- 1College of Civil Engineering and Architecture, Zhejiang University, Hangzhou, China
- 2Department of Civil and Environmental Engineering, College of Engineering, Majmaah University, Al Majma’ah, Saudi Arabia
- 3Department of Civil and Environmental Engineering, Universiti Teknologi PETRONAS, Tronoh, Perak, Malaysia
- 4Architectural Engineering Department—College of Engineering—Taibah University, Madina, Saudi Arabia
- 5Architecture and Planning Department, Faculty of Engineering, King Khalid University (KKU), Abha, Saudi Arabia
- 6Department of Civil Engineering, College of Engineering, Prince Sattam Bin Abdulaziz University, Al-Kharj, Saudi Arabia
The growing demand for sustainable and energy-efficient buildings, particularly in the context of tall structures, has prompted increased attention to innovative solutions. Despite advancements in Building Information Modelling (BIM) technology, there exists a critical gap in understanding its comprehensive application for achieving net-zero energy consumption in tall buildings, particularly in the Malaysian construction industry. This research addresses this gap by presenting a novel strategy that integrates BIM technology with energy analysis tools for net-zero tall buildings in Malaysia. The aim of the study is to contribute valuable insights to the construction industry, policymakers, and researchers by conducting empirical research, utilizing case studies, validating the proposed framework, advancing sustainable design practices, and supporting the transition towards net-zero energy tall buildings in Malaysia. The methodology involves a three-phase approach, including qualitative analysis, a pilot survey, and a main questionnaire. Exploratory factor analysis (EFA) validates the categorization derived from qualitative interviews, while Partial Least Squares Structural Equation Modelling (PLS-SEM) assesses the convergent and discriminant validity of the measurement model. Hypotheses testing using bootstrapping establishes the significance of correlations between BIM deployment and key factors such as early design integration, enhanced energy efficiency, optimized system integration, predictive performance analysis, and validation of sustainable design. The research findings support the positive associations between BIM deployment and the mentioned factors, providing statistical significance through T-statistics and p-values. The implications of this research extend beyond the Malaysian context, offering valuable insights for architects, engineers, and stakeholders involved in designing and managing sustainable tall buildings. By addressing the identified gaps and leveraging BIM technology effectively, stakeholders can contribute to the construction of net-zero energy structures, aligning with global efforts towards sustainable and energy-efficient building practices.
Introduction
With the growing demand for sustainable and energy-efficient buildings, the construction industry has prioritised achieving net-zero energy consumption. According to a report, facilities are responsible for approximately 36% of global final energy consumption and nearly 39% of energy-related carbon dioxide (CO2) emissions (Cao et al., 2022; Waqar et al., 2023a). The complex architectural design, high energy requirements, and substantial environmental impact of tall buildings contribute significantly to these figures (Waqar et al., 2023b).
Integration of Building Information Modelling (BIM) technology provides a plausible solution for addressing the challenges posed by lofty buildings (Waqar et al., 2023c). BIM, a digital representation of a building’s physical and functional characteristics, provides a collaborative platform for architects, engineers, contractors, and stakeholders to share and exchange information throughout the tenure of the building. BIM enables professionals to simulate, analyse, and optimise various aspects of building design, including energy performance (Waqar et al., 2023d; Waqar et al., 2023e).
In selecting tall buildings as the focal point of our study, we recognize the need to substantiate this choice with empirical evidence. Malaysia has experienced a substantial increase in urbanization over the past five decades, with the urbanization rate rising from 28.4% in 1970% to 75.1% in 2020 (Wang and Liu, 2020; Liu et al., 2021). This rapid urbanization is reflected in the construction of tall buildings by more than 26%, especially in major cities like Kuala Lumpur (Miranda and Safarik, 2021). For instance, Kuala Lumpur is home to the Telekom Tower (310 m), Kempinski Hotel and Residences (307.8 m), and numerous other skyscrapers in Malaysia (Hamidavi et al., 2020). The increase in tall buildings is a direct response to the urbanization trend and represents a significant portion of Malaysia’s urban landscape. This trend highlights the relevance of focusing on tall buildings in our study on sustainable and net-zero energy practices. Implementing BIM-Driven Energy Simulation and Optimization for Net-Zero Tall Buildings involves utilising BIM models to perform comprehensive energy simulations, thereby enabling accurate prediction of energy consumption, thermal comfort, daylighting, and other crucial sustainability factors (Cao et al., 2022; Waqar et al., 2023f). These simulations comprehensively consider building geometry, materials, systems, occupancy patterns, meteorological data, and operational strategies to analyse the building’s energy performance (Cao et al., 2022; Liao et al., 2022).
Further statistics demonstrate the urgency and significance of sustainable design in towering buildings. According to a study by the Council on Tall Buildings and Urban Habitat (CTBUH), tall buildings’ average annual energy use intensity (EUI) ranges from 150 to 350 kWh/m2 (Tahmasebinia et al., 2022; Vahdatikhaki et al., 2022). On the other hand, a net-zero energy building obtains a near-zero EUI, ensuring minimal reliance on external energy sources and reducing the overall carbon footprint (Habibi, 2022).
By employing BIM-driven energy simulation and optimisation techniques, designers and stakeholders can make informed decisions regarding selecting energy-efficient technologies, incorporating renewable energy, and enhancing the building envelope (Habibi, 2022). This contributes to climate change mitigation and has economic benefits (Sarkisian, 2022; Weber et al., 2022). According to a report, energy-efficient buildings have the potential to achieve 30 to 50 per cent energy savings, resulting in significant cost savings over the lifespan of the building (Davidson and Gales, 2021).
Successful implementation of “BIM-Driven Energy Simulation and Optimization for Net-Zero Tall Buildings” necessitates resolving several critical voids. There is a need to bridge the awareness and comprehension divide among construction industry professionals (Applegath et al., 2021; Miranda and Safarik, 2021). This approach’s extensive adoption is hindered by the ignorance of many stakeholders regarding its capabilities and advantages, which prevents them from embracing it (Liu et al., 2021). To close this knowledge divide, investing in education and training programs that increase awareness and promote comprehension of BIM-driven energy simulation and optimisation is necessary (Shalabi and Turkan, 2020; Wang and Liu, 2020).
This manuscript comprehensively analyses the success outcomes of implementation of BIM-Driven Energy Simulation and Optimization for Net-Zero Tall Buildings. This manuscript seeks to contribute to the corpus of knowledge on BIM-driven energy simulation and optimisation for achieving net-zero energy consumption in tall buildings through a comprehensive primary study. The presented findings and insights are focused to aid architects, engineers, and decision-makers in employing sustainable design practices and making educated decisions to improve the energy efficiency of towering structures.
The construction industry emphasises sustainable and energy-efficient structures, with net-zero energy consumption as the primary objective. Due to their intricate architectural design, high energy demands, and significant environmental impact, tall buildings present unique challenges. BIM technology provides a collaborative platform for simulating, analysing, and optimising various aspects of building design, including energy performance (Pučko et al., 2020; Sarkisian, 2020). However, some voids must be addressed for implementation to be successful. These include limited awareness and comprehension among professionals, data integration challenges, complex software and tools, and the need for enhanced collaboration and stakeholder engagement (Rodrigues et al., 2020). This study also seeks to advance sustainable design practices by incorporating BIM-driven energy simulation and optimisation and support the transition towards net-zero energy towering buildings.
