlitz et al., 2012). From a sequence of ordinal patterns, the probabilities of occurrence of specific patterns, given a lag l and length w, can be used to characterize the underlying time series. Commonly, complexity measures as permutation entropy (Bandt and Pompe, 2002; Parlitz et al., 2012) or conditional entropy (Unakafov, 2015) are applied to the resulting pattern distributions.
Here, the question asked is not about the complexity of a univariate time series, but about the similarity of channels in one multivariate EEG recording. The similarity measure that is used here is the mutual information (MI) (Shannon, 1948; Cover and Thomas, 1991). Mutual information can be expressed by the Kullback-Leibler divergence (Kullback and Leibler, 1951) between the joint probability distribution pX, Y of two jointly varying random variables X and Y and the product of their marginal distributions:
For independent variables, the joint distribution is equal to the product of the marginal ones, resulting in a mutual information of I(X; Y) = 0. Accordingly, mutual information can be interpreted as a quantity that measures to what degree two random variables are not independent.
3.1.2. Coherence
In contrast to OP statistics and MI, coherency (Bastos and Schoffelen, 2016) measures functional connectivity in the frequency domain. The coherency of two time series yi and yj, for example two EEG channels i and j, is defined as the normed expectation value of the cross-spectrum
where ŷi(f) is the Fourier transform of the signal yi(t) and * denotes the complex conjugate.
The expectation value 〈·〉 is usually approximated by taking the average over multiple trials from an EEG sample. In this study, we will consider the absolute value of Equation (2), and call it coherence.
3.2. Single-Channel Features in EEG Recordings
We compare the introduced FC measures to measures only taking into account single channels, but no relations between them. For this, we consider the PSDs as done by Suetani and Kitajo (2020), as well as OP distributions for each channel. In both cases, (not necessarily normalized) distributions per channel are considered. As a metric to compare them, we use the generalized KL-divergence. A vectorized, symmetric version of this was introduced by Suetani and Kitajo (2020) as a metric, which is given by
where the generalized KL-divergence DB(P||Q) between two not necessarily normalized densities P and Q is given according to
dnm gives the distance between two vectors of dimension Nch, where each dimension contains a distribution , which will either be a PSD or a OP distribution. It is a special case of the beta-divergence (Basu et al., 1998; Mihoko and Eguchi, 2002) used in Suetani and Kitajo (2020) with β = 1.
3.3. Dimensionality Reduction
Dimensionality reduction aims to visualize such high-dimensional data in a low-dimensional space, preferably by extracting the most important features and representing each data point only by those. While the idea of dimensionality reduction dates back more than 100 years (Pearson, 1901), recently, more and more techniques have surfaced (van der Maaten et al., 2007).
In this study, the non-linear algorithm t-distributed stochastic neighbor embedding (t-SNE, van der Maaten and Hinton, 2008) is used to project features extracted from EEG time series. These features are adjacency matrices in case of functional connectivity and vectors of distributions in case of single-channel measures.
3.3.1. Nonlinear Dimension Reduction (t-SNE)
T-distributed stochastic neighbor embedding was first introduced by van der Maaten and Hinton (2008) as an extension of stochastic neighbor embedding (SNE) (Hinton and Roweis, 2003) to avoid the crowding problem and simplify optimization.
The algorithm projects a set of L samples x1, x2, …, xL from a high-dimensional into a low-dimensional space so that , considering the so-called neighbor probabilities
for high-dimensional data points xn and xm. Here, ||·|| is typically the Euclidean norm. For projections of single-channel features, where one high-dimensional data point consists of Nch distributions, we use ||·|| = dnm in Equation (3). In case of connectivity matrices, we flatten1 the upper triangular part of the matrix and use the Euclidean norm as a measure of distance. N is an arbitrary integer value and M ∈ {2, 3} in general, we use M = 2 in this study. The probability pm|n describes the probability that xm is a neighbor of xn and is proportional to a Gaussian centered at xn. The standard deviation σn of the high-dimensional probability distributions is calculated so that it satisfies a given value of the perplexity k. More specifically, the entropy of the conditional probability pm|n as a function of m must be approximately equal to log2(k), or
where H is the Shannon entropy (Shannon, 1948). The goal of t-SNE is now to minimize the sum of the Kullback-Leibler divergences between the symmetric probabilities pnm = (pm|n+pm|n)/2 in the high-dimensional space and the neighbor probabilities qnm of the projections into low-dimensional space,
where qnm is proportional to a Student-t-distribution (Student, 1908) with mean yn. The cost-function then becomes
This cost-function is minimized using the gradient descent method, thus aligning the high- and low-dimensional neighbor probabilities. As a consequence, data points that are close in high-dimensional space will be projected closely together.
Because t-SNE is a stochastic method that starts out the projection with randomly assigning low-dimensional coordinates to data points and then minimizing the cost-function given in Equation (8), the resulting projection depends on the initial conditions of the projection. If one only considers a single projection, it is possible that this projection is not necessarily representative for all projections. Thus, if one wants to quantify effects in the t-SNE projections, one must average over many projections with different initial conditions.
3.4. Analysis Scheme
The EEG recordings were cut into trials. These trials were 1.5 s long, either directly before or directly after the subjects were shown a picture. These steps were done both for the raw and preprocessed versions of the data set.
