- 1Department of Civil Engineering, Ghulam Ishaq Khan Institute of Engineering Sciences and Technology, Topi, Pakistan
- 2University of Rostock, Faculty of Mechanical Engineering and Marine Technology, Rostock, Germany
- 3School of Interdisciplinary Engineering and Sciences (SINES), National University of Sciences and Technology (NUST), Islamabad, Pakistan
- 4University of Rostock, Department of Life, Light and Matter, Rostock, Germany
Steel bearings have been commonly used to counteract induced loading from thermal and traffic conditions in numerous bridges. However, their effectiveness has been compromised due to aging and maintenance limitations, potentially impacting the overall bridge system performance. Existing monitoring techniques for detecting malfunctioning steel bearings lack automation and precision, making them inadequate for long-term and real-time bridge dynamics assessment. This study proposes a response-based approach to identify bearing malfunction by analyzing the traffic-induced response in the bearing vicinity. To implement this approach, laser displacement sensors and wireless acceleration sensors were employed to monitor both malfunctioning and well-functioning steel bridge bearings. Significant differences in bearing performance were observed through response analysis and comparison. Laser sensor measurements revealed larger vertical deflections in the girder at malfunctioned bearing under traffic loading. Moreover, the investigation of the acceleration response in the bearing locality indicated that bearing malfunction could alter the vibrational characteristics of the vicinity, significantly affecting Cross Power Spectral Density (CPSD) and cross-correlation. To quantitatively evaluate the performance of steel bearings, a Condition Score (CS) was introduced. The CS exhibited a strong correlation with bearing damage, providing valuable insights for maintenance and decision-making processes in bridge asset management. This study offers a comprehensive and automated method for identifying steel bridge bearing malfunction by utilizing advanced monitoring techniques and introducing the CS for assessment. The results obtained from this approach can enhance bridge maintenance strategies and contribute to effective bridge asset management.
1 Introduction
Bridges constitute a crucial element of a transportation system and have an enormous impact on the overall economy of a country. The structural health of bridge systems not only plays a significant role in the socioeconomic development of a nation but also has a significant impact on the livelihood and prosperity of its residents (Zhao et al., 2015a). As per bridge design specifications, bridges are modeled as ‘simply supported’, assuming no lateral demands on the bridge girder or slab. It is common knowledge that due to extreme temperature variations, horizontal displacements in a bridge can be significant (Helmicki et al., 1999; Rohrmann et al., 2000; Catbas et al., 2008; Bell and Sipple, 2009; Sanayei et al., 2011). Wipf et al. noted the tilt of the piers of a bridge located in Iowa, US. They found that the bearing restraints could induce horizontal forces on the bridge piers due to ambient temperature variations (Wipf, 1990). Fu and DeWolf observed that a temperature change alters the natural frequency of a bridge and is mainly due to the thermal effects on bridge bearings when they are not entirely free to rotate (Fu and DeWolf, 2001). Bakht and Jaeger demonstrated that when bearings are not entirely free due to malfunctioning, horizontal demands are significant, even under normal traffic loads (Bakht and Jaeger, 1988).
A large inventory of bridges worldwide is becoming older, deeming that these infrastructures came into existence mostly in high growth periods of many developed countries. For instance, more than 50 percent of bridges in Japan will be older than 50 years by 2030 (Shirato and Tamakoshi, 2013) and currently, in the US, the average age of bridges is 42 years (American Society of Civil Engineers, 2017). Most of these bridges are equipped with steel bearings, a popular bearing type of that era (Galindo et al., 2010; Rahman Bhuiyan and Alam, 2013; Dezfuli and Alam, 2016; Hedayati Dezfuli and Alam, 2017; Xiang et al., 2018; Xiang et al., 2019; Deng et al., 2020; Sheikhi et al., 2021). Along with other components of bridges, steel bearings are relatively sizeable victims of corrosion and other environmental disintegrations since these are located under the bridge joints where water ingress and debris growth, etc., are moderately higher (FIB Bulletins, 2003). These aspects and aging issues are significant factors in bearing function loss.
Factually, the envisioned purposes of providing bridge bearings are to allow the smooth and safe flow of structural load effects and compensate movements attributed to thermal effects and interim loads (Greimann et al., 1982). In addition, these also play their role in lessening the creep and shrinkage consequences and so forth (Sagara and Pane, 2015). Lack of maintenance and aging effects are two of the main reasons for bearing function loss, which mainly restricts the bearing movements, and this could potentially affect the bridge performance (Bakht and Jaeger, 1988; Kayser and Nowak, 1989; Dicleli and Hindi, 2005; Lindquist et al., 2008; Khurram et al., 2014; Fan and Mccormick, 2022). Under restrained conditions, bearings attract more loads and subsequently transfer them to the substructure or superstructure, depending upon the load path. Under normal operational conditions with stuck bearings, thermal and live load effects could not be relieved, which could lead to repetitive or cyclic force applications with the consequences of stress concentrations near the bearing areas (Konstantinidis et al., 2009; Steelman et al., 2014a; Algohi et al., 2017; Noury and Eriksson, 2017; Parool and Rai, 2020; Sengsri and Kaewunruen, 2020). During a seismic event, restrained bearings will not offer energy dissipation mechanisms that could have been realized while exhibiting movements and hence will attract and transfer higher seismic forces to a superstructure, which may, in turn, cause pounding and unseating (Kim et al., 2006). Further, supporting piers may also have more considerable displacement demands and could experience a plastic response, causing substantial damage to the overall structure (Steelman et al., 2014b).
To elaborate on the aforementioned issue and enumerate the severity of the problem, many studies have looked into this matter in the past. Mazroi et al. investigated the effective coefficient of friction of various steel bridge bearings in the laboratory (Mazroi et al., 1980). Under monotonic loads, the bearings were tested in the installed, rusted and in-situ conditions. Corroded bearings with sand spread over the sliding surface showed a significant increase in the coefficient of friction. Bakht et al. demonstrated that bridges with restraint bearings could appear stiffer than their design (Bakht and Jaeger, 1988). Mander et al. studied the cyclic behavior of high and low-type steel bearings used in New York, US bridges (Mander et al., 1996). Bearing samples were retrieved from the actual bridges and tested in the laboratory under large cyclic horizontal and constant gravity loads. The behavior of most bearings tested in this study was later simulated with Coulomb’s friction model.
Fu and DeWolf performed the nonlinear dynamic finite element analysis of two-span bridges with partially restrained bearings (Fu and DeWolf, 2001). They found that the bearing restraints influence the natural frequencies of the bridges. Kim et al. studied the effect of bearing damage on the global seismic response of bridges (Kim et al., 2006). They concluded that bearing damage plays a significant role in the unseating failure of a bridge system. Sato et al. also investigated the effects of steel bearing performance on the global seismic response of the bridge (Sato et al., 2008). A fixed steel pin bearing was tested, and a slip-type hysteresis response was identified. A numerical model of the steel bearing was developed and employed to evaluate the nonlinear global response of the bridge. They found that the global response of the bridge is well assessed with a developed numerical model of bearing compared to the traditional nodal approach, which is adopted in typical structural analysis and design. Fan and McCormick studied the cyclic behavior of corroded steel bearings (Fan and McCormick, 2013; Fan and McCormick, 2015). Bolster and rocker bearing types salvaged from Central and Eastern US bridges were studied under the longitudinal and transverse cyclic loads. Furthermore, Yang et al. demonstrated the effectiveness of altering bearing support stiffness in reducing bridge vibrations. They studied the cancellation of resonance in elastically supported beams under successive moving loads, focusing on optimal design conditions for bridges (Yang et al., 2024). Experimental and computational finite element modeling results revealed that corrosion significantly changes the deformation mode, secant stiffness, and lateral resistance of bearings and could lead to an undesirable response from the bridges.
