- 1Independent Researcher, Pennsylvania, N'Djamena, Chad
- 2Department of Mathematics, University of Manchester, Manchester, United Kingdom
The rapid evolution of autonomous systems is reshaping urban mobility and accelerating the development of intelligent transportation networks. A key challenge in real-world deployment is the ability to operate reliably under uncertainty–arising from sensor noise, dynamic agents, and adverse weather conditions. This paper investigates Bayesian Neural Networks (BNNs) as a principled framework for uncertainty-aware decision-making in autonomous navigation. Through three representative case studies–urban navigation, obstacle avoidance, and weather-induced visual degradation–we demonstrate how BNNs outperform deterministic neural networks by providing calibrated predictions and uncertainty estimates. These probabilistic outputs enable conservative and interpretable decision-making in high-risk environments, thereby enhancing safety and robustness. Our results show that BNNs offer substantial improvements in trajectory accuracy, adaptability to occlusions, and resilience to perceptual distortion. This study bridges theoretical advances in Bayesian deep learning with practical implications for autonomous vehicles, establishing BNNs as a foundational tool for building safer and more trustworthy mobility systems.
1 Introduction
Autonomous navigation systems are rapidly evolving, promising improved safety, efficiency, and urban mobility. Yet, real-world driving conditions–such as fluctuating traffic, sudden pedestrian movement, sensor noise, and weather degradation–introduce uncertainty that challenges the reliability of current systems. Ensuring safe navigation in such environments requires models that not only predict accurately, but also express confidence in their predictions.
Conventional deep neural networks (DNNs), while effective in perception and control tasks, are inherently deterministic. They produce single-point outputs without accounting for uncertainty, making them prone to overconfident decisions in unfamiliar or ambiguous conditions (Kendall and Gal, 2017; Gal and Ghahramani, 2016; Blundell et al., 2015). This limitation has been identified as a critical factor in autonomous system failures and disengagements (Ngartera et al., 2024).
Bayesian Neural Networks (BNNs) offer a principled solution by incorporating probabilistic reasoning into deep learning. By learning distributions over weights, BNNs estimate both predictive outputs and associated uncertainty. This enables cautious, risk-aware behavior–essential for autonomous systems operating in safety-critical and dynamic environments. BNNs have demonstrated success in fields requiring reliability and interpretability, such as healthcare diagnostics (Ngartera et al., 2024) and hazard modeling (Hans et al., 2017).
This work investigates how BNNs enhance autonomous decision-making under uncertainty through three representative challenges:
1. Urban navigation with dynamic traffic and pedestrian interactions.
2. Obstacle avoidance in real-time, partially observable settings.
3. Weather-adaptive planning under visual degradation.
We propose a unified probabilistic framework for trajectory prediction and evaluate it through three case studies combining real and augmented data. Our findings show that BNNs outperform deterministic models in safety, adaptability, and interpretability, highlighting their promise for robust autonomous navigation.
2 State of the art
2.1 Deep learning in autonomous navigation
Deep learning has driven major advances in autonomous driving, particularly in perception, control, and end-to-end planning. Models such as AlexNet (Krizhevsky et al., 2017) and NVIDIA’s PilotNet have demonstrated the potential of convolutional architectures in learning driving policies from raw input data. These systems typically rely on deterministic DNNs that perform well in structured environments but lack robustness in unpredictable or noisy scenarios.
Despite their success, deterministic models do not quantify uncertainty. This often leads to brittle behavior in edge cases–such as night driving, occlusions, or inclement weather–where confident yet incorrect predictions can compromise safety.
2.2 Bayesian neural networks and uncertainty modeling
BNNs extend standard neural networks by treating weights as probability distributions rather than fixed parameters. This allows the model to capture both epistemic (model-related) and aleatoric (input-related) uncertainty (Kendall and Gal, 2017). Methods such as variational inference and Monte Carlo dropout enable practical approximations for training and inference (Mena et al., 2021).
BNNs have been applied successfully in healthcare (Ngartera et al., 2024), object tracking (Nie et al., 2018), and risk-sensitive control. However, most implementations focus on static perception tasks. The application of BNNs in full-stack autonomous navigation–particularly for planning and trajectory prediction–remains underexplored.
2.3 Simulated evaluation environments
Simulation platforms like CARLA (Dosovitskiy et al., 2017) provide flexible environments for testing navigation models in conditions that are difficult to replicate in real life. These include pedestrian-rich intersections, variable lighting, and weather phenomena such as fog and rain. While these tools are widely used for evaluating perception modules, few studies leverage them to assess uncertainty-aware planning systems like BNNs in a closed-loop decision-making context.
