- Business Analytics and Information Systems, Quinnipiac University, Hamden, CT, United States
The rise of Web 4.0 marks a shift toward decentralized, autonomous AI-driven ecosystems, where intelligent agents interact, transact, and self-govern across digital and physical environments. This paper presents a layered framework outlining the infrastructural, behavioral, and governance dimensions required for enabling autonomous AI agents in decentralized ecosystems. It also explores how enterprises can strategically adopt Web 4.0 applications while mitigating risks related to decentralization and AI coordination. A conceptual approach is adopted, synthesizing research on blockchain-enabled AI, decentralized governance, and autonomous agent interactions. The paper introduces a six-layer framework visualizing key dimensions for Web 4.0 adoption, alongside a framework focusing on enterprise integration guidelines. The study identifies six essential dimensions – spanning infrastructure, trust, and governance – that collectively enable Web 4.0. AI agents require decentralized coordination, transparent behavioral norms, and scalable governance structures to operate autonomously and ethically. Enterprises adopting Web 4.0 must address challenges in data privacy, AI training, multi-agent interaction, and governance. The findings highlight that successful enterprise adoption will depend on trust mechanisms, regulatory alignment, and scalable AI deployment models that balance autonomy with accountability.
1 Introduction
The Web 4.0 paradigm represents a transformative concept that aspires to seamlessly integrate physical and digital realms. This integration is facilitated by advancements in emerging technologies and their interplay such as artificial intelligence (AI), distributed ledger technologies (DLT), the internet of things (IoT), extended reality (XR), and quantum computing (QC). The International Organization for Standardization (ISO) defines Web 4.0 as the anticipated evolution of the internet, building upon the decentralized Web 3.0 and emphasizing applications such as the fully automated, long-distance initiation and control of actions (ISO, 2017). Although the concept of Web 4.0 is still in its early stages, with most enterprises still focused on adapting to and realizing the potential of dezentralization presented by Web 3.0, the European Commission presents its strategic vision for Web 4.0 highlighting transformative potentials of the beforementioned technologies. This vision foresees an open, secure, trustworthy, fair, and inclusive digital environment for citizens, enterprises, and public administrations. It focuses on empowered human-machine interactions altering commerce and societal structures, while protecting people’s rights and economic growth (EU4Digital, 2024; European Commission, 2023). Similarly, the Web4Hub presents a Web 4.0 definition that adds the use “of advanced artificial and ambient intelligence, the internet of things, and trusted blockchain transactions to enable truly intuitive, immersive experiences” (Web4Hub, 2024). According to Zhou et al., the advanced use of AI agents – as distinctive entities in the network – is the biggest differentiator compared to the service-only AI applications in Web 3.0 (Zhou et al., 2023).
While the global Web 4.0 and virtual worlds market is projected to grow from $27 billion in 2022 to over $800 billion by 2030 (European Commission, 2025), the integration of Web 4.0 applications still brings forth numeours challenges, particularly related to the integration of AI agents capable of operating within decentralized infrastructures (La Roche et al., 2025; Zhou et al., 2023). AI agents play a pivotal role in enabling network intelligence but still require a network evolution of integrated computing and networking nodes with decentralized controllers. Key issues therefore include ensuring interoperability, maintaining security, and developing robust governance frameworks (Gürpinar et al., 2024; Zhou et al., 2023). In light of these developments, this paper addresses the following question:
Which foundational layers enable Web 4.0 and facilitate the deployment of autonomous AI agents within decentralized enterprise networks?
To explore this question, the paper explores the following sections:
• Web 4.0 foundational layers: This section introduces a layered framework detailing the infrastructural, behavioral, and governance dimensions essential for enabling autonomous AI agents within decentralized ecosystems.
• Web 4.0 enterprise readiness: This section examines critical considerations for enterprises aiming to integrate Web 4.0 applications into their networks, focusing on strategic adoption, risk mitigation, and alignment with relevant regulations.
• Discussion, conclusion, and future outlook: This section synthesizes the insights from the previous sections, discussing the findings and offering recommendations and future outlooks on the integration of Web 4.0 applications in enterprise contexts.