All these previous studies were mainly based on either experimental lab results, which require sophisticated instrumentation and expensive test configurations, or numerical analysis results that are greatly affected by assumptions to make the investigation straightforward. Furthermore, laboratory experiments cannot exactly simulate the in-situ conditions, no matter how detailed they are, because of implicit reasons. For instance, experimental results of bearings are compromised when bearing samples are salvaged for laboratory testing. These may lose the site-specific conditions, e.g., dust, corrosion, and field stress conditions, which will unquestionably influence the test outputs. Therefore, the current situation demands the in-situ condition evaluation of the bearings on full-scale bridges. Accordingly, this paper proposes a response-based bearing condition evaluation methodology, which identifies the problem of bearing malfunction in sliding bearing types. Thus, a bridge having both examples of malfunctioned and good condition bearings was favored. Within the framework of the measurement scheme, an efficient malfunction identifier, the Condition Score (CS), is introduced. This identifier potentially yields beneficial effects compared to previously discussed experimental and numerical strategies when investigating real-world scenarios. In this context, the CS could emerge as a promising tool with the potential to enhance efficiency in identifying bearing malfunctions. Its applications are anticipated to constitute a valuable contribution to the field of structural health monitoring, offering potential benefits in practical scenarios.
2 Materials and methods
This study aimed to develop a response-based approach for identifying the malfunctioning steel bearings of a bridge. The approach is designed to overcome the limitations of existing monitoring techniques, which have low degrees of automation and insufficient precision, and to offer the potential for real-time monitoring of bridge structures. To achieve this goal, the study involved the selection of a sample of both healthy and malfunctioning bearings at a target bridge site, installing sensors on these bearings, and collecting and analyzing the response of bearings to traffic-induced loadings. The overall methodology adopted during this study is depicted by a characteristic flow chart shown in Figure 1.
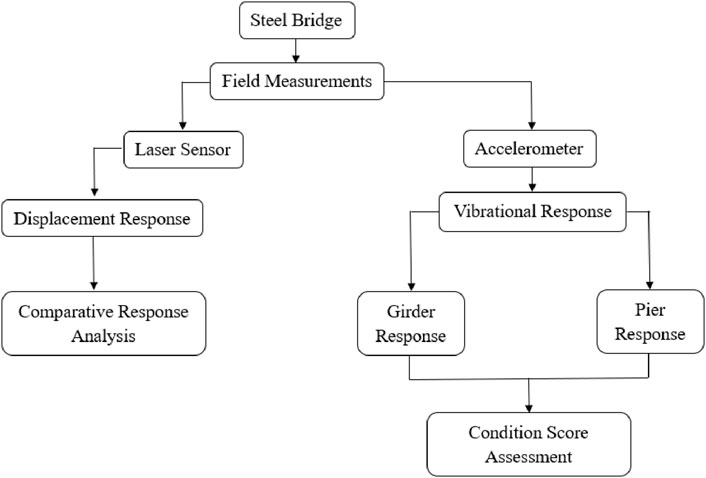
Figure 1. Characteristic flow chart depicting the bearing malfunction monitoring techniques and overall methodology.
The selected bearings were instrumented with laser displacement sensors and wireless acceleration sensors to collect data on the response of the bearings to traffic-induced loadings over a period of time. The signal processing techniques were employed, and comparative analysis was performed on collected data to identify differences in the movements and vibrational characteristics of the healthy and malfunctioning bearings. The results of this analysis were used to discuss the potential of the proposed method in automated damage identification of steel bearings for improved maintenance and repair programs for bridges.
2.1 Overview of experimental bridge
The experimental bridge is a typical steel box girder bridge. The total length of the bridge is 172.5 m, and its width is 10.25 m. It has pin bearings at fixed ends and pin-roller bearings at movable sides. One of the movable bearings was found damaged with partial unseating, which is a typical issue of these types of bearings due to earthquakes (Dicleli and Bruneau, 1995; Kim et al., 2006; Santo et al., 2012; Ellenberg et al., 2016). The first span of the bridge from the south side, bound for incoming traffic, was the target span for the response measurements since the malfunctioned bearing had been discovered there. Figure 2 shows the site condition of the damaged bearing where the roller support was partially moved out, and additional damage was prevented with temporary retrofitting, done with a wooden stopper on both sides. This arrangement restricted the longitudinal movement of the bearing and imparted the sliding malfunction (Lu et al., 2017; Chu et al., 2022; Sun et al., 2022). The condition of the neighboring bearing was found to be satisfactory by visual inspection and thus chosen for comparison purposes.
2.2 Field measurements for evaluation of bearing performance
In order to investigate the performance of both bearings in similar conditions, an identical test vehicle weight of 1.78 ton was driven closer to each bearing in each lane to input the same effects on both bearings. For clarity, the location of both bearings is shown in Figure 3. The malfunctioned bearing is located at the end of the slow lane, and the bearing in good condition is positioned at the end of the fast lane. Since the movable side of the bridge with pin-roller bearings is the only end that has both standard and malfunctioning bearings, further discussion will, therefore, be purely pertinent to this side of the bridge span, unless stated otherwise. This span was chosen for investigation because it was found to have both malfunctioned and standard bearings located side by side. The favorable situation was exploited to develop a rational approach.
2.3 Sensor installation scheme and response measurement
Microelectromechanical Systems (MEMS) accelerometers and laser displacement sensors (Gangone et al., 2011; Xiao et al., 2017; Whelan et al., 2008) were installed on the structure to record the response of both malfunctioned and good condition bearings. The Complementary Metal Oxide Semiconductor (CMOS) Multi-Function Analog Laser Sensor of the IL-300 series from KEYENCE corporation of America was used with a sampling frequency of 100 Hz. The M-A351AU triaxial MEMS wireless accelerometer of EPSON Japan recorded the acceleration response of the structure with a sampling frequency of 1,000 Hz. The wireless accelerometer is an IoT-based sensor designed explicitly for analyzing vibrations and monitoring the structural health of a system. It is a low-power device that uses Wi-Fi to communicate and has a built-in 2.4 GHz antenna that allows it to transmit data wirelessly over a maximum range of 200 m. These sensors measured outputs as voltage values and then converted them to the standard displacement unit (mm) and acceleration (m/sec2). Objectively, a longer measurement duration was chosen, which allowed for recording enough information on imposed actions. A test vehicle was used to collect the response of the structure, and the data was captured using a data acquisition system connected to the laser sensors and accelerometer sensors. The data was continuously recorded and stored in the data acquisition system during the 6-h test period. Figure 4A shows the typical installation of laser displacement sensors using special stands, and Figure 4B displays the placement of wireless sensors with firmly placed double-sided tape. The reference axis of these sensors is also shown in their respective figures with additional information on sensor placement.
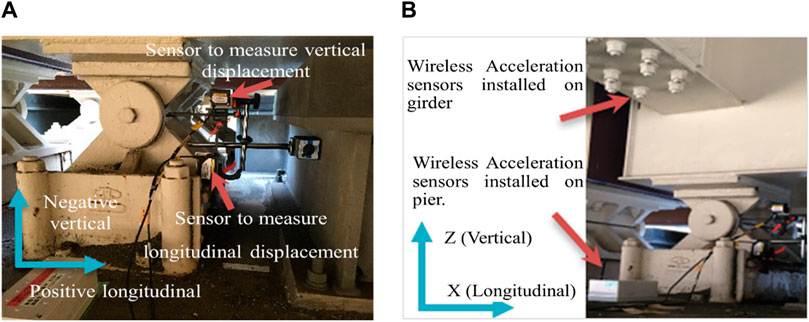
Figure 4. Placement of sensors; (A) Placement of laser displacement sensors; (B) Placement of wireless acceleration sensor.
For purposeful sensor placement, two laser displacement sensors were installed at each bearing location. These sensors were affixed to a steel component near the bearing using strong magnetic support, as depicted in Figure 4A. The horizontal laser sensors were positioned at the sliding component of the bearing to capture longitudinal displacement and assess sliding performance. Similarly, vertical laser sensors were oriented towards the main girders, approximately 80 mm away from the bearing, to measure the vertical displacement of the girder. This vertical displacement is indicative of the rotational performance of the bearings. The rotational performance of the girder and bearing components is estimated by computing the arctangent function of the girder’s vertical response, divided by the distance between the bearing and the specific measurement point on the girder. Furthermore, wireless accelerometers were installed using double taps with strong adhesive characteristics to ensure proper placement on the girders and piers, as illustrated in Figure 4B. The acceleration response of the near-bearing girder and pier was recorded to analyze the vibration characteristics of these components. This approach allows for the development of an efficient bearing performance evaluation methodology, circumventing the cumbersome laser displacement sensor placement procedures. Additionally, it bypasses the potential errors or uncertainties associated with numerical integration for displacement calculations. The experimental results are discussed in subsequent sections.
