- 1Faculty of Information Engineering and Automation, Kunming University of Science and Technology, Kunming, China
- 2Brain Cognition and Brain-Computer Intelligence Integration Group, Kunming University of Science and Technology, Kunming, China
- 3Faculty of Science, Kunming University of Science and Technology, Kunming, China
- 4Institute of Quantitative and Technological Economics, Chinese Academy of Social Sciences, Beijing, China
- 5Institute of Biomedical Engineering, Chinese Academy of Medical Sciences and Peking Union Medical College, Tianjin, China
- 6School of Artificial Intelligence, South China Normal University, Foshan, China
Brain-computer interface (BCI) is a novel human-computer interaction technology, and its rapid development has led to a growing demand for skilled BCI professionals, culminating in the emergence of the BCI major. Despite its significance, there is limited literature addressing the curriculum design for this emerging major. This paper seeks to bridge this gap by proposing and discussing a curricular framework for the BCI major, based on the inherently multidisciplinary nature of BCI research and development. The paper begins by elucidating the primary factors behind the emergence of the BCI major, the increasing demand for both medical and non-medical applications of BCI, and the corresponding need for specialized talent. It then delves into the multidisciplinary nature of BCI research and offers principles for curriculum design to address this nature. Based on these principles, the paper provides detailed suggestions for structuring a BCI curriculum. Finally, it discusses the challenges confronting the development of the BCI major, including the lack of consensus and international collaboration in the construction of the BCI major, as well as the inadequacy or lack of teaching materials. Future work needs to improve the curriculum design of the BCI major from a competency-oriented perspective. It is expected that this paper will provide a reference for the curriculum design and construction of the BCI major.
1 Introduction
Brain-computer interface (BCI) is a revolutionizing human-computer interface (HCI) that bypasses or does not rely on peripheral nerves or muscles. Instead, it establishes a direct communication and control channel between the brain (or central nervous system, CNS) and computer using signals generated in the CNS (referred to as central nervous signals) (Allison et al., 2012; Graimann et al., 2010; Ramsey and Millán, 2020; Wolpaw and Wolpaw, 2012), as shown in Figure 1 (Chen et al., 2024; Luo et al., 2022). The goal of BCI is to improve or further enhance the quality of life or work efficiency of individuals, including patients, people with disabilities, and healthy individuals (Allison et al., 2012; Collura, 2014; Ramsey and Millán, 2020).
The rapid advances in computer technology and artificial intelligence, the progress in understanding the brain and acquiring brain signals, and the new understanding of the needs and capabilities of patients with neurological or psychiatric disorders and individuals with disabilities are major factors driving scientific interest and research activities in the BCI field (Wolpaw and Wolpaw, 2012). These factors have also driven the emergence of the BCI major. As BCI technologies develop rapidly and move toward industrial applications, the demand for trained BCI professionals has increased significantly, leading to an emerging BCI major, as illustrated in Figure 2 (Luo et al., 2022).
The BCI major is inherently multidisciplinary and requires extensive collaboration and integration across disciplines, unlike traditional or existing majors. Figure 2 also highlights the necessity for multidisciplinary cooperation in BCI research and applications. However, few publications have addressed the design of BCI curriculum based on its multidisciplinary nature. To fill this gap, this paper first discusses the emergence of the BCI major, followed by an explanation of the multidisciplinary nature of BCI research and development. The paper proposes principles for designing a BCI curriculum and suggests a proposed course structure based on this multidisciplinary nature. Finally, the paper discusses the challenges in the development of the BCI major and explores its prospects. It is anticipated that this paper will provide valuable suggestions for the BCI community regarding the curriculum design of the BCI major.
2 The emergence of the brain-computer interface major
2.1 The history of brain-computer interface research: an overview
BCI is a novel form of human-computer interaction, a new thing under the sun (Wolpaw and Wolpaw, 2012), and one of humanity’s dreams. Early research related to BCI is shown in Table 1.
In Table 1, the BCI system developed by Vidal aligns with the modern, narrow definition of BCI (Wolpaw and Wolpaw, 2012). Contemporary VEP-based BCI still fundamentally builds upon this idea (Chen et al., 2024). The possibility of using signals recorded from the brain for communication and control gained traction and scientific interest in the decades leading up to 2011 (Birbaumer et al., 1999; Chapin et al., 1999; Millan et al., 2004; Musallam et al., 2004; Nicolelis, 2001; Taylor et al., 2002). However, sustained research only began in the 25 years between 1986 and 2011, and a recognizable field of BCI research and development emerged in the 15 years between 1996 and 2011 (Wolpaw and Wolpaw, 2012). By 2011, this emerging field had already seen several hundred research groups established worldwide, with new groups continuing to emerge (Wolpaw and Wolpaw, 2012). The development of the BCI field has been extraordinary, with 2006–2011 marking a “golden era” of BCI research, during which many seminal BCI studies were published (Daly and Wolpaw, 2008; Hochberg et al., 2006; LaConte, 2011; Santhanam et al., 2006; Scott, 2006). Since 2012, there has been a significant surge in BCI-related publications in prestigious international journals (Aflalo et al., 2015; Coulter and Kemere, 2023; Flesher et al., 2021; Hochberg et al., 2012; Lai et al., 2023; Metzger et al., 2023; Minev et al., 2015; Ramsey and Crone, 2023; Willett et al., 2021; Willett et al., 2023). Notably, the field has seen accelerated progress since 2015, when the BCI community established the EU roadmap for BCI development. This momentum has been further strengthened by investments from major companies, such as Neuralink, which have contributed to the advancement of BCI research and development. As previously stated, while most BCI research has focused on non-invasive approaches, invasive BCI has also undergone significant advancements since early studies first demonstrated the feasibility of directly linking neural activity to external devices. Pioneering work by Lebedev and Nicolelis (2006) and Nicolelis (2003) laid the foundation for invasive BCIs by demonstrating their ability to control external devices through animal models and preliminary human trials. Currently, invasive BCI research is focused on continuous optimization, with an emphasis on improving signal quality, system stability, and long-term biocompatibility to support clinical applications such as precise control of prostheses, wheelchairs, and communication devices. Recent studies (Lorach et al., 2023; Metzger et al., 2023; Willett et al., 2021) have further enhanced system safety and performance by integrating advanced signal processing techniques, deep learning algorithms, and novel biomaterials, paving the way for high-resolution, closed-loop control systems. In the future, invasive BCIs are expected to enable functional restoration, neurorehabilitation, and cognitive enhancement, potentially driving revolutionary breakthroughs in neuroscience and rehabilitation medicine.