OP sequences with patterns of length w = 4 were calculated for each trial. From all trials belonging to the same condition, histograms of the probability of occurrence were extracted, giving one histogram per EEG-channel, per condition and per subject. On the basis of the OP sequences, one single-channel feature and one functional connectivity feature were extracted:
• The marginal ordinal pattern distributions were used as single-channel features for dimensionality reduction with t-SNE. Here, we calculated the distance between two samples, each characterized by Nch OP distributions, with Equation (3).
• We also used (joint) OP distributions to calculate MI-values between each pair of EEG channels, resulting in an Nch × Nch symmetric adjacency matrix per stimulus type per subject, containing the MI before or after each stimulus.
Additional to the time-domain measures, features were extracted from the frequency domain. For each trial, power spectra were calculated by performing a fast Fourier transform (FFT). Based on this, we again extracted one single-channel feature and one functional connectivity feature:
• The power spectral densities of each electrode were calculated as the squared absolute value of the Fourier transform of the signal and averaged over all trials. Again, we calculated the distance between two samples, each characterized by Nch PSDs (either full PSD or only the bins of specific frequency bands), with Equation (2) where only the frequency bins of specific frequency bands were contained (alpha: 8 Hz–12 Hz, beta: 15 Hz–30 Hz, theta: 3 Hz–7 Hz, gamma: 30 Hz–50 Hz). We consider different frequency bands for comparability with findings in literature.
• Adjacency matrices based on the coherence between EEG channels were composed by calculating the average coherence over all trials per frequency-band.
The above described dimensionality reduction algorithms were applied to each feature set. In case of the adjacency matrices, the flattened upper triangular part of the matrices was used as input for the algorithms with the Euclidean distance as a metric. We also projected the vectors containing PSDs and OP distributions with the vectorized KL-divergence as distance measure as introduced in Equation (3).
It is important to mention that the different feature sets (FC and SC) involved in the comparison have different numbers of features which itself might influence dimensionality-reduction methods.
To quantify the effect of subject separation in the 2d-projections the ratio ρ between the average distances within a subject-cluster to the average distances to its three next neighbors was calculated. This ratio gives insight on how much closer data points of the same subject are projected together than data points of different individuals.
As a quantification of the separation of age groups we used a kernel density estimation (KDE; Silverman, 1986) with a Gaussian kernel and a bandwidth selection according to Scott (2015) for the distributions of the two age groups in the projections. An illustration of such estimated densities is given in Figure 4.
We then calculated the Jensen-Shannon divergence (Lin, 1991) between the two distributions. The Jensen-Shannon divergence quantifies differences between probability distributions. It is bound by unity in case of a base-2 logarithm and can be derived from the KL divergence via
The square root of the Jensen-Shannon divergence is a metric that is often called the Jensen-Shannon distance (JSD, Endres and Schindelin, 2003). This was done, in all cases, for 100 t-SNE projections of the ensemble with different random seeds.