2.4 Cross power spectral density (CPSD) and cross-correlation for malfunction identification
The methods used to extract information about the frequency content and similarity between two signals are CPSD and cross-correlation. CPSD and Cross-correlation provide information about the similarities and dissimilarities in the frequency content of vibration signals. CPSD is a frequency domain analysis technique used to examine the relationship between two vibration signals. The CPSD is obtained by multiplying each signal’s Fourier transform and multiplying their complex conjugates. Mathematically, the CPSD can be expressed using Eq. 1:
Here,
where x(t) represents the time-domain signal, t denotes time, j is the imaginary unit, and f denotes frequency. The expression in Eq. 1 provides information about how the spectral content of one signal relates to the other signal across various frequencies. CPSD is employed to analyze girders’ vertical acceleration response and piers’ longitudinal acceleration response for both healthy and damaged bearing locations. Whereas cross-correlation is a time domain analysis technique that measures the similarity between two signals as a function of time lag. It represents the impulse response between the girders’ vertical acceleration response and the piers’ longitudinal acceleration response. It provides information about the time lag and strength of the correlation between these two signals. In this case, the inverse fast Fourier transform (IFFT) is performed on the CPSD signals to obtain the results in the time domain. Mathematically, the cross-correlation function, CC(t), is calculated using Eq. 3 as follows:
where
The Inverse Fourier Transform operator,
where X(f) represents the frequency-domain signal, f denotes frequency, and t represents time. The utility of this function has been discussed in Section 3.2.
2.5 Condition score based bearing malfunction assesment
This study further proposes the CS as a quantitative estimation approach for assessing bearing performance in bridge systems. The mathematical expression for CS estimation is provided below in Eq. 5.
where CS represents the condition score, the sum is taken over all the correlation values. The absolute value (|cross-correlation|) is calculated for each correlation value, ensuring a positive outcome. The square root (√) is then applied to each absolute correlation value. Finally, the square root is taken of the sum of the square roots of the absolute correlation values, resulting in the CS.
To demonstrate the application of the proposed CS analysis, a bridge model previously investigated (Farrar et al., 2000) was utilized to assess a wide range of bearing conditions. The Abaqus software (Abaqus Analysis user’s guide, 2013) was used to develop a detailed Finite Element Model (FEM) of the bridge, which includes a concrete deck supported by six steel beams. The bridge has a width of 7.3 m and a length of 15.2 m. The bridge pier measures 2.5 m in height and 1 m in width. The steel beams in the model are W30 × 116 wide-flange beams, and four evenly spaced cross braces with C12 × 25 channel sections are positioned along the span’s length. Figure 5 provides various perspectives of the bridge model and its meshed geometry. The material properties for concrete and steel used in the study are presented in Table 1.
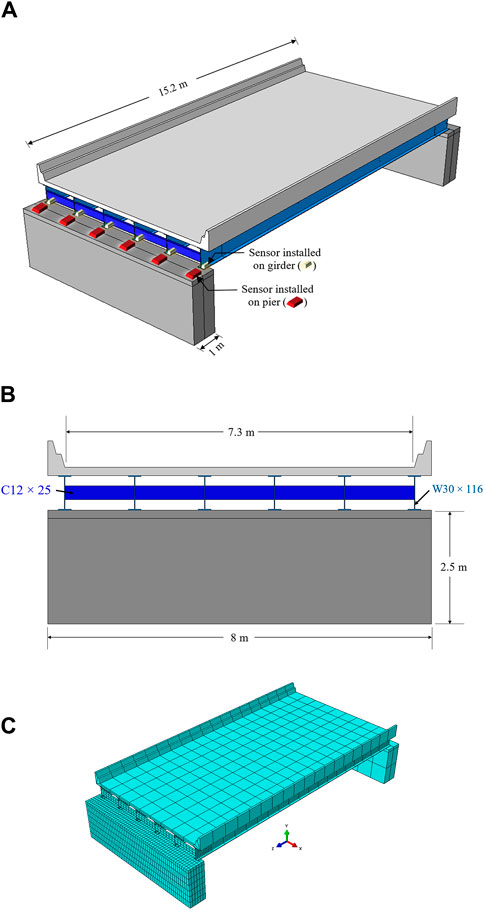
Figure 5. (A) 3D View with locations of sensors for data acquisition (B) Front View (C) Meshed geometry of FEM Model.
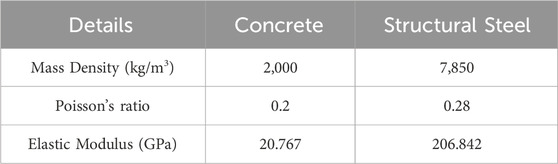
Table 1. Material properties of concrete and steel (data from Farrar et al., 2000).
The bridge model used in this study incorporates essential boundary conditions, with one end supported by sliding bearings and the other end supported by pin bearings. A tie constraint was implemented to establish the interconnection between the bridge deck slab, main girder, and cross braces. For the finite element analysis, the bridge structure was discretized using 3D solid elements with a refined mesh employed at the locations of contact interaction.
A contact interaction with friction formulation was defined to simulate the sliding bearing performance. This formulation allowed for the simulation of the sliding behavior of the bearing with different coefficients of friction. A range of coefficient of friction values was used, assuming that a higher coefficient of friction indicates a malfunctioned bearing. The contact interaction was assigned to the corresponding surfaces of the bridge pier and main girder, incorporating friction to account for the sliding bearing in the analysis.
To replicate the effects of traffic, the recorded traffic acceleration was applied as a loading condition at the bottom of the main girder near the pier. The longitudinal acceleration response of the pier was then estimated from the analysis to calculate the CS and establish the relationship between the CS and the coefficient of friction. By employing this numerical modeling approach, a comprehensive investigation of the relationship between the CS and the coefficient of friction becomes possible, offering valuable insights into the bearing performance of the bridge.
3 Results and discussion
3.1 Laser displacement sensor response
The response of the bearings under the passage of the test vehicle that was driven closer to either bearing is presented in Figure 6. From the figure of longitudinal displacement response, the sliding malfunction of the retrofitted bearing can be identified as consistent with the in-situ condition. This bearing exhibited negligibly small longitudinal displacements even though the test vehicle passed closer to it (see Figures 6A, B). Figures 6C, D display the vertical displacement response of the girders at a malfunctioned bearing location. Both downward and upward displacements can be observed in contrast to their counterparts, which exhibited mostly downward displacements. Sliding malfunction of the bearing altered the rotational response of the girder and resulted in greater vertical movements. Usually, under standard vehicle passage, the sliding and rotation phenomenon simultaneously takes place at the bearing location. Nevertheless, in case of sliding malfunctioning, the only mechanism left behind to compensate for the imposed actions is rotation, which is reflected in terms of more vertical movements of the girder. Likewise, the girder at a malfunctioned bearing revealed more considerable rotational demands and supported this conclusion, even though the test vehicle was not passing closer to the malfunctioned bearing (Figure 6D).
