Characterization of the evolutionary and virological aspects of mutations in the receptor binding motif of the SARS-CoV-2 spike protein
- 1Division of Systems Virology, Department of Microbiology and Immunology, The Institute of Medical Science, The University of Tokyo, Tokyo, Japan
- 2Division of Molecular Virology and Genetics, Joint Research Center for Human Retrovirus Infection, Kumamoto University, Kumamoto, Japan
- 3Department of Clinical Pathology, Faculty of Medicine, Suez Canal University, Ismailia, Egypt
- 4First Medical Faculty at Biocev, Charles University, Vestec, Czechia
- 5Department of Biomolecular Sciences, Weizmann Institute of Science, Rehovot, Israel
- 6Department of Veterinary Science, Faculty of Agriculture, University of Miyazaki, Miyazaki, Japan
- 7Department of Hematology and Oncology, Kyoto University, Kyoto, Japan
- 8Center for Animal Disease Control, University of Miyazaki, Miyazaki, Japan
- 9Graduate School of Medicine and Veterinary Medicine, University of Miyazaki, Miyazaki, Japan
- 10Graduate School of Medicine, The University of Tokyo, Tokyo, Japan
- 11International Research Center for Infectious Diseases, The Institute of Medical Science, The University of Tokyo, Tokyo, Japan
- 12Core Research for Evolutionary Science and Technology (CREST), Japan Science and Technology Agency, Kawaguchi, Japan
- 13Laboratory of Virus Control, Research Institute for Microbial Diseases, Osaka University, Suita, Japan
- 14International Vaccine Design Center, The Institute of Medical Science, The University of Tokyo, Tokyo, Japan
- 15Collaboration Unit for Infection, Joint Research Center for Human Retrovirus Infection, Kumamoto University, Kumamoto, Japan
Severe acute respiratory syndrome coronavirus 2 (SARS-CoV-2) has substantially diversified during the pandemic, resulting in the successive emergence of variants characterized by various mutations. It has been observed that several epidemic variants, including those classified as variants of concern, share mutations at four key residues (L452R, T478K, E484K, and N501Y) within the receptor binding motif (RBM) region of the spike protein. However, the processes through which these four specific RBM mutations were acquired during the evolution of SARS-CoV-2, as well as the degree to which they enhance viral fitness, remain unclear. Moreover, the effect of these mutations on the properties of the spike protein is not yet fully understood. In this study, we performed a comprehensive phylogenetic analysis and showed that the four RBM mutations have been convergently acquired across various lineages throughout the evolutionary history of SARS-CoV-2. We also found a specific pattern in the order of acquisition for some of these mutations. Additionally, our epidemic dynamic modeling demonstrated that acquiring these mutations leads to an increase in the effective reproduction number of the virus. Furthermore, we engineered mutant spike proteins with all feasible combinations of the four mutations, and examined their properties to uncover the influence that these mutations have on viral characteristics. Our results provide insights into the roles these four mutations play in shaping the viral characteristics, epidemic proliferation, and evolutionary pathway of SARS-CoV-2.
Introduction
Severe acute respiratory syndrome coronavirus 2 (SARS-CoV-2) was first identified in China at the end of 2019 and subsequently spread worldwide, leading to a pandemic (1). Subsequently, various SARS-CoV-2 variants that acquired mutations and altered viral properties such as transmissibility, immune evasion capability, and pathogenicity have emerged one after another. Particularly, variants of concern (VOCs), such as the Alpha, Beta, Delta, and Omicron variants, have caused severe epidemic surges around the world (2).
SARS-CoV-2 uses angiotensin-converting enzyme 2 (ACE2) as a viral receptor. When the viral spike (S) protein binds to ACE2, the viral envelope membrane fuses with the cellular membrane, resulting in viral entry into the cell. The protein domain of the S protein essential for interaction with ACE2 is called the receptor binding domain (RBD; residues 305–534 in the S protein of the reference strain of Wuhan-Hu-1), and the part specifically critical for binding to ACE2 is called the receptor binding motif (RBM; residues 437–508 in the S protein of Wuhan-Hu-1) (3). Importantly, RBD and RBM are also critical targets for neutralizing antibodies, which inhibit the binding of the S protein to ACE2 and block viral infection to cells (4). Therefore, mutations in RBD and RBM have crucial effects on binding affinity to ACE2 and infectivity, as well as escape ability from neutralizing antibodies, both of which are strongly associated with viral fitness (2).
By examining the connection between viral mutations and phenotypic alterations, we can clarify why certain epidemic variants have proliferated rapidly, and further forecast potential epidemic variants based on distinct mutation patterns. The evolution of the SARS-CoV-2 S protein appears to be following a pattern where the S protein enhances its ability to evade the host’s humoral immunity while preserving its capacity to bind to ACE2 (5, 6). Since the RBD or RBM of the S protein plays a vital role in ACE2 binding and escaping humoral immunity, critical mutations are often observed in these regions (3). Mutations at four key sites in RBM (L452, T478, E484, and N501) are observed in various epidemic variants, including VOCs. Particularly, L452R, T478K, E484K, and N501Y have been observed in multiple lineages. For instance, L452R is in Delta and Omicron BA.5; T478K is in Omicron and Delta; E484K is in Beta and Gamma; and N501Y is in Alpha, Beta, Gamma, and Omicron (2, 3, 7).
Previous studies have revealed that these four individual mutations in RBM enhance functions of the S protein that are tied to viral fitness, such as infectivity, ACE2 binding ability, and evasion from neutralizing antibodies (4, 8–14). These findings imply that the mutations may contribute to the observed increase in viral fitness. However, the extent of the contribution of these mutations to the increased viral fitness remains unclear. Also, the pattern in which these mutations were acquired during SARS-CoV-2 evolution is not thoroughly understood. Furthermore, while the impacts of singular mutations on the function of the S proteins have been studied, the effects of two or more combined mutations have not been explored in depth. Here, we carried out phylogenetic and epidemic dynamics analyses using viral genome surveillance data to investigate how these mutations have facilitated the spread of the virus. We also engineered mutant S proteins with all possible combinations of the (L452R, T478K, E484K, and N501Y; 16 mutants, including the wild type) to investigate their properties, and thereby unravel the effects that these mutations exert on viral characteristics.
Methods
Ethics statement
All protocols involving specimens from human subjects recruited at Kyoto University were reviewed and approved by the Institutional Review Board of Kyoto University (approval ID: G1309). All human subjects provided written informed consent. All protocols for the use of human specimens were reviewed and approved by the Institutional Review Boards of The Institute of Medical Science, The University of Tokyo (approval IDs: 2021-1-0416 and 2021-18-0617), Kyoto University (approval ID: G0697) and Kumamoto University (approval IDs: 2066 and 2074).
Epidemiological data
In this investigation, we utilized genomic epidemiological survey information, as obtained from GISAID (https://www.gisaid.org/) (15). The epidemiological survey information was procured on March 23, 2022, and incorporates information relating to the virus, such as location, patient, and mutation information. It is important to note that we solely concentrated on human hosts in our research.
Moreover, we also utilized two kinds of phylogenetic information available in GISAID. The first one is a worldwide phylogenetic tree created by Audacity (https://www.epicov.org/epi3/frontend#4579b6). The data were developed utilizing all high-quality sequences enlisted in GISAID. Newly enrolled sequences were included in the current tree utilizing maximum parsimony. This data was downloaded on March 17th, 2022. The second data set depicts the phylogeny of the PANGO lineage, which has been arranged by grouping together similar lineages. We obtained this data from CoVizu (16) on January 19th, 2022.
Ancestral state reconstruction
To investigate how 4 mutations in RBM (S:L452R, T478K, E484K, N501Y) have been acquired in the SARS-CoV-2 phylogeny, we counted how many times the mutations are acquired by estimating whether each node has mutations with ancestral state reconstruction. Ancestral state reconstruction was performed by maximum parsimony with phylogenetic data from Audacity and epidemiological survey data from GISAID. We defined the mutation acquisition node as the node which is estimated to have a mutation and whose parental node is estimated not to have the mutation. This analysis was performed using the asr_max_parsimony function provided in the castor (17) package in R v.2.0.3.
Analysis of mutation coexistence
Based on the result of the ancestral state reconstruction, we tested whether some of the 4 mutations tend to coexist (i.e. if the probability of obtaining mutation B changes based on the presence or absence of mutation A). We classified the acquisition events of the 4 mutations into 4 groups generated by a 2 × 2 contingency table based on whether 1) it was after acquiring mutation A and 2) whether it was a mutation B acquisition. We tested the association between the two terms by Fisher’s exact test.
Modeling the dynamics of SARS-CoV-2 lineage
To compare the viral spread rate in the human population of SARS-CoV-2 subgroups, we estimated the relative effective reproduction number of each viral lineage according to the lineage dynamics calculated on the basis of viral genomic surveillance data.