As in many other regions, the construction industry in Malaysia is increasingly prioritising sustainable and energy-efficient buildings, with an emphasis on reaching net-zero energy consumption. Tall structures present unique challenges regarding the complexity of architectural design, energy requirements, and environmental impact. BIM technology offers a collaborative framework for modelling, assessing, and maximising several facets of building design, such as energy efficiency. Yet, to apply BIM-driven energy simulation and optimisation for net-zero tall buildings in Malaysia, it is required to solve region-specific gaps and difficulties. This research offers an innovative strategy that adapts to the Malaysian setting by combining BIM technology with energy analysis tools. The goal of the research is to create a thorough framework involving success outcomes of implementing BIM driven energy simulations for tall buildings. This study contributes valuable insights to the Malaysian construction industry, policymakers, and researchers by conducting empirical research, utilising case studies, validating the proposed framework, advancing sustainable design practices, and supporting the transition towards net-zero energy tall buildings in Malaysia.
Literature review
The world population has rapidly increased during the last 10 years, along with economic growth and extreme weather. As a result, the demand for energy with a large carbon footprint has increased. Buildings account for 30% of global energy consumption and 28% of global greenhouse gas emissions. This requirement is expected to soon increase to 55% by 2050 due to an increase in the demand for heating and cooling. An energy-efficient enclosure is required, however, to reduce energy use (Waqar et al., 2023g; Waqar et al., 2023h). This transformation has resulted in the development of Nearly Zero Energy Buildings (NZEBs) which is any building that utilizes community electricity networks that maintains an annual or lifetime energy balance between consumption and production. In the construction industry, the concept of energy savings has grown in popularity (Waqar et al., 2023i; Waqar et al., 2023j). The study on net-zero buildings suggests incorporating non-integrated systems into the system limits of future building guidelines and procedures.
Positive Energy Buildings (PEBs) are a new paradigm that strives to positively contribute to the energy support of other buildings rather than only concentrating on attaining energy balance. The objective of these structures is to produce more energy than they consume. PEBs can be linked to a smart community grid or energy storage collection when renewable energy sources are unavailable. The architectural, engineering, and construction (AEC) industry has witnessed an increase in research into automated optimization approaches. These strategies are used to map out and identify the paths to building designs with desirable features such as aesthetics, geometry, structure, comfort, energy efficiency, and cost. The focus is on reaching a lot of positive outcomes rather than focusing on a single goal (Waqar et al., 2023k; Waqar et al., 2023l). Optimization studies are often carried out at the early phases of design, when the bulk of design options have yet to be chosen. However, in the late design stages, optimization strategies may be equally useful for selecting and fine-tuning control techniques and HVAC design. They may also be used to find the optimal building control during construction activities using model-predictive control approaches. The optimal modelling approaches and search algorithms are selected depending on the application domain. There are many and growing applications for optimization methodologies in building design and control. One of the most important applications in recent years, optimization is currently being used to provide input for energy policy and incentive measures. A review of the literature on BIM-driven energy simulation and optimization for net-zero tall structures was done. The keywords were “Bim-driven OR energy OR simulation AND net-zero OR buildings”. The year range (2013–2024), topic area (engineering), document type (article), and language (English) criteria resulted in a total of 56,328 documents being discovered. Net-zero tall building design and operation might be significantly impacted by BIM-driven energy simulation and optimization, an area that is quickly expanding. China, the United States, and the United Kingdom have been the countries with the most publications on this subject in recent years as shown in Figure 1.
Using BIM, a building may be represented digitally, and its energy efficiency can be predicted. Energy simulation may be used to uncover methods to lower a building’s energy usage, and optimization can be used to determine the ideal set of design and operating parameters to meet a certain goal, such as minimizing energy use or maximizing the generation of renewable energy. The most commonly occurring terms in these papers are shown in Figure 2. “Bim-driven,” “energy,” “simulation,” “net-zero,” and “buildings” are a few of the keywords.
BIM makes energy efficiency prediction and digital representation of a building possible. Energy planning and optimization methods can be used to determine the optimal way to construct and operate components to reduce energy consumption or increase the efficiency of renewable energy production. Designing and operating large buildings to consume zero energy is possible using BIM-driven energy models and efficiency methods. Changing the shape of a building’s exterior may be done primarily to conserve energy. Perform a comprehensive analysis of all the available design options, and then select the one with the lowest energy consumption. Furthermore, the structure’s most efficient heating, ventilation, and air conditioning (HVAC) system can be selected. Getting closer to this objective may be facilitated by considering the size, population density, and temperature of the structure’s environs. The building’s energy requirements could be met using renewable energy sources, which would be facilitated by adding a third component. Sun, wind, or other renewable sources can accomplish this objective (Waqar et al., 2023m; Waqar et al., 2023n). Utilizing the most effective control methods advances energy systems over time. Sensors might be utilized to monitor energy use and make the required system modifications to accomplish this goal. The following methods could be used to plan and operate large buildings to make them energy-free and environmentally beneficial. BIM technology can facilitate modelling and managing energy consumption. BIM requires additional research into the currently employed energy planning tools, efficiency methods, and combined processes. Energy simulation and optimization outcomes based on BIM must be validated using empirical data. BIM-driven energy modelling and optimization is a powerful tool for designing and operating net-zero tall structures. The creation of new and improved tools, algorithms, and methods, as well as the validation of findings using real data, are all ways that the scientific community may contribute to the construction of net-zero tall structures.
Methodology
Framework design
This study involved seventeen qualitative interviews in devising a research framework cantered on implementing BIM-driven energy simulation and optimization for net-zero tall buildings. The primary purpose of these interviews was to gain insights and perspectives from industry professionals and experts. Throughout the interview process, the interviewers meticulously analysed the collected data, modifying the existing framework as necessary. Variables were meticulously classified to ensure a streamlined and effective research strategy, and irrelevant or inconsequential factors were eliminated (Kincelova et al., 2020). Respondents with more than 15 years of experience in the field explicitly sought to provide a thorough comprehension of the topic. Their extensive knowledge and expertise contributed to refining the research framework with invaluable insights (Salimzadeh et al., 2020).
This study has effectively developed a rigorous research framework by incorporating the findings of 17 qualitative interviews, the modifications made to the framework, and the valuable input of experienced respondents. The framework is intended to facilitate the implementation of BIM-driven energy simulation and optimization for net-zero towering buildings to promote sustainable industry practices. The designed factors involved in this study are shown in Table 1.
