- 1Department of Psychology, University of Oslo, Oslo, Norway
- 2Department of Language and Literature, Norwegian University of Science and Technology, Trondheim, Norway
- 3Department of Culture Studies and Oriental Languages, University of Oslo, Oslo, Norway
Directing visual attention toward items mentioned within utterances can optimize understanding the unfolding spoken language and preparing appropriate behaviors. In several languages, numeral classifiers specify semantic classes of nouns but can also function as reference trackers. Whereas all classifier types function to single out objects for reference in the real world and may assist attentional guidance, we propose that only sortal classifiers efficiently guide visual attention by being inherently attached to the nouns' semantics, since container classifiers are pragmatically attached to the nouns they classify, and the default classifiers index a noun without specifying the semantics. By contrast, container classifiers are pragmatically attached, and default classifiers index a noun without specifying the semantics. Using eye tracking and the “visual world paradigm”, we had Chinese speakers (N = 20) listen to sentences and we observed that they looked spontaneously within 150 ms after offset of the Sortal classifier. After about 200 ms the same occurred for the container classifiers, but with the default classifier only after about 700 ms. This looking pattern was absent in a control group of non-Chinese speakers and the Chinese speakers' gaze behavior can therefore only be ascribed to classifier semantics and not to artifacts of the visual objects. Thus, we found that classifier types affect the rapidity of spontaneously looking at the target objects on a screen. These significantly different latencies indicate that the stronger the semantic relatedness between a classifier and its noun, the more efficient the deployment of overt attention.
1. Introduction
Language provides a bridge between communicating minds and the physical world and therefore it can also have a profound effect on the direction and distribution of attention in physical space (Altmann and Kamide, 2007). Previous psycholinguistic studies have shown that visual attention toward items can be mediated during the unfolding of spoken language. That is, people tend to focus attention spontaneously onto the objects or agents corresponding to the meaningful elements in a sentence. Cooper (1974) labeled this behavior “an active online anticipative process” and stressed the role of what he called “highly informative words,” but also found that function words like “and” instigated listeners to direct their gaze to a second item in the visual scene. A constructive way to view this function of speech was suggested by Talmy (2000) in the “windowing of attention”; i.e., selecting one portion of the present scene for reference. In many real-world situations, a plurality of things could potentially be highlighted and become the focus of verbal communication and (in turn) of attention.
Specifically, a single sentence can foreground a portion of the here-and-now world by the explicit mentioning of something and by simultanously placing the remainder of the situation in the background. Talmy refers to foregrounded segments as “event frames”, for example Figure-Ground relationships; foregrounded portions are windowed and backgrounded portions are gapped. That is, the Figure and the Ground in a spatial scene are relative concepts necessarily characterized with respect to each other, where Figure is defined entirely in terms of which segment is highlighted for attention, as opposed to the rest as Ground (Stocker and Laeng, 2017). For example, in a situation where the paint is peeling from the wall, one could select as Figure either the paint or the wall (“the paint is peeling” vs. “the wall is peeling”; Talmy, 2000, p. 290), with clear consequences for the scope of attention (e.g., the attentional window can expand or contract to encompass the referent). In this way, the choice of linguistic expression can impose differential attentional distribution onto objects or events in the external world.
Thus, there can be a direct match between external objects and a concept, allowing a moment-by-moment link between the current physical environment and a mind's state. Typically, meaning is first established by “joint attention” (Moore and Dunham, 1995); that is, semantics is confirmed by overt attention when speakers look at the same object at the same time (Tomasello and Farrar, 1986). However, minds may create additional “units” that will ease communication in everyday situations by using specialized labels for either containment or measurement. The above aspects are reflected in specific linguistic devices known as classifiers. Specifically, there are three main types of classifiers (i.e., sortal, container, default), which are present in several human languages, spoken by billions of people (e.g., Mandarin). Classifiers are relevant to psycholinguistics because they can reveal how cognition structure semantic knowledge, as shown by the similarity between semantic categories identified by classifiers in speakers and semantic-specific deficits in neurological patients (Lobben et al., 2020).
Here, we hypothesize that one of the psycholinguistic roles of classifiers within an utterance is to guide the visual attention of the listener, so that the link between a referent and joint attention are facilitated. In general, a classifier can be founded on a semantic relationship to the noun it classifies (sortal classifiers), it can be a device of measurement (mensural classifiers, e.g., a container), or it can refer to an object in the most general sense, as “a thing” (default classifiers). Considering how these classifier types relate to the nouns they “classify”, thereby to their referents in the world, we should expect visual overt attention to behave differently in each case. Our present goal is to reveal this link between mind and world as it is deployed in time.