4. Results
T-SNE projections of similar experimental conditions from the Image Recognition data set, i.e., samples after a high-contrast stimulus, are displayed in Figure 3. Four different feature types were projected. The results for the two FC features MI and Coherence (alpha band) are displayed in the left column, and the projections of the single-channel features, OP distributions and filtered PSDs (theta band), are depicted in the right column. In all cases, separations of age groups and individuals can be observed visually. We observe that the three conditions (face, car, scrambled image) are projected together closely for each individual. This effect is similar to the one observed in Suetani and Kitajo (2020) and is further quantified in Figure A1 in the Appendix. The two age groups (Elderly in red and Young in blue) appear to be loosely separated.
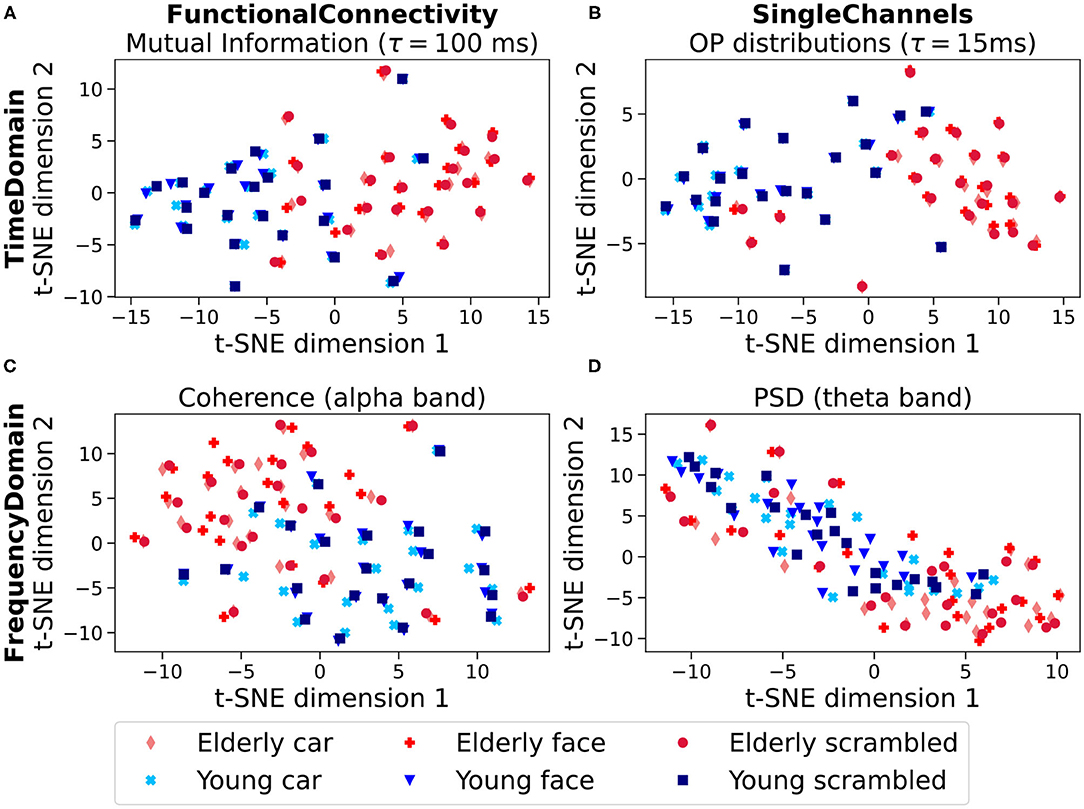
Figure 3. t-SNE projections of features obtained from the EEG time series of the Image Recognition data set (k = 30). We compare time-domain measures (A,B) to frequency domain measures (C,D). The time-domain measures are extracted from raw data by calculating OP sequences, the frequency-domain measures are taken from frequency bands of the preprocessed data. In each case, a projection for the parameter (lag/frequency band) yielding the best group separation for the method is shown. (A) Projection of functional connectivity vectors obtained from OP statistics (τ = 100 ms), (B) Projection of OP distribution-vectors (τ = 15 ms) with the generalized KL-divergence as a metric, (C) Projection of functional connectivity vectors obtained from average coherence (alpha-band), (D) projection of power spectral density-vectors (theta-band) with the generalized KL-divergence as a metric.
As will be detailed in the following section we find that the separation of the two age groups, based on the JSD, are comparable for all feature sets, with a tendency toward higher separations for the OP based methods. The separation of individuals is clearly more distinct for OP based measures than for frequency based measures, both for FC and single channels.
4.1. Age Group Separation
In Figure 3, a separation of the two age groups in the t-SNE projections can be observed visually. As an example, in Figure 4, the estimated densities of the age groups are plotted for the same parameters as in Figure 3A. One can observe that the densities barely overlap.
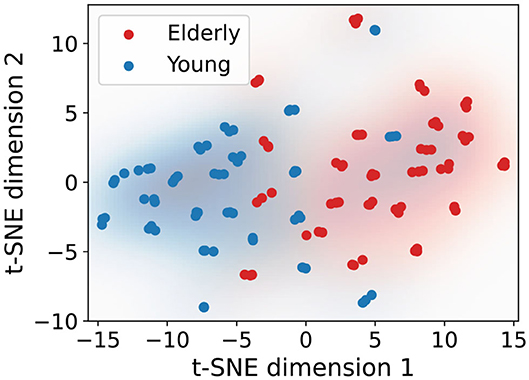
Figure 4. Exemplary plot of a the estimated kernel densities for the same parameters as in Figure 3A; a distinction is made only between age groups, not conditions.
The Jensen-Shannon distances between the two estimated distributions, depending on time or frequency scales, are displayed in Figure 5 for both raw and preprocessed data. For all feature sets, an average JSD larger than zero can be observed, with the highest values being achieved by the OP based measures.
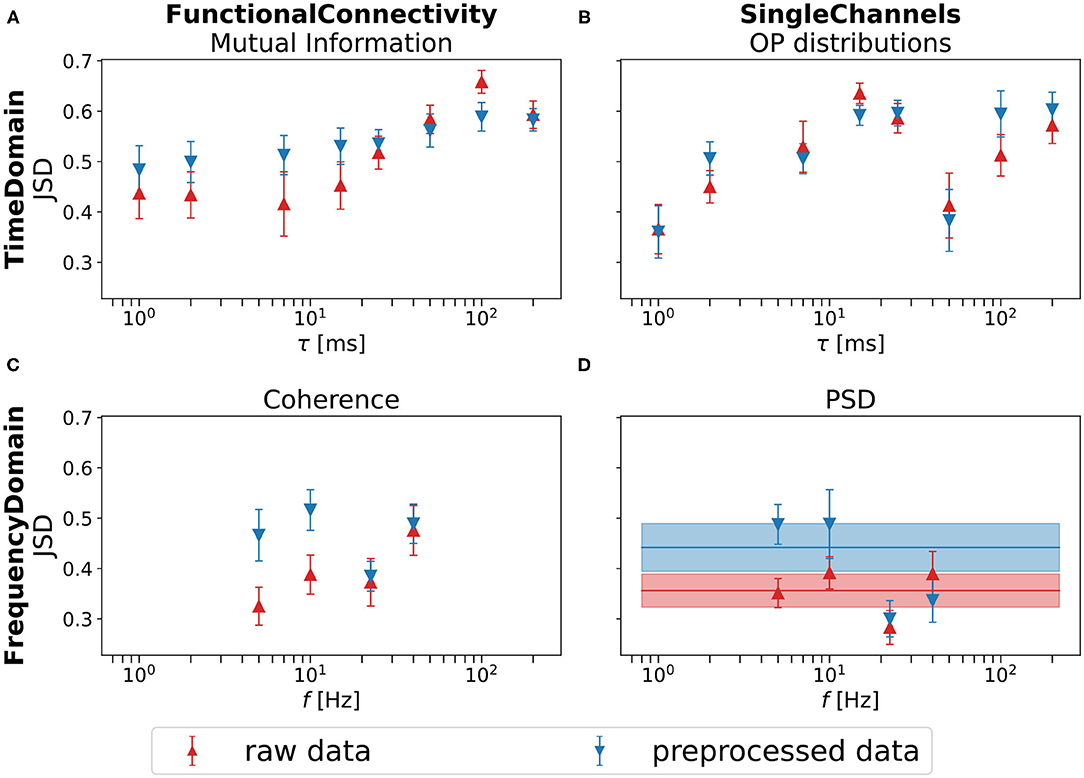
Figure 5. Quantification of the separation of Elderly and Young in 2d t-SNE projections (k = 30) for both raw (red) and preprocessed (blue) EEG data. We consider group separation based on (A,C) functional connectivity and (B,D) single channels. Results from OP based measures are displayed in the upper row, and from frequency-based measures in the lower row. The solid lines describe the separation when using the full PSD, otherwise only the frequency bins of one specific band are considered. The error bars display the standard deviation over 100 t-SNE projections with different random seeds.