We compared the relative effective reproduction number among viruses with and without the mutation at position E484 in the Alpha variant. The surveillance data contains Alpha variant which has N501Y mutation registered from March 12th,2020 to March 14th, 2022. Data collected at quarantine and sequences with higher than 20 percent undefined bases in their genome were eliminated. The data regions were limited to where the amount of Alpha variant sequences harboring a mutation at E484 is more than 50 per mutation. The data for each region were classified according to the type of amino acid mutation at E484 or the absence of mutation at E484, and time series data were generated by counting the number of observed sequences per fixed number of days (bin size=7 days) for each of the taxonomic groups (cluster). For the estimation of the relative effective reproduction number of each cluster, we constructed a Bayesian multinomial logistic model as described in our previous study (18). The model is:
in which b0, b1, μt, θt and Yt are vectors with K elements, and the k-th element in the vector represents the value for viral cluster k. The explanatory variable is time bin t, and the outcome variable Yt represents the counts of the respective viral clusters at time t. In the model, the linear estimator μt, consisting of the intercept b0 and the slope b1 for t, is converted to the simplex θt, which represents the probability of occurrence of each viral cluster, by the softmax link function. Yt is generated from θt, and Nt, which represents the total count of all lineages at t, according to a multinomial distribution. According to the previous study, the relative effective reproduction number of each cluster (r, a vector with K elements) was calculated according to the slope parameter b1 in the model above with the assumption of a fixed generation time. Γ is the average viral generation time (5 days) and ω is the time bin size (7 days). For the parameter estimation, the intercept and slope parameters of the Alpha variant with no mutation at E484 were fixed at 0. Consequently, the relative effective reproduction number of the Alpha variant with no mutation at E484 was fixed at 1, and those of the respective lineages were estimated relative to them.
Parameter estimation was performed by the framework of Bayesian statistical inference with Markov chain Monte Carlo (MCMC) methods implemented in CmdStan v.2.92.2 (https://mc-stan.org) with cmdstanr v.0.5.2 (https://mc-stan.org/cmdstanr/). Noninformative priors were set for all parameters. 4 independent MCMC chains were run with 1,000 steps of each warmup and sampling iteration. In the MCMC runs, the target average acceptance probability was set at 0.99, and the maximum tree depth parameter was set at 20. We confirmed that all estimated parameters had<1.01 convergence diagnostic and more than 400 effective sampling size values (ESS), indicating that the MCMC runs were successfully convergent. The analyses above were performed in R v.4.1.0 (https://www.r-project.org/).
Measuring the effect of mutations on viral transmissibility
To investigate whether the acquisition of the 4 mutations in RBM (L452R, T478K, E484K, N501Y) alters the transmissibility of the virus, we used a Bayesian hierarchical multinomial logistic model, established in our previous study (19). In this model, the relative Re and the effect of mutations on the Re are estimated based on the virus dynamics and the mutation patterns. We used the surveillance data registered in GISAID collected from March 12th,2020 to March 14th, 2022. The data elimination was conducted in the same condition as the former analysis. The data were categorized by the profile of the 500 most frequent mutations, and a group of viral sequences with the same mutation profiles was designated as the mutant haplotype cluster. Mutations with a correlation coefficient of the observed mutation pattern higher than 0.90 were grouped together as one mutation group. Time series data were generated by counting the number of observed sequences per fixed number of days (bin size=7 days) for each mutant haplotype cluster. For the estimation of the relative effective reproduction number of each cluster, we constructed a Bayesian hierarchical multinomial logistic model. The model is:
in which b0, b1, μt, θt and Yt are vectors with K elements, and the k-th element in the vector represents the value for viral cluster k. The explanatory variable is time bin t, and the outcome variable Yt represents the counts of the respective viral clusters at time t. The linear estimator μt, consisting of the intercept b0 and the slope b1 for t, is converted to the simplex θt, which represents the probability of occurrence of each viral cluster, by the softmax link function. Yt is generated from θt, and Nt, which represents the total count of all lineages at t, according to a multinomial distribution. According to the previous study, the relative effective reproduction number of each cluster (r, a vector with K elements) was calculated according to the slope parameter b1 in the model above with the assumption of a fixed generation time. Γ is the average viral generation time (5 days) and ω is the time bin size (3 days). Here we further expressed the slope b1 as the sum of the effects of the mutations of the cluster. The b1 is assumed to be a Student’s t distribution with 4 degrees of freedom, mean Aw, and standard deviation σ. A is the profile matrix of mutations (K×D matrix) of each mutation haplotype cluster, and w is the effect size (vector of length D) of each mutation/mutation group. D is the total number of mutations/mutation groups. Thus, Aw represents the linear combination of the effects of each mutation. As a prior distribution of w, we set a Laplace distribution with mean 0 and standard deviation 1. As a prior distribution of σ, we set a semi-stochastic t-distribution with 4 degrees of freedom, mean 0 and standard deviation 1. Noninformative priors were set for the other parameters.
Parameter estimation was performed by the framework of Bayesian statistical inference with Markov chain Monte Carlo (MCMC) methods implemented in CmdStan v.2.92.2 (https://mc-stan.org) with cmdstanr v.0.5.2 (https://mc-stan.org/cmdstanr/). Four independent MCMC chains were run with 500 and 1,000 steps of warmup and sampling iteration, respectively. In the MCMC runs, the target average acceptance probability was set at 0.99, and the maximum tree depth exceeded was set at 15. We confirmed that all estimated parameters had<1.01 value).
Cell culture
The Lenti-X 293T cell line (Takara, Cat# 632180), HEK293 cells (a human embryonic kidney cell line; ATCC, CRL-1573), HEK293T cells (a human embryonic kidney cell line; ATCC, CRL-3216) and HOS-ACE2/TMPRSS2 cells (kindly provided by Dr. Kenzo Tokunaga), a derivative of HOS cells (a human osteosarcoma cell line; ATCC CRL-1543) stably expressing human ACE2 and TMPRSS2 (20, 21), were maintained in Dulbecco’s modified Eagle’s medium (DMEM) (high glucose) (Wako, Cat# 044-29765) containing 10% fetal bovine serum (Sigma-Aldrich Cat# 172012-500ML), 100 units penicillin and 100 ug/ml streptomycin (P/S) (Sigma-Aldrich, Cat# P4333-100ML). Calu-3/DSP1-7 cells (Calu-3 cells stably expressing DSP1-7) (22) were maintained in EMEM (Wako, Cat# 055-08975) containing 20% FBS and P/S.
Plasmid construction
Plasmids expressing the mutant spike proteins harboring all combinations of the four RBM mutations (L452R, T478K, E484K, and N501Y) were generated by site-directed overlap extension PCR using specific combinations of the primers listed in Table S5. As the PCR template, the plasmid expressing the codon-optimized SARS-CoV-2 S protein of B.1.1 (the parental D614G-bearing variant) [PMID: 33558493] was used. The resulting PCR fragment was subcloned into the KpnI-NotI site of the pCAGGS vector (23) using In-Fusion® HD Cloning Kit (Takara, Cat# Z9650N). Nucleotide sequences were determined by DNA sequencing services (Eurofins), and the sequence data were analyzed by Sequencher v5.1 software (Gene Codes Corporation).
Yeast surface display
The peptidase domain of ACE2 (Q18 – S740) was generated in Expi293F cells (ThermoFisher). The plasmid pCAGGS-ACE2 was transfected into the cells using the ExpiFectamine 293 Transfection Kit (ThermoFisher), following the manufacturer’s protocol. After 72 hours of expression post-transfection, the supernatant was harvested through centrifugation (1500 rpm, 15 minutes, 4°C), underwent sterile filtration (0.45 µm Nalgene Rapid-Flow™), and was then applied to a 5 ml HisTrap Fast Flow column (Cytiva (GE, USA), cat. 17-5255-01) connected to an ÄKTA pure FPLC system (Cytiva, USA). The column was subsequently rinsed with 5 column volumes (CV) of a 25 mM Tris, 200 mM NaCl, 20 mM imidazole pH 7.2 buffer. Subsequently, the ACE2 protein was eluted with a 2 CV gradient using the same buffer with a gradient ranging from 20 to 1000 nM imidazole. The protein was dialyzed in PBS and concentrated using Amicon® concentrators (3K MWCO, Merck Millipore Ltd, cat. UFC900324).
Plasmids carrying either WT or mutated RBDs (L452R, T478K, E484K, N501Y, or their combinations) were introduced into electrocompetent EBY100 Saccharomyces cerevisiae as described in the paper by Benatuil et al. (DOI: 10.1093/protein/gzq002) via electroporation. The transformed cells were then plated on selective SD-Trp agar plates and incubated for 48 hours at 30°C. Subsequently, the colonies were inoculated into 1.0 ml of liquid SD-CAA media and cultured overnight at 30°C with agitation at 220 rpm. Following the overnight culture, the cells were subjected to centrifugation (3000 g, 3 minutes), and the supernatant was discarded. The cell pellets were utilized to inoculate an expression culture in which they were suspended in a mixture of 1/9 media and 1 nM DMSO-solubilized bilirubin to OD 1. This culture was maintained at 20°C with agitation at 220 rpm overnight. The expressed cells were then washed with ice-cold PBSB buffer (PBS with 1 g/L BSA) and resuspended in the same buffer. Cell aliquots (100 ul) were incubated in PBSB buffer containing a range of CF®640R succinimidyl ester-labeled ACE2 concentrations at 4°C. The timing and volume of the incubation solutions were adjusted to attain equilibrium and minimize ligand depletion effects. After incubation, the cells were washed twice with ice-cold PBSB buffer. The fluorescence characteristics of a minimum of 30,000 cells were analyzed using a BD Accuri™ C6 Flow Cytometer (BD Biosciences, USA) and the C6 Plus Analysis Software. The mean CF640 fluorescence signals of the RBD+ cell population were subtracted from the fluorescence of the RBD- population, and the data were fitted to the non-cooperative Hill equation using nonlinear least-squares regression in Python 3.7. The fitting also incorporated the total concentration of yeast-exposed protein, along with two additional parameters that described the titration curve.