By providing a comprehensive analysis of the frameworks and key considerations for Web 4.0, this paper aims to contribute to the discourse on effectively harnessing the potential of autonomous AI agents within decentralized enterprise environments.
2 Web 4.0 characteristics enabling AI agents in decentralized systems
Unlike previous iterations of the web, Web 4.0 emphasizes interoperability, intelligent automation, and immersive human-machine collaboration, allowing AI agents to initiate and execute complex transactions along human team members (Dennis et al., 2023). A key enabler of this transformation is the integration of AI and DLT infrastructures to provide a trustless environment for AI agents to interact securely. These technologies should work in tandem to enhance autonomy, transparency, and security as crucial elements for systems coordinating multi-agent and human teams.
This section explores (and visualizes in Figure 1) the essential characteristics of Web 4.0 that empower AI agents, highlighting how decentralization, advanced knowledge systems, and seamless machine-to-machine (M2M) interactions create an ecosystem where intelligent agents can function efficiently while maintaining alignment with ethical, legal, and economic considerations (Almeida, 2017; Gürpinar et al., 2022; Hawlitschek et al., 2018; Trausan-Matu, 2020). The framework serves as a conceptual suggestion for progressing toward the highest governance layer, where AI agents operate on DLT and evolve into self-governing DAOs with natural language-based brain-computer interfaces enabling direct human interaction.
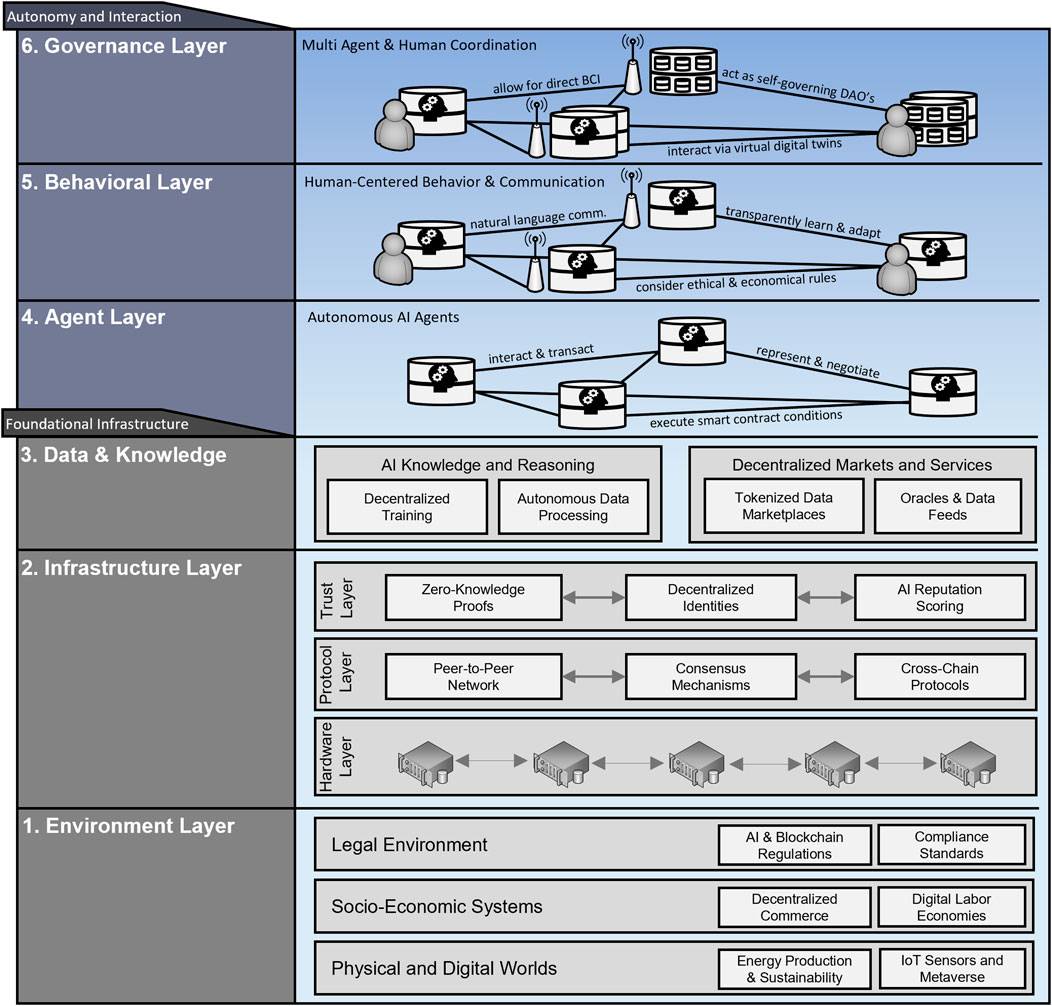
Figure 1. Web 4.0 characteristics enabling AI agents in decentralized systems. Own graphic. Inspired by (Dennis et al., 2023; Gürpinar et al., 2022; Hawlitschek et al., 2018)
The environmental layer forms the foundation of Web 4.0, integrating physical and digital worlds to create an ecosystem where autonomous AI agents can operate effectively. The expansion of decentralized AI systems and blockchain networks brings energy demands, highlighting the need for energy-efficient solutions (Grünewald et al., 2024). Without sustainable energy strategies, the large deployment of AI agents in decentralized environments may become both economically and environmentally unfeasible. At the same time, IoT sensors, edge computing, and extended reality (XR) play a critical role in gathering and processing data, enabling AI agents to perceive, interpret, and respond to real-world conditions (Dennis et al., 2023; Küpeli and Gürpinar, 2023). To fully realize Web 4.0, its underlying economic structures must evolve to accommodate AI-driven decentralized commerce and digital labor economies. AI agents will increasingly participate in autonomous transactions, supply chain optimization, and decentralized finance (DeFi), shifting away from traditional economic models toward self-executing smart contracts and