While for the OP based measures, no increase of the best performance across time scales through artifact removal can be observed, artifact correction clearly leads to an overall increase of performance for frequency based measures. This is yet another illustration of the robustness of OP statistics.
We found no significant dependency of the separation of age groups on the perplexity k for the t-SNE projections. In case of individuals, we found the same dependency as Suetani and Kitajo (2020), where for larger values of k (up to k = 100), the separation is less distinct than for smaller values, but still observable.
5. Discussion
In this study, we obtained differences between age groups (Elderly, Young) and individuals subjected to similar experimental conditions based on both functional connectivity and single-channel measures obtained from multichannel EEG time series. We found that t-SNE as a method for dimensionality reduction and feature extraction does not only reflect individuality but also appears to represent inter-individual relationships, given by age groups in this case.
It should be emphasized that the separation of individuals is restricted to the separation of recordings from the same individual under similar conditions (post high contrast stimulus). Separate checks using pre-stimulus recordings and resting state recordings from the same session, revealed that the data points of the same individual are not necessarily projected closely together.
Regarding the separation between age groups, one could consider that a difference between brain age, which is a descriptor of the physiological condition of the brain, and the chronological age of the subject has been hypothesized (Irimia et al., 2015; Steffener et al., 2016). If the chronological age and the brain age of some individuals in the study differ, this could explain apparent outliers in the projections.
Since the aim of t-SNE is to find a low-dimensional projection of a high-dimensional data set that represents the distances between high-dimensional points, it can be assumed that the projected ensemble does not only represent the two features with highest variance and omits the others, but is rather a representation of the whole feature set.
We showed that OP analysis can obtain results comparable to classical EEG feature sets, and even outperform them if both methods are applied to raw data. For the OP based measures, the applied preprocessing pipeline partially even leads to a decrease in performance regarding the separation of age groups, while for the frequency-based measures, there is always an increase observable. This supports previous observations that OP based methods yield promising results if applied to raw data sets. Preprocessing could thus be reduced to avoid potential differences of analyses in different labs.
The question answered here is “Is the average or best performance of one feature set comparable to the average or best performance of another feature set?” This appears to be the case for the age group separation, and also for the separation of subjects.
Given the observation that there appears to be no significant difference between FC and SC measures, the question arises whether the obtained functional networks actually contain the information that we assume they do. In light of these findings, the interpretation of other studies that obtained age group differences based on functional connectivity (Wada et al., 1998; McIntosh et al., 2013; Al Zoubi et al., 2018, amongst others) could be reconsidered.
If the age differences are also contained in single-channel measures, and the separation power of functional connectivity does not outperform the single channel measures, it must be thoroughly investigated how these group differences are related to one another.
A possible explanation for the lack of differences between functional connectivity and single-channel measures would be that the main information that is contained in the functional connectivity measures that are considered here is due to shared sources between the different EEG channels. This information would also be contained in single-channel features. To verify this, further tests must be done.
Furthermore, future studies should include a comparison with other measures of network physiology like time delay stability (Bartsch et al., 2015; Liu et al., 2015; Lin et al., 2016) and transfer entropy (Schreiber, 2000; Staniek and Lehnertz, 2008; Vicente et al., 2011; Wibral et al., 2013) measures.
Data Availability Statement
The datasets presented in this article are not readily available due to data protection rules. Requests to access the datasets should be directed to Melanie Wilke (melanie.wilke@med.uni-goettingen.de).
Ethics Statement
The studies involving human participants were reviewed and approved by medical ethics committees of the University Medical Center Göttingen, Germany. The patients/participants provided their written informed consent to participate in this study.