Pseudovirus infection
Pseudoviruses were prepared as previously described (24). Briefly, lentivirus (HIV-1)-based, luciferase-expressing reporter viruses were pseudotyped with the SARS-CoV-2 S protein. One prior day of transfection, the LentiX-293T cells were seeded at a density of 5 × 105 cells per well in a 6-well plate. The cells were cotransfected with 800 ng psPAX2-IN/HiBiT (a packaging plasmid encoding the HiBiT-tag-fused integrase) (21), 800 ng pWPI-Luc2 (a reporter plasmid encoding a firefly luciferase gene) (21), and 400 ng plasmids expressing parental S protein or its derivatives using TransIT-293 transfection reagent (Mirus, Cat# MIR2704) according to the manufacturer’s protocol. Two days post transfection, the culture supernatants were harvested and filtrated. The amount of produced pseudovirus particles was quantified by the HiBiT assay using the Nano Glo HiBiT lytic detection system (Promega, Cat# N3040) as previously described (21).
To measure viral infectivity, the same amount of pseudovirus normalized with the HIV-1 p24 capsid protein was inoculated into HOS-ACE2/TMPRSS2 cells. At two days postinfection, the infected cells were lysed with a britelite plus (PerkinElmer, Cat#6066769), and the luminescent signal produced by firefly luciferase reaction was measured using a GloMax explorer multimode microplate reader 3500 (Promega). The pseudoviruses were stored at –80°C until use.
Neutralization assay
Neutralization assays were performed as previously described (24). The SARS-CoV-2 S pseudoviruses (counting ~50,000 relative light units) were incubated with serially diluted (40-fold to 29,160-fold dilution at the final concentration) heat-inactivated sera at 37°C for 1 hour. Pseudoviruses without sera were included as controls. Then, a 20 μl mixture of pseudovirus and serum was added to HOS-ACE2/TMPRSS2 cells (10,000 cells/100 μl) in a 96-well white plate. Two days post infection, the infected cells were lysed with a Bright-Glo luciferase assay system (Promega, Cat# E2620), and the luminescent signal was measured using a CentroXS3 LB960 (Berthold Technologies). The assays were performed in triplicate, and the 50% neutralization titer (NT50) was calculated using Prism 9 (GraphPad Software). The details of the convalescent sera are summarized in Table S6.
SARS-CoV-2 S-based fusion assay
A SARS-CoV-2 S-based fusion assay with dual split protein (DSP) encoding Renilla luciferase (RL) and GFP genes was performed as previously described (25, 26). On day 1, effector cells (i.e., S-expressing) and target cells (Calu-3/DSP1-7 cells) were prepared at a density of 0.6-0.8 × 106 cells/well in a 6 well plate. On day 2, to prepare effector cells, HEK293 cells were cotransfected with the S expression plasmids (400 ng) and pDSP8-11 (27) (400 ng) using TransIT-LT1 (Takara, Cat# MIR2306). On day 3 (24 hours post transfection), effector cells were detached by pipetting and 16,000 effector cells/well were reseeded into a 96-well black plate (PerKinElmer, Cat# 6005225), and target cells were reseeded at a density of 1 × 106 cells/2 ml/well in a 6-well plate. On day 4 (48 hours post transfection), the target cells were incubated with Enduren live cell substrate (Pomega, Cat# E6481) for 3 hours and then detached, and 32,000 target cells/well were added to a 96 well plate with effector cells. RL activity was measured at the indicated time points using a Centro XS3 LB960 (Berthhold Technologies). To measure the surface expression level of the S protein, effector cells were stained with rabbit anti-SARS-CoV-2 S S1/S2 polyclonal antibody (Thermo Fisher Scientific, Cat#PA5-112048, 1:100 dilution). Normal rabbit IgG (SouthernBiotech, Cat# 0111-01, 1:100) was used as a negative control, and APC-conjugated goat anti-rabbit IgG polyclonal antibody (Jackson immunoresearch, Cat# 111-136-144, 1:50) was used as a secondary antibody. The expression level of the surface S proteins was detected by FACS Canto II (BD Biosciences) and analyzed by FlowJo software v10.7.1 (BD Biosciences). RL activity was normalized to the mean fluorescence intensity (MFI) of surface S proteins, and the normalized values are shown as fusion activity.
Statistical analysis for the experimental data
To compare the values of the results of each experiment, the value of log2 fold change was determined. The value of the S without the mutation was used as a reference. For the result of the resistance to neutralizing antibodies, the average of the two values obtained with Pfizer and Moderna vaccine sera was used.
To investigate the effect of mutation on the function of the virus, we performed multiple regression with mutations as variables. The regression used the lm function from the stat library v.4.0.3 on R v.4.0.3. To obtain the effect of the mutation alone and the effect of the combination of mutations respectively, we built two models without interaction terms and a model with first-order interaction terms.
Results
Prevalence of the four RBM mutations
We first traced the prevalence of mutations at the four aforementioned amino acid positions in the RBM (452, 478, 484, and 501) in the GISAID data (15) (https://gisaid.org/) during the period from December 2019 to March 2022 (Figures 1A, B). The L452R mutation, for instance, was observed with the spread of the Delta variant, while the two separate peaks of the N501Y mutation were associated with first the Alpha and then the Omicron variant epidemics (Figure 1B).
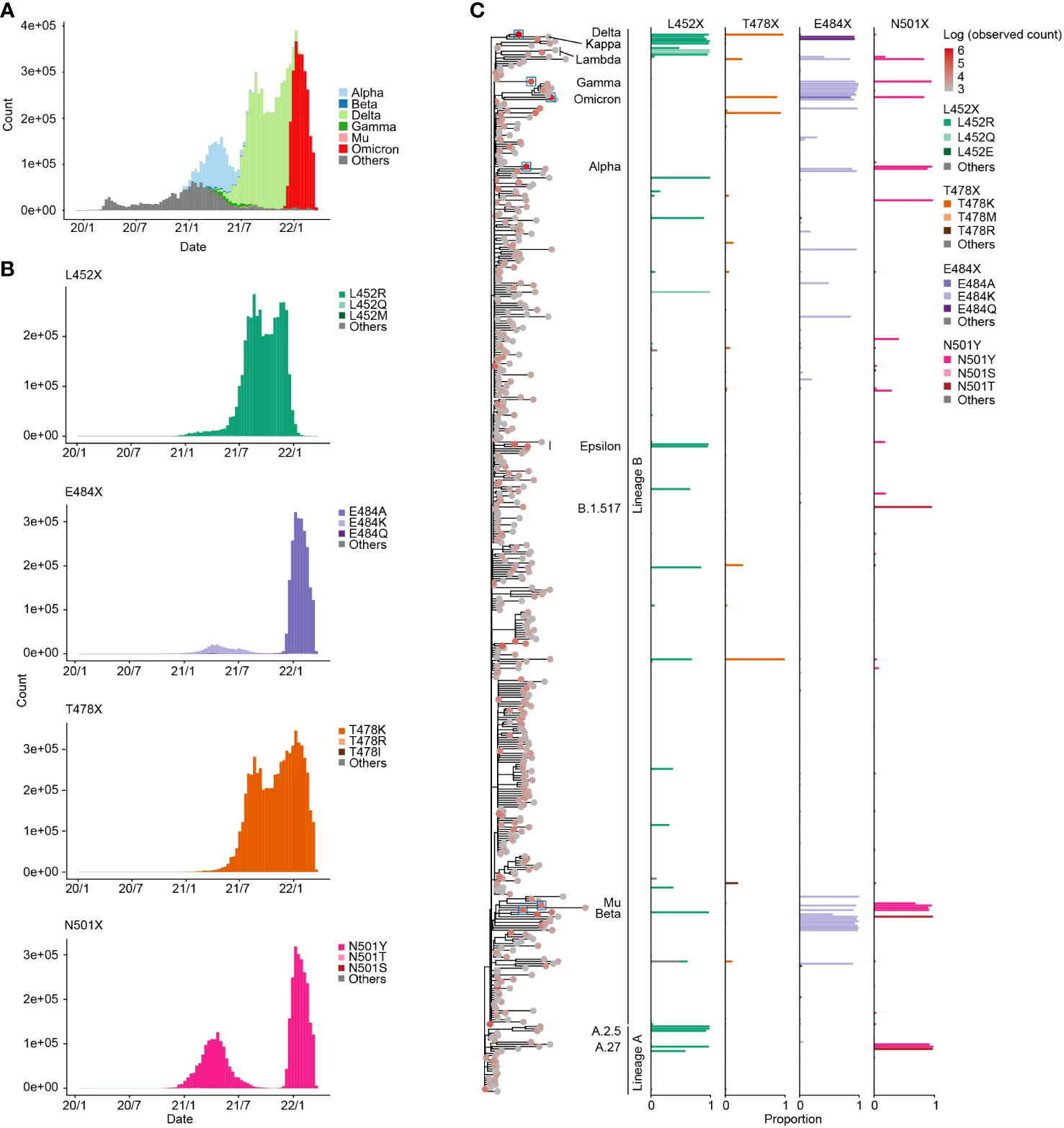
Figure 1 Prevalence of specific mutations at the four RBM sites. (A) Transition in the frequency of major epidemic variants, including VOCs in the GISAID genome surveillance data spanning December 2019 to March 2022. The X-axis represents the collection date, and the Y-axis indicates the number of sequences collected per ten-day period. (B) Transition in the frequency of sequences containing specific mutations at the four RBM sites. (C) Proportion of sequences containing specific mutations at the four RBM sites in each PANGO lineage. The left tree displays the phylogeny of PANGO lineages from CoVizu (16). The tip color represents the total number of sequences for each PANGO lineage. Tips related to epidemic variants presented in (A) are highlighted with boxes. In the right bar plots, bar colors denote specific mutation types.