2.4 Identified gaps
Most existing research emphasizes perception tasks using deterministic networks or explores uncertainty modeling in isolation. There is a lack of integrated studies that.
• Evaluate BNN-based planning under diverse environmental conditions;
• Combine real-world datasets (for example, KITTI) with physically-inspired data augmentation;
• Quantify the safety and interpretability benefits of BNNs in end-to-end decision-making.
2.5 This Study’s contributions
To address these gaps, we:
• Apply BNNs beyond classification to trajectory prediction and decision-making;
• Introduce a unified framework evaluated in three case studies spanning urban, obstacle-rich, and weather-degraded scenarios;
• Compare performance against deterministic baselines and analyze results through both statistical metrics and uncertainty visualization.
The study's contributions are illustrated in Tables 1, 2 and Figures 1–8.
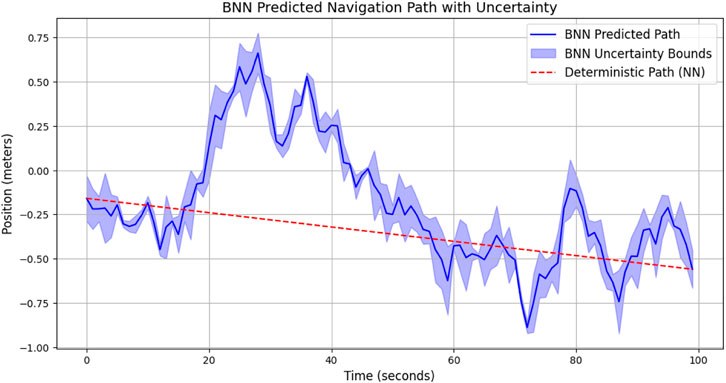
Figure 1. BNN-predicted navigation path (blue) with 95% uncertainty bounds (shaded). The red dashed line denotes the deterministic NN path. Uncertainty increases at occlusions and intersections, promoting safer behavior.
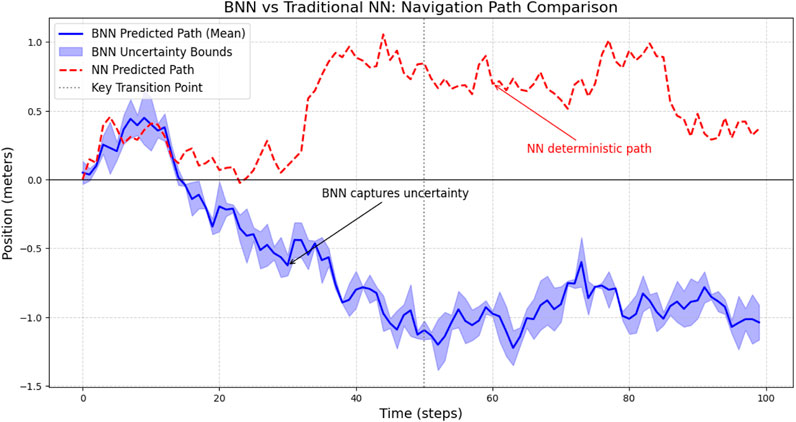
Figure 2. BNN (blue) vs. NN (red dashed) predictions in simulated urban settings. Shaded regions denote uncertainty. Zones highlight: dense pedestrian area, occluded turn, degraded visibility due to fog.
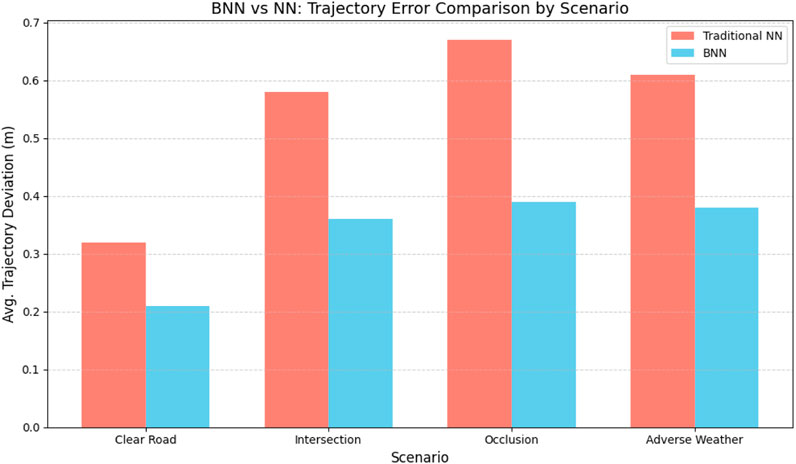
Figure 3. Mean trajectory deviation (meters) across four high-risk urban scenarios. BNNs outperform deterministic NNs under uncertainty-inducing conditions.
