1
Amsterdam Center for the Study of Adaptive Control in Brain and Behavior, Department of Psychology, University of Amsterdam, Amsterdam, The Netherlands
2
Department of Physiology, University of Arizona, Tucson, AZ, USA
3
Cognitive Neuroscience Group, Department of Psychology, University of Amsterdam, Amsterdam, The Netherlands
The medial prefrontal cortex (MFC) is critical for our ability to learn from previous mistakes. Here we provide evidence that neurophysiological oscillatory long-range synchrony is a mechanism of post-error adaptation that occurs even without conscious awareness of the error. During a visually signaled Go/No-Go task in which half of the No-Go cues were masked and thus not consciously perceived, response errors enhanced tonic (i.e., over 1–2 s) oscillatory synchrony between MFC and occipital cortex (OCC) leading up to and during the subsequent trial. Spectral Granger causality analyses demonstrated that MFC → OCC directional synchrony was enhanced during trials following both conscious and unconscious errors, whereas transient stimulus-induced occipital → MFC directional synchrony was independent of errors in the previous trial. Further, the strength of pre-trial MFC-occipital synchrony predicted individual differences in task performance. Together, these findings suggest that synchronous neurophysiological oscillations are a plausible mechanism of MFC-driven cognitive control that is independent of conscious awareness.
Throughout life, we try to improve our performance on goal-directed tasks, in part by learning from our previous mistakes. Monitoring our actions for errors and fine-tuning performance accordingly is a key function of the cognitive control system, a neural network in which the medial prefrontal cortex (MFC) plays a prominent and crucial role (Ridderinkhof et al., 2004a
; Carter and van Veen, 2007
). Myriad studies spanning several species have implicated the MFC in action monitoring, conflict detection, error signaling, and reinforcement learning (Nieuwenhuis et al., 2004
; Carter and van Veen, 2007
). Generally, these studies show that MFC activity increases following errors or negative feedback (Ridderinkhof et al., 2004a
,b
). MFC activity also predicts adjustments in performance or decision-making in the subsequent trial (Gehring et al., 1993
; Ridderinkhof et al., 2003
; Debener et al., 2005
; Cohen and Ranganath, 2007
; Gentsch et al., 2009
), although this pattern is not always observed (Gehring and Fencsik, 2001
; Nieuwenhuis et al., 2001
).
And yet, little is known about the neurobiological mechanisms by which processes in the MFC can lead to adjustments in performance. Researchers using fMRI (Egner and Hirsch, 2005
) have revealed that activity in regions involved in task-relevant stimulus processing is enhanced following errors or conflicts. This is in line with a large body of evidence revealing preparatory top-down modulatory effects in visual cortex when attentional demands are increased (Kastner and Ungerleider, 2000
; Pessoa et al., 2003
). We hypothesize that this top-down control is realized through synchronized electrophysiological oscillations (Cavanagh et al., 2009
). Oscillations reflect rhythmic fluctuations in population-level dendritic activity and action potentials, and accompany memory, decision-making, and other cognitive processes (Klimesch, 1999
; Engel et al., 2001
; Buzsaki and Draguhn, 2004
; Cohen et al., 2007
). Further, synchronized oscillations across brain regions have been linked to conditioning and choice behavior (Pesaran et al., 2008
; Sauseng and Klimesch, 2008
; Cohen et al., 2009
; Popescu et al., 2009
). Here we examined whether long-range neurophysiological oscillatory synchrony is a plausible mechanism by which the MFC engages top-down control over sensory processing following errors.
The adaptive change in cognitive control that occurs after errors has traditionally been conceptualized as an effortful process, requiring conscious awareness that an error was made or negative performance feedback was given. However, conscious awareness may not be necessary for all aspects of cognitive control. Indeed, non-consciously perceived conflict or error signals modulate activity in the motor system (Dehaene et al., 1998
) and prefrontal cortex (Nieuwenhuis et al., 2001
; Hester et al., 2005
; Endrass et al., 2007
; Klein et al., 2007
; Ursu et al., 2009
). Moreover, some aspects of high-level cognitive control, such as response inhibition and task-switching, may also occur in absence of conscious awareness (Lau and Passingham, 2007
; van Gaal et al., 2008
). However, such unconscious processes are thought to be ephemeral, lasting only a few hundred milliseconds (Greenwald et al., 1996
; Rossetti, 1998
; Dehaene and Naccache, 2001
). The extent to which unconsciously made errors can engage top-down control remains unknown.
We recorded EEG from human subjects while they performed a visually signaled Go/No-Go task, in which one half of the No-Go cues were presented in a way that evaded conscious awareness. We examined oscillatory phase synchrony – a measure of frequency-band specific functional connectivity – between the MFC and occipital cortex (OCC) on correctly responded Go trials that followed conscious errors, unconscious errors, or other correct Go trials. Enhanced MFC–OCC synchrony occurred on trials following unconscious and conscious errors, and predicted subjects’ behavioral performance. Pre-trial synchrony was dominated by an MFC → OCC directional flow; OCC → MFC directed synchrony was maximal following stimulus onset and was unrelated to errors in the previous trial. These findings suggest that the MFC uses synchronized oscillations to entrain sensory regions following errors to improve sensory processing. Further, these findings demonstrate that top-down control over sensory cortex occurs even when errors are made unconsciously.