We next investigated the prevalence of the four RBM mutations in each PANGO lineage (Figure 1C; Table S1). We found the L452R, E478K, E484K, and N501Y mutations in various lineages, including major epidemic variants such as VOCs. For instance, the L452R mutation is present not only in the Delta variant but also in the Epsilon variant (B.1.427 and B.1.429). SARS-CoV-2 is classified into lineages A and B, currently circulating and extinct lineages, respectively (28, 29), and these four mutations were present not only variants in lineage B but also in lineage A. For instance, A.27 had both the L452R and N501Y mutations. These findings suggest that the four RBM mutations have been independently acquired in various lineages, including lineages A and B, during SARS-CoV-2 diversification.
Furthermore, we identified minor types of amino acid substitutions in the four RBM sites. For example, the L452Q mutation was detected in certain strains such as the Lambda variant (C37; Figure 1C). The most diverse amino acid types were detected at position 484 among the four RBM sites. The E484A mutation, which is highly conserved in the Omicron variant, was the most observed amino acid mutation in the dataset. In addition, the E484K mutation is also prevalent in many PANGO lineages, including the Beta and Gamma variants. The E484Q mutation is conserved in the Kappa variant (B.1.617.1). At position 501, the N501Y mutation was present in numerous lineages including Alpha and Omicron variants, while the N501T mutation was highly conserved in some minor lineages, such as B.1.517.
Convergent acquisitions of the four RBM mutations
Next, we quantified how frequently the four RBM mutations (L452R, T478K, E484K, and N501Y) were acquired using a phylogenetic tree of SARS-CoV-2 provided by the Audacity Global Phylogeny, a phylogenic tree constructed from all high-quality sequences registered in GISAID as of March 23, 2022 [https://zenodo.org/record/4289383]. We inferred the ancestral branch where the acquisition of the four RBM mutations occurred using a maximum parsimony method (see Methods). We show that these four RBM mutations have been acquired multiple times in the phylogeny, including VOCs such as the Alpha and Delta variants (Figure 2A). The E484K mutation was acquired the most frequently, 31 times, among the four RBM mutations (Figure 2B). On the other hand, T478K was the least, acquired only 7 times. L452R and N501Y were acquired 25 and 17 times, respectively. There is also a branch that acquired E484K and N501Y simultaneously.
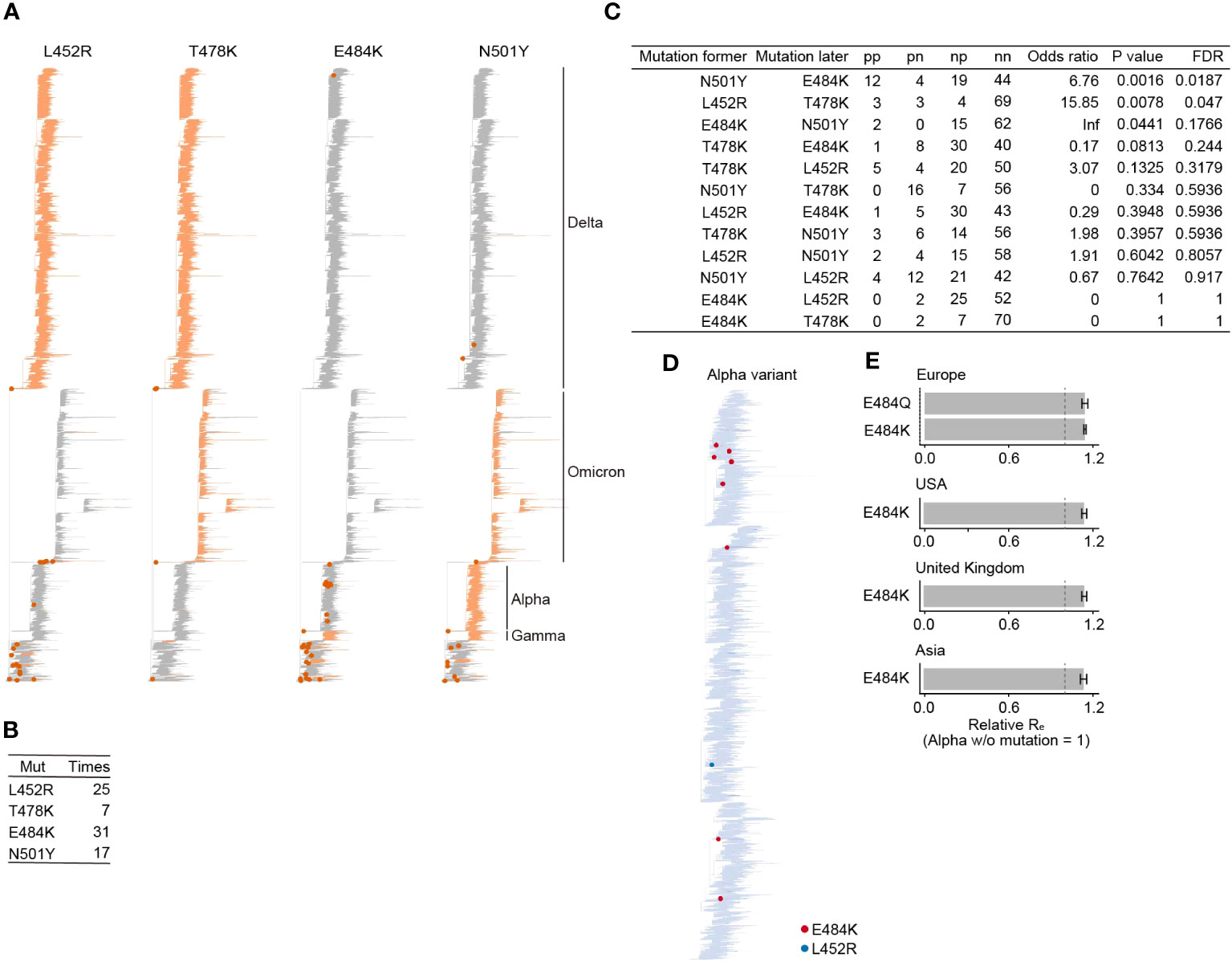
Figure 2 Acquisition frequency of mutations at the four RBM sites during SARS-CoV-2 evolution. (A) Ancestral state reconstruction for the four RBM mutations. Branches with mutations are colored in orange. A dot marks branches where a mutation was acquired. VOC clades are labeled. The phylogenetic tree is derived from Audacity Global Phylogeny, downloaded on March 23, 2022 (https://zenodo.org/record/4289383). (B) Count of inferred mutation acquisition events for the four RBM mutations. (C) The pattern of acquisition order of the four RBM mutations. We assessed if the likelihood of acquiring one mutation depends on the presence of another mutation, using Fisher’s exact test. The false discovery rate (FDR) was computed using the Holm method. (D) Tracking the acquisition events of the four RBM mutations throughout the diversification of the Alpha variant. (E) Relative Re of the Alpha variant subpopulations with or without mutations at the E484 site. Re of the Alpha variant lacking the E484 mutation is set to one. Error bars represent the 95% Bayesian confidence interval.
Some VOCs are known to have multiple mutations in the RBM. For example, the Delta variant has two mutations, L452R and T478K. To investigate the coexistence pattern of the four RBM mutations, we performed a statistical test to examine whether the acquisition probability of one mutation depends on the existence of another mutation using Fisher’s exact test. We detected two statistically significant pairs, N501Y preceding E484K, and L452R preceding T478K (Figure 2C). In other words, viruses with N501Y and T478K tend to acquire E484K and L452R, respectively. Interestingly, of the 31 acquisition events of E484K, 12 events occurred after the N501Y acquisition. Of these, eight events occurred in the Alpha variant, in which N501Y was highly conserved (Figure 2D). This frequent acquisition pattern of E484K in N501Y-bearing viruses, such as the Alpha variant, raises a hypothesis that the acquisition of E484K in N501Y-bearing viruses may increase viral fitness. To test this hypothesis, we then estimated the relative effective reproduction number (Re), representing the viral fitness (18), of the Alpha variant with or without mutations at the E484 site. This result showed that the subpopulation of Alpha variants with E484K or E484Q exhibited a significantly higher Re than those without E484K in the UK and the USA, where a certain number of Alpha variant sequences with E484 mutations were observed (Figure 2E; Table S2). Together, we showed that a mutation at the E484 site has been acquired repeatedly in the Alpha variant lineage, which harbors N501Y, and increases viral fitness in this lineage (see Discussion).