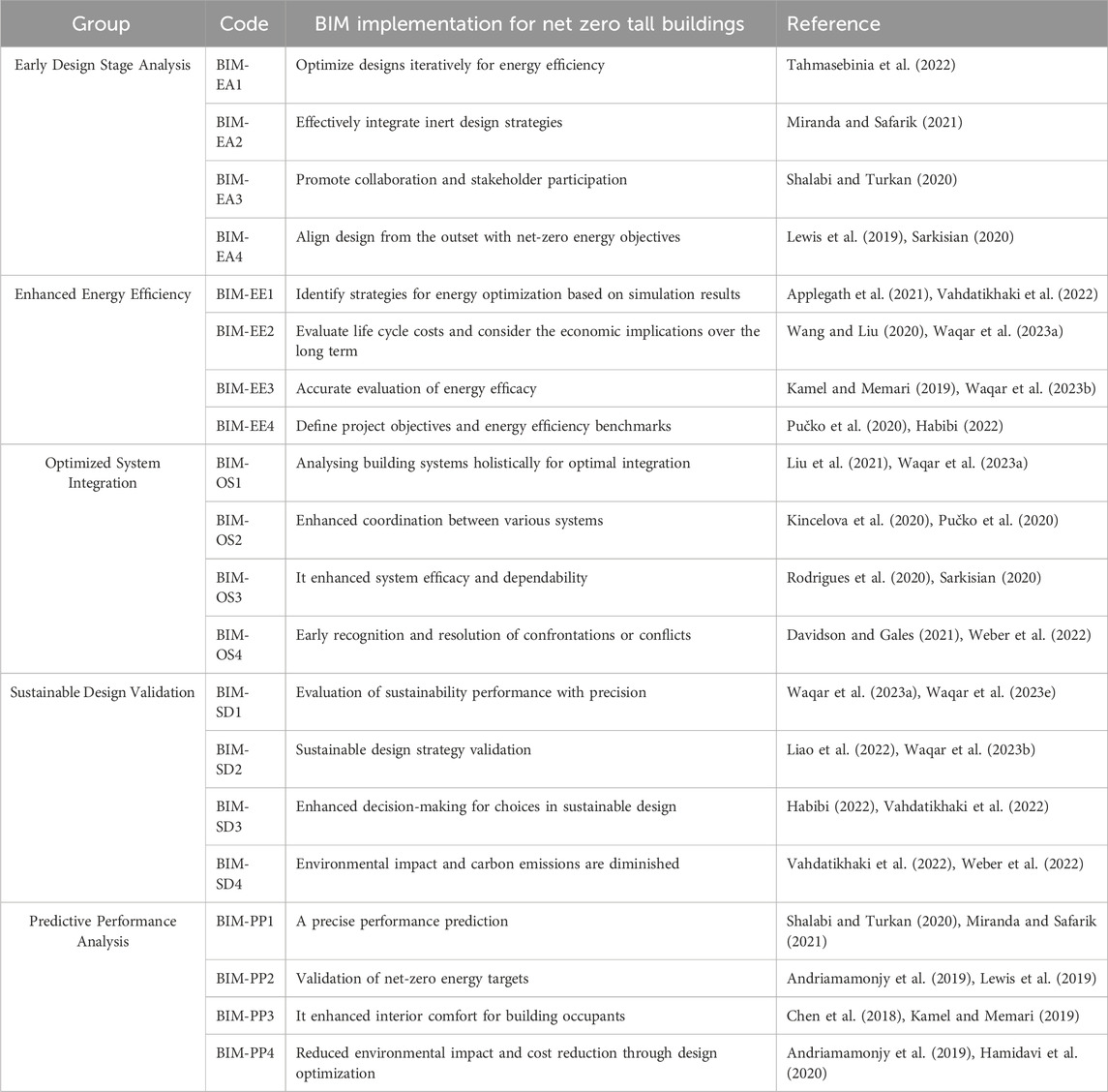
TABLE 1. Designed variables and factors from qualitative interviews and literature for BIM implementation for net-zero tall buildings.
Based on qualitative interviews and designed categories for each item, the hypothesis of the study has been developed. Figure 3 shows the five assumptions of the study.
Research method
This study involves three primary phases: the first includes qualitative analysis, the second consists of a pilot survey, and the third comprises a preliminary questionnaire survey. The study in this research is the Perak region of Malaysia. The significant respondent involved in both surveys are contractors, clients, and consultants. Figure 4 indicates the overall flow of the study.
Pilot survey
The pilot survey aimed to validate categories and items derived from qualitative interviews. To ensure a robust representation of expertise, 101 potential respondents were purposefully selected from the target population of contractors, clients, and consultants in the Perak region of Malaysia. Selection criteria included a minimum of 5 years of experience in construction projects involving BIM-driven energy simulation and optimization for tall buildings. Respondents were identified through professional networks, industry associations, and project databases, ensuring diverse expertise and experiences within the construction sector. The pilot survey was administered using a mixed-methods approach, combining manual administration facilitated by local experts in the field and electronic dissemination via email. This dual approach aimed to reach a broad spectrum of respondents, considering different preferences and accessibility. The selection process, contact methodology, and selection criteria were meticulously designed to ensure a representative and engaged cohort for the pilot study.
EFA analysis
Exploratory factor analysis (EFA) validated the expert-designed categorisation against the quantitative pilot survey data. EFA aids in revealing the latent factors that explain the patterns and relationships of the observed variables. The analysis is depicted by the Eq. 1 below:
• X represents the observed variables,
• F represents the latent factors,
• L represents the factor loadings, and
• E represents the error elements in this equation.
The objective is to estimate the factor loadings (L) that best characterise the associations between the observed variables (X) and the latent factors (F).
For EFA, the principal component analysis (PCA) technique was utilized, identifying the main components (i.e., latent factors) that account for a tremendous variance in the observed variables. This reduces the dimensionality of the data while preserving the essential information (Miranda and Safarik, 2021; Vahdatikhaki et al., 2022).
In addition, a Varimax rotation was used to facilitate the interpretation of the factor loadings. The Varimax rotation maximizes the factor loading variance within each latent factor, resulting in more distinct and interpretable factors (Miranda and Safarik, 2021; Tahmasebinia et al., 2022). To ensure a manageable and informative analysis, the variables were categorized into no more than five fixed categories. This classification organizes the observed variables and facilitates the identification of distinct factors that explain the underlying data structure (Miranda and Safarik, 2021). The decision to conduct EFA is consistent with previous studies that advocated this methodology in similar research fields (Applegath et al., 2021). EFA is a potent instrument for investigating and comprehending the factors that influence the implementation of BIM-driven energy simulation and optimization for net-zero tall structures. By conducting EFA, we can obtain valuable insights into the underlying structure and relationships of the quantitative data acquired, thereby enhancing our understanding of the essential factors in this context.
Main questionnaire
Utilizing a survey approach, the methodology involved quantitative analysis. There were 178 participants in the study, with 126 responses declared legitimate and included in the calculation. In some cases, the survey was administered manually by enlisting nearby experts in the discipline. The survey was disseminated predominantly via email (Kincelova et al., 2020). This study has a 70.8% response rate. This response rate indicates a satisfactory level of participant engagement, improving the findings’ reliability and generalizability. The study’s questionnaire was constructed using a Likert scale of 5. Respondents can designate their level of agreement or disagreement with a set of statements or items using the Likert scale. It provides a standardized measurement approach that captures respondents’ opinions or perceptions regarding specific aspects related to the research topic. Previous research supports the decision to have enough respondents for this study. According to studies such as (Applegath et al., 2021) and (Weber et al., 2022), a sample size of 100–200 respondents is generally deemed sufficient for quantitative analyses in comparable research fields. With 126 valid responses, this study meets the recommended sample size range. It can provide insightful information regarding implementing BIM-driven energy simulation and optimization for zero-energy towering buildings.
