1
Biomagnetic Imaging Lab, Department of Radiology, University of California San Francisco (UCSF), San Francisco, CA, USA
2
Department of Neurology, University of Berne, Bern, Switzerland
3
Joint Graduate Group in Bioengineering, University of California, San Francisco, and University of California Berkeley, USA
We examine the relative timing of numerous brain regions involved in human decisions that are based on external criteria, learned information, personal preferences, or unconstrained internal considerations. Using magnetoencephalography (MEG) and advanced signal analysis techniques, we were able to non-invasively reconstruct oscillations of distributed neural networks in the high-gamma frequency band (60–150 Hz). The time course of the observed neural activity suggested that two-alternative forced choice tasks are processed in four overlapping stages: processing of sensory input, option evaluation, intention formation, and action execution. Visual areas are activated first, and show recurring activations throughout the entire decision process. The temporo-occipital junction and the intraparietal sulcus are active during evaluation of external values of the options, 250–500 ms after stimulus presentation. Simultaneously, personal preference is mediated by cortical midline structures. Subsequently, the posterior parietal and superior occipital cortices appear to encode intention, with different subregions being responsible for different types of choice. The cerebellum and inferior parietal cortex are recruited for internal generation of decisions and actions, when all options have the same value. Action execution was accompanied by activation peaks in the contralateral motor cortex. These results suggest that high-gamma oscillations as recorded by MEG allow a reliable reconstruction of decision processes with excellent spatiotemporal resolution.
Choosing plays an important role in our daily life, since it not only reflects our current environment and internal state, but it also determines our future situation. The advent of modern functional brain imaging methods and electrophysiological techniques during the last two decades has given us the unprecedented opportunity to investigate the underlying neural mechanisms. Different sets of brain areas that mediate choice have been revealed and accurate models of neural decision processing have been formulated.
It has been shown that rational decision making, during which the available information about the eligible options is rationally evaluated with regard to their respective value (Montague et al., 2006
; Sanfey et al., 2006
; Sugrue et al., 2004
), is primarily mediated by the parietal cortex (Andersen and Buneo, 2002
; Platt and Glimcher, 1999
; Sugrue et al., 2004
). Some rational decisions – such as choosing a larger sensory stimulus – depend uniquely on the externally given sensory information (Romo and Salinas, 2003
). Yet, the most conspicuous feature of decision making that distinguishes it phenomenologically from reflexive behavior is the faculty of acting not only according to current sensory representations of external conditions, but additionally according to internal self-related values and preferences (Montague et al., 2006
; Sugrue et al., 2004
). This internal value-system depends on complex neural mechanisms. Correspondingly, imaging studies in humans have shown that self-related judgments are associated with an activation of cortical midline structures ranging from the ventromedial prefrontal cortex (VMPFC) to the medial parietal cortex (MPC) (Gusnard et al., 2001
; Northoff and Bermpohl, 2004
; Northoff et al., 2006
; Zysset et al., 2002
). In cases of rational uncertainty about the value of the options, healthy individuals were found to be capable of making advantageous decisions and correct guesses (Bechara et al., 1997
; Maia and McClelland, 2004
), as mediated primarily by prefrontal brain areas (Bechara et al., 1997
; Elliott et al., 1999
). Studies investigating the neural processes underlying internal movement selection reported that the supplementary motor area (SMA) plays a crucial role in these cases (Deiber et al., 1991
, 1996
;Shibasaki and Hallett, 2006
; Thaler et al., 1995
).
In spite of this large body of knowledge about the localization of brain regions mediating decisions, our understanding of human volition and decision making is constrained by a lack of information about how the temporally distributed activities in spatially distributed neural networks are integrated into a coherent decision and action. For instance, although we know that cortical midline structures are involved during preference judgments (Gusnard et al., 2001
; Northoff and Bermpohl, 2004
; Northoff et al., 2006
; Zysset et al., 2002
), we are ignorant about the specific role of each of these areas (Northoff et al., 2006
), and about how and when personal preferences influence the decision process. Moreover, although we know that explicit memory retrieval is associated with activations in dorsolateral prefrontal and parietal cortices (Buckner and Koutstaal, 1998
; Schott et al., 2005
), it is unclear how and when memories are integrated into current sensory representations of options and, ultimately, into actions. Similarly, although we have detailed knowledge about the timing of neural activity in primary motor cortex (MC) and SMA during “free” internally generated actions (Kornhuber and Deecke, 1965
; Libet et al., 1982
; Shibasaki and Hallett, 2006
; Sochurkova et al., 2006
), we do not know whether and how these motor areas interact over time with sensory and associative areas.
This lack of empirical data is mainly due to inherent limitations of the techniques currently used for the assessment of neural activity. Functional imaging techniques such as functional magnetic resonance imaging (fMRI) or positron emission tomography (PET) provide insufficient temporal resolution for reconstructing the dynamics of neural activity. Intracerebral electrophysiological methods are unable to record simultaneously from multiple distributed brain regions and are rarely possible in humans. Finally, electroencephalography (EEG) studies relying on movement related slow potential shifts such as the Bereitschaftspotential (Kornhuber and Deecke, 1965
) are only sensitive to neural activity in motor areas (MC) (Shibasaki and Hallett, 2006
; Sochurkova et al., 2006
) and often suffer from insufficient spatial resolution.
