- 1Cecil H. and Ida Green Center for Reproductive Biology Sciences, University of Texas Southwestern Medical Center, Dallas, TX, United States
- 2Division of Basic Reproductive Biology Research, Department of Obstetrics and Gynecology, Department of Bioinformatics, University of Texas Southwestern Medical Center, Dallas, TX, United States
Large scale cancer genomic studies in patients have unveiled millions of non-coding variants. While a handful have been shown to drive cancer development, the vast majority have unknown function. This review describes the challenges of functionally annotating non-coding cancer variants and understanding how they contribute to cancer. We summarize recently developed high-throughput technologies to address these challenges. Finally, we outline future prospects for non-coding cancer genetics to help catalyze personalized cancer therapy.
The challenges of interpreting non-coding variant function in cancer
Understanding the molecular mechanism of a cancer variant has clear implications to treatment. For example, KRAS is frequently mutated in several cancers and the G12C mutation locks KRAS into an active oncogenic state. Functional profiling of mutant KRAS led to the development of a new class of inhibitors that were recently FDA approved for patients with KRAS G12C mutations (Ostrem et al., 2013; Lito et al., 2016; Janes et al., 2018; Canon et al., 2019). This example stresses the need to 1) map cancer variants and 2) understand their mechanisms. There are different kinds of cancer variants, and they can be grouped based on the technologies used to identify them.
• Somatic mutation identified by sequencing studies: Somatic mutations are variants found by comparing the tumor and non-tumor samples from the same patient, including point mutations, copy number variants and structural variants. In this review, we focus on the point mutations category. Most of the somatic mutations are acquired. The Cancer Genome Atlas (TCGA) has mapped many coding somatic mutations in cancer with whole exome sequencing, focusing on protein coding regions of the genome (Weinstein et al., 2013; Bailey et al., 2018; Ding et al., 2018). The KRAS G12C example mentioned above is one example of a coding somatic mutation. Recently, the International Cancer Genome Consortium (ICGC) broadly applied whole genome sequencing (WGS) to identify millions of somatic mutations in non-coding regions, which span >95% of the human genome (ICGC/TCGA Pan-Cancer Analysis of Whole Genomes Consortium, 2020; Rheinbay et al., 2020). In addition, sequencing studies can also identify germline variants with cancer relevance (Huang et al., 2018). Excellent online resources such as COSMIC, the cBio cancer genome portal, and CNCDatabase now catalog these sequenced variants (Cerami et al., 2012; Tate et al., 2019; Liu et al., 2021).
• Single nucleotide polymorphisms (SNPs) identified by Genome-wide association studies (GWAS): By comparing the allelic frequency in large populations of patients and controls using genotyping arrays, GWAS have identified many inherited cancer-associated SNPs, including breast cancer, ovarian cancer and prostate cancer (Turnbull et al., 2010; Michailidou et al., 2013; Michailidou et al., 2015; Michailidou et al., 2017; Milne et al., 2017; Zhang et al., 2020). Like somatic mutations, many GWAS SNPs are also enriched in non-coding parts of the genome (Corradin and Scacheri, 2014). Public resources for cancer-relevant GWAS SNPs include the GWAS Catalog and PLCO (Ruan et al., 2022; Sollis et al., 2023).
Somatic variants and GWAS variants have different implications for cancer development. First, GWAS variants are associated with increased cancer risk while somatic variants can drive cancer development. GWAS variants are derived from comparing the blood samples from patient and control populations, which are mostly inherited, but somatic mutations compare the tumor and non-tumor samples from the same patient, reflecting personalized acquired variation. Second, somatic mutations precisely point to single base pair change of function during cancer development while most GWAS variants indicate a risk locus. Although somatic mutations are at single-base resolution, the sample size is smaller than cancer GWAS studies, which makes it difficult to distinguish passenger mutations and driver mutations.
While numerous cancer-associated variants have been identified by different studies (Table 1), elucidating the role of the majority, especially those in non-coding regions, remains a challenge. Unlike coding variants with predictable effects on amino acids, non-coding variants pose unique challenges. First, non-coding variants impact diverse elements in the genome with unique functions. Thus, non-coding variants can influence cancer development through different mechanisms, for example by altering the activity of regulatory elements, modifying gene splicing, and altering miRNA function (described in the next section). Second, the number of non-coding variants far surpasses that of coding variants (Figure 1 (ICGC/TCGA Pan-Cancer Analysis of Whole Genomes Consortium, 2020)). Problematically, many non-coding variants could be passengers rather than drivers. Functionally distinguishing the two possibilities is of utmost importance and will require new approaches for systematic functional analysis. Third, while many non-coding variants influence disease by modifying the expression of cancer genes, the assignment of variants to cancer genes may not be straightforward. For example, non-coding variants often localize to intergenic regions, which complicates efforts to understand how they link to cancer-relevant genes, pathways, and phenotypes. Addressing these challenges to a functional understanding of non-coding variants will be important to personalized medicine in cancer.
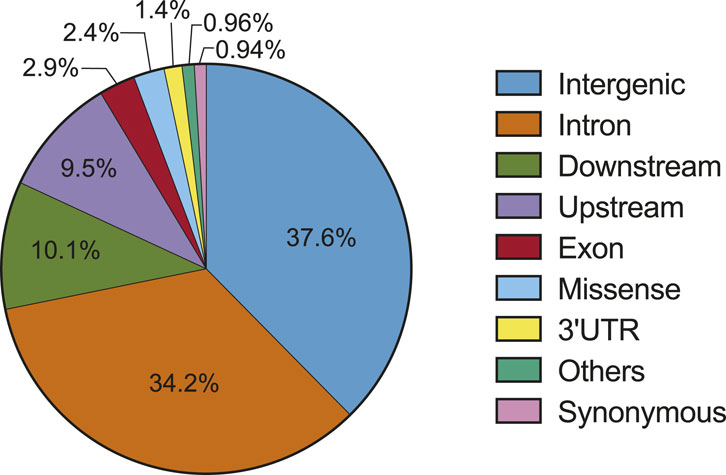
Figure 1. Distribution of somatic variants in the genome (source: PCAWG) (ICGC/TCGA Pan-Cancer Analysis of Whole Genomes Consortium, 2020).
This review describes current challenges to non-coding variant interpretation in cancer and introduces recent technological innovations that will decode the non-coding landscape of cancer genomes.
Diverse functions of non-coding variants in cancer
Non-coding cancer variants can be found at broad classes of regulatory elements including promoters, enhancers, and microRNAs. This functional diversity presents complicates efforts to understand their impact on cancer development.
Promoter activity alteration
Gene expression is regulated by promoters, which recruit transcription factors and RNA polymerase to initiate transcription. Promoter variants may alter transcription factor binding sites to impact the rate of transcriptional initiation and/or elongation (Perera et al., 2016). One notable example is the telomerase reverse transcriptase (TERT) promoter, which is a hotspot of mutation in multiple cancer types (Landa et al., 2013; Vinagre et al., 2013; Borah et al., 2015). In melanoma, TERT promoter mutations lead to increased transcription due to the generation of new binding motifs for ETS transcription factors (Horn et al., 2013). The mutant TERT promoter also harbors epigenetic features of activity, including decreased DNA methylation and increased enrichment of the histone modification H3K4me3 (Stern et al., 2017). Another example is the SNP309 variant located in the MDM2 promoter, which increases the affinity of transcription activator SP1 (Bond et al., 2004). Since MDM2 is a negative regulator of tumor suppressor p53, this variant indirectly leads to a lower level of TP53, and is associated with accelerated tumor formation. These examples highlight the impact of promoter variants on cancer development.
