- 1Department of Physics and Astronomy, McMaster University, Hamilton, ON, Canada
- 2Origins Institute, McMaster University, Hamilton, ON, Canada
Red blood cells (RBCs) are the most abundant cell type in the human body and critical suppliers of oxygen. The cells are characterized by a simple structure with no internal organelles. Their two-layered outer shell is composed of a cytoplasmic membrane (RBCcm) tethered to a spectrin cytoskeleton allowing the cell to be both flexible yet resistant against shear stress. These mechanical properties are intrinsically linked to the molecular composition and organization of their shell. The cytoplasmic membrane is expected to dominate the elastic behavior on small, nanometer length scales, which are most relevant for cellular processes that take place between the fibrils of the cytoskeleton. Several pathologies have been linked to structural and compositional changes within the RBCcm and the cell’s mechanical properties. We review current findings in terms of RBC lipidomics, lipid organization and elastic properties with a focus on biophysical techniques, such as X-ray and neutron scattering, and Molecular Dynamics simulations, and their biological relevance. In our current understanding, the RBCcm’s structure is patchy, with nanometer sized liquid ordered and disordered lipid, and peptide domains. At the same time, it is surprisingly soft, with bending rigidities κ of 2–4 kBT. This is in strong contrast to the current belief that a high concentration of cholesterol results in stiff membranes. This extreme softness is likely the result of an interaction between polyunsaturated lipids and cholesterol, which may also occur in other biological membranes. There is strong evidence in the literature that there is no length scale dependence of κ of whole RBCs.
Introduction
Red blood cells (RBCs) are critical suppliers of oxygen to tissues and transport waste carbon dioxide. This unique cell-type thus plays a crucial role in the mammalian metabolism. Healthy RBCs have a biconcave shape, i.e., they appear as round disks with a diameter of ≈ 7 μm and a central dimple under the microscope (as sketched in Figure 1A). Several pathologies, such as sickle cell anemia (Herrick, 1910; Rees et al., 2010), hereditary spherocytosis (Perrotta et al., 2008) or hereditary elliptocytosis (Dresbach, 1904; Palek, 1985), but also infectious diseases, such as malaria (Mohandas and An, 2012), are known to alter the shape of the cells. Unlike most other human cells, RBCs lack complexer internal structures, such as a nucleus or mitochondria, in favor of a larger volume for the oxygen binding protein, hemoglobin.
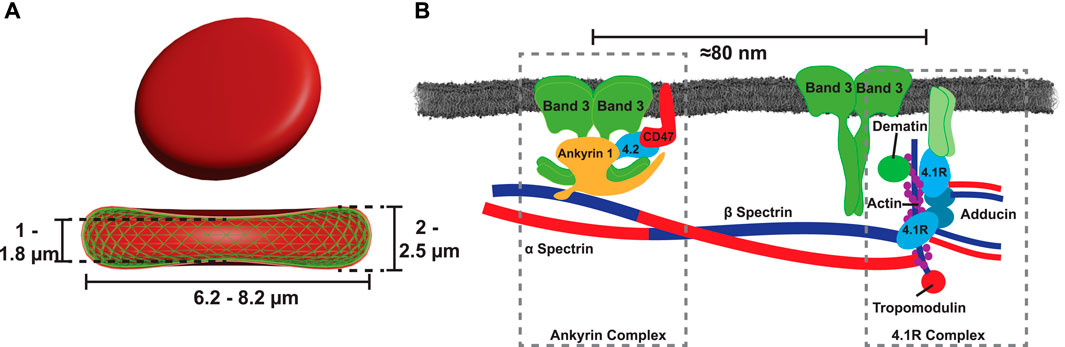
FIGURE 1. (A) Healthy RBCs have a discocyte shape, which assembles the minimal elastic energy of the RBC’s shell. This two layered shell is comprised of a cytoplasmic membrane tethered to a spectrin-based cytoskeleton. (B) The cytoplasmic membrane is tethered to the cytoskeleton via two protein complexes, the ankyrin-based complex, and the 4.1R-based complex (Gallagher, 20052005).
A particularly long debated question concerns the shape of RBCs. In equilibrium, the biconcave shape must correspond to the total minimum elastic energy of the RBC’s outer shell for a given volume and surface area (Viallat and Abkarian, 2014). This outer shell is comprised of two layers as illustrated in Figure 1B: a cytoplasmic membrane (RBCcm) and a spectrin-based cytoskeleton (Liu et al., 1987). The RBC’s cytoskeleton is a two-dimensional structure. It is formed by triangularly arranged spectrin filaments parallel to the RBCcm. The distance between tethers is ≈ 80 nm (Liu et al., 1987). The cytoskeleton is anchored to the RBCcm through tethering sites that are formed by two macromolecular complexes of membrane proteins, the ankyrin-based complex, and the 4.1R-based complex (Mohandas and Gallagher, 2008). A schematic illustration of the molecular tethering sites is shown in Figure 1B. Both complexes are highly mobile within the lipid bilayer and allow the RBCcm to slide against the cytoskeleton (Discher et al., 1994; Viallat and Abkarian, 2014). Although the cell’s discocyte shape represents the minimal state of the shell’s elastic energy, the cytoskeleton is not considered to be stress free (Viallat and Abkarian, 2014): the spectrin network is believed to be slightly stretched in the resting biconcave shape and to exert a compression force on the lipid bilayer (Sens and Gov, 2007; Viallat and Abkarian, 2014). The cytoplasmic membrane is typically described using the fluid mosaic model (Singer and Nicolson, 1972), which pictures this structure as a two-dimensional fluid-like lipid bilayer with embedded proteins. More than 50 of these membrane proteins have been characterized for the RBCcm (Mohandas and Gallagher, 2008).
The RBC lipidome and pathological lipidomic changes
The lipid bilayer is a ≈ 5 nm (Himbert et al., 2017) thick membrane formed by two lipid layers (leaflets). A three-dimensional render of a Molecular Dynamics (MD) simulation of a RBCcm is shown in Figure 2A. There is a variety of different lipids found within the RBCcm. Lipid molecules are amphiphilic and appear in a wide range of molecular structures giving rise to a manifold of influences onto the membrane’s biophysical properties. Notable examples include glycerophospholipids (PL), sphingomyelin (SM) and cholesterol. The chemical composition of these molecules is well known to the scientific community for more than a century.
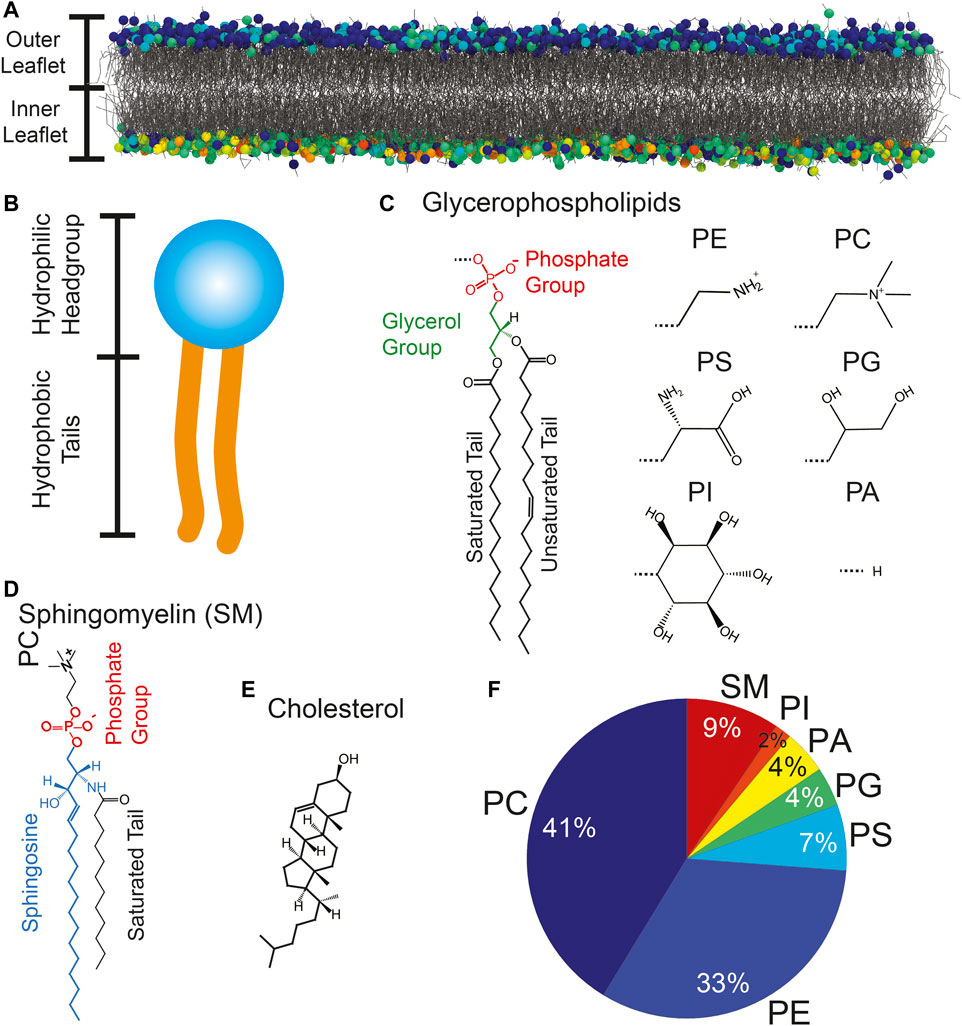
FIGURE 2. (A) The RBCcmis formed by two leaflets of lipids molecules. (B) Glycerophospholipids and sphingomyelin consist of a hydrophilic head group and two hydrophobic tails. (C–E) Chemical structures of glycerophospholipids, sphingomyelin and cholesterol. Common head groups are: Phosphatidylethanolamine (PE), Phosphatidylcholine (PC), Phosphatidylserine (PS), Phosphatidylglycerol (PG), Phosphatidylinositol (PI), and Phosphatidic Acid (PA). (F) Lipid distribution of the RBC cytoplasmic membrane not including cholesterol.
The molecular structure of PL and SM is characterized by a hydrophilic head group and flexible hydrophobic tails (Figure 2B). PLs are built around a glycerol moiety as depicted in Figure 2C. The majority of PLs have two fatty acid tails. Lysophospholipid (LPL) are produced by removing one of the tails through hydrolysis. The structure of SM is visualized in Figure 2D. This molecule is built around sphingosine with an attached fatty acid chain and a phosphatidylcholine (PC) head group. The fatty acid tails can vary in length and degree of saturation. Unlike PL and SM, cholesterol is dominated by a rigid structure formed by hydrocarbon rings (Figure 2E). It can account for up to 50 mol% (Dodge and Phillips, 1967) of the membrane’s lipid content, and typically aligns itself upright along the bilayer normal. The lipidome of the RBCcm was first determined by Dodge & Phillips in 1967 using gas-liquid chromatography (Dodge and Phillips, 1967). Modern mass spectroscopy experiments allow for high throughput analysis (D’Alessandro et al., 2021; Reisz et al., 2019; Stefanoni et al., 2020; Thomas et al., 2020a). Figure 2F shows the contribution of most lipid species not including cholesterol. PC ( ≈ 40%) and phosphatidylethanolamine (PE) ( ≈ 30%) PLs are the most abundant molecules in the membrane followed, by SM ( ≈ 9%) (Dodge and Phillips, 1967; Stefanoni et al., 2020; Himbert et al., 2021). Phosphatidylserine (PS), phosphatidylglycerol (PG), phosphatidylinositol (PI) and phosphatidic acid (PA) lipids account for ≈ 20% of the membrane. Particularly important to the membrane’s biophysical structure and especially its mechanical properties is also the level of saturation of the fatty acids within the membrane. Most fatty acid tails contain between 16 and 18 methylene moieties but tails with as little as 8 and as much as 36 CH2 groups have been detected (Dodge and Phillips, 1967; Stefanoni et al., 2020; Himbert et al., 2021). About 40% of the tails are unsaturated. 21% have one or two double bonds, and 29% have more than three double bonds.