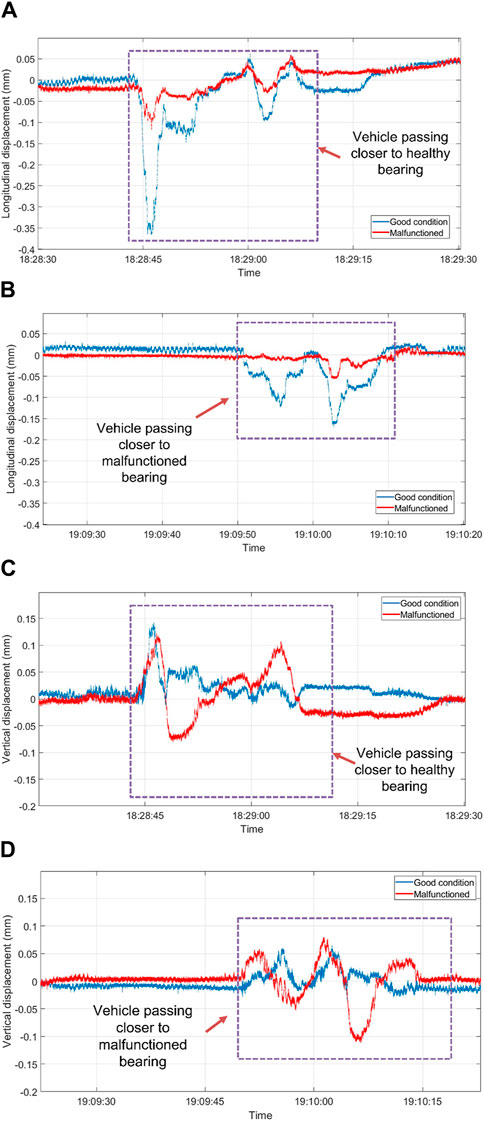
Figure 6. Response comparison of damaged and healthy bearings, (A,B) longitudinal displacement response; (C,D) vertical displacement response.
3.2 Wireless acceleration sensor response
An overview of the typical acceleration response recorded at the bearing vicinity under traffic loading is presented in Figure 7. From the overall response, it is difficult to identify the various characteristics of response and hard to understand the different mechanisms. Displacement and rotation estimates from acceleration response under the test vehicle are expected to infer the equivalent information described in the laser displacement sensor response discussions. Additionally, the aforementioned technique involves the use of a test vehicle drive by approach. It requires the absence of any other vehicle on the bridge, which is sometimes burdensome to realize, and it is onerous to avoid the influence of other traffic. Therefore, keeping in view the concerns associated with the drive by approach, the ambient acceleration response of bearing locality under any arbitrary traffic was further utilized and presented in the following discussion.
Ideally, bridge bearings should receive the same input excitations when their corresponding bridge girder midspans have similar acceleration responses (Yamamura and Tanaka, 1990; Tsai et al., 2007; Zhu et al., 2014). In view of this notion, the acceleration response of the girder midspans was also acquired by placing sensors in each lane, as shown in Figure 3. Figure 7 shows the almost identical mid span acceleration response of both girders obtained with in-depth exploration. Additionally, the corresponding response of both bearings with observable discrepancies is presented in Figure 8, and details are discussed further. Figure 8 shows the combined plots of the acceleration response of the girder in the vertical direction and the acceleration response of the pier in the longitudinal direction at both malfunctioned and good condition bearing locations. Response of only stated directions was considered since other response information may not be significant. For instance, a pier in the vertical direction is appreciably rigid and will not exhibit any noteworthy excitations. Response in the transverse direction also may not be of interest as no major force is acting under normal operations, and these bearings are not intended to accommodate movements in the lateral direction. Response of the girder in either the vertical or longitudinal direction at the bearing vicinity could be considered. The vertical direction response was selected because of its direct relation with bearing malfunction, as illustrated next.
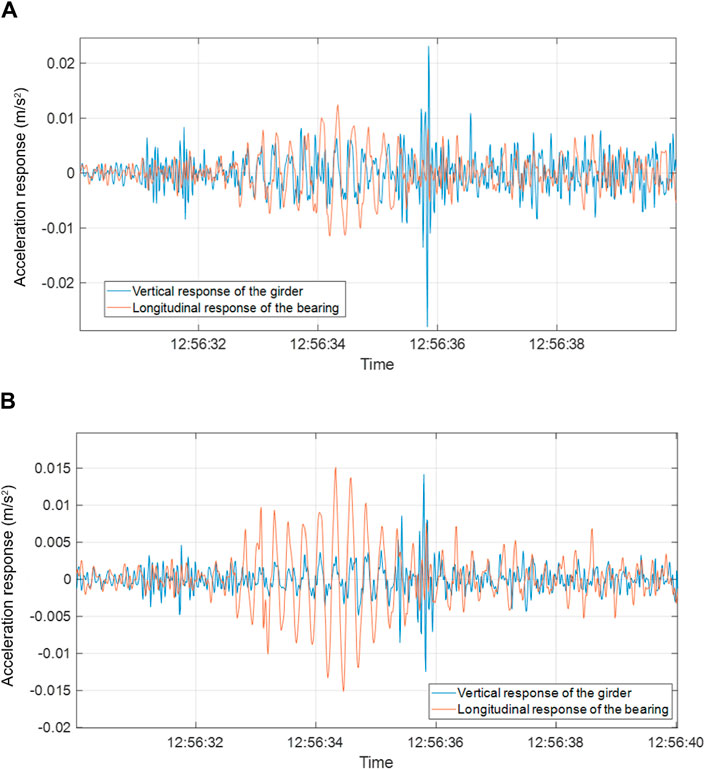
Figure 8. Comparison of girder and pier acceleration responses: (A) response at good condition bearing; (B) response at malfunctioned bearing.
It can be seen from the plots that the acceleration response of the girder in the vertical direction at the location of the healthy bearing is larger than the vertical response of the girder at the malfunctioned bearing location (see Figures 8A, B). The conceivable reason for this phenomenon is the increased rotational demand of the girder, as per the previous discussion. The frequent change in the vertical displacement direction of the girder caused the comparatively reduced acceleration response (Roberts et al., 2015; Martínez et al., 2017; Xia et al., 2017). Furthermore, increased bearing fixity attracted a relatively larger share of input actions at the malfunctioned bearing location, which was reflected in terms of the enlarged acceleration response of the pier in a longitudinal direction. The current approach to identifying malfunctioning bearings has limitations, such as the difficulty of finding similar inputs to bearings and the influence of multiple vehicles on the bridge. However, using CPSD and cross-correlation, we can develop a more systematic approach to analyze the near bearing vertical acceleration response of girders and the longitudinal acceleration response of piers. This will allow us to better understand the performance of bearings and identify any malfunctions.
The preceding discussion emphasized the importance of analyzing the near bearing vertical acceleration response of girders and the longitudinal acceleration response of piers to understand the performance of bearings. In order to develop a more comprehensive approach, the mutual characteristics of these response parameters using CPSD and Cross-correlation were considered in the context of bearing malfunctioning. Figure 9A shows the CPSD plots of these signals normalized by their corresponding maximum amplitudes. This frequency domain plot could reveal the dissimilarity of these signals, which might need to be clarified. The cross-correlation function is shown in Figure 9B.
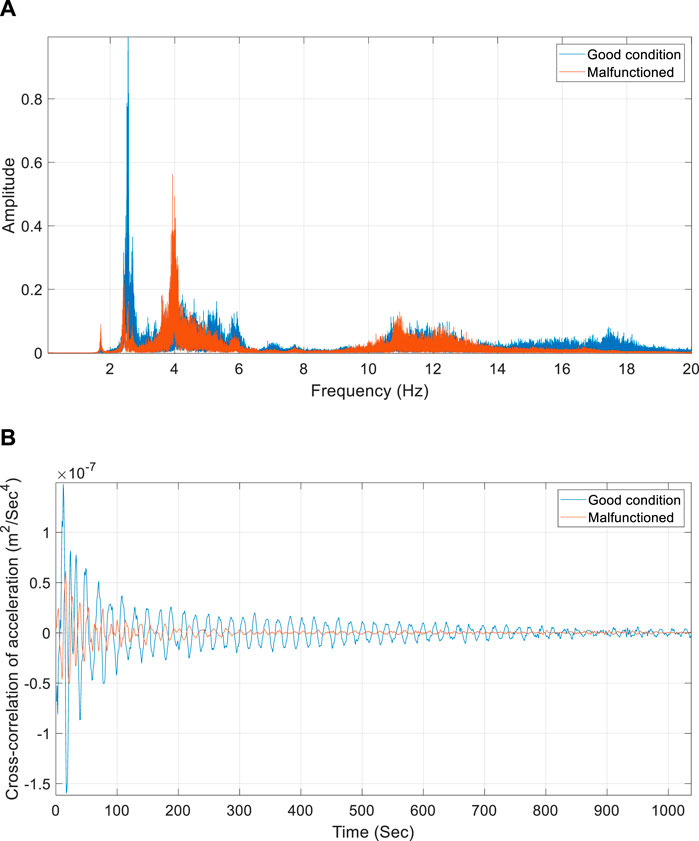
Figure 9. (A) Normalized cross power spectral density of acceleration signals (B) cross-correlation of accelerations obtained through IFFT of CPSD.