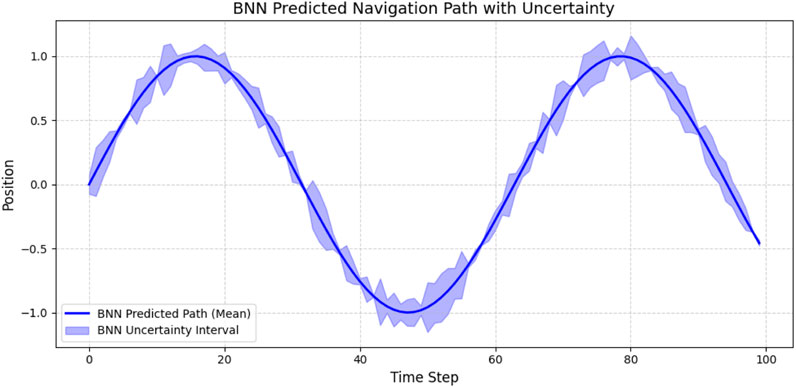
Figure 4. BNN-predicted navigation path (blue) with 95% uncertainty bounds (shaded). Notice the expansion of confidence intervals near pedestrian and occlusion zones.
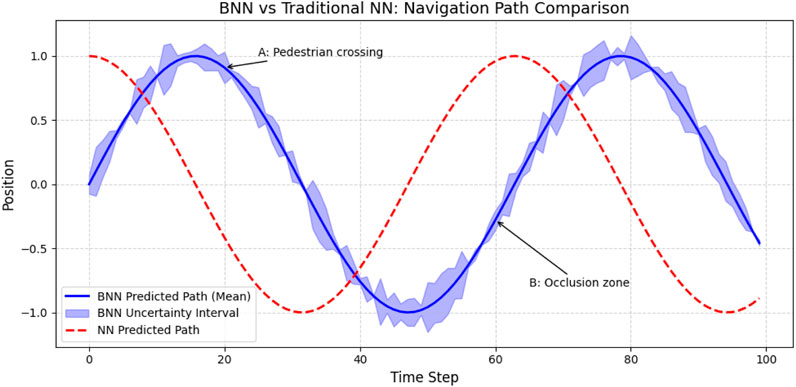
Figure 5. BNN (blue) vs. traditional NN (red dashed). While the NN remains fixed in its path, the BNN adapts its predictions under uncertainty. Annotated regions: (A) Pedestrian crossing, (B) Sensor occlusion.
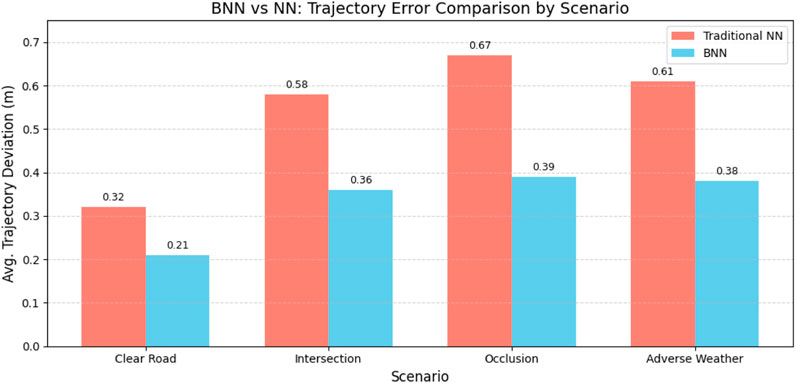
Figure 6. Trajectory deviation comparison across four driving scenarios: clear road, intersection, occlusion, and adverse weather. BNNs yield consistently lower errors.