Pathological changes in the RBCcm’s lipidomic have been widely described in the literature. Excellent reviews of RBCcm related diseases can be found in (Gallagher, 2005) and (Narla and Mohandas, 2017). Both, oxidative stress and mechanical stress have been recognized to impair the RBCcm. They lead to lipid oxidation and may trigger phagocytosis and the consequent clearance of damaged RBC. This process is sometimes referred to as eryptosis (Föller et al., 2008) and is characterized by increased intracellular Ca2+, microparticle release and cell shrinkage (Nemkov et al., 2021). Microparticle release is the emission of small vesicles into the blood. Among others, increased microparticle concentration is a key marker for prolonged eryptosis and has been recognized to affect the RBCcm’s composition (Nemkov et al., 2021). Sickle cell anemia is an inherited genetic disease that causes the formation of hemoglobin fibrils resulting in a loss in the cells’ ability to transport oxygen. Abnormal membrane lipid composition in sickle cell RBCs has been observed (Lubin et al., 1981; Franck et al., 1985; Connor et al., 1997), and was correlated with increased intracellular calcium (Eaton et al., 1978), increased binding of hemoglobin (Asakura et al., 1977), enhanced flip-flop of PC and the exposure of PS on the outer leaflet (Franck et al., 1985), and enhanced susceptibility of sickled erythrocytes to lipid peroxidation (Chiu and Lubin, 1979).
Aside from genetic diseases, infectious diseases have also been reported to alter the composition of the RBCcm. A particularly important, RBC targeting parasite, is malaria (Mohandas and An, 2012). Fundamental differences between erythrocytes infected with the different parasite stages were revealed. In mature gametocytes many lipids that decrease in the trophozoite and early gametocyte infected RBCs are regained (Tran et al., 2016). In particular, regulators of membrane fluidity, cholesterol and sphingomyelin, increased significantly during gametocyte maturation (Tran et al., 2016). Neutral lipids increased from 3% of total lipids in uninfected to 27% in stage V gametocyte infected RBCs. PL content decreased during gametocyte development (Tran et al., 2016).
Very recently, effects of the SARS-CoV-2 virus onto the concentrations of circulating fatty acids in the blood plasma were reported (Thomas et al., 2020b). Importantly, elevated levels of polyunsaturated fatty acids (PUFAs) were related to an increased activity of phospholipases A2 which is required for viral replication (Müller et al., 2018; Thomas et al., 2020b). An inhibition of phospholipases A2 activity was suggested to reduce disease severity (Thomas et al., 2020b).
Alterations to the RBCcm’s composition can also be non-pathological. Exercising increases both, shear stress and oxidative stress, but changes of the RBCcm strongly depend on the exercise intensity. Short maximal exercise tests do not show signs of microvesicle release (Nader et al., 2018; Tomschi et al., 2018). Ten kilometer running trials also did not elevate eryptosis markers in endurance-trained athletes (Tripette et al., 2011). However, high-intensity 30 min cycling tests showed an increased generation of microparticles even in well-trained subjects (Nader et al., 2020). Consequently, a decrease in several lipids such as LPC(18:1), PC(36:5), SM(42:2), LPC(18:3), LPC(20:5) were observed (Nemkov et al., 2021). Quite interestingly, these were predominantly lipids with polyunsaturated fatty tails.
Lipid organization within the RBC cytoplasmic membrane
The lipid organization within the RBCcm is largely affected by the distribution of head groups, the degree of saturation of the fatty acid tails and the concentration of sterols, such as cholesterol. The molecules are asymmetrically distributed between the two leaflets (Mohandas and Gallagher, 2008). This assymetry is maintained by flippase (Devaux et al., 2008), floppase, and scramblase (Bevers et al., 1999; Zwaal et al., 2005; Daleke, 2008) proteins. PC and SM lipids are predominantly found in the outer leaflet of the membrane while the majority of PE and PI lipids, as well as, all PS and PG lipids are located on the inner leaflet (Mohandas and Gallagher, 2008). Little is known about the distribution of cholesterol within the RBCcm. It is well established that the molecule can diffuse between both leaflets. Cholesterol flip-flop between the membrane leaflets is an active field of research. Flip-flop rates determined from MD simulations are typically in the microsecond to millisecond time range (Gu et al., 2019; Baral et al., 2020). These rates are typically determined from all-atom simulations that run for tens of μs and by dividing the number of cholesterol molecules that have flipped between the leaflets during that period by the total number of cholesterol molecules in the system. Rates in the order of 10 × 104 s−1 are obtained, at the sub-millisecond time scale, with about 40% of molecules undertaking a flip, and most of the flip-flop events take less than ∼50 ns. Millisecond all-atom simulations can be run but are still scarce in the literature, however, it thus seems that longer simulations will not change these rates.
While several experiments confirm these findings (Steck et al., 2002; Bruckner et al., 2009), recent neutron scattering experiments determined flip-flop rates of tens to hundreds of minutes (Garg et al., 2011). Most of these studies were performed on membranes with a simple composition (less than 5 lipid species). The slower cholesterol flipping rates would explain the higher cholesterol concentration in the cytoplasmic leaflet over that in the extracellular leaflet (Gibson Wood et al., 2011), however, are in contradiction to other studies in the literature. Experiments on multicomponent and more complex membranes, such as the RBCcm, are missing at this point, likely due to experimental challenges. In any case, the flip flop rate was found to be strongly dependent on the membrane’s composition and especially the presence of polyunsaturated lipids substantially increased flip-flop rates. The closest approximation to a RBCcm was reported in a recent study by Baral et al. with an all-atom MD model of a RBCcm mimic, and flip flop rates between 1.6 × 103–1.9 × 104 1/s have been determined (Baral et al., 2020). This is interesting, as it quantifies the highly mobile nature of cholesterol within the RBCcm. At the same time, such a high flip-flop rate suggests a rapid equilibration of the distribution within the membrane. In the absence of any active transport process, one can thus likely assume cholesterol being symmetrically distributed between both leaflets. We note that the apparent contradiction between the flip-flop rates and its biological impact, has yet to be resolved.
The membrane’s asymmetry can be impaired in various conditions. Of particular importance is the exposure of PS on the outer leaflet as it is a strong marker for cell eryptosis (Franck et al., 1985; Nguyen et al., 2011; Vermes et al., 1995; Daleke, 2008). This change in the membrane’s asymmetry is regulated by three mechanisms (Nguyen et al., 2011): 1) Ca2+-stimulated scramblase activation (and flippase inhibition) by lysophosphatidic acid, 4-bromo-A23187, and phorbol-12 myristate-13 acetate; 2) protein kinase C activation by lysophosphatidic acid and phorbol-12 myristate-13 acetate; and 3) enhanced lipid flop caused by lysophosphatidic acid. Such molecular changes are often probed in flow cytometry experiments (Vermes et al., 1995) where the Ca2+ dependent PL-binding protein Annexin V is used to detect exposed external PS.
While these high-throughput measurements allow for an indirect measurement of the molecular composition and structure of the RBCcm, analysis of the organization of the individual components requires high resolution biophysical techniques, such as high resolution microscopy (Mikhalyov and Samsonov, 2011; Ciana et al., 2014) or electron microscopy (Liu et al., 1987). Scattering techniques, and especially X-ray diffraction, have become standard methods to investigate membrane structure on molecular length scales. The first X-ray diffraction studies on human erythrocyte membranes date back to 1970 (Knutton et al., 1970; Stamatoff et al., 1975; McCaughan and Krimm, 1980), where RBC ghosts, i.e., empty RBC vesicles, were prepared using the Dodge protocol (Dodge et al., 1963) and pellets of the final preparation were measured. Lamellar periodicities between ≈ 55 and ≈ 70 Å were observed for hemoglobin free membranes. Large amounts of hemoglobin were reported to result in much larger lamellar periodicities of ≈ 110 Å (Knutton et al., 1970). Early electron density profiles predicted a head-to-head thickness of 44 Å (Stamatoff et al., 1975). However, the low purity and low degree of order in the RBC pellets likely prohibited a more detailed structural analysis at this time.
X-ray diffraction experiments are typically conducted on stacks of RBCcms applied on solid substrates, such as silicon wafers (Nagle and Tristram-Nagle, 2000; Reviakine and Brisson, 2000; Chen et al., 2001; Rheinstädter et al., 2004; Daillant et al., 2005; Tanaka and Sackmann, 2005; Rheinstädter et al., 2006; Zhou et al., 2007; Pabst et al., 2010; Kučerka et al., 2011; Himbert et al., 2017; Himbert et al., 2020; Himbert et al., 2021). The periodic arrangement of the membranes on the chip maximizes the amount of structural information that can be collected, similar to protein crystallography. A typical high-resolution X-ray diffraction scan of stacked RBCcm is shown in Figure 3A. Most scattering signal is specular (q‖ = 0 Å−1) and a series of pronounced lamellar peaks is apparent. These peaks are the results of the periodic stacking of the membranes and the lamellar spacing dz = 2π/q can be determined from the peak position. This lamellar spacing comprises the thickness of a single membrane layer plus the thickness of a surrounding water layer that separates the membranes within the stack. Each lamellar peak represents a measurement of the membrane’s form factor, i.e., the Fourier representation of the membrane’s electron density, at a discrete point in the reciprocal space. This allows calculating the RBCcm’s electron density profile from the specular X-ray scattering through a one-dimensional Fourier analysis, as for instance detailed in (McCaughan and Krimm, 1980; Nagle and Wiener, 1989; Barrett et al., 2012; Himbert et al., 2017; Himbert et al., 2020; Himbert et al., 2021). A typical electron density profile of the RBCcm is shown in Figure 3B. These electron density profiles show a central minima in the bilayer center (z = 0 Å) and two maxima (|z| ≈ 22 Å) which originate from the electron rich phosphate group of PLs and SM. The membrane thickness, dHH, and the thickness of the water layer, dw = dz − dHH, can be determined from the distance of the peaks. Values reported in the literature range from dHH = 39 to 48 Å (Himbert et al., 2017; Himbert et al., 2020; Himbert et al., 2021).