In summary, the field has evolved over more than 60 years since the pioneering BCI research of Walter et al. (1964). The accumulated research achievements indicate that humanity is steadily moving closer to realizing the dream of BCI. The emergence of the BCI major and its associated talent cultivation will further drive the realization of this vision.
2.2 Key factors in the emergence of the brain-computer interface major
The significant surge in scientific interest and research activities in the field of BCI can be attributed to the convergence of the following three factors (Wolpaw and Wolpaw, 2012), which are also the key factors for the emergence of the BCI major (Yang et al., 2024).
The first and most apparent factor is the availability of powerful and affordable computer hardware and software capable of supporting the complex and high-speed analysis of brain activity that is essential for real-time BCI operation (Wolpaw and Wolpaw, 2012).
The second factor lies in the advancements over the past 60 years in understanding CNS through studies on both animals and humans. These efforts have yielded a wealth of new information about the nature and functionality of brain signals, such as EEG activity and neuronal action potentials (Wolpaw and Wolpaw, 2012). Simultaneously, significant advances have been made in brain signal recording technologies. Revolutionary breakthroughs have been achieved in understanding the extraordinary adaptability and plasticity of the brain and CNS. Harnessing these adaptive properties has made it possible to create novel interactions between the brain and computer-based devices. These interactions can replace, restore, enhance, supplement, or improve the natural interactions between the brain and its internal and external environments, a profoundly exciting prospect (Wolpaw and Wolpaw, 2012).
The third factor is the growing recognition of the needs and capabilities of individuals with disabilities. These disabilities often result from conditions such as cerebral palsy, spinal cord injury, stroke, amyotrophic lateral sclerosis (ALS), multiple sclerosis, and muscular dystrophy. Technologies such as home ventilators and other life-support systems now allow the most severely disabled individuals to live for many years. However, some of these individuals experience a significantly reduced quality of life due to difficulties in communicating with the outside world. Providing even the most basic means of communication and control to individuals with minimal voluntary muscle control could enable them to lead enjoyable and productive lives, which is a central goal of BCI. Even in its current limited state of development, BCI can also address this critical need (Wolpaw and Wolpaw, 2012).
The societal demand for BCI serves as a fundamental driving force behind its development. The construction of the BCI major and the refinement of its curriculum may be urgently needed to meet the growing demand for skilled professionals in BCI research, development, and applications (Yang et al., 2024).
2.3 Medical and non-medical application demands for brain-computer interface
The medical and non-medical application demands of BCI are the fundamental driving forces behind the emergence of the BCI major (Yang et al., 2024).
2.3.1 Medical application demands for brain-computer interface
Patients with severe motor dysfunctions often lose the ability to communicate with the external world, directly reducing their quality of life and placing a heavy burden on their families and society. BCI can provide these patients with critical new opportunities for communication and control. As previously mentioned, this is the most important and primary goal of BCI (Flesher et al., 2021; Hochberg et al., 2012; Lai et al., 2023; Metzger et al., 2023; Ramsey and Crone, 2023; Willett et al., 2021; Willett et al., 2023). Other severely disabled patients also have potential needs for BCI technology, such as those with severe neurological and psychiatric disorders including spinal cord injury, stroke, Alzheimer’s disease, Parkinson’s disease, depression, and schizophrenia (Ramsey and Millán, 2020).
Numerous studies have demonstrated that BCI can replace, restore, enhance, supplement, or improve the natural output of the CNS for clinical patients and individuals with disabilities (Wolpaw and Wolpaw, 2012). For instance, BCI has been applied in stroke rehabilitation, the treatment of ALS, brain injury therapy, spinal cord injury rehabilitation, communication for locked-in syndrome patients, control of robots and prosthetics, neurorehabilitation training, enhancement of brain plasticity and attentional control, consciousness assessment and communication for patients with severe brain injury, and intelligent neuromodulation for movement disorders (Ramsey and Millán, 2020). In addition, research has explored the use of bidirectional BCI technology for spinal cord injury patients, the integration of BCI with virtual reality (VR) for neurorehabilitation, and the combination of BCI with functional electrical stimulation (FES) for motor recovery (Ramsey and Millán, 2020). Finally, as wearable neurotechnology, BCI holds promise for self-health monitoring to prevent diseases, representing a preventive medical application of BCI (Ramsey and Millán, 2020).
2.3.2 Non-medical application demands for brain-computer interface
In addition to medical applications, BCI also has non-medical application demands or objectives. With the advancement of BCI technology, its non-medical applications are also growing, including applications in virtual reality (VR) and augmented reality (AR) (Kohli et al., 2022), gaming and entertainment (Prapas et al., 2023; Vasiljevic et al., 2024), education and learning (Jamil et al., 2021), as well as occupational safety and productivity enhancement (Al-Hudhud et al., 2019; Powers et al., 2015).
2.4 Demand for brain-computer interface professionals
BCI research, development and applications require BCI professionals to realize the human dream of BCI and to meet the demand for BCI medical and non-medical applications. With the rapid development of BCI technology and the acceleration of its industrial transformation, the demand for BCI professionals has increased significantly. The key factors driving this demand include: (1) The BCI industry’s need for interdisciplinary and innovative talent. (2) The growing application demands and industrialization of BCI require skilled professionals. (3) The need for experts capable of analyzing and interpreting complex brain signals. (4) The potential of BCI in future intelligent systems, which further emphasizes the importance of professional expertise (Yang et al., 2024).
As previously mentioned, the rapid development of BCI technology and its translation into industrial contexts has led to the emergence of an emerging major: the BCI major. The establishment of this major, in conjunction with the refinement of its curriculum and the formulation of training programs, appears imperative to meet the growing demand for BCI professionals in research, development and applications (Yang et al., 2024). The BCI major is distinguished by its inherent interdisciplinary character, necessitating extensive collaboration and integration across multiple fields to nurture talent that can make innovative and original contributions.
3 Multidisciplinary nature of brain-computer interface research and development and curriculum design of the brain-computer interface major
3.1 Multidisciplinary nature of brain-computer interface research and development
Since 2000, BCI has achieved remarkable progress, largely due to its inherent and indispensable multidisciplinary nature, which also defines the uniqueness of BCI research and development (Wolpaw and Wolpaw, 2012). Figure 1 illustrates the operational sequence from the user’s brain to the BCI, clearly demonstrating this characteristic. Table 2 lists the main disciplines involved in BCI research and development (Wolpaw and Wolpaw, 2012; Yang et al., 2024).
