- 1Faculty of Science and Technology, Physics Department, Universidad Nacional Pedro Henríquez Ureña, Santo Domingo, Dominican Republic
- 2Centre for Medical Visualization, National Institute for Bioengineering, INABIO, Universidad Central de Venezuela, Caracas, Venezuela
- 3Centro de Diagnóstico Docente Las Mercedes, Caracas, Venezuela
Tumor interface dynamics is a complex process determined by cell proliferation and invasion to neighboring tissues. Parameters extracted from the tumor interface fluctuations allow for the characterization of the particular growth model, which could be relevant for an appropriate diagnosis and the correspondent therapeutic strategy. Previous work, based on scaling analysis of the tumor interface, demonstrated that gliomas strictly behave as it is proposed by the Family-Vicsek ansatz, which corresponds to a proliferative-invasive growth model, while for meningiomas and acoustic schwannomas, a proliferative growth model is more suitable. In the present work, other morphological and dynamical descriptors are used as a complementary view, such as surface regularity, one-dimensional fluctuations represented as ordered series and bi-dimensional fluctuations of the tumor interface. These fluctuations were analyzed by Detrended Fluctuation Analysis to determine generalized fractal dimensions. Results indicate that tumor interface fractal dimension, local roughness exponent and surface regularity are parameters that discriminate between gliomas and meningiomas/schwannomas.
1 Introduction
Tumor interface exhibits a complex and irregular geometry due to the dynamics involved in the tumor growth process, which in general takes into account tumor cell proliferation and invasion into the surrounding tissue. To characterize its complexity, fractal analysis has been used routinely for tumor detection (Iftekharuddin et al., 2009) and therapy monitoring (Di Ieva et al., 2012). In the case of brain tumors, magnetic resonance imaging techniques give detailed geometrical information with excellent spatial resolution and quality for the evaluation of the tumor interface. Parameters extracted from the tumor interface by scaling and fractal analysis have given relevant clues about the complex tumor growth dynamics (Brú et al., 2012) (Brú et al., 2003) (Brú et al., 2008) (Torres Hoyos and Martín-Landrove, 2012) which in turn can be used to further validate tumor growth models (Brú et al., 2014) for therapy simulation and prognosis. In a previous work (Martín-Landrove et al., 2020) it was demonstrated that scaling analysis provided a clear difference in the tumor growth model for gliomas, which follow a ballistic growth model in completely agreement with the Family-Vicsek ansatz (Family and Vicsek, 1991) (Barabasi and Stanley, 1995), compared to meningiomas/schwannomas. A different approach that includes fractal properties of the tumor interface or surface has been proposed by defining surface regularity measures (Pérez-Beteta et al., 2018) (Popadic et al., 2021). So far, scaling analysis parameters, such as fractal dimension and local roughness exponent, and surface regularity measures give a global picture of the fractal properties of the tumor interface. Since the tumor growth process is heterogeneous it is expected that its fractal properties should be heterogeneous as well and a more general approach is needed and general multifractal analysis methods (Kantelhardt et al., 2002) (Lopes and Betrouni, 2009) (Gu and Zhou, 2006) applied to the fluctuations over the interface have to be used. In the present work, an extended image database is used to determine an extended group of parameters that characterize the tumor interface, including those previously determined (Martín-Landrove et al., 2020).
Related to the assessment of the tumor interface, several methods have been proposed for the segmentation of brain tumors (Işın et al., 2016) (Wadhwa et al., 2019) (Zhao et al., 2020) from magnetic resonance images, which include conventional methods such as thresholding and region growing, supervised methods mainly represented by support vectors machines and artificial neural networks and unsupervised methods which include clustering methods and deformable models. Even though supervised methods perform better than unsupervised ones (Rao et al., 2018), for the purpose of the present work, unsupervised methods, such as K-means or Fuzzy C-means are preferable since these methods do not need any training set and in many cases are simpler in its numerical implementation. In the present work, an unsupervised method based on dynamic quantum clustering (Weinstein and Horn, 2009), (Horn and Gottlieb, 2001) is used for image segmentation to determine the tumor interface.
The article is organized as follows, in Section 2 it is described the selection of images for this study, the segmentation method employed for determination of the tumor interface and the different morphological parameters that describe the tumor interface such as fractal dimension and lacunarity, growth dynamics exponents, regularity measures, complex visibility graphs and parameters derived from multifractal analysis. The results are discussed in Section 3 and the conclusions are presented in Section 4.
2 Materials and Methods
2.1 Image Selection
Images for high grade gliomas were extracted from different collections in The Cancer Imaging Archive (Chang et al., 2011), (Clark et al., 2013); The Cancer Genome Atlas Low Grade Glioma (TCGA-LGG) data collection (Pedano et al., 2020), the Repository of Molecular Brain Neoplasia Data (REMBRANDT) (Scarpace et al., 2019) for astrocytomas and oligodendrogliomas of grades 2 and 3, and The Cancer Genome Atlas Glioblastoma multiforme [TCGA-GBM] collection (Scarpace et al., 2016) for glioblastoma multiforme. Also, data coming from the RSNA-ASNR-MICCAI Brain Tumor Segmentation (BraTS) Challenge 2021 (Baid et al., 2021) (Menze et al., 2015) (Bakas et al., 2017). For other brain neoplasias, such as meningiomas and acoustic schwannomas, local image datasets were used. Among these collections, only contrast enhanced T1-weighted images, with tumor lesions clearly identified as such and separated from anatomical structures, were selected for analysis.