In order to get an integrated picture of the complex spatiotemporal interactions among neural systems and networks taking place during human decision making, we therefore need techniques that allow us to observe the entire human cortex with high resolution in both space and time. Magnetoencephalography (MEG) seems to be a promising tool in this regard. Recent studies of averaged task-evoked fields recorded with MEG have yielded new insights into the temporal aspects of human perception (Poghosyan and Ioannides, 2007
; Poghosyan et al., 2005
) and decision making (Amano et al., 2006
; Periáñez et al., 2004
). Electrocorticographical (ECoG) studies have demonstrated that oscillations of neural networks in the “high-gamma” frequency range (>60 Hz) are reliable markers of cortical activity that outperform traditional EEG and fMRI markers in combined spatiotemporal resolution; they are spatially more focal and task-specific than slow neural oscillations and event-related potentials (Brovelli et al., 2005
; Canolty et al., 2006
; Crone et al., 2006
; Edwards et al., 2005
), and they correlate with the fMRI hemodynamic response (Brovelli et al., 2005
; Logothetis et al., 2001
; Niessing et al., 2005
) while having much better time resolution. Whereas ECoG still has the disadvantage of being invasive and limited to a small brain region covered by an electrode grid, there is increasing evidence that neural oscillations, including high-gamma rhythms, can be recorded non-invasively and simultaneously from the entire human cortex with MEG, and subsequently be reconstructed in space, time, and the frequency domain with advanced signal analysis techniques (Dalal et al., 2008
; Hoogenboom et al., 2006
; Luo et al., 2007
; Medendorp et al., 2007
; Osipova et al., 2006
; Vidal et al., 2006
).
In this study, we optimized existing MEG signal analysis methods for reconstruction of high-gamma frequency band activity in order to gain insights into the spatiotemporal patterns of neural activity during different types of decisions. We designed a two-alternative forced choice paradigm that allowed us to analyze and compare the neural correlates of rational, externally cued and self-related judgments, as well as of “free” internally generated choices, while controlling for differences in visual option-stimuli and subject responses. In order to study unconstrained internal decisions, we confronted the participants with the choice situation posed to Buridan’s ass in the famous paradox commonly ascribed to the 14th century philosopher Jean Buridan. The hypothetical rational donkey was unable to choose between two equally near, large, and appetizing stacks of hay, and therefore faced starvation. It was hoped that this analysis would shed light on the neural mechanisms that prevent us from suffering the same fate and that enable us to make unconstrained internal decisions.
Subjects
Ten healthy subjects (aged 26–44, 4 females) gave their written informed consent to participate in the experiments. All procedures were approved by the UCSF Committee on Human Research and conducted in accordance with the Declaration of Helsinki.
Recordings
The participants were seated in a magnetically shielded room, while their MEG was recorded with a 275 channel whole-head CTF Omega 2000 system (VSM MedTech, Coquitlam, BC, Canada), using a sampling rate of 1200 Hz.
Experimental Paradigm
The subjects were asked to perform two-alternative forced choice tasks according to 5 different experimental conditions indicated in Table 1
. Choosing according to external cues was studied by letting the participants choose between 2 visually presented single digit numbers, either the number that was printed in bold (Visual condition) or the number with the higher numerical value (Numerical condition). The processing of memories during a decision task was investigated with an explicit memory retrieval paradigm: the participants were instructed to choose the number that they had memorized 12–24 hours before the main experiment on a list of 5 single digit numbers (Memory condition). The neural integration of preferences and internal values into a decision and action was analyzed by asking the participants to choose the number that they personally preferred (Preference condition). In order to study the neural processing during choices that are completely independent of external variables and determined only by the agent’s current internal state, we confronted the participants with 2 exactly identical numbers (Free condition).
Each task condition consisted of 100 trials (in total 500 trials per subject) with a visual stimulus followed by a button response (Figure 1
A). During each trial, subjects had to fixate a cross in their central visual field, which was presented 0.2 seconds after a 1 kHz warning tone. After an interval varying randomly between 1.2 and 1.4 seconds, the fixation cross disappeared and two single digit numbers were projected 1.5° to the left and right of the subject’s central visual field, respectively. Subjects were asked to choose one of the two numbers by pressing a button on the corresponding side with their right (dominant) index or middle finger within a time interval of 1.2 seconds. Each trial ended with a 750 Hz tone signaling the possibility to blink within the next 1.2 seconds (before the next warning tone). Motor responses were obtained from the right hand only, because we were interested in the cognitive aspects of decision making rather than movement selection, and therefore wanted to ensure a constant motor response in all conditions and trials. Congruency effects due to responses to visual stimuli with the ispilateral vs. contralateral hand would have been equally distributed across conditions.
Figure 1. Flowchart of study paradigm, applied signal analyses, and statistical procedures. Example images from the Preference condition are shown. (A) Ten healthy volunteers performed visuo-motor, two-alternative, forced choice tasks as shown in Table 1
. Oscillation power changes were calculated relative to a baseline before presentation of the options for a series of moving time windows after presentation of the options, and for each of the frequency bands 60–90, 90–120, and 120–150 Hz. (B) A set of adaptive spatial filters was used to reconstruct the sources of power changes at each voxel, time window, and frequency band from the recordings of all MEG sensors. For contrasts between condition pairs, the difference in power changes between the conditions at each time-frequency point was used: (active1–baseline1) – (active2–baseline2). (C) For each voxel, the frequency band(s) that showed significant power increases across time were selected and averaged. (D) The spatially normalized power change values at each voxel and at each time window were averaged across subjects and (E) statistically thresholded with statistical non-parametric mapping (SnPM).