Participants
Subjects were 15 right-handed undergraduate psychology students (14 female) at the University of Amsterdam, with normal or corrected-to-normal vision. Subjects gave written informed consent prior to participation, and the experiment was approved by the local ethical committee. Non-overlapping results from these subjects have been reported elsewhere (van Gaal et al., 2008
).
Stimuli
Stimuli were presented on a gray box (59.1 cd/m2, visual angle of 3.78°) against a black background (2.17 cd/m2) at the center of a 15-inch BenQ TFT monitor with a refresh rate of 60 Hz. The monitor was placed at a distance of approximately 90 cm in front of the participant, so that each centimeter subtended a visual angle of 0.64°. Participants were told that they would see a black annulus (the Go signal, 2.17 cd/m2, visual angle of 1.30°, duration 100 ms) and that they would have to respond as quickly as possible by pressing a button with their right index finger. Participants were instructed to withhold their response when they perceived a gray circle (the No-Go signal, 41.85 cd/m2, visual angle of 0.60°, duration 16.7 ms) preceding the Go signal. The stimulus onset asynchrony between the No-Go signal and the Go signal was either 16.7 ms or 83 ms. The No-Go circle exactly fitted within the Go annulus, which typically results in efficient metacontrast masking. Trial duration was jittered between 1400 and 2200 ms (in steps of 200 ms), randomly drawn from a uniform distribution, rendering the presentation of the stimuli temporally unpredictable. Participants were not informed about the presence of the unconscious No-Go cue and received no feedback about performance on these trials during testing. The experiment was programmed with Presentation (Neurobehavioral Systems, Albany, USA). There were seven blocks of 200 trials each (140 Go, 30 conscious No-Go, and 30 unconscious No-Go). The average number (and standard deviation) of trials included in the EEG analyses for Go following Go trials, Go following conscious errors, and Go following unconscious errors, was 654 (34), 53 (15), and 142 (8). The minimum number of trials for any subject was 29.
Experiment Conditions
We distinguished three types of trials: Go trials following correct Go trials (“Go”); Go trials following trials that contained a visible No-Go cue, but in which subjects committed a response (“conscious errors”); and Go trials following trials that contained a masked No-Go cue, but in which subjects committed a response (“unconscious errors”) (see Figure 1
A). Note that all trials included in the present analyses are Go trials, and that there was a response on the previous trial; differences among conditions lie solely in whether the previous trial contained a conscious, nonconscious, or no No-Go cue. Further, because the unconscious No-Go cue on the previous trial did not reach subjective awareness, Go trials following other Go trials are also identical to Go trials following unconscious errors in terms of the subjective experience. In other words, all trials included in these analyses, and the trials that preceded those, contained a stimulus and response.
Figure 1. Overview of task design and behavioral results. (A) Visual representation of trial events for the three conditions. Note that all data reported here are from correctly responded Go trials (“trial n”) in which the previous trial also contained a response, separated according to whether the previous trial contained a consciously visible No-Go cue (conscious error, top), an unconsciously presented No-Go cue (unconscious error, middle), or a Go cue (correct go, bottom). (B) Subjects were significantly slower on Go trials following unconscious and conscious errors compared to Go trials following correct Go trials, over two behavioral testing sessions (1, 2), and the EEG recording session (3).
Visibility of No-Go Signals
To test whether subjects were truly unaware of the unconscious No-Go signals, they performed two discrimination tasks after the main task. In both tasks, the stimulus display and trial timing were identical to the main task. In the first yes/no detection task, the number of trials per condition was the same as one block in the Go/No-Go task (140 Go trials, 30 unconscious No-Go trials, 30 conscious No-Go trials, pseudo-random order). However, subjects were instructed to press a button only when they perceived a No-Go signal. The hit rate of 2% on unconscious No-Go trials did not exceed the false alarm rate of 0.8% on Go trials (the hit rate on conscious No-Go trials was 93.3%). In the second, more conservative, forced-choice discrimination task (2 blocks of 100 trials), subjects were informed about the presence of a very brief No-Go signal shortly before the Go signal on some trials. No participants reported awareness of these No-Go signals during the Go/No-Go experiment. In this task, each block consisted of 50 unconscious No-Go trials and 50 Go trials (pseudo-random order). Participants were instructed to press the left button when they thought a brief No-Go signal preceded the Go signal and to press the right button when they thought this was not the case. Participants were told that in 50% of all trials, a Go signal was preceded by a No-Go signal and were instructed to consider this in their response. Participants were unable to discriminate between Go trials and unconscious No-Go trials (d’ = 0.05, mean percentage correct = 51%), as evidenced by chance-level performance (p > 0.2 for both discrimination tasks, see van Gaal et al., 2008
, for more details).
EEG Recording and Processing
EEG data were recorded at 256 Hz using a BioSemi ActiveTwo amplifier from 48 scalp electrodes and 4 peri-occular electrodes. All analyses were conducted in Matlab, using in-house written code supplemented by EEGLAB (Delorme and Makeig, 2004
) (independent components analysis and topographical plotting) and BSMART (Cui et al., 2008
) (spectral Granger causality estimates). Data were re-referenced off-line to the average of the activity recorded at the two ear lobes. All data were visually inspected, and trials containing artifacts were identified and removed. Blink artifacts were removed from the data using independent components analyses in EEGLAB.