2.2 Clustering of Data and Image Segmentation
Image digital levels were clustered using the Dynamic Quantum Clustering algorithm (DQC) (Weinstein and Horn, 2009) (Horn and Gottlieb, 2001) which assumes that data are described by a set of points, each one defined with some uncertainty, σ and the distribution for all points in data space is given by a Parzen estimator, φ, which satisfies the time independent Schrödinger equation for its ground state,
which leads to the evaluation of an energy potential
The number of potential minima (Horn and Gottlieb, 2001) was previously used (Martín-Landrove et al., 2020) to determine the number of classes in a K-means algorithm alone. In the present work, the full dynamic quantum clustering algorithm is used and by Ehrenfest theorem, digital levels evolve in time toward the potential minima according to the following equation of motion (Lafata et al., 2018),
which is a second order Langevin equation with a dissipative term γ. The clustering process is performed with a suitable selection of parameters such as σ2, which is an equivalent to a “mass”, and determines the number of classes among the digital levels (Horn and Gottlieb, 2001), dissipation, γ and time interval. These parameters are estimated according to the magnitude of the digital levels in the image and assess the evolution of all the data to the potential minima. An example of clustering of digital levels is shown in Figure 1. For image segmentation the dynamics is performed as a two step process (Sánchez and Martín-Landrove, 2021):
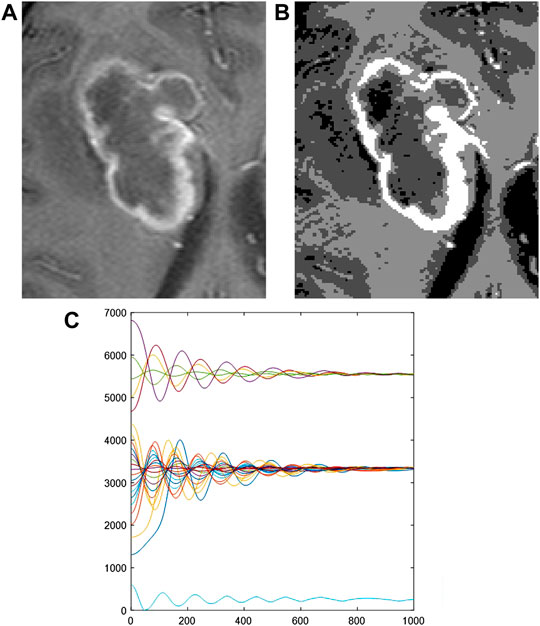
FIGURE 1. Clustering of digital levels. (A) Original image, (B) clustered image, (C) Time evolution of digital levels.
Dynamic A: First application of the dynamics upon the original image using the potential calculated from the original image histogram. At the end of this step, a Parzen estimator is evaluated using the clustered image histogram, allowing for the calculation of a “trap” potential, which defines the number of classes if a further classification algorithm, such as K-means, is to be used. It has been proved that the dynamic quantum clustering algorithm provides the same set of centroids as the K-means algorithm (Sánchez and Martín-Landrove, 2021).
Dynamic B: Second application of the dynamics upon the original image using the “trap” potential.
In Figure 2 it is shown how the selection of the appropriate σ2 determines the number of classes and therefore the image segmentation. It is important to note that the “trap” potential compared to the original Schrödinger potential, exhibit a well defined minima, which allows for an unsupervised definition of the number of clusters and an improvement in the assessment of the tumor interface.
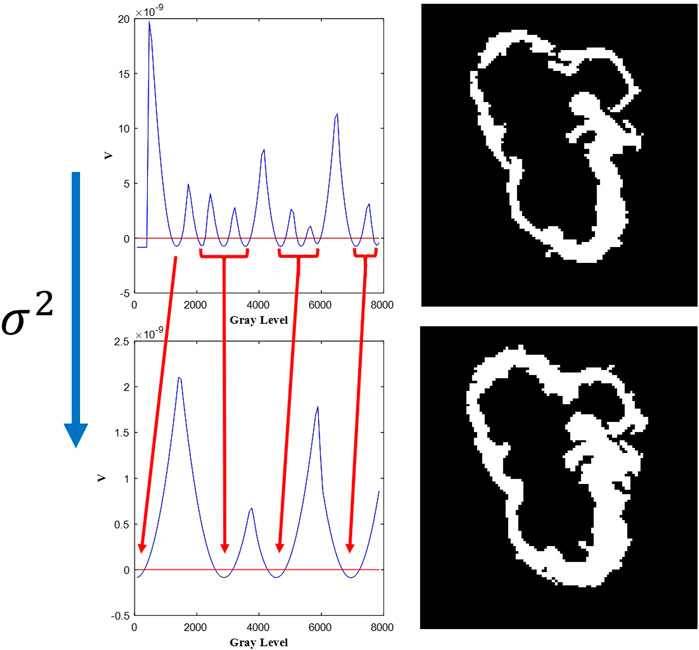
FIGURE 2. Dependence of the segmentation procedure on the value of the ‘mass’ of the particle, σ2. Arrows indicate how the potential energy minima, in the ‘trap’ potential, collapse as σ2 is increased from top to bottom by a 3-fold factor. On the right, the corresponding segmentation.
2.3 Morphological Parameters
2.3.1 Fractal Dimension and Lacunarity of the Tumor Interface
Fractal dimension (Iftekharuddin et al., 2003) and lacunarity (Plotnick et al., 1993) have been used as morphological parameters to characterize tumor interface and grading of brain tumors (Smitha et al., 2015) (Park et al., 2020). Both quantities can be calculated using a box counting algorithm and are defined as
with N (ϵ), the number of boxes containing the fractal object and ϵ the size of the box. In a similar way, lacunarity is defined as
where μ (ϵ) is the mean of point density within the box of size ϵ and σ (ϵ), the standard deviation.
2.3.2 Scaling Analysis and Tumor Growth Dynamics
Tumor interface, in both resected and in vitro samples (Brú et al., 2012) (Brú et al., 2003), and in vivo (Torres Hoyos and Martín-Landrove, 2012) (Martín-Landrove et al., 2016) (Martín-Landrove et al., 2020) has been characterized using scaling analysis techniques. These studies have shown that tumor contours exhibit super-rough scaling dynamics described by the Family–Vicsek ansatz (Family and Vicsek, 1991) (Barabasi and Stanley, 1995) that corresponds to a ballistic growth process or a proliferative-invasive tumor growth model. The roughness of the tumor interface can be parameterized at the global level, with an exponent α, and at the local level by a roughness exponent, αloc. In three dimensions, the local roughness exponent relates the scale-averaged width of the interface between tumor and host to the scale of growth s, exhibiting a power-law behavior for small s (Torres Hoyos and Martín-Landrove, 2012) (Martín-Landrove et al., 2016):
with W given by (Brú et al., 2008),
where
Also, the saturation value of the interface width, Wsat, scales with average of the tumor size, ⟨R⟩ as
where α is the global roughness exponent.