Contribution of the four RBM mutations to the increased Re
To continue investigating whether the acquisition of the four mutations in RBM (L452R, T478K, E484K, and N501Y) alters the fitness (i.e., Re) of the virus, we estimated the impact of each mutation on viral fitness using a Bayesian hierarchical multinomial logistic model we previously established (19) (Figure 3A; Table S1). This model estimates the effect sizes of individual mutations or mutation groups (groups of strongly coexisting mutations) on Re, assuming linear relationships among the mutations (19, 30). Using this model, we analyzed the GISAID data samples from the USA, the country with the most abundant viral genome sequence data, collected from November 9, 2020, to September 4, 2021 (300 days), roughly corresponding to the epidemic periods from Alpha to Delta variants in the chosen country. This analysis focused on the pre-Omicron period since almost all Omicron variants possess the mutations at three out of the four RBM sites (results for the post-Omicron period are shown in Figure S1, Table S2). We showed that the four RBM mutations had a relatively greater impact on Re compared to most of the other mutations or mutation groups (Figure 3A). Particularly, L452R, E484K, and N501Y have statistically significant positive effects on Re. These findings suggest that these mutations contribute to an increase in viral fitness. In addition to the four RBM mutations, we identified other mutations associated with increased viral fitness and present in many sequences, such as the A1537S mutation in NSP3 (see Discussion) (Figure 3B).
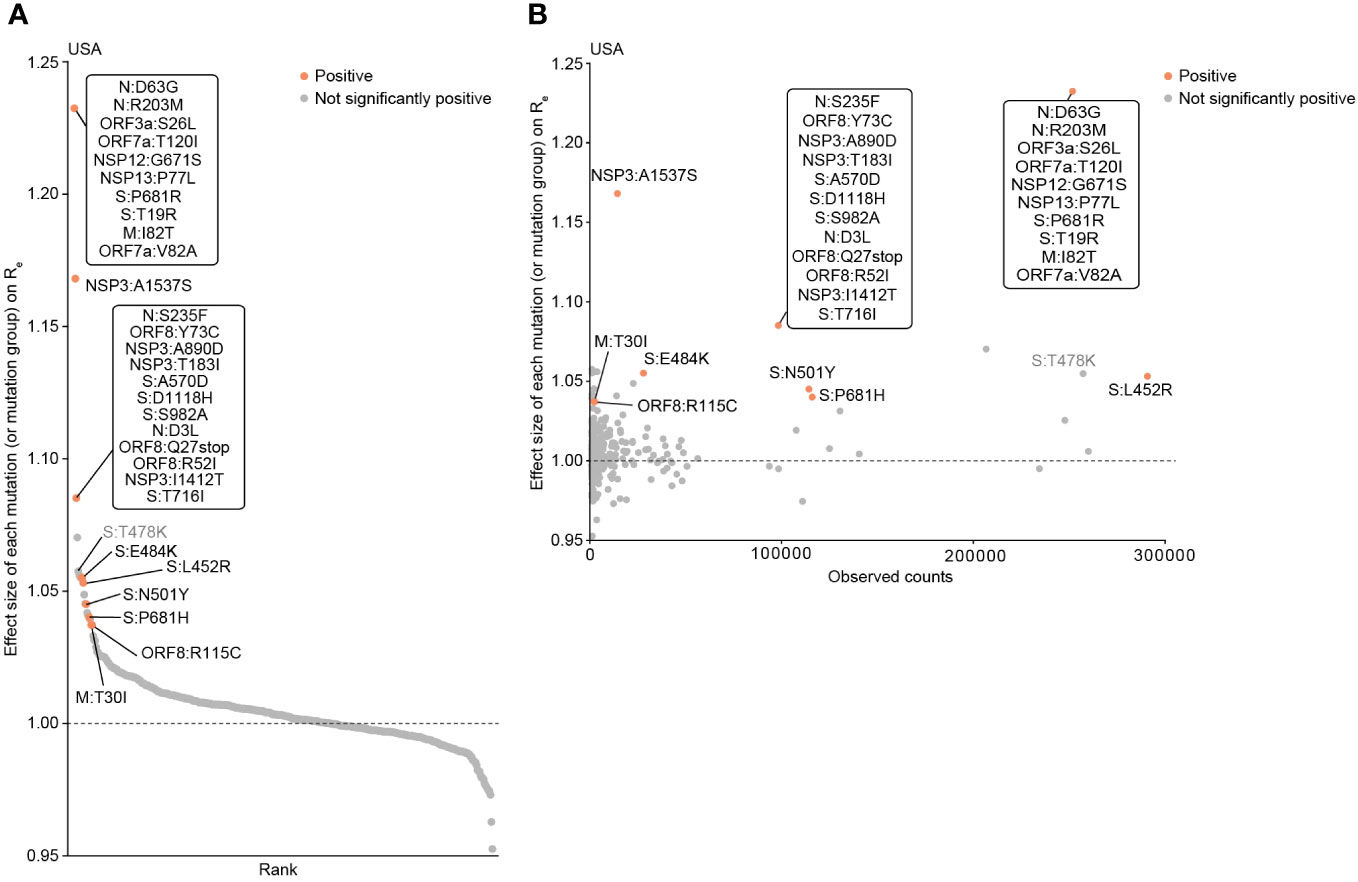
Figure 3 Epidemic dynamics modeling to determine the influence of mutations on viral fitness (Re). (A) Effect size of each mutation (or mutation group) on relative Re, determined by a Bayesian hierarchical model. The posterior mean value is shown. Groups of mutations that frequently co-occur are treated as a single mutation group. Mutations with significant positive effects are highlighted. The viral genome surveillance data in the USA collected from November 9, 2020 to September 4, 2021 was analyzed. (B) Scatter plot showing the prevalence of mutations and their respective effect sizes on Re.
The effects of the four RBM mutations on S protein functions
Next, we evaluated how the functions of the SARS-CoV-2 S protein are altered by the four RBM mutations and their combinations. We reconstructed a total of 16 mutants of the S protein, harboring all possible combinations of the four mutations (24 = 16 combinations; including the wild type), from the S protein of B.1.1 (the parental D614G-bearing variant). We subsequently performed four experiments: ACE2 binding assay, pseudoviral infection assay, fusion assay, and neutralization assay using sera from individuals vaccinated with the 2nd dose monovalent mRNA vaccine for ancestral SARS-CoV-2 (Figures 4A, B).
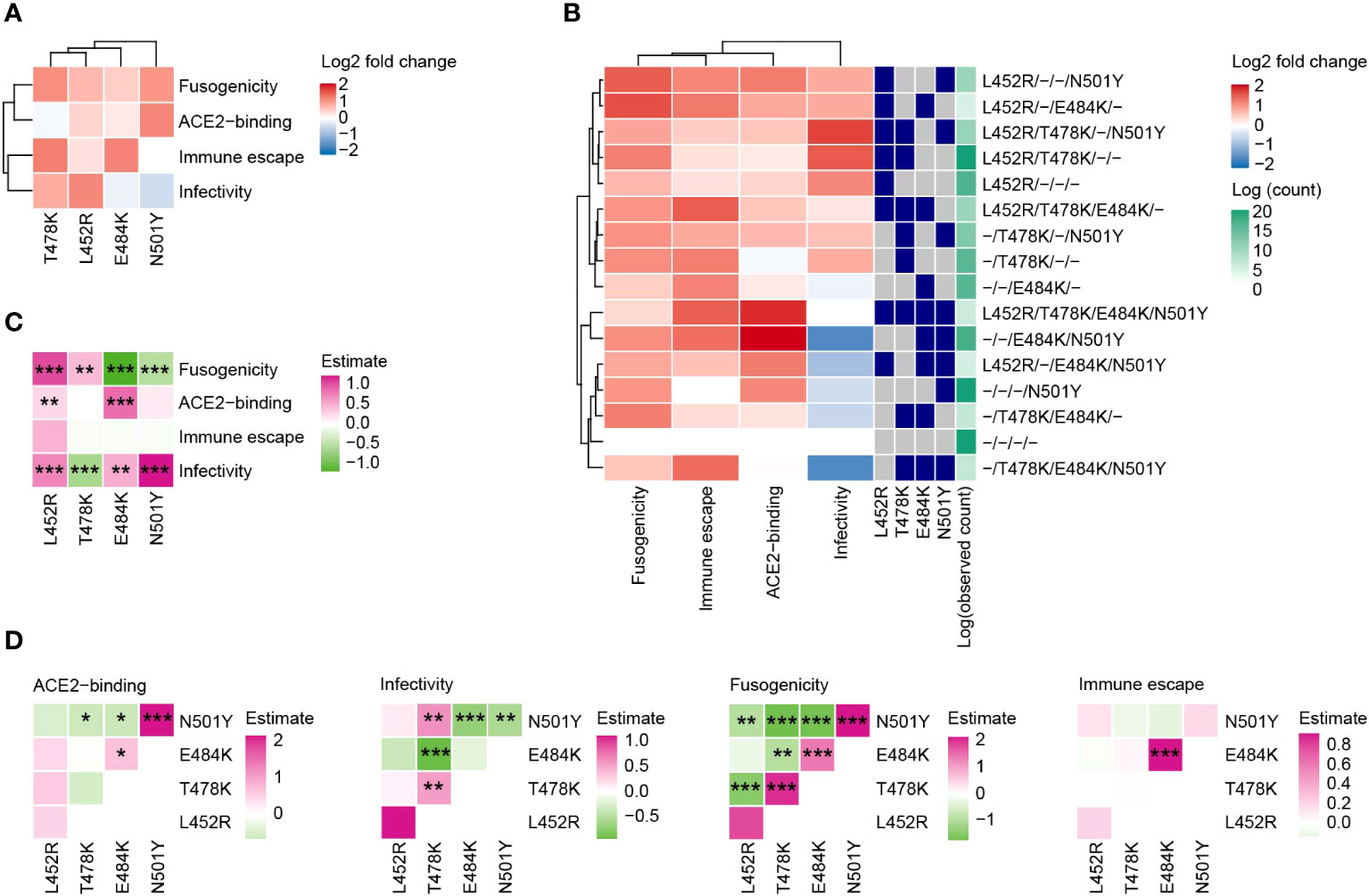
Figure 4 Effect of the four RBM mutations on S protein’s functions. (A) The impact of each individual mutation on S protein’s functions. The color represents the log2 fold change value in comparison to the wild type. (B) Phenotypic variations in mutant S proteins containing all possible combinations of the four RBM mutations (24 = 16 combinations, including the wild type). (C, D) Linear regression analyses to gauge mutation effects on the functions of each S protein. Results without (C) or with (D) interaction terms between pairs of mutations are displayed. Colors denote standardized effect sizes. Statistical significance was assessed using the Wald test, followed by FDR calculations using the Holm method. Symbols represent significance levels: *, FDR<0.1; **, FDR<0.01; ***, FDR<0.001.