Enhancer activity modification
Non-coding variants are especially enriched at transcriptional enhancers (Corradin and Scacheri, 2014), which are promoter-distal regulatory elements that serve as a platform to bind transcription factors and activate gene expression from a distance (Schoenfelder and Fraser, 2019). Since enhancer activity is exquisitely cell type and tissue type specific (Heintzman et al., 2009; Visel et al., 2009), these regulatory elements drive the diverse expression patterns of different cell types. Genome-wide mapping of enhancers through chromatin profiling have consistently illustrated the strong enrichment of enhancers with GWAS variants across many disease contexts including cancer (Visel et al., 2009; Creyghton et al., 2010; Rada-Iglesias et al., 2011; Pradeepa et al., 2016). Several examples underscore the important roles that enhancers play in cancer development (Mansour et al., 2014; Morton et al., 2019; Huang et al., 2021; Leeman-Neill et al., 2023). For example, a gain-of-function non-coding variant in leukemia creates a new MYB binding site, activating a new enhancer that induces the oncogene TAL1 (Mansour et al., 2014). As another example, the FOXC1 enhancer regulates invasion in triple negative breast cancer cells (Huang et al., 2021). Non-coding mutations convert this enhancer to target a different gene (ZCCHC7), which contributes to cancer development by rewiring protein synthesis (Leeman-Neill et al., 2023). Finally, a more dramatic mechanism of enhancer dysregulation in cancer is enhancer hijacking, where chromosomal rearrangements cause enhancer-mediated activation of oncogenes such as MYC (Xu et al., 2022). In summary, genetic variants can alter enhancer activity or enhancer position, propelling cancer development.
Transcript splicing alternation
Non-coding variants can also drive cancer through alternative splicing. Abnormal splicing widely occurs in multiple cancer types (Jung et al., 2015; Jayasinghe et al., 2018). For example, mutations of BCL2L1 gene induce apoptotic resistance in breast cancer and prostate cancer through up-regulating the anti-apoptotic form of alternative splicing transcripts (Boise et al., 1993; Bauman et al., 2010; Sveen et al., 2016). Another mechanism is that abnormal splicing leads to intron retention of tumor suppressor genes such as ARID1A, PTEN and TP53, which further inactive the function of those tumor suppressor genes (Jung et al., 2015).
miRNAs dysfunction
Non-coding variants in miRNAs can also contribute to cancer development. miRNAs fine tune gene expression post-transcriptionally by binding to the 3′UTR of target mRNA with complementary sequence, with impacts on translation inhibition or transcript degradation (Lujambio and Lowe, 2012; Bartel, 2018). Cancer associated variants can alter miRNA seed sequences or miRNA binding sites on the 3′UTRs of target transcripts. Systematic analysis has identified cancer mutations enriched in specific miRNAs, which are strongly correlated to cancer gene programs (Urbanek-Trzeciak et al., 2020). One example is hsa-let-7d, which is implicated in breast cancer, ovarian cancer and colorectal cancer (Jiang et al., 2018; Wei et al., 2018; Chen et al., 2019; Urbanek-Trzeciak et al., 2020). hsa-let-7d post-transcriptionally regulates multiple oncogenes and tumor suppressors. In breast cancer, has-let-7d negatively regulates the expression of Jab1, a proliferation pathway regulator. In ovarian cancer, has-let-7d blocks the p53 signaling pathway through HMGA1.
In summary, non-coding mutations affect cancer development through several mechanisms. Linking non-coding variants to cancer genes and pathways is a key step to understanding how they contribute to cancer.
High throughput approaches to functionally annotate cancer variants
The abundance of non-coding mutations in cancer necessitates advanced technologies for comprehensive functional studies (Figure 1). Here, we summarize contemporary technologies for the high-throughput analysis of non-coding variants, with a focus on characterizing the impact of variants and regulatory elements, particularly promoters and enhancers (Figure 2).
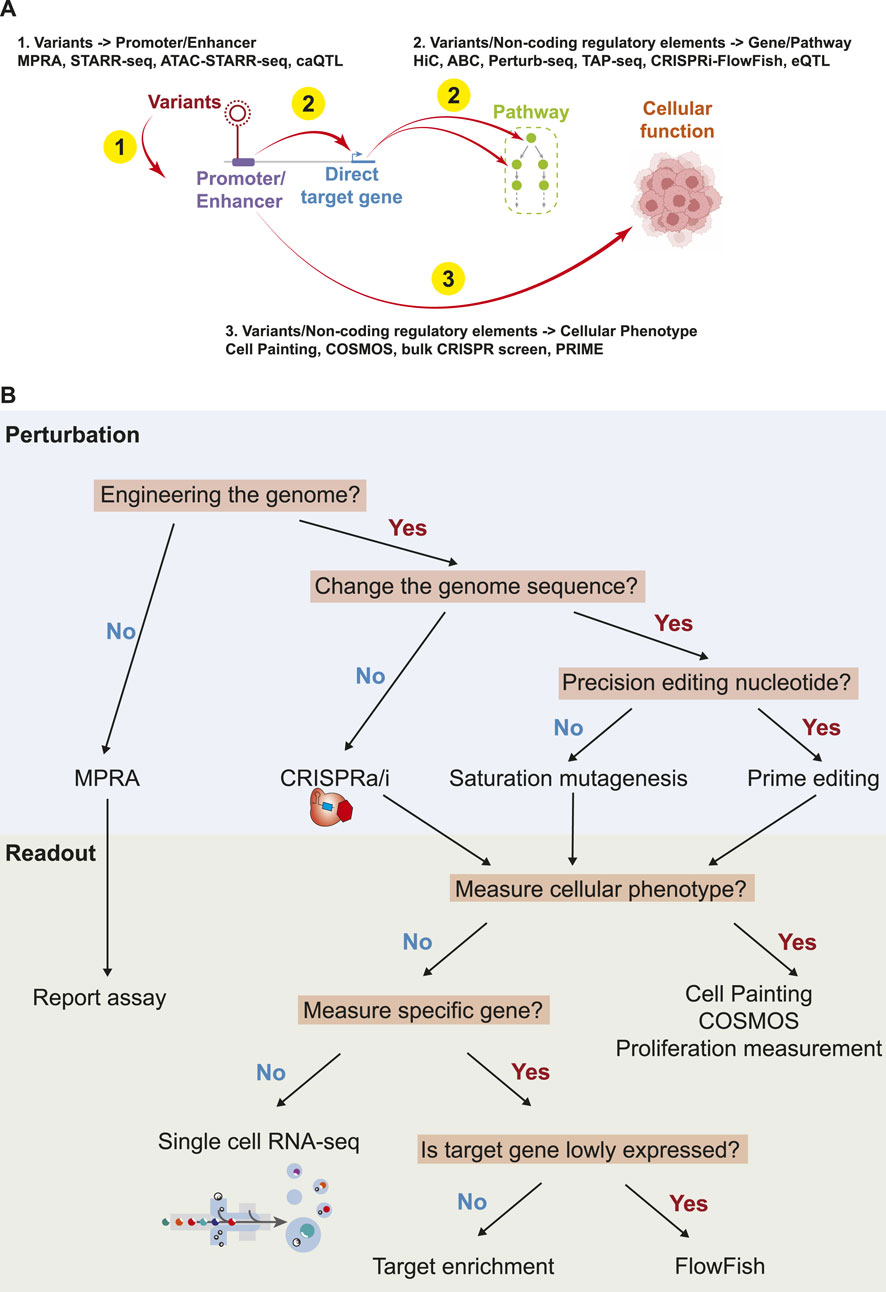
Figure 2. Summary of biological links, and the technologies to understand the links. (A). Summary of technologies to understand the function of non-coding variants/regulatory elements. (B). An overview of perturbations and readouts for high throughput technologies.