2.3.3 Surface Regularity Measures
A surface regularity measure has been proposed to characterize glioblastoma multiforme (Pérez-Beteta et al., 2018) by the following equation,
where TV is the total volume of the tumor and TVeq is the volume of a sphere which has the same surface area as the tumor, TS. Thus
In a similar way, a surface factor has been proposed (Popadic et al., 2021) for grading meningioma tumors. the surface factor is defined as,
where TSeq is the surface area of an sphere with a volume equal to the tumor volume, TV and TS is the surface area of the tumor. In terms of TV and TS, SF can be written,
So any of the proposed regularity measures SR or SF are equivalent for the description of the surface regularity and therefore SR will be used in the present work. According to (Pérez-Beteta et al., 2018), SR is related to the fractality or roughness of the tumor surface, i.e., if SR ≪ 1, tumor exhibits a distinct fractal dimension with a rough surface, while otherwise its fractal dimension is close to the Euclidean one and the tumor surface is smooth. Nevertheless, the actual value of SR must be corrected by a shape factor due to the fact that the distribution of points over the tumor surface could be elongated along certain directions, departing from the roughed sphere condition,
fshape is calculated in a likewise way as SR,
where Vellipsoid is the volume of an ellipsoid obtained by principal component analysis of the point distribution over the tumor surface and Vsphere is the volume of a sphere with the same surface area as the ellipsoid. For the tumor interface, the Fractional Anisotropy is defined as,
where λi, i = 1, 2, 3 are the ellipsoid axes. In Figure 3 it is shown how FA determines the tumor interface correction that leads to Equation 14. Another factor can be defined which takes into account the distribution of contrast inside the tumor,
where TVC is the volume of the region with contrast and TSC is its total surface area, including inner and outer surfaces.
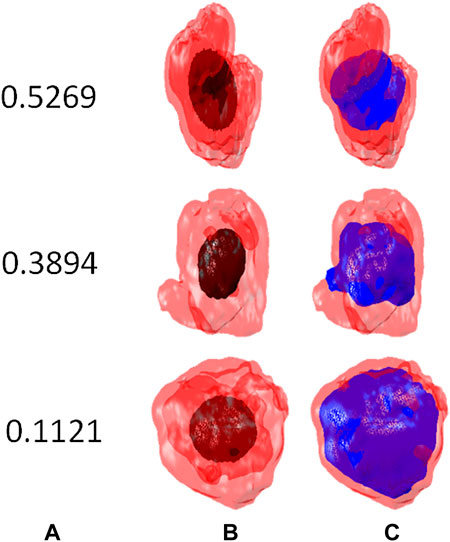
FIGURE 3. Principal component analysis of the tumor interface. (A) fractional anisotropy of the ellipsoid obtained by PCA, (B) PCA ellipsoids, (black), located inside the tumor interface (red) and (C) tumor interface correction (blue).
2.3.4 Ordered Series and Visibility Graphs
Ordered series and the associated visibility graphs (Lacasa et al., 2008) (Lacasa et al., 2009) that can be extracted from the tumor interface has been used to further discriminate between the dynamical models that describe tumor growth (Brú et al., 2014). In a previous work (Martín-Landrove et al., 2020), it has been demonstrated that the associated visibility graph, through its connectivity distribution function P (k) discriminates between gliomas and meningiomas/schwannomas, in the exponent of its power law behavior,
In the present work, the analysis of the ordered series and its associated graph is extended one step further to determine its scaling properties (Pérez-Beteta et al., 2018). Similarly to Equation 7, the local standard deviation of the vertex degree for a subset ϕ on the ordered series can be written as (Brú et al., 2014) (Estrada, 2010),
It exhibits a power-law behaviour for small ϕ (Brú et al., 2014),
where a represents the local variance exponent and for ϕ = 2π, Wk is related to the global variance or heterogeneity of the associated visibility graph, as shown in Figure 4.
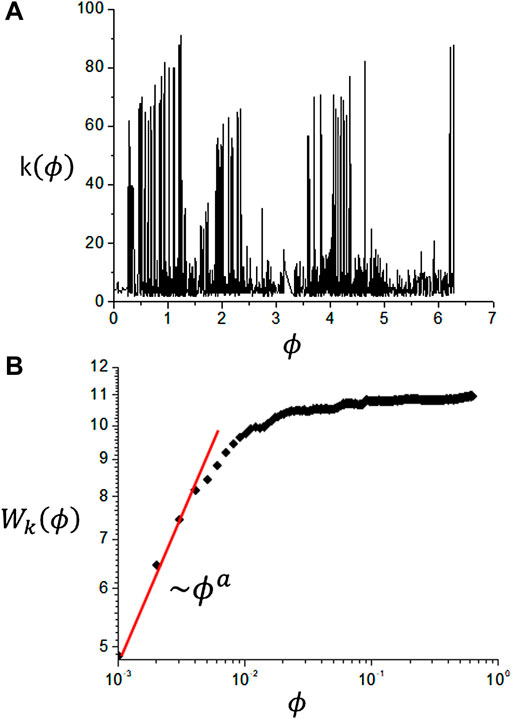
FIGURE 4. Analysis of angular ordered series (A) Connectivity series. (B) local standard deviation of the connectivity series showing a power-law dependence on ϕ.
2.3.5 Multifractal Analysis
In order to determine the multifractal scaling exponents for the one-dimensional ordered series extracted from the tumor interface, a general procedure of fluctuation analysis is used (Kantelhardt et al., 2002) (Lopes and Betrouni, 2009). First, the profile of the ordered series is determined by a cumulative sum,
where ⟨r⟩ represents the mean radius of the tumor interface. The profile series is then partitioned into Ns ≡int (N/s) segments of equal length s, the box probability ps (v), which is the sum of the values rk within each segment v of size s, is defined as,
The scaling properties and exponents can be obtained through the partition function,
For large values of s, a power law behavior is obtained for Zq (s), allowing for a definition of the scaling exponent τ1 (q) that characterizes the one-dimensional fluctuations,
The generalized fractal dimensions are then defined as,
and the generalized Hurst exponents can be obtained from the relation,
The ordered series that can be extracted from any slice represent a one dimensional sampling of the tumor interface and as a consequence an incomplete picture of the tumor interface fluctuations. A more general approach is possible if a two dimensional detrended fluctuation analysis (Gu and Zhou, 2006) is performed. In this case, the tumor interface is parameterized as a two dimensional array with elements r (nϕ, nZ), the radii of the tumor interface, and is partitioned in two dimensional segments of size s. The detrended fluctuation in each segment is given by,
where ϵv,w (i, j) is the difference between the cumulative sum of r (i, j) and its trend over the segment (v, w). The average of the detrended fluctuation over all the segments is,
for q ≠ 0 and for q = 0,
For large values of s, Fq behaves as a power law,
The multifractal nature of the fluctuation is characterized by the scaling exponents τ2 (q) and related to the exponents h2 (q) by,
and Equation 25 holds for the generalized fractal dimensions D2 (q).