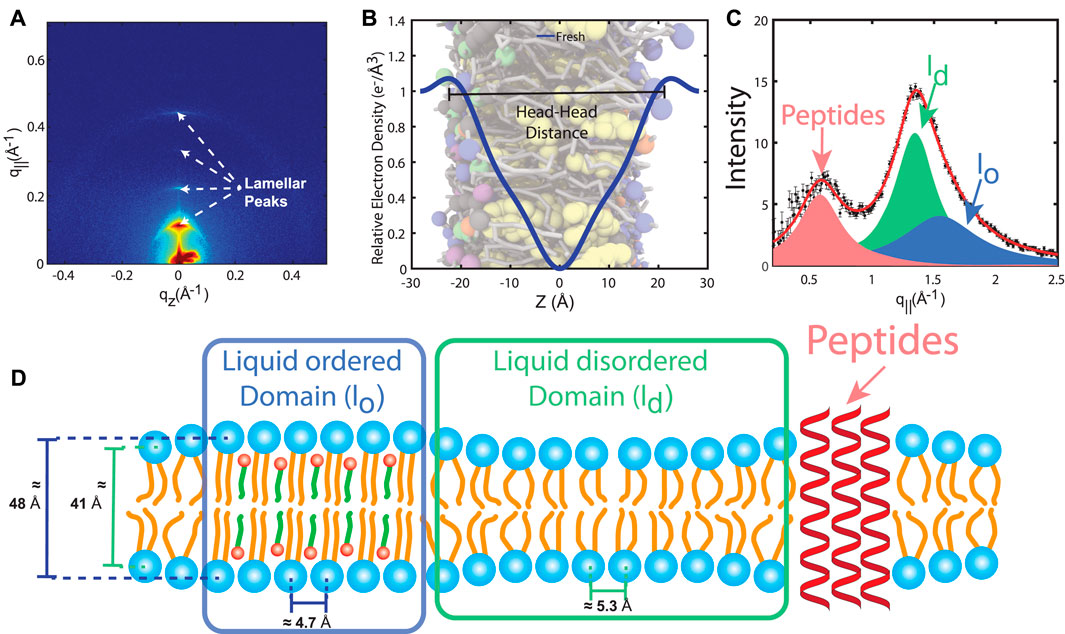
FIGURE 3. (A) Two-dimensional X-ray intensity map recorded on a stack of RBCcm, measured at 37°C and 88% relative humidity. The lamellar peaks are the result of the lamellar stacking of the membranes. (B) Electron density profile reconstructed from the specular peak intensities. The profile is characterized by a global minimum in the bilayer center and two maxima that indicate the location of the electron rich phosphate group of PLs and SMs. (C) In-plane X-ray scan reveals three signals that can be assigned to liquid ordered lo, liquid disordered ld and peptide domains. (D) Schematic of the RBCcm structure, as determined from the high-resolution X-ray diffraction measurements.
While specular X-ray diffraction signal provides structural information along the bilayer normal, in-plane scattering (Figure 3C) allows measuring distances between neighboring lipids, as well as, the area per lipid tail. Three in-plane signals can be observed in RBCcm samples, and can be assigned to two lipid domains which are interpreted as manifestations of liquid ordered lo and liquid disordered ld phases, and α-helical coiled-coil peptide domains (integral proteins), at ratios of 30.2% lo, 45.0% ld and 24.8% coiled peptides (Himbert et al., 2017) (Figure 3D). The lo domains were found to be thicker (dHH = 48 Å), with more densely packed lipid tails (area per lipid tail, AT = 19 Å2) (Himbert et al., 2017). In contrast, the ld domains are significantly thinner (41 Å) with a greater lipid tail area (AT = 25 Å2), typical for a fluid structure (Himbert et al., 2017). The average thickness of the peptide domains of 40.6 Å is compatible with the thickness of the membranes and supports the assignment to integral peptides. The patch sizes of both lipid domains are small, on molecular length scales, about 20 and 30 Å (Himbert et al., 2017). The experimentally determined structure of the RBCcm is sketched in Figure 3D).
The observation of such heterogeneities is interesting as it agrees well with the raft hypothesis (Simons and Ikonen, 1997; Simons and Ikonen, 2000; Lingwood and Simons, 2009; Simons and Gerl, 2010). Cholesterol is preferably located in areas with saturated lipid tails where it straightens the lipid tails and leads to a reduced area per lipid (Pan et al., 2008). These cholesterol-rich patches are referred to as rafts, which are a manifestation of the lo lipid phase (Simons and Gerl, 2010). The rigid cholesterol molecule has indeed been found to form patches with increased lipid tail order within lipid bilayers (Armstrong et al., 2014; Armstrong et al., 2013; Levental et al., 2020; Nickels et al., 2019; Rheinstädter and Mouritsen, 2013a; Toppozini et al., 2014), surrounded by ld domains (Simons and Gerl, 2010). The de-mixing of lipid molecules and the formation of lipid rafts is now well established in synthetic lipid membranes that contain saturated, unsaturated PLs and cholesterol (often referred to as raft-forming mixture) (Kučerka et al., 2010; Rheinstädter and Mouritsen, 2013b; Nickels et al., 2019). Lipid rafts are speculated to be relevant for cell signaling events. Properties and even existence of rafts in biological membranes are, however, a topic of intense debate in the literature (Simons and Gerl, 2010; Rheinstädter and Mouritsen, 2013b; Richard et al., 2016). The reason is that we picture rafts as very small, nanometer sized, and highly dynamic structures, which are very difficult to observe (Armstrong et al., 2014; Toppozini et al., 2014). While rafts were initially pictured as more solid patches floating in a less well ordered, fluid membrane environment, in our current understanding, rafts are the result of temporal and spatial inhomogeneities in the membrane (similar to a microemulsion), which form and dissolve spontaneously through the motion of membrane molecules, which is determined by their properties (such as degree of saturation, head group properties), but also topological properties of the membrane, such as curvature or asymmetry (Meinhardt et al., 2013; Sadeghi et al., 2014; Toppozini et al., 2014; Schick, 2019).
Membrane domains have been reported in the RBCcm. They have been shown to regulate the entry of malarial parasites through mediating the β2-adregenic receptor signaling and increasing cAMP levels (Harrison et al., 2003). Techniques such as detergent-resistant-membranes (DRM) (Ciana et al., 2014) or fluorescent labeling (Mikhalyov and Samsonov, 2011; Ciana et al., 2014), but also atomic force microscopy on whole cells in physiological buffer support the presence of rafts in RBCs (Cai et al., 2012). The size of the reported membrane domains ranged between 100 and 300 nm (Cai et al., 2012). This is well below the resolution limit of optical microscopes, however, significantly larger than the speculated size and dynamic range of rafts (Ciana et al., 2014).
Modern in-silico methods open new possibilities to investigate mixing and demixing processes within the RBCcm. Previous MD simulations on large scale models show a similar de-mixing of lipid species in biological cell membranes. Ingolfsson and co-authors demonstrated that these patches form and disappear on nano to microsecond time scales (Ingólfsson et al., 2014). These fluctuations typically average out on longer length and time scales leading to a uniform membrane structure. The observed small dynamic domains are, therefore, not the result of a static phase separation between both membrane species but the result of nanoscopic molecular fluctuations (Ingólfsson et al., 2014; Levental et al., 2016; Ingólfsson et al., 2017; Marrink et al., 2019; Ingólfsson et al., 2020). We note that typical lipid diffusion constants are in the order of 60 × 10−12 m2/s and the underlying time scale is picoseconds. The lifetime of these patches is therefore about 1,000 times longer than the typical lipid diffusion time scale (Armstrong et al., 2011).
Mechanical properties of the RBC’s cytoplasmic membrane
RBCs are known for their high deformability. The cells are constantly exposed to mechanical stress as they pass through the vascular system. It is remarkable that the cells can pass through constrictions that are much smaller than their own diameter. Considering that RBCs make up ≈ 45% of the blood’s volume, it is little surprising that the cells mechanical properties strongly correlate with blood’s hydrodynamic properties. When discussing the deformation of cells, there are four distinct forms of mechanical stress: out-of-plane compressional forces, bending forces, shear stress and in-plane compression forces. These four deformation modes are sketched in Figure 4.
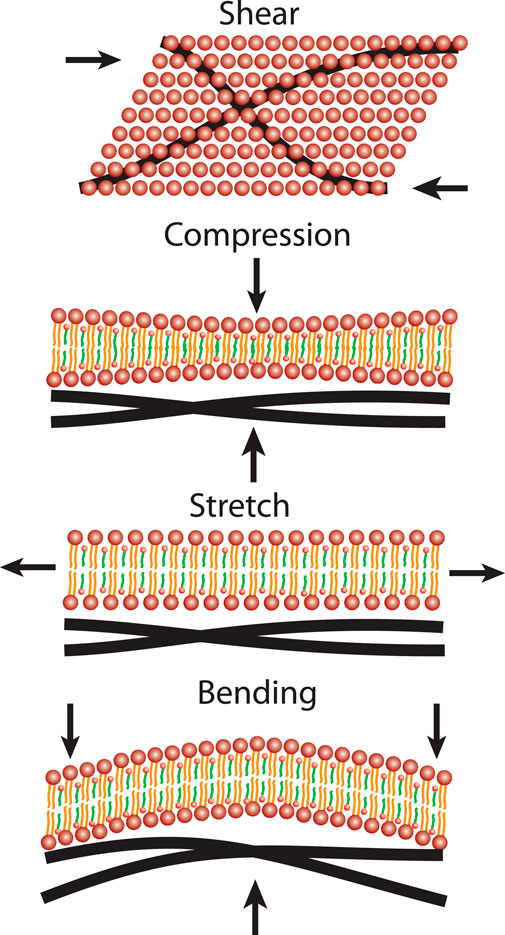
FIGURE 4. Four deformations of the RBC’s outer shell need to be considered for cells in flow: Shear stress, out-of-plane compressional forces, in-plane stretching forces as well as membrane bending.
The unusual deformability of the RBC’s outer shell is the result of softness combined with a resistance against shear stress and stretching. It is inherently related to the shell’s two layer nature. The shear stability is predominently provided by the cytoskeleton. The network can locally be sheared or stretched by the relative displacement of anchor proteins, while the total surface area of the membrane remains constant. The spectrin network is believed to be slightly stretched in the resting biconcave shape and to exert a compression force on the lipid bilayer (Viallat and Abkarian, 2014). The cytoskeleton elastic response displays strain-stiffening effects (Viallat and Abkarian, 2014). At small deformation, its shear modulus is of the order of 2.5 pN/μm (Viallat and Abkarian, 2014). Spectrin typical bond stretching energies are of the order of kBT (Auth et al., 2007) and bond lengths are in the range of 80 nm (Liu et al., 1987). The cytoskeleton’s constant bending modulus is small compared to the typical bending moduli of lipid bilayer membranes (Auth et al., 2007). On the other hand, the RBCcm is primarily composed of a lipid bilayer. As such the RBCcm is characterized by a bending and in-plane stretch rigidity and vanishing shear rigidity. The RBCcm is characterized by a high viscosity (0.1–1 Pa⋅s) (Viallat and Abkarian, 2014).
A simple model of the deformation of lipid bilayers are lipid molecules connected by springs between neighboring molecules, and leaflets that undergo compression and stretching when the membrane is deformed (Phillips et al., 2012). In-plane compression can then be modeled as the compression of springs between the lipid molecules. The corresponding energy cost can be expressed as (Phillips et al., 2012):
where Δa refers to the change of the membrane area relative to the uncompressed area a0. KA is a material property known as the area compression modulus (Phillips et al., 2012). FCompress has the unit of an energy and KA has the unit of force/length. KA can be understood as a measure of the force needed, per dimension, to compress a lipid bilayer by a certain length.
The in-plane compression deforms both leaflets evenly. This changes when a bilayer is bent from its flat state. Bending requires an uneven stretching and compression of springs along the out-of-plane coordinate. This can be understood by springs that are compressed in the lower leaflet, while those in the upper leaflet are stretched. The energy cost resulting from bilayer bending is described by (Phillips et al., 2012):
where u(x, y) describes local spatial deviations of the bilayer center in the out-of-plane direction, κ is the membrane’s bending modulus, and A is the area covered by the membrane. Eq. 2 is the Helfrich-Canham-Evans functional (Phillips et al., 2012). κ is a material property that measures the amount of energy that is needed to bend a membrane. Both KA and κ characterize the membrane’s deformability and several experimental methods have been developed to measure these quantities.