Assessing the impact of non-coding variants on promoter/enhancer activity
Massive Parallel Reporter Assays (MPRA) can simultaneously quantify the activities of millions of promoters and enhancers to drive gene expression (Kircher et al., 2019; Shigaki et al., 2019; Choi et al., 2020; Long et al., 2022). MPRAs are carried out by high throughput cloning of synthetic elements (typically <300 bp) together with transcribed genetic barcodes into a plasmid with a reporter gene, followed by transduction into cells and RNA readout of barcode expression. Importantly, since MPRAs compare the activities of synthesized sequences, they are compatible with the high throughput assessment of non-coding variants compared to control sequences. Although the throughput of MPRAs can be extremely high, one disadvantage is the lack of genomic context. For example, one study applied MPRAs to several hundred melanoma variants and verified multiple variants regulating MX2 activity (Choi et al., 2020). Another study examined more than 1,000 multiple myeloma variants and identified causal variants at six loci (Ajore et al., 2022). Like MPRAs, STARR-seq also quantifies the transcriptional activity of regulatory elements through high throughput reporter assays, with the key difference being that tested sequences are isolated using a biochemical assay like ChIP-Seq or ATAC-Seq (Arnold et al., 2013; Hansen and Hodges, 2022). One study used STARR-seq to systematically identify hundreds of SNPs with the ability to regulate gene expression and to verify that the rs11055880 SNP regulates ATF7IP in breast cancer (Liu et al., 2017). Another recent study applied STARR-seq to find that transposable elements have functional enhancer activity in cancer (Karttunen et al., 2023). The strength of MPRAs and STARR-Seq is the low cost to functionally examine non-coding sequences, which enables large-scale studies of enhancers, promoters, and their variants. However, one key disadvantage is that MRPAs test sequences outside of their native genomic context.
Chromatin accessibility quantitative trait loci (caQTL) studies test the association of genetic variants and chromatin accessibility by performing ATAC-Seq in a large cohort of genetically diverse individuals (Tehranchi et al., 2019; Ajore et al., 2022; Wang et al., 2022). By profiling the chromatin accessibility from cancer patients, one can test if SNPs at promoters and enhancers are associated with gain or loss of function. The approach can be applied with somatic mutation as well. One study in bladder cancer patients identified a somatic variant that generates new binding sites for NKX2-8 with a dramatic increase in open chromatin accessibility, which results in FGD4 upregulation and low patient survival rate (Corces et al., 2018).
Assessing the impact of variants/non-coding regulatory elements on gene expression
Non-coding variants that alter the activity of promoters and enhancers (previous section) can lead to downstream changes in gene expression and pathway activity to influence cancer development (Bauer et al., 2013). For example, one study documented a non-coding cancer variant that converts an enhancer to target ZCCHC7, leading to protein synthesis rewiring and cancer development (Leeman-Neill et al., 2023). In this section, we discuss both computational and experimental approaches to dissect the impact of variants or non-coding regulatory elements to genes and pathways.
While non-coding variants are enriched within enhancers (Corradin and Scacheri, 2014), a key unresolved question is: what are the target genes of these enhancers? Several computational approaches have been developed to address this question. One approach uses three-dimensional chromatin confirmation information, for example with genome-wide HiC data (Lieberman-Aiden et al., 2009), to link enhancers to target genes. One study used HiC to demonstrate that the chromatin structure of the androgen receptor (AR) locus is altered in prostate cancer (Rhie et al., 2019). HiC with single-cell resolution has also been developed to identify cell type specific enhancer regulation (Zhang et al., 2022). One computational approach, the ABC (Activity-by-contact) model, predicts enhancer target genes across the genome as a function of enhancer strength and the 3D chromatin contact frequency (Fulco et al., 2019; Ying et al., 2023). One study demonstrated that an enhancer with variant rs4810856 regulates PREX1, CSE1L and STAU1 expression and activates p-AKT signaling in colorectal cancer (Ying et al., 2023). A recent advance is the development of ENCODE-rE2G, an improved algorithm for predicting enhancer to gene activity with supervised machine learning (Gschwind et al., 2023). However, despite these innovations, current computational approaches are not perfect and are limited by available datasets. As such, the prediction of enhancer targets remains an open problem.
Advances in genome engineering and genomics have catalyzed the development of new approaches to evaluate the functions of non-coding regulatory elements. CRISPR activation (CRISPRa) and repression (CRISPRi) has been frequently employed as a robust tool to modulate the activity of non-coding regulatory elements. One key readout is how these regulatory perturbations impact the expression of target genes and the activity of pathways, by profiling the expression of specific genes, gene subsets, or whole transcriptomes. Perturb-seq combines CRISPRa/i and single cell RNA-seq to measure the impact of non-coding regulatory element perturbation at high throughput (Adamson et al., 2016; Xie et al., 2017; Xie et al., 2019; Gasperini et al., 2019). Perturb-seq has also been applied in cancer cells to facilitate the construction of gene regulatory networks (Dietlein et al., 2022; Ursu et al., 2022; Wang Y. et al., 2023). By measuring whole transcriptomes, Perturb-Seq also enables the exploration of secondary effects from enhancer perturbation. These indirect linkages between disease associated enhancers and disease genes may explain how genetic variants that are far from disease-causing genes can influence complex diseases (Boyle et al., 2017; Wang Y. et al., 2023). For example, we have shown that variants associated enhancers regulate the cell cycle pathway globally in breast cancer (Wang Y. et al., 2023). Another study demonstrates that enhancers within breast cancer risk loci regulate cell proliferation (Tuano et al., 2023).
While Perturb-Seq is a powerful tool, one disadvantage is its high cost. To address this issue, Targeted Perturb-seq (TAP-seq) has been developed to specifically enrich and sequence a subset of genes in Perturb-seq experiments (Schraivogel et al., 2020). This approach reduces cost and increases the sensitivity to detect lowly expressed genes. However, one disadvantage is that loss of transciptome-wide readout precludes unbiased analyses, which could be addressed by expanding the pool of enriched transcripts (Estilo et al., 2009). An even more specific approach is CRISPRi-FlowFish, which perturbs regulatory elements and uses FACS to sensitively measure changes in gene expression (Arrigucci et al., 2017; Fulco et al., 2019). One study identified five non-coding regulatory elements of XBP1 in breast cancer cells, which are the hotspots of breast cancer mutations (Dietlein et al., 2022). The sensitivity of FlowFish is high, which enables the analysis of lowly expressed genes. In addition, this method reduces the cost of sequencing. However, disadvantages include being limited to analyzing a handful of genes at a time and the need to optimize and validate the FISH probes used to detect transcript expression.
Recent advances have enabled a new suite of tools to examine non-coding functions at nucleotide resolution. Computationally, eQTL analysis can infer the impact of variants on genes, and has been widely used in the cancer setting (Li et al., 2013; Nica and Dermitzakis, 2013; Gong et al., 2018). By correlating variant status and nearby gene expression levels across a large cohort of patients, eQTL analysis assigns non-coding variants to the genes likely being misregulated. For example, ESR1, MYC and KLF4 have been linked to three different risk loci in breast cancer with eQTL analysis (Li et al., 2013). Experimentally, traditional reporter assays and higher throughput MPRAs have been widely used to test the impact of non-coding variants on the expression of a reporter gene in vitro (Pomerantz et al., 2009; Long et al., 2010; Dietlein et al., 2022). Finally, variant editing methods such as prime editing/base editing combined with target gene measurement provide a means to precisely quantify the effects of genetic variants in an endogenous genomic context (Canver et al., 2015; Dixit et al., 2016; Martyn et al., 2023). These methods directly edit the genome and measure gene expression, offering a more accurate reflection of variant function. By directly knocking-in disease variants, these experiments can give more relevant insights on the impact of variants compared to approaches like CRISPRa or CRISPRi. For example, correcting TERT promoter mutation using base editing can inhibit the cancer phenotype in vivo (Li et al., 2020). In parallel, new developments in saturation genome editing are enabling the functional characterization of all possible single-nucleotide genetic variants of cancer genes such as BRCA1 (Findlay et al., 2018).
Assessing the impact of variants/non-coding regulatory elements on cellular phenotypes
Cancer cells exhibit multiple hallmarks, and studies have used these features as cellular readouts to quantify the impact of variants (Hanahan and Weinberg, 2000; Hanahan and Weinberg, 2011; Hanahan, 2022). One study has shown that cellular morphology correlates with cancer hallmarks such as metastasis, and morphology can be a predictive marker of cancer cell state (Alizadeh et al., 2020; Wu et al., 2020). There are several methods to measure the morphological phenotype such as Cell Painting and COSMOS (Bray et al., 2016; Salek et al., 2022). Both methods can evaluate cellular morphology in a high-throughput and high-content way. For example, high throughput Cell Painting experiments are able to capture variant phenotypes in lung cancer, and are highly correlated with transcriptional phenotypes (Caicedo et al., 2022). Integrating perturbation and morphological measurement could be a powerful tool to understand the variant impact on cellular level (Way et al., 2021; Haghighi et al., 2022).