Conditions 1–4 (Table 1
) were presented in 4 recurring blocks of 25 trials each, with a possibility to rest between the blocks as long as considered necessary by the subject. Trials of the Free condition were randomly interleaved with the other conditions in order to prevent the subjects from making their decision before the stimuli were actually presented. In addition, subjects were instructed to make their choices spontaneously in each Free trial rather than according to preselected patterns, and to press each button overall in about 50% of cases. Incorrect responses given in conditions 1–3 were excluded from further analysis. The sequence of digits presented in each condition was randomized for each subject by a computer.
Behavioral Analyses
The response latency was determined for each trial. The randomness of the left vs. right choices was assessed by calculating the normalized entropy U of all sequences of 5 consecutive responses occurring within the total of 100 responses of a condition, according to the formula
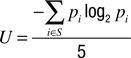
where S designates all sequences of 5 left/right responses produced by the subject, and p is the probability of each combination i (Hopkinson and Neuringer, 2003
). The resulting U-values are bound between 0 and 1, with higher values indicating a higher degree of randomness.
Functional Imaging with MEG
Figure 1
shows a flowchart of the applied analysis procedures. Neural activity during decision making was reconstructed in space and time by using a time-frequency beamformer technique (Dalal et al., 2008
). An adaptive spatial filter (Sekihara et al., 2001
, 2002
, 2004
, 2005
;Vrba and Robinson, 2001
) was used to estimate, at each volume element (voxel) in the brain, the mean oscillation power change across trials between a given active and a baseline time window and within a given frequency band. Separate filter weights were calculated for each time window of 200 ms duration and for each frequency band. Active windows were shifted in overlapping steps of 50 ms from 0 to 1000 ms after option presentation, and each was compared to a baseline period ranging from 200 to 0 ms before presentation of the options, during which the participants attended to a central fixation cross. A power increase in the frequency bands 60–90, 90–120, and 120–150 Hz was used as an index of neural activation. Lower frequency bands (8–30 Hz) were explored in single subjects but not used for the main analyses, because the polarity of power changes was ambiguous in these bands. Whereas central and parietal areas showed activation induced power decreases up to the gamma band, activation in frontal and temporal areas was sometimes associated with power increases in the beta band. Moreover, the neighboring frequency bands 30–60 and 150–180 Hz were found to yield no additional information in the average maps of all subjects and were therefore not included in the main analyses. A multiple spheres head model was used. Based on the coregistered structural MRI, individual data was spatially normalized to standard MNI (Montreal Neurological Institute) voxel space at 5 mm resolution.
Frequency bands. Since there was considerable inter-subject variability in the frequency bands that were activated at a given voxel, all 3 high-gamma bands analyzed were combined to a single time-series as follows. For each subject, the time-series of each frequency band at each voxel were tested against the null-hypothesis of 0 power change by using right-tailed t-tests for one sample. Parametric statistical tests were chosen for this analysis step, since the log-transformed power change values at a given voxel of a given subject were approximately normally distributed. Right-tailed hypotheses were used, since we statistically tested for activations in the combined 3 frequency bands and therefore had to ensure that all bands showed power changes with the same polarity. Left-tailed hypotheses would have tested for greater activations during the baseline time window, which was not the interest of our study. A Bonferroni correction was applied at each voxel and time window to correct for testing multiple frequency bands (a correction for multiple voxels and time windows is applied separately at a later stage, see below). Then, the mean power change of frequency bands showing significant high-gamma activity was calculated for each voxel and at each time window. Voxels that did not have significant power changes in any frequency band were set to 0. This method yielded comparable results as when calculating the mean of all frequency bands and as when using only one broad high-gamma frequency band. However, the statistical method introduced here was more sensitive, especially at central and parietal brain regions where beta-desynchronization sometimes extended up to the high-gamma band and interfered with high-gamma activity.
Analysis across subjects. The significance of the obtained time-series of power change in all high-gamma frequency bands was tested across subjects with statistical non-parametric mapping (SnPM) (Singh et al., 2003
). SnPM does not depend on a normal distribution of power change values across subjects, and allows correcting for the familywise error of testing at multiple voxels and time windows. At each time window, average and variance maps across subjects were calculated, and the variance maps were spatially smoothed with a 20 × 20 × 20 mm Gaussian kernel. From this, a pseudo-t statistic was obtained for each voxel and time window. In addition, a distribution of pseudo-t statistics was also calculated from 2N permutations of the original N datasets (subjects). Each permutation consisted of two steps: inverting the power change values of some subjects (with 2N possible combinations of negations), and finding the current maximum pseudo-t value among all voxels and time windows. Instead of estimating the significance of each non-permuted pseudo-t value from an assumed normal distribution, it is then calculated from the position within the distribution of these maximum permuted pseudo-t values. The comparison against maximum values effectively corrects for the familywise error of testing multiple voxels and time windows. Power change values were thresholded at a corrected p < 0.05.