Table 2 shows that the development of BCI has been quite different from previous or existing technologies. It requires the collaboration of multiple disciplines to create a successful BCI system. In particular, BCI research and development are directly linked to the brain that a highly complex system. This connection is the most distinctive and challenging aspect of BCI development. Neuroscience or Brain Science serves as the foundation for the scientific principles of BCI. Brain science includes areas such as central nervous system plasticity, psychological science, and cognitive neuroscience. It is particularly important to emphasize that the development of BCI is not merely an engineering challenge involving the implementation and optimization of BCI systems. Instead, it also relies on more fundamental and critical scientific principles, as shown in Figure 3A. Figure 3B illustrates the scientific principles underlying BCI systems. These principles involve identifying a specific set of psychological tasks, attentional tasks, and external stimuli that elicit highly separable brain signals for BCI applications. This is reflected in BCI paradigms and neural encoding (Tai et al., 2024). Additionally, the adaptability of the user’s brain, or central nervous system plasticity, plays a critical role in BCI systems. It serves as the scientific basis for modulating users’ neural activity and adapting BCI algorithms.
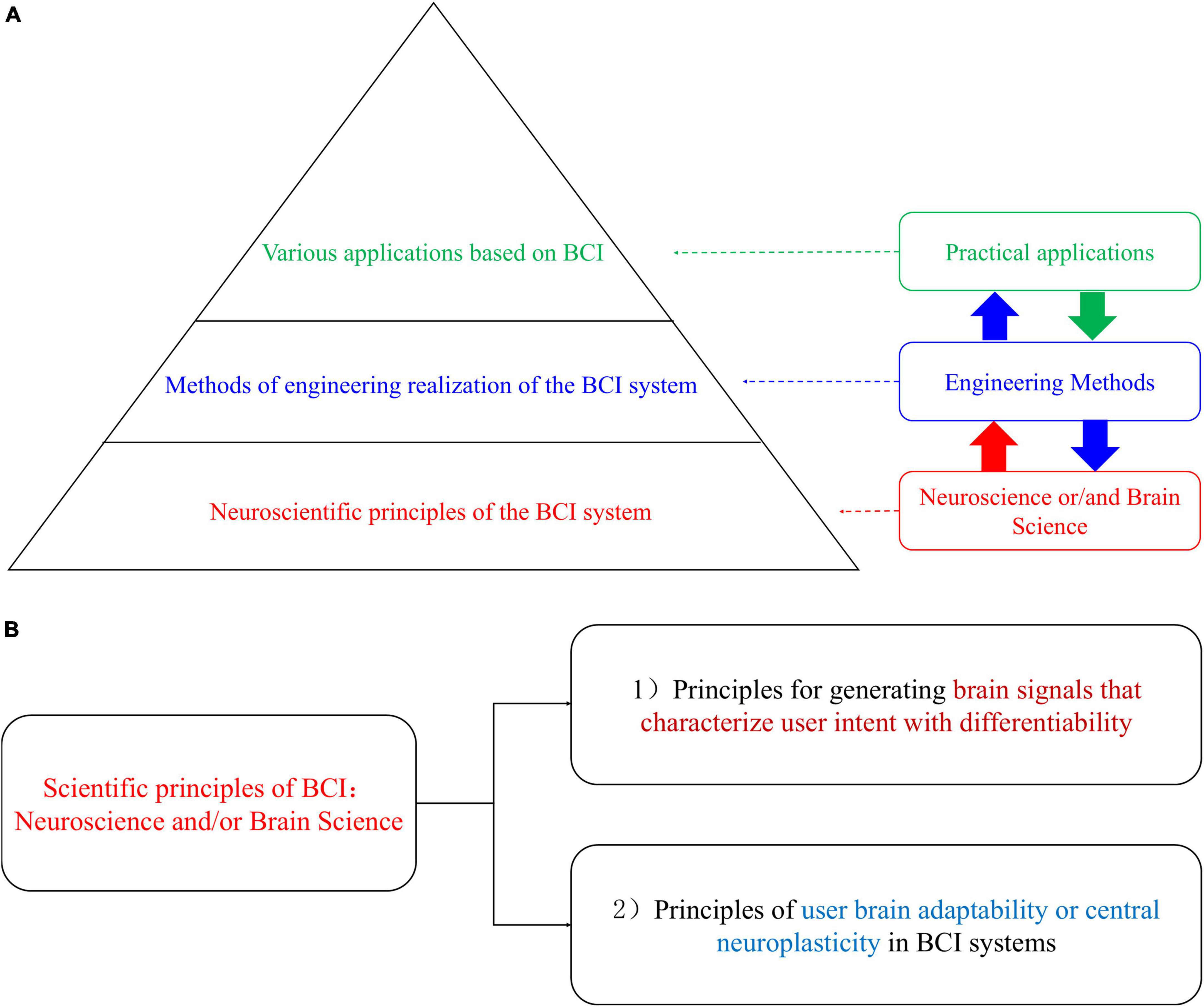
Figure 3. Three important aspects are involved in BCI research and development. (A) The relationship between neuroscientific principles, engineering methods of realization, and practical applications in BCI research and development; (B) the scientific principles of BCI.
In addition to being directly related to neuroscience or brain science, BCI is also closely related to advanced computer science and technology, and artificial intelligence. This includes areas such as computer programming, software engineering, algorithm analysis and design, modern signal processing, pattern recognition, machine learning, deep learning, and artificial intelligence (Yang et al., 2024). Computer science and AI serve as tools or engineering methods for the implementation of BCI systems. In addition, user-centered BCI design is directly associated with human ergonomics (Lu et al., 2021) and is also closely related to fields such as biomedical engineering, neural and rehabilitation engineering, and intelligent robotic control. Figure 4 illustrates the multidisciplinary nature of BCI research and development (Yang et al., 2024).
In conclusion, given the multidisciplinary and unique nature of BCI research and development (particularly its direct connection with the brain). Effective collaboration among these diverse disciplines is essential for achieving the primary goal of BCI: providing significant new communication and control options for individuals with severe disabilities (Wolpaw and Wolpaw, 2012). Effective interdisciplinary collaboration is also required to achieve other goals of BCI beyond the primary goal (e.g., additional medical or non-medical applications) (Yang et al., 2024).
3.2 Principles of curriculum design for the brain-computer interface major
As mentioned above, BCI research and development is inherently multidisciplinary. Therefore, the curriculum design of the BCI major must fully consider its multidisciplinary characteristics and integrate different knowledge systems and practical methodologies. In this unique context, the following eight curriculum design principles are proposed to effectively construct the BCI major.