An alternative method for assessing cellular phenotype involves a focus on biological phenomena, particularly cancer-associated processes such as proliferation, migration, and apoptosis. For example, traditional (bulk) CRISPR screens (Tsherniak et al., 2017). Identify genetic perturbations that either increase or decrease the proliferation of cancer cells. DepMap contains whole genome CRISPR screens across a wide panel of diverse cancer cell lines (Tsherniak et al., 2017). Extending this approach to individual variants, PRIME uses prime editing to install variants into cancer cells and then identify the variants that accelerate proliferation in a cancer context (Ren et al., 2023).
Perspectives
The challenges and future prospects of functionally characterizing enhancer variants in cancer
Mapping the variant-gene-pathway-disease network is an active area of current research. But several key challenges remain.
• First: effect size. Numerous studies confirm the limited impact of one single cancer-associated variant (Park et al., 2010; Freedman et al., 2011). The vast majority of variants exhibit low penetrance, contributing to the “missing heritability” problem. This phenomenon extends beyond cancer to complex traits (Purcell et al., 2009; Yang et al., 2010).
• Second: population diversity. Large-scale efforts like whole genome sequencing (WGS) and genome-wide association studies (GWAS) to profile genetic variants have been biased toward certain ancestral populations. Increasing cohort diversity will expand the catalog of variants linked with disease.
• Third: indirect regulation. Many studies have shown that non-coding regulatory regions can regulate genes indirectly (Bauer et al., 2013; Wang Y. et al., 2023). Variant-associated regulatory regions may regulate cancer genes indirectly, through non-obvious mechanisms. New studies using Perturb-Seq and related approaches are needed to comprehensively map these regulatory interactions.
• Fourth: the synergistic variant effects. Multiple non-coding variants can synergistically act on the same gene, complicating variant functional studies. Studies have shown that multiple enhancers can synergistically co-regulate the same target gene (Corradin et al., 2014). Since a single enhancer usually does not fully control a target gene’s expression, it is likely that multiple variants across multiple enhancers are required to alter expression, with each individual variant contributing a mild effect. These attributes add to the difficulty of functionally mapping variant effects. Addressing this challenge requires simultaneous characterization of multiple variants, which is extremely difficult due to the combinatorial complexity of this analysis.
• Fifth: cell type specificity. A tumor is a heterogeneity entity. Variants in non-cancerous cells like fibroblasts and immune cells can also impact therapeutic outcomes by altering the tumor microenvironment (Dhainaut et al., 2022). Thus, it is also important to examine variant functions across cellular contexts. Recognizing the intrinsic heterogeneity of tumors, understanding cell-cell interactions within the tumor microenvironment becomes pivotal. Importantly, current studies have mainly studied variant functions in cancer cell lines and mouse models. Future efforts will need to leverage co-culture or 3D cancer organoid models consisting of multiple cell types, which enhances the likelihood of identifying hits in crucial cancer pathways such as angiogenesis, migration, and immune response (Yuan et al., 2022; Polak et al., 2024).
The omnigenic model posits that a disease or a trait is controlled by a small number of ‘core genes’, and many ‘peripheral genes’ (Boyle et al., 2017; Wray et al., 2018). Core genes directly lead to disease progression, such as tumor suppressors and oncogenes in cancer. Peripheral genes influence core genes, and can include genes like transcriptional regulators. Viewed in this way, variants integrate into the omnigenic model by directly or indirectly influencing core genes or peripheral genes. In this way, the omnigenic model can be readily extended to cancer development. This complex variant-gene regulatory network could possibly explain the small effect size of most cancer variants.
Concluding remarks
Interpreting non-coding variants remains a significant problem in cancer genetics. Powerful new technologies will facilitate the systematic functional characterization of non-coding variants. However, increases in experimental throughput alone will not be sufficient to understand the function of all cancer variants across all cell states. New computational approaches that learn from these datasets to derive accurate predictions will also be an integral component to a comprehensive understanding of how non-coding variants contribute to cancer (but are outside the scope of this review) (Ostroverkhova et al., 2023; Wang Z. et al., 2023).
Author contributions
YW: Writing–original draft, Writing–review and editing. GH: Funding acquisition, Writing–original draft, Writing–review and editing.
Funding
The author(s) declare that financial support was received for the research, authorship, and/or publication of this article. GH is supported by CPRIT (RP190451), NIH (DP2GM128203, UM1HG011996), the Welch Foundation (I-2103-20220331), and the Green Center for Reproductive Biology.
Acknowledgments
The authors acknowledge the support from CPRIT (RP190451), NIH (DP2GM128203, UM1HG011996), the Burroughs Wellcome Fund (1019804), the Welch Foundation (I-2103-20220331), and the Green Center for Reproductive Biology.
Conflict of interest
The authors declare that the research was conducted in the absence of any commercial or financial relationships that could be construed as a potential conflict of interest.
Publisher’s note
All claims expressed in this article are solely those of the authors and do not necessarily represent those of their affiliated organizations, or those of the publisher, the editors and the reviewers. Any product that may be evaluated in this article, or claim that may be made by its manufacturer, is not guaranteed or endorsed by the publisher.
References
Adamson, B., Norman, T. M., Jost, M., Cho, M. Y., Nuñez, J. K., Chen, Y., et al. (2016). A multiplexed single-cell CRISPR screening platform enables systematic dissection of the unfolded protein response. Cell 167, 1867–1882. doi:10.1016/j.cell.2016.11.048
Ajore, R., Niroula, A., Pertesi, M., Cafaro, C., Thodberg, M., Went, M., et al. (2022). Functional dissection of inherited non-coding variation influencing multiple myeloma risk. Nat. Commun. 13, 151. doi:10.1038/s41467-021-27666-x
Alizadeh, E., Castle, J., Quirk, A., Taylor, C. D. L., Xu, W., and Prasad, A. (2020). Cellular morphological features are predictive markers of cancer cell state. Comput. Biol. Med. 126, 104044. doi:10.1016/j.compbiomed.2020.104044