Distinct information on bearing performances could be observed in terms of differences in amplitude, phase, and even total response duration. The malfunctioned bearing seems to have very stiff behavior and smaller excitations along with lesser excitation duration (about three times smaller) when compared to its counterpart. The disparity in energy dissipation mechanisms was found to be the leading cause of the discrepancy between the responses. Suitable condition bearings respond to incoming actions through a combination of sliding and rotation, leading to a longer response duration. Whereas a malfunctioned bearing uses the rotational ability alone to act against the imposed actions that have relatively limited capability to respond, and henceforth, it transfers the energy to the underlying pier that could also be seen from its larger longitudinal response.
The proposed CS methodology is next employed herein to demonstrate its superior functionality over the conventional techniques. While traditional methods rely on subjective evaluations, leading to inconsistencies, the CS considers parameters such as amplitude and response duration from cross-correlation of accelerations to provide a comprehensive evaluation metric. By combining these parameters, a holistic assessment of the bearing condition is obtained. The CS enhances the objectivity and standardization of evaluations, aiding in maintenance decisions and ensuring long-term bridge integrity. The obtained CS values for healthy and malfunctioned bearings were found to be 1.682 and 1.311, respectively. The noticeable difference in these values indicates contrasting conditions between the two types of bearings. The CS value is expected to be larger for healthy and properly functioning bearings, indicating a higher structural integrity and performance level. The lower CS value associated with the malfunctioned bearings suggests a compromised condition with reduced capabilities to withstand external forces and vibrations. This finding highlights the importance of the CS as a quantitative measure for distinguishing between healthy and malfunctioned bearings, providing valuable insights for maintenance and decision-making processes in bridge asset management. The subsequent section expounds on applying the proposed approach through the utilization of numerical modeling techniques.
3.3 Numerical modeling based demonstration of condition score assessment technique
The bridge model prescribed in Section 2.5 was simulated with varying conditions of bearing degradation, including both healthy and malfunctioning states. To establish a correlation between the CS and the coefficient of friction, different models with distinct friction coefficients were employed. The aim was to investigate the relationship between the CS and the health of the bearings. A lower coefficient of friction indicated a healthier bearing, while a higher coefficient pointed towards a malfunctioning bearing.
During the analysis, parameters such as the amplitude and response duration of cross correlation of acceleration response were considered to calculate the CS values for each bearing condition. This quantitative assessment allowed for direct comparisons among different conditions, enabling an objective evaluation of bearing performance. The findings, presented in Figure 10A, clearly demonstrate the relationship between the CS and the coefficient of friction. The graph illustrates how variations in the coefficient of friction correspond to changes in the CS.
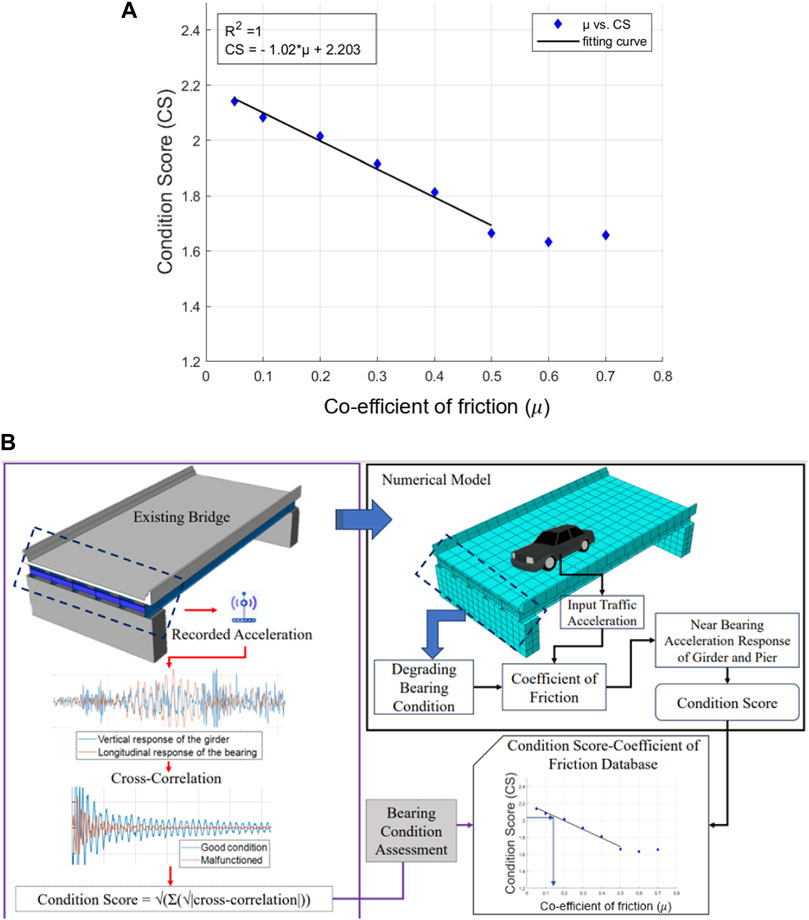
Figure 10. (A) Relationship between the coefficient of friction and CS (B) schematic framework of proposed bearing condition assessment approach.
It was observed that a lower coefficient of friction correlated with a higher CS, indicating a healthier bearing. Conversely, a higher coefficient of friction was associated with a lower CS, indicating a malfunctioning bearing. Within the range of 0–0.5 coefficient of friction, the CS exhibited a direct decrease. However, beyond a coefficient of friction of 0.5, the acceleration response became minimal, resulting in an insignificant change in the CS, which reached a nearly constant value.
It should be noted that the current methodology for CS assessment relies on acceleration response data. However, for bearings that are severely malfunctioning, estimating the CS may be challenging due to the smaller amplitude of the acceleration response. In such cases, the damage is often visually apparent and can be readily identified. It is crucial to address significant damage and implement preventive maintenance measures to mitigate the effects of severe damage. The current methodology is recommended for identifying damage in bearings that may not be visually apparent and serves as a valuable tool in maintenance and decision-making processes.
The research presented in this study demonstrates the practical application of the proposed CS estimation method through numerical modeling, providing a convenient approach for assessing bearing conditions. The obtained CS values offer valuable quantitative indicators that bridge owners and engineers can use to prioritize maintenance actions and make informed decisions regarding repair or replacement strategies. Additionally, establishing a database of CS-coefficient of friction relationships using numerical modeling enables estimating the current state of bearing function, enhancing the evaluation of bearing conditions and improving our understanding of bearing performance for proactive maintenance and the long-term durability and safety of bridge structures.
3.4 Application of the current research study
The comparison of the response of bearings with different health conditions paved the way for the applicability of the presented method. The damage identification methods based on comparative assessment of damaged and undamaged cases have already been used in past research. Typically, the artificial damage states had been induced in the structure for devising the damage identification methodologies (Li et al., 2021). On the contrary, the propitious availability of damaged and undamaged bearings at the target bridge site made it possible to devise a more realistic response-based bearing damage identification scheme.