Contrasts between conditions. A statistical comparison between conditions was performed by subtracting the power change values at each voxel, time window, and frequency band. This corresponds to a double contrast (active1 – baseline1) – (active2 – baseline2). The difference maps were then subjected to the same statistical procedure as used for the activation maps.
Software. Spatial normalization was done with the toolbox SPM2 for Matlab (http://www.fil.ion.ucl.ac.uk/spm/software/spm2/). The algorithms for SnPM were adapted from the toolbox SnPM3 for Matlab (http://www.sph.umich.edu/ni-stat/SnPM/). All analysis algorithms are implemented in our Matlab toolbox NUTMEG (Dalal et al., 2004
), which can be freely downloaded at http://bil.ucsf.edu. Three-dimensional renderings of the activation maps were created with the freely available program mri3dX written by K. D. Singh (http://imaging.aston.ac.uk/mri3dX/).
Data Driven Region-of-Interest (ROI) Analysis
Spatiotemporal activation peaks within the 4-dimensional, averaged, and statistically thresholded activation maps were defined by repetitively moving the center of an observation sphere with 10 to 20 mm radius to the maximum value within the sphere’s boundaries and within the entire time range until the sphere remained stable. Peaks of all 5 conditions were then manually assigned to common ROIs, if they were located in the same functional area and within a radius of less than 20 mm. The center of a ROI was defined by the centroid of all its peak locations, and the radius was defined as 5 mm greater than the distance from the center to the farthest peak of a given ROI (15 mm at minimum). The neural activity of a ROI was calculated as the mean power change in dB across its voxels.
Additional Statistical Analyses
A one-way ANOVA was used to test response latencies, U-values, and ROI time-series for differences between conditions. The statistical probabilities of differences between ROI time-series were corrected for testing multiple time windows with a false discovery rate of 10% (Genovese et al., 2002
).
Behavioral Data
The mean response time of all subjects and of all 5 experimental conditions is shown in Table 1
. There was a significant difference between conditions [F(4,45) = 8.0, p < 0.0001], but in a pairwise comparison only the Numerical condition differed significantly from other conditions (p < 0.05, Tukey-Kramer HSD). The left/right responses showed a high degree of randomness for all conditions (U-values >0.9, Table 1
), with no significant difference between conditions [F(4,45) = 1.5, p = 0.21].
Spatiotemporal Reconstruction of Neural Activity
Active brain areas (relative to baseline) and their time courses were reconstructed from increases in MEG oscillations in the high-gamma range, between 60 and 150 Hz, using an adaptive spatial filtering technique (Sekihara et al., 2001
, 2002
, 2004
, 2005
; Vrba and Robinson, 2001
). All decision conditions elicited complex spatiotemporal brain activation patterns, the dynamics of which are shown in real time in Video S1a–e (supplementary material can be found at http://www.mrsc.ucsf.edu/∼aguggis/frontiers/suppinfo.html). Video S2a–e presents the same data in 30× slow motion, and with the anatomical locations of all spatiotemporal activation peaks labeled. Figure 2
gives a static overview of the cortical areas with significant activations during different decision stages and in each of the 5 conditions, and shows that, as expected, numerous and spatially distributed neural systems are required for choosing tasks, including primary and associative visual areas, prefrontal areas, the limbic system, the parietal cortex, the motor and premotor cortex, and the SMA. The spatiotemporal pattern of neural activity during decisions was also visualized by defining ROIs from activation peaks in each of the conditions, and displaying the resulting average time-series of each ROI in Figure 3
.
Figure 2. Spatiotemporal reconstruction of synchronized high-gamma activity during choosing. Average activation maps of 10 healthy subjects are superimposed on a 3-dimensional template brain. Colored brain areas show the reconstructed sources of significant (corrected p < 0.05, SnPM) high-gamma activity during the 5 task conditions. Rows represent different stages in the decision process.
Figure 3. Time course of induced high-gamma power in regions of interest (ROI). ROIs were derived from spatiotemporal activity peaks in all 5 choosing conditions. Occipital areas showed the first activity peaks, but were also reactivated later in the decision process. The DLPFC contributed to preference and memory judgments during the option evaluation stage of choosing. Activity in cortical midline structures was most prominent during the Preference condition, and progressed over time from posterior to anterior parts. Parietal regions showed consistent activity peaks during the intention stage of choosing, with the intentions being mediated by a distinct set of posterior parietal and superior occipital subregions for each condition. Activity in the bilateral MC started to build up immediately after visual presentation of the options, and peaked roughly 50 ms before the average behavioral button press latency. High-gamma power changes in the SMA were of similar intensity for all conditions during the option evaluation stage of choosing, but became significantly greater in the Preference and Free condition than in the other conditions during and after action execution.
All conditions first induced activation in the medial occipital cortex (MOC) of Brodmann area (BA) 17/18, peaking 200 ms after visual presentation of the options. With the exception of the Numerical condition, all conditions also showed activation around the temporo-occipital junction (TOJ; BA 19, 37, 39, and 22) and the occipito-parietal junction (OPJ; BA 18, 19, and 7) peaking within 250 ms after stimulus presentation. In contrast, the Numerical condition elicited an early activation of the right dorsolateral prefrontal cortex (DLPFC; BA 6). Additional early activation peaks (∼200 ms) could be found in the right superior parietal lobule (SPL; BA 7) during Memory judgments, and in the left supraparietal lobule/intraparietal sulcus (SPL/IPS; BA 7) as well as in the left cerebellar (Cb) hemisphere during Free decisions.