All of Us Research Program Genomics Investigators (2024). Genomic data in the all of us research program. Nature 627, 340–346. doi:10.1038/s41586-023-06957-x
Arnold, C. D., Gerlach, D., Stelzer, C., Boryń, Ł. M., Rath, M., and Stark, A. (2013). Genome-wide quantitative enhancer activity maps identified by STARR-seq. Science 339, 1074–1077. doi:10.1126/science.1232542
Arrigucci, R., Bushkin, Y., Radford, F., Lakehal, K., Vir, P., Pine, R., et al. (2017). FISH-Flow, a protocol for the concurrent detection of mRNA and protein in single cells using fluorescence in situ hybridization and flow cytometry. Nat. Protoc. 12, 1245–1260. doi:10.1038/nprot.2017.039
Bailey, M. H., Tokheim, C., Porta-Pardo, E., Sengupta, S., Bertrand, D., Weerasinghe, A., et al. (2018). Comprehensive characterization of cancer driver genes and mutations. Cell 173, 371–385.e18. doi:10.1016/j.cell.2018.02.060
Bauer, D. E., Kamran, S. C., Lessard, S., Xu, J., Fujiwara, Y., Lin, C., et al. (2013). An erythroid enhancer of BCL11A subject to genetic variation determines fetal hemoglobin level. Science 342, 253–257. doi:10.1126/science.1242088
Bauman, J. A., Li, S.-D., Yang, A., Huang, L., and Kole, R. (2010). Anti-tumor activity of splice-switching oligonucleotides. Nucleic Acids Res. 38, 8348–8356. doi:10.1093/nar/gkq731
Boise, L. H., González-García, M., Postema, C. E., Ding, L., Lindsten, T., Turka, L. A., et al. (1993). bcl-x, a bcl-2-related gene that functions as a dominant regulator of apoptotic cell death. Cell 74, 597–608. doi:10.1016/0092-8674(93)90508-n
Bond, G. L., Hu, W., Bond, E. E., Robins, H., Lutzker, S. G., Arva, N. C., et al. (2004). A single nucleotide polymorphism in the MDM2 promoter attenuates the p53 tumor suppressor pathway and accelerates tumor formation in humans. Cell 119, 591–602. doi:10.1016/j.cell.2004.11.022
Borah, S., Xi, L., Zaug, A. J., Powell, N. M., Dancik, G. M., Cohen, S. B., et al. (2015). Cancer. TERT promoter mutations and telomerase reactivation in urothelial cancer. Science 347, 1006–1010. doi:10.1126/science.1260200
Boyle, E. A., Li, Y. I., and Pritchard, J. K. (2017). An expanded view of complex traits: from polygenic to omnigenic. Cell 169, 1177–1186. doi:10.1016/j.cell.2017.05.038
Bray, M.-A., Singh, S., Han, H., Davis, C. T., Borgeson, B., Hartland, C., et al. (2016). Cell Painting, a high-content image-based assay for morphological profiling using multiplexed fluorescent dyes. Nat. Protoc. 11, 1757–1774. doi:10.1038/nprot.2016.105
Caicedo, J. C., Arevalo, J., Piccioni, F., Bray, M.-A., Hartland, C. L., Wu, X., et al. (2022). Cell Painting predicts impact of lung cancer variants. Mol. Biol. Cell 33, ar49. doi:10.1091/mbc.E21-11-0538
Canon, J., Rex, K., Saiki, A. Y., Mohr, C., Cooke, K., Bagal, D., et al. (2019). The clinical KRAS(G12C) inhibitor AMG 510 drives anti-tumour immunity. Nature 575, 217–223. doi:10.1038/s41586-019-1694-1
Canver, M. C., Smith, E. C., Sher, F., Pinello, L., Sanjana, N. E., Shalem, O., et al. (2015). BCL11A enhancer dissection by Cas9-mediated in situ saturating mutagenesis. Nature 527, 192–197. doi:10.1038/nature15521
Cerami, E., Gao, J., Dogrusoz, U., Gross, B. E., Sumer, S. O., Aksoy, B. A., et al. (2012). The cBio cancer genomics portal: an open platform for exploring multidimensional cancer genomics data. Cancer Discov. 2, 401–404. doi:10.1158/2159-8290.CD-12-0095
Chen, Y.-N., Ren, C.-C., Yang, L., Nai, M.-M., Xu, Y.-M., Zhang, F., et al. (2019). MicroRNA let-7d-5p rescues ovarian cancer cell apoptosis and restores chemosensitivity by regulating the p53 signaling pathway via HMGA1. Int. J. Oncol. 54, 1771–1784. doi:10.3892/ijo.2019.4731
Choi, J., Zhang, T., Vu, A., Ablain, J., Makowski, M. M., Colli, L. M., et al. (2020). Massively parallel reporter assays of melanoma risk variants identify MX2 as a gene promoting melanoma. Nat. Commun. 11, 2718. doi:10.1038/s41467-020-16590-1
Corces, M. R., Granja, J. M., Shams, S., Louie, B. H., Seoane, J. A., Zhou, W., et al. (2018). The chromatin accessibility landscape of primary human cancers. Science 362, eaav1898. doi:10.1126/science.aav1898
Corradin, O., Saiakhova, A., Akhtar-Zaidi, B., Myeroff, L., Willis, J., Cowper-Sal lari, R., et al. (2014). Combinatorial effects of multiple enhancer variants in linkage disequilibrium dictate levels of gene expression to confer susceptibility to common traits. Genome Res. 24, 1–13. doi:10.1101/gr.164079.113
Corradin, O., and Scacheri, P. C. (2014). Enhancer variants: evaluating functions in common disease. Genome Med. 6, 85. doi:10.1186/s13073-014-0085-3
Creyghton, M. P., Cheng, A. W., Welstead, G. G., Kooistra, T., Carey, B. W., Steine, E. J., et al. (2010). Histone H3K27ac separates active from poised enhancers and predicts developmental state. Proc. Natl. Acad. Sci. U. S. A. 107, 21931–21936. doi:10.1073/pnas.1016071107
Dentro, S. C., Leshchiner, I., Haase, K., Tarabichi, M., Wintersinger, J., Deshwar, A. G., et al. (2021). Characterizing genetic intra-tumor heterogeneity across 2,658 human cancer genomes. Cell 184, 2239–2254.e39. doi:10.1016/j.cell.2021.03.009
Dhainaut, M., Rose, S. A., Akturk, G., Wroblewska, A., Nielsen, S. R., Park, E. S., et al. (2022). Spatial CRISPR genomics identifies regulators of the tumor microenvironment. Cell 185, 1223–1239.e20. doi:10.1016/j.cell.2022.02.015
Dietlein, F., Wang, A. B., Fagre, C., Tang, A., Besselink, N. J. M., Cuppen, E., et al. (2022). Genome-wide analysis of somatic noncoding mutation patterns in cancer. Science 376, eabg5601. doi:10.1126/science.abg5601
Ding, L., Bailey, M. H., Porta-Pardo, E., Thorsson, V., Colaprico, A., Bertrand, D., et al. (2018). Perspective on oncogenic processes at the end of the beginning of cancer genomics. Cell 173, 305–320.e10. doi:10.1016/j.cell.2018.03.033
Dixit, A., Parnas, O., Li, B., Chen, J., Fulco, C. P., Jerby-Arnon, L., et al. (2016). Perturb-seq: dissecting molecular circuits with scalable single-cell RNA profiling of pooled genetic screens. Cell 167, 1853–1866. doi:10.1016/j.cell.2016.11.038
Estilo, C. L., O-charoenrat, P., Talbot, S., Socci, N. D., Carlson, D. L., Ghossein, R., et al. (2009). Oral tongue cancer gene expression profiling: identification of novel potential prognosticators by oligonucleotide microarray analysis. BMC Cancer 9, 11. doi:10.1186/1471-2407-9-11
Findlay, G. M., Daza, R. M., Martin, B., Zhang, M. D., Leith, A. P., Gasperini, M., et al. (2018). Accurate classification of BRCA1 variants with saturation genome editing. Nature 562, 217–222. doi:10.1038/s41586-018-0461-z
Freedman, M. L., Monteiro, A. N. A., Gayther, S. A., Coetzee, G. A., Risch, A., Plass, C., et al. (2011). Principles for the post-GWAS functional characterization of cancer risk loci. Nat. Genet. 43, 513–518. doi:10.1038/ng.840