3 Results and Discussion
A total of 609 tumor interfaces were analyzed, discriminated as follows, 176 benign tumors including 99 meningiomas and 77 acoustic schwannomas, all of them coming from local databases, 46 Grade II and Grade III astrocytomas and oligodendrogliomas (Pedano et al., 2020) (Scarpace et al., 2019) and 387 glioblastoma multiforme Grade IV tumors (Scarpace et al., 2016) (Baid et al., 2021). The tumor interfaces were selected by its size, i.e., the number of points in the tumor interface must be greater than a certain threshold, allowing for adequate statistics in the evaluation of morphological parameters such as fractal dimension dF or local roughness exponent, αloc. Also, the distribution of points must be as isotropic as possible, with values of the fractional anisotropy, equation (Işın et al., 2016), closest to zero. This is performed by the procedure described in Section 2.3.3 and shown in Figure 3.
3.1 Scaling Analysis Results
Results are shown in Figure 5 which exhibits a high dispersion of the data. Average values are indicated by large circles and correspond from left to right to meningioma, acoustic schwannoma, Grade II and Grade III glioma TCGA-LGG and REMBRANDT databases), glioblastoma multiforme and high grade glioma (BraTS 2021 database) and glioblastoma multiforme (TCGA-GBM database). These average values are summarized in Table 1. There is a clear difference between meningiomas and acoustic schwannomas, top of Table 1, with average parameters dF = 1.97 ± 0.08 and αloc = 0.68 ± 0.11 and gliomas, bottom of Table 1, with dF = 2.11 ± 0.12 and αloc = 0.80 ± 0.14, a result that is consistent with previous research using segmentation schemes based on k-means classification alone and smaller data sets (Torres Hoyos and Martín-Landrove, 2012) (Martín-Landrove et al., 2020) (Martín-Landrove et al., 2016). This fact is also shown in Figure 6 in the comparison of the corresponding histograms for dF and αloc. The values of the local roughness exponent αloc and the fractal dimension dF give important information about what proliferative-invasive process is taking place in the dynamics of tumor growth, if the sum of these parameters is close to the Euclidean dimension, dE, as reflected by Equation 8, the tumor growth dynamics corresponds to a ballistic growth model, characterized by the ansatz of Family-Vicsek (Family and Vicsek, 1991) (Barabasi and Stanley, 1995). Inspection of Table 1 reveals that this is indeed the case for Grade II and III gliomas and glioblastoma multiforme, dF + αloc = 2.92 ± 0.22. In the case of meningiomas and acoustic schwannomas, the tumor growth dynamics corresponds to a different growth model since dF + αloc = 2.65 ± 0.16. Also, from Table 1, lacunarity values, λ, exhibits a variation that it is in correspondence with fractal dimension, dF. Another important feature comes from the results shown in Figure 7 which depicts the dependence of Wsat with respect to the average size of the tumor lesion, ⟨R⟩. Trend of the data conforms to a power law according to Equation 9 and the results are summarized in Table 2. There is a clear difference between tumor groups, for gliomas, α = 0.948 ± 0.038, and for meningiomas and acoustic schwannomas, α = 0.730 ± 0.087, which is what has to be expected if the growth dynamics governing the tumor interface corresponds to a more invasive process as it happens to be the case for malignant tumors.
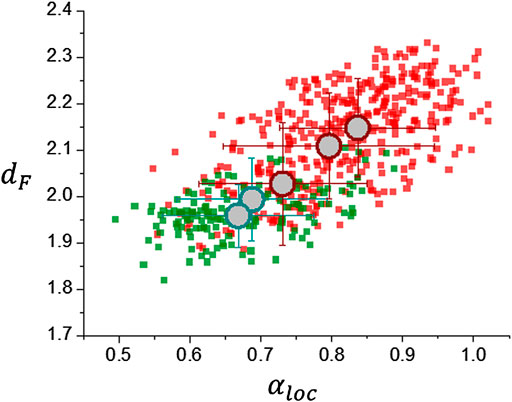
FIGURE 5. Scaling analysis results for different brain tumor databases: Glioblastoma multiforme:TCGA-GBM and BraTS 2021, and Glioma: TCGA-LGG and REMBRANDT, are represented in red color; Meningioma and Acoustic Schwannoma correspond to local databases, represented in green color. Large circles indicate average values of data points.
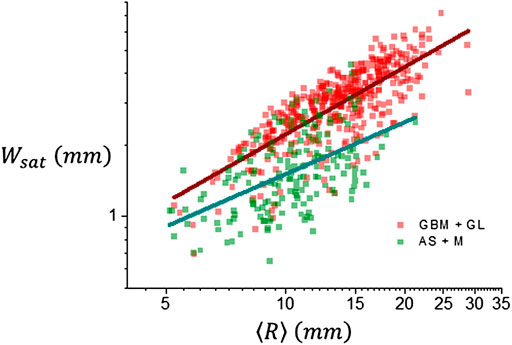
FIGURE 7. Scaling behavior of Wsat with tumor size R, according to Equation 9. Lines represent the trend of the data Values of the global roughness exponent α are 0.74 ± 0.09 for meningiomas and acoustic schwannomas (AS + M) and 0.94 ± 0.04 for high grade gliomas and glioblastoma multiforme (GBM + GL).