Subsequently, various brain regions showed an activity peak during a time window between 250 and 500 ms after option presentation, depending on the choice condition, suggesting a second stage of choice processing. All conditions elicited activity peaks in supplementary motor area (SMA; BA 6) and right motor cortex (MC; BA 4 and 6), as well as reactivations of TOJ and OPJ, with no significant intensity differences between conditions in these regions during this stage. Moreover, additional specific activations were observed in the Visual, Numerical, Memory and Preference conditions. In the Visual condition, the superior parietal lobule/intraparietal sulcus (SPL/IPS) was activated on both sides; in the Numerical condition, activations were seen only in the left IPS. The Explicit Memory condition was associated with activation in right DLPFC (BA 6 and 8). During the Preference condition, activations were observed in cortical midline structures progressing from medial parietal cortex (MPC; BA 7 and 31) to supragenual anterior cingulate cortex (SACC, BA 24 and 32; see Video S2d), as well as in left DLPFC (BA 6 and 8). The Free condition showed reactivations of the left cerebellar (Cb) hemisphere and the left SPL, as well as activation of the right posterior inferior parietal lobule (IPL; BA 39).
In a third stage, (re-)activations of parietal and occipital brain areas peaking between 500 and 600 ms after presentation of the options occurred, with each condition being associated with a distinct subset of parieto-occipital regions (Figure 2
, Video S2a–e): the Visual condition with the MPC (BA 7) and the left IPL (BA 40); the Numerical condition with the MPC (BA 7); the Memory condition with the right IPL (BA 40), left SPL, and left OPJ; the Preference condition with the left IPL (BA 40), left SPL, and bilateral OPJ; and the Free condition with the left IPL (BA 40) and the right SPL/OPJ (BA 7 and 19). Furthermore, the Cb showed activation peaks during this time window in all conditions. During preference judgments, the activity in the cortical midline structures migrated further in an anterior direction, and induced a peak in the pregenual anterior cingulate cortex (PACC; BA 32), as visualized in Figure 3
.
Finally, activity in the left (contralateral) premotor and primary MC that has been building up during the second and third processing stages converges in the precentral gyrus (BA 4) and peaks between 550 and 650 ms after stimulus presentation in all conditions, about 50 ms before the average button press latency of the subjects (Table 1
). During and immediately after the button press, the Preference and Free conditions were additionally associated with reactivations of the SMA, the left SPL/IPS, and the SACC (Figure 3
), which were significantly greater than in the other conditions (p < 0.05, corrected). Ventromedial prefrontal cortex (VMPFC; BA 11) was significantly activated only during the Free condition, and only after the activation peak in the motor cortex (Figures 2 and 3
, Video S2e).
The MNI coordinates of all spatiotemporal activation peaks are indicated in Table S1 (http://www.mrsc.ucsf.edu/∼aguggis/frontiers/suppinfo.html).
Contrasts
In order to better isolate the neural networks being specifically involved during the different choosing conditions, we additionally performed four planned statistical comparisons between condition pairs. The Numerical, Memory, Preference, and Free conditions were each contrasted to the Visual condition, since we hypothesized that the former four conditions each relied on one or several neural systems in addition to visual shape processing required for the Visual condition (Table 1
). Figure 4
displays the location and time course of activations that were specific to each condition. The dynamics of the activation differences are shown in Video S3 (real time) and S4 (30× slow motion with labels). Numerical choices induced a specific activation of the left IPL (BA 40) 250 ms after option presentation, as well as of the left IPS/OPJ (BA 7/19) and right DLPFC (BA 9) 450 ms after option presentation. Explicit memory retrieval and memory judgments were associated with specific sequential activations of right DLPFC (BA 8), left DLPFC (BA 8), and the right IPL (BA 40). The contrast Preference > Visual condition showed significantly greater high-gamma activity in the SACC (BA 24) and left DLPFC (BA 8) peaking 450 ms after stimulus onset, and in the right SPL (BA 7) peaking 650 ms after stimulus onset. Finally, the Free condition was associated with a significantly larger activation of, in chronological order, the left IPL (BA 40), the left DLPFC (BA 8), the right posterior IPL (BA 39), and the left Cb, than the Visual condition. In contrast, the Visual condition depended on a greater activation of the right IPS (BA 7), the left fusiform gyrus (FusG; BA 37), and left lateral orbitofrontal cortex (OFC; BA 11) than the Free condition.
Figure 4. Comparison of high-gamma activity between choosing conditions. The 3-dimensional maps show areas with significant differences in high-gamma activity between condition pairs of 10 healthy subjects. The line plots display the time course of power differences in the region marked with corresponding color.
The MNI coordinates of regions with significant contrast between condition pairs are listed in Table S2.