PLS-SEM algorithm
In addition to the quantitative analysis, the software SmartPLS 4 was used to assess the Partial Least Squares Structural Equation Modelling (PLS-SEM) algorithm. PLS-SEM is a statistical method for analysing a structural model’s complex relationships between latent variables. In this investigation, the PLS-SEM analysis served two purposes (Cao et al., 2022). The first objective was to assess the convergence validity of the measurement model. Convergent validity evaluates the extent to which measurement items within each latent construct measure the same concept (Waqar et al., 2023o; Waqar et al., 2023p). This was achieved by examining the factor loadings, composite reliability, and average extracted variance (AVE) for each construct. Second, PLS-SEM was used to assess the measurement model’s discriminant validity. Investigating the degree to which different notions may be distinguished is what discriminant validity does (Sarkisian, 2020; Waqar et al., 2023e). This was done by comparing the squared inter-construct correlations to each construct’s AVE values. Because SmartPLS 4 can handle complex structural models and provide precise findings, it was chosen for these investigations. It offers complex capabilities for calculating route coefficients, testing model fit, and determining the validity and reliability of the measurement model (Shalabi and Turkan, 2020; Weber et al., 2022). This research used the PLS-SEM technique and SmartPLS 4 software to assess the convergent and discriminant validity of the measurement model. The insights into the dependability and distinctiveness of the latent constructs provided by these analyses aid in a thorough understanding of the interactions between variables in the study framework.
Structural model analysis
The findings of the research were evaluated using both a structural model analysis and a measurement model analysis. In the inquiry, bootstrapping—a resampling technique—was employed to estimate the sampling distribution of data.
The five hypotheses of the research were tested using the bootstrap method. For each hypothesis, the original sample (O), sample mean (M), sample standard deviation (STDEV), t-statistic, and p-value were compared. These metrics highlight the importance and potency of the connections between the latent components in the theoretical framework (Andriamamonjy et al., 2019; Rodrigues et al., 2020).
In order to create fresh samples with the same size as the first sample, the bootstrapping approach requires several random resampling of the data (Davidson and Gales, 2021). For each set of resampled data, the structural model is then calculated, resulting in a statistical distribution. It is possible to ascertain the importance of the correlations between variables by evaluating the distribution of these statistics, including the t-statistic and p-value.
In this equation,
• Y stands for observed variables.
• B stands for path coefficients.
• X stands for latent variables.
• E stands for the error term.
The objective of the structural model Eq. 2, is to estimate the path coefficients (B) that characterize the strength and direction of the relationships between the latent variables (X) and the observed variables (Y). Using the bootstrapping method, researchers can draw inferences about the population based on the resampled data. It provides accurate and trustworthy estimates of the relationships and permits the testing of hypotheses (Andriamamonjy et al., 2019; Lewis et al., 2019).
This study tested hypotheses and evaluated the significance and intensity of the relationships between latent constructs by employing bootstrap analysis on the structural model (Waqar et al., 2023e). The t-statistics, p-values, and other measures obtained through this analysis contribute to an in-depth comprehension of the research findings and support the study’s conclusions.
Predictive relevance
The predictive validity Q2 evaluates a model’s capacity to foresee future outcomes or new data. It considers the model’s ability to explain and predict the dependent variable using latent constructs and observed variables. Higher Q2 values indicate a greater prognostic relevance of the model, indicating its ability to forecast or generalize new data.
Results and analysis
Demographic details
The demographic information contained in the primary questionnaire reveals crucial information about the respondents. Regarding occupation, the survey received responses from various positions within the construction industry. Quantity Surveyors constituted the most significant proportion of participants (14.9%), followed by Civil Engineers (19.4%) and Architects (6.9%). Other professionals contributed to the survey, including M&E Engineers and Project Managers. Regarding organizational affiliations, the survey included respondents from various construction industry sectors. Most participants, 33.1%, were Contractors, whereas 26.3% were Consultants. Clients, who play an essential role in construction initiatives, represented 12.6% of respondents shown in Table 2—additionally, the questionnaire collected information regarding the respondents’ experience in the construction industry. The distribution of respondents by experience level revealed a wide variety of expertise. 16.0% of the sample consisted of professionals with 0–5 years percentage of respondents with 11–15 years of experience was 18.3%, while those with 16–20 years and over 20 years of experience each accounted for 9.1%. Most respondents were conversant with BIM based on an evaluation of their knowledge of this technology. Approximately 68.6% of participants indicated a positive comprehension of BIM, while 3.4% responded with uncertainty or ignorance.
These demographic details provide valuable insights into the composition of the respondent population, providing a comprehensive overview of the professionals’ backgrounds, affiliations, industry experience, and BIM knowledge.
EFA analysis
The EFA (Exploratory Factor Analysis)’s objective on the provided variables was to identify underlying factors and evaluate their reliability. The Principal Component Analysis extraction method and Varimax rotation method with Kaiser normalization were utilized in the analysis. They examined the eigenvalues to ascertain the number of retained factors. Only factors with eigenvalues greater than one were controlled in this analysis, indicating their importance in explaining the observed variables’ variance (Sarkisian, 2020; Weber et al., 2022). The relationship between the variables and the identified factors was determined using factor loadings (Waqar et al., 2023a; Waqar et al., 2023q). Variables greater than 0.6 or 0.7 loadings are significant and indicate a strong relationship with the corresponding element. The Cronbach’s alpha coefficient was calculated to assess each factor’s internal consistency or reliability. Higher Cronbach’s alpha values indicate that the variables within a factor are more internally consistent (Sarkisian, 2022; Weber et al., 2022).
Five factors were extracted from the analysis and labelled Component 1, Component 2, Component 3, Component 4, and Component 5. Based on their factor loadings, the variables were designated to the components. In addition, one variable (BIM-EE4, BIM-PP4) was excluded from the analysis due to its incorrect positioning in the group and loading error, ensuring the accuracy and reliability of the results is indicated in Table 3.
The variables within each component have satisfactory factor loadings, and Cronbach’s alpha coefficients indicate acceptable internal consistency. This suggests that the identified factors can accurately represent the underlying constructs defined by the variables.
Overall, the EFA analysis provided insights into the structure of the variables and contributed to the identification of significant factors that contribute to the understanding of the research topic.
PLS-SEM algorithm
Convergent validity evaluates the degree to which the indicators (variables) within each construct (group) measure the same underlying concept. It assures that the variables designed to assess a specific construct effectively capture that construct (Sarkisian, 2020; Waqar et al., 2023e). The loadings represent the intensity and direction of each variable’s relationship with its corresponding construct. The higher the loading value, the stronger the relationship between the variable and the construct. For instance, a loading value of 0.89 for BIM-EA1 indicates a significant association with the Early Design Stage Analysis construct.