Fulco, C. P., Nasser, J., Jones, T. R., Munson, G., Bergman, D. T., Subramanian, V., et al. (2019). Activity-by-contact model of enhancer-promoter regulation from thousands of CRISPR perturbations. Nat. Genet. 51, 1664–1669. doi:10.1038/s41588-019-0538-0
Gao, J., Aksoy, B. A., Dogrusoz, U., Dresdner, G., Gross, B., Sumer, S. O., et al. (2013). Integrative analysis of complex cancer genomics and clinical profiles using the cBioPortal. Sci. Signal. 6, pl1. doi:10.1126/scisignal.2004088
Gasperini, M., Hill, A. J., McFaline-Figueroa, J. L., Martin, B., Kim, S., Zhang, M. D., et al. (2019). A genome-wide framework for mapping gene regulation via cellular genetic screens. Cell 176, 1516. doi:10.1016/j.cell.2019.02.027
Gong, J., Mei, S., Liu, C., Xiang, Y., Ye, Y., Zhang, Z., et al. (2018). PancanQTL: systematic identification of cis-eQTLs and trans-eQTLs in 33 cancer types. Nucleic Acids Res. 46, D971–D976. doi:10.1093/nar/gkx861
Gschwind, A. R., Mualim, K. S., Karbalayghareh, A., Sheth, M. U., Dey, K. K., Jagoda, E., et al. (2023). An encyclopedia of enhancer-gene regulatory interactions in the human genome. bioRxiv. doi:10.1101/2023.11.09.563812
Haghighi, M., Caicedo, J. C., Cimini, B. A., Carpenter, A. E., and Singh, S. (2022). High-dimensional gene expression and morphology profiles of cells across 28,000 genetic and chemical perturbations. Nat. Methods 19, 1550–1557. doi:10.1038/s41592-022-01667-0
Hanahan, D. (2022). Hallmarks of cancer: new dimensions. Cancer Discov. 12, 31–46. doi:10.1158/2159-8290.CD-21-1059
Hanahan, D., and Weinberg, R. A. (2000). The hallmarks of cancer. Cell 100, 57–70. doi:10.1016/s0092-8674(00)81683-9
Hanahan, D., and Weinberg, R. A. (2011). Hallmarks of cancer: the next generation. Cell 144, 646–674. doi:10.1016/j.cell.2011.02.013
Hansen, T. J., and Hodges, E. (2022). ATAC-STARR-seq reveals transcription factor-bound activators and silencers within chromatin-accessible regions of the human genome. Genome Res. 32, 1529–1541. doi:10.1101/gr.276766.122
Heintzman, N. D., Hon, G. C., Hawkins, R. D., Kheradpour, P., Stark, A., Harp, L. F., et al. (2009). Histone modifications at human enhancers reflect global cell-type-specific gene expression. Nature 459, 108–112. doi:10.1038/nature07829
Horn, S., Figl, A., Rachakonda, P. S., Fischer, C., Sucker, A., Gast, A., et al. (2013). TERT promoter mutations in familial and sporadic melanoma. Science 339, 959–961. doi:10.1126/science.1230062
Huang, H., Hu, J., Maryam, A., Huang, Q., Zhang, Y., Ramakrishnan, S., et al. (2021). Defining super-enhancer landscape in triple-negative breast cancer by multiomic profiling. Nat. Commun. 12, 2242. doi:10.1038/s41467-021-22445-0
Huang, K.-L., Mashl, R. J., Wu, Y., Ritter, D. I., Wang, J., Oh, C., et al. (2018). Pathogenic germline variants in 10,389 adult cancers. Cell 173, 355–370.e14. doi:10.1016/j.cell.2018.03.039
Hudson, T. J., Anderson, W., Artez, A., Barker, A. D., Bell, C., Bernabe, R. R., et al. (2010). International network of cancer genome projects. Nature 464, 993–998. doi:10.1038/nature08987
ICGC/TCGA Pan-Cancer Analysis of Whole Genomes Consortium (2020). Pan-cancer analysis of whole genomes. Nature 578, 82–93. doi:10.1038/s41586-020-1969-6
Janes, M. R., Zhang, J., Li, L.-S., Hansen, R., Peters, U., Guo, X., et al. (2018). Targeting KRAS mutant cancers with a covalent G12C-specific inhibitor. Cell 172, 578–589. doi:10.1016/j.cell.2018.01.006
Jayasinghe, R. G., Cao, S., Gao, Q., Wendl, M. C., Vo, N. S., Reynolds, S. M., et al. (2018). Systematic analysis of splice-site-creating mutations in cancer. Cell Rep. 23, 270–281.e3. doi:10.1016/j.celrep.2018.03.052
Jiang, J., Liu, H.-L., Tao, L., Lin, X.-Y., Yang, Y.-D., Tan, S.-W., et al. (2018). Let-7d inhibits colorectal cancer cell proliferation through the CST1/p65 pathway. Int. J. Oncol. 53, 781–790. doi:10.3892/ijo.2018.4419
Jung, H., Lee, D., Lee, J., Park, D., Kim, Y. J., Park, W.-Y., et al. (2015). Intron retention is a widespread mechanism of tumor-suppressor inactivation. Nat. Genet. 47, 1242–1248. doi:10.1038/ng.3414
Karttunen, K., Patel, D., Xia, J., Fei, L., Palin, K., Aaltonen, L., et al. (2023). Transposable elements as tissue-specific enhancers in cancers of endodermal lineage. Nat. Commun. 14, 5313. doi:10.1038/s41467-023-41081-4
Kircher, M., Xiong, C., Martin, B., Schubach, M., Inoue, F., Bell, R. J. A., et al. (2019). Saturation mutagenesis of twenty disease-associated regulatory elements at single base-pair resolution. Nat. Commun. 10, 3583. doi:10.1038/s41467-019-11526-w
Landa, I., Ganly, I., Chan, T. A., Mitsutake, N., Matsuse, M., Ibrahimpasic, T., et al. (2013). Frequent somatic TERT promoter mutations in thyroid cancer: higher prevalence in advanced forms of the disease. J. Clin. Endocrinol. Metab. 98, E1562–E1566. doi:10.1210/jc.2013-2383
Leeman-Neill, R. J., Song, D., Bizarro, J., Wacheul, L., Rothschild, G., Singh, S., et al. (2023). Noncoding mutations cause super-enhancer retargeting resulting in protein synthesis dysregulation during B cell lymphoma progression. Nat. Genet. 55, 2160–2174. doi:10.1038/s41588-023-01561-1
Li, Q., Seo, J.-H., Stranger, B., McKenna, A., Pe’er, I., Laframboise, T., et al. (2013). Integrative eQTL-based analyses reveal the biology of breast cancer risk loci. Cell 152, 633–641. doi:10.1016/j.cell.2012.12.034
Li, X., Qian, X., Wang, B., Xia, Y., Zheng, Y., Du, L., et al. (2020). Programmable base editing of mutated TERT promoter inhibits brain tumour growth. Nat. Cell Biol. 22, 282–288. doi:10.1038/s41556-020-0471-6
Lieberman-Aiden, E., van Berkum, N. L., Williams, L., Imakaev, M., Ragoczy, T., Telling, A., et al. (2009). Comprehensive mapping of long-range interactions reveals folding principles of the human genome. Science 326, 289–293. doi:10.1126/science.1181369
Lito, P., Solomon, M., Li, L.-S., Hansen, R., and Rosen, N. (2016). Allele-specific inhibitors inactivate mutant KRAS G12C by a trapping mechanism. Science 351, 604–608. doi:10.1126/science.aad6204
Liu, E. M., Martinez-Fundichely, A., Bollapragada, R., Spiewack, M., and Khurana, E. (2021). CNCDatabase: a database of non-coding cancer drivers. Nucleic Acids Res. 49, D1094–D1101. doi:10.1093/nar/gkaa915
Liu, S., Liu, Y., Zhang, Q., Wu, J., Liang, J., Yu, S., et al. (2017). Systematic identification of regulatory variants associated with cancer risk. Genome Biol. 18, 194. doi:10.1186/s13059-017-1322-z
Long, E., Yin, J., Funderburk, K. M., Xu, M., Feng, J., Kane, A., et al. (2022). Massively parallel reporter assays and variant scoring identified functional variants and target genes for melanoma loci and highlighted cell-type specificity. Am. J. Hum. Genet. 109, 2210–2229. doi:10.1016/j.ajhg.2022.11.006
Long, J., Cai, Q., Shu, X.-O., Qu, S., Li, C., Zheng, Y., et al. (2010). Identification of a functional genetic variant at 16q12.1 for breast cancer risk: results from the Asia Breast Cancer Consortium. PLoS Genet. 6, e1001002. doi:10.1371/journal.pgen.1001002