tokenized economic interactions. For this transformation, it will be crucial to reduce reliance on intermediaries while ensuring transparency, fairness in and regulatory compliance of AI-powered business ecosystems. It should be noted that AI-driven decision-making in decentralized environments introduces new risks related to accountability, bias, and legality, necessitating clear guidelines on how AI agents can autonomously transact and interact. Regulatory frameworks, therefore, have to evolve in tandem with technological advancements, providing clarity on data privacy, liability, and algorithmic developments or changes.
The infrastructure layer forms the backbone for autonomous AI agents in decentralized ecosystems. As agents become more sophisticated, they require significant computational power and data storage to process vast amounts of real-time information (Hwang, 2018). DLT, storage solutions, and edge devices will be necessary to support AI workloads while reducing latency. Innovations such as neuromorphic computing and quantum-resistant cryptographic hardware could further enhance the efficiency and security of these networks (Liu et al., 2023). Peer-to-peer (P2P) networks eliminate central points of failure, allowing AI agents to communicate and transact without intermediaries. Consensus mechanisms and zero-knowledge proofs (ZKPs), play their role in maintaining data integrity, ensuring transaction validity, and enhancing privacy while optimizing computational efficiency. Additionally, cross-chain interoperability protocols and standards are essential to enable AI agents to interact across multiple networks, facilitating exchanges of data and value while relying on verifiable digital identities to authenticate and negotiate, and transact autonomously (Wasserman, 2023).
The data and knowledge layer allows agents to access and exchange information securely, fostering collaborative intelligence without reliance on centralized authorities. Research into decentralized AI learning, including federated and swarm learning, is essential as these methods enable AI agents to be trained on distributed datasets while preserving privacy and ensuring that no single entity controls the flow of knowledge (Abdulrahman et al., 2021; Zhu et al., 2023). Decentralized markets will facilitate AI-to-AI transactions, allowing agents to negotiate, execute contracts, and provide services in a trustless manner. Establishing mechanisms for verifying AI reasoning, accountability, and ethical compliance will be critical to maintaining trust. On-chain knowledge validation frameworks can help assess AI-generated insights, while zero-knowledge proofs and cryptographic attestations can ensure data integrity without exposing sensitive information. Furthermore, aligning decentralized AI models with human values and governance frameworks will be crucial to preventing bias, misinformation, and unintended consequences in AI decision-making.