One limitation of surface EEG is the spatial spread of large field potentials through volume conduction. This limits interpretability of inter-regional synchrony, because it is possible that strong synchrony results from the same deep sources projecting to multiple electrodes, and not to synchronous activity across spatially disparate brain regions. Current-source-density (CSD) transform acts as a high-pass spatial filter of voltage data, thus effectively subtracting volume-conducted common activity due to deep and/or distant sources. CSD therefore measures superficial cortical activity from the tissue lying directly underneath the electrode. The use of CSD transform has been validated for inter-regional synchrony (Srinivasan et al., 2007
) as well as in cognitive control (Allain et al., 2004
; Cavanagh et al., 2009
). Thus, EEG data were first CSD transformed (Kayser and Tenke, 2006
). We note that despite increased spatial selectivity, precise anatomical localization remains uncertain. For analyses with pooled data, unfiltered time-domain EEG data were averaged together from FC1, FCz, and FC2, and from O1, Oz, and O2. For convenience, we refer to these pooled electrodes as MFC and OCC, respectively. Pooling data across several electrodes has the advantage of increasing signal-to-noise ratio and minimizing any possible noise fluctuations in a single electrode. We also conducted all analyses using a single electrode (FCz and Oz); the patterns of results were the same as those reported here.
Phase Synchrony Analyses
Phase synchrony was computed by first extracting the phase angle time series from the data via complex Morlet wavelet convolution (Cavanagh et al., 2009
; Cohen et al., 2009
), and computing the magnitude of the average phase angle difference between two electrodes over trials at each time/frequency point. Data were first convolved with a family of complex Morlet wavelets, defined as
where t is time and f is frequency, which increased from 2 to 60 Hz in 50 logarithmically spaced steps. σ defines the width of each frequency band, and was set according to 4/(2πf). Power is defined as the modulus of the resulting complex signal Zt [real(zt)2 + imag(zt)2], and phase angle is defined as arctan[imag(zt)/real(zt)]. Inter-site phase synchrony (Lachaux et al., 1999
) measures the extent to which oscillation phase angle differences between electrode pairs are consistent over trials at each time/frequency point, and varies from 0 (completely random phase angle differences across trials) to 1 (identical phase angle differences across trials):
where n is the number of points, and ϕj and ϕk are the phase angles of electrodes j and k.

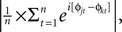
Statistics were conducted in two ways. First, we computed paired-sample t-tests on the differences between conditions in the 2–12 Hz band at each time point across subjects (Figure 2
B). This frequency band was selected based on the condition-averaged synchrony plot (Figure 2
B), and on the spectral Granger causality plots (Figure 5
A). Temporal regions were highlighted if each time point achieved a significance of at least p < 0.05, with at least 156 ms (40 samples) of contiguously significant p < 0.05 points. These analyses are mainly for illustration purposes. Results were confirmed through repeated-measures ANOVAs, in which average synchrony was taken from three temporal windows (first averaging from 2–12 Hz): −1200 to −300 ms preceding stimulus onset, 100 to 300 post-stimulus onset (MFC–OCC synchrony peaked at 200 ms; we refer to this time window as “peak synchrony”), and 500 to 1400 ms post-stimulus onset. These windows were selected, based on the timing of the experiment and on the synchrony results, to examine activity pre-trial, peak synchrony, and post-trial. Repeating the analyses using different time windows did not appreciably alter the results. Averaged data were entered into a 3 (condition) × 3 (time window) repeated-measures ANOVA. Greenhouse-Geisser correction for nonsphericity was used; however, unadjusted degrees of freedom are reported for readability.
Figure 2. Oscillatory phase synchrony between prefrontal and occipital cortices was significantly enhanced following unconscious and conscious errors. (A) Time-frequency plot of oscillatory phase synchrony averaged across all conditions demonstrates a “burst” of synchrony between a pool of medial frontal electrodes (MFC) and a pool of occipital electrodes (OCC) from around 2–12 Hz following the onset of the Go stimulus (data are converted to percent change from a −300 to −100 ms baseline at each frequency). (B) Oscillatory phase synchrony from 2–12 Hz plotted separately for each condition (values are the magnitude of the average projection vector over phase angle differences; see Materials and Methods). Phase synchrony was significantly stronger following unconscious and conscious errors compared to Go trials, and was significantly stronger following conscious compared to unconscious errors. Gray regions reflect time windows in which at least 156 contiguous ms (40 time points) survived a paired-samples t-test at p < 0.05 (see text for complementary results from ANOVAs).
Topographical (Whole-Head) Synchrony Analyses
In these analyses, phase synchrony was computed over trials between each possible electrode pair within a frequency band of 2–12 Hz, as described above. Here, we extracted phase angles by applying the Hilbert transform to band-pass filtered data, which produces comparable results to wavelet convolution (Le Van Quyen et al., 2001
), and is computationally simpler for analyses involving one a priori defined frequency band. To pool these data, we averaged the phase synchrony values for each condition among FC1, FCz, and FC2, and among O1, Oz, and O2. Statistics were performed by computing a paired-sample t-test across subjects at each electrode for differences between conditions. Any electrode that was not significant at p < 2.22 × 10−5 (corresponding to 0.001 with Bonferroni correction for 45 electrodes) was assigned a value of 0 (gray in Figures 4
A,B).
Spectral Granger Causality
Spectral Granger causality estimates the amount of variance in signal X that can be explained by variance in signal Y previously in time, at a particular frequency band. It was implemented here using Matlab code available in the BSMART toolbox (Cui et al., 2008
), which has previously been validated for neurophysiological data (Zhang et al., 2008
; Gaillard et al., 2009
). Time-domain, unfiltered data from prefrontal and occipital sites were pooled prior to the analyses, as described above. Statistics were performed using a repeated-measures ANOVA, as described for the phase synchrony analyses. Because spectral Granger causality uses an autoregressive approach whereas oscillatory phase synchrony relies on consistency of phase values estimated from wavelet convolution, these methods provide converging evidence from different mathematical fronts.