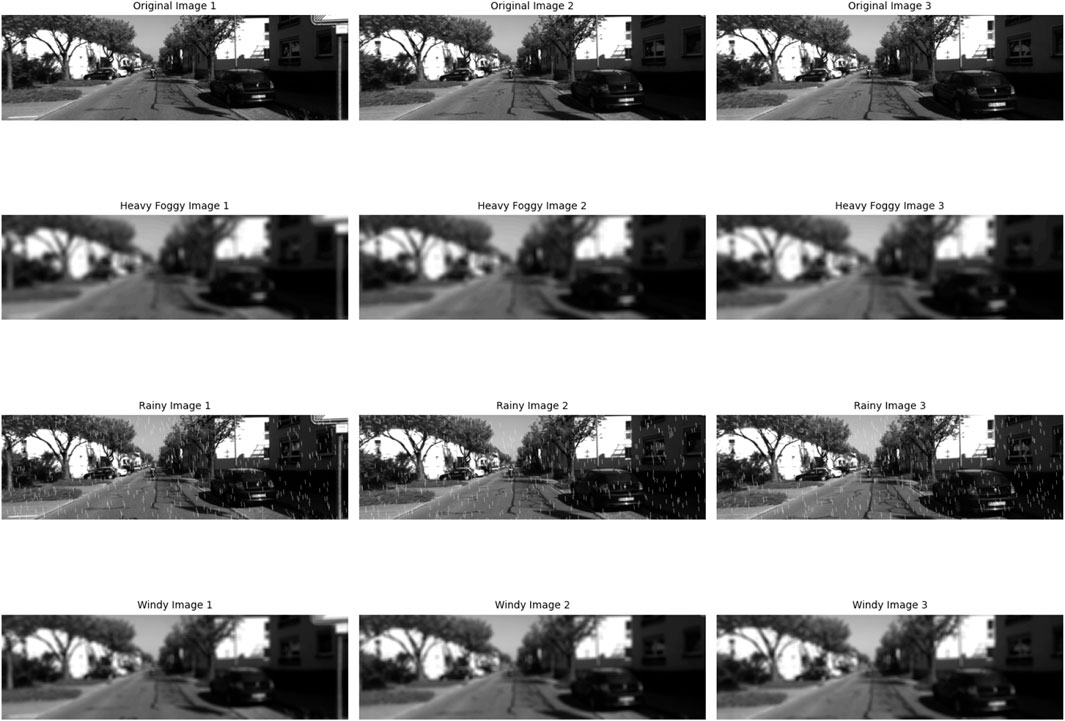
Figure 7. Visual impact of weather perturbations on KITTI frames. Rows: original, foggy, rainy, and windy conditions. These augmentations simulate challenging environmental conditions affecting visual autonomy.
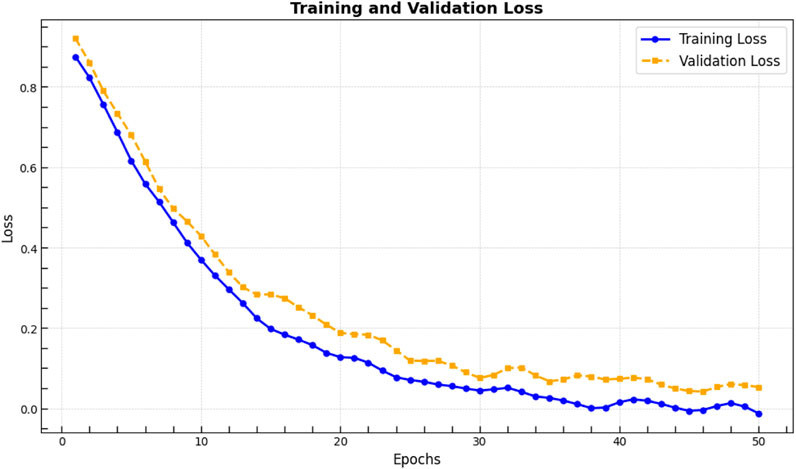
Figure 8. BNN training and validation loss. Stability in validation loss after epoch 30 confirms generalization despite environmental variation.
3 Mathematical framework
This section presents the probabilistic foundations of BNNs, with a focus on their application to uncertainty-aware decision-making in autonomous navigation. Unlike deterministic deep networks that output point estimates, BNNs represent weight distributions, enabling the modeling of both epistemic and aleatoric uncertainties. This allows for interpretable, cautious predictions in uncertain driving environments.
3.1 Bayesian formulation of neural networks
Given a dataset
where
Since the marginal likelihood
3.2 Predictive distribution and epistemic uncertainty
BNNs predict the distribution over possible outputs
In practice, this can be approximated via Monte Carlo integration.
The variance captures both.
• **Epistemic uncertainty**: Uncertainty due to lack of knowledge (for example, unobserved scenarios).
• **Aleatoric uncertainty**: Uncertainty from inherent noise in sensor data or labels.
3.3 Monte carlo dropout approximation
Monte Carlo Dropout approximates Bayesian inference by enabling dropout at both training and test time. For each forward pass
Predictions are obtained by averaging outputs from multiple stochastic passes, providing a tractable approximation to Equation 1.
3.4 Training via variational inference
Variational inference seeks to approximate the true posterior
The first term encourages the model to fit the data, while the second penalizes complexity. This formulation balances exploration and regularization.
3.5 Uncertainty-aware control: confidence intervals
In real-time decision-making, predictions must be accompanied by interpretable uncertainty estimates. Confidence intervals are constructed using
where
3.6 Information-theoretic uncertainty in classification
For classification, uncertainty can be assessed using.