Lujambio, A., and Lowe, S. W. (2012). The microcosmos of cancer. Nature 482, 347–355. doi:10.1038/nature10888
Mansour, M. R., Abraham, B. J., Anders, L., Berezovskaya, A., Gutierrez, A., Durbin, A. D., et al. (2014). Oncogene regulation. An oncogenic super-enhancer formed through somatic mutation of a noncoding intergenic element. Science 346, 1373–1377. doi:10.1126/science.1259037
Martyn, G. E., Montgomery, M. T., Jones, H., Guo, K., Doughty, B. R., Linder, J., et al. (2023). Rewriting regulatory DNA to dissect and reprogram gene expression. bioRxiv. doi:10.1101/2023.12.20.572268
Michailidou, K., Beesley, J., Lindstrom, S., Canisius, S., Dennis, J., Lush, M. J., et al. (2015). Genome-wide association analysis of more than 120,000 individuals identifies 15 new susceptibility loci for breast cancer. Nat. Genet. 47, 373–380. doi:10.1038/ng.3242
Michailidou, K., Hall, P., Gonzalez-Neira, A., Ghoussaini, M., Dennis, J., Milne, R. L., et al. (2013). Large-scale genotyping identifies 41 new loci associated with breast cancer risk. Nat. Genet. 45, 353–361e3612. doi:10.1038/ng.2563
Michailidou, K., Lindström, S., Dennis, J., Beesley, J., Hui, S., Kar, S., et al. (2017). Association analysis identifies 65 new breast cancer risk loci. Nature 551, 92–94. doi:10.1038/nature24284
Milne, R. L., Kuchenbaecker, K. B., Michailidou, K., Beesley, J., Kar, S., Lindström, S., et al. (2017). Identification of ten variants associated with risk of estrogen-receptor-negative breast cancer. Nat. Genet. 49, 1767–1778. doi:10.1038/ng.3785
Morton, A. R., Dogan-Artun, N., Faber, Z. J., MacLeod, G., Bartels, C. F., Piazza, M. S., et al. (2019). Functional enhancers shape extrachromosomal oncogene amplifications. Cell 179, 1330–1341. doi:10.1016/j.cell.2019.10.039
Nica, A. C., and Dermitzakis, E. T. (2013). Expression quantitative trait loci: present and future. Philos. Trans. R. Soc. Lond. B Biol. Sci. 368, 20120362. doi:10.1098/rstb.2012.0362
Ostrem, J. M., Peters, U., Sos, M. L., Wells, J. A., and Shokat, K. M. (2013). K-Ras(G12C) inhibitors allosterically control GTP affinity and effector interactions. Nature 503, 548–551. doi:10.1038/nature12796
Ostroverkhova, D., Przytycka, T. M., and Panchenko, A. R. (2023). Cancer driver mutations: predictions and reality. Trends Mol. Med. 29, 554–566. doi:10.1016/j.molmed.2023.03.007
Park, J.-H., Wacholder, S., Gail, M. H., Peters, U., Jacobs, K. B., Chanock, S. J., et al. (2010). Estimation of effect size distribution from genome-wide association studies and implications for future discoveries. Nat. Genet. 42, 570–575. doi:10.1038/ng.610
Perera, D., Poulos, R. C., Shah, A., Beck, D., Pimanda, J. E., and Wong, J. W. H. (2016). Differential DNA repair underlies mutation hotspots at active promoters in cancer genomes. Nature 532, 259–263. doi:10.1038/nature17437
Polak, R., Zhang, E. T., and Kuo, C. J. (2024). Cancer organoids 2.0: modelling the complexity of the tumour immune microenvironment. Nat. Rev. Cancer 24, 523–539. doi:10.1038/s41568-024-00706-6
Pomerantz, M. M., Ahmadiyeh, N., Jia, L., Herman, P., Verzi, M. P., Doddapaneni, H., et al. (2009). The 8q24 cancer risk variant rs6983267 shows long-range interaction with MYC in colorectal cancer. Nat. Genet. 41, 882–884. doi:10.1038/ng.403
Pradeepa, M. M., Grimes, G. R., Kumar, Y., Olley, G., Taylor, G. C. A., Schneider, R., et al. (2016). Histone H3 globular domain acetylation identifies a new class of enhancers. Nat. Genet. 48, 681–686. doi:10.1038/ng.3550
Purcell, S. M., Wray, N. R., Stone, J. L., Visscher, P. M., O’Donovan, M. C., Sullivan, P. F., et al. (2009). Common polygenic variation contributes to risk of schizophrenia and bipolar disorder. Nature 460, 748–752. doi:10.1038/nature08185
Rada-Iglesias, A., Bajpai, R., Swigut, T., Brugmann, S. A., Flynn, R. A., and Wysocka, J. (2011). A unique chromatin signature uncovers early developmental enhancers in humans. Nature 470, 279–283. doi:10.1038/nature09692
Ren, X., Yang, H., Nierenberg, J. L., Sun, Y., Chen, J., Beaman, C., et al. (2023). High-throughput PRIME-editing screens identify functional DNA variants in the human genome. Mol. Cell 83, 4633–4645.e9. doi:10.1016/j.molcel.2023.11.021
Rheinbay, E., Nielsen, M. M., Abascal, F., Wala, J. A., Shapira, O., Tiao, G., et al. (2020). Analyses of non-coding somatic drivers in 2,658 cancer whole genomes. Nature 578, 102–111. doi:10.1038/s41586-020-1965-x
Rhie, S. K., Perez, A. A., Lay, F. D., Schreiner, S., Shi, J., Polin, J., et al. (2019). A high-resolution 3D epigenomic map reveals insights into the creation of the prostate cancer transcriptome. Nat. Commun. 10, 4154. doi:10.1038/s41467-019-12079-8
Ronquillo, J. G., and Lester, W. T. (2022). Precision medicine landscape of genomic testing for patients with cancer in the National Institutes of Health All of Us database using informatics approaches. JCO Clin. Cancer Inf. 6, e2100152. doi:10.1200/CCI.21.00152
Ruan, E., Nemeth, E., Moffitt, R., Sandoval, L., Machiela, M. J., Freedman, N. D., et al. (2022). PLCOjs, a FAIR GWAS web SDK for the NCI prostate, lung, colorectal and ovarian cancer genetic Atlas project. Bioinformatics 38, 4434–4436. doi:10.1093/bioinformatics/btac531
Salek, M., Li, N., Chou, H.-P., Saini, K., Jovic, A., Jacobs, K. B., et al. (2022). Realtime morphological characterization and sorting of unlabeled viable cells using deep learning. bioRxiv. doi:10.1101/2022.02.28.482368
Schoenfelder, S., and Fraser, P. (2019). Long-range enhancer-promoter contacts in gene expression control. Nat. Rev. Genet. 20, 437–455. doi:10.1038/s41576-019-0128-0
Schraivogel, D., Gschwind, A. R., Milbank, J. H., Leonce, D. R., Jakob, P., Mathur, L., et al. (2020). Targeted Perturb-seq enables genome-scale genetic screens in single cells. Nat. Methods 17, 629–635. doi:10.1038/s41592-020-0837-5
Shigaki, D., Adato, O., Adhikari, A. N., Dong, S., Hawkins-Hooker, A., Inoue, F., et al. (2019). Integration of multiple epigenomic marks improves prediction of variant impact in saturation mutagenesis reporter assay. Hum. Mutat. 40, 1280–1291. doi:10.1002/humu.23797
Sollis, E., Mosaku, A., Abid, A., Buniello, A., Cerezo, M., Gil, L., et al. (2023). The NHGRI-EBI GWAS Catalog: knowledgebase and deposition resource. Nucleic Acids Res. 51, D977–D985. doi:10.1093/nar/gkac1010
Stern, J. L., Paucek, R. D., Huang, F. W., Ghandi, M., Nwumeh, R., Costello, J. C., et al. (2017). Allele-specific DNA methylation and its interplay with repressive histone marks at promoter-mutant TERT genes. Cell Rep. 21, 3700–3707. doi:10.1016/j.celrep.2017.12.001
Sveen, A., Kilpinen, S., Ruusulehto, A., Lothe, R. A., and Skotheim, R. I. (2016). Aberrant RNA splicing in cancer; expression changes and driver mutations of splicing factor genes. Oncogene 35, 2413–2427. doi:10.1038/onc.2015.318