3.2 Regularity Measures Results
The relationship between SC and SR are shown in Figure 8. Data points are dispersed below the diagonal since SC takes into account both inner and outer surfaces while SR only takes into account the outer surface (Pérez-Beteta et al., 2018). Data points along the diagonal correspond to tumors that lack the presence of either contrast free or necrotic volumes, a condition that occurs more frequently for meningiomas and acoustic schwannomas than for gliomas as seen in Figure 8. Large circles in Figure 8 correspond to average values that are summarized in Table 3. The average values of SR for meningiomas and acoustic schwannomas, 0.64 ± 0.16 and gliomas, 0.57 ± 0.23, do not differ significantly. On the other hand, average values of SC are 0.61 ± 0.17 for meningiomas and acoustic schwannomas, and 0.31 ± 0.17 for gliomas, have a difference that clearly discriminates between these groups. In order to enhance the difference among tumor groups, the ratio SC/SR is used, as seen from Table 3. Figure 9 shows frequency distributions for meningiomas and acoustic schwannomas, with an average value ⟨SC/SR⟩ = 0.94 ± 0.11, and gliomas with ⟨SC/SR⟩ = 0.58 ± 0.23. Since regularity measures are related to the fractal properties of the tumor interface (Pérez-Beteta et al., 2018) there must be a certain correlation to the scaling parameters dF and αloc. For tumors with SC/SR close to 1, fractal dimension, dF, should be close to 2 and αloc should have its lowest value, i.e., tumor surface is regular and smooth. As SC/SR decreases it is expected an increase in the scaling parameters. Figure 10 shows this trend for the dependence of dF and αloc on SC/SR. The slopes that characterize the linear trend are summarized in Table 4.
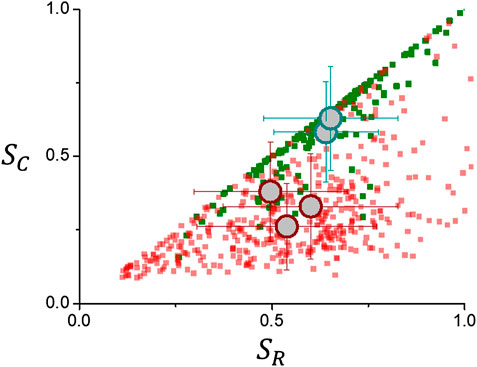
FIGURE 8. Regularity measures for brain tumors. Circles represent average values for meningiomas and acoustic schwannomas (green) and high grade gliomas and glioblastoma multiforme (red).
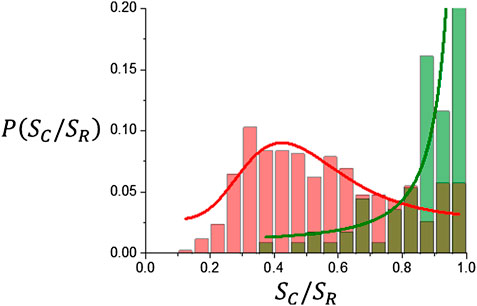
FIGURE 9. Distribution histograms for SC/SR. Meningiomas and acoustic schwannomas are indicated in green and gliomas in red.
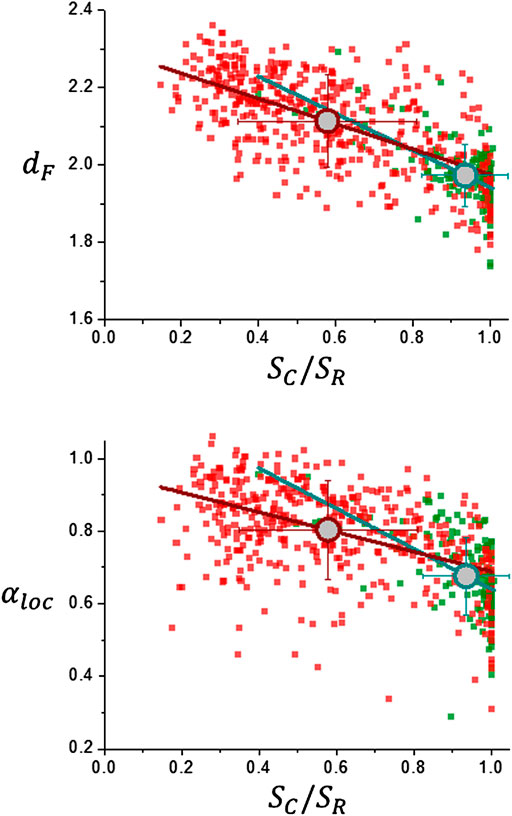
FIGURE 10. Relationship between scaling analysis parameters dF and αloc with regularity measure ratio SC/SR. Large circles represent average values: meningiomas and acoustic schwannomas (green) and gliomas (red).
3.3 Ordered Series, Visibility Graphs and Multifractal Analysis Results
Ordered series were extracted from the tumor interface for those slices that contain the maximum number of interface points, visibility graphs were generated and the degree distribution functions were obtained. Results for the average of P (k) distributions obtained for each of the different tumor types are shown in Figure 11A. For values of the connectivity index k > 20, P (k) decays abruptly due to the fact that the ordered series has a finite size which limits the probability P (k). For values of the connectivity index k < 20 all distributions exhibit a power law behavior as shown in Figure 12A with slopes that are summarized in Table 5 and shown in Figure 12B. If the tumor types are discriminated only into two classes: one including meningiomas and acoustic schwannomas and the other including high grade gliomas and glioblastoma multiforme, the slope distributions are clearly distinct for each tumor class as shown in Figure 11B. This result suggest the possibility of using γ as a possible parameter that characterizes tumor interface dynamics supported by the result shown in Figure 12B. Multifractal analysis results are summarized in Figure 13. As expected, the evaluation of generalized fractal dimensions for the one dimensional sampling of the tumor interface, Figure 13A, does not provide with enough information to discriminate between these two classes as seen in Figure 13B and summarized in Table 6. On the other hand, two dimensional detrended fluctuation analysis of the tumor interface yield some differences in the average values of D (q), as shown in Table 6 and Figure 13D, that possibly could be improved by an adequate sampling of the tumor interface, i.e., higher image longitudinal and transverse resolution. In any case, generalized fractal dimensions do not discriminate appropriately between tumor types.