Laser displacement sensor-based assessment could provide a detailed comparative study and differentiate the bridge structure’s bearing malfunction under undamaged and damaged conditions. These findings are consistent with the work of Zhao et al. (Zhao et al., 2015b), which has demonstrated the accuracy and viability of measurements of displacements response and the ability to operate using non-contact measurements for safe and efficient monitoring. However, this method depends on the known performance of healthy/undamaged bearing to compare with. This unavailability of performance data of a healthy bearing could be recorded from similar bridge structures, and analytical and numerical modeling approaches could be utilized otherwise. Further, the application and erection of laser sensors pose practical challenges, including the need for access to a bearing locality, special stands for instrument erection, and sufficient space for data acquisition setup. These requirements may hinder the feasibility and practicality of utilizing laser sensors for displacement measurements in bridge monitoring applications. As an alternative, accelerometers offer a promising solution for displacement estimation, as they do not necessitate direct contact with the bearing locality and can be installed in a more straightforward manner. However, it is essential to consider potential errors or uncertainties associated with displacement calculations based on acceleration data using double integration. Factors such as noise, drift, and integration errors can affect the accuracy of displacement estimates derived from acceleration data, highlighting the importance of robust signal processing techniques and calibration procedures to minimize these sources of error. Therefore, while accelerometers offer advantages in terms of ease of installation, it became imperative to explore alternative approaches that circumvent these limitations while ensuring the reliable bearing health assessment.
In the absence of laser sensor data, CPSD and Cross-correlation of acceleration data collected from the bridge girder and abutment could be utilized for acceleration response signals. The vibration response of a location caused by traffic excitation at a particular location and its proximity in the system could be effectively utilized for bridge-bearing performance evaluation. The CPSD and Cross-correlation relationship between the input signal and the output signal in the system corresponding to measured responses of damaged and undamaged conditions deviate from each other. From this result, the presence of damage in the bearing and the extent of damage greatly influence the vibrational response of the structure and the Cross-correlation of acceleration data in the structural system. The practicality of the proposed correlation-based assessment method has already been explored by different researchers (Yoon et al., 2017; Wang et al., 2021) and could be employed as a potential framework for bridge-bearing damage identification.
Initial measurement data on the target structure revealed that bearing damage significantly impacted the CPSD and cross-correlation. The damage from the original state of the structure alters the magnitude of the cross-correlation of accelerations, and its value is reduced from the undamaged state. The cross-correlation is inversely related to the presence of damage. It could be linked with the extent of the damage if sufficient data on various damage states and vibrational characteristics of the structure are available. The required data sets could be gathered from the numerical modeling of bridge structures with bearings of various damage states to collect data on vibrational characteristics and acceleration response. The experimental findings make it clear that this method needs reference cross-correlation data of undamaged structures for further applications in the current phase.
Furthermore, regarding the specific conditions of the vehicle running over the bridge for condition assessment of bearings, it is essential to consider several factors. Firstly, while the proposed methodology is designed to be less influenced by the presence of multiple vehicles on the bridge, it is recommended to avoid the presence of multiple vehicles during testing. Additionally, maintaining an average speed of 30 km/h during the test is crucial, as driving too slowly or too quickly may compromise the accuracy of the methodology. Therefore, it is recommended that the speed of the test vehicle closely aligns with the specified speed of 30 km/h. Furthermore, for effective bearing performance evaluation, the test vehicle should move closer to the steel bearings under investigation and over the main girder directly supported by that bearing, if feasible. Thus, adherence to these specific test conditions would ensure the reliability and accuracy of the proposed methodology for bearing condition assessment.
While the current study primarily focuses on the identification of individual bearing health status, it is pertinent to note that plate girder bridges commonly feature multiple bridge bearings arranged on the piers. The method proposed in this article relies on the test vehicle passing closer to the bearing or over the main girder directly resting over the bearing. Consequently, this methodology is deemed more suitable for assessing the performance of individual bearings rather than groups of bearings. Future research efforts should aim to explore the applicability of this method for evaluating the health status of groups of bearings in plate girder bridges. Moreover, with the easy installation of acceleration sensors and data acquisition, the proposed approach could be further gradually matured. It should strive to become a dependable damage identifier in bridge bearings based on extensive data set correlations. Figure 10B illustrates the schematic framework of the proposed approach for bearing condition assessment.
4 Conclusion
This study investigates the performance of steel bearings that could lose their function in bridges due to aging and maintenance limitations. The existing monitoring techniques for steel bearings often lack automation and precision and need to account adequately for the real-time dynamics of bridges. Therefore, this research proposed a response-based approach for identifying malfunctioning steel bearings that mainly utilize the traffic-induced response in the bearing vicinity. By instrumenting malfunctioning and suitable condition steel bearings with measurement sensors, the comparative analysis is performed to demonstrate the applicability of presented approaches to distinguish different bearing performances and identify bearing malfunction. The following conclusions were thus obtained from the current study.
• The comparative response investigation of displacement response reveals that the sliding malfunction of a bearing could alter the rotational demands of the girder. The girder at the malfunctioned bearing exhibited comparatively enlarged vertical deflections.
• Laser displacement sensor-based assessment could provide a detailed comparative study and clearly differentiate the bridge structure’s bearing malfunction under undamaged and damaged conditions. This method is dependent on the known performance of healthy bearings for comparison, and erecting laser sensors needs access to bearing locality, special stands, and sufficient space for data acquisition setup.
• From the acceleration response of the bearing locality, it was reported that the bearing malfunction could alter the vibrational characteristics of both the pier and girder.
• A generalized approach was introduced, independent of the use of a test vehicle and based on CPSD and cross-correlation functions of acceleration response near the bearing. The results revealed that the CS showed a significant correlation with the presence of damage in the bearing.
• A lower CS value indicated the presence of damage or malfunction in the bearing, while a higher CS value was associated with a healthier and properly functioning bearing. A CS value of 1.7 or below indicates severe bearing malfunction in the demonstrated case. A similar methodology for a specific bridge project would involve determining the applicable range of CS for properly functioning and severely malfunctioned cases of bearings. In this manner, the CS value would serve as a quantitative measure to assess the health and performance of the bearings, providing crucial insights for maintenance and decision-making processes in bridge asset management.
The presented approaches identified the bearing malfunctioning issue, and it revealed that the proposed methodology could be utilized in machine learning applications for the early identification of bearing issues and any subsequent need for prompt repairs. In the future, the collected dataset could be used in machine learning methods. The acquired data could be trained with a suitable machine-learning tool for predicting the likelihood of a bridge-bearing malfunction. This could be done by training a model on a dataset of a bridge with functioning and malfunctioning bearings. The trained model could then be employed for the identification of bearing malfunction on bridges based on their sensor readings. This augmentation of sensor data and machine learning algorithms will allow for the early identification of issues and prompt repairs before the malfunction becomes more severe. In addition, the data could also be used to optimize bridge maintenance schedules, allowing maintenance teams to prioritize their efforts and reduce the overall cost of bridge maintenance. In general, the use of machine learning methods on bridge bearing malfunction dataset has the potential to significantly improve the safety and efficiency of bridge maintenance and repair operations.
Data availability statement
The original contributions presented in the study are included in the article/Supplementary material, further inquiries can be directed to the corresponding author.
Author contributions
HW: Writing–review and editing, Writing–original draft, Visualization, Validation, Software, Resources, Methodology, Investigation, Formal Analysis, Data curation, Conceptualization. MS: Writing–review and editing, Writing–original draft, Visualization, Software, Methodology, Investigation, Formal Analysis, Data curation, Conceptualization. AR: Writing–review and editing, Writing–original draft, Validation, Methodology, Investigation, Formal Analysis, Data curation, Conceptualization. SA: Writing–review and editing, Writing–original draft, Visualization, Methodology, Investigation, Formal Analysis. MW: Writing–review and editing, Writing–original draft, Validation, Supervision, Resources, Project administration, Methodology, Investigation, Conceptualization. HS: Writing–review and editing, Validation, Supervision, Resources, Project administration, Investigation, Funding acquisition, Conceptualization.
Funding
The author(s) declare that financial support was received for the research, authorship, and/or publication of this article. Article processing charges were funded by Deutsche Forschungsgemeinschaft (DFG) and the Open Access Publication Fund of the University of Rostock.
Acknowledgments
Authors thankfully acknowledge the support of the Department of Civil Engineering, Ghulam Ishaq Khan Institute of Engineering Sciences and Technology, Pakistan.
Conflict of interest
The authors declare that the research was conducted in the absence of any commercial or financial relationships that could be construed as a potential conflict of interest.