VIF (Variance Inflation Factor) assesses multicollinearity, the degree of correlation between variables within a construct. VIF values are not provided for all variables in the table but are typically employed to determine whether variables are overly correlated. Values exceeding 5 or 10 may indicate potential multicollinearity problems (Sarkisian, 2022; Weber et al., 2022).
Composite reliability assesses the internal consistency or reliability of a construct’s indicators. It indicates the degree to which variables within a construct measure the same underlying concept reliably. Ideal CA values exceed 0.7 and indicate increased reliability. For instance, a CA value of 0.813 for the Early Design Stage Analysis construct indicates that its variables are internally consistent.
Like CA, CR (Composite Reliability) is another measure of internal consistency. It evaluates the construct’s dependability by examining the correlations between its variables. Ideal CR values exceed 0.7 and indicate increased reliability. For instance, a CR value of 0.89 for the Sustainable Design Validation construct indicates that its variables exhibit high levels of internal consistency.
AVE (Average Variance Extracted) is the variance the indicators explain relative to measurement error. It indicates how well the variables within a construct convey the underlying concept. AVE values greater than 0.5 are typically regarded as adequate (Waqar et al., 2023c). For instance, an AVE value of 0.727 for the Early Design Stage Analysis construct indicates that the variables within this construct account for a significant proportion of the variance in this construct.
In summary, the Table 3 values provide insight into the intensity of relationships, internal consistency, and variance captured by each construct’s variables (Sarkisian, 2020; Waqar et al., 2023c). These values aid in evaluating the convergent validity of the measurement model, ensuring that the variables measure their intended constructs accurately.
Table 4 shows the HTMT (Heterotrait-Monotrait Ratio) values provide insight into the discriminant validity of the study’s various constructs. A value less than 0.85 is generally considered adequate, indicating that the two constructs are distinct. The HTMT values in the provided table range from 0.111 to 0.501. The values below 0.3 indicate relatively feeble relationships between constructs, demonstrating acceptable discriminant validity. However, the moderate values (0.432, 0.501, 0.278, and 0.265) suggest that distinct construct pairings share a certain amount of variance (Lewis et al., 2019; Li et al., 2020). These findings highlight the significance of contemplating the interrelationships between constructs when interpreting the study’s results and comprehending their uniqueness.
Table 5 shows the Fornell lacker criterion for discriminant validity; by comparing the square roots of the average variance extracted (AVE) values to the correlations between constructs, the Fornell-Larcker criterion is used to evaluate the discriminant validity of constructs (Sarkisian, 2022). The diagonal values in the provided table represent the square roots of the AVERAGE for each construct, indicating the amount of variance captured by the indicators (Waqar et al., 2023r). According to the Fornell-Larcker criterion, the square root of the AVE for each construct should be greater than the correlation between that construct and other constructs for discriminant validity (Shalabi and Turkan, 2020; Waqar et al., 2023e).
Based on the table values, the square root of the AVE for Early Design Stage Analysis (EA) is 0.853, indicating that the construct itself accounts for more than 85% of the variance in the indicators. The correlations between EA and other constructs (EE, OS, PP, and SD) are below 0.853, indicating discriminant validity. Similarly, the square root of the AVE for Enhanced Energy Efficiency (EE) is 0.84, and correlations with other constructs are less than 0.84, supporting discriminant validity.
The Optimized System Integration (OS) construct has a square root of AVE of 0.784 and correlations with other constructs smaller than 0.784, indicating discriminant validity. However, the square root of AVE for the Predictive Performance Analysis (PP) construct is marginally lower than its correlation with Sustainable Design Validation (SD) (0.856) (Sarkisian, 2022). This hints at a possible overlap or shared variance between these constructs, indicating the need for additional research.
Most constructs’ discriminant validity is supported by the Fornell-Larcker criterion, indicating that they are distinct. However, the relationship between PP and SD must be investigated in greater detail to comprehend the possibility of measurement overlap (Sarkisian, 2020).
Table 6 shows showing cross-loadings provide information regarding the degree to which each variable contributes to multiple constructs, thereby indicating potential discriminant validity issues. The values in the supplied table represent the correlations between each variable and the respective constructs (Andriamamonjy et al., 2019; Hamidavi et al., 2020). The cross-loading values show that most variables have higher correlations with their separate constructs than others, indicating discriminant validity (Lewis et al., 2019; Shalabi and Turkan, 2020). Variables such as BIM-EA1, BIM-EA3, and BIM-EA4 exhibit stronger correlations with the Early Design Stage Analysis (EA) construct than other constructs. Likewise, BIM-EE1, BIM-EE2, and BIM-EE3 demonstrate stronger correlations with the Enhanced Energy Efficiency (EE) construct.
Structural model development
Table 7 shows that all five of the study’s hypotheses receive substantial support from the bootstrapping analysis. Early Design Stage (ES), Enhanced Energy Efficiency (EE), Optimized System Integration (OS), Predictive Performance Analysis (PP), Sustainable Design Validation (SD), and BIM have statistically significant relationships (Andriamamonjy et al., 2019). The coefficients derived from the original sample (O) and the sample mean (M) reveal positive and consistent relationships between the constructs. The standard deviations (STDEV) represent the data’s variability, whereas the t-statistics indicate the significance of the connections. The p-values of 0 indicate high confidence in the hypotheses’ acceptance (Hamidavi et al., 2020; Shalabi and Turkan, 2020). These results provide strong evidence supporting the theoretical framework and contribute to a better understanding of the associations between the variables within the study context.
Figure 5 illustrates the path loadings and p-values correlating to the correlations between the various constructs investigated in this research. Higher values for the path loadings show that the linkages are more substantial. They also reflect the direction in which the relationships are moving. The p-values reflect the statistical importance of the associations; lower values show outcomes that are more dependable and significant for the p-values (Andriamamonjy et al., 2019; Shalabi and Turkan, 2020). The image offers a condensed and graphical depiction of the path loadings and p-values, making it possible to get a rapid and intuitive understanding of the interactions between the variables investigated in the research (Waqar et al., 2023b; Sajjad et al., 2023).
The path loadings and accompanying T-statistic values for the relationships between the constructs investigated in this research are shown in Figure 6, which can be found here. The path loadings show both the strength of the linkages and the direction they are going, with higher values suggesting stronger correlations (Shalabi and Turkan, 2020; Waqar et al., 2023e). The T-statistic values determine the associations’ importance, and outcomes with larger values indicate that they are more dependable and statistically significant. The image offers a condensed and graphical depiction of the route loadings and T-statistic values, enabling a speedy and natural comprehension of the correlations between the variables investigated in the research.
Discussion
We concentrated on using BIM for energy simulation and optimization in Net Zero Tall Buildings during our research. The findings highlight the linkages between the various structures and the implementations of those constructs in the real world.
According to the first hypothesis, there is a favourable correlation between the Early Design Stage (ES) and the deployment of BIM. The result of 0.442 for route loading demonstrates a substantial and robust correlation. Both the T-statistics value, which comes in at 15.253, and the p-value, which sits at 0, provide evidence that this link is statistically significant. The sample mean (M) was calculated to be 0.436, and the standard deviation (STDEV) was estimated at 0.029. These numbers imply that the observed values are consistent and near the sample mean. This suggests that incorporating BIM early in the design process is reliable for applying energy simulation and optimization in Net Zero Tall Buildings (Waqar et al., 2023c; Waqar et al., 2023s).