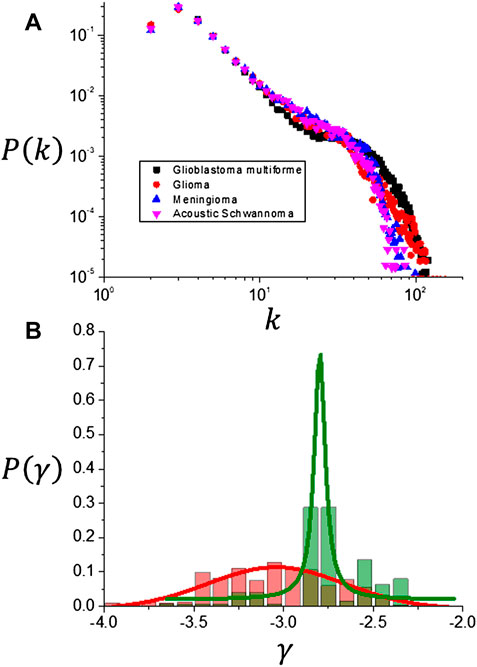
FIGURE 11. (A) Visibility graph degree distributions P (k) for different tumor types. A power law behavior region is observed for k < 20 and the probability decreases abruptly beyond that value. (B) Frequency distributions for the exponent γ of the power law behavior.
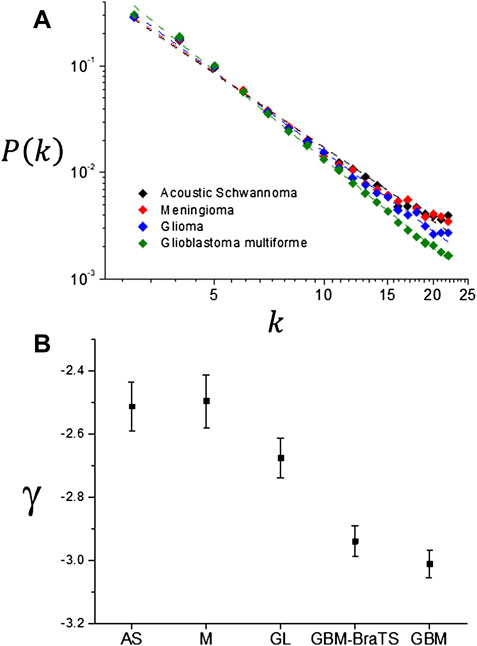
FIGURE 12. (A) Detail of the power law region for the visibility graph degree distributions. (B) Exponent γ dependence on tumor type.
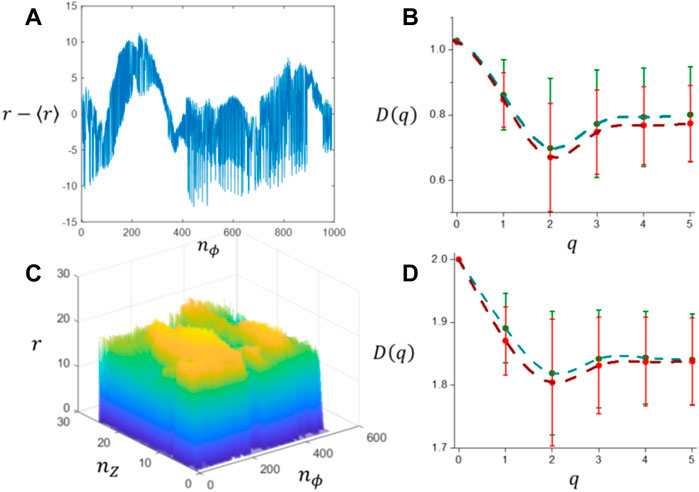
FIGURE 13. Multifractal and detrended fluctuation analysis results. (A) Typical ordered series extracted from an slice; (B) Generalized fractal dimensions associated to the ordered series obtained by multifractal analysis using the partition function Z; (C) Two dimensional landscape for r as a function of nZ and nϕ, and (D) Generalized fractal dimensions obtained by 2D Detrended Fluctuation Analysis. In (B,D), meningiomas and acoustic schwannomas are represented in green and gliomas in red.

TABLE 6. Generalized fractal dimensions obtained by Detrended Fluctuation Analysis on one dimensional ordered series, D1 (1) and D1 (2), and two dimensional interface data space r (nϕ, nZ).
4 Conclusion
A method based on dynamic quantum clustering is used to perform contrast enhanced MRI of brain tumors. Tumor interfaces can be classified according to scaling analysis parameters such as the fractal dimension, dF and the local roughness exponent, αloc which clearly differentiate between the growth dynamics of different tumor types adding support of a ballistic growth model for gliomas and glioblastomas, following the Family-Vicsek ansatz and a non-ballistic growth model for other neoplasias such as meningiomas and acoustic schwannomas. Among the regularity measures, the ratio SC/SR exhibit some correlation with the scaling parameters and clearly discriminates between gliomas and meningiomas or acoustic schwannomas. The relation between dF, αloc and SC/SR is shown in Figure 14. Parameters obtained in series extracted from the tumor interface are size sensitive but nevertheless exhibit differences that could be used for tumor classification and in particular its growth dynamics, through the exponent γ. Generalized fractal dimensions obtained by two dimensional detrended fluctuation analysis could possibly give significant differences if the tumor interface could be sampled with higher resolution. Further research should take into account combination of different MRI modalities.
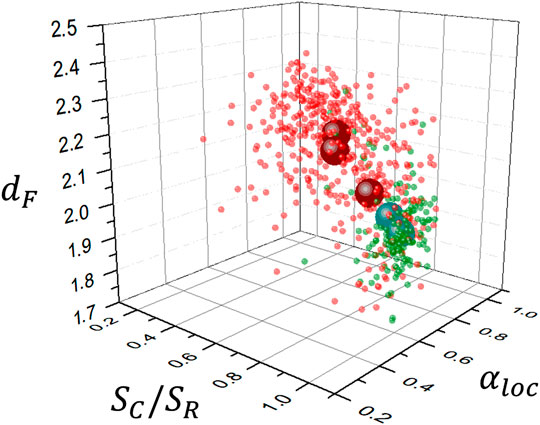
FIGURE 14. Relevant morphological and scaling parameters used to discriminate between meningiomas and acoustic schwannomas (green) and gliomas (red). Average values are represented as large dots.