Publisher’s note
All claims expressed in this article are solely those of the authors and do not necessarily represent those of their affiliated organizations, or those of the publisher, the editors and the reviewers. Any product that may be evaluated in this article, or claim that may be made by its manufacturer, is not guaranteed or endorsed by the publisher.
References
Abaqus, S., Fallis, A. G., and Techniques, D. (2013). Abaqus analysis user’s guide. Abaqus 6.14, 53.
Algohi, B., Bakht, B., and Mufti, A. (2017). Long-term study on bearing restraint of a girder bridge. J. Civ. Struct. Health Monit. 7, 45–55. doi:10.1007/s13349-017-0207-x
American Society of Civil Engineers (2017). Infrastructure report card of bridges. Available at: https://Www.Infrastructurereportcard.Org/Cat-Item/Bridges/ (Accessed February 04, 2021).
Bakht, B., and Jaeger, G. (1988). Bearing restraint in slab-on- girder bridges. J. Struct. Eng. 114 (12), 2724–2740. doi:10.1061/(asce)0733-9445(1988)114:12(2724)
Bell, E. S., and Sipple, J. D. (2009). “Accounting for the impact of thermal loads in nondestructive bridge testing,” in Proceedings of the structures congress 2009 (Reston, VA: American Society of Civil Engineers), 1–10.
Catbas, F. N., Susoy, M., and Frangopol, D. M. (2008). Structural health monitoring and reliability estimation: long span truss bridge application with environmental monitoring data. Eng. Struct. 30, 2347–2359. doi:10.1016/j.engstruct.2008.01.013
Chu, K., Hossain, K. M. A., and Lachemi, M. (2022). Joint-free bridges with engineered cementitious composite sliding approach slabs under static and fatigue loading. Eng. Struct. 268, 114787. doi:10.1016/J.ENGSTRUCT.2022.114787
Deng, P., Gan, Z., Hayashikawa, T., and Matsumoto, T. (2020). Seismic response of highway viaducts equipped with lead-rubber bearings under low temperature. Eng. Struct. 209, 110008. doi:10.1016/J.ENGSTRUCT.2019.110008
Dezfuli, F. H., and Alam, M. S. (2016). Seismic vulnerability assessment of a steel-girder highway bridge equipped with different SMA wire-based smart elastomeric isolators. Smart Mater Struct. 25, 075039. doi:10.1088/0964-1726/25/7/075039
Dicleli, M., and Bruneau, M. (1995). An energy approach to sliding of single-span simply supported slab-on-girder steel highway bridges with damaged bearings. Earthq. Eng. Struct. Dyn. 24, 395–409. doi:10.1002/EQE.4290240307
Dicleli, M., and Hindi, R. (2005). Seismic retrofitting of bridges by response modification techniques based on altering bearing fixities. J. Earthq. Eng. 9 (4), 483–495. doi:10.1080/13632460509350552
Ellenberg, A., Kontsos, A., Moon, F., and Bartoli, I. (2016). Bridge related damage quantification using unmanned aerial vehicle imagery. Struct. Control Health Monit. 23, 1168–1179. doi:10.1002/STC.1831
Fan, X., and McCormick, J. (2022). Seismic performance evaluation of corroded steel bridge bearings.
Fan, X., and McCormick, J. P. (2013). “Experimental characterization of aging effects on steel bridge bearings under cyclic loads,” in Proceedings of the ASCE structures congress.
Fan, X., and McCormick, J. P. (2015). Characterisation of the behaviour of steel bridge bearings under cyclic load reversal. Struct. Infrastructure Eng. 11 (6), 744–760. doi:10.1080/15732479.2014.905963
Farrar, C. R., Cornwell, P. J., Doebling, S. W., and Michael, B. (2000). Prime structural health monitoring studies of the alamosa canyon and I-40 bridges.
FIB Bulletins (2003). Monitoring and safety evaluation of existing concrete structures: state-of-art report. Fib Fédération Internationale Du Béton. doi:10.35789/fib.BULL.0022
Fu, Y., and DeWolf, J. (2001). Monitoring and analysis of a bridge with partially restrained bearings. J. Bridge Eng. 6 (1), 23–29. doi:10.1061/(asce)1084-0702(2001)6:1(23)
Galindo, C. M., Belda, J. G., and Hayashikawa, T. (2010). Non-linear seismic dynamic response of curved steel bridges equipped with LRB supports. Steel Constr. 3, 34–41. doi:10.1002/STCO.201010005
Gangone, M. v., Whelan, M. J., and Janoyan, K. D. (2011). Wireless monitoring of a multispan bridge superstructure for diagnostic load testing and system identification. Computer-Aided Civ. Infrastructure Eng. 26, 560–579. doi:10.1111/J.1467-8667.2010.00711.X
Greimann, L. F., Yang, P. S., and Wolde-Tinsae, A. M. (1982). Conference: 62nd Annual Meeting of the Transportation Research Board. Washington District of Columbia, United States: Transportation Research Board.
Hedayati Dezfuli, F., and Alam, M. S. (2017). Smart lead rubber bearings equipped with ferrous shape memory alloy wires for seismically isolating highway bridges. J. Earthq. Eng. 22, 1042–1067. doi:10.1080/13632469.2016.1269692
Helmicki, A., Hunt, V., Shell, M., Lenett, M., Türer, A., Dalal, V., et al. (1999). “Multidimensional performance monitoring of a recently constructed steel-stringer bridge,” in Proceedings of the 2nd international workshop on structural health monitoring (California) (https://hdl.handle.net/11511/54335).
Kayser, J. R., and Nowak, A. S. (1989). Capacity loss due to corrosion in steel girder bridges. J. Struct. Eng. 115, 1525–1537. doi:10.1061/(ASCE)0733-9445(1989)115:6(1525)
Khurram, N., Sasaki, E., Katsuchi, H., and Yamada, H. (2014). Experimental and numerical evaluation of bearing capacity of steel plate girder affected by end panel corrosion. Int. J. Steel Struct. 14 (3), 659–676. doi:10.1007/S13296-014-3023-8
Kim, S.-H., Mha, H.-S., and Lee, S.-W. (2006a). Effects of bearing damage upon seismic behaviors of a multi-span girder bridge. Eng. Struct. 28, 1071–1080. doi:10.1016/j.engstruct.2005.11.015
Kim, S. H., Mha, H. S., and Lee, S. W. (2006b). Effects of bearing damage upon seismic behaviors of a multi-span girder bridge. Eng. Struct. 28, 1071–1080. doi:10.1016/J.ENGSTRUCT.2005.11.015
Konstantinidis, D., Makris, N., and Kelly, J. M. (2009). Experimental investigation on the seismic response of bridge bearings brownian motion and statistical mechanics view project historic structures and monuments view project experimental investigation on the seismic response of bridge bearings.
Li, B., Lei, Y., Zhou, D., Deng, Z., Yang, Y., and Huang, M. (2021). Bearing damage detection of a bridge under the uncertain conditions based on the bayesian framework and matrix perturbation method. Shock Vib. 2021, 1–17. doi:10.1155/2021/5576362
Lindquist, L., Kahn, L. F., Singh, P., and Kurtis, K. E. (2008). Corrosion of steel bridge girder anchor bolts.
Lu, W., Teng, J., Wen, R., Zhu, J., and Li, C. (2017). Malfunction diagnosis of sensors based on correlation of measurements. Meas. Sci. Technol. 28, 024004. doi:10.1088/1361-6501/AA52ED
Mander, J. B., Kim, D.-K., Chen, S. S., and Premus, G. J. (1996). “Response of steel bridge bearings to reversed cyclic loading,” in Technical report NCEER-96-0014, national centre for earthquake engineering research, department of Civil engineering (Buffalo, NY: State University of New York).
Martínez, D., Obrien, E. J., and Sevillano, E. (2017). “Damage detection by drive-by monitoring using the vertical displacements of a bridge,” in Sixth international conference on structural engineering, mechanics and computation (SEMC 2016), 1915–1918. doi:10.1201/9781315641645-316
Mazroi, A., Ru-liang Wang, L., and Murray, T. M. (1980). Effective coefficient of friction of steel bridge bearings. Eng. Ext. Ser., 27–36.