Brain-Brain and Brain-Behavior Correlations
For correlations between pre-trial and synchrony peak activity, we used robust regression (Holland and Welsch, 1977
) as implemented in the statistics toolbox in Matlab. Robust regression uses an iterative weighting least-squares approach to minimize the contribution of potential outliers, and therefore minimizes the possibility of finding statistically significant relationships that are driven by a small number of data points.
For correlations between synchrony and task performance, we computed an “efficiency” index (Townsend and Ashby, 1983
) by dividing the response times during Go trials by one minus the proportion of errors on conscious No-Go trials (i.e., the inhibition rate). This provides a composite performance measure that takes into account both correct responses and successful inhibition rates. Response times from error trials were not included because they are faster than correct responses and thus may introduce bias. Correlations were computed using Spearman’s rho.
Unconscious No-Go Cues Slow Response Times on Subsequent Trials
Although the unconscious No-Go cue was not perceived, subjects were slower on Go trials following unconscious errors, compared to Go trials following Go trials (Figure 1
B). Although small in magnitude (on average 3.1 ms), this effect was reliable across participants and testing sessions (F1,14 = 5.98; p = 0.028). Subjects also were slower on Go trials following conscious errors (on average 19.6 ms; F1,14 = 13.45; p = 0.003), which replicates many previous post-error slowing studies. Post-error slowing was greater following conscious compared to unconscious errors (F1,14 = 10.472, p = 0.006).
MFC–OCC Synchrony Following Response Errors
We examined oscillatory phase synchrony between a pool of prefrontal electrodes (FC1, FCz, and FC2; hereafter referred to as MFC) and a pool of occipital electrodes (O1, Oz, O2; hereafter referred to as OCC); pooling ensures high signal-to-noise and minimal chance of finding effects due to noise fluctuations in any single electrode. Data were first CSD transformed to maximize spatial resolution and minimize volume conduction (see Materials and Methods). When averaging across conditions, we found a strong “burst” of MFC–OCC synchrony around 100–400 ms following the onset of the Go stimulus, in a relatively broad low-frequency range of about 2–12 Hz (Figure 2
A). Based on the experimental design and time-course of inter-site phase synchrony, we defined three time windows of interest for subsequent investigations: “pre-trial” (−1200 to −300 ms before stimulus onset), “peak synchrony” (100 to 300 ms following stimulus onset; peak synchrony was observed at ∼200 ms), and “post-trial” (500 to 1400 ms following stimulus onset).
Upon closer inspection of patterns of MFC–OCC synchrony in different conditions, we found that when subjects committed either conscious or unconscious errors in the previous trial, MFC–OCC synchrony significantly increased, both before the trial started and throughout the trial (with the exception of the time of the peak synchrony, around 200 ms post-stimulus; see Figure 2
B). A 3 (condition) × 3 (time window: pre-trial, peak synchrony, post-trial; see Materials and Methods) repeated-measures ANOVA confirmed the significant main effects of condition (F2,28 = 78.5, p < 0.001) and time window (F2,28 = 46.78, p < 0.001), and a significant condition × time window interaction (F4,56 = 4.33, p = 0.021), which was driven by differences among conditions being smaller immediately following stimulus onset (100 to 300 ms). During the pre-stimulus period, MFC–OCC synchrony was significantly stronger for trials following unconscious errors compared to trials following Go trials (t14 = 8.1, p < 0.001), and for trials following conscious errors compared to trials following Go trials (t14 = 8.5, p < 0.001). Trials following conscious errors also had significantly stronger synchrony compared to trials following unconscious errors (t14 = 6.1, p < 0.001). The pattern of results was not affected by our choice of frequency band, because similar effects were observed when using narrower frequency ranges (2–5 Hz; data not shown).
One potential alternative explanation of changes in phase synchrony is that with increased oscillation power, phase values are better estimated, and this may spuriously increase phase synchrony. However, such an account does not explain our findings: There was no significant main effect of or interaction with condition with occipital oscillation power (p’s > 0.5), nor was there a main effect of condition with MFC power (F2,28 = 2.57, p = 0.115). There was a time window × condition interaction for MFC power (F4,56 = 15.28, p < 0.001), which was driven by increased power following conscious error trials (t14 = 4.2, p = 0.001) (see Figure 3
). This was due to enhanced MFC activity during conscious response errors on the preceding trial (the error-related negativity); indeed, the response on the previous trial occurred on average around 1500 ms before stimulus onset of the current trial.
Figure 3. Oscillation power from MFC and OCC. These effects were uncorrelated with changes in oscillation synchrony, suggesting that inter-regional phase synchrony reflects a mechanism of brain interactions that is independent of the overall amount of localized activity.