3.2.1 Multidisciplinary collaboration based on brain-computer interface research and development
As shown in Figure 4, BCI system development involves numerous disciplines. Successful development of a BCI system (i.e., one that provides high usability and user satisfaction) requires multidisciplinary collaboration. The curriculum should strive to include essential disciplines in the course structure. Consideration should also be given to additional disciplinary support in the curriculum if needed.
3.2.2 Content selection and necessity principle
Given the wide range of disciplines involved in BCI, each containing extensive content, it is essential to prioritize the knowledge most relevant to BCI system development. This means not simply including all knowledge from related disciplines in the curriculum. For example, careful consideration must be given to which aspects of neuroscience, psychology, and cognitive science are essential for BCI education, as well as which specific content from computer science and artificial intelligence should be integrated into the curriculum.
3.2.3 Fostering interdisciplinary innovation as a core principle
BCI is a fundamental driver of innovation as a revolutionizing human-computer interaction technology. The curriculum should focus on cultivating students’ ability to innovate across disciplines. Modules such as thematic seminars, open-ended experiments, and capstone projects can be incorporated to inspire students’ innovative thinking and encourage interdisciplinary research and technological exploration.
3.2.4 Integration of brain-computer interface principles, practices, and project-based learning
The curriculum should embed practical BCI development projects into the coursework, in addition to teaching the theoretical principles of BCI. This can be achieved through BCI experiments, training, internships, and research projects. Collaborating with BCI companies on projects can provide students with real-world scenarios to apply their theoretical knowledge. This project-based learning approach increases students’ interest in learning and their ability to address practical challenges in BCI development. It also helps them develop teamwork skills and the ability to make decisions under uncertain conditions.
3.2.5 Flexibility and dynamic updates in curriculum design
Several seminal works in the field of BCI have been published (Allison et al., 2012; Graimann et al., 2010; Ramsey and Millán, 2020; Wolpaw and Wolpaw, 2012), and these publications serve as essential teaching references for senior undergraduate and graduate students at various universities and research institutes worldwide, including in China. They provide critical support for the curriculum design of the BCI major, enabling students to systematically master the underlying principles and practical applications of BCI technology. However, as BCI technology and its associated disciplines continue to evolve, the curriculum must remain flexible and be periodically updated. For instance, it is imperative to promptly incorporate the latest advances in neuroscience, psychology, and cognitive science, as well as emerging engineering methods and application cases, into the curriculum. Extensive exchanges within the BCI community facilitate this process, allowing both educators and students to stay current with the latest developments. For example, master classes of the tenth international brain-computer interface meeting in 2023 (Cernera et al., 2025) showcased recent research outcomes across diverse areas—from signal acquisition and decoding to system implementation—thus fostering interdisciplinary interaction and providing cutting-edge insights and practical experience to further advance BCI applications in clinical rehabilitation, assistive communication, and intelligent control. This dynamic exchange platform not only enables students to keep pace with evolving technologies and receive expert feedback on their research but also establishes a solid foundation for the continual update and improvement of the BCI major curriculum.
3.2.6 Fostering self-directed learning and critical thinking
Adhering to a student-centered approach that values individuality, the curriculum should emphasize cultivating independent thinking, free inquiry, and questioning scientific spirit in the learning and research process. To achieve this, students’ ability to learn in a self-directed manner can be enhanced through flexible elective modules and personalized learning plans. Additionally, course content and teaching methods should encourage students to question and critically evaluate existing results or conclusions, and foster their ability to propose and test new ideas.
3.2.7 Humanities and social sciences literacy
In addition to the interdisciplinary nature of BCI, students in the BCI major are expected to develop literacy in the humanities and social sciences. They are encouraged to take courses either related to or entirely different from their major to broaden their perspectives. Because of the significant social impact of BCI, the curriculum must include education in ethics and social responsibility. Students should understand the challenges posed by BCI in areas such as mental and physical health, privacy, and the misuse of technology, and cultivate a strong sense of social responsibility.
3.2.8 Assessment based on learning processes and outcomes
Student assessment should go beyond final exam scores to include diverse methods such as class participation, group collaboration, research reports, and practical performance. This multidimensional assessment approach emphasizes a comprehensive evaluation of students’ learning processes and abilities.
By implementing these principles, the BCI curriculum can meet the demands of a multidisciplinary educational environment and support the development of students into innovative and practice-oriented interdisciplinary professionals.
3.3 Curriculum design for the brain-computer interface major
It is recommended that the curriculum design for the BCI major consider the principles to establish a structured and well-organized knowledge system. This system could include disciplinary basic courses, introductory courses (including brain science, computer science, and artificial intelligence), core courses of the major, practice training for the major (including BCI experiments, training, and internships), and comprehensive training for the dissertation, as illustrated in Figure 5. Through systematic progression from basic courses to specialized courses and then to practical courses, students can gradually deepen their understanding of BCI technology during their studies.
In Figure 5, the inclusion of introductory courses for major disciplines ensures that students gain a broad understanding of brain science, computer science, and artificial intelligence as they relate to BCI development. This fosters research interest and lays the foundation for subsequent courses. The integration of practice training helps students combine BCI theories, principles, and methods with practice experience, thereby enhancing their understanding of specialized BCI course content. This also improves their ability to solve practical problems and nurtures innovation in real-world BCI application scenarios. The inclusion of comprehensive training for the dissertation component aids in developing students’ mastery of key skills such as BCI research methodologies, data analysis, and results interpretation. This process enhances their innovation capacity and critical thinking abilities.
In the practical teaching of the BCI major, phased objectives, project-based tasks, and theoretical integration are combined to enhance students’ research capabilities and problem-solving skills effectively. Practical training is structured into three stages. the foundational skills phase, where students use EEG devices to acquire and preprocess motor imagery-related brain signals while mastering noise filtering and feature extraction techniques; the system development phase, exemplified by the creation of a P300-based speller system; and the application innovation phase, where VR technology is integrated to develop neurorehabilitation training systems, fostering interdisciplinary collaboration and real-world application in complex scenarios. Practical projects encompass classical paradigms such as steady-state visual evoked potentials (SSVEP) and MI-BCI, advanced research topics (e.g., invasive BCI signal acquisition and deep learning-based decoding), and interdisciplinary applications (e.g., brain-controlled robotics and AR-BCI game development). Regarding resource utilization, open-source hardware such as OpenBCI and virtual simulation platforms are employed to reduce experimental costs. Additionally, a multifaceted evaluation framework—including project defenses, user testing, and competitive events—is implemented to comprehensively assess students’ technical proficiency, innovative thinking, and teamwork abilities.