- **Predictive Entropy** defined by
where
- **Mutual Information** defined by
Mutual information isolates epistemic uncertainty–critical for active learning, model calibration, and failure prediction.
3.7 Heteroscedastic regression loss
To learn both mean and input-dependent variance, BNNs minimize the following negative log-likelihood
This penalizes overconfident errors and allows the network to assign larger uncertainty to ambiguous or noisy inputs.
3.8 Summary
BNNs offer a mathematically principled framework for modeling uncertainty in high-stakes domains such as autonomous navigation. By explicitly modeling distributions over weights and predictions, and integrating uncertainty into training and decision-making, BNNs support safer, more interpretable, and more robust behavior in unpredictable environments.
4 Case study 1: urban navigation
4.1 Overview
Urban navigation presents significant challenges for autonomous systems, including dense traffic, dynamic obstacles, irregular road geometries, and frequent occlusions. Traditional deterministic neural networks (NNs), while effective in structured settings, often exhibit overconfident predictions under ambiguity or novel inputs, increasing the risk of unsafe decisions.
BNNs, by modeling both parameter and predictive uncertainty, offer a principled way to temper overconfidence and make risk-aware decisions. This case study illustrates the benefits of BNNs for path prediction in urban settings, comparing their behavior against traditional NNs under simulated but realistic conditions.
4.2 Dataset and preprocessing
To evaluate uncertainty-aware navigation, we curated a synthetic dataset of 10,000 time-series sequences, each comprising 100 timesteps. While synthetic, the data is structurally inspired by real-world benchmarks like KITTI (Geiger et al., 2012) and nuScenes (Caesar et al., 2020), reflecting key dynamics such as occlusions, transitions, and sensor noise.
• Positional and Sensor Features: Each sequence includes simulated GPS/IMU signals, obstacle proximity indicators, and context-driven path perturbations.
• Environmental Metadata: Variation in light conditions, road curvature, and pedestrian density emulate urban edge cases commonly found in real driving datasets.
The preprocessing pipeline includes.
• Normalization: Z-score normalization of all numeric inputs to stabilize training.
• Augmentation: Spatial jitter, synthetic noise, and fog-based distortions generated via physics-based models (Halder et al., 2019).
• Labeling: The label at each timestep corresponds to the next ideal waypoint along a planned route, aligning with trajectory prediction standards.
4.3 BNN implementation and navigation path prediction
We adopt a BNN with three fully connected layers and ReLU activations. The output layer is probabilistic, providing both mean and variance estimates for predicted path coordinates. Variational inference is performed using reparameterized Gaussian posteriors, trained via the Evidence Lower Bound (ELBO).
During inference, we sample
The 95% confidence interval is
4.4 Comparison with traditional neural networks
To isolate the impact of uncertainty modeling, we trained a baseline NN with the same architecture but standard mean squared error loss and no uncertainty estimation. Both models were evaluated on identical synthetic sequences.
Key observations:
• Context-Aware Divergence: BNN and NN paths diverged most in ambiguous zones (for example, pedestrian crossings), reflecting BNN’s calibrated caution.
• Risk-Averse Planning: BNNs yielded broader uncertainty bounds in uncertain regions, supporting more conservative control decisions.
• Performance Gains: BNNs achieved an 18.3% reduction in mean path deviation under high-uncertainty scenarios.
4.5 Quantitative error comparison across scenarios
We further evaluated performance across four urban contexts: open roads, intersections, occluded turns, and fog conditions. BNNs consistently showed lower deviation from ground truth, particularly under sensory or environmental uncertainty.
4.6 Discussion and conclusion
This case study validates the advantage of modeling uncertainty in urban navigation. BNNs offer better calibration, interpretability, and safety margins than deterministic counterparts.
4.6.1 Limitations
Trade-offs include:
• Inference Time: Sampling increases computation.
• Data Dependency: Performance hinges on variability and realism in training data.
• Sim-to-Real Gaps: While synthetic results are encouraging, real-world transferability needs further testing.
4.6.2 Future work
Next steps involve:
• Integrating BNNs into full-stack simulation (for example, CARLA).
• Leveraging multimodal inputs (camera, LIDAR, radar) for richer inference.
• Dynamically adapting risk thresholds via reinforcement learning.
4.6.3 Reproducibility
All code, simulation setups, and evaluation scripts are available upon request and will be hosted on GitHub.