The alternative explanation of power driving phase synchrony differences also predicts that individual differences in power would be correlated with individual differences in synchrony. However, we found no evidence for such an effect: The difference in inter-site phase synchrony between unconscious errors and Go trials was not significantly correlated with the difference in power between these conditions at MFC (Spearman’s rho: r = 0.089, p = 0.75) or OCC (r = −0.07, p = 0.79) sites; correlations between power and synchrony were also not significantly correlated when comparing trials following conscious errors and Go trials at MFC (r = −0.09, p = 0.73) or OCC (r = 0.14, p = 0.60) sites. Further, inter-site synchrony did not correlate with OCC or MFC power in any condition independently (not computing condition differences) (all p’s > 0.35). The lack of complementary effects in power or significant power-synchrony correlations demonstrate that there is information available in the synchrony across sites beyond what is available at either site alone.
This set of analyses demonstrated that oscillatory synchrony between MFC and OCC increases following conscious and unconscious errors, extending several seconds after the error into the following trial. These analyses were anatomically constrained according to our a priori hypotheses regarding the roles of medial prefrontal and occipital cortices in the visually cued Go/No-Go task; in the next set of analyses, we examined the topographical distribution of post-error oscillatory functional connectivity.
Topographical Analyses of Phase Synchrony
To examine the more global spatial distribution of these effects, we computed inter-site synchrony between MFC and all other electrodes, and between OCC and all other electrodes, for each condition and for the three time windows of interest (pre-trial, peak synchrony, and post-trial). To remove global patterns of inter-regional synchrony, we subtracted seeded synchrony maps during Go trials following Go trials, from those during Go trials following unconscious and conscious errors. As seen in Figures 4
A,B, synchrony between OCC and prefrontal regions was significantly greater during trials following both unconscious and conscious errors compared to trials following Go trials; similarly, synchrony between MFC and occipital regions was significantly greater following errors compared to following Go trials. To threshold these maps statistically, a paired-samples t-test was conducted at each electrode across subjects; electrodes with synchrony differences that were not significant at p < 2.22 × 10−5 (0.001 with Bonferroni correction for 45 electrode pairs) had their values set to zero (gray color). MFC–OCC synchrony differences were prominent pre-trial and post-trial; in contrast, strong topographical differences were not observed during the time of stimulus-related activity (100–300 ms).
Figure 4. Topographical analyses of oscillatory phase synchrony reveal spatial distribution of frontal-occipital connectivity following unconscious and conscious errors. In these analyses, phase synchrony between the MFC seed and every other electrode (top row of A and B), and between the OCC seed and every other electrode (bottom row of A and B) was calculated; differences between trials following unconscious errors and Go trials (A) and between trials following conscious errors and Go trials (B) are plotted. Electrodes with differences not statistically significant at p < 2.22 × 10−5 had their values set to 0 (gray color). It can be seen that the MFC seed was significantly more strongly synchronized with occipital regions, and that the OCC seed was significantly more strongly synchronized with frontal regions, following unconscious and conscious errors. (C) Synchrony with MFC and OCC seeds when averaging across all time windows and conditions. These plots show that there is minimal contribution of volume conduction due to current-source-density transform.
These findings confirm the specificity of long-range, prefrontal-occipital synchrony following unconscious and conscious errors. As seen in Figure 4
C, CSD transform (see Materials and Methods) was successful at minimizing the effects of volume conduction; when averaging across conditions, phase synchrony was strong only with the electrodes used for pooling, and was minimal even with neighboring electrodes.
Directional Influences Estimated from Spectral Granger Causality
In our next set of analyses, we used spectral Granger causality to estimate the directional influence of the MFC–OCC interactions. Go trials following errors had significantly enhanced pre-trial MFC → OCC directional synchrony compared to trials following Go trials (Figure 5
A). A 3 (condition) × 3 (time window) repeated-measures ANOVA revealed a significant main effect of condition (F2,28 = 9.58, p = 0.003), and no effect of or interaction with time window (p’s > 0.4). Follow-up t-tests confirmed that during the pre-trial time window, unconscious (t14 = 2.64, p = 0.019) and conscious (t14 = 3.6, p = 0.003) errors elicited greater MFC → OCC directional synchrony compared to Go trials. In contrast, OCC → MFC directional synchrony was not statistically significantly modulated by events in the previous trial (F2,28 = 2.62, p = 0.12). There was a main effect of time, with OCC → MFC directional synchrony being maximal in the 100–300 ms time window (F2,28 = 5.27, p = 0.03; see Figure 5
B).
Figure 5. Spectral Granger causality reveals both top-down (MFC → OCC) directed influence, which was significantly increased following unconscious and conscious errors, and bottom-up (OCC → MFC) influence, which was strongest during stimulus presentation and was not significantly modulated by events in the previous trial. (A) Time-frequency plots for each condition for MFC → OCC directed synchrony (top row) and OCC → MFC directed synchrony (bottom row). Deeper red colors indicate that more variance in OCC (top) or MFC (bottom) can be explained by variance in MFC (top) or OCC (bottom) in each frequency band. (B) Line plots (average from 2–12 Hz) demonstrate time courses of directed synchrony effects. (C) Difference maps between trials following unconscious errors and Go trials (left side) and between trials following conscious errors and Go trials (right side) demonstrates time-frequency regions in which condition differences were maximal.
Further ANOVAs confirmed that during the pre-trial period, there was a significant directionality × condition interaction (F2,28 = 7.17, p = 0.01), such that MFC → OCC synchrony was characterized by a significant increase in synchrony strength from trials following correct Go trials, to those following unconscious errors, to those following conscious errors (F1,14 = 6.97, p = 0.019) (Figure 5
C). In contrast, pre-trial OCC → MFC directional synchrony was not significantly different among the three conditions (F1,14 = 2.79, p = 0.11).