1.1. An overview of classifier types
In several languages, classifiers are linguistic devices that label and categorize referents in the external world according to certain semantic domains. These domains are often referred to as extralinguistic, while they also serve specific linguistic and pragmatic functions such as reference tracking of real-world objects as well as the individuation of mass nouns (Aikhenvald, 2000, p. 321, 329). Classifiers are known for characterizing semantic aspects of objects that reflect underlying, near universal conceptual domains, either as kinds that describe objects at the unit level or in terms of configurations that extract abstract qualities (e.g., overall shape) that generalize across objects that may be otherwise dissimilar (Denny, 1979; Denny and Creider, 1986). Importantly, both these types reflect essential semantic aspects that groups of nouns have in common, such as size, shape, dimension, material, consistency, or animacy. Kinds may be in the animate domain (humans, animals), in the living inanimate domain (plants, fruit), or as inanimate objects (artifacts; tools). For reasons of their semantic specificity, some linguists (e.g., Tse et al., 2007) refer to sortal classifiers as “specific classifiers”, hinting at their use being curbed by a well-defined semantic content. It can be argued that sortal classifier semantics can be more specific than mensural (including container) classifiers and this may strengthen the link between classifier and noun. However, it should be noted that specificity can refer also to the number of noun members within a class; the fewer members a classifier has, the more specific it can be with respect to its referents. It is in this sense, Aikhenvald (2021) uses the term “specific classifiers” to cover subgroups of sortal as well as of mensural classifiers, on grounds that they classify a very limited set of referents. In the extreme case, a classifier may be used only with one referent and be a unique classifier (Aikhenvald discusses large classifier systems that cover limited “cultural concerns and practices” Aikhenvald, 2021, p. 243).
To provide an example, Lao has a classifier daang for “nets with evenly spaced holes”—fishing nets, mosquito nets” (Enfield, 2004, p. 122). Such classifiers are specific in the sense of being characterized by “restricted semantics”. They are dependent on certain cultural practices to continue to exist. Hence, to avoid confusion, we refrain here from using the term “specific classifiers”. However, it should be noted that sortal classifiers not only overlap semantically with the nouns they classify but they can clearly define semantically the nouns they group (i.e., they are “specific”). Thus, “sortal” may be better than mensural classifiers in predicting their referents.
Configurational classes distinguish extended from non-extended in space, shapes, and dimensions (one-, two- and three dimensional). Often, natural kinds (like “plant”) are the basis for extracting the configurational classes (Conklin, 1981, p. 136, 341). For example, like in many other classifier languages, the Chinese animal classifier 只 zhı̄ (e.g., 一 只老虎 Yı̄ zhı̄ lǎohǔ “a tiger”) uses “body” in the sense of “container/hollow objects”, as in 一 只桶 Yı̄ zhı̄ tǒng “one bucket”. These types of classifiers are known as sortal.
In numeral classifier systems, sortal classifiers reflect semantic characteristics of the noun being counted, in possessive systems of the noun being owned. This information is redundantly specified in addition to the noun itself, as in this Chinese example:
(1) 一 尾 鮀
yı̄ wěi tuó
one CLASS:FISH catfish
“A (fish) catfish”
However, classifiers can also function as measure terms that combine with mass nouns like sand or water to render them countable, as in (2) below. In contrast to the sortal classifiers, classifiers used for measurement, for example container classifiers, are not usually considered classifiers in a strict sense since they do not in any way “echo” a semantic feature of the noun (Hopper, 1986, p. 316). Their main function may be to deploy them as units in discourse. In other words, the classifier imposes a certain structure on a naturally occurring substance (note that the container classifiers we discuss here are not the same as sortal classifiers for containers, which are found e.g., in Panare and a few other languages; Aikhenvald, 2000, p. 128).