Author Contributions
IK, AS, and UP prepared the design of the manuscript. MW and DT-T designed the behavioral tasks. DT-T performed the experiments and was responsible for data acquisition. DT-T and IS performed data preprocessing. AS, IK, SB, UP, and IS developed the conceptional design of the data analysis. IK and SB implemented the analysis algorithms and performed the data analysis. MB, SL, and MW were in charge of project administration and funding acquisition. All authors edited and reviewed the article and approved the manuscript.
Funding
We acknowledge EKFS Seed Funding by the Else Kröner-Fresenius Foundation and support through the German Center for Cardiovascular Research (DZHK), partner site Göttingen. MW, IS, and DT-T were funded by the Herman and Lilly Schilling Foundation.
Conflict of Interest
The authors declare that the research was conducted in the absence of any commercial or financial relationships that could be construed as a potential conflict of interest.
Acknowledgments
IK would like to thank Steffen Korn for helpful discussions regarding machine learning techniques. All authors would like to thank Aishwarya Bhonsle for interesting discussions. We would like to thank Kristina Miloserdov for the task design and support with data collection and Carsten Schmidt-Samoa for his support in task programming and technical assistance.
Footnotes
1. ^We “flatten” a matrix by concatenating all column vectors into one long vector.
References
Al Zoubi, O., Ki Wong, C., Kuplicki, R. T., Yeh, H.-w., Mayeli, A., Refai, H., et al. (2018). Predicting age from brain EEG signals-a machine learning approach. Front. Aging Neurosci. 10:184. doi: 10.3389/fnagi.2018.00184
Amigó, J. M., Keller, K., and Unakafova, V. A. (2010). Permutation Complexity in Dynamical Systems: Ordinal Patterns, Permutation Entropy and All That. Heidelberg: Springer Science & Business Media. doi: 10.1007/978-3-642-04084-9_3
Amigó, J. M., Keller, K., and Unakafova, V. A. (2015). Ordinal symbolic analysis and its application to biomedical recordings. Philos. Trans. Ser. A Math. Phys. Eng. Sci. 373:20140091. doi: 10.1098/rsta.2014.0091
Amigó, J. M., Monetti, R., Graff, B., and Graff, G. (2016). Computing algebraic transfer entropy and coupling directions via transcripts. Chaos 26:113115. doi: 10.1063/1.4967803
Arditi, A. (2005). Improving the design of the letter contrast sensitivity test. Invest. Ophthalmol. Vis. Sci. 46, 2225–2229. doi: 10.1167/iovs.04-1198
Babiloni, C., Binetti, G., Cassarino, A., Dal Forno, G., Del Percio, C., Ferreri, F., et al. (2006). Sources of cortical rhythms in adults during physiological aging: a multicentric EEG study. Hum. Brain Mapp. 27, 162–172. doi: 10.1002/hbm.20175
Bandt, C., and Pompe, B. (2002). Permutation entropy: a natural complexity measure for time series. Phys. Rev. Lett. 88:174102. doi: 10.1103/PhysRevLett.88.174102
Bartsch, R. P., Liu, K. K. L., Bashan, A., and Ivanov, P. C. (2015). Network physiology: how organ systems dynamically interact. PLoS ONE 10:e142143. doi: 10.1371/journal.pone.0142143
Bastos, A. M., and Schoffelen, J. (2016). A tutorial review of functional connectivity analysis methods and their interpretational pitfalls. Front. Syst. Neurosci. 9:175. doi: 10.3389/fnsys.2015.00175
Basu, A., Harris, I. R., Hjort, N. L., and Jones, M. C. (1998). Robust and efficient estimation by minimising a density power divergence. Biometrika 85, 549–559. doi: 10.1093/biomet/85.3.549
Beck, A., Steer, R., Ball, R., and Ranieri, W. (1996). Comparison of beck depression inventories -IA and -II in psychiatric outpatients. J. Pers. Assess. 67, 588–597. doi: 10.1207/s15327752jpa6703_13
Broschmann, D., and Kuchenbecker, J. (2011). Tafeln zur Prüfung des Farbensinnes. Stuttgart: Thieme.
Cover, T. M., and Thomas, J. A. (1991). Elements of Information Theory. New York, NY: Wiley-Interscience. doi: 10.1002/0471200611
Cui, D., Wang, J., Wang, L., Yin, S., Bian, Z., and Gu, G. (2016). Symbol Recurrence Plots based resting-state eyes-closed EEG deterministic analysis on amnestic mild cognitive impairment in type 2 diabetes mellitus. Neurocomputing 203, 102–110. doi: 10.1016/j.neucom.2016.03.056
Demuru, M., and Fraschini, M. (2020). EEG fingerprinting: subject-specific signature based on the aperiodic component of power spectrum. Comput. Biol. Med. 120:103748. doi: 10.1016/j.compbiomed.2020.103748
Endres, D. M., and Schindelin, J. E. (2003). A new metric for probability distributions. IEEE Trans. Inform. Theory 49, 1858–1860. doi: 10.1109/TIT.2003.813506
Folstein, M. F. (1975). “Mini-mental state”: a practical method for grading the cognitive state of patients for the clinician. J. Psychiatr. Res. 12, 189–198. doi: 10.1016/0022-3956(75)90026-6
Graff, G., Graff, B., Kaczkowska, A., Makowiec, D., Amigó, J., Piskorski, J., et al. (2013). Ordinal pattern statistics for the assessment of heart rate variability. Eur. Phys. J. Spec. Top. 222. doi: 10.1140/epjst/e2013-01857-4
Hinton, G. E., and Roweis, S. T. (2003). “Stochastic neighbor embedding,” in Advances in Neural Information Processing Systems 15, eds S. Becker, S. Thrun, and K. Obermayer (Cambridge, MA: MIT Press), 857–864.