These spectral Granger causality analyses revealed both “bottom-up” (OCC → MFC) and “top-down” (MFC → OCC) directional coupling; pre-trial synchrony was dominated by an MFC → OCC flow and was significantly enhanced by conscious and unconscious errors on the previous trial. In contrast, OCC → MFC flow was strongest shortly after stimulus onset, and was not significantly modulated by errors in the previous trial. These findings are consistent with the idea that the prefrontal cortex uses oscillatory synchrony to “instruct” visual cortex to improve sensory processing. We therefore hypothesized that pre-trial top-down directed synchrony should predict the strength of subsequent bottom-up directed synchrony.
To test this, we regressed pre-trial MFC → OCC directed synchrony on peak OCC → MFC directed synchrony. We found significant positive relations in all conditions, indicating that individuals with stronger pre-trial MFC → OCC directed synchrony had stronger OCC → MFC directed synchrony during subsequent stimulus presentation (Figure 6
A). This was confirmed using robust regression (t13: 2.91, 2.1, and 2.22; p-values: 0.01, 0.05, and 0.04 for trials following Go, conscious errors, and unconscious errors). These effects were not due to an alternative explanation of global individual differences in synchrony, because the strength of pre-trial OCC → MFC synchrony did not predict the strength of peak synchrony MFC → OCC synchrony (all p’s > 0.17) (Figure 6
A).
Figure 6. Top-down synchrony predicts stimulus-related bottom-up synchrony and task performance. (A) Subjects with stronger pre-stimulus MFC → OCC directed synchrony also had stronger peak synchrony (100–300 ms) OCC → MFC directed synchrony. This was statistically significant in all conditions (left panel). In contrast, pre-stimulus OCC → MFC directed synchrony was not significantly correlated with MFC → OCC directed synchrony (right panel), demonstrating the specificity of pre-trial top-down control. (B) Subjects with stronger MFC–OCC phase synchrony performed significantly better on the task (using a unitary performance measure that takes into account both response times on Go trials, and inhibition rate; smaller numbers indicate better performance). These synchrony-performance correlations were significant following conscious error trials, and increased in magnitude from Go trials following Go trials, to Go trials following unconscious errors, to Go trials following conscious errors (see inset; asterisks indicate statistical significance at p < 0.05).
Top-Down Synchrony Strength Predicts Inhibition Performance
In our final set of analyses, we examined the link between MFC–OCC oscillatory phase synchrony and behavioral performance. We computed a unitary performance measure that takes into account both speed (response times on correctly responded Go trials) and accuracy (errors on No-Go trials). For each subject, we divided the average of Go trial response times by conscious No-Go inhibition rate (see Materials and Methods); thus, subjects with lower numbers are better performers. We then correlated this efficiency measure with oscillatory phase synchrony, and found that subjects with stronger MFC–OCC phase synchrony during Go trials following conscious errors performed significantly better on the task (Figure 6
B) (Spearman’s rho: −0.56, −0.63, and −0.74, p-values: 0.03, 0.01, and 0.002, for pre-trial, peak synchrony, and post-trial). These correlations remained significant when excluding the possible outlier from the correlations (p-values: 0.02, 0.01, 0.01). As seen in Figure 6
B inset, synchrony-performance correlations became increasingly negative from Go trials following Go trials, to those following unconscious errors, to those following conscious errors. We also computed correlations between MFC–OCC synchrony and post-error reaction time slowing, but no correlations were significant (all p’s > 0.2). No correlations between MFC–OCC synchrony and average reaction time on Go trials (not scaled by inhibition rate) were significant (all p’s > 0.23). In other words, the strength of MFC–OCC phase synchrony predicted task performance, but was not correlated with overall motor speed.
Granger Causality Estimates with Lateral PFC
Because the lateral PFC has also been implicated in cognitive control (Kerns et al., 2004
), we additionally examined whether lateral PFC may play a role in guiding top-down control following errors (Figure 7
A). For these analyses, we pooled electrodes AF4, F4, and FC6. In general, results from spectral Granger causality analyses with lateral PFC → OCC were in the same direction but weaker compared to MFC → OCC (Figure 7
B). Indeed, there was no statistically robust effect of condition (F2,28 = 3.6, p = 0.064), time (F2,28 = 0.70, p = 0.449), or condition × time interaction (F4,56 = 2.78, p = 0.09). Further, when directly comparing the MFC → OCC and lateral PFC → OCC causal influence estimates, we found a significant main effect of “region” (stronger MFC → OCC casual influence than lateral PFC → OCC causal influence) (F1,14 = 13.6, p = 0.002) and a region × condition interaction (F2,28 = 5.27, p = 0.014), which was driven by directed synchrony increasing for Go trials following Go trials, Go trials following unconscious errors, and go trials following conscious errors.
Figure 7. Synchrony with lateral PFC (LPFC). (A) LPFC oscillatory phase synchrony with OCC (left) and MFC (right) averaged over conditions. (B) Spectral Granger causality estimates between LPFC and OCC. Differences among conditions were only marginally significant, and MFC → OCC directed synchrony was significantly stronger than was LPFC → OCC directed synchrony. (C) Spectral Granger causality demonstrates strong activation flow from LPFC → MFC, which was not significantly different according to condition. In contrast, MFC → LPFC directed synchrony was relatively weaker, but was significantly different across conditions, in a manner similar to that observed for MFC → OCC.