A particularly popular technique is ektacytometry. For this technique, an RBC concentrate is filled into the gap between two concentric transparent cylinders (Figure 5A). A laser beam is guided through the RBC solution and scattered. The diffraction pattern is recorded by a CCD camera outside the outer cylinder and provides a quantitative analysis of the cell’s shape. The cells are then exposed to a shear flow by rotating the inner cylinder and cell elongation is measured from relative changes in the measured diffraction pattern. The elongation allows conclusions on the cell mechanical properties. Importantly, ektacytometry measures an ensemble average and information about individual cells is not recorded. This disadvantage can be overcome by a microfludic approach. Several methods have been employed in the literature such as wedging in tapered constrictions (Gifford et al., 2003) or by measuring the transit time through constrictions by means of pressure change (Abkarian et al., 2006) or electrical impedance (Zheng et al., 2013). An excellent overview over the different methods is presented in a recent review article (Matthews et al., 2022). The method is visualized in Figure 5B. The single-cell elongation in a micro channel with a rectangular cross-section (dimensions between 7 and 15 μm) is visually determined through microscopic techniques. The shape of the RBC depends on the membrane’s deformability and may be quantified through a deformability index (Cluitmans et al., 2014). Recent approaches also enable the in-flow three-dimensional characterization of RBC (Quint et al., 2017) and quantify the observed shapes using machine learning algorithms and demonstrated the importance of the RBC’s deformability for the diagnosis of chorea acanthocytosis/VSP13A disease, McLeod Syndrome or the quality control of stored RBC (Kihm et al., 2018; Recktenwald et al., 2022; Simionato et al., 2021). Another common technique is micropipette aspiration (MA), which can be applied to whole cells and artificially formed liposomes (Figure 5C). A small bulge is formed by sucking a section of the membrane into a micropipette with an opening of a few micrometers. This deformation is then visually inspected under a microscope and both material properties can be determined from a shape analysis of the formed bulge (Evans et al., 1976; Evans, 1983; Evans and Rawicz, 1990; Evans et al., 2008; Dimova, 2014). This approach is taken further by the formation of membrane nanotubes (NT). A nanometer-sized section (diameter ≈ 100 nm) of the membrane is pulled out of a liposome with an optical tweezer and κ can be determined from the applied pulling force (Li et al., 2011; Campillo et al., 2013; Dimova, 2014).
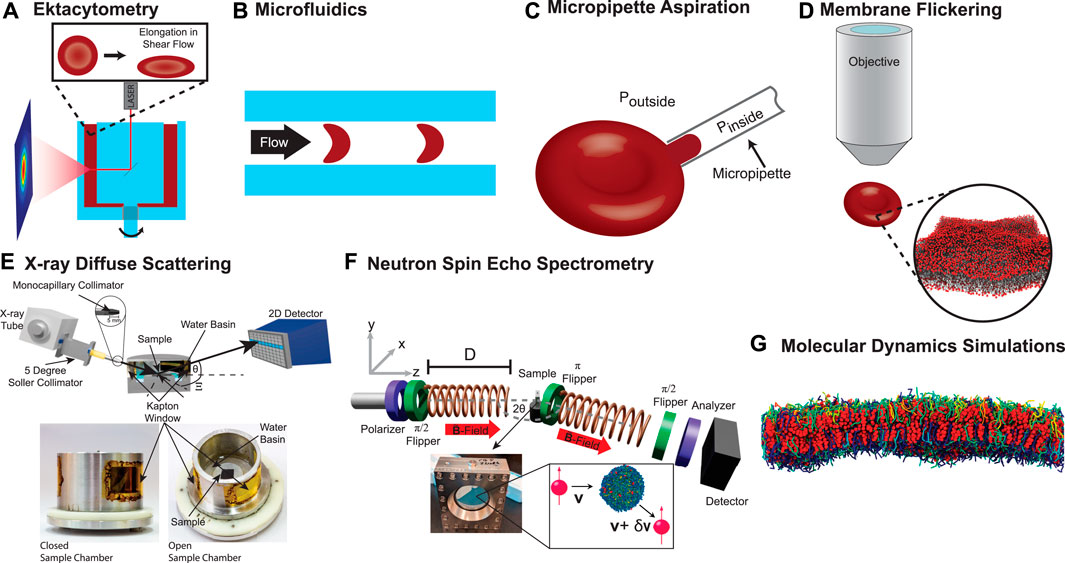
FIGURE 5. RBC’s deformability can be probed in different techniques: (A) Ektacytometry optically probes the elongation of RBC in shear flow. (B) The RBC’s deformability can also be determined by visually inspecting single-cell elongation when the cells pass through microcapillaries of a microfluidic device. (C) In Micropipette aspiration, a small bulge is formed by means of a pressure gradient between the pipette and the surrounding medium. The deformation is then visually inspected under a microscope and material properties can be determined from a shape analysis. (D) Membrane fluctuations can be observed optically and the fluctuation spectrum can be calculated and allows the determination of the membrane’s material characteristics. (E) X-ray diffuse scattering probes the membrane’s bending modulus of solid supported, tension-free RBCcm. Undulating membranes result in off-specular diffuse scattering signal that varies with the membrane’s mechanical properties. (F) Neutron spin echo spectrometry probes the membrane’s dynamical properties by measuring the energy transfer of scattered neutrons. (G) Molecular Dynamics (MD) simulations allow direct access to the molecules trajectories and fluctuation spectra.
The membrane’s elastic properties can also be measured indirectly from a spectral analysis of flickering of cells under a microscope (Brochard and Lennon, 1975; Zilker et al., 1992; Strey et al., 1995), as well as optical interferometric techniques (Popescu et al., 2006; Park et al., 2010b), shown in Figure 5D. Methods that have been employed only recently include X-ray diffuse scattering (XDS), neutron spin echo (NSE) spectrometry and MD simulations. These methods probe the mechanical properties of the RBC’s cytoplasmic membrane on length scales of less than 80 nm, between the pillars of the spectrin network.
In XDS (Kučerka et al., 2006; Tristram-Nagle et al., 2010), elastic properties are determined by diffuse X-ray scattering, which is the result of thermal membrane fluctuations. While (static) structure is typically determined by Bragg scattering, diffuse scattering occurs when molecules move, and scattering occurs at positions away from the resting position. The envelope of diffuse scattering then allows the modeling of dynamical processes. As diffuse scattering is typically orders of magnitude weaker than Bragg scattering, these measurements can only be done at synchrotron X-ray sources or very powerful rotating anode in-house sources. As shown in Figure 5E, solid supported stacks of membranes are used to increase the diffuse signal. In contrast to protein crystallography for instance, membranes must be measured at high temperature (>30°C) and highly hydrated in a humidity chamber (at 99.999% relative humidity). The elastic properties are then determined by fitting the diffuse scattering intensity to a model that calculates the XDS from the membranes’ height-height correlation function. It should be noted that this process is mathematically and computationally intensive and requires high powered GPU supported computers (Himbert, 2021).
These techniques model membrane fluctuations as thermally activated and will deliver incorrect results in the presence of non-equilibrium, activated processes. In this context, ATP has sometimes been thought to affect membrane fluctuations (Betz et al., 2009; Park et al., 2010a), but not always (Evans et al., 2008). Non-thermal noise within experimental error was found at some point from optical tweezer experiments, which could hint to non-thermal sources of membrane motion (Yoon et al., 2011). Turlier et al. (Turlier et al., 2016) more recently reported a violation of the fluctuation–dissipation relation from active and passive microrheology, as a result of the non-equilibrium nature of flickering and the existence of active fluctuations. We note that the experiments discussed below have all been conducted in the explicit absence of ATP such that the origin of the observed fluctuations can be considered as purely thermal.
Relaxations due to membrane undulations on small unilamellar liposomes can be measured directly by inelastic neutron scattering using NSE spectrometry (Rheinstädter et al., 2006; Nagao et al., 2017; Kelley et al., 2020). The corresponding relaxation times are in the order of tens of nanoseconds, with excitation energies in the nano-electron volt range. To measure such a small energy transfers, the velocity of neutrons before and after the interaction with RBC liposomes must be determined with neV precision. To do so, the number of precessions of the neutron spin in a well defined and homogenous magnetic field is “counted” before and behind the sample. A schematic is shown in Figure 5F. With that, the energy transfer between neutron and membrane can be determined as a function of scattering vector, i.e., length scale. The membrane bending rigidity, κ, can then be calculated by measuring the membrane relaxation on different length scales. This technique has in the past been limited to artificial lipid bilayers (Pabst et al., 2010; Bouvrais, 2012; Nagle et al., 2015), as they either require a large volume (a typical NSE sample consists of 20 ml with a membrane mass concentration of ≈ 20 mg/ml). The latest generation of NSE instruments now allows to apply this technique to RBCcm liposomes, which are available in small concentrations, only, and produce smaller scattering signals due to the increased complexity of real biological membranes, as compared to synthetic bilayers (Himbert et al., 2022). Importantly, the membrane composition and especially the presence of cholesterol has to be considered (Nagle, 2021; Nagle et al., 2021) in the analysis.
With the ever-increasing computing power and refined algorithms, MD simulations have become indispensable tools in biomedical research. While experiments are typically done using a large number of molecules (at millimolar concentrations equivalent to
Table 1 lists values for the bending rigidity, κ, and the area compression modulus, KA, for RBCs and RBCcm. Values for κ are reported over a wide range, from 210 kBT down to 2 kBT and the disparate experimental results have been appropriately described as puzzling (Strey et al., 1995; Auth et al., 2007). It was suggested that these apparent controversial results can be explained by the complex interplay between the membrane bilayer and the spectrin network (Strey et al., 1995). Correlation of the magnitude of κ with the length scale of the experiments has been suggested (Lipowsky and Girardet, 1990; Auth et al., 2007), and one would associate a crossover length scale with the 80 nm mesh of the spectrin network. At length scales substantially greater than that, the composite RBC shell is homogeneous and would be characterized by a bending modulus for both the cytoplasmic membrane and the spectrin network.
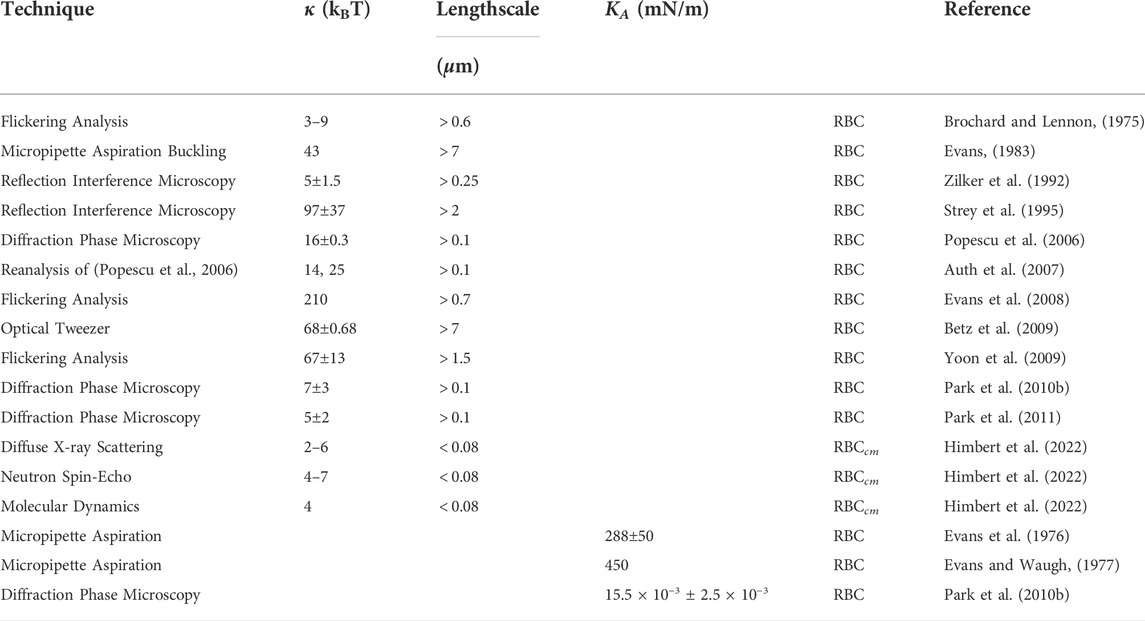
TABLE 1. Summary of values reported for the bending rigidity, κ, and the area compression modulus, KA, of discocytic RBCs and RBCcm. Also included is an estimate of the length scale on which the different techniques work.