Irimia, A., Torgerson, C. M., Matthew Goh, S. Y., and Van Horn, J. D. (2015). Statistical estimation of physiological brain age as a descriptor of senescence rate during adulthood. Brain Imag. Behav. 9, 678–689. doi: 10.1007/s11682-014-9321-0
Keller, K., Lauffer, H., and Sinn, M. (2007a). Ordinal analysis of EEG time series. Chaos Complex. Lett. 2, 247–258.
Keller, K., Sinn, M., and Emonds, J. (2007b). Time series from the ordinal viewpoint. Stochast. Dyn. 07, 247–258. doi: 10.1142/S0219493707002025
Keller, K., Unakafov, A., and Unakafova, V. (2014). Ordinal patterns, entropy, and EEG. Entropy 16, 6212–6239. doi: 10.3390/e16126212
Kullback, S., and Leibler, R. A. (1951). On information and sufficiency. Ann. Math. Stat. 22, 79–86. doi: 10.1214/aoms/1177729694
Kulp, C. W., Chobot, J. M., Freitas, H. R., and Sprechini, G. D. (2016). Using ordinal partition transition networks to analyze ECG data. Chaos 26:073114. doi: 10.1063/1.4959537
Lin, A., Liu, K. K. L., Bartsch, R. P., and Ivanov, P. C. (2016). Delay-correlation landscape reveals characteristic time delays of brain rhythms and heart interactions. Philos. Trans. R. Soc. A Math. Phys. Eng. Sci. 374:20150182. doi: 10.1098/rsta.2015.0182
Lin, J. (1991). Divergence measures based on the Shannon entropy. IEEE Trans. Inform. Theory 37, 145–151. doi: 10.1109/18.61115
Liu, K. K. L., Bartsch, R. P., Lin, A., Mantegna, R. N., and Ivanov, P. C. (2015). Plasticity of brain wave network interactions and evolution across physiologic states. Front. Neural Circuits 9:62. doi: 10.3389/fncir.2015.00062
McCullough, M., Small, M., Iu, H. H. C., and Stemler, T. (2017). Multiscale ordinal network analysis of human cardiac dynamics. Philos. Trans. R. Soc. A Math. Phys. Eng. Sci. 375:20160292. doi: 10.1098/rsta.2016.0292
McCullough, M., Small, M., Stemler, T., and Iu, H. H.-C. (2015). Time lagged ordinal partition networks for capturing dynamics of continuous dynamical systems. Chaos 25:053101. doi: 10.1063/1.4919075
McIntosh, A. R., Vakorin, V., Kovacevic, N., Wang, H., Diaconescu, A., and Protzner, A. B. (2013). Spatiotemporal dependency of age-related changes in brain signal variability. Cereb. Cortex 24, 1806–1817. doi: 10.1093/cercor/bht030
Mihoko, M., and Eguchi, S. (2002). Robust blind source separation by beta divergence. Neural Comput. 14, 1859–1886. doi: 10.1162/089976602760128045
Miloserdov, K., Schmidt-Samoa, C., Williams, K., Weinrich, C. A., Kagan, I., Bürk, K., et al. (2020). Aberrant functional connectivity of resting state networks related to misperceptions and intra-individual variability in parkinson's disease. NeuroImage 25:102076. doi: 10.1016/j.nicl.2019.102076
O'Hora, D., Schinkel, S., Hogan, M. J., Kilmartin, L., Keane, M., Lai, R., et al. (2013). Age-related task sensitivity of frontal EEG entropy during encoding predicts retrieval. Brain Topogr. 26, 547–557. doi: 10.1007/s10548-013-0278-x
Oldfield, R. (1971). The assessment and analysis of handedness: the Edinburgh inventory. Neuropsychologia 9, 97–113. doi: 10.1016/0028-3932(71)90067-4
Oostenveld, R., Fries, P., Maris, E., and Schoffelen, J.-M. (2010). Fieldtrip: open source software for advanced analysis of MEG, EEG, and invasive electrophysiological data. Comput. Intell. Neurosci. 2011:156869. doi: 10.1155/2011/156869
Ouyang, G., Dang, C., Richards, D. A., and Li, X. (2010). Ordinal pattern based similarity analysis for EEG recordings. Clin. Neurophysiol. 121, 694–703. doi: 10.1016/j.clinph.2009.12.030
Parlitz, U., Berg, S., Luther, S., Schirdewan, A., Kurths, J., and Wessel, N. (2012). Classifying cardiac biosignals using ordinal pattern statistics and symbolic dynamics. Comput. Biol. Med. 42, 319–327. doi: 10.1016/j.compbiomed.2011.03.017
Pearson, K. F. R. S. (1901). LIII. on lines and planes of closest fit to systems of points in space. Lond. Edinburgh Dublin Philos. Mag. J. Sci. 2, 559–572. doi: 10.1080/14786440109462720
Quintero-Quiroz, C., Montesano, L., Pons, A. J., Torrent, M. C., García-Ojalvo, J., and Masoller, C. (2018). Differentiating resting brain states using ordinal symbolic analysis. Chaos 28:106307. doi: 10.1063/1.5036959