In Figure 5, the inclusion of disciplinary basic courses aims to meet the demands of interdisciplinary collaboration and integration inherent in BCI development, laying a solid foundation for future research, development, and application in the field. Table 3 lists the proposed disciplinary basic courses for the BCI major and their necessity (Yang et al., 2024). These courses include essential mathematical foundations for quantitative analysis of brain signals and system modeling in BCI system development, such as calculus, linear algebra, and probability and statistics. Additionally, courses supporting BCI hardware development are included, such as physics and brain signal acquisition technologies. Furthermore, the curriculum also includes foundational courses for BCI software development, such as algorithm analysis and design, digital signal processing algorithms, and data structures, which are also part of the curriculum.
The inclusion of specialized core courses in Figure 5 helps students gain a solid understanding of the fundamental principles and practical skills specific to the BCI field. Table 4 outlines the proposed core courses for the BCI major and their necessity (Yang et al., 2024). These courses include principles fundamental to BCI systems, such as BCI principles, neuroscience principles and applications, and fundamentals of cognitive neuroscience. Additionally, courses related to BCI system development and application, such as human-computer interaction, assistive technologies, and neural and rehabilitation engineering, are also part of the curriculum.
From the above, it is evident that the curriculum design for the BCI major, based on an interdisciplinary collaboration for BCI development, includes courses from various academic disciplines. While ensuring that students acquire the necessary foundational knowledge and essential skills closely related to BCI, it is also critical to identify and prioritize core courses in key disciplines.
In curriculum design, foundational courses, core courses, and practical training collectively form a comprehensive knowledge system through progressive layering and interdisciplinary collaboration. Foundational courses provide mathematical and engineering tools for BCI technology. For instance, probability and statistics lay the groundwork for modeling noise in brain signals, linear algebra underpins the matrix operations necessary for feature extraction in machine learning, and digital signal processing algorithms are directly applied to filtering and denoising EEG signals. Core courses (e.g., brain signal processing, human-computer interaction, and neural engineering) focus on cultivating the essential skills of BCI by bridging foundational knowledge with industry requirements through both theoretical deepening and practical application (e.g., developing algorithms for classifying motor imagery-related brain signals and optimizing user interfaces). Practical training, incorporating BCI experiments and industry partnership projects, further integrates interdisciplinary knowledge. This three-tiered framework, which spans from fundamental principles to technological integration and finally to innovative applications, serves to enhance the logical coherence among courses. Moreover, it establishes a closed-loop competency development system through interdisciplinary collaboration (from mathematical modeling to algorithm implementation to clinical validation), ensuring that students systematically master the skills required, from brain signal decoding to system optimization.
4 Discussion
4.1 What are the challenges in constructing the brain-computer interface major?
There are many challenges in constructing the BCI major, particularly because of its highly multidisciplinary nature, its status as a frontier research area, and its rapid pace of development. Below are some key challenges and suggested strategies for addressing them.
4.1.1 The lack of consensus and international collaboration in the construction of the brain-computer interface major
The BCI major is an emerging major, and there is currently no consensus on how to construct this major especially regarding curriculum design. This lack of consensus results in limited references for major construction. Therefore, international collaboration within the BCI community is essential. Drawing on the advanced experiences of BCI research and education in different regions or countries can help to promote cooperation in constructing the BCI major. In particular, the establishment of an internationally oriented BCI curriculum based on its multidisciplinary nature is crucial. The BCI community has already published several significant works on the topic (Allison et al., 2012; Collura, 2014; Graimann et al., 2010; Ramsey and Millán, 2020; Wolpaw and Wolpaw, 2012).
Existing literature has preliminarily discussed the framework for a BCI major, including the academic system, degree awards, educational objectives, curriculum design, and credit distribution (Yang et al., 2024). However, this literature does not provide detailed syllabi for each course (including learning objectives, content, and teaching methods). This omission may be partly due to space limitations and partly because of the differences in syllabi across regions or countries. For course syllabi, the selection of teaching content is critical. Only the essential content should be included, avoiding the inclusion of all possible topics or a simple compilation of existing materials. Additionally, the literature may have overlooked certain courses that are essential for the BCI major, such as Human-Computer Interaction and Assistive Technology. Finally, aspects such as the prerequisites for each course (i.e., the logical sequence of courses), the objectives of each course and their alignment with the overall educational goals of the major, teaching content, assessment methods, textbooks, and reference materials all require careful consideration.
4.1.2 Inadequate or lack of course materials for brain-computer interface major
As an emerging major, the BCI major is still in its infancy and developmental stages. At present, and for the foreseeable future, there remains a lack of comprehensive or specialized textbooks for its curriculum (Yang et al., 2024). Although the BCI community has already published several significant works (Allison et al., 2012; Collura, 2014; Graimann et al., 2010; Ramsey and Millán, 2020; Wolpaw and Wolpaw, 2012), the development of textbooks for BCI courses still faces the following main challenges.
4.1.2.1 Mismatch between existing brain-computer interface books and the demands of the brain-computer interface curriculum
Existing edited volumes or monographs on BCI primarily focus on research and academics. They often summarize and integrate the work of prominent international research teams in the BCI field. While these are valuable and rich as reference materials for BCI teaching, they may not be entirely suitable as textbooks. It is recommended to fully consider teaching objectives, methods, and principles, organizing the content in a progressive and logical sequence. Although BCI theories, principles, and methods have evolved into a relatively stable and widely accepted framework over the past 50 years, existing BCI books were published at specific points in time and may need to be updated to reflect the latest advances in BCI research. In addition, these publications often lack thought-provoking questions and exercises designed to engage students and inspire innovation.
4.1.2.2 Lack of brain-computer interface experimental material
BCI is rooted in experimental science and technology research as opposed to purely theoretical research. As previously mentioned, the BCI major not only focuses on teaching BCI theories, principles, and methods but also emphasizes the combination of theory and practice through experimental teaching. However, there is currently a lack of complementary experimental manuals tailored for BCI education.
It is advisable to consider incorporating the three classical BCI paradigms (SSVEP-BCI, MI-BCI, and P300-BCI) as the core experimental teaching content, while also adding some of the latest BCI paradigms. For each BCI paradigm in experimental tutorials, the content could include the following: Experimental objectives, required hardware and software, Experimental design, Step-by-step procedures, Full-system implementation and Provide support for computer technology in BCI systems, to understand the fundamentals of computer systems, and to support the design and optimization of BCI system. Developing such experimental manuals will be essential for fostering hands-on skills and practical understanding among students.