Enhanced Energy Efficiency (EE) and BIM Implementation are hypothesized to have favourable associations in H2. A substantial relationship may be shown from the path loading value being 0.342. The statistical importance of this association is supported by the value of the T-statistics, which is 9.77, and the p-value, which is 0. The sample mean (M) was calculated to be 0.335, and the standard deviation (STDEV) was estimated at 0.035. This information indicates that the observed values are below the mean, with moderate variability. This suggests that utilizing BIM for better energy efficiency is a realistic technique for generating energy savings in Net Zero Tall Buildings. BIM stands for building information modelling.
The third hypothesis investigates the possible connection between Optimized System Integration (OS) and the execution of BIM. The significance of the link may be inferred from the route loading value of 0.336. The statistical importance of this association is further supported by the fact that its T-statistics value is 9.833, and its p-value is 0. The sample mean (M) is 0.331, and the standard deviation (STDEV) is 0.034. These numbers imply that the observed values are consistent and near the sample mean. This implies that using BIM for optimized system integration is a dependable strategy to enhance the performance of building systems in Net Zero Tall Buildings. This is especially true for buildings that are taller than 100 m.
The Predictive Performance Analysis (PP) and Business Integrated Modelling (BIM) implementation link are investigated in Hypothesis Number Four. The result of 0.214 for route loading demonstrates a substantial correlation. Both the T-statistics value, which comes in at 4.807, and the p-value, which sits at 0, provide evidence that this link is statistically significant. The observed values are somewhat higher than the mean with moderate variability, as shown by the sample mean (M) of 0.218 and the standard deviation (STDEV) of 0.044. This indicates that using BIM for predictive performance analysis is a good strategy for analysing the energy implications of design choices in Net Zero Tall Buildings. This is because BIM is a building information modelling tool.
The fifth hypothesis investigates a possible connection between BIM and Sustainable Design Validation (SD). A substantial connection may be shown from the path loading value of 0.278. Both the T-statistics value, which comes in at 6.338, and the p-value, which sits at 0, indicate that this association is statistically significant. The sample mean (M) was calculated to be 0.272, and the standard deviation (STDEV) was estimated at 0.044. These numbers show that the observed values are moderately variable and below the mean. This demonstrates the importance of BIM in verifying sustainable design features and supporting sustainable practices in Net Zero Tall Buildings.
In general, our research supports the hypothesis that there are good associations between the various constructs and the use of BIM in the context of energy simulation and optimization for Net Zero Tall Buildings. These findings, backed by the T-statistics, p-values, sample means, and standard deviations, provide light on the statistical relevance and practical consequences of integrating BIM into different phases and components of building design and energy management. By harnessing BIM technology and practices, stakeholders may efficiently accomplish net-zero energy targets and develop more sustainable buildings.
The implications of this research extend beyond the immediate context of renewable energy integration in the Perak region of Malaysia. Decision-makers, energy strategists, and grid managers can leverage the insights gained from this study to inform resilient and enduring energy frameworks that accommodate a significant proportion of renewable energy sources. By addressing the challenges identified in this research, such as intermittency and variability, stakeholders can implement efficient grid management approaches and sophisticated control mechanisms. This not only contributes to the stability of power grids but also aligns with global efforts to transition towards sustainable energy solutions, mitigating climate change concerns.
Conclusion
This research has extensively investigated the application of Building Information Modelling (BIM) for energy simulation and optimization in Net Zero Tall Buildings. The study has yielded significant and valuable insights into the interrelationships among different building structures and the practical utilization of BIM to achieve net-zero energy objectives. The findings strongly support the positive correlations between BIM deployment and key factors such as early design integration, improved energy efficiency, optimised system integration, predictive performance analysis, and validation of sustainable design. Extensive statistical research has shown that the correlations are significant and statistically significant, as demonstrated by high path-loading values, higher T-statistics, and low p-values. The research found that including Building Information planning (BIM) from the start of a project makes energy planning and optimisation easier. This integration enables designers and architects to make educated choices, resulting in considerable energy savings.
Building Information Modelling (BIM) has shown its effectiveness in optimising system integration, improving coordination, and improving the performance of building systems, eventually leading to increased energy efficiency. Predictive performance analysis has been critical in analysing and maximising the energy implications of design choices, allowing stakeholders to make informed decisions and maximise energy efficiency. BIM has made it easier to validate sustainable design components, promote sustainable practises, and ultimately achieve net-zero energy in tall buildings. This study unequivocally establishes the efficacy of BIM-driven energy simulation and optimization in Net Zero Tall Buildings. The practical applications of BIM during the early design phase, the enhancement of energy efficiency, system integration, predictive performance analysis, and the validation of sustainable design elements are indispensable for achieving sustainable and energy-efficient buildings. These findings significantly contribute to the expanding knowledge of BIM implementation and provide invaluable insights for architects, engineers, and other stakeholders involved in the design and management of Net Zero Tall Buildings.
Limitations and future recommendations
Despite the valuable insights generated by this study, it is essential to acknowledge its inherent limitations. Firstly, it is crucial to recognize that the research was explicitly conducted within the context of Net Zero Tall Buildings, which may restrict the generalizability of the findings to other building types or capacities. Caution should be exercised when extrapolating the results to different contexts, and further investigation is necessary to assess their applicability. Secondly, the study relied on self-reported data collected from a relatively small sample size, introducing the possibility of response biases, and limiting the broader generalizability of the findings. To enhance future research’s reliability and external validity, it is recommended to employ more extensive and diverse samples that better represent the target population. Furthermore, the study primarily focused on examining the relationships between BIM implementation, specific energy simulation, and optimization-related constructs, while other crucial variables and factors influencing net-zero energy objectives, such as site characteristics, occupant behaviour, and regulatory frameworks, needed to be thoroughly considered. Future research endeavours should adopt a more comprehensive approach that investigates the complex interaction between BIM and these additional factors to provide a more holistic understanding.
Several robust recommendations for future research in BIM-driven energy simulation and optimization for Net Zero Tall Buildings can be proposed based on the identified limitations. Firstly, conducting longitudinal studies that comprehensively monitor the actual energy performance of buildings throughout their lifecycle would provide more robust evidence regarding the effectiveness of BIM in achieving net-zero energy objectives. These studies should encompass long-term monitoring of energy consumption, occupant behaviour, and system performance to evaluate the enduring impact of BIM on energy efficiency. Also, exploring the integration of BIM with emerging technologies such as the Internet of Things (IoT) and Artificial Intelligence (AI) holds great potential for improving energy simulation and optimization capabilities. Valuable research would investigate how BIM can effectively leverage these technologies to enhance energy prediction, optimize control systems, and improve occupant comfort, thereby advancing the field.
Furthermore, a comprehensive understanding of BIM implementation’s social and economic aspects in net-zero buildings is essential. Future research should investigate the cost-effectiveness of BIM-driven energy simulation and optimization and the social acceptability and adoption barriers encountered in various regions and contexts. These factors will provide valuable insights into the broader impact and feasibility of implementing BIM in different settings.