Quintero-Quiroz, C., Pigolotti, S., Torrent, M. C., and Masoller, C. (2015). Numerical and experimental study of the effects of noise on the permutation entropy. New J. Phys. 17:093002. doi: 10.1088/1367-2630/17/9/093002
Ricci, G. (2019). Social aspects of dementia prevention from a worldwide to national perspective: a review on the international situation and the example of Italy. Behav. Neurol. 2019:8720904. doi: 10.1155/2019/8720904
Robbins, K. A., Touryan, J., Mullen, T., Kothe, C., and Bigdely-Shamlo, N. (2020). How sensitive are EEG results to preprocessing methods: a benchmarking study. IEEE Trans. Neural Syst. Rehabil. Eng. 28, 1081–1090. doi: 10.1109/TNSRE.2020.2980223
Rocca, D. L., Campisi, P., Vegso, B., Cserti, P., Kozmann, G., Babiloni, F., et al. (2014). Human brain distinctiveness based on EEG spectral coherence connectivity. IEEE Trans. Biomed. Eng. 61, 2406–2412. doi: 10.1109/TBME.2014.2317881
Rummel, C., Abela, E., Hauf, M., Wiest, R., and Schindler, K. (2013). Ordinal patterns in epileptic brains: analysis of intracranial EEG and simultaneous EEG-fMRI. Eur. Phys. J. Spec. Top. 222, 569–585. doi: 10.1140/epjst/e2013-01860-9
Sakkalis, V. (2011). Review of advanced techniques for the estimation of brain connectivity measured with EEG/MEG. Comput. Biol. Med. 41, 1110–1117. doi: 10.1016/j.compbiomed.2011.06.020
Scally, B., Burke, M. R., Bunce, D., and Delvenne, J.-F. (2018). Resting-state EEG power and connectivity are associated with alpha peak frequency slowing in healthy aging. Neurobiol. Aging 71, 149–155. doi: 10.1016/j.neurobiolaging.2018.07.004
Schinkel, S., Marwan, N., and Kurths, J. (2017). Order patterns recurrence plots in the analysis of ERP data. Cogn. Neurodyn. 1, 317–325. doi: 10.1007/s11571-007-9023-z
Schinkel, S., Zamora-López, G., Dimigen, O., Sommer, W., and Kurths, J. (2012). Order Patterns Networks (ORPAN)-a method to estimate time-evolving functional connectivity from multivariate time series. Front. Comput. Neurosci. 6:91. doi: 10.3389/fncom.2012.00091
Schreiber, T. (2000). Measuring information transfer. Phys. Rev. Lett. 85, 461–464. doi: 10.1103/PhysRevLett.85.461
Scott, D. W. (2015). Multivariate Density Estimation: Theory, Practice, and Visualization. Hoboken, NJ: John Wiley & Sons. doi: 10.1002/9781118575574
Shalbaf, R., Behnam, H., Sleigh, J. W., Steyn-Ross, D. A., and Steyn-Ross, M. L. (2015). Frontal-temporal synchronization of EEG signals quantified by order patterns cross recurrence analysis during propofol anesthesia. IEEE Trans. Neural Syst. Rehabil. Eng. 23, 468–474. doi: 10.1109/TNSRE.2014.2350537
Shannon, C. E. (1948). A mathematical theory of communication. Bell Syst. Techn. J. 27, 379–423. doi: 10.1002/j.1538-7305.1948.tb01338.x
Silverman, B. W. (1986). Density Estimation for Statistics and Data Analysis. Boca Raton, FL; London: Chapman & Hall, London. doi: 10.1007/978-1-4899-3324-9
Staniek, M., and Lehnertz, K. (2008). Symbolic transfer entropy. Phys. Rev. Lett. 100:158101. doi: 10.1103/PhysRevLett.100.158101
Steffener, J., Habeck, C., O'Shea, D., Razlighi, Q., Bherer, L., and Stern, Y. (2016). Differences between chronological and brain age are related to education and self-reported physical activity. Neurobiol. Aging 40, 138–144. doi: 10.1016/j.neurobiolaging.2016.01.014
Suetani, H., and Kitajo, K. (2020). A manifold learning approach to mapping individuality of human brain oscillations through beta-divergence. Neurosci. Res. 156, 188–196. doi: 10.1016/j.neures.2020.02.004
Unakafov, A. M. (2015). Ordinal-patterns-based segmentation and discrimination of time series with applications to EEG data (Ph.D. thesis). University of Lübeck, Lübeck, Germany.
van der Maaten, L., and Hinton, G. (2008). Visualizing data using t-SNE. J. Mach. Learn. Res. 9, 2579–2605.
van der Maaten, L., Postma, E., and van der Herik, J. (2007). Dimensionality reduction: a comparative review. J. Mach. Learn. Res. 10, 2579–2605.