The L452R enhanced all four evaluated functions of the S protein (Figure 4A). T478K enhanced infectivity, fusogenicity, and the ability to escape neutralization by the sera of vaccinated individuals. E484K had a particularly high impact on the neutralization escape ability. The N501Y mutant increased ACE2 binding affinity and fusogenicity. We found that individual mutations or their combinations generally upregulated the functions of the S protein we investigated except for the pseudoviral infectivity (Figure 4B). The S protein mutant with L452R+T478K+N501Y had the highest effect on infectivity among all the combinations. The mutant with L452R+T478K had the second-highest effect on infectivity and these mutations are highly conserved in the Delta variant. The mutant with E484K+N501Y, which is a combination observed in the Beta variant, had the highest effect on ACE2 binding affinity, while this mutant showed a highly negative effect on pseudoviral infectivity.
To further assess the effects of each mutation on viral function, we performed multiple regression analyses on the experiment results. We conducted the analysis using a model with interaction terms between two mutations (Figures 4C, D). Notably, although the effects of individual mutations on fusogenicity are significantly positive, most interaction terms between mutations on fusogenicity were significantly negative. This result indicates that the buildup of multiple mutations does not linearly enhance fusogenicity. For example, the S protein with all four mutations had weaker fusogenicity than that with single mutations (Figure 4B).
Discussion
In this study, we conducted an evolutionary and virological analysis to determine whether the four RBM mutations contributed to the increase in viral fitness. First, we demonstrated that these four mutations were acquired independently multiple times during SARS-CoV-2 evolution, which is a characteristic of mutations having a positive effect on viral fitness (19, 31) (Figures 1, 2). Second, using an epidemic modeling analysis, we showed that these RBM mutations have a positive effect on viral fitness (Re) (Figure 3). Finally, we demonstrated that each or combination of these mutations can modify the S protein functions that are strongly associated with viral fitness (Figure 4). Particularly, these mutations enhance the S protein’s ability to evade humoral immunity, which likely contributes to the reduced effectiveness of vaccinations observed in VOCs (32). Together, our results suggest that these four RBM mutations increase viral fitness by altering specific functions of the S protein.
We showed that the four RBM mutations were acquired independently multiple times, with E484K being acquired 31 times in total (Figure 2B). Interestingly, 12 out of 31 E484K acquisition events occurred after the N501Y acquisition (Figures 2C, D). This acquisition pattern was frequently observed in the Alpha variant, where N501Y was highly conserved. Furthermore, we showed that a subpopulation of Alpha harboring E484K exhibited a higher Re than the original Alpha variant (Figure 2D). These results suggest that the E484K acquisition following the N501Y acquisition increases viral fitness. Our experiments using mutant S proteins showed that N501Y increases ACE2-binding ability most strongly, and E484K mostly increased the escape ability from humoral immunity induced by vaccination, aligning with previous reports (Figure 4) (10–13). Furthermore, the mutant with both N501Y and E484K exhibited the highest ACE2 binding ability among all mutants and relatively higher escape ability from vaccine-induced humoral immunity. Together, these functional alterations by N501Y and E484K would contribute to the observed elevation of viral fitness by these mutations.
We showed that most of the 15 S mutants harboring the combination of these four mutations increased S protein functions, suggesting that these mutations contribute to the elevation of viral fitness (Figures 4A, B). Also, we showed that the effect that the combination of these mutations has on the S protein functions is not necessarily additive, suggesting the presence of epistatic effects between these mutations (Figure 4D). For instance, each mutation has the capacity to increase the fusogenicity of the S protein, but the interactions between these mutations reverse this effect, resulting in the mutant with all mutations displaying the lowest fusogenicity among the mutants (Figures 4B, D). For future research, the approach used in this study increased from 16 combinations of 4 mutations to all combinations of mutations of interest could be utilized to elucidate the complex interactions between mutations.
Our statistical modeling analysis suggests that all four mutations in RBM have an effect on increasing viral fitness (Figure 3B). In addition to these mutations, we found several mutations and groups of mutations that are associated with increased viral fitness. A1537S in NSP3 has the second-highest effect of all mutations or mutation groups. This NSP3 mutation was particularly observed in the Delta subvariants such as AY.44, AY.100, and AY.103, suggesting that this mutation is convergently acquired during the diversification of the Delta variant. Although most studies on SARS-CoV-2 variants are focusing on the S protein, mutational effects on non-S proteins should be elucidated in future studies, and our results provide a clue to identify critical non-S mutations for viral fitness.
Several limitations are present in this study. First, in our statistical analysis, it is difficult to separately estimate the effects between mutations whose presence is highly correlated to each other (referred to as a mutation group), such as mutations specifically observed in a certain strain (19). For instance, since T478K has a strong linkage disequilibrium with a group of mutations specific to the Delta variant, the estimated confidence interval of the effect of the T478K mutation on Re was wider than that of other mutations, leading to a non-significant score (Figure 3B; Table S3). Additionally, since the effect of a mutation group is estimated as a sum of the single mutation effects, the estimated effect size of a mutation group is subject to be higher than that of single mutations. To identify the causal mutation(s) increasing viral fitness within a mutation group, a sequence dataset containing sequences with a more diverse array of mutation combinations or experiments of viral function focusing on these mutations are needed. Another limitation relates to the lentiviral pseudoviral system used in this study. While one might expect pseudoviral infectivity to correlate with ACE2 binding affinity, these two viral properties have sometimes shown inconsistencies (24, 33, 34). For instance, in this study, the virus with E484K+N501Y mutations had the largest positive effect on the ACE2 binding affinity but a significant negative effect on pseudoviral infectivity (Figure 4B). To evaluate the effect of mutations on the authentic infectivity, experiments using other systems such as a replication-competent virus harboring specific mutations would be useful.
In conclusion, we showed that the four RBM mutations studied here increase viral fitness and impact multiple functionalities of the S protein, including improved ACE2 receptor binding and enhanced immune evasion capabilities. Indeed, the Omicron variant (BA.1) emerged with mutations at three out of the four RBM sites, and certain Omicron lineages—such as BA.4 and BA.5—harbor mutations at the four RBM sites (2, 33, 35), supporting the importance of these mutations. Understanding how RBM mutations affect viral fitness and S protein functions, as investigated in our study, can aid in the early identification of high-risk variants and facilitate more efficient vaccine development. Through continued analysis of epidemic dynamics and detailed experimental investigations, it may become possible to unravel the genotype-phenotype relationship of the virus. In the future, this could allow for evaluating the characteristics and risks of newly emerged variants directly from their sequences.
Data availability statement
The viral genome surveillance datasets of SARS-CoV-2 isolatesare available from the GISAID database (https://www.gisaid.org;EPI_SET_230220ow and EPI_SET_230220vu). The SupplementaryTable for each GISAID dataset is available in the GitHub repository(https://github.com/TheSatoLab/RBM_mutations).
Ethics statement
All protocols involving specimens from human subjects recruited at Kyoto University were reviewed and approved by the Institutional Review Board of Kyoto University (approval ID: G1309). All human subjects provided written informed consent. All protocols for the use of human specimens were reviewed and approved by the Institutional Review Boards of The Institute of Medical Science, The University of Tokyo (approval IDs: 2021-1-0416 and 2021-18-0617), Kyoto University (approval ID: G0697) and Kumamoto University (approval IDs: 2066 and 2074).