The agent, behavioral, and governance layers define how AI agents operate autonomously, interact with humans, and participate in larger governance structures, ensuring a functional and ethically aligned decentralized system. At the agent layer, AI agents act as independent economic and decision-making entities capable of interacting and transacting with other agents, representing and negotiating on behalf of users or organizations, and executing smart contracts to automate agreements without intermediaries. These agents must be equipped with adaptive learning models, secure identity frameworks, and the ability to verify and enforce contractual obligations in a decentralized setting. The behavioral layer extends agent capabilities by integrating human-AI interactions through natural language communication, transparent learning, and ethical decision-making frameworks. AI agents must learn, adapt, and maintain transparency to gain trust and adoption. They must also align their actions with ethical and economic principles, ensuring they contribute positively to digital and real-world economies without reinforcing biases or engaging in harmful activities (Real de Oliveira and Rodrigues, 2021). At the governance layer, AI agents function as self-governing decentralized autonomous organizations (DAOs) or collaborate in multi-agent systems, interacting with digital twins to optimize complex systems. This layer ensures that AI-driven ecosystems remain coordinated, compliant, and scalable by embedding governance mechanisms that allow agents to self-regulate or collectively make decisions. Additionally, advancements in brain-computer interfaces (BCI) will enable direct human-agent interaction, opening new frontiers for AI-enhanced cognition and decision-making.
Together, these layers characterize a Web 4.0 ecosystem, where AI agents autonomously engage in decentralized economic and governance structures while maintaining alignment with human values and societal objectives. Building on this foundation, the next section shifts focus to the practical considerations enterprises must address when integrating and leveraging Web 4.0 applications.
3 Guidelines for integrating Web 4.0 application into enterprises
In this section, guidelines are presented and summarized in Figure 2 for enterprises seeking to adopt Web 4.0 applications and integrating autonomous AI agents in a decentralized manner. These guidelines are structured across two core dimensions: foundational, infrastructure, and trust, as well as autonomy, interaction, and governance. Each dimension plays a critical role in ensuring that enterprises can harness the full potential of Web 4.0 while navigating the complexities of decentralized AI deployment.
The foundational, infrastructure, and trust dimension emphasizes the importance of creating a robust technical and regulatory environment that supports the operation of autonomous AI agents. This includes the development of a reliable infrastructure for data and computing resources, as well as establishing trust frameworks that ensure transparency, accountability, and security (Grosse et al., 2021). Enterprises need to focus on ensuring that the underlying technological layers, such as decentralized hardware and communication protocols, are designed to operate seamlessly while adhering to legal standards and compliance requirements. A clear, consistent approach to trust-building within the decentralized network will be essential, especially as autonomous agents begin to interact and transact within complex, open systems (Gillespie et al., 2023).
On the other hand, the autonomy, interaction, and governance dimension focuses on the role of AI agents in autonomous decision-making, their ability to engage in decentralized governance, and their interactions within enterprise networks. Autonomous AI agents need to operate independently yet in alignment with human values, legal regulations, and organizational goals. Furthermore, enterprises must ensure that agents can effectively interact with each other and with human stakeholders through intuitive interfaces, including natural language processing and transparent communication methods (Chen et al., 2024). Web 4.0 enables DAOs and facilitates interactions with digital twins, which necessitate new governance frameworks that support both AI and human-led decision-making processes.
In the center of this Web 4.0 ecosystem lies the enterprise network, which consists of multiple stakeholders, each contributing to and interacting with the shared system. These stakeholders are interconnected through the flow of products, information, and financial transactions, which must be efficiently managed to ensure smooth operations (Düdder et al., 2021). Web 4.0’s decentralized nature allows these flows to occur autonomously, reducing the reliance on traditional intermediaries and increasing overall efficiency. However, to make this a reality, enterprises must adopt the proper strategies to ensure that these complex interactions are transparent, ethical, and aligned with long-term objectives.