(2) 一 杯 牛奶
yı̄ bēi niúnǎi
one CLASS:CUP cow's milk
“A cup of cow's milk”
A distinct divide in classifier systems runs between sortal classifiers on the one hand and classifiers for measurement, such as container classifiers, on the other. Cheung (2016) distinguishes four mensural classifiers in Mandarin Chinese, among which the container classifiers constitute one subtype. Importantly, while non-configurational sortal classifiers generalize over groups of nouns by extracting their semantic characteristics, typical semantic aspects communicated by classifiers of measurement regard their consistency (fluid as in a cup of milk, solid as in a chunk of meat, etc.) or shape (square and flat, as in a sheet of paper). This aspect is only indirectly communicated by the way these objects can be measured. Note that this indirect semantic relationship is far less specific than that of sortal classifiers, since substances can be measured or contained in many ways (e.g., berries can be contained in a bowl, as a handful or piled up on a table).
Thus, it is useful to think of classifiers in terms of their semantic relatedness (Cooper, 1974; Huettig and Altmann, 2005). Semantic relatedness indicates how much two concepts are related in a taxonomic hierarchy by using all relations between them, e.g., hyperonymic/hyponymic (generic term/specific instance), meronymic (a constituent part of a whole) and any kind of functional relations including is-made-of, is-an-attribute-of, has-part, etc. (Strube and Ponzetto, 2006). Hyperonymy/hyponymy refers to is-a relations and characterizes semantic similarity. A sortal classifier is a hyperonym to the nouns it classifies, i.e., a word whose semantic field is included within that of another word, here the noun it classifies (the hyponym). Put differently, the noun's specification implies the classifier's specification because the classifier's specification is weaker, or more general. The range of references for the hyperonym is therefore wider, and inversely correlated with the strength of its semantic specification. A parallel could be “color”, which is a hyperonym to red, green, and blue; and blue is again a hyperonym to cyan, periwinkle and aquamarine. The narrower the specification, the more limited range of references, and vice versa, but all relationships in the taxonomy imply subsumption, and overlap. Sortal classifiers may also have a meronymic (part-whole) relationship to the nouns they classify, as in heads of cattle. The is-an-attribute-of relationship is quite common in sortal classifiers, as demonstrated in the near omnipresent shape classifiers.
More rarely, sortal classifiers refer to material (e.g., wood) and an is-made-of relation appears. An overlap specification applies in all these cases. Container classifiers, however, are rather characterized by a metonymic relationship between classifier and noun and are not intrinsically attached to the nouns they classify, resulting in a much looser relationship. Metonymy can occur when two concepts are associated because of their contiguity, e.g., when we say that the kettle is boiling (not its content). Containment is one of the broad fields where metonymy is frequently used.
The primary purpose of mensural classifiers is to function as unitizers for things that do not come in natural units (a liter of milk). Therefore, they may assist creating larger units than the naturally occurring ones (two bunches of carrots) or present parts of natural units (two fronds of a palm). Aggregated count nouns may imply a certain arrangement (a row of trees). However, the reference of these concepts is imposed instead of corresponding to naturally occurring units.
If the sortal classifiers seem redundant, the default (general) classifiers, that are devoid of meaning and can be paired with a wide range of nouns, may seem even more so. In fact, at a first glance, they would seem to be superfluous. Hence, to better understand their function, it may be helpful to look at how this part of grammar was established. In a classifier language, its presence is a regular occurrence and noun phrases typically include a “slot” specified for the classifier in the noun phrase syntax. At times, there may not be a noun that is appropriate for any of the semantic categories at one's disposal. In these cases, there are two ways in which the classifier slot can be filled: (a) to use repeaters, by doubling the noun (Aikhenvald, 2000, p. 334); or (b) to use general classifiers.
Linguists have suggested that classifiers that were once quite specific and meaningful in some semantic context became progressively used with other types of items, for the sake of semantic similarity to the original meaning. As a result, they turned out to be semantically bleached or void of content altogether and to function as mere placeholders that fill up the classifier slot. It seems likely that their role is to render a noun phrase grammatical. In Chinese, the general classifier works by default, since it complements across all semantic domains (e.g., in cases where attention is shifted away from the nature of the referent; Erbaugh, 1986; Zubin and Shimojo, 1993). We will therefore reserve the term “default classifier” for present-day use of the Chinese classifier 个 ge and among the general classifier types it is the default classifier that is the topic of this study. Note that, despite this complementary function, a very high number of nouns do not occur with any other classifier than the default classifier in Chinese.