Author contributions
YM: Formal Analysis, Investigation, Methodology, Software, Validation, Visualization, Writing – review & editing. HN: Investigation, Writing – review & editing. JZ: Investigation, Writing – review & editing. SM: Investigation, Writing – review & editing. RS: Investigation, Writing – review & editing. KN: Investigation, Writing – review & editing. AT-K: Resources, Supervision, Writing – review & editing. GS: Supervision, Writing – review & editing. KSh: Investigation, Supervision, Writing – review & editing. AS: Investigation, Supervision, Writing – review & editing. TI: Investigation, Supervision, Writing – review & editing. JI: Conceptualization, Funding acquisition, Project administration, Software, Supervision, Writing – original draft. KSa: Funding acquisition, Supervision, Writing – review & editing.
Group members of The Genotype to Phenotype Japan (G2P-Japan) Consortium
Keita Matsuno1, Keita Mizuma1, Isshu Kojima1, Jingshu Li1, Hirofumi Sawa1, Naganori Nao1, Tomoya Tsubo1, Shinya Tanaka1, Masumi Tsuda1, Lei Wang1, Yoshikata Oda1, Zannatul Ferdous1, Kenji Shishido1, Takasuke Fukuhara1, Tomokazu Tamura1, Rigel Suzuki1, Saori Suzuki1, Shuhei Tsujino1, Hayato Ito1, Yu Kaku2, Naoko Misawa2, Arnon Plianchaisuk2, Ziyi Guo2, Alfredo A Hinay Jr.2, Kaoru Usui2, Wilaiporn Saikruang2, Keiya Uriu2, Yusuke Kosugi2, Shigeru Fujita2, Jarel Elgin M. Tolentino2, Luo Chen2, Lin Pan2, Mai Suganami2, Mika Chiba2, Ryo Yoshimura2, Kyoko Yasuda2, Keiko Iida2, Adam P. Strange2, Naomi Ohsumi2, Shiho Tanaka2, Kaho Okumura2, Kazuhisa Yoshimura3, Kenji Sadamasu3, Mami Nagashima3, Hiroyuki Asakura3, Isao Yoshida3, So Nakagawa4, Ryosuke Nomura5, Yoshihito Horisawa5, Yusuke Tashiro5, Yugo Kawai5, Kazuo Takayama5, Rina Hashimoto5, Sayaka Deguchi5, Yukio Watanabe5, Yoshitaka Nakata5, Hiroki Futatsusako5, Ayaka Sakamoto5, Naoko Yasuhara5, Takao Hashiguchi5, Tateki Suzuki5, Kanako Kimura5, Jiei Sasaki5, Yukari Nakajima5, Hisano Yajima5, Takashi Irie6, Ryoko Kawabata6, Kaori Tabata7, MST Monira Begum8, Michael Jonathan8, Yuka Mugita8, Sharee Leong8, Otowa Takahashi8, Kimiko Ichihara8, Takamasa Ueno8, Chihiro Motozono8, Mako Toyoda8, Maya Shofa9, Yuki Shibatani9, Tomoko Nishiuchi9, Prokopios Andrikopoulos10, Miguel Padilla-Blanco10, Aditi Konar10
Funding
The author(s) declare financial support was received for the research, authorship, and/or publication of this article. This study was supported in part by AMED SCARDA Japan Initiative for World-leading Vaccine Research and Development Centers “UTOPIA” (JP223fa627001, to KSa), AMED SCARDA Program on R&D of new generation vaccine including new modality application (JP223fa727002, to KSa); AMED Research Program on Emerging and Re-emerging Infectious Diseases (JP21fk0108574, to HN; JP21fk0108465, to AS; JP22fk0108146, to KSa; JP21fk0108494 to G2P-Japan Consortium, TI and KSa; JP21fk0108425, to KSa; JP21fk0108432, to KSa; JP22fk0108534, to TI, and KSa; JP22fk0108511, to TI and KSa); AMED Research Program on HIV/AIDS (JP22fk0410033, to AS; JP22fk0410047, to AS; JP22fk0410055, to TI; and JP22fk0410039, to KSa); AMED CRDF Global Grant (JP22jk0210039 to AS); AMED Japan Program for Infectious Diseases Research and Infrastructure (JP22wm0325009, to AS); JST PRESTO (JPMJPR22R1, to JI); JST CREST (JPMJCR20H4, to KSa); JSPS KAKENHI Grant-in-Aid for Scientific Research C (22K07103, to TI); JSPS KAKENHI Grant-in-Aid for Early-Career Scientists (22K16375, to HN; 20K15767, JI); JSPS Core-to-Core Program (A. Advanced Research Networks) (JPJSCCA20190008, to KSa); JSPS Leading Initiative for Excellent Young Researchers (LEADER) (to TI); International Joint Research Project of the Institute of Medical Science, the University of Tokyo (to TI, JZ, and AS); The Tokyo Biochemical Research Foundation (to KSa); Takeda Science Foundation (to TI); Mochida Memorial Foundation for Medical and Pharmaceutical Research (to TI); The Naito Foundation (to TI); and the project of National Institute of Virology and Bacteriology, Programme EXCELES, funded by the European Union, Next Generation EU (LX22NPO5103, to JZ).
Acknowledgments
We would like to thank all members belonging to The Genotype to Phenotype Japan (G2P-Japan) Consortium. We thank Dr. Kenzo Tokunaga (National Institute for Infectious Diseases, Japan) and Dr. Jin Gohda (The University of Tokyo, Japan) for providing reagents. We gratefully acknowledge all data contributors, i.e. the Authors and their Originating laboratories responsible for obtaining the specimens, and their submitting laboratories for generating the genetic sequence and metadata and sharing via the GISAID Initiative, on which this research is based. The super-computing resource was provided by the Human Genome Center at The University of Tokyo.
Conflict of interest
JI has consulting fees and honoraria for lectures from Takeda Pharmaceutical Co. Ltd. KSa has consulting fees from Moderna Japan Co., Ltd. and Takeda Pharmaceutical Co. Ltd. and honoraria for lectures from Gilead Sciences, Inc., Moderna Japan Co., Ltd., and Shionogi & Co., Ltd.
The remaining authors declare that the research was conducted in the absence of any commercial or financial relationships that could be construed as a potential conflict of interest.
The author(s) declared that they were an editorial board member of Frontiers, at the time of submission. This had no impact on the peer review process and the final decision.
Publisher’s note
All claims expressed in this article are solely those of the authors and do not necessarily represent those of their affiliated organizations, or those of the publisher, the editors and the reviewers. Any product that may be evaluated in this article, or claim that may be made by its manufacturer, is not guaranteed or endorsed by the publisher.
Supplementary material
The Supplementary Material for this article can be found online at: https://www.frontiersin.org/articles/10.3389/fviro.2023.1328229/full#supplementary-material
Supplementary Figure 1 | Epidemic analysis including successive Omicron subvariants. (A) Transition in the frequency of major epidemic variants including VOCs in the GISAID data from December 2019 to August 2022. (B) Transition in the frequency of sequences with specific mutations at the four RBM sites. (C) Effect size of each mutation (or mutation group) on relative Re, determined by a Bayesian hierarchical model. The posterior mean value is shown. Groups of mutations that frequently co-occur are treated as a single mutation group. Mutations with significant positive effects are highlighted.