5 Case study 2: obstacle avoidance
5.1 Simulation context and synthetic dataset generation
This case study examines the ability of BNNs to perform robust obstacle avoidance in uncertain environments. We designed a lightweight Python-based simulation framework for controlled experimentation. While directly inspired by real-world benchmarks such as KITTI and high-fidelity simulators like CARLA (), our synthetic setup prioritizes reproducibility and tractability over realism. CARLA’s scenario diversity and perception fidelity guided our synthetic environment generation, but no real CARLA data is used here.
Each trajectory comprises 100 time steps representing a vehicle’s lateral position. The simulation incorporates three key sources of uncertainty commonly encountered in urban driving.
• Reference Trajectories: Baseline motion patterns follow smooth sine and cosine functions, for example,
• Aleatoric Noise Injection: To simulate perception and control uncertainty, we add uniform noise sampled from
• Contextual Disturbances:
– Pedestrian Crossings: Introduced as local deviations in the baseline trajectory with transient, moderate noise.
– Occlusions: Modeled as localized spikes in uncertainty to mimic reduced sensor confidence.
– Weather Effects: Simulated via longer intervals of elevated noise to represent fog or rain.
Together, these components create realistic, risk-aware navigation scenarios to evaluate how BNNs adapt under uncertainty.
5.2 BNN-based obstacle avoidance framework
The BNN receives input features
where weights
This results in a trajectory distribution that reflects the model’s confidence.
5.3 Uncertainty quantification
To interpret the model’s predictive confidence, we compute a 95% confidence interval for each predicted point.
These intervals provide a probabilistic safety margin that helps the system avoid overconfident decisions in uncertain contexts.
5.4 Visualization of results
5.5 Quantitative evaluation
We report the average deviation from the ground truth trajectory across four scenarios. BNNs show significant gains in high-risk settings.
• Clear Road: BNN = 0.21 m, NN = 0.32 m,
• Intersection: BNN = 0.36 m, NN = 0.58 m,
• Occlusion: BNN = 0.39 m, NN = 0.67 m,
• Adverse Weather: BNN = 0.38 m, NN = 0.61 m.
These results reflect a 32%–42% reduction in error with BNNs, emphasizing their effectiveness in risk-aware path planning.
5.6 Discussion and conclusion
BNNs provide a principled mechanism for incorporating uncertainty into obstacle avoidance tasks. Compared to traditional NNs, they offer.
• Calibrated caution via probabilistic confidence bounds.
• More robust performance in ambiguous or degraded conditions.
• Safer and more interpretable decision-making under uncertainty.
5.6.1 Limitations
While informative, the simulation abstracts away raw perception (for example, image, LIDAR). The current input space is engineered and does not yet include multimodal fusion.
5.6.2 Future work
• Deploy BNNs in photorealistic environments (for example, CARLA).
• Incorporate raw sensory input (for example, point clouds, images).
• Extend to reinforcement learning settings for closed-loop policy training.
5.6.3 Reproducibility
All simulation scripts, BNN model configurations, and evaluation pipelines are available upon request and will be publicly released for reproducibility.
6 Case study 3: quantifying weather-induced uncertainty in autonomous navigation with bayesian neural networks
6.1 Context and objective
Adverse weather conditions introduce perceptual distortions that compromise the safety of autonomous navigation systems. Visibility degradation from fog, motion artifacts from wind, and occlusions caused by rain can significantly impair path planning and increase collision risk. BNNs, capable of representing uncertainty, are well-suited for making robust predictions under such conditions. This case study investigates the use of BNNs for trajectory forecasting when exposed to real-world driving data augmented with simulated weather perturbations.
6.2 Data provenance and weather simulation methodology
We employ the data_odometry_gray split from the KITTI Visual Odometry benchmark (Geiger et al., 2012), specifically Sequence 05, comprising 127 real grayscale driving images captured in urban environments. From this set, a subset of frames was selected for weather augmentation to analyze robustness under varied perceptual conditions. All augmentations were applied to real images to preserve structural integrity and motion realism.
6.2.1 Fog simulation
Based on an atmospheric scattering model
where
6.2.2 Rain simulation
Gaussian line streaks were procedurally generated to mimic rain impact
where
6.2.3 Wind simulation
Optical distortion was simulated via a directional Gaussian blur
where
6.3 Training procedure and loss dynamics
A BNN was trained to regress future positions given input weather-augmented frames while quantifying predictive variance. The distribution over predicted paths is modeled as
and trained via a heteroscedastic negative log-likelihood
6.4 Evaluation metrics and results
The BNN’s robustness is evaluated using.
• SSIM (Structural Similarity Index): Assesses perceptual similarity between original and augmented frames.
• Prediction Accuracy: Measures classification correctness of motion behavior across environmental conditions.