1.2. The sortal, container, and default classifiers in Chinese
In the present study, a fundamental theoretical assumption is that sortal classifiers in Chinese have a tight semantic bond to their following noun. We hypothesize that this aspect allows them to guide attention effectively. That is, sortal classifiers highlight semantic features of the noun just before this is uttered, as in these examples of Chinese sortal classifiers:
- 位 wèi honorific humans; 只 zhı̄ animals; 张 zhāng flat shape; 所 suǒ build7ings; 条 tiáo long; 根 gēn long cylindrical; and 头 tóu—“head” classifier for pigs or livestock.
Container classifiers in Chinese typically “scoop up”, “shut in”, or “box up” mass noun referents like liquids, food, paper, seed and so on. However, for container classifiers there is no direct semantic relationship between the classifier and the ensuing noun, other than defining its borders and thus rendering them countable. The classifier therefore has an independent reference and does not overlap with the semantic field of the noun. Examples of container classifiers in Chinese are:
- 碗 wǎn bowl; 盘 pán plate; 杯 bēi cup; 瓶 píng bottle; 壶 hú kettle; 锅 guō cooking pot; 罐 guàn jar; 听 tı̄ng can; 盒 hé box; and 包 bāo packet.
Finally, the 个 gè default classifier in Chinese is both highly productive and applied with a heterogeneous set of nouns, including abstract nouns. Today, gè is by far the most used compared to sortal classifiers (Erbaugh, 1986, p. 406). Indeed, this classifier may be used even where a sortal classifier should be preferred and it often replaces sortal classifiers in preschool children as well as adult conversation. No single semantic content can be ascribed to it, although historically it was derived from a concrete meaning, “bamboo” (Erbaugh, 1986). The MDBG Free Online Chinese English Dictionary, ©2018 the Netherlands (https://www.mdbg.net) lists nouns that can be allocated to an ample variety of categories, such as (1) people/life attribute/emotions (author, friend, reporter, child, hero/way of life, a life, outer appearance, a person's age/laugh, feeling, experience), (2) locations (region, market, a home, a place, an altitude), (3) organized human activities/effects of such activities (union, association, administrative section, government, system, society, project, plan, old practice, habit, doctrine, policy, nationality/merchandise, price, a result, money), (4) mental concepts (purpose, aim, science, word, idea, opinion, secret), (5) time units (a date, a century, an afternoon, a period, an hour). However, the above lists do not exhaust the nouns classified by 个 gè and, most importantly, there seem to be no internal semantic coherence or motivation for semantic extensions between these subgroups of nouns.
Finally, there are no formal markers in the Chinese grammar that distinguish these classifier types. Thus, directed attention to the referent is bound to occur on semantic grounds.
1.3. The mutual influence of visual cues and linguistic interpretation
The present study puts to test the above hypothesized role of Chinese classifiers by use of the so-called “visual world paradigm” (Tanenhaus et al., 1995; Salverda and Michael, 2017; Porretta et al., 2018). Cooper's (1974) seminal study on visual attention and unfolding spoken language indicated that a “privileged” mental representation of the category member is mediated through semantic relatedness to the heard word. Huettig and Altmann (2005) showed that semantic relatedness between words can guide overt attention toward semantically related objects in the visual environment. For example, upon hearing the word “piano”, there were clearly more looks toward a line drawing of the piano than toward three semantically unrelated distractors, a goat, a carrot, and a hammer. Moreover, the direction of gaze was modulated by the semantic overlap between the word and target (e.g., toward a trumpet upon hearing the word “piano”). Supposedly, hearing the word “piano” activated semantic information that overlapped with the semantic representation of “trumpet”, which again triggered saccades toward the trumpet. Furthermore, in the condition where the piano and the trumpet were both present, there were less gaze movements away from the trumpet than from distractors upon hearing “piano”.
About a potential difference between the sortal vs. container classifiers, it is interesting that Huettig et al. (2006) found that conceptual overlap could be different from “associative relatedness”; i.e., associations based on contiguity, proximity, and containment (as in “boat” and “lake”). Participants directed overt attention toward a depicted object when a semantically related but not associatively related target word acoustically unfolded (Huettig and Altmann, 2005). Most interestingly, they point out that “increased attention directed to conceptually related items was proportional to the degree of conceptual overlap”. In other words, they surmise that semantic relatedness can be gradual and that this can be detected within the visual world paradigm.
Hence, it seems clear that the monitoring of eye movements can be used to observe the “online” mental processes underlying spoken language comprehension, since searching in a visual scene for items that semantically match a current linguistic expression appears to be part of the natural interpretation process in linguistic communication.