Measurements on the length scale of the whole cell, such as buckling in an aspiration pipette experiment (43 kBT) (Evans, 1983) and deformations induced by optical tweezers (κ = 67 kBT (Betz et al., 2009)) would provide these values. For length scales smaller than the crossover length scale, most of the bending would be situated in the cytoplasmic membrane between the ribs of the spectrin network, and κ values from measurements on those length scales would approach those of just the cytoplasmic membrane, which is likely to be homogeneous down to a length scale of 10 nm. However, measurements on molecular length scales do not support the preceding scenario. The smaller values in Table 1 by Brochard et al. [κ = 3–9 kBT (Brochard and Lennon, 1975)], and Park et al. [κ = 7 kBT (Park et al., 2010b)], Zilker et al. [κ = 5 kBT (Zilker et al., 1992)] are from measurements with length scales of the order of the wavelength 400 nm of the optical methods employed. That is not smaller than the spectrin network length scale of 80 nm, so crossover to the value of κ for the cytoplasmic membrane would only be expected to have just begun. Instead, those κ values are much smaller than the values obtained from the largest length scale measurements. At even smaller length scales one would further expect complete crossover to a still smaller value of κ. That value would be just that of the cytoplasmic membrane. Contrarily, values for the cytoplasmic membrane from XDS, NSE and MD are roughly equal to the small values obtained at the optical length scales (Brochard and Lennon, 1975; Zilker et al., 1992; Park et al., 2010b; Park et al., 2011). There is thus strong evidence that there is no length scale dependence in κ. This implies that there is no contribution of the spectrin network to the RBC bending modulus, in agreement with (Gov et al., 2003). The bending modulus of the RBCcm is relatively small, in the range of 4 kBT to 6 kBT. Even though this is a rather large uncertainty range, it is still significant and points to an extreme softness of RBCs on small length scales. The only conclusions that can be drawn by reviewing the existing literature at this point seem to be 1: The bending rigidity of red blood cells is reported over a large range, from 3 kBT to about 100 kBT, which does not depend on the technique 2: The spread in values cannot be explained by a length scale dependence of κ, as techniques which measure on large length scales report small and large values and vice versa 3: Techniques that isolate the RBC cytoplasmic membrane and only measure κ of the RBCcm report very small values, between 4–6 kBT 4: Because of these measurements, the contribution of the spectrin network is likely negligible as there are measurements of entire RBCs which also report such small values.
The nanoscopic regime is most relevant for cellular processes which take place between the ribs of the spectrin network. Especially the passive transport of small molecules is intrinsically related to the membrane’s properties on small length scales (Ghysels et al., 2019; Angles et al., 2021). Of course, RBCs are required to efficiently exchange oxygen and carbon dioxide across the membrane. One may speculate that such permeability is enhanced in a softer membrane, and a standard measure of softness is having a smaller bending modulus. As such, a smaller bending modulus of the RBCcm would generally indicate physiological advantage. While a correlation between the area compressibility modulus and diffusion is obvious as a larger area creates more free volume for particles to move, a correlation between bending rigidity and passive water diffusion has been found (Mathai et al., 2007). However, no experiments regarding membrane stiffness and gas permeability have been reported at this point.
If one accepts a small value of κ for the RBCcm, the question remains if it could be beneficial for biological membranes to be so soft. A possible advantage of a small bending modulus of the cytoplasmic membrane might be that it reduces the energy cost for the process of squeezing the RBC through small capillaries. This hypothesis is based on the possibility that such mechanical processes might require local area changes in the cytoplasmic membrane. Such changes could be slaved to changes in the local area of the spectrin network if the latter changes were required. Even if the spectrin network is rigid with respect to local area changes, changes in its local curvature would necessarily change the local area of the attached cytoplasmic membrane. It is usual to think of the free energy for area change in terms of the area compressibility modulus KA, which is a fairly stiff modulus, typically 250 mN/m. This modulus is associated with area changes per molecule in a flat membrane and the work done to change the molecular packing. However, in the flaccid, low surface tension regime, the membrane has thermally induced undulations that make the cell’s projected area smaller than the local area (Rawicz et al., 2000). Small increases in the tension pulls out these undulations, resulting in an increase in projected area that corresponds to a much smaller apparent KA than the one usually reported. This process is sketched in Figure 6. Indeed, such an apparent KA of only 15 μN/m has been reported for the RBC (Park et al., 2010b). This means that there is a regime of area strain that costs very little energy. How far the area can change in this low cost regime varies nearly inversely with the bending modulus κ. This regime has been measured to extend up to an area increase of about 2% for a lipid bilayer with κ = 10 kBT (Rawicz et al., 2000). The smaller κ of the RBCcm thus increases the low-cost regime and would therefore provide a greater range of mechanical flexibility that may be advantageous for blood flow.
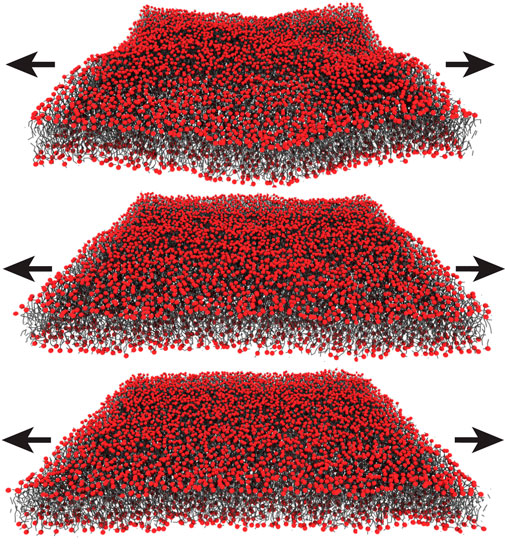
FIGURE 6. The area compressibility modulus of lipid bilayer is typically a stiff modulus between 200 and 300 mN/m. However, much lower values have been also reported for the RBCcm (Park et al., 2010b). This controversy may be explained when considering conformational states of the membrane. A tension free membrane with a low bending rigidity disclose flickering. Wrinkles of the bilayer must be flattened first when stretching the membrane, characterized by a low stretch resistance. In a subsequent regime, the lipid bilayer itself will be stretched which results in a much larger area compressibility modulus KA.
If one accepts the above conclusion that κ of the RBCcm is small, even 10 times smaller than typical bending rigidities of much simpler synthetic membranes, an important point is the role of cholesterol for the mechanical properties of the RBCcm. It is interesting that κ of the RBCcm is so small when it has 50% cholesterol, which is of often found to stiffen bilayers composed of pure lipids while our current belief is that a high concentration of cholesterol leads to stiff membranes. It has been shown that the stiffening effect of cholesterol decreases with increasing unsaturation (Pan et al., 2009) and vanishes already in DOPC and diC22:1PC that have just one unsaturated double bond in each chain. Extrapolation would then suggest that cholesterol might even decrease the bending modulus of membranes with a significant concentration of lipids with multiple double bonds. Cholesterol’s rigid molecular structure contrasts the flexible nature of fatty acyl tails and increases the membrane’s bending modulus in fully saturated model membranes (Pan et al., 2008; Pan et al., 2009). However, little is known about the effect of cholesterol on the bending rigidity of multi-component membranes. Only ≈ 1/3 of the lipids within the RBCcm were found to be fully saturated and experiments on synthetic membranes found a negligible effect of cholesterol on the membrane’s bending rigidity when there are unsaturated molecules present.
However, a particularly interesting observation in this context is the increase in both the bending rigidity in MD simulation for which the polyunsaturated lipids or cholesterol have been removed from a RBCcm mimic (Himbert et al., 2022), respectively. The absence of either of poly-unsaturated lipids or cholesterol made κ increase from 4 kBT to about 13 kBT. This strongly suggests that the softness of the RBCcm can at least partially be explained by the interplay between lipids with higher degrees of tail unsaturation and the stiff cholesterol molecule. While this hypothesis sheds light on some of the confusing results that have been reported in simpler model membranes, it needs to be confirmed in other biological membranes. Cholesterol is an essential component of eucaryotic membranes and makes up to ≈ 50% of the membrane’s content. If it is also true that there is an advantage for biological membranes to be soft at the same time to support membrane diffusion and support cell mechanics, an interaction between unsaturated and cholesterol could provide a missing piece in our current understanding of cell membrane properties.
Conclusion
Modern biophysical approaches can contribute to our understanding of the red blood cell’s cytoplasmic membrane. They picture the RBCcm as patchy, with nanometer sized liquid ordered and disordered lipid and peptide domains. The membrane is at the same time surprisingly soft, with bending rigidities κ of 2–6 kBT, only. This extreme softness is the result of an interaction between poly unsaturated lipids and cholesterol, and in strong contrast to the current belief that a high concentration of cholesterol in general results in stiff membranes. As permeability is typically enhanced in a softer membrane, a smaller bending modulus of the RBCcm would generally indicate a physiological advantage. A small bending rigidity can also reduce the energy for the process of squeezing the RBC through small capillaries by providing a low-cost mechanism, where small increases in the tension pull out membrane undulations, resulting in an increase in projected membrane area without actually stretching the RBCcm (which is more costly in terms of energy). By reviewing the current literature, we present convincing evidence that there is no length scale dependence in κ of whole RBCs. This also implies that there is no contribution of the spectrin network to the RBC bending modulus.
Author contributions
All authors listed have made a substantial, direct, and intellectual contribution to the work and approved it for publication.
Conflict of interest
The authors declare that the research was conducted in the absence of any commercial or financial relationships that could be construed as a potential conflict of interest.
The handling editor declared a past co-authorship with the authors SH and MR.
Publisher’s note
All claims expressed in this article are solely those of the authors and do not necessarily represent those of their affiliated organizations, or those of the publisher, the editors and the reviewers. Any product that may be evaluated in this article, or claim that may be made by its manufacturer, is not guaranteed or endorsed by the publisher.