In our next set of analyses, we computed Granger causality between MFC and lateral PFC (Figure 7
C). Here we found that the causal influence of lateral PFC → MFC was stronger than that from MFC → lateral PFC (F1,14 = 13.39, p = 0.003), although the causal influence was not significantly different among conditions (F2,28 = 1.1, p = 0.335). In contrast, the MFC → lateral PFC causal estimates were significantly different among conditions (F2,28 = 4.61, p = 0.021; no interaction with time, p = 0.39). In other words, a robust flow of activity travels from lateral PFC to MFC, but only is the information flowing from MFC to lateral PFC significantly different among conditions.
Learning to adapt behavior following mistakes is critical to our biological and social survival in a fast-paced, ever-changing world. Although considerable evidence points to a role of the MFC in detecting errors or negative feedback and adapting subsequent performance (Ridderinkhof et al., 2004a
; Yeung et al., 2004
; Carter and van Veen, 2007
; Cohen, 2008
), less is known about the mechanisms by which the prefrontal cortex is able to adapt performance. Our results suggest that at least one mechanism by which MFC adapts performance following errors is by engaging top-down control mechanisms to increase the tuning or efficiency of stimulus processing (Egner, 2008
). This top-down control might be expressed through synchronized electrophysiological oscillations. This is consistent with, and offers a neurobiologically plausible mechanism for, previous neuroimaging work suggesting that prefrontal cognitive control mechanisms amplify cortical responses to task relevant information in sensory cortex (Egner and Hirsch, 2005
; Crottaz-Herbette and Menon, 2006
; Scerif et al., 2006
).
Long-range synchronized oscillations are thought to reflect a mechanism by which spatially disparate neural networks become functionally connected, and neural activities across regions are coordinated (Engel et al., 2001
; Fries et al., 2007
). Oscillatory synchrony between widespread brain regions has been linked to conflict/error processing (Cavanagh et al., 2009
), learning (Popescu et al., 2009
), decision-making (Pesaran et al., 2008
; Cohen et al., 2009
; Gaillard et al., 2009
), visual priming (Ghuman et al., 2008
), and memory retrieval (Summerfield and Mangels, 2005
). The present findings provide novel evidence that such long-range communication may underlie a cognitive control process by which top-down regulation over sensory regions is triggered by response errors. The specificity of this mechanism to error adaptation per se (as opposed to more general attentional or cognitive control processes) remains to be tested in future studies.
Spectral Granger causality revealed separable top-down and bottom-up directional synchrony. Bottom-up (OCC → MFC) directional coupling was strongest immediately following the onset of the visual stimulus, whereas top-down (MFC → OCC) directional coupling was strong before the trial began, and again from 500 to 1400 ms following stimulus onset. Interestingly, top-down directional coupling was observed in a relatively lower frequency range whereas bottom-up directional coupling was additionally observed in higher frequencies (alpha, around 8–13 Hz). This finding is consistent with electrophysiological recordings in monkeys (Buschman and Miller, 2007
), which showed that bottom-up (in that report, parietal → prefrontal) synchronization occurs in a higher frequency band compared to top-down synchronization. Although there are several differences between our study and theirs (experimental design, species, recording site, etc.), together these findings suggest that different kinds of information (e.g., bottom-up vs. top-down) may be transferred using different frequency bands.
Our results suggest that cognitive control is realized, at least in part, through MFC–mediated enhancement of sensory processing. Signals generated in the MFC may travel to early visual sensory regions via the parietal cortex, inferior temporal cortex, the thalamus, the superior colliculus, or other subcortical regions (Shipp, 2004
; Corbetta et al., 2008
). However, enhancement of the relevant stimulus domain is not the only means of adaptation thought to reside in the PFC’s toolbox. Previous modeling work has suggested that adjusting the response threshold after errors/conflict may account for some post-error/conflict control mechanisms (Botvinick et al., 2001
; Nieuwenhuis and Yeung, 2005
). In our experiment, as in others (Egner and Hirsch, 2005
), we find that top-down biasing signals on visual cortex may be most relevant for post-error adaptation. In part, this may be due to the fact that the conscious gray No-Go cues are difficult to perceive, whereas there is no ambiguity of the meaning of the No-Go stimulus in terms of response inhibition. That is, in our experiment, increasing the response threshold after errors may not improve performance, but better perceiving the No-Go stimulus will. Visual versus response selection processes may occur independently but partially serially, which would mean that longer visual processing leads to longer response times, even if response selection is not affected be different processes (Woodman et al., 2008
).
Relatedly, amygdala activity is enhanced (perhaps under control of rostral cingulate zone within the MFC) when emotional responses must be controlled (Etkin et al., 2006
), and extrastriate responses to attended stimuli are enhanced by the prefrontal cortex (Barcelo et al., 2000
; Pessoa et al., 2003
). Together, these findings suggest that modulating response thresholds may be only one of several strategies for realizing cognitive control-based adaptations.
Interestingly, this top-down control mechanism is activated even in absence of conscious awareness that an erroneous response was made to an unconscious No-Go cue. Previous studies have demonstrated that unconscious errors or conflict can increase activity in the MFC (Nieuwenhuis et al., 2001
; Hester et al., 2005
; Endrass et al., 2007
; Klein et al., 2007
; Ursu et al., 2009
). However, the implications of such unconsciously triggered activity increases have thus far remained unknown. Some researchers have suggested that such unconscious errors do not induce post-error or post-conflict performance adjustments (Nieuwenhuis et al., 2001
; Kunde, 2003
; Endrass et al., 2007
; Klein et al., 2007
), although it is possible that the magnitude of the effect is relatively subtle. Importantly, our EEG synchrony results demonstrate that unconscious errors are able to activate a top-down control mechanism. This provides an important new window into the extent to which unconscious processes can affect high-level cognitive control processes.