Acknowledging the limitations of this study and pursuing the recommended future research directions will contribute to a more vital and nuanced understanding of the role of BIM in energy simulation and optimization for Net Zero Tall Buildings. These findings will provide valuable guidance for architects, engineers, and stakeholders involved in the design and management of sustainable buildings.
Data availability statement
The original contributions presented in the study are included in the article/Supplementary Material, further inquiries can be directed to the corresponding authors.
Author contributions
MS: Writing–review and editing, Visualization, Data curation, Software, Validation. AH: Funding acquisition, Writing–review and editing, Visualization, Software. AA: Investigation, Resources, Supervision, Validation, Writing–review and editing. AW: Conceptualization, Methodology, Validation, Visualization, Formal Analysis, Investigation, Project administration, Supervision, Writing–review and editing. AK: Conceptualization, Methodology, Validation, Visualization, Writing–original draft, Writing–review and editing. AB: Investigation, Methodology, Software, Validation, Writing–review and editing. YE: Data curation, Project administration, Supervision, Writing–review and editing. AS: Data curation, Formal Analysis, Funding acquisition, Resources, Writing–review and editing. OB: Funding acquisition, Resources, Supervision, Visualization, Writing–review and editing.
Funding
The author(s) declare financial support was received for the research, authorship, and/or publication of this article. This study is supported via funding from Prince sattam bin Abdulaziz University project number (PSAU/2023/R/1445).
Conflict of interest
The authors declare that the research was conducted in the absence of any commercial or financial relationships that could be construed as a potential conflict of interest.
Publisher’s note
All claims expressed in this article are solely those of the authors and do not necessarily represent those of their affiliated organizations, or those of the publisher, the editors and the reviewers. Any product that may be evaluated in this article, or claim that may be made by its manufacturer, is not guaranteed or endorsed by the publisher.
Supplementary material
The Supplementary Material for this article can be found online at: https://www.frontiersin.org/articles/10.3389/fbuil.2024.1296817/full#supplementary-material
References
Andriamamonjy, A., Saelens, D., and Klein, R. (2019). A combined scientometric and conventional literature review to grasp the entire BIM knowledge and its integration with energy simulation. J. Build. Eng. 22, 513–527. doi:10.1016/j.jobe.2018.12.021
Applegath, C., Veres, C., and Wu, T. (2021). The zero-carbon hybrid future of tall timber. CTBUH J.,
Cao, Y., Kamaruzzaman, S. N., and Aziz, N. M. (2022). Building information modeling (BIM) capabilities in the operation and maintenance phase of green buildings: a systematic review. Buildings 12, 830. doi:10.3390/buildings12060830
Chen, S., Jin, R., and Alam, M. (2018). Investigation of interoperability between building information modelling (BIM) and building energy simulation (BES). Int. Rev. Appl. Sci. Eng. 9, 137–144. doi:10.1556/1848.2018.9.2.9
Davidson, A., and Gales, J. (2021). BIM and fire safety engineering – overview of state of the art. Int. J. High-Rise Build., doi:10.21022/IJHRB.2021.10.4.251
Habibi, S. (2022). Role of BIM and energy simulation tools in designing zero-net energy homes. Constr. Innov. 22, 101–119. doi:10.1108/CI-12-2019-0143
Hamidavi, T., Abrishami, S., and Hosseini, M. R. (2020). Towards intelligent structural design of buildings: a BIM-based solution. J. Build. Eng. 32, 101685. doi:10.1016/j.jobe.2020.101685
Kamel, E., and Memari, A. M. (2019). Review of BIM’s application in energy simulation: tools, issues, and solutions. Autom. Constr. 97, 164–180. doi:10.1016/j.autcon.2018.11.008
Kincelova, K., Boton, C., Blanchet, P., and Dagenais, C. (2020). Fire safety in tall timber building: a BIM-based automated code-checking approach. Buildings 10, 121. doi:10.3390/BUILDINGS10070121
Lewis, A. M., Valdes-Vasquez, R., and Clevenger, C. (2019). Understanding the perceived value of using bim for energy simulation. J. Green Build. 14, 79–92. doi:10.3992/1943-4618.14.1.79
Li, H. X., Ma, Z., Liu, H., Wang, J., Al-Hussein, M., and Mills, A. (2020). Exploring and verifying BIM-based energy simulation for building operations. Eng. Constr. Archit. Manag. 27, 1679–1702. doi:10.1108/ECAM-06-2019-0314
Liao, L., Zhou, K., Fan, C., and Ma, Y. (2022). Evaluation of complexity issues in building information modeling diffusion research. Sustain 14, 3005. doi:10.3390/su14053005
Liu, Z., Yuan, C., Wang, Y., Zhang, A., and Shi, G. (2021). BIM-based intelligent monitoring method for super tall building with multi-source information. Beijing Gongye Daxue Xuebao/Journal Beijing Univ. Technol., doi:10.11936/bjutxb2020110035
Pučko, Z., Maučec, D., and Šuman, N. (2020). Energy and cost analysis of building envelope components using BIM: a systematic approach. Energies 13, 2643. doi:10.3390/en13102643
Rodrigues, F., Isayeva, A., Rodrigues, H., and Pinto, A. (2020). Energy efficiency assessment of a public building resourcing a BIM model. Innov. Infrastruct. Solut. 5, 41. doi:10.1007/s41062-020-00291-1
Sajjad, M., Hu, A., Waqar, A., Falqi, I. I., Alsulamy, S. H., Bageis, A. S., et al. (2023). Evaluation of the success of industry 4.0 digitalization practices for sustainable construction management: Chinese construction industry. Buildings 13, 1668. doi:10.3390/buildings13071668
Salimzadeh, N., Vahdatikhaki, F., and Hammad, A. (2020). Parametric modeling and surface-specific sensitivity analysis of PV module layout on building skin using BIM. Energy Build. 216, 109953. doi:10.1016/j.enbuild.2020.109953
Sarkisian, M. P. (2020). “China’s important influence on world-wide life-cycle engineering,” in Proceedings of the Life-Cycle Civil Engineering: Innovation, Theory and Practice - Proceedings of the 7th International Symposium on Life-Cycle Civil Engineering, IALCCE 2020, Shanghai, China, October, 2020.