In an attempt to obtain a more integrated and dynamic picture of the neural processes underlying visuo-motor choosing tasks in humans, this study used MEG recordings of neural oscillations in the high-gamma frequency band and advanced signal analysis techniques to reconstruct the activity of the entire cortex with high resolution in both space and time. This approach has the potential limitation that the inference of brain sources from MEG data has no unique solution and depends on assumptions that introduce uncertainty about the correctness of the results. However, previous studies have demonstrated the reliability of the applied signal analysis techniques (Dalal et al., 2008
; Sekihara et al., 2005
), and we will show in the following sections that the location of brain activations found in this study are largely in accordance with previous fMRI and PET studies, which confirms the validity of our results. Moreover, the improved temporal resolution allowed us to disambiguate the specific role of different brain areas, particularly of subregions in parietal, frontal, and limbic cortex, and to determine how and when sensory information, memories, and preferences are integrated to decisions and actions.
Timing and Interpretation of Choice-Related Neural Activity
Choosing requires an integration of multiple sensory inputs to a single output of intention and action. “Cartesian” models of decision making have long assumed that decisions are processed sequentially, with a sensory representation of the options occurring in a first stage, and mental/neural processing as well as motor output occurring in successive stages (Glimcher, 2003
). The pattern and time course of cerebral activity observed in our study can indeed be conceptually divided into 4 distinct but overlapping stages (rows in Figure 2
). It is, however, noteworthy that most brain regions did not show a monophasic time course of activation, but rather complex patterns of recurring activation peaks, which is in accordance with previous studies (Periáñez et al., 2004
). For instance, activation of sensory (visual) networks was not limited to the early perceptive stage of choosing, but could be observed throughout the entire decision process including during the button press. Moreover, the high-gamma power increase in the MC and SMA also started several hundred milliseconds before the actual finger movement, which is in agreement with previous Bereitschaftspotential studies (Kornhuber and Deecke, 1965
; Libet et al., 1982
; Shibasaki and Hallett, 2006
), and which suggests that motor areas actively participate in the perceptive decision stages that precede an action rather than being merely responsible for its execution. Indeed, recent data has shown that perception of action-related stimuli activates both sensory and motor networks in parallel (Lahav et al., 2007
; Pizzamiglio et al., 2005
; Tettamanti et al., 2005
). Thus, although the neural processing of decisions occurs in sequential cognitive steps, sensory, motor, and associative networks seem to activate mostly in parallel (Freeman, 1997
; Varela et al., 1992
).
We observed four stages of choice-related brain activation. During the first stage, primary and associative visual cortices showed activation peaks, suggesting a perception and processing of the sensory input (“stimulus processing stage”). Similarly as in previous event related potential/field (ERP/ERF) studies (Poghosyan and Ioannides, 2007
), we observed a progression of activity from the MOC around V1 to associative visual areas (Videos S1 and S2). It is, however, noteworthy that high-gamma power peaks in the visual cortex were reached only about 200 ms after presentation of the visual stimulus, i.e., considerably later than the activity peaks observed with evoked potentials/fields in humans (Poghosyan and Ioannides, 2007
; Poghosyan et al., 2005
) and cortical recordings in monkeys (Lamme and Roelfsema, 2000
). The gamma activity during this stage therefore seems to include both early and late visual processes.
In the second stage, we observed neural activation peaks in a wide variety of brain areas, depending on the experimental condition, which we interpret as an evaluation of the value of each option according to different choosing criteria (“option evaluation stage”). These criteria include external features as well as memories and internal personal preferences.
In the third stage, we found activation peaks in parietal brain areas, which, according to previous studies, mediate processing of intentions and action planning (Andersen and Buneo, 2002
; Chapman et al., 2007
; Connolly et al., 2003
), thus suggesting that the available information is integrated and actions are prepared during this step (“intention stage”). While it is well established that different subregions of the posterior parietal cortex are dedicated to the planning of different actions, e.g., eye movements, reaching movements, or grasping movements (Andersen and Buneo, 2002
; Connolly et al., 2003
), we also observed activations in a distinct set of subregions in posterior parietal cortex and OPJ for each choosing condition (Figure 2
), even though the prepared actions as well as the hand positions were exactly the same for all of them. This suggests a functional specialization of the parietal cortex depending not only on action parameters and the sensory representation of spatial coordinates (Andersen and Buneo, 2002
), but also on the cognitive context.
Finally, in the last stage, activation peaks occurred in the contralateral sensorimotor cortex corresponding to the execution of a button press (“action execution stage”).
Value Constrained Decisions
Conditions 1–4 of our study represented rational decision types, which differed with regard to the neural systems required to choose, whereas the perceived stimuli (single digit numbers) and the actions (button press) were kept the same (Table 1
). In contrast to the Visual and Numerical conditions, the Explicit Memory and Preference conditions depended on internal neural representations of personal preferences and memories in addition to the processing of external stimuli. Thus, our setup enabled us to study the neural networks that are primarily responsible for internally motivated as compared to externally cued choices.