The behavioral and neural oscillation results suggests that unconscious errors do not trigger adaptive control processes as strongly as do conscious errors (e.g. 3 vs. 20 ms post-error slowing). This may be due to differences in how conscious and unconscious stimuli are processed in the human brain, as well as to the design of our study. Masked (unconscious) stimuli can be deeply processed in the brain (for a review, see Kouider and Dehaene, 2007
), including in the prefrontal cortex (Lau and Passingham, 2007
; van Gaal et al., 2008
), and can trigger specific behaviors. However, the strength, life-time and scope of processing of masked stimuli is generally lower than that of conscious (unmasked) stimuli (Greenwald et al., 1996
; Lamme and Roelfsema, 2000
; Dehaene and Naccache, 2001
; Kunde, 2003
). One reason for this is that in masking studies, the unconscious stimulus is generally presented for less time, or is followed by a mask after a shorter period of time than is the conscious stimulus. In this case, the conscious signal has more time to be processed, and this may determine the neural propagation extent (Lau and Passingham, 2007
; Lau, 2009
) and the impact on behavior (Vorberg et al., 2003
). Thus, although in the present experiment, conscious error adaptations were stronger than unconscious error adaptations, we are not able to determine whether this difference was due to the nature of conscious awareness, or the depth of processing (due to different intervals before the mask appeared).
It may seem surprising that MFC–OCC synchrony was sustained over an unusually long time window (1–2 s); results from masked priming studies suggest that the effects of unconscious stimuli on behavior and brain activity are ephemeral, and typically decay within ∼500 ms (Greenwald et al., 1996
; Rossetti, 1998
; Dehaene and Naccache, 2001
). Our results demonstrate that not only do unconscious errors enhance activity over MFC, they also activate top-down tonic control processes that extend over several seconds, even following the Go cue in the current trial. The duration of this effect is consistent with recent studies showing that masked (unconscious) words modulate neural activity up to approximately 1 s after stimulus presentation (Naccache et al., 2005
; Gaillard et al., 2009
). Even longer effects of unconscious priming (several minutes) have been reported, for example in the “mere exposure” effect (Elliott and Dolan, 1998
; Gaillard et al., 2007
). The combination of these results suggests that unconscious information can influence cognitive and neural processes for longer periods of time than previously thought. This adds to the ongoing debate about the neural foundations of conscious and unconscious information processing (Dehaene and Naccache, 2001
; Lamme, 2006
; Kouider and Dehaene, 2007
).
Although MFC plays a prominent role in adaptive behavior, error processing, and cognitive control (Ridderinkhof et al., 2004a
; Carter and van Veen, 2007
), other prefrontal regions also contribute to executive functioning, including lateral prefrontal cortex. Patterns of synchrony between OCC and a pooled right lateral prefrontal group (electrodes AF4, F4, and FC6) were somewhat similar to OCC synchrony with MFC, but overall less robust. Lateral PFC → OCC directed synchrony was generally weaker compared to MFC → OCC, and was only marginally significantly (p = 0.064) affected by condition. Further, MFC → lateral PFC directed synchrony was significantly influenced by condition. This suggests that MFC plays a larger role than lateral PFC in directing top-down synchrony over visual processing, consistent with previous studies implicating a strong role for MFC in implementing top-down control (Taylor et al., 2007
).
Our findings cannot be due to differences in overall oscillation power, because the effects in the power domain did not mirror the MFC–OCC synchrony effects, nor were power changes correlated with inter-site synchrony. The findings were also not related to the number of trials in each condition, because trial count was uncorrelated with synchrony strength across subjects (all p’s > 0.57), and the pattern of results was not different when randomly selecting trials in each condition according to the number of trials in the smallest condition: We still found a significant main effect of condition (F2,28 = 8.1, p = 0.002) and time (F2,28 = 35.8, p < 0.001), which was driven by the same pattern of increasing MFC–OCC synchrony for Go trials following Go trials, Go trials following unconscious No-Go errors, and Go trials following conscious No-Go errors. More generally, this dissociation between inter-site synchrony and power demonstrates that important insights into neurocognitive function can be gained from an examination of the interactions among brain regions; in some cases, limiting analyses to average activity in localized brain regions may miss important information. This set of analyses also revealed an important advantage of electrophysiological measures such as EEG and MEG over functional MRI; functional MRI cannot resolve interactions on such short time scales, nor can it be used to determine oscillatory characteristics of brain activity (Axmacher et al., 2009
).
In conclusion, we have provided novel evidence that the prefrontal cortex utilizes oscillatory synchrony as a means of enacting top-down control over sensory regions to enhance stimulus processing. This mechanism is engaged even in absence of conscious awareness that an error was made.
The authors declare that the research was conducted in the absence of any commercial or financial relationships that could be construed as a potential conflict of interest.
We thank Sandra Cornelisse for assistance with data acquisition. Michael X Cohen is supported by a Human Frontiers in Science Program fellowship. K. Richard Ridderinkhof is supported by a Vici grant from the Netherlands Organization for Scientific Research (NWO). Victor A. F. Lamme is supported by an Advanced Investigator grant from the European Research Council, and by grants from the NWO.