Tate, J. G., Bamford, S., Jubb, H. C., Sondka, Z., Beare, D. M., Bindal, N., et al. (2019). COSMIC: the catalogue of somatic mutations in cancer. Nucleic Acids Res. 47, D941–D947. doi:10.1093/nar/gky1015
Tehranchi, A., Hie, B., Dacre, M., Kaplow, I., Pettie, K., Combs, P., et al. (2019). Fine-mapping cis-regulatory variants in diverse human populations. Elife 8, e39595. doi:10.7554/eLife.39595
Tsherniak, A., Vazquez, F., Montgomery, P. G., Weir, B. A., Kryukov, G., Cowley, G. S., et al. (2017). Defining a cancer dependency map. Cell 170, 564–576. doi:10.1016/j.cell.2017.06.010
Tuano, N. K., Beesley, J., Manning, M., Shi, W., Perlaza-Jimenez, L., Malaver-Ortega, L. F., et al. (2023). CRISPR screens identify gene targets at breast cancer risk loci. Genome Biol. 24, 59. doi:10.1186/s13059-023-02898-w
Turnbull, C., Ahmed, S., Morrison, J., Pernet, D., Renwick, A., Maranian, M., et al. (2010). Genome-wide association study identifies five new breast cancer susceptibility loci. Nat. Genet. 42, 504–507. doi:10.1038/ng.586
Urbanek-Trzeciak, M. O., Galka-Marciniak, P., Nawrocka, P. M., Kowal, E., Szwec, S., Giefing, M., et al. (2020). Pan-cancer analysis of somatic mutations in miRNA genes. EBioMedicine 61, 103051. doi:10.1016/j.ebiom.2020.103051
Ursu, O., Neal, J. T., Shea, E., Thakore, P. I., Jerby-Arnon, L., Nguyen, L., et al. (2022). Massively parallel phenotyping of coding variants in cancer with Perturb-seq. Nat. Biotechnol. 40, 896–905. doi:10.1038/s41587-021-01160-7
Vinagre, J., Almeida, A., Pópulo, H., Batista, R., Lyra, J., Pinto, V., et al. (2013). Frequency of TERT promoter mutations in human cancers. Nat. Commun. 4, 2185. doi:10.1038/ncomms3185
Visel, A., Blow, M. J., Li, Z., Zhang, T., Akiyama, J. A., Holt, A., et al. (2009). ChIP-seq accurately predicts tissue-specific activity of enhancers. Nature 457, 854–858. doi:10.1038/nature07730
Wang, D., Wu, X., Jiang, G., Yang, J., Yu, Z., Yang, Y., et al. (2022). Systematic analysis of the effects of genetic variants on chromatin accessibility to decipher functional variants in non-coding regions. Front. Oncol. 12, 1035855. doi:10.3389/fonc.2022.1035855
Wang, Y., Armendariz, D., Wang, L., Zhao, H., Xie, S., and Hon, G. C. (2023). Enhancer regulatory networks globally connect non-coding breast cancer loci to cancer genes. bioRxiv. doi:10.1101/2023.11.20.567880
Wang, Z., Zhao, G., Li, B., Fang, Z., Chen, Q., Wang, X., et al. (2023). Performance comparison of computational methods for the prediction of the function and pathogenicity of non-coding variants. Genomics Proteomics Bioinforma. 21, 649–661. doi:10.1016/j.gpb.2022.02.002
Way, G. P., Kost-Alimova, M., Shibue, T., Harrington, W. F., Gill, S., Piccioni, F., et al. (2021). Predicting cell health phenotypes using image-based morphology profiling. Mol. Biol. Cell 32, 995–1005. doi:10.1091/mbc.E20-12-0784
Wei, Y., Liu, G., Wu, B., Yuan, Y., and Pan, Y. (2018). Let-7d inhibits growth and metastasis in breast cancer by targeting jab1/cops5. Cell. Physiol. Biochem. 47, 2126–2135. doi:10.1159/000491523
Weinstein, J. N., Collisson, E. A., Mills, G. B., Mills, K. R., Shaw, K. R. M., Ozenberger, B. A., et al. (2013). The cancer genome Atlas pan-cancer analysis project. Nat. Genet. 45, 1113–1120. doi:10.1038/ng.2764
Wray, N. R., Wijmenga, C., Sullivan, P. F., Yang, J., and Visscher, P. M. (2018). Common disease is more complex than implied by the core gene omnigenic model. Cell 173, 1573–1580. doi:10.1016/j.cell.2018.05.051
Wu, P.-H., Gilkes, D. M., Phillip, J. M., Narkar, A., Cheng, T. W.-T., Marchand, J., et al. (2020). Single-cell morphology encodes metastatic potential. Sci. Adv. 6, eaaw6938. doi:10.1126/sciadv.aaw6938
Xie, S., Armendariz, D., Zhou, P., Duan, J., and Hon, G. C. (2019). Global analysis of enhancer targets reveals convergent enhancer-driven regulatory modules. Cell Rep. 29, 2570–2578. doi:10.1016/j.celrep.2019.10.073
Xie, S., Duan, J., Li, B., Zhou, P., and Hon, G. C. (2017). Multiplexed engineering and analysis of combinatorial enhancer activity in single cells. Mol. Cell 66, 285–299. doi:10.1016/j.molcel.2017.03.007
Xu, Z., Lee, D.-S., Chandran, S., Le, V. T., Bump, R., Yasis, J., et al. (2022). Structural variants drive context-dependent oncogene activation in cancer. Nature 612, 564–572. doi:10.1038/s41586-022-05504-4
Yang, J., Benyamin, B., McEvoy, B. P., Gordon, S., Henders, A. K., Nyholt, D. R., et al. (2010). Common SNPs explain a large proportion of the heritability for human height. Nat. Genet. 42, 565–569. doi:10.1038/ng.608
Ying, P., Chen, C., Lu, Z., Chen, S., Zhang, M., Cai, Y., et al. (2023). Genome-wide enhancer-gene regulatory maps link causal variants to target genes underlying human cancer risk. Nat. Commun. 14, 5958. doi:10.1038/s41467-023-41690-z
Yuan, J., Li, X., and Yu, S. (2022). Cancer organoid co-culture model system: novel approach to guide precision medicine. Front. Immunol. 13, 1061388. doi:10.3389/fimmu.2022.1061388
Zhang, H., Ahearn, T. U., Lecarpentier, J., Barnes, D., Beesley, J., Qi, G., et al. (2020). Genome-wide association study identifies 32 novel breast cancer susceptibility loci from overall and subtype-specific analyses. Nat. Genet. 52, 572–581. doi:10.1038/s41588-020-0609-2
Zhang, J., Bajari, R., Andric, D., Gerthoffert, F., Lepsa, A., Nahal-Bose, H., et al. (2019). The international cancer genome Consortium data portal. Nat. Biotechnol. 37, 367–369. doi:10.1038/s41587-019-0055-9
Zhang, J., Baran, J., Cros, A., Guberman, J. M., Haider, S., Hsu, J., et al. (2011). International cancer genome Consortium data portal--a one-stop shop for cancer genomics data. Database 2011, bar026. doi:10.1093/database/bar026
Keywords: functional genomics, enhancers and promoters, genetic variants, cancer, gene regulation, noncoding regulatory regions
Citation: Wang Y and Hon GC (2024) Towards functional maps of non-coding variants in cancer. Front. Genome Ed. 6:1481443. doi: 10.3389/fgeed.2024.1481443
Received: 15 August 2024; Accepted: 22 October 2024;
Published: 31 October 2024.
Edited by:
Xuebing Wu, Columbia University, United StatesReviewed by:
Hemangi Chaudhari, Stealth BioTherapeutics, United StatesPei-Chen Peng, Cedars Sinai Medical Center, United States
Copyright © 2024 Wang and Hon. This is an open-access article distributed under the terms of the Creative Commons Attribution License (CC BY). The use, distribution or reproduction in other forums is permitted, provided the original author(s) and the copyright owner(s) are credited and that the original publication in this journal is cited, in accordance with accepted academic practice. No use, distribution or reproduction is permitted which does not comply with these terms.
*Correspondence: Gary C. Hon, Z2FyeS5ob25AdXRzb3V0aHdlc3Rlcm4uZWR1