Data Availability Statement
The raw data supporting the conclusions of this article will be made available by the authors, without undue reservation.
Author Contributions
All authors listed have made a substantial, direct, and intellectual contribution to the work and approved it for publication.
Conflict of Interest
The authors declare that the research was conducted in the absence of any commercial or financial relationships that could be construed as a potential conflict of interest.
Publisher’s Note
All claims expressed in this article are solely those of the authors and do not necessarily represent those of their affiliated organizations, or those of the publisher, the editors and the reviewers. Any product that may be evaluated in this article, or claim that may be made by its manufacturer, is not guaranteed or endorsed by the publisher.
Acknowledgments
This research was supported by Universidad Central de Venezuela, INABIO and Universidad Nacional Pedro Henríquez Ureña. We thank our colleagues from these institutions who provided insight and expertise that greatly assisted the research.
References
Baid U., Ghodasara S., Mohan S., Bilello M., Calabrese E., Colak E., et al. (2021). The Rsna-Asnr-Miccai Brats 2021 Benchmark on Brain Tumor Segmentation and Radiogenomic Classification. Ithaca, NY, United States: arXiv:2107.02314v2 [cs.CV].
Bakas S., Akbari H., Sotiras A., Bilello M., Rozycki M., Kirby J. S., et al. (2017). Advancing the Cancer Genome Atlas Glioma Mri Collections with Expert Segmentation Labels and Radiomic Features. Sci. Data 4, 170117. doi:10.1038/sdata.2017.117
Barabasi A., Stanley H. (1995). Fractal Concepts in Surface Growth. Cambridge, UK: Cambridge University Press.
Brú A., Albertos S., Luis Subiza J., García-Asenjo J. L., Brú I. (2003). The Universal Dynamics of Tumor Growth. Biophysical J. 85, 2948–2961. doi:10.1016/S0006-3495(03)74715-8
Brú A., Alós E., Nuño J. C., de Dios M. F. (2014). Scaling in Complex Systems: a Link between the Dynamics of Networks and Growing Interfaces. Sci. Rep. 4, 7550. doi:10.1038/srep07550
Brú A., Casero D., de Franciscis S., Herrero M. A. (2008). Fractal Analysis and Tumour Growth. Math. Comput. Model. 47, 546–559. doi:10.1016/j.mcm.2007.02.033
Brú A., Pastor J. M., Fernaud I., Brú I., Melle S., Berenguer C. (2012). Super-rough Dynamics on Tumor Growth. Phys. Rev. Lett. 81, 4008–4011. doi:10.1103/PhysRevLett.81.4008
Chang H., Fontenay G. V., Han J., Cong G., Baehner F. L., Gray J. W., et al. (2011). Morphometic Analysis of Tcga Glioblastoma Multiforme. BMC Bioinforma. 12, 484. doi:10.1186/1471-2105-12-484
Clark K., Vendt B., Smith K., Freymann J., Kirby J., Koppel P., et al. (2013). The Cancer Imaging Archive (Tcia): Maintaining and Operating a Public Information Repository. J. Digit. Imaging 26, 1045–1057. doi:10.1007/s10278-013-9622-7
Di Ieva A., Matula C., Grizzi F., Grabner G., Trattnig S., Tschabitscher M. (2012). Fractal Analysis of the Susceptibility Weighted Imaging Patterns in Malignant Brain Tumors during Antiangiogenic Treatment: Technical Report on Four Cases Serially Imaged by 7 T Magnetic Resonance during a Period of Four Weeks. World Neurosurg. 77, e11–785. e11–e21. doi:10.1016/j.wneu.2011.09.006
Estrada E. (2010). Quantifying Network Heterogeneity. Phys. Rev. E 82, 066102. doi:10.1103/PhysRevE.82.066102
Gu G.-F., Zhou W.-X. (2006). Detrended Fluctuation Analysis for Fractals and Multifractals in Higher Dimensions. Phys. Rev. E 74, 061104. doi:10.1103/PhysRevE.74.061104
Horn D., Gottlieb A. (2001). Algorithm for Data Clustering in Pattern Recognition Problems Based on Quantum Mechanics. Phys. Rev. Lett. 88, 018702. doi:10.1103/PhysRevLett.88.018702
Iftekharuddin K. M., Jia W., Marsh R. (2003). Fractal Analysis of Tumor in Brain Mr Images. Mach. Vis. Appl. 13, 352–362. doi:10.1007/s00138-002-0087-9
Iftekharuddin K. M., Zheng J., Islam M. A., Ogg R. J. (2009). Fractal-based Brain Tumor Detection in Multimodal Mri. Appl. Math. Comput. 207, 23–41. doi:10.1016/j.amc.2007.10.063
Işın A., Direkoğlu C., Şah M. (2016). Review of Mri-Based Brain Tumor Image Segmentation Using Deep Learning Methods. Procedia Comput. Sci. 102, 317–324. doi:10.1016/j.procs.2016.09.407
Kantelhardt J. W., Zschiegner S. A., Koscielny-Bunde E., Havlin S., Bunde A., Stanley H. E. (2002). Multifractal Detrended Fluctuation Analysis of Nonstationary Time Series. Phys. A Stat. Mech. its Appl. 316, 87–114. doi:10.1016/S0378-4371(02)01383-3
Lacasa L., Luque B., Ballesteros F., Luque J., Nuño J. C. (2008). From Time Series to Complex Networks: The Visibility Graph. Proc. Natl. Acad. Sci. U.S.A. 105, 4972–4975. doi:10.1073/pnas.0709247105
Lacasa L., Luque B., Luque J., Nuño J. C. (2009). The Visibility Graph: A New Method for Estimating the Hurst Exponent of Fractional Brownian Motion. Europhys. Lett. 86, 30001. doi:10.1209/0295-5075/86/30001
Lafata K., Zhou Z., Liu J. G., Yin F. F. (2018). Data Clustering Based on Langevin Annealing with a Self-Consistent Potential. Ithaca, NY, United States: arXiv:1806.10597v1 [physics.comp-ph].