Our results show essentially the same spatial activation pattern as previous fMRI and PET studies, but additionally demonstrate that memories and preferences are processed during the same early decision stage as information coming from external sensory stimuli. The Visual condition was associated with early activations in the TOJs (particularly the left FusG; see Figure 4
), which are thought to mediate shape recognition (Fujimaki et al., 1999
; Fulbright et al., 2003
; Op de Beeck et al., 2000
), with the peak activity occurring between 250 and 400 ms after stimulus onset (Figure 2
). The Numerical condition induced activity peaks within 400 ms after presentation of the options in right DLPFC and left IPL (Figures 2 and 4
), which have previously been related to numerical processing (Fulbright et al., 2003
; Pinel et al., 1999
; Zago and Tzourio-Mazoyer, 2002
). Both external conditions (Visual and Numerical) also showed early activations in the SPL/IPS on both sides, which fits well with the notion that these cortical areas are important in determining stimulus magnitude (Cohen Kadosh et al., 2005
; Fulbright et al., 2003
; Kaufmann et al., 2005
) and for encoding the relative value of each option (Platt and Glimcher, 1999
; Sugrue et al., 2004
). Similarly, bilateral activity in DLPFC, which is related to explicit memory retrieval (Buckner and Koutstaal, 1998
; Schott et al., 2005
), and in cortical midline structures, which mediate preference judgments (Gusnard et al., 2001
; Northoff and Bermpohl, 2004
; Northoff et al., 2006
; Zysset et al., 2002
), peaked in the same time range between 250 and 400 ms after stimulus presentation (Figures 3 and 4
). Thus, our results demonstrate that memories and preferences influence the decision in a similar manner as choice-related external cues, with all of them being evaluated with regard to the relative value of each option by specialized brain regions during a time window of 250 to 500 ms after presentation of the options.
Activations in cortical midline structures during preference judgments were found to begin in MPC and then to migrate in an anterior direction along the cingulate gyrus up to its pregenual part (Figures 2 and 3
). This time course suggests that the posterior and supragenual parts of cortical midline structures might be mostly concerned with evaluation of options with regard to self-related internal values, whereas the pregenual part could be more involved in preparation and execution of self-related actions.
Unconstrained Internal Decisions
Although preference judgments allow us “to act as we will and to act differently if we had willed differently” and thus fulfill John Locke’s influential definition of freedom (Locke, 1975
), it is generally agreed that the “will” for an action, i.e., the preferences underlying preference judgments, are, at least in part, (pre-)determined by environmental conditions and past experience (Montague et al., 2006
; Strawson, 1986
; Sugrue et al., 2004
), and hence not freely chosen. In contrast, the “Buridan’s ass” paradigm used for our Free condition allowed us to study choices that were completely unconstrained by external cues or predetermined internal preferences, and depended only on current internal considerations. In order to further verify that the study participants indeed made their decisions independent of systematic influences for this condition, we analyzed the randomness of the response sequences produced by the participants. The observed values of normalized entropy were high and statistically not distinguishable from the sequences observed in the rational conditions, which in turn depended on computer-randomized stimulus sequences. The behavioral data thus suggested that the decisions in this condition were indeed spontaneous and unconstrained. We can, however, not exclude the possibility that – despite instructions to choose spontaneously in each trial – some subjects applied predefined rules in this condition, which escaped the randomness measure applied here.
The results in Figure 4
, Video S3d, and Video S4d show that the left cerebellar hemisphere and the IPL on both sides were the most prominently activated areas during unconstrained internal compared to externally cued decisions, which is in agreement with a previous PET study using a similar experimental paradigm [“None” condition in (Deiber et al., 1996
)]. The Cb and the IPL were also found to be important during decision making under uncertainty and probabilistic inference tasks (Blackwood et al., 2004
; Elliott et al., 1999
), which are different from our paradigm in that our Free condition required choices among alternatives with known equal value rather than among alternatives with different but uncertain value. The common activation of the Cb and IPL in both choice situations therefore suggests that they might be involved in the internal generation of choice and intention in the absence of sufficient information from external sources, past experience, and internal preferences. This conclusion is also supported by their activation time courses with peaks during the “evaluation” and “intention” stages.
In order to exclude the possibility that the participants made their unconstrained decisions before seeing the options, we randomly interleaved all trials of the Free condition within the blocks of the other conditions. The specific activity observed in this condition could therefore also partly reflect task switching and first trial effects (Konishi et al., 1998
, 2001
; Periáñez et al., 2004
; Rubinstein et al., 2001
). However, in the absence of corresponding behavioral evidence or imaging data, we consider this possibility unlikely. Task-switching effects are typically associated with longer response latencies. We did not observe greater response times in the Free condition than in most other conditions (Table 1
), nor in the trials following Free tasks [t(9) = 0.24, p = 0.82]. Moreover, set shifting and first trial effects have consistently been reported to induce robust activity in the bilateral inferior frontal gyri (Konishi et al., 1998
, 2001
;Periáñez et al., 2004
), which was not the case in our Free task condition. On the contrary, more activation could be observed in the Visual condition than in the Free condition (Figure 4
), although it required less task switching.
At variance with studies of decision making under uncertainty (Bechara et al., 1997
; Blackwood et al., 2004
; Elliott et al., 1999
), we did not find a significant activation of VMPFC during the decision stages of unconstrained internal choices, and only a weak activation during the action execution stage. This supports the notion that VMPFC is involved in probabilistic reasoning and outcome monitoring (Blackwood et al., 2004
; Elliott et al., 1999
), which are important for rationally uncertain but less for rationally indeterminate decisions.
The greater activation in left DLPFC during early stages of rationally inderminate decisions most likely reflects working memory processes required for creating left-right response sequences (Lau et al., 2004b
).