Sarkisian, M. P. (2022). The impact of jin mao tower on life-cycle Civil engineering of tall buildings. Struct. Infrastruct. Eng. 18, 895–932. doi:10.1080/15732479.2022.2038644
Shalabi, F., and Turkan, Y. (2020). Bim-energy simulation approach for detecting building spaces with faults and problematic behavior. J. Inf. Technol. Constr. 25, 342–360. doi:10.36680/J.ITCON.2020.020
Tahmasebinia, F., Jiang, R., Sepasgozar, S., Wei, J., Ding, Y., and Ma, H. (2022). Implementation of BIM energy analysis and Monte Carlo simulation for estimating building energy performance based on regression approach: a case study. Buildings 12, 449. doi:10.3390/buildings12040449
Vahdatikhaki, F., Salimzadeh, N., and Hammad, A. (2022). Optimization of PV modules layout on high-rise building skins using a BIM-based generative design approach. Energy Build. 258, 111787. doi:10.1016/j.enbuild.2021.111787
Wang, Z., and Liu, J. (2020). A seven-dimensional building information model for the improvement of construction efficiency. Adv. Civ. Eng. 2020, 1–17. doi:10.1155/2020/8842475
Waqar, A., Almujibah, H., Khan, M. B., Alotaibi, S., and Elhassan, A. A. M. (2023a). Factors influencing adoption of digital twin advanced technologies for smart city development: evidence from Malaysia. Buildings 13, 775. doi:10.3390/buildings13030775
Waqar, A., Alshehri, A. H., Alanazi, F., Alotaibi, S., and Almujibah, H. R. (2023b). Evaluation of challenges to the adoption of intelligent transportation system for urban smart mobility. Res. Transp. Bus. Manag. 51, 101060. doi:10.1016/j.rtbm.2023.101060
Waqar, A., Bheel, N., Shafiq, N., Othman, I., Khan, M. B., Mansoor, M. S., et al. (2023c). Effect of volcanic pumice powder ash on the properties of cement concrete using response surface methodology. J. Build. Pathol. Rehabil. 8, 17. doi:10.1007/s41024-023-00265-7
Waqar, A., Hannan Qureshi, A., Othman, I., Saad, N., and Azab, M. (2023d). Exploration of challenges to deployment of blockchain in small construction projects. Ain Shams Eng. J. 2023, 102362. doi:10.1016/j.asej.2023.102362
Waqar, A., Khan, M. B., Shafiq, N., Skrzypkowski, K., Zagórski, K., and Zagórska, A. (2023e). Assessment of challenges to the adoption of IOT for the safety management of small construction projects in Malaysia: structural equation modeling approach. Appl. Sci. 13, 3340. doi:10.3390/app13053340
Waqar, A., Mateen Khan, A., and Othman, I. (2023f). Blockchain empowerment in construction supply chains: enhancing efficiency and sustainability for an infrastructure development. J. Infrastruct. Intell. Resil. 2023, 100065. doi:10.1016/j.iintel.2023.100065
Waqar, A., Othman, I., Almujibah, H., and Hayat, S. (2023g). Implementing building information modeling (BIM) for the success of geotechnical offshore construction projects: Malaysian construction industry. Qual. Quant., doi:10.1007/s11135-023-01730-8
Waqar, A., Othman, I., Almujibah, H. R., Sajjad, M., Deifalla, A., Shafiq, N., et al. (2023h). Overcoming implementation barriers in 3D printing for gaining positive influence considering PEST environment. Ain Shams Eng. J. 2023, 102517. doi:10.1016/j.asej.2023.102517
Waqar, A., Othman, I., Falqi, I., Almujibah, H., Alshehri, A., Alsulamy, S., et al. (2023i). Assessment of barriers to robotics process automation (RPA) implementation in safety management of tall buildings. Buildings 13, 1663. doi:10.3390/buildings13071663
Waqar, A., Othman, I., Hamah Sor, N., Alshehri, A. M., Almujibah, H. R., Alotaibi, B. S., et al. (2023j). Modeling relation among implementing AI-based drones and sustainable construction project success. Front. Built Environ. 9, 9. doi:10.3389/fbuil.2023.1208807
Waqar, A., Othman, I., Hayat, S., Radu, D., Khan, M. B., Galatanu, T. F., et al. (2023k). Building information modeling—empowering construction projects with end-to-end life cycle management. Buildings 13, 2041. doi:10.3390/buildings13082041
Waqar, A., Othman, I., and Pomares, J. C. (2023l). Impact of 3D printing on the overall project success of residential construction projects using structural equation modelling. Int. J. Environ. Res. Public Health 20, 3800. doi:10.3390/ijerph20053800
Waqar, A., Othman, I., Radu, D., Ali, Z., Almujibah, H., Hadzima-Nyarko, M., et al. (2023m). Modeling the relation between building information modeling and the success of construction projects: a structural-equation-modeling approach. Appl. Sci. 13, 9018–9023. doi:10.3390/app13159018
Waqar, A., Othman, I., Saad, N., Azab, M., and Khan, A. M. (2023n). BIM in green building: enhancing sustainability in the small construction project. Clean. Environ. Syst. 11, 100149. doi:10.1016/j.cesys.2023.100149
Waqar, A., Othman, I., Saad, N., Qureshi, A. H., Azab, M., and Khan, A. M. (2023o). Complexities for adopting 3D laser scanners in the AEC industry: structural equation modeling. Appl. Eng. Sci. 16, 100160. doi:10.1016/j.apples.2023.100160
Waqar, A., Othman, I., Shafiq, N., Altan, H., and Ozarisoy, B. (2023p). Modeling the effect of overcoming the barriers to passive design implementation on project sustainability building success: a structural equation modeling perspective. Sustain 15, 8954. doi:10.3390/su15118954
Waqar, A., Othman, I., Shafiq, N., Deifalla, A., Ragab, A. E., and Khan, M. (2023q). Impediments in BIM implementation for the risk management of tall buildings. Results Eng. 20, 101401. doi:10.1016/j.rineng.2023.101401
Waqar, A., Othman, I., Shafiq, N., and Mansoor, M. S. (2023r). Applications of AI in oil and gas projects towards sustainable development: a systematic literature review. Artif. Intell. Rev. 56, 12771–12798. doi:10.1007/s10462-023-10467-7
Waqar, A., Qureshi, A. H., and Alaloul, W. S. (2023s). Barriers to building information modeling (BIM) deployment in small construction projects: Malaysian construction industry. Sustainability 15, 2477. doi:10.3390/su15032477
Keywords: sustainable design, BIM technology, net-zero energy, tall buildings, energy simulation
Citation: Sajjad M, Hu A, Alshehri AM, Waqar A, Khan AM, Bageis AS, Elaraki YG, Shohan AAA and Benjeddou O (2024) BIM-driven energy simulation and optimization for net-zero tall buildings: sustainable construction management. Front. Built Environ. 10:1296817. doi: 10.3389/fbuil.2024.1296817
Received: 19 September 2023; Accepted: 15 January 2024;
Published: 01 February 2024.
Edited by:
Ha Tran, The University of Melbourne, AustraliaReviewed by:
Hossein Omrany, University of Adelaide, AustraliaPedro Mêda, University of Porto, Portugal
Copyright © 2024 Sajjad, Hu, Alshehri, Waqar, Khan, Bageis, Elaraki, Shohan and Benjeddou. This is an open-access article distributed under the terms of the Creative Commons Attribution License (CC BY). The use, distribution or reproduction in other forums is permitted, provided the original author(s) and the copyright owner(s) are credited and that the original publication in this journal is cited, in accordance with accepted academic practice. No use, distribution or reproduction is permitted which does not comply with these terms.
*Correspondence: Anfeng Hu, YW5mZW5naHVAemp1LmVkdS5jbg==; Abdul Mateen Khan, YWJkdWxfMjEwMTExMzFAdXRwLmVkdS5teQ==