Lopes R., Betrouni N. (2009). Fractal and Multifractal Analysis: A Review. Med. Image Anal. 13, 634–649. doi:10.1016/j.media.2009.05.003
Martín-Landrove M., Brú A., Rueda-Toicen A., Torres-Hoyos F. (2016). “Tumor Growth in the Brain: Complexity and Fractality,” in The Fractal Geometry of the Brain. Editor A. D. Ieva (New York: Springer), 351–369. doi:10.1007/978-1-4939-3995-4_22
Martín-Landrove M., Torres-Hoyos F., Rueda-Toicen A. (2020). Complexity of Brain Tumors. Phys. A Stat. Mech. its Appl. 537, 122696. doi:10.1016/j.physa.2019.122696
Menze B. H., Jakab A., Bauer S., Kalpathy-Cramer J., Farahani K., Kirby J., et al. (2015). The Multimodal Brain Tumor Image Segmentation Benchmark (Brats). IEEE Trans. Med. Imaging 34, 1993–2024. doi:10.1109/TMI.2014.2377694
Park Y. W., Kim S., Ahn S. S., Han K., Kang S.-G., Chang J. H., et al. (2020). Magnetic Resonance Imaging-Based 3-dimensional Fractal Dimension and Lacunarity Analyses May Predict the Meningioma Grade. Eur. Radiol. 30, 4615–4622. doi:10.1007/s00330-020-06788-8
[Dataset] Pedano N., Flanders A. E., Scarpace L., Mikkelsen T., Eschbacher J. M., Hermes B., et al. (2020). The Cancer Genome Atlas Low Grade Glioma [tcga-Lgg] Collection. Ithaca, NY, United States: The cancer imaging archive. doi:10.7937/K9/TCIA.2016.L4LTD3TK
Pérez-Beteta J., Molina-García D., Ortiz-Alhambra J. A., Fernández-Romero A., Luque B., Arregui E., et al. (2018). Tumor Surface Regularity at Mr Imaging Predicts Survival and Response to Surgery in Patients with Glioblastoma. Radiology 288, 218–225. doi:10.1148/radiol.2018171051
Plotnick R. E., Gardner R. H., O'Neill R. V. (1993). Lacunarity Indices as Measures of Landscape Texture. Landsc. Ecol. 8, 201–211. doi:10.1007/BF00125351
Popadic B., Scheichel F., Pinggera D., Weber M., Ungersboeck K., Kitzwoegerer M., et al. (2021). The Meningioma Surface Factor: a Novel Approach to Quantify Shape Irregularity on Preoperative Imaging and its Correlation with Who Grade. J. Neurosurg. 136, 1–7. doi:10.3171/2021.5.JNS204223
Rao B. D., Goswami M. M. (2018). “Performance Analysis of Supervised & Unsupervised Techniques for Brain Tumor Detection and Segmentation from Mr Images,” in Proceedings of the International Conference on Intelligent Systems and Signal Processing. Advances in Intelligent Systems and Computing. Editors R. Kher, D. Gondaliya, M. Bhenasiya, L. Ladid, and M. Atiquzzaman (Singapore: Springer), 35–44. doi:10.1007/978-981-10-6977-2_4
Sánchez J., Martín-Landrove M. (2021). A Dynamic Quantum Clustering Approach to Brain Tumor Segmentation. Ithaca, NY, United States: arXiv:2107.07698v2 [physics.med-ph].
[Dataset] Scarpace L., Flanders A., Jain R., Mikkelsen T., Andrews D. (2019). Data from Rembrandt [data Set]. Ithaca, NY, United States: The cancer imaging archive. doi:10.7937/K9/TCIA.2015.588OZUZB
[Dataset] Scarpace L., Mikkelsen T., Cha S., Rao S., Tekchandani S., Gutman D., et al. (2016). Radiology Data from the Cancer Genome Atlas Glioblastoma Multiforme [tcga-Gbm] Collection [data Set]. Ithaca, NY, United States: The cancer imaging archive. doi:10.7937/K9/TCIA.2016.RNYFUYE9
Smitha K. A., Gupta A. K., Jayasree R. S. (2015). Fractal Analysis: Fractal Dimension and Lacunarity from Mr Images for Differentiating the Grades of Glioma. Phys. Med. Biol. 60, 6937–6947. doi:10.1088/0031-9155/60/17/6937
Torres Hoyos F., Martín-Landrove M. (2012). 3-d In Vivo Brain Tumor Geometry Study by Scaling Analysis. Phys. A Stat. Mech. its Appl. 391, 1195–1206. doi:10.1016/j.physa.2011.09.034
Wadhwa A., Bhardwaj A., Singh Verma V. (2019). A Review on Brain Tumor Segmentation of Mri Images. Magn. Reson. Imaging 61, 247–259. doi:10.1016/j.mri.2019.05.043
Weinstein M., Horn D. (2009). Dynamic Quantum Clustering: A Method for Visual Exploration of Structures in Data. Phys. Rev. E 80, 066117. doi:10.1103/PhysRevE.80.066117
Keywords: fractal dimension, scaling analysis, visibility graphs, local roughness exponent, tumor interface, tumor growth dynamics, morphological parameters, tumor surface regularity
Citation: Sánchez J and Martín-Landrove M (2022) Morphological and Fractal Properties of Brain Tumors. Front. Physiol. 13:878391. doi: 10.3389/fphys.2022.878391
Received: 18 February 2022; Accepted: 31 May 2022;
Published: 27 June 2022.
Edited by:
Marina Vladimirovna Zueva, Helmholtz Moscow Research Institute of Eye Diseases (NMITS GB), RussiaReviewed by:
Camillo Sherif, Karl Landsteiner University of Health Sciences, AustriaMartin Ostoja-Starzewski, University of Illinois at Urbana-Champaign, United States
Copyright © 2022 Sánchez and Martín-Landrove. This is an open-access article distributed under the terms of the Creative Commons Attribution License (CC BY). The use, distribution or reproduction in other forums is permitted, provided the original author(s) and the copyright owner(s) are credited and that the original publication in this journal is cited, in accordance with accepted academic practice. No use, distribution or reproduction is permitted which does not comply with these terms.
*Correspondence: Miguel Martín-Landrove, bWdsbXJ0bkBnbWFpbC5jb20=
†These authors have contributed equally to this work and share first authorship