4 Conclusion
In summary, this paper outlines the critical characteristics of Web 4.0 that enable the deployment of autonomous AI agents within decentralized systems. It explores the foundational, infrastructure, and trust dimensions that provide the necessary technical and regulatory environment, as well as the autonomy, interaction, and governance dimensions that ensure effective operation and collaboration among agents within enterprise networks. By addressing these dimensions, enterprises can build robust, decentralized ecosystems that leverage AI agents for efficient, transparent, and ethical decision-making. Looking ahead, the continued evolution of Web 4.0 technologies will require further research in all presented dimensions, particularly in scalable solutions for decentralization, enhanced trust mechanisms, and novel governance structures to ensure that autonomous AI agents operate in alignment with human values. As enterprises navigate these advancements, they will play a key role in shaping the future of decentralized AI-driven ecosystems, creating new opportunities for innovation and collaboration across industries.
Data availability statement
The original contributions presented in the study are included in the article/supplementary material, further inquiries can be directed to the corresponding author.
Author contributions
TG: Writing – original draft, Writing – review and editing.
Funding
The author(s) declare that no financial support was received for the research and/or publication of this article.
Conflict of interest
The author declares that the research was conducted in the absence of any commercial or financial relationships that could be construed as a potential conflict of interest.
Generative AI statement
The authors declare that no Generative AI was used in the creation of this manuscript.
Publisher’s note
All claims expressed in this article are solely those of the authors and do not necessarily represent those of their affiliated organizations, or those of the publisher, the editors and the reviewers. Any product that may be evaluated in this article, or claim that may be made by its manufacturer, is not guaranteed or endorsed by the publisher.
References
Abdulrahman, S., Tout, H., Ould-Slimane, H., Mourad, A., Talhi, C., and Guizani, M. (2021). A survey on federated learning: the journey from centralized to distributed on-site learning and beyond. IEEE Internet Things J. 8 (7), 5476–5497. doi:10.1109/jiot.2020.3030072
Almeida, F. L. (2017). Concept and dimensions of web 4.0. Int. J. Comput. Technol. 16 (7), 7040–7046. doi:10.24297/ijct.v16i7.6446
Chen, Y., Wang, H., Yu, K., and Zhou, R. (2024). Artificial intelligence methods in natural language processing: a comprehensive review. Highlights Sci. Eng. Technol. 85, 545–550. doi:10.54097/vfwgas09
Dennis, A. R., Lakhiwal, A., and Sachdeva, A. (2023). AI agents as team members: effects on satisfaction, conflict, trustworthiness, and willingness to work with. J. Manag. Inf. Syst. 40 (2), 307–337. doi:10.1080/07421222.2023.2196773
Düdder, B., Fomin, V., Gürpinar, T., Henke, M., Iqbal, M., Janavičienė, V., et al. (2021). Interdisciplinary blockchain education: utilizing blockchain technology from various perspectives. Front. Blockchain 3. doi:10.3389/fbloc.2020.578022
EU4Digital (2024). Join the global conversation on the future of Web 4.0 and virtual worlds. Available online at: https://eufordigital.eu/join-the-global-conversation-on-the-future-of-web-4-0-and-virtual-worlds/.
European Commission (2023). Towards the next technological transition: commission presents EU strategy to lead on Web 4.0 and virtual worlds. Available online at: https://ec.europa.eu/commission/presscorner/detail/en/ip_23_3718.
European Commission (2025). Virtual worlds. Available online at: https://digital-strategy.ec.europa.eu/en/node/12802/printable/pdf.
Gillespie, N., Lockey, S., Curtis, C., Pool, J., and Akbari, A. (2023). Trust in artificial intelligence. A Glob. study. doi:10.14264/00d3c94
Grosse, N., Guerpinar, T., and Henke, M. (2021). “Blockchain-enabled trust in intercompany networks applying the agency theory,” in 2021 3rd Blockchain and Internet of Things Conference, July, 2021, Ho Chi Minh, Vietnam (New York, NY: ACM).
Grünewald, A., Gürpinar, T., Culotta, C., and Guderian, A. (2024). Archetypes of blockchain-based business models in enterprise networks. Inf. Syst. E-Business Manag. 22 (4), 633–665. doi:10.1007/s10257-024-00673-3
Gürpinar, T., Große, N., Schwarzer, M., Burov, E., Stammes, R., Ioannidis, P. A., et al. (2022). “Blockchain technology in supply chain management – a discussion of current and future research topics,” in Science and technologies for smart cities. Editors S. Paiva, X. Li, S. I. Lopes, N. Gupta, D. B. Rawat, A. Patelet al. (Cham, Switzerland: Springer International Publishing), 482–503.