References
Abkarian M., Faivre M., Stone H. A. (2006). High-speed microfluidic differential manometer for cellular-scale hydrodynamics. Proc. Natl. Acad. Sci. U. S. A. 103, 538–542. doi:10.1073/pnas.0507171102
Aimon S., Callan-Jones A., Berthaud A., Pinot M., Toombes G. E., Bassereau P. (2014). Membrane shape modulates transmembrane protein distribution. Dev. Cell 28, 212–218. doi:10.1016/j.devcel.2013.12.012
Angles G., Hail A., Dotson R. J., Pias S. C. (2021). Atomistic simulations modify interpretation of spin-label oximetry data. part 1: Intensified water–lipid interfacial resistances. Appl. Magn. Reson. 52, 1261–1289. doi:10.1007/s00723-021-01398-z
Armstrong C. L., Häußler W., Seydel T., Katsaras J., Rheinstädter M. C. (2014). Nanosecond lipid dynamics in membranes containing cholesterol. Soft Matter 10, 2600–2611. doi:10.1039/c3sm51757h
Armstrong C. L., Marquardt D., Dies H., Kučerka N., Yamani Z., Harroun T. A., et al. (2013). The observation of highly ordered domains in membranes with cholesterol. PLOS ONE 8, e66162. doi:10.1371/journal.pone.0066162
Armstrong C. L., Trapp M., Peters J., Seydel T., Rheinstädter M. C. (2011). Short range ballistic motion in fluid lipid bilayers studied by quasi-elastic neutron scattering. Soft Matter 7, 8358–8362. doi:10.1039/c1sm05691c
Asakura T., Minakata K., Adachi K., Russell M. O., Schwartz E. (1977). Denatured hemoglobin in sickle erythrocytes. J. Clin. Invest. 59 (4), 633–640. doi:10.1172/JCI108681
Auth T., Safran S., Gov N. S. (2007). Fluctuations of coupled fluid and solid membranes with application to red blood cells. Phys. Rev. E Stat. Nonlin. Soft Matter Phys. 76, 051910. doi:10.1103/PhysRevE.76.051910
Baral S., Levental I., Lyman E. (2020). Composition dependence of cholesterol flip-flop rates in physiological mixtures. Chem. Phys. Lipids 232, 104967. doi:10.1016/j.chemphyslip.2020.104967
Barrett M. A., Zheng S., Roshankar G., Alsop R. J., Belanger R. K., Huynh C., et al. (2012). Interaction of aspirin (acetylsalicylic acid) with lipid membranes. PLoS ONE 7, e34357. doi:10.1371/journal.pone.0034357
Betz T., Lenz M., Joanny J.-F., Sykes C. (2009). Atp-dependent mechanics of red blood cells. Proc. Natl. Acad. Sci. U. S. A. 106, 15320–15325. doi:10.1073/pnas.0904614106
Bevers E. M., Comfurius P., Dekkers D. W., Zwaal R. F. (1999). Lipid translocation across the plasma membrane of mammalian cells. Biochim. Biophys. Acta 1439, 317–330. doi:10.1016/s1388-1981(99)00110-9
Bouvrais H. (2012). Bending rigidities of lipid bilayers: Their determination and main inputs in biophysical studies. Adv. Planar Lipid Bilayers Liposomes 15, 1–75. doi:10.1016/B978-0-12-396533-2.00006-9
Brochard F., Lennon J. (1975). Frequency spectrum of the flicker phenomenon in erythrocytes. J. Phys. Fr. 36, 1035–1047. doi:10.1051/jphys:0197500360110103500
Bruckner R., Mansy S., Ricardo A., Mahadevan L., Szostak J. (2009). Flip-flop-induced relaxation of bending energy: Implications for membrane remodeling. Biophys. J. 97, 3113–3122. doi:10.1016/j.bpj.2009.09.025
Cai M., Zhao W., Shang X., Jiang J., Ji H., Tang Z., et al. (2012). Direct evidence of lipid rafts by in situ atomic force microscopy. Small 8, 1243–1250. doi:10.1002/smll.201102183
Campillo C., Sens P., Köster D., Pontani L.-L., Lévy D., Bassereau P., et al. (2013). Unexpected membrane dynamics unveiled by membrane nanotube extrusion. Biophys. J. 104, 1248–1256. doi:10.1016/j.bpj.2013.01.051
Chen S., Liao C., Huang H., Weiss T., Bellisent-Funel M., Sette F. (2001). Collective dynamics in fully hydrated phospholipid bilayers studied by inelastic x-ray scattering. Phys. Rev. Lett. 86, 740–743. doi:10.1103/PhysRevLett.86.740
Chiu D., Lubin B. (1979). Abnormal vitamin e and glutathione peroxidase levels in sickle cell anemia: Evidence for increased susceptibility to lipid peroxidation in vivo. J. Lab. Clin. Med. 94, 542–548.
Ciana A., Achilli C., Minetti G. (2014). Membrane rafts of the human red blood cell. Mol. Membr. Biol. 31, 47–57. doi:10.3109/09687688.2014.896485
Cluitmans J. C., Chokkalingam V., Janssen A. M., Brock R., Huck W. T., Bosman G. J. (2014). Alterations in red blood cell deformability during storage: A microfluidic approach. Biomed. Res. Int. 2014, 764268. doi:10.1155/2014/764268
Connor W. E., Lin D. S., Thomas G., Ey F., DeLoughery T., Zhu N. (1997). Abnormal phospholipid molecular species of erythrocytes in sickle cell anemia. J. Lipid Res. 38, 2516–2528. doi:10.1016/s0022-2275(20)30036-5
Daillant J., Bellet-Amalric E., Braslau A., Charitat T., Fragneto G., Graner F., et al. (2005). Structure and fluctuations of a single floating lipid bilayer. Proc. Natl. Acad. Sci. U. S. A. 102, 11639–11644. doi:10.1073/pnas.0504588102
Daleke D. L. (2008). Regulation of phospholipid asymmetry in the erythrocyte membrane. Curr. Opin. Hematol. 15, 191–195. doi:10.1097/MOH.0b013e3282f97af7
D’Alessandro A., Fu X., Kanias T., Reisz J. A., Culp-Hill R., Guo Y., et al. (2021). Donor sex, age and ethnicity impact stored red blood cell antioxidant metabolism through mechanisms in part explained by glucose 6-phosphate dehydrogenase levels and activity. Haematologica 106, 1290–1302. doi:10.3324/haematol.2020.246603
Devaux P. F., Herrmann A., Ohlwein N., Kozlov M. M. (2008). How lipid flippases can modulate membrane structure. Biochim. Biophys. Acta 1778, 1591–1600. doi:10.1016/j.bbamem.2008.03.007
Dimova R. (2014). Recent developments in the field of bending rigidity measurements on membranes. Adv. Colloid Interface Sci. 208, 225–234. doi:10.1016/j.cis.2014.03.003
Discher D., Mohandas N., Evans E. (1994). Molecular maps of red cell deformation: Hidden elasticity and in situ connectivity. Science 266, 1032–1035. doi:10.1126/science.7973655
Dodge J. T., Mitchell C., Hanahan D. J. (1963). The preparation and chemical characteristics of hemoglobin-free ghosts of human erythrocytes. Arch. Biochem. Biophys. 100, 119–130. doi:10.1016/0003-9861(63)90042-0
Dodge J. T., Phillips G. B. (1967). Composition of phospholipids and of phospholipid fatty acids and aldehydes in human red cells. J. Lipid Res. 8, 667–675. doi:10.1016/s0022-2275(20)38890-8
Dresbach M. (1904). Elliptical human red corpuscles. Science 19, 469–470. doi:10.1126/science.19.481.469
Eaton J. W., Berger E., White J. G., Jacob H. S. (1978). Calcium-induced damage of haemoglobin ss and normal erythrocytes. Br. J. Haematol. 38, 57–62. doi:10.1111/j.1365-2141.1978.tb07108.x
Evans E. A. (1983). Bending elastic modulus of red blood cell membrane derived from buckling instability in micropipet aspiration tests. Biophys. J. 43, 27–30. doi:10.1016/S0006-3495(83)84319-7
Evans E. A., Waugh R., Melnik L. (1976). Elastic area compressibility modulus of red cell membrane. Biophys. J. 16, 585–595. doi:10.1016/S0006-3495(76)85713-X
Evans E., Rawicz W. (1990). Entropy-driven tension and bending elasticity in condensed-fluid membranes. Phys. Rev. Lett. 64, 2094–2097. doi:10.1103/PhysRevLett.64.2094
Evans E., Waugh R. (1977). Osmotic correction to elastic area compressibility measurements on red cell membrane. Biophys. J. 20, 307–313. doi:10.1016/S0006-3495(77)85551-3
Evans J., Gratzer W., Mohandas N., Parker K., Sleep J. (2008). Fluctuations of the red blood cell membrane: Relation to mechanical properties and lack of atp dependence. Biophys. J. 94, 4134–4144. doi:10.1529/biophysj.107.117952
Föller M., Huber S. M., Lang F. (2008). Erythrocyte programmed cell death. IUBMB life 60, 661–668. doi:10.1002/iub.106
Fowler P. W., Hélie J., Duncan A., Chavent M., Koldsø H., Sansom M. S. (2016). Membrane stiffness is modified by integral membrane proteins. Soft Matter 12, 7792–7803. doi:10.1039/c6sm01186a
Franck P., Bevers E., Lubin B., Comfurius P., Chiu D., den Kamp J. O., et al. (1985). Uncoupling of the membrane skeleton from the lipid bilayer. the cause of accelerated phospholipid flip-flop leading to an enhanced procoagulant activity of sickled cells. J. Clin. Invest. 75, 183–190. doi:10.1172/JCI111672
Gallagher P. G. (2005). Red cell membrane disorders. Hematol. Am. Soc. Hematol. Educ. Program 2005, 13–18. doi:10.1182/asheducation-2005.1.13
Garg S., Porcar L., Woodka A., Butler P., Perez-Salas U. (2011). Noninvasive neutron scattering measurements reveal slower cholesterol transport in model lipid membranes. Biophys. J. 101, 370–377. doi:10.1016/j.bpj.2011.06.014
Ghysels A., Krämer A., Venable R. M., Teague W. E., Lyman E., Gawrisch K., et al. (2019). Permeability of membranes in the liquid ordered and liquid disordered phases. Nat. Commun. 10, 5616. doi:10.1038/s41467-019-13432-7
Gibson Wood W., Igbavboa U., Müller W. E., Eckert G. P. (2011). Cholesterol asymmetry in synaptic plasma membranes. J. Neurochem. 116, 684–689. doi:10.1111/j.1471-4159.2010.07017.x
Gifford S. C., Frank M. G., Derganc J., Gabel C., Austin R. H., Yoshida T., et al. (2003). Parallel microchannel-based measurements of individual erythrocyte areas and volumes. Biophys. J. 84, 623–633. doi:10.1016/S0006-3495(03)74882-6
Gov N., Zilman A., Safran S. (2003). Cytoskeleton confinement and tension of red blood cell membranes. Phys. Rev. Lett. 90, 228101. doi:10.1103/PhysRevLett.90.228101
Gu R.-X., Baoukina S., Tieleman D. P. (2019). Cholesterol flip-flop in heterogeneous membranes. J. Chem. Theory Comput. 15, 2064–2070. doi:10.1021/acs.jctc.8b00933
Harrison T., Samuel B. U., Akompong T., Hamm H., Mohandas N., Lomasney J. W., et al. (2003). Erythrocyte g protein-coupled receptor signaling in malarial infection. Science 301, 1734–1736. doi:10.1126/science.1089324
Herrick J. B. (1910). Peculiar elongated and sickle-shaped red blood corpuscles in a case of severe anemia. Arch. Intern. Med. 6, 517–521. doi:10.1001/archinte.1910.00050330050003
Himbert S., Alsop R. J., Rose M., Hertz L., Dhaliwal A., Moran-Mirabal J. M., et al. (2017). The molecular structure of human red blood cell membranes from highly oriented, solid supported multi-lamellar membranes. Sci. Rep. 7, 39661. doi:10.1038/srep39661
Himbert S. (2021). Biophysics of blood membranes. Ph.D. thesis. Hamilton, Canada: McMaster University.