Our present goal is to explore the role of the classifiers' semantic relationships that are inherently conceptual, is-a relationships, as different from thematic associations that are the result of contiguity, has-a relationships. As part of this prediction, we surmise that the link between semantic interpretation and overt attention processes may be bidirectional; that is, anticipatory saccades would be directed at object properties hauled from semantic memory. A semantic relationship based on semantic overlap would therefore be stronger than one based on regularly co-occurring contiguity, because it would be stored within shared semantic fields in long-term, semantic memory. For example, in Cooper's (1974) study, the adjective “striped” referred to a temporary characteristic of the King's forehead in the phrase “his forehead was striped with wrinkles”, while listeners instead looked at the zebra, an entity whose stripes are essential characteristics to the concept “zebra”. Similarly, established semantic features in the classifiers could instigate a visual search, such as an object's overall shape, or the visual search could activate semantic properties in semantic memory of classifiers.
Indeed, linguists have hypothesized that classifiers function as reference trackers (see Löbel, 2000, about numeral classifiers in Vietnamese; Martins, 1994, for noun classifiers in Dâw; Heath, 1983, for Nunggubuyu; and Aikhenvald and Green, 1998, for verbal classifiers in Palikur). However, despite this general understanding of a basic function of classifiers, this assumption has not been put to empirical test.
On the basis of former linguistic research on classifier typology, general semantic research on conceptually overlapping vs. metonymic relationships and the methodology of the visual world paradigm (i.e. experiments that are based on both visual and auditory input, Huettig et al., 2011), we make the following predictions: that (1) Chinese numeral classifiers guide overt attention of the Chinese speakers to the classified reference objects; (2) The total amount of time fixating on the visual referent of the classifier-noun pair is longest for the trials with sortal classifiers, shorter for the container classifiers and shortest for the default classifier, indicating the time necessary for tracking the correct referent; and (3) The proportions of looks to the visual referent of the noun will increase fastest after the onset of the sortal classifiers, reflecting the facilitatory effect of the tight semantic relatedness, later for the container classifiers and latest for the default classifier.
2. Methods
We used naturalistic photographs, which allows a more realistic world set-up than most studies that used this paradigm. We monitored gaze fixations with eye tracking onto five different objects shown on screen. We computed how long listeners looked at each of them, while sentences containing linguistic stimuli in the form of numeral classifier phrases were heard through headphones. The objects belonged to either sortal, container, or default numeral classifiers in Chinese.
2.1. Participants
Twenty right-handed native speakers of Mandarin Chinese (mean age = 25.6; SD = 2.9; 14 females) participated in the experiment. They were recruited online through a website for Chinese students living in Oslo, Norway. In addition, 48 individuals with no knowledge of Chinese participated as a Control group (Non-Chinese speakers). The control group was matched on chronological age with the target group. All participants had normal vision and hearing by self-report and they provided informed consent for their voluntary participation in the study.
2.2. Auditory stimuli
We selected 10 Sortal and 10 Container classifiers that fit semantically into a set of template sentences that were neutral in content but meaningful after insertions of any of the classifier + noun phrases. Ten additional sentences were constructed using the Default classifier (Table 1).
Each of the classifiers was inserted into four different sentence templates, as exemplified below with the classifier 顶 dǐng for headwear, as in (3). The classifier phrase appears in bold types (see the Supplementary material for the complete set of sentences):
(3) 2. HAT, CL 顶 dǐng
(2A) 桌子 上 摆着 一 顶 帽子。
zhuōzi shang bǎizhe yì dǐng màozi
table on place a CLASS hat
“There is (lies/stands) a hat on the table.”
All sentences were read by a native female speaker of Mandarin Chinese and pre-recorded by use of the open-source audio software Audacity (www.audacityteam.org).
2.3. Visual stimuli
The visual stimuli in the experiment included photographs of the real-world objects. Sixteen color photographs were taken at the same distance and viewpoint with a Pentax K100 digital camera of the sets of various small objects layed out on a white table surface for maximum visibility. Each picture contained five objects, two that matched the nouns preceded by Sortal classifiers, two by Container classifiers and one by the Default classifier as a control (Figure 1). In each trial one of these objects (the Target) corresponded to the noun from the stimulus sentence currently heard. Each of the 16 photographs was shown together with six different sentences (two sentences with a Sortal classifier-noun pair, two sentences with a Container classifier-noun pair and two sentences with the Default classifier-noun pair), which resulted in 96 trials in the experiment. The objects used for referencing the nouns' meanings in each classifier type are listed in Table 2. The actual Chinese nouns are listed in Supplementary material.