Noury, P., and Eriksson, K. (2017). Failures of high strength stainless steel bridge roller bearings: a review. Eng. Fract. Mech. 180, 315–329. doi:10.1016/J.ENGFRACMECH.2017.06.004
Parool, N., and Rai, D. C. (2020). Seismic fragility of multi-span simply supported bridge with drop spans and steel bearings.
Rahman Bhuiyan, A., and Alam, M. S. (2013). Seismic performance assessment of highway bridges equipped with superelastic shape memory alloy-based laminated rubber isolation bearing. Eng. Struct. 49, 396–407. doi:10.1016/J.ENGSTRUCT.2012.11.022
Roberts, G. W., Brown, C. J., Meng, X., Ogundipe, O., Atkins, C., and Colford, B. (2015). Deflection and frequency monitoring of the forth road bridge, scotland, by GPS. 165, 105–123. doi:10.1680/BREN.9.00022
Rohrmann, R. G., Baessler, M., Said, S., and Schmid, W. (2000). “Ruecker WF structural causes of temperature affected modal data of Civil structures obtained by long time monitoring,” in Proceedings of the 18th international modal analysis conference.
Sagara, A., and Pane, I. (2015). A study on effects of creep and shrinkage in high strength concrete bridges. Procedia Eng. 125, 1087–1093. doi:10.1016/J.PROENG.2015.11.089
Sanayei, M., Brenner, B. R., Santini-Bell, E., Ha, D., Sipple, J. D., Iplikcioglu, M., et al. (2011). “Picard E temperature based monitoring of a composite steel girder bridge,” in Proceedings of the ASCE (Structures Congress), 14–16.
Santo, T., Saito, J., and Masuda, K. (2012). “Transition and recent knowledge of bridge bearings in Japan,” in The 15th world conference on earthquake engineering.
Sato, Y., Sakai, M., and Ohtomo, K. (2008). “Effects of steel bearing performance on global seismic response of a bridge,” in Proceeding of the 14th world conference on earthquake engineering.
Sengsri, P., and Kaewunruen, S. (2020). Additive manufacturing meta-functional composites for engineered bridge bearings: a review. Constr. Build. Mater 262, 120535. doi:10.1016/J.CONBUILDMAT.2020.120535
Sheikhi, J., Fathi, M., Rahnavard, R., and Napolitano, R. (2021). Numerical analysis of natural rubber bearing equipped with steel and shape memory alloys dampers. Structures 32, 1839–1855. doi:10.1016/J.ISTRUC.2021.03.115
Shirato, M., and Tamakoshi, T. (2013). Bridge inspection standards in Japan and US. 29th US - Japan bridge engineering workshop.
Steelman, J. S., Filipov, E. T., Fahnestock, L. A., Revell, J. R., LaFave, J. M., Hajjar, J. F., et al. (2014a). Experimental behavior of steel fixed bearings and implications for seismic bridge response. J. Bridge Eng. 19, A4014007. doi:10.1061/(ASCE)BE.1943-5592.0000540
Steelman, J. S., Filipov, E. T., Fahnestock, L. A., Revell, J. R., LaFave, J. M., Hajjar, J. F., et al. (2014b). Experimental behavior of steel fixed bearings and implications for seismic bridge response. J. Bridge Eng. 19 (8). A4014007. doi:10.1061/(asce)be.1943-5592.0000540
Sun, Z., Siringoringo, D. M., Chen, S., and Lu, J. (2022). Cumulative displacement-based detection of damper malfunction in bridges using data-driven isolation forest algorithm. Eng. Fail Anal. 143, 106849. doi:10.1016/J.ENGFAILANAL.2022.106849
Tsai, M. H., Wu, S. Y., Chang, K. C., and Lee, G. C. (2007). Shaking table tests of a scaled bridge model with rolling-type seismic isolation bearings. Eng. Struct. 29, 694–702. doi:10.1016/J.ENGSTRUCT.2006.05.025
Wang, Y., Yang, D.-H., and Yi, T.-H. (2021). Accurate correlation modeling between wind speed and bridge girder displacement based on a multi-rate fusion method. Sensors 21, 1967. doi:10.3390/s21061967
Whelan, M., Gangone, M., Janoyan, K., Jha Matthew Whelan, R. J., Gangone, M. v., Janoyan, K. D., et al. (2008). Wireless vibration monitoring for damage detection of highway bridges, 6933, 144–154. doi:10.1117/12.776606
Wipf, T. (1990). Use of tilt sensing equipment for monitoring long term bridge movement. Can. J. Civ. Eng. 18, 1033–1046. doi:10.1139/l91-126
Xia, Q., Zhang, J., Tian, Y., and Zhang, Y. (2017). Experimental study of thermal effects on a long-span suspension bridge. J. Bridge Eng. 22, 04017034. doi:10.1061/(ASCE)BE.1943-5592.0001083
Xiang, N., Alam, M. S., and Li, J. (2018). Shake table studies of a highway bridge model by allowing the sliding of laminated-rubber bearings with and without restraining devices. Eng. Struct. 171, 583–601. doi:10.1016/J.ENGSTRUCT.2018.05.121
Xiang, N., Shahria Alam, M., and Li, J. (2019). Yielding steel dampers as restraining devices to Control seismic sliding of laminated rubber bearings for highway bridges: analytical and experimental study. Anal. Exp. Study 24. doi:10.1061/(ASCE)BE.1943-5592.0001487
Xiao, F., Chen, G. S., and Leroy Hulsey, J. (2017). Monitoring bridge dynamic responses using fiber bragg grating tiltmeters. Sensors 17, 2390. doi:10.3390/S17102390
Yamamura, N., and Tanaka, H. (1990). Response analysis of flexible MDF systems for multiple-support seismic excitations. Earthq. Eng. Struct. Dyn. 19, 345–357. doi:10.1002/EQE.4290190305
Yang, Y. B., Chen, L., Wang, Z. L., Liu, Z. Y., Liu, D.-H., Yao, H., et al. (2024). Cancellation of resonance for elastically supported beams subjected to successive moving loads: optimal design condition for bridges. Eng. Struct. 307, 117950. doi:10.1016/j.engstruct.2024.117950
Yoon, H., Hoskere, V., Park, J.-W., and Spencer, B. (2017). Cross-correlation-based structural system identification using unmanned aerial vehicles. Sensors 17, 2075. doi:10.3390/s17092075
Zhao, X., Liu, H., Yu, Y., Xu, X., Hu, W., Li, M., et al. (2015a). Bridge displacement monitoring method based on laser projection-sensing Technology. Sensors 15, 8444–8463. doi:10.3390/S150408444
Zhao, X., Liu, H., Yu, Y., Xu, X., Hu, W., Li, M., et al. (2015b). Bridge displacement monitoring method based on laser projection-sensing Technology. Sensors 15, 8444–8463. doi:10.3390/s150408444
Keywords: bearing malfunction, steel bearings, traffic loading, response measurement, acceleration
Citation: Waqas HA, Sahil M, Riaz A, Ahmed S, Waseem M and Seitz H (2024) Efficient bridge steel bearing health monitoring using laser displacement sensors and wireless accelerometers. Front. Built Environ. 10:1396815. doi: 10.3389/fbuil.2024.1396815
Received: 06 March 2024; Accepted: 20 May 2024;
Published: 10 June 2024.
Edited by:
Xiaohua Li, Chongqing University, ChinaCopyright © 2024 Waqas, Sahil, Riaz, Ahmed, Waseem and Seitz. This is an open-access article distributed under the terms of the Creative Commons Attribution License (CC BY). The use, distribution or reproduction in other forums is permitted, provided the original author(s) and the copyright owner(s) are credited and that the original publication in this journal is cited, in accordance with accepted academic practice. No use, distribution or reproduction is permitted which does not comply with these terms.
*Correspondence: Abdullah Riaz, YWJkdWxsYWgucmlhekB1bmktcm9zdG9jay5kZQ==