Gürpinar, T., Henke, M., and Ashraf, R. (2024). “Integrating blockchain technology in supply chain management – a process model with evidence from current implementation projects,” in Proceedings of the 57th Hawaii International Conference on System Sciences, Hawaii International Conference on System Sciences, January, 2024, Kauai, HI.
Hawlitschek, F., Notheisen, B., and Teubner, T. (2018). The limits of trust-free systems: a literature review on blockchain technology and trust in the sharing economy. Electron. Commer. Res. Appl. 29, 50–63. doi:10.1016/j.elerap.2018.03.005
Hwang, T. (2018). Computational power and the social impact of artificial intelligence. SSRN Electron. J., 47. doi:10.2139/ssrn.3147971
ISO (2017). 2017 - web 4.0. Available online at: https://www.iso.org/obp/ui/?utm_source=chatgpt.com#iso:std:iso:5127:ed-2:v1:en:term:3.1.9.07.
Küpeli, O., and Gürpinar, T. (2023). “Towards a definition of the industrial metaverse applied in context of the blockchain and web3 ecosystem,” in Blockchain and Cryptocurrency Conference, June, 2025, Rotkreuz, Switzerland.
La Roche, M. d., Voloder, E., Banerjee, A., Guerra, C., Cataldo Dell'Accio, D., Budris, F., et al. (2025). Report on artificial intelligence and blockchain convergences. SSRN Electron. J., 55. doi:10.2139/ssrn.5023415
Liu, A., Chen, X.-B., Xu, G., Wang, Z., Feng, X., and Feng, H. (2023). Quantum-enhanced blockchain: a secure and practical blockchain scheme. Comput. Mater. Continua 76 (1), 259–277. doi:10.32604/cmc.2023.039397
Real de Oliveira, E., and Rodrigues, P. (2021). A review of literature on human behaviour and artificial intelligence: contributions towards knowledge management. Electron. J. Knowl. Manag. 19 (2), 165–179. doi:10.34190/ejkm.19.2.2459
Trausan-Matu, S. (2020). Ethics in artificial intelligence. Int. Joural User-System Interact. 13 (3), 136–148. doi:10.37789/ijusi.2020.13.3.2
Wasserman, N. H. (2023). “The value of a systems architecture for disaster risk reduction”,in 2023 IEEE Global Humanitarian Technology Conference (GHTC), Radnor, PA, October, 2023 (IEEE).
Web4Hub (2024). Virtual world and the transition to Web 4.0. Available online at: https://digital-strategy.ec.europa.eu/en/library/workshop-toward-virtual-world-and-web-40-governance-action-oriented-workshop-26-november-2024.
Zhou, Z., Li, Z., Zhang, X., Sun, Y., and Xu, H. (2023). “A review of gaps between web 4.0 and web 3.0 intelligent network infrastructure,” in 2023 IEEE 9th World Forum on Internet of Things (WF-IoT), October, 2023, Aveiro, Portugal (Aveiro, Portugal: IEEE).
Keywords: artificial intelligence, AI agents, AI governance, distributed ledger technology, enterprise networks, business ecosystems, blockchain technology
Citation: Gürpinar T (2025) Towards web 4.0: frameworks for autonomous AI agents and decentralized enterprise coordination. Front. Blockchain 8:1591907. doi: 10.3389/fbloc.2025.1591907
Received: 11 March 2025; Accepted: 28 April 2025;
Published: 08 May 2025.
Edited by:
Meghana Kshirsagar, University of Limerick, IrelandReviewed by:
Neil H. Wasserman, George Washington University, United StatesCopyright © 2025 Gürpinar. This is an open-access article distributed under the terms of the Creative Commons Attribution License (CC BY). The use, distribution or reproduction in other forums is permitted, provided the original author(s) and the copyright owner(s) are credited and that the original publication in this journal is cited, in accordance with accepted academic practice. No use, distribution or reproduction is permitted which does not comply with these terms.
*Correspondence: Tan Gürpinar, dGFuLmd1cnBpbmFyQHF1LmVkdQ==