4.1.2.3 How to select, integrate, and develop systematic textbooks from multidisciplinary content closely related to brain-computer interface
As previously mentioned, BCI involves multiple disciplines, including neuroscience, psychology and cognitive science, computer science and technology, signal processing and pattern recognition, machine learning and artificial intelligence, human factors engineering, and neurorehabilitation engineering. Based on existing textbooks in these disciplines, it is challenging to determine what to include, omit, or integrate in a systematic BCI textbook. This challenge is particularly significant in ensuring that the content covers the foundational knowledge required for BCI research and development. The design of a BCI curriculum must balance breadth and depth within the constraints of limited class time. It is essential to avoid merely combining materials or attempting to cover everything comprehensively. Instead, a selective and focused approach is recommended. To achieve this, a multidisciplinary team of experts from related fields should collaborate to develop the textbook, with each expert contributing content from their area of expertise while ensuring coherence and integration across disciplines.
4.1.2.4 How to ensure the brain-computer interface curriculum reflects the rapid advancements in brain-computer interface technology
BCI technology is advancing rapidly as neuroscientific, hardware, algorithmic, and applicational advances continue to be made. In particular, advancements in brain signal acquisition technologies, new processing algorithms, machine learning and deep learning, and brain-machine interfaces are occurring at a fast pace. This necessitates frequent adjustments to course content to keep up with the latest developments.
It is recommended to create a “stable edition” textbook that covers the basics, core principles, and methodologies, while periodically updating course content to reflect new technologies and research findings. For example: Introduce elective courses and special-topic seminars to include emerging technologies and the latest research. Supplement textbooks with online resources and up-to-date scientific literature. Periodically update or release digital chapters to facilitate content adjustments in response to advancements. Additionally, students should be encouraged to participate in research projects and academic competitions to deepen their understanding of frontier technologies. This dynamic approach will ensure that the BCI curriculum remains relevant and aligned with the rapid progress in the field.
4.1.3 High-cost brain-computer interface experimental equipment and resources
The construction of BCI as an academic discipline requires the establishment of teaching-oriented BCI laboratories. Most existing BCI laboratories are research-focused rather than tailored for teaching purposes at present. In addition, the BCI hardware and software systems available in the commercial market are generally costly. Given that teaching usually demands multiple experimental platforms, this consequently results in high configuration expenses.
It is recommended to develop BCI experimental systems specifically designed for teaching with significant cost and price reductions to support bulk deployment. In addition, university-industry collaborations and resource-sharing initiatives can address the shortage of experimental facilities. The establishment of virtual simulation platforms for experiments can also help students to simulate experimental procedures and data analysis via software, thus reducing costs (Yang et al., 2024).
4.1.4 Shortage of faculty in the brain-computer interface major
Faculty for the BCI major needs to have a multidisciplinary background. However, there is currently a shortage of full-time faculty in universities with expertise in both neuroscience and computer technology, which impacts the quality of teaching. It is proposed to invite experts with multidisciplinary BCI backgrounds to serve as guest lecturers or mentors. Existing faculty members should be encouraged to participate in multidisciplinary BCI training programs to enhance their expertise and contribute to high-quality teaching at the same time (Yang et al., 2024).
4.2 What is the prospect of the BCI major?
The prospects of the BCI major are closely tied to the development of BCI technology and its transition into practical applications. With continuous advancements in fields such as neuroscience, psychology and cognitive science, computer science and technology, artificial intelligence, and ergonomics, BCI technology is expected to move gradually from the laboratory to practical applications. Its use cases will expand beyond medical rehabilitation to encompass education, entertainment, intelligent control, augmented reality, and other domains.
In medical applications, BCI technology will provide new diagnostic, therapeutic, and rehabilitative solutions for individuals with severe motor impairments and disabilities, such as paralyzed patients, individuals with aphasia, and those with other neurological or psychiatric conditions. In non-medical applications, such as brain-controlled games and brain-machine collaborative robotics, BCI technology will bring novel experiences and services to healthy individuals. These applications will provide diverse industries for BCI students to showcase their talents.
4.3 Limitations of the study and future work
Based on the multidisciplinary nature of BCI technology, this paper proposes eight principles for designing the BCI curriculum and develops the hierarchical structure of curriculum design for the BCI major that includes basic courses in discipline, introductory courses, core courses of the major, practice training, and comprehensive training for dissertations. This hierarchical structure provides a reference for the construction of the BCI major. However, the guidelines for designing the BCI curriculum still require in-depth consideration, supplementation, and refinement; it is especially necessary to evaluate the curriculum design of the BCI major from a competency-oriented perspective, which may include the following:
(1) Clearly defining the skills and knowledge covered by the BCI major. This includes supporting evidence of these skills and knowledge through needs assessment studies, interviews, analysis of future jobs, market research, job positions, etc.
(2) Matching the skills and knowledge with the appropriate teaching methods and techniques.
(3) Designing a structured curriculum grid. The grid can provide insights into how to weave and interleave the skills and knowledge across different courses, including the workload of classroom and independent assignments.
(4) Integrating ethical issues related to BCI throughout the curriculum. Because of the direct connection between BCI and the medical practice and the human brain, Ethical issues should be covered across the curriculum, with putting the human in the center rather than the technology.
5 Conclusion
This paper focuses on the emerging BCI major and based on the inherent and essential multidisciplinary nature of BCI system research and development, proposes curriculum design principles for the BCI major and provides recommendations for its curriculum structure. These principles and recommendations are preliminary. The next step is to improve the BCI major’s curriculum design from a competency-oriented perspective. As BCI technology continues to evolve, the curriculum design and development of the BCI major will be further refined. The challenges in the construction of the BCI major will be gradually overcome, paving the way for a brighter future for this major.
Author contributions
HY: Investigation, Writing – original draft. TL: Writing – review & editing, Investigation. LZ: Writing – review & editing, Investigation. YW: Writing – review & editing, Funding acquisition, Investigation, Project administration. XC: Writing – review & editing, Investigation, Methodology. JP: Writing – review & editing, Investigation, Methodology. YF: Conceptualization, Funding acquisition, Investigation, Project administration, Supervision, Writing – review & editing, Methodology.
Funding
The author(s) declare that financial support was received for the research and/or publication of this article. This study was partially funded by the National Natural Science Foundation of China (grant numbers 82172058, 62376112, 81771926, 61763022, and 62366026).