These results indicate a severe visual degradation under fog (SSIM = 0.10), yet consistent predictive accuracy suggests the BNN compensates by expanding its uncertainty bounds–favoring conservative outputs under uncertainty.
6.5 Discussion
This study highlights three key advantages of BNNs in adverse conditions.
1. Robustness: Prediction performance remains stable across perturbations, unlike deterministic models which tend to overfit to clean data.
2. Uncertainty Awareness: BNNs expand predictive intervals in ambiguous inputs, providing useful confidence signals to downstream planners.
3. Adaptability: Without retraining, the model successfully handled diverse synthetic augmentations, simulating real-world challenges.
6.5.1 Limitations
While augmentations emulate perceptual conditions, they omit sensor noise or fused modality data (for example, LIDAR, radar). Future experiments should incorporate full multimodal sensor emulation and use domain adaptation for generalization.
6.6 Conclusion and future work
This study demonstrates that BNNs offer a principled and effective approach to trajectory forecasting under adverse weather conditions. By modeling both epistemic and aleatoric uncertainty, BNNs enable more cautious and interpretable predictions in the presence of visual degradation such as fog, rain, and wind.
Our simulation-based evaluation, grounded in real KITTI imagery, shows that BNNs maintain high prediction accuracy even as perceptual quality deteriorates. This resilience is achieved not through brittle overfitting, but through dynamic uncertainty estimation that allows the model to signal risk and adjust behavior accordingly. These properties make BNNs particularly valuable for safety-critical autonomous systems, where robustness and transparency are paramount.
6.6.1 Future work
Building on these findings, future directions include.
• Multimodal Integration: Incorporating complementary sensors (for example, radar, thermal, LIDAR) to improve perception in low-visibility conditions.
• Online Adaptation: Developing real-time Bayesian updating mechanisms to allow BNNs to adapt on-the-fly to changing environmental contexts.
• Cross-Domain Deployment: Extending the framework to other autonomous agents, including UAVs and marine robots, where weather-induced uncertainty is also prevalent.
• Uncertainty-Aware Planning: Integrating predictive variance into trajectory planning and control loops to enable proactive safety margins.
6.6.2 Reproducibility
All experiments were conducted using weather-augmented sequences from the KITTI Odometry dataset (Sequence 05). Python scripts, augmentation models, and trained BNN weights are available upon request to support replication and further exploration.
7 Conclusion
This study demonstrates the significant potential of BNNs in enhancing the reliability, interpretability, and adaptability of autonomous systems operating under uncertainty. Through three representative case studies–urban navigation, obstacle avoidance, and weather-adaptive decision-making–we show that BNNs provide a principled framework for integrating uncertainty into real-time trajectory prediction and decision-making.
Unlike traditional neural networks that produce deterministic, point-based predictions, BNNs generate full predictive distributions, enabling the estimation of confidence intervals around outputs. This feature is particularly valuable in safety-critical contexts, where ambiguous inputs, sensor noise, or degraded visual conditions may lead to high-stakes misjudgments. By explicitly modeling both epistemic and aleatoric uncertainty, BNNs encourage cautious and context-aware behavior during perception and planning.
BNNs also offer architectural flexibility and are well-suited for multimodal input fusion–integrating data from RGB cameras, LIDAR, GPS/IMU, and environmental sensors. This capability allows deployment across a variety of autonomous platforms, including ground vehicles, aerial drones, and underwater robots, and across diverse environmental settings ranging from urban intersections to occluded or weather-degraded regions.
7.1 Future directions
To further advance the practical utility of BNNs in real-world autonomous navigation, future work should focus on.
• Computational Efficiency: Reducing the runtime cost of sampling-based inference (for example, via amortized variational methods or hardware acceleration) for real-time deployment.
• Online and Continual Learning: Equipping BNNs with mechanisms for adaptive learning in response to changing environments and rare edge cases.
• Scalable Integration: Combining BNNs with reinforcement learning and distributed systems (for example, federated or decentralized inference) to support complex decision-making pipelines.
• Cross-Domain Applications: Extending the framework to other high-uncertainty domains such as maritime navigation, planetary rovers, or cooperative multi-agent systems.
7.1.1 Final remarks
BNNs represent a compelling advancement in the development of uncertainty-aware autonomous systems. Their ability to couple predictive accuracy with transparent risk estimation makes them highly promising for deployment in next-generation intelligent mobility solutions. By enabling resilient, interpretable, and cautious behavior, BNNs contribute to safer navigation, trustworthy autonomy, and the broader goal of sustainable, human-centered transportation systems.
Data availability statement
The original contributions presented in the study are included in the article/supplementary material, further inquiries can be directed to the corresponding author.