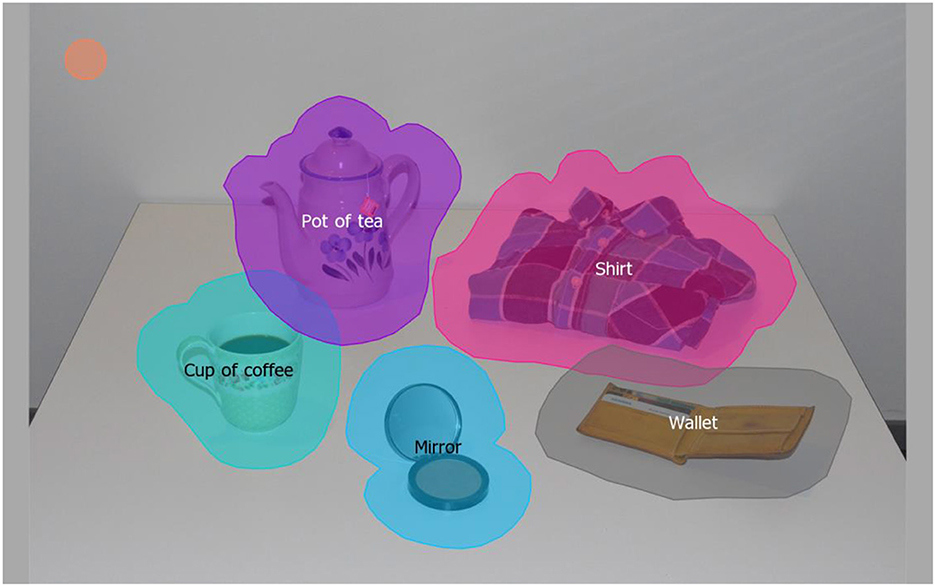
Figure 1. An example of the visual stimulus with overlayed colored Areas of Interest (AOIs; Nota Bene: these areas were not visible to the participants during the task). The AOIs extended from the boundaries of the object to around 2 degrees of visual angle, which corresponded to the size of the fovea (see the orange circle in the left upper corner representing the size of the fovea).
2.4. Apparatus
Eye monitoring was obtained with an iView Remote Eye-Tracking Device (R.E.D.) from Senso-Motoric Instruments (SMI, Berlin, Germany) set at a sampling rate of 120 Hz; iView 3.2®. The SMI Experiment Center software was used for data collection and stimulus presentation. R.E.D. has an automatic compensation for head movements at a 70 cm distance and in a range of 40 × 20 cm; nevertheless, a chinrest was used to keep the participant's head as stable as possible. The distance of the screen from participants' cornea when the head was placed in the chinrest was 55 cm. The light condition in the room was kept constant throughout the experiment. The visual stimuli were shown on a color, flat Dell LCD monitor. The size of the monitor was 47 cm. The resolution of the screen was set to 1,680 × 1,050 pixels. Sentence stimuli were played through headphones at a stable volume across all testing sessions.
2.5. Procedure
Participants were seated in front of the monitor screen and a standard 5-points eye-tracking calibration procedure was conducted. After successful calibration, the instructions were viewed on the screen. Participants were told to carefully read the instructions and press the space bar when they were ready to start the experiment. Each trial consisted of a blank screen (1,000 ms), a fixation cross and a visual stimulus, i.e., an image of five objects. The image was accompanied by an auditorily presented sentence in Chinese (Figure 2). Participants' task was to look at the images of objects while listening to the sentences. They were instructed to keep always looking at the screen while listening to the sentences; however, they were not told in the instructions that they had to look at the object mentioned in each of the sentences.