Conflict of interest
The authors declare that the research was conducted in the absence of any commercial or financial relationships that could be construed as a potential conflict of interest.
The author(s) declared that they were an editorial board member of Frontiers, at the time of submission. This had no impact on the peer review process and the final decision.
Generative AI statement
The authors declare that no Generative AI was used in the creation of this manuscript.
Publisher’s note
All claims expressed in this article are solely those of the authors and do not necessarily represent those of their affiliated organizations, or those of the publisher, the editors and the reviewers. Any product that may be evaluated in this article, or claim that may be made by its manufacturer, is not guaranteed or endorsed by the publisher.
References
Aflalo, T., Kellis, S., Klaes, C., Lee, B., Shi, Y., Pejsa, K., et al. (2015). Decoding motor imagery from the posterior parietal cortex of a tetraplegic human. Science 348, 906–910. doi: 10.1126/science.aaa5417
Al-Hudhud, G., Alqahtani, L., Albaity, H., Alsaeed, D., and Al-Turaiki, I. (2019). Analyzing passive BCI signals to control adaptive automation devices. Sensors (Basel) 19:3042. doi: 10.3390/s19143042
Allison, B. Z., Dunne, S., Leeb, R., Millán, J. D. R., and Nijholt, A. (eds) (2012). Towards practical brain-computer interfaces: Bridging the gap from research to real-world applications. Berlin: Springer Science & Business Media.
Birbaumer, N., Ghanayim, N., Hinterberger, T., Iversen, I., Kotchoubey, B., Kübler, A., et al. (1999). A spelling device for the paralysed. Nature 398, 297–298. doi: 10.1038/18581
Blankertz, B., Dornhege, G., Krauledat, M., Müller, K. R., and Curio, G. (2007). The non-invasive Berlin brain–computer interface: Fast acquisition of effective performance in untrained subjects. NeuroImage 37, 539–550. doi: 10.1016/j.neuroimage.2007.01.051
Cernera, S., Gemicioglu, T., Berezutskaya, J., Csaky, R., Verwoert, M., Polyakov, D., et al. (2025). Master classes of the tenth international brain–computer interface meeting: Showcasing the research of BCI trainees. J. Neural Eng. 22:022001. doi: 10.1088/1741-2552/adb335
Chapin, J. K., Moxon, K. A., Markowitz, R. S., and Nicolelis, M. A. (1999). Real-time control of a robot arm using simultaneously recorded neurons in the motor cortex. Nat. Neurosci. 2, 664–670. doi: 10.1038/10223
Chen, Y., Wang, F., Li, T., Zhao, L., Gong, A., Nan, W., et al. (2024). Considerations and discussions on the clear definition and definite scope of brain-computer interfaces. Front. Neurosci. 18:1449208. doi: 10.3389/fnins.2024.1449208
Coulter, M. E., and Kemere, C. (2023). The neural basis of mental navigation in rats. Science 382, 517–518. doi: 10.1126/science.adl0806
Daly, J. J., and Wolpaw, J. R. (2008). Brain–computer interfaces in neurological rehabilitation. Lancet Neurol. 7, 1032–1043. doi: 10.1016/S1474-4422(08)70223-0
Donoghue, J. P. (2002). Connecting cortex to machines: Recent advances in brain interfaces. Nat. Neurosci. 5(Suppl.), 1085–1088. doi: 10.1038/nn947
Fetz, E. E. (1969). Operant conditioning of cortical unit activity. Science 163, 955–958. doi: 10.1126/science.163.3870.955
Fetz, E. E., and Finocchio, D. V. (1971). Operant conditioning of specific patterns of neural and muscular activity. Science 174, 431–435. doi: 10.1126/science.174.4007.431
Flesher, S. N., Downey, J. E., Weiss, J. M., Hughes, C. L., Herrera, A. J., Tyler-Kabara, E. C., et al. (2021). A brain-computer interface that evokes tactile sensations improves robotic arm control. Science 372, 831–836. doi: 10.1126/science.abd0380
Graimann, B., Allison, B., and Pfurtscheller, G. (2010). “Brain–computer interfaces: A gentle introduction,” in Brain-computer interfaces: Revolutionizing human-computer interaction, eds B. Graimann, G. Pfurtscheller, and B. Allison (Berlin: Springer Berlin Heidelberg), 1–27.
Hochberg, L. R., Bacher, D., Jarosiewicz, B., Masse, N. Y., Simeral, J. D., Vogel, J., et al. (2012). Reach and grasp by people with tetraplegia using a neurally controlled robotic arm. Nature 485, 372–375. doi: 10.1038/nature11076
Hochberg, L. R., Serruya, M. D., Friehs, G. M., Mukand, J. A., Saleh, M., Caplan, A. H., et al. (2006). Neuronal ensemble control of prosthetic devices by a human with tetraplegia. Nature 442, 164–171. doi: 10.1038/nature04970
İnce, R., Adanır, S. S., and Sevmez, F. (2021). The inventor of electroencephalography (EEG): Hans berger (1873–1941). Child’s Nerv. Syst. 37, 2723–2724. doi: 10.1007/s00381-020-04564-z
Jamil, N., Belkacem, A. N., Ouhbi, S., and Guger, C. (2021). Cognitive and affective brain–computer interfaces for improving learning strategies and enhancing student capabilities: A systematic literature review. IEEE Access 9, 134122–134147. doi: 10.1109/ACCESS.2021.3115263
Kohli, V., Tripathi, U., Chamola, V., Rout, B. K., and Kanhere, S. S. (2022). A review on virtual reality and augmented reality use-cases of brain computer interface based applications for smart cities. Microprocess. Microsyst. 88:104392. doi: 10.1016/j.micpro.2021.104392
LaConte, S. M. (2011). Decoding fMRI brain states in real-time. Neuroimage 56, 440–454. doi: 10.1016/j.neuroimage.2010.06.052
Lai, C., Tanaka, S., Harris, T. D., and Lee, A. K. (2023). Volitional activation of remote place representations with a hippocampal brain-machine interface. Science 382, 566–573. doi: 10.1126/science.adh5206
Lebedev, M. A., and Nicolelis, M. A. (2006). Brain–machine interfaces: Past, present and future. Trends Neurosci. 29, 536–546. doi: 10.1016/j.tins.2006.07.004