Himbert S., Blacker M. J., Kihm A., Pauli Q., Khondker A., Yang K., et al. (2020). Hybrid erythrocyte liposomes: Functionalized red blood cell membranes for molecule encapsulation. Adv. Biosyst. 4, 1900185. doi:10.1002/adbi.201900185
Himbert S., D’Alessandro A., Qadri S. M., Majcher M. J., Hoare T., Sheffield W. P., et al. (2022). The bending rigidity of the red blood cell cytoplasmic membrane. PLOS ONE 17, e0269619. doi:10.1371/journal.pone.0269619
Himbert S., Qadri S. M., Sheffield W. P., Schubert P., D’Alessandro A., Rheinstädter M. C. (2021). Blood bank storage of red blood cells increases RBC cytoplasmic membrane order and bending rigidity. PLOS ONE 16, e0259267. doi:10.1371/journal.pone.0259267
Ingólfsson H. I., Bhatia H., Zeppelin T., Bennett W. D., Carpenter K. A., Hsu P.-C., et al. (2020). Capturing biologically complex tissue-specific membranes at different levels of compositional complexity. J. Phys. Chem. B 124, 7819–7829. doi:10.1021/acs.jpcb.0c03368
Ingólfsson H. I., Carpenter T. S., Bhatia H., Bremer P.-T., Marrink S. J., Lightstone F. C. (2017). Computational lipidomics of the neuronal plasma membrane. Biophys. J. 113, 2271–2280. doi:10.1016/j.bpj.2017.10.017
Ingólfsson H. I., Melo M. N., Van Eerden F. J., Arnarez C., Lopez C. A., Wassenaar T. A., et al. (2014). Lipid organization of the plasma membrane. J. Am. Chem. Soc. 136, 14554–14559. doi:10.1021/ja507832e
Kelley E. G., Butler P. D., Ashkar R., Bradbury R., Nagao M. (2020). Scaling relationships for the elastic moduli and viscosity of mixed lipid membranes. Proc. Natl. Acad. Sci. U. S. A. 117, 23365–23373. doi:10.1073/pnas.2008789117
Kihm A., Kaestner L., Wagner C., Quint S. (2018). Classification of red blood cell shapes in flow using outlier tolerant machine learning. PLoS Comput. Biol. 14, e1006278. doi:10.1371/journal.pcbi.1006278
Knutton S., Finean J., Coleman R., Limbrick A. (1970). Low-angle x-ray diffraction and electron-microscope studies of isolated erythrocyte membranes. J. Cell Sci. 7, 357–371. doi:10.1242/jcs.7.2.357
Kučerka N., Marquardt D., Harroun T., Nieh M.-P., Wassall S., de Jong D., et al. (2010). Cholesterol in bilayers with PUFA chains: Doping with DMPC or POPC results in sterol reorientation and membrane-domain formation. Biochemistry 49, 7485–7493. doi:10.1021/bi100891z
Kučerka N., Nieh M.-P., Katsaras J. (2011). Fluid phase lipid areas and bilayer thicknesses of commonly used phosphatidylcholines as a function of temperature. Biochim. Biophys. Acta 1808, 2761–2771. doi:10.1016/j.bbamem.2011.07.022
Kučerka N., Tristram-Nagle S., Nagle J. F. (2006). Structure of fully hydrated fluid phase lipid bilayers with monounsaturated chains. J. Membr. Biol. 208, 193–202. doi:10.1007/s00232-005-7006-8
Levental I., Levental K. R., Heberle F. A. (2020). Lipid rafts: Controversies resolved, mysteries remain. Trends Cell Biol. 30, 341–353. doi:10.1016/j.tcb.2020.01.009
Levental K. R., Lorent J. H., Lin X., Skinkle A. D., Surma M. A., Stockenbojer E. A., et al. (2016). Polyunsaturated lipids regulate membrane domain stability by tuning membrane order. Biophys. J. 110, 1800–1810. doi:10.1016/j.bpj.2016.03.012
Li Y., Lipowsky R., Dimova R. (2011). Membrane nanotubes induced by aqueous phase separation and stabilized by spontaneous curvature. Proc. Natl. Acad. Sci. U. S. A. 108, 4731–4736. doi:10.1073/pnas.1015892108
Lingwood D., Simons K. (2009). Lipid rafts as a membrane-organizing principle. Science 327, 46–50. doi:10.1126/science.1174621
Lipowsky R., Girardet M. (1990). Shape fluctuations of polymerized or solidlike membranes. Phys. Rev. Lett. 65, 2893–2896. doi:10.1103/PhysRevLett.65.2893
Liu S.-C., Derick L. H., Palek J. (1987). Visualization of the hexagonal lattice in the erythrocyte membrane skeleton. J. Cell Biol. 104, 527–536. doi:10.1083/jcb.104.3.527
Lubin B., Chiu D., Bastacky J., Roelofsen B., Van Deenen L. (1981). Abnormalities in membrane phospholipid organization in sickled erythrocytes. J. Clin. Invest. 67 (6), 1643–1649. doi:10.1172/jci110200
Marrink S. J., Corradi V., Souza P. C., Ingolfsson H. I., Tieleman D. P., Sansom M. S. (2019). Computational modeling of realistic cell membranes. Chem. Rev. 119, 6184–6226. doi:10.1021/acs.chemrev.8b00460
Mathai J. C., Tristram-Nagle S., Nagle J. F., Zeidel M. L. (2007). Structural determinants of water permeability through the lipid membrane. J. Gen. Physiol. 131, 69–76. doi:10.1085/jgp.200709848
Matthews K., Lamoureux E. S., Myrand-Lapierre M.-E., Duffy S. P., Ma H. (2022). Technologies for measuring red blood cell deformability. Lab. Chip 22, 1254–1274. doi:10.1039/d1lc01058a
McCaughan L., Krimm S. (1980). X-ray and neutron scattering density profiles of the intact human red blood cell membrane. Science 207, 1481–1483. doi:10.1126/science.7361101
Meinhardt S., Vink R. L. C., Schmid F. (2013). Monolayer curvature stabilizes nanoscale raft domains in mixed lipid bilayers. Proc. Natl. Acad. Sci. U. S. A. 110, 4476–4481. doi:10.1073/pnas.1221075110
Mikhalyov I., Samsonov A. (2011). Lipid raft detecting in membranes of live erythrocytes. Biochim. Biophys. Acta–1939 1808, 1930–1939. doi:10.1016/j.bbamem.2011.04.002
Mohandas N., An X. (2012). Malaria and human red blood cells. Med. Microbiol. Immunol. 201, 593–598. doi:10.1007/s00430-012-0272-z
Mohandas N., Gallagher P. G. (2008). Red cell membrane: Past, present, and future. Blood 112, 3939–3948. doi:10.1182/blood-2008-07-161166
Müller C., Hardt M., Schwudke D., Neuman B. W., Pleschka S., Ziebuhr J. (2018). Inhibition of cytosolic phospholipase a2α impairs an early step of coronavirus replication in cell culture. J. Virol. 92, e01463–17. doi:10.1128/JVI.01463-17
Nader E., Guillot N., Lavorel L., Hancco I., Fort R., Stauffer E., et al. (2018). Eryptosis and hemorheological responses to maximal exercise in athletes: Comparison between running and cycling. Scand. J. Med. Sci. Sports 28, 1532–1540. doi:10.1111/sms.13059
Nader E., Monedero D., Robert M., Skinner S., Stauffer E., Cibiel A., et al. (2020). Impact of a 10 km running trial on eryptosis, red blood cell rheology, and electrophysiology in endurance trained athletes: A pilot study. Eur. J. Appl. Physiol. 120, 255–266. doi:10.1007/s00421-019-04271-x
Nagao M., Kelley E. G., Ashkar R., Bradbury R., Butler P. D. (2017). Probing elastic and viscous properties of phospholipid bilayers using neutron spin echo spectroscopy. J. Phys. Chem. Lett. 8, 4679–4684. doi:10.1021/acs.jpclett.7b01830
Nagle J. F., Evans E. A., Bassereau P., Baumgart T., Tristram-Nagle S., Dimova R. (2021). A needless but interesting controversy. Proc. Natl. Acad. Sci. U. S. A. 118, e2025011118. doi:10.1073/pnas.2025011118
Nagle J. F., Jablin M. S., Tristram-Nagle S., Akabori K. (2015). What are the true values of the bending modulus of simple lipid bilayers? Chem. Phys. Lipids 185, 3–10. doi:10.1016/j.chemphyslip.2014.04.003
Nagle J. F. (2021). Measuring the bending modulus of lipid bilayers with cholesterol. Phys. Rev. E 104, 044405. doi:10.1103/PhysRevE.104.044405
Nagle J. F., Tristram-Nagle S. (2000). Structure of lipid bilayers. Biochim. Biophys. Acta 1469, 159–195. doi:10.1016/s0304-4157(00)00016-2
Nagle J. F., Wiener M. C. (1989). Relations for lipid bilayers. Connection of electron density profiles to other structural quantities. Biophys. J. 55, 309–313. doi:10.1016/S0006-3495(89)82806-1
Narla J., Mohandas N. (2017). Red cell membrane disorders. Int. J. Lab. Hematol. 39, 47–52. doi:10.1111/ijlh.12657
Nemkov T., Skinner S. C., Nader E., Stefanoni D., Robert M., Cendali F., et al. (2021). Acute cycling exercise induces changes in red blood cell deformability and membrane lipid remodeling. Int. J. Mol. Sci. 22, 896. doi:10.3390/ijms22020896
Netz R. R., Pincus P. (1995). Inhomogeneous fluid membranes: Segregation, ordering, and effective rigidity. Phys. Rev. E Stat. Phys. Plasmas Fluids Relat. Interdiscip. Top. 52, 4114–4128. doi:10.1103/physreve.52.4114
Nguyen D. B., Wagner-Britz L., Maia S., Steffen P., Wagner C., Kaestner L., et al. (2011). Regulation of phosphatidylserine exposure in red blood cells. Cell. Physiol. biochem. 28, 847–856. doi:10.1159/000335798
Nickels J. D., Smith M. D., Alsop R. J., Himbert S., Yahya A., Cordner D., et al. (2019). Lipid rafts: Buffers of cell membrane physical properties. J. Phys. Chem. B 123, 2050–2056. doi:10.1021/acs.jpcb.8b12126
Pabst G., Kučerka N., Nieh M.-P., Rheinstädter M., Katsaras J. (2010). Applications of neutron and x-ray scattering to the study of biologically relevant model membranes. Chem. Phys. Lipids 163, 460–479. doi:10.1016/j.chemphyslip.2010.03.010
Palek J. (1985). Hereditary elliptocytosis and related disorders. Clin. Haematol. 14, 45–87. doi:10.1016/s0308-2261(21)00462-8
Pan J., Mills T. T., Tristram-Nagle S., Nagle J. F. (2008). Cholesterol perturbs lipid bilayers nonuniversally. Phys. Rev. Lett. 100, 198103. doi:10.1103/PhysRevLett.100.198103
Pan J., Tristram-Nagle S., Nagle J. F. (2009). Effect of cholesterol on structural and mechanical properties of membranes depends on lipid chain saturation. Phys. Rev. E Stat. Nonlin. Soft Matter Phys. 80, 021931. doi:10.1103/PhysRevE.80.021931
Park Y., Best C. A., Auth T., Gov N. S., Safran S. A., Popescu G., et al. (2010). Metabolic remodeling of the human red blood cell membrane. Proc. Natl. Acad. Sci. U. S. A. 107, 1289–1294. doi:10.1073/pnas.0910785107
Park Y., Best C. A., Badizadegan K., Dasari R. R., Feld M. S., Kuriabova T., et al. (2010). Measurement of red blood cell mechanics during morphological changes. Proc. Natl. Acad. Sci. U. S. A. 107, 6731–6736. doi:10.1073/pnas.0909533107
Park Y., Best C. A., Kuriabova T., Henle M. L., Feld M. S., Levine A. J., et al. (2011). Measurement of the nonlinear elasticity of red blood cell membranes. Phys. Rev. E Stat. Nonlin. Soft Matter Phys. 83, 051925. doi:10.1103/PhysRevE.83.051925
Perrotta S., Gallagher P. G., Mohandas N. (2008). Hereditary spherocytosis. Lancet 372, 1411–1426. doi:10.1016/S0140-6736(08)61588-3
Phillips R., Kondev J., Theriot J., Garcia H. G., Orme N. (2012). Physical biology of the cell. New York, US: Garland Science.