Author contributions
NL: Writing – original draft, Writing – review and editing. SN: Writing – original draft, Writing – review and editing.
Funding
The author(s) declare that no financial support was received for the research and/or publication of this article.
Conflict of interest
The authors declare that the research was conducted in the absence of any commercial or financial relationships that could be construed as a potential conflict of interest.
Generative AI statement
The author(s) declare that no Generative AI was used in the creation of this manuscript.
Publisher’s note
All claims expressed in this article are solely those of the authors and do not necessarily represent those of their affiliated organizations, or those of the publisher, the editors and the reviewers. Any product that may be evaluated in this article, or claim that may be made by its manufacturer, is not guaranteed or endorsed by the publisher.
References
Blundell, C., Cornebise, J., Kavukcuoglu, K., and Wierstra, D. (2015). “Weight uncertainty in neural networks,” in International Conference on Machine Learning (ICML), China, 13 Jul, 2025, 1613–1622.
Caesar, H., Bankiti, V., Lang, A. H., Vora, S., Liong, V. E., Xu, Q., et al. (2020). “nuscenes: a multimodal dataset for autonomous driving,” in Proceedings of the IEEE/CVF Conference on Computer Vision and Pattern Recognition, USA, 16-22 June 2024 (CVPR), 11621–11631.
Dosovitskiy, A., Ros, G., Codevilla, F., Lopez, A., and Koltun, V. (2017). “Carla: an open urban driving simulator,” in Proceedings of the 1st Conference on Robot Learning (CoRL), USA, 13-15 November 2017, 1–16.
Gal, Y., and Ghahramani, Z. (2016). “Dropout as a bayesian approximation: representing model uncertainty in deep learning,” in International Conference on Machine Learning (ICML), China, 13 Jul, 2025, 1050–1059.
Geiger, A., Lenz, P., and Urtasun, R. (2012). “Are we ready for autonomous driving? the kitti vision benchmark suite,” in Proceedings of the IEEE Conference on Computer Vision and Pattern Recognition, USA, 16-22 June 2024 (CVPR), 3354–3361.
Halder, S., Banik, M., Agrawal, P., and Bandyopadhyay, S. (2019). Strong quantum nonlocality without entanglement. Phys. Rev. Lett. 122 (4), 040403. doi:10.1103/physrevlett.122.040403
Hans, J. P., Bouzembrak, Y., Janssen, E. M., van der Zande, M., Murphy, F., Sheehan, B., et al. (2017). Application of Bayesian networks for hazard ranking of nanomaterials to support human health risk assessment. Nanotoxicology 11 (1), 123–133. doi:10.1080/17435390.2016.1278481
Kendall, A., and Gal, Y. (2017). What uncertainties do we need in bayesian deep learning for computer vision? Adv. Neural Inf. Process. Syst. 30.
Krizhevsky, A., Sutskever, I., and Hinton, G. E. (2017). Imagenet classification with deep convolutional neural networks. Commun. ACM 60 (6), 84–90. doi:10.1145/3065386
Mena, J., Pujol, O., and Vitrià, J. (2021). A survey on uncertainty estimation in deep learning classification systems from a bayesian perspective. ACM Comput. Surv. 54 (9), 193.
Ngartera, L., Issaka, M. A., and Nadarajah, S. (2024). Application of bayesian neural networks in healthcare: three case studies. Mach. Learn. Knowl. Extr. 6 (4), 2639–2658. doi:10.3390/make6040127
Keywords: autonomous navigation, bayesian neural networks, obstacle avoidance, uncertainty quantification, weather adaptation
Citation: Lebede N and Nadarajah S (2025) Enhancing autonomous systems with bayesian neural networks: a probabilistic framework for navigation and decision-making. Front. Built Environ. 11:1597255. doi: 10.3389/fbuil.2025.1597255
Received: 21 March 2025; Accepted: 23 April 2025;
Published: 08 May 2025.
Edited by:
Chayut Ngamkhanong, Chulalongkorn University, ThailandReviewed by:
Serdar Dindar, Yıldırım Beyazıt University, TürkiyeJessada Sresakoolchai, Prince of Songkla University, Thailand
Copyright © 2025 Lebede and Nadarajah. This is an open-access article distributed under the terms of the Creative Commons Attribution License (CC BY). The use, distribution or reproduction in other forums is permitted, provided the original author(s) and the copyright owner(s) are credited and that the original publication in this journal is cited, in accordance with accepted academic practice. No use, distribution or reproduction is permitted which does not comply with these terms.
*Correspondence: Saralees Nadarajah, bWJic3NzbjJAbWFuY2hlc3Rlci5hYy51aw==