Vicente, R., Wibral, M., Lindner, M., and Pipa, G. (2011). Transfer entropy–a model-free measure of effective connectivity for the neurosciences. J. Comput. Neurosci. 30, 45–67. doi: 10.1007/s10827-010-0262-3
Wada, Y., Nanbu, Y., Kikuchi, M., Koshino, Y., Hashimoto, T., and Yamaguchi, N. (1998). Abnormal functional connectivity in Alzheimer's disease: intrahemispheric EEG coherence during rest and photic stimulation. Eur. Arch. Psychiatry Clin. Neurosci. 248, 203–208. doi: 10.1007/s004060050038
Waschke, L., Wöstmann, M., and Obleser, J. (2017). States and traits of neural irregularity in the age-varying human brain. Sci. Rep. 7:17381. doi: 10.1038/s41598-017-17766-4
Wibral, M., Pampu, N., Priesemann, V., Siebenhühner, F., Seiwert, H., Lindner, M., et al. (2013). Measuring information-transfer delays. PLoS ONE 8:e55809. doi: 10.1371/journal.pone.0055809
Wilaiprasitporn, T., Ditthapron, A., Matchaparn, K., Tongbuasirilai, T., Banluesombatkul, N., and Chuangsuwanich, E. (2020). Affective EEG-based person identification using the deep learning approach. IEEE Trans. Cogn. Dev. Syst. 12, 486–496. doi: 10.1109/TCDS.2019.2924648
Zhang, J., Zhou, J., Tang, M., Guo, H., Small, M., and Zou, Y. (2017). Constructing ordinal partition transition networks from multivariate time series. Sci. Rep. 7:7795. doi: 10.1038/s41598-017-08245-x
Appendix
To quantify the effect of subject separation and its dependency on considered time scales, the ratio ρ of intra- and inter-cluster-distances was calculated on different time scales. For each projection, the ratio between the mean intra- and inter-cluster-distances was calculated. The average ratio ρ and the standard deviation, depending on the lag for the OPs or the frequency band, is displayed in Figure A1.
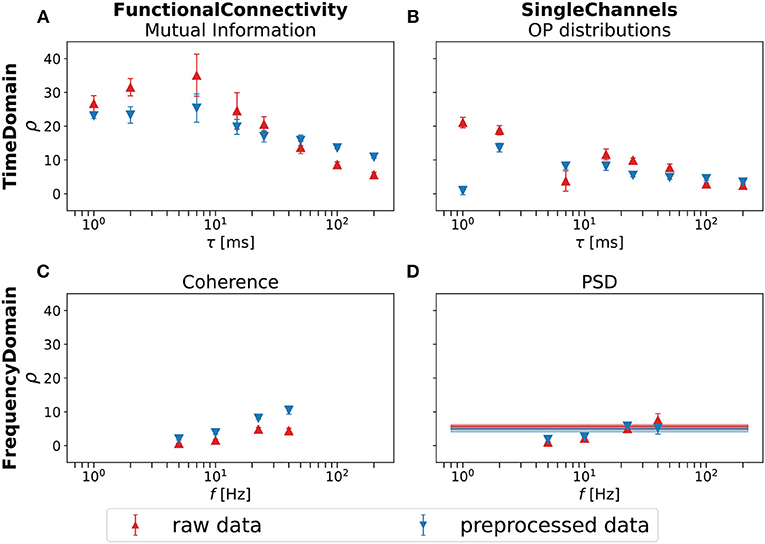
Figure A1. Quantification of separation of subjects in the t-SNE projections (perplexity k = 30) data set for raw and pre-processed EEG data. Both FC measures (A,C) and single-channel measures (B,D) were projected. For each projection, the ratio between the mean intra- and inter-cluster-distances was calculated. We call the Euclidean distances between points belonging to the same subject intra-cluster-distances, and the distances between the center of one cluster and its three next neighbors inter-cluster-distances. The error bars display the standard deviation over 100 t-SNE projections with different random seeds.
Keywords: EEG - Electroencephalogram, t-SNE (t-distributed stochastic neighbor embedding), ordinal pattern statistics, nonlinear dimensionality reduction, biomarkers, functional connectivity, coherence, mutual information
Citation: Kottlarz I, Berg S, Toscano-Tejeida D, Steinmann I, Bähr M, Luther S, Wilke M, Parlitz U and Schlemmer A (2021) Extracting Robust Biomarkers From Multichannel EEG Time Series Using Nonlinear Dimensionality Reduction Applied to Ordinal Pattern Statistics and Spectral Quantities. Front. Physiol. 11:614565. doi: 10.3389/fphys.2020.614565
Received: 06 October 2020; Accepted: 16 December 2020;
Published: 01 February 2021.
Edited by:
Plamen Ch. Ivanov, Boston University, United StatesReviewed by:
Hiromichi Suetani, Oita University, JapanCristina Masoller, Universitat Politecnica de Catalunya, Spain
Klaus Lehnertz, University of Bonn, Germany
Copyright © 2021 Kottlarz, Berg, Toscano-Tejeida, Steinmann, Bähr, Luther, Wilke, Parlitz and Schlemmer. This is an open-access article distributed under the terms of the Creative Commons Attribution License (CC BY). The use, distribution or reproduction in other forums is permitted, provided the original author(s) and the copyright owner(s) are credited and that the original publication in this journal is cited, in accordance with accepted academic practice. No use, distribution or reproduction is permitted which does not comply with these terms.
*Correspondence: Alexander Schlemmer, alexander.schlemmer@ds.mpg.de