Popescu G., Ikeda T., Goda K., Best-Popescu C. A., Laposata M., Manley S., et al. (2006). Optical measurement of cell membrane tension. Phys. Rev. Lett. 97, 218101. doi:10.1103/PhysRevLett.97.218101
Quint S., Christ A., Guckenberger A., Himbert S., Kaestner L., Gekle S., et al. (2017). 3d tomography of cells in micro-channels. Appl. Phys. Lett. 111, 103701. doi:10.1063/1.4986392
Rangamani P., Mandadap K. K., Oster G. (2014). Protein-induced membrane curvature alters local membrane tension. Biophys. J. 107, 751–762. doi:10.1016/j.bpj.2014.06.010
Rawicz W., Olbrich K. C., McIntosh T., Needham D., Evans E. (2000). Effect of chain length and unsaturation on elasticity of lipid bilayers. Biophys. J. 79, 328–339. doi:10.1016/S0006-3495(00)76295-3
Recktenwald S. M., Lopes M. G., Peter S., Hof S., Simionato G., Peikert K., et al. (2022). Erysense, a lab-on-a-chip-based point-of-care device to evaluate red blood cell flow properties with multiple clinical applications. Front. Physiol. 13, 884690. doi:10.3389/fphys.2022.884690
Rees D. C., Williams T. N., Gladwin M. T. (2010). Sickle-cell disease. Lancet 376, 2018–2031. doi:10.1016/S0140-6736(10)61029-X
Reisz J. A., Zheng C., D’Alessandro A., Nemkov T. (2019). Untargeted and semi-targeted lipid analysis of biological samples using mass spectrometry-based metabolomics. Methods Mol. Biol. 1978, 121–135. doi:10.1007/978-1-4939-9236-2_8
Reviakine I., Brisson A. (2000). Formation of supported phospholipid bilayers from unilamellar vesicles investigated by atomic force microscopy. Langmuir 16, 1806–1815. doi:10.1021/la9903043
Rheinstädter M. C., Häussler W., Salditt T. (2006). Dispersion relation of lipid membrane shape fluctuations by neutron spin-echo spectrometry. Phys. Rev. Lett. 97, 048103. doi:10.1103/PhysRevLett.97.048103
Rheinstädter M. C., Mouritsen O. G. (2013). Small-scale structure in fluid cholesterol–lipid bilayers. Curr. Opin. Colloid & Interface Sci. 18, 440–447. doi:10.1016/j.cocis.2013.07.001
Rheinstädter M. C., Mouritsen O. G. (2013). Small-scale structure in fluid cholesterol–lipid bilayers. Curr. Opin. Colloid & Interface Sci. 18, 440–447. doi:10.1016/j.cocis.2013.07.001
Rheinstädter M. C., Ollinger C., Fragneto G., Demmel F., Salditt T. (2004). Collective dynamics of lipid membranes studied by inelastic neutron scattering. Phys. Rev. Lett. 93, 108107. doi:10.1103/PhysRevLett.93.108107
Richard J., Alsop M. C. R. (2016). “Lipid rafts in binary lipid/cholesterol bilayers,” in Membrane organization and lipid rafts in the cell and artificial membranes. Editor A. Catalá (Hauppauge, New York, US: Nova Science Publisher), 17–42.
Sadeghi S., Müller M., Vink R. L. (2014). Raft formation in lipid bilayers coupled to curvature. Biophys. J. 107, 1591–1600. doi:10.1016/j.bpj.2014.07.072
Schick M. (2019). 17 on the origin of “rafts”: The plasma membrane as a microemulsion. Charact. Biol. Membr. 499–514. doi:10.1515/9783110544657-017
Sens P., Gov N. (2007). Force balance and membrane shedding at the red-blood-cell surface. Phys. Rev. Lett. 98, 018102. doi:10.1103/PhysRevLett.98.018102
Simionato G., Hinkelmann K., Chachanidze R., Bianchi P., Fermo E., van Wijk R., et al. (2021). Red blood cell phenotyping from 3D confocal images using artificial neural networks. PLoS Computational Biology 17 (5), e1008934.
Simons K., Gerl M. J. (2010). Revitalizing membrane rafts: New tools and insights. Nat. Rev. Mol. Cell Biol. 11, 688–699. doi:10.1038/nrm2977
Simons K., Ikonen E. (1997). Functional rafts in cell membranes. Nature 387, 569–572. doi:10.1038/42408
Simons K., Ikonen E. (2000). How cells handle cholesterol. Science 290, 1721–1726. doi:10.1126/science.290.5497.1721
Singer S., Nicolson G. (1972). The fluid mosaic model of the structure of cell membranes. Science 175, 720–731. doi:10.1126/science.175.4023.720
Stamatoff J. B., Krimm S., Harvie N. R. (1975). X-ray diffraction studies of human erythrocyte membrane structure. Proc. Natl. Acad. Sci. U. S. A. 72, 531–534. doi:10.1073/pnas.72.2.531
Steck T. L., Ye J., Lange Y. (2002). Probing red cell membrane cholesterol movement with cyclodextrin. Biophys. J. 83, 2118–2125. doi:10.1016/S0006-3495(02)73972-6
Stefanoni D., Shin H. K. H., Baek J. H., Champagne D. P., Nemkov T., Thomas T., et al. (2020). Red blood cell metabolism in rhesus macaques and humans: Comparative biology of blood storage. Haematologica 105, 2174–2186. doi:10.3324/haematol.2019.229930
Strey H., Peterson M., Sackmann E. (1995). Measurement of erythrocyte membrane elasticity by flicker eigenmode decomposition. Biophys. J. 69, 478–488. doi:10.1016/S0006-3495(95)79921-0
Tanaka M., Sackmann E. (2005). Polymer-supported membranes as models of the cell surface. Nature 437, 656–663. doi:10.1038/nature04164
Thomas T., Stefanoni D., Dzieciatkowska M., Issaian A., Nemkov T., Hill R. C., et al. (2020). Evidence of structural protein damage and membrane lipid remodeling in red blood cells from Covid-19 patients. J. Proteome Res. 19, 4455–4469. doi:10.1021/acs.jproteome.0c00606
Thomas T., Stefanoni D., Reisz J. A., Nemkov T., Bertolone L., Francis R. O., et al. (2020). Covid-19 infection alters kynurenine and fatty acid metabolism, correlating with il-6 levels and renal status. JCI insight 5, 140327. doi:10.1172/jci.insight.140327
Tomschi F., Bizjak D., Bloch W., Latsch J., Predel H. G., Grau M. (2018). Deformability of different red blood cell populations and viscosity of differently trained young men in response to intensive and moderate running. Clin. Hemorheol. Microcirc. 69, 503–514. doi:10.3233/CH-189202
Toppozini L., Meinhardt S., Armstrong C. L., Yamani Z., Kučerka N., Schmid F., et al. (2014). Structure of cholesterol in lipid rafts. Phys. Rev. Lett. 113, 228101. doi:10.1103/PhysRevLett.113.228101
Tran P. N., Brown S. H., Rug M., Ridgway M. C., Mitchell T. W., Maier A. G. (2016). Changes in lipid composition during sexual development of the malaria parasite plasmodium falciparum. Malar. J. 15, 73–13. doi:10.1186/s12936-016-1130-z
Tripette J., Hardy-Dessources M.-D., Beltan E., Sanouiller A., Bangou J., Chalabi T., et al. (2011). Endurance running trial in tropical environment: A blood rheological study. Clin. Hemorheol. Microcirc. 47, 261–268. doi:10.3233/CH-2011-1388
Tristram-Nagle S., Kim D. J., Akhunzada N., Kučerka N., Mathai J. C., Katsaras J., et al. (2010). Structure and water permeability of fully hydrated diphytanoylpc. Chem. Phys. Lipids 163, 630–637. doi:10.1016/j.chemphyslip.2010.04.011
Turlier H., Fedosov D. A., Audoly B., Auth T., Gov N. S., Sykes C., et al. (2016). Equilibrium physics breakdown reveals the active nature of red blood cell flickering. Nat. Phys. 12, 513–519. doi:10.1038/nphys3621
Vermes I., Haanen C., Steffens-Nakken H., Reutellingsperger C. (1995). A novel assay for apoptosis flow cytometric detection of phosphatidylserine expression on early apoptotic cells using fluorescein labelled annexin v. J. Immunol. Methods 184, 39–51. doi:10.1016/0022-1759(95)00072-i
Viallat A., Abkarian M. (2014). Red blood cell: From its mechanics to its motion in shear flow. Int. J. Lab. Hematol. 36, 237–243. doi:10.1111/ijlh.12233
Yoon Y.-Z., Hong H., Brown A., Kim D. C., Kang D. J., Lew V. L., et al. (2009). Flickering analysis of erythrocyte mechanical properties: Dependence on oxygenation level, cell shape, and hydration level. Biophys. J. 97, 1606–1615. doi:10.1016/j.bpj.2009.06.028
Yoon Y. Z., Kotar J., Brown A. T., Cicuta P. (2011). Red blood cell dynamics: From spontaneous fluctuations to non-linear response. Soft Matter 7, 2042–2051. doi:10.1039/c0sm01117g
Zheng Y., Nguyen J., Wang C., Sun Y. (2013). Electrical measurement of red blood cell deformability on a microfluidic device. Lab. Chip 13, 3275–3283. doi:10.1039/c3lc50427a
Zhou X., Moran-Mirabal J. M., Craighead H. G., McEuen P. L. (2007). Supported lipid bilayer/carbon nanotube hybrids. Nat. Nanotechnol. 2, 185–190. doi:10.1038/nnano.2007.34
Zilker A., Ziegler M., Sackmann E. (1992). Spectral analysis of erythrocyte flickering in the 0.3–4-μm−1 regime by microinterferometry combined with fast image processing. Phys. Rev. A 46, 7998–8001. doi:10.1103/physreva.46.7998
Keywords: red blood cells, RBCs, RBC membrane, RBC biophysics, RBC mechanical properties, RBC membrane structure, RBC cytoplasmic membrane
Citation: Himbert S and Rheinstädter MC (2022) Structural and mechanical properties of the red blood cell’s cytoplasmic membrane seen through the lens of biophysics. Front. Physiol. 13:953257. doi: 10.3389/fphys.2022.953257
Received: 26 May 2022; Accepted: 15 August 2022;
Published: 12 September 2022.
Edited by:
Angelo D'Alessandro, University of Colorado Denver, United StatesReviewed by:
Stephan Quint, Universität des Saarlandes, GermanyDmitry A. Fedosov, Julich Research Center (HZ), Germany
Copyright © 2022 Himbert and Rheinstädter. This is an open-access article distributed under the terms of the Creative Commons Attribution License (CC BY). The use, distribution or reproduction in other forums is permitted, provided the original author(s) and the copyright owner(s) are credited and that the original publication in this journal is cited, in accordance with accepted academic practice. No use, distribution or reproduction is permitted which does not comply with these terms.
*Correspondence: Sebastian Himbert, aGltYmVydHNAbWNtYXN0ZXIuY2E=; Maikel C. Rheinstädter, cmhlaW5zdGFkdGVyQG1jbWFzdGVyLmNh