It is a matter of long-standing debate whether “Buridan’s ass” type decisions as studied here represent manifestations of a “will power” (Hodgson, 1999
; O’Connor, 1995
; Reid, 1969
) or merely (pseudo-)random processes (Strawson, 1986
). Our results do not resolve this issue. However, the finding that the generation of unconstrained internal choices is associated with an activation of specialized brain regions cannot easily be explained by random fluctuations in value encoding networks, but rather favors models that assume specialized neural networks responsible for internal choosing and reasoning.
Decision-Related Preparatory Activity
In agreement with the notion that SMA subregions are important for action preparation, we found an activation of the SMA during the “evaluation” and “intention” stages in all conditions. However, whereas previous studies have observed a greater SMA activation during preparation of internally generated movements (Deiber et al., 1991
, 1996
; Shibasaki and Hallett, 2006
) and during preference judgments (Lau et al., 2004b
) than during externally cued movements and choices, we did not observe a significantly greater activation of the SMA in the Free and Preference conditions than in the other conditions before action execution. We argue that this discrepancy is due to different experimental paradigms used in different studies, as well as to different temporal resolutions of the applied analysis techniques.
The internal movement generation and selection paradigms used in most previous studies did not involve any sensory processing of options and sensory-motor integration, but depended only on motor planning and execution, whereas the externally cued conditions required an evaluation of sensory cues. Similarly, all choosing conditions studied here required that the subjects inspected and integrated the visual appearance of the options before preparing an action. The different structures found to be important in our and previous studies could therefore reflect differences in sensory-motor integration. The SMA seems to be responsible for general action preparation and execution, and, specifically, for motor selection and movement initiation in the absence of sensory processing. Conversely, if an evaluation of sensory information is necessary for the choice, then the parietal cortex and the Cb seem to be more suitable for unconstrained internal decisions, possibly due to their abundant structural connections with both sensory and motor areas.
Rather than before the action, we found a reactivation of the SMA, the SACC, and of parietal areas that was specific and limited to the Preference and Free conditions (Figure 3
), during and after action execution. A similar differential time course of the SMA has also been reported in a recent time-resolved fMRI study [Figure 5
in Cunnington et al. (2006
)]. In agreement with Cunnington et al., we therefore suggest that significant contrasts between preference judgments and externally cued decisions in the SMA (Lau et al., 2004b
) are mostly due to differences occurring during and after the corresponding action.
Postdiction of Internal Decisions
The greater activation of the SMA, SACC, and parietal areas during and after execution of internally generated actions suggests that an important feature of internal decisions is specific neural processing taking place during and after the corresponding action. This retrospective assessment might be related to evaluation of causality and the awareness that the preceding action was internally generated. Previous studies have indeed shown that the SMA, SACC, and parietal regions are important for reporting the subjective timing of internally produced intentions and actions (Lau et al., 2004a
, 2006
; Sirigu et al., 2004
). Moreover, the percept of an event was found to be a function of what happens in the ∼80 ms after the event (Eagleman and Sejnowski, 2000
), and transcranial magnetic stimulation of the SMA after execution of spontaneous actions was reported to be capable of disturbing perception of the timing of intentions (Lau et al., 2007
). Therefore, awareness of intention timing seems to be fully established only after execution of the corresponding action, in agreement with the time course of neural activity observed here.
Deep Brain Structures
Decision making depends not only on the cerebral cortex, but is also mediated by deep brain structures such as the basal ganglia (Balleine et al., 2007
; Seymour et al., 2007
). Due to the greater distance of deep nuclei to the MEG sensors with resulting spatial blur and low signal-to-noise ratio, we are currently reluctant to use MEG based methods for the study of these structures. Moreover, the adaptive spatial filters used for source reconstruction tend to erroneously localize highly correlated and spatially distributed neural activity in the middle of the brain (Sekihara et al., 2002
, 2004
, 2005
). The significant activations in the thalamus and brain stem shown in Figure 2
could therefore represent artifacts introduced by the source reconstruction technique rather than true brain activity. On the other hand, we are convinced that the reconstructed sources in the depth of the cingulate gyrus are real, because they follow exactly the complex anatomical shape of the cingulate gyrus, and are specific to the Preference task condition (Figure 2
). Previous studies have also reported trustworthy MEG reconstructions of neural activity in the amygdala (Luo et al., 2007
). Future work will have to assess the anatomical limits of MEG techniques for reconstructing deep brain sources.
The authors declare that the research was conducted in the absence of any commercial of financial relationships that could be construed as potential conflict of interest.
A.G.G. was supported by Swiss National Fund grant PBBEB-109055, S.S.D. was supported by NIH grant F31 DC006762, and S.S.N. was supported by NIH grants R01DC004855 and R01DC006435. We would like to thank the reviewers for helpful comments.
Videos S1–S4 and Tables S1 and S2 can be found online at: http://www.mrsc.ucsf.edu/∼aguggis/frontiers/suppinfo.html
Dalal, S. S., Guggisberg, A. G., Edwards, E., Sekihara, K., Findlay, A. M., Canolty, R. T., Berger, M. S., Knight, R. T., Barbaro, N. M., Kirsch, H. E., and Nagarajan, S. S. (2008). Five-dimensional neuroimaging: Localization of the time-frequency dynamics of cortical activity. Neuroimage (in press).