Lorach, H., Galvez, A., Spagnolo, V., Martel, F., Karakas, S., Intering, N., et al. (2023). Walking naturally after spinal cord injury using a brain-spine interface. Nature 618, 126–133. doi: 10.1038/s41586-023-06094-5
Lu, X., Ding, P., Li, S., Gong, A., Zhao, L., Qian, Q., et al. (2021). Human factors engineering of brain-computer interface and its applications: Human-centered brain-computer interface design and evaluation methodology. J. Biomed. Eng. 38, 210–223. doi: 10.7507/1001-5515.202101093
Luo, J., Ding, P., Gong, A., Tian, G., Xu, H., Zhao, L., et al. (2022). Applications, industrial transformation and commercial value of brain-computer interface technology. J. Biomed. Eng. 39, 405–415. doi: 10.7507/1001-5515.202108068
Metzger, S. L., Littlejohn, K. T., Silva, A. B., Moses, D. A., Seaton, M. P., Wang, R., et al. (2023). A high-performance neuroprosthesis for speech decoding and avatar control. Nature 620, 1037–1046. doi: 10.1038/s41586-023-06443-4
Millan, J. R., Renkens, F., Mourino, J., and Gerstner, W. (2004). Noninvasive brain-actuated control of a mobile robot by human EEG. IEEE Trans. Biomed. Eng. 51, 1026–1033. doi: 10.1109/TBME.2004.827086
Minev, I. R., Musienko, P., Hirsch, A., Barraud, Q., Wenger, N., Moraud, E. M., et al. (2015). Electronic dura mater for long-term multimodal neural interfaces. Science 347, 159–163. doi: 10.1126/science.1260318
Musallam, S., Corneil, B. D., Greger, B., Scherberger, H., and Andersen, R. A. (2004). Cognitive control signals for neural prosthetics. Science 305, 258–262. doi: 10.1126/science.1097938
Nicolelis, M. A. (2003). Brain-machine interfaces to restore motor function and probe neural circuits. Nat. Rev. Neurosci. 4, 417–422. doi: 10.1038/nrn1105
Powers, J. C., Bieliaieva, K., Wu, S., and Nam, C. S. (2015). The human factors and ergonomics of P300-based brain-computer interfaces. Brain Sci. 5, 318–356. doi: 10.3390/brainsci5030318
Prapas, G., Glavas, K., Tzimourta, K. D., Tzallas, A. T., and Tsipouras, M. G. (2023). Mind the move: Developing a brain-computer interface game with left-right motor imagery. Information 14:354. doi: 10.3390/info14070354
Ramsey, N. F., and Crone, N. E. (2023). Speech-enabling brain implants pass milestones. Nature 620, 954–955. doi: 10.1038/d41586-023-02546-0
Santhanam, G., Ryu, S. I., Yu, B. M., Afshar, A., and Shenoy, K. V. (2006). A high-performance brain–computer interface. Nature 442, 195–198. doi: 10.1038/nature04968
Schwartz, A. B., Moran, D. W., and Reina, G. A. (2004). Differential representation of perception and action in the frontal cortex. Science 303, 380–383. doi: 10.1126/science.1087788
Scott, S. H. (2006). Neuroscience: Converting thoughts into action. Nature 442, 141–142. doi: 10.1038/442141a
Tai, P., Ding, P., Wang, F., Gong, A., Li, T., Zhao, L., et al. (2024). Brain-computer interface paradigms and neural coding. Front. Neurosci. 17:1345961. doi: 10.3389/fnins.2023.1345961
Taylor, D. M., Tillery, S. I. H., and Schwartz, A. B. (2002). Direct cortical control of 3D neuroprosthetic devices. Science 296, 1829–1832. doi: 10.1126/science.1070291
Vasiljevic, G. A. M., and Cunha de Miranda, L. (2024). The CoDIS taxonomy for brain-computer interface games controlled by electroencephalography. Int. J. Human–Comp. Interact. 40, 3908–3935. doi: 10.1080/10447318.2023.2203006
Vidal, J. J. (1973). Toward direct brain-computer communication. Annu. Rev. Biophys. Bioeng. 2, 157–180. doi: 10.1146/annurev.bb.02.060173.001105
Vidal, J. J. (1977). Real-time detection of brain events in EEG. Proc. IEEE 65, 633–641. doi: 10.1109/PROC.1977.10542
Walter, W. G., Cooper, R., Aldridge, V. J., McCallum, W. C., and Winter, A. L. (1964). Contingent negative variation: An electric sign of sensori-motor association and expectancy in the human brain. Nature 203, 380–384. doi: 10.1038/203380a0
Willett, F. R., Avansino, D. T., Hochberg, L. R., Henderson, J. M., and Shenoy, K. V. (2021). High-performance brain-to-text communication via handwriting. Nature 593, 249–254. doi: 10.1038/s41586-021-03506-2
Willett, F. R., Kunz, E. M., Fan, C., Avansino, D. T., Wilson, G. H., Choi, E. Y., et al. (2023). A high-performance speech neuroprosthesis. Nature 620, 1031–1036. doi: 10.1038/s41586-023-06377-x
Wolpaw, J. R., and Wolpaw, E. W. (2012). “Brain-computer interfaces: Something new under the sun,” in Brain-computer interfaces: Principles and practice, eds J. R. Wolpaw and E. W. Wolpaw (Oxford: Oxford University Press).
Wolpaw, J. R., McFarland, D. J., Neat, G. W., and Forneris, C. A. (1991). An EEG-based brain-computer interface for cursor control. Electroencephalogr. Clin. Neurophysiol. 78, 252–259. doi: 10.1016/0013-4694(91)90040-b
Keywords: brain-computer interface, BCI major, multidisciplinary nature of BCI, curriculum design, construction of BCI major
Citation: Yang H, Li T, Zhao L, Wei Y, Chen X, Pan J and Fu Y (2025) Guiding principles and considerations for designing a well-structured curriculum for the brain-computer interface major based on the multidisciplinary nature of brain-computer interface. Front. Hum. Neurosci. 19:1554266. doi: 10.3389/fnhum.2025.1554266
Received: 01 January 2025; Accepted: 23 April 2025;
Published: 15 May 2025.
Edited by:
Jianjun Meng, Shanghai Jiao Tong University, ChinaCopyright © 2025 Yang, Li, Zhao, Wei, Chen, Pan and Fu. This is an open-access article distributed under the terms of the Creative Commons Attribution License (CC BY). The use, distribution or reproduction in other forums is permitted, provided the original author(s) and the copyright owner(s) are credited and that the original publication in this journal is cited, in accordance with accepted academic practice. No use, distribution or reproduction is permitted which does not comply with these terms.
*Correspondence: Yunfa Fu, ZnlmQHludS5lZHUuY24=