Footnotes
- ^ Hokkaido University, Hokkaido, Japan
- ^ The University of Tokyo, Tokyo, Japan
- ^ Tokyo Metropolitan Institute of Public Health, Tokyo, Japan
- ^ Tokai University, Kanagawa, Japan
- ^ Kyoto University, Kyoto, Japan
- ^ Hiroshima University, Hiroshima, Japan
- ^ Kyushu University, Fukuoka, Japan
- ^ Kumamoto University, Kumamoto, Japan
- ^ University of Miyazaki, Miyazaki, Japan
- ^ Charles University, Vestec, Czechia
References
1. Wu F, Zhao S, Yu B, Chen YM, Wang W, Song ZG, et al. A new coronavirus associated with human respiratory disease in China. Nature (2020) 579:265–9. doi: 10.1038/s41586-020-2008-3
2. Carabelli AM, Peacock TP, Thorne LG, Harvey WT, Hughes J, Peacock SJ, et al. SARS-CoV-2 variant biology: immune escape, transmission and fitness. Nat Rev Microbiol (2023) 21:162–77. doi: 10.1038/s41579-022-00841-7
3. Tao K, Tzou PL, Nouhin J, Gupta RK, de Oliveira T, Kosakovsky Pond SL, et al. The biological and clinical significance of emerging SARS-CoV-2 variants. Nat Rev Genet (2021) 22:757–73. doi: 10.1038/s41576-021-00408-x
4. Cao Y, Yisimayi A, Jian F, Song W, Xiao T, Wang L, et al. BA.2.12.1, BA.4 and BA.5 escape antibodies elicited by Omicron infection. Nature (2022) 608:593–602. doi: 10.1038/s41586-022-04980-y
5. Hie B, Zhong ED, Berger B, Bryson B. Learning the language of viral evolution and escape. Science (2021) 371:284–8. doi: 10.1126/science.abd7331
6. Cao Y, Jian F, Wang J, Yu Y, Song W, Yisimayi A, et al. Imprinted SARS-CoV-2 humoral immunity induces convergent Omicron RBD evolution. Nature (2023) 614:521–9. doi: 10.1038/s41586-022-05644-7
7. Viana R, Moyo S, Amoako DG, Tegally H, Scheepers C, Althaus CL, et al. Rapid epidemic expansion of the SARS-CoV-2 Omicron variant in southern Africa. Nature (2022) 603:679–86. doi: 10.1038/s41586-022-04411-y
8. Motozono C, Toyoda M, Zahradnik J, Saito A, Nasser H, Tan TS, et al. SARS-CoV-2 spike L452R variant evades cellular immunity and increases infectivity. Cell Host Microbe (2021) 29:1124–1136.e11. doi: 10.1016/j.chom.2021.06.006
9. Deng X, Garcia-Knight MA, Khalid MM, Servellita V, Wang C, Morris MK, et al. Transmission, infectivity, and neutralization of a spike L452R SARS-CoV-2 variant. Cell (2021) 184:3426–3437.e8. doi: 10.1016/j.cell.2021.04.025
10. Jangra S, Ye C, Rathnasinghe R, Stadlbauer D, Krammer F, Simon V, et al. SARS-CoV-2 spike E484K mutation reduces antibody neutralisation. Lancet Microbe (2021) 2:e283–4. doi: 10.1016/S2666-5247(21)00068-9
11. Weisblum Y, Schmidt F, Zhang F, DaSilva J, Poston D, Lorenzi JC, et al. Escape from neutralizing antibodies by SARS-CoV-2 spike protein variants. Elife (2020) 9. doi: 10.7554/eLife.61312
12. Liu Y, Liu J, Plante KS, Plante JA, Xie X, Zhang X, et al. The N501Y spike substitution enhances SARS-CoV-2 infection and transmission. Nature (2022) 602:294–9. doi: 10.1038/s41586-021-04245-0
13. Tian F, Tong B, Sun L, Shi S, Zheng B, Wang Z, et al. N501Y mutation of spike protein in SARS-CoV-2 strengthens its binding to receptor ACE2. Elife (2021) 10. doi: 10.7554/eLife.69091
14. Starr TN, Greaney AJ, Hilton SK, Ellis D, Crawford KHD, Dingens AS, et al. Deep mutational scanning of SARS-CoV-2 receptor binding domain reveals constraints on folding and ACE2 binding. Cell (2020) 182:1295–1310.e20. doi: 10.1016/j.cell.2020.08.012
15. Khare S, Gurry C, Freitas L, Schultz MB, Bach G, Diallo A, et al. GISAID’s role in pandemic response. China CDC Wkly (2021) 3:1049–51. doi: 10.46234/ccdcw2021.255
16. Ferreira RC, Wong E, Gugan G, Wade K, Liu M, Baena LM, et al. CoVizu: Rapid analysis and visualization of the global diversity of SARS-CoV-2 genomes. Virus Evol (2021) 7:veab092. doi: 10.1093/ve/veab092
17. Louca S, Doebeli M. Efficient comparative phylogenetics on large trees. Bioinformatics (2018) 34:1053–5. doi: 10.1093/bioinformatics/btx701
18. Suzuki R, Yamasoba D, Kimura I, Wang L, Kishimoto M, Ito J, et al. Attenuated fusogenicity and pathogenicity of SARS-CoV-2 Omicron variant. Nature (2022) 603:700–5. doi: 10.1038/s41586-022-04462-1
19. Ito J, Suzuki R, Uriu K, Itakura Y, Zahradnik J, Kimura KT, et al. Convergent evolution of SARS-CoV-2 Omicron subvariants leading to the emergence of BQ.1.1 variant. Nat Commun (2023) 14:2671. doi: 10.1038/s41467-023-38188-z
20. Ozono S, Zhang Y, Ode H, Sano K, Tan TS, Imai K, et al. SARS-CoV-2 D614G spike mutation increases entry efficiency with enhanced ACE2-binding affinity. Nat Commun (2021) 12:848. doi: 10.1038/s41467-021-21118-2
21. Ozono S, Zhang Y, Tobiume M, Kishigami S, Tokunaga K. Super-rapid quantitation of the production of HIV-1 harboring a luminescent peptide tag. J Biol Chem (2020) 295:13023–30. doi: 10.1074/jbc.RA120.013887
22. Yamamoto M, Kiso M, Sakai-Tagawa Y, Iwatsuki-Horimoto K, Imai M, Takeda M, et al. The anticoagulant nafamostat potently inhibits SARS-CoV-2 S protein-mediated fusion in a cell fusion assay system and viral infection in vitro in a cell-type-dependent manner. Viruses (2020) 12. doi: 10.3390/v12060629
23. Niwa H, Yamamura K, Miyazaki J. Efficient selection for high-expression transfectants with a novel eukaryotic vector. Gene (1991) 108:193–9. doi: 10.1016/0378-1119(91)90434-D
24. Yamasoba D, Uriu K, Plianchaisuk A, Kosugi Y, Pan L, Zahradnik J, et al. Virological characteristics of the SARS-CoV-2 omicron XBB.1.16 variant. Lancet Infect Dis (2023) 23:655–6. doi: 10.1016/S1473-3099(23)00278-5
25. Nasser H, Shimizu R, Ito J, Saito A, Sato K, Ikeda T. Monitoring fusion kinetics of viral and target cell membranes in living cells using a SARS-CoV-2 spike-protein-mediated membrane fusion assay. STAR Protoc (2022) 3:101773. doi: 10.1016/j.xpro.2022.101773
26. Begum MM, Ichihara K, Takahashi O, Nasser H, Jonathan M, Tokunaga K, et al. Virological characteristics correlating with SARS-CoV-2 spike protein fusogenicity. bioRxiv (2023). doi: 10.1101/2023.10.03.560628:2023.10.03.560628
27. Kondo N, Miyauchi K, Matsuda Z. Monitoring viral-mediated membrane fusion using fluorescent reporter methods. Curr Protoc Cell Biol (2011) Chapter 26:Unit 26.9. doi: 10.1002/0471143030.cb2609s50
28. Rambaut A, Holmes EC, O’Toole Á, Hill V, McCrone JT, Ruis C, et al. A dynamic nomenclature proposal for SARS-CoV-2 lineages to assist genomic epidemiology. Nat Microbiol (2020) 5:1403–7. doi: 10.1038/s41564-020-0770-5
29. Pekar JE, Magee A, Parker E, Moshiri N, Izhikevich K, Havens JL, et al. The molecular epidemiology of multiple zoonotic origins of SARS-CoV-2. Science (2022) 377:960–6. doi: 10.1126/science.abp8337
30. Obermeyer F, Jankowiak M, Barkas N, Schaffner SF, Pyle JD, Yurkovetskiy L, et al. Analysis of 6.4 million SARS-CoV-2 genomes identifies mutations associated with fitness. Science (2022) 376:1327–32. doi: 10.1126/science.abm1208
31. Bloom JD, Neher RA. Fitness effects of mutations to SARS-CoV-2 proteins. bioRxiv (2023). doi: 10.1101/2023.01.30.526314
32. Zeng B, Gao L, Zhou Q, Yu K, Sun F. Effectiveness of COVID-19 vaccines against SARS-CoV-2 variants of concern: a systematic review and meta-analysis. BMC Med (2022) 20:200. doi: 10.1186/s12916-022-02397-y
33. Kimura I, Yamasoba D, Tamura T, Nao N, Suzuki T, Oda Y, et al. Virological characteristics of the SARS-CoV-2 Omicron BA.2 subvariants, including BA.4 and BA.5. Cell (2022) 185:3992–4007.e16. doi: 10.1016/j.cell.2022.09.018
34. Saito A, Tamura T, Zahradnik J, Deguchi S, Tabata K, Anraku Y, et al. Virological characteristics of the SARS-CoV-2 Omicron BA.2.75 variant. Cell Host Microbe (2022) 30:1540–1555.e15. doi: 10.1101/2022.08.07.503115
Keywords: SARS-CoV-2, evolution, receptor binding motif, variants of concerns, epidemic dynamics modeling
Citation: Masuda Y, Nasser H, Zahradnik J, Mitoma S, Shimizu R, Nagata K, Takaori-Kondo A, Schreiber G, The Genotype to Phenotype Japan (G2P-Japan) Consortium, Shirakawa K, Saito A, Ikeda T, Ito J and Sato K (2023) Characterization of the evolutionary and virological aspects of mutations in the receptor binding motif of the SARS-CoV-2 spike protein. Front. Virol. 3:1328229. doi: 10.3389/fviro.2023.1328229
Received: 26 October 2023; Accepted: 29 November 2023;
Published: 22 December 2023.
Edited by:
Alex Compton, National Cancer Institute at Frederick (NIH), United StatesReviewed by:
Veronica Ueckermann, University of Pretoria, South AfricaWajihul Hasan Khan, All India Institute of Medical Sciences, India
Copyright © 2023 Masuda, Nasser, Zahradnik, Mitoma, Shimizu, Nagata, Takaori-Kondo, Schreiber, The Genotype to Phenotype Japan (G2P-Japan) Consortium, Shirakawa, Saito, Ikeda, Ito and Sato. This is an open-access article distributed under the terms of the Creative Commons Attribution License (CC BY). The use, distribution or reproduction in other forums is permitted, provided the original author(s) and the copyright owner(s) are credited and that the original publication in this journal is cited, in accordance with accepted academic practice. No use, distribution or reproduction is permitted which does not comply with these terms.
*Correspondence: Jumpei Ito, jampei@g.ecc.u-tokyo.ac.jp; Kei Sato, KeiSato@g.ecc.u-tokyo.ac.jp
†These authors have contributed equally to this work