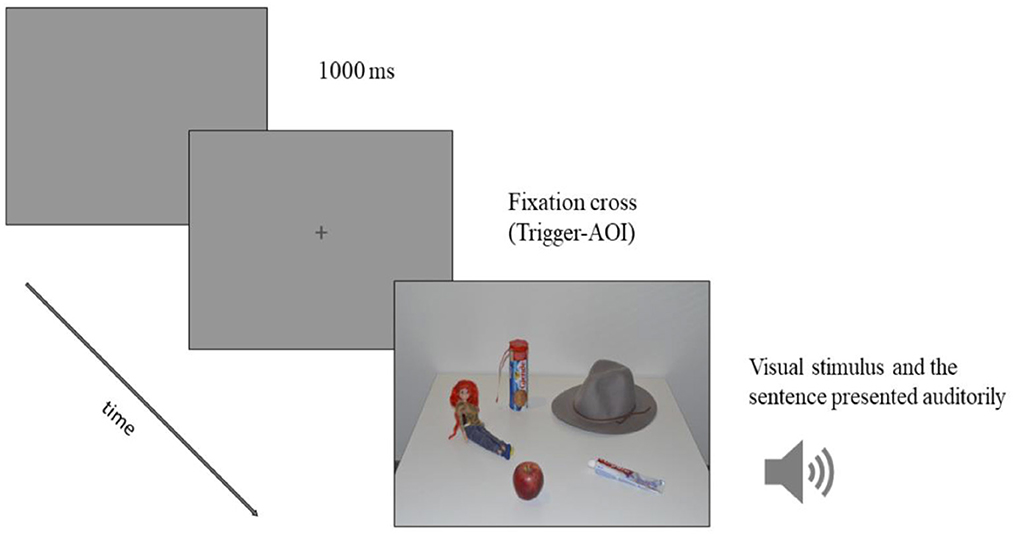
Figure 2. The timeline of the trial: first, a blank screen (1,000 ms), followed by a fixation cross with a trigger Area of Interest, that initiated the presentation of the stimulus when a participant's gaze fixated on the cross for 500 ms. When the visual stimulus appeared, the auditory stimulus was played out (i.e., a sentence with the classifier-noun pair corresponding to only one of the objects).
3. Results
We first compared participants' dwell times in the Target and Other (Non-Target) Areas of Interest (AOIs) throughout the whole stimulus presentation. Dwell times were defined as the total amount of time (in milliseconds) fixating within an AOI in each trial. We ran a 2 × 2 ANOVA with AOI (Target, Other) as within-subject factor and LanguageGroup (Chinese, Non-Chinese) as between-subjects factor. The analysis revealed a significant main effect of AOI, F(1, 66) = 142.6, p < 0.001, = 0.68, as well as a significant effect of LanguageGroup, F(1, 66) =38.9, p < 0.001, = 0.67, and a significant interaction of AOI * LanguageGroup, F(1, 66) = 132.5, p < 0.001, = 0.37.
The post-hoc comparisons (with Bonferroni correction) revealed a significant difference between the dwell times in Target AOI and Other AOI in the Chinese speakers, t(1, 19) = 7.76, p < 0.001, but not in the non-Chinese speakers, t(1, 47) = 1.27, p = 0.208 (Figure 3), suggesting that, as expected, Chinese speakers were looking overall more to the relevant objects mentioned in the sentences, while Non-Chinese speakers looked at the Target and Other AOIs for a similar amount of time. This shows that, without being explicitly instructed to do so, Chinese speakers looked at the relevant referents of the classifier-noun pairs mentioned in the sentences.
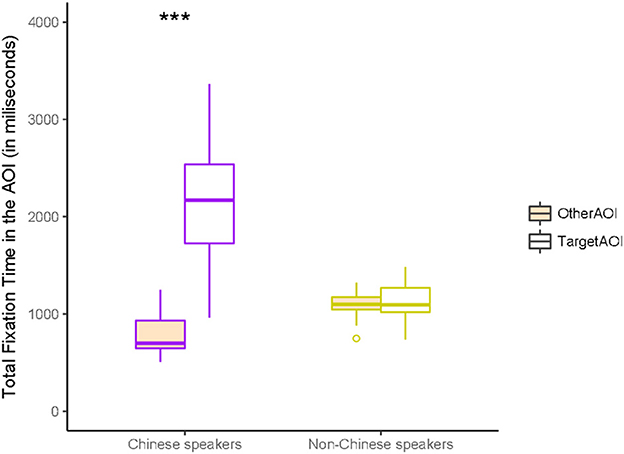
Figure 3. The graphs show differences in fixation times between target-AOI vs. Other-AOI in the Chinese group and controls (“non-Chinese speakers”). X-axis represents the LanguageGroup and y-axis represents the total fixation times within AOIs in milliseconds. ***p < 0.001.
Next, we examined the total time spent by the two language groups looking at the Target AOIs throughout the stimulus presentation, depending on which type of classifier was heard. We ran a 3 × 2 ANOVA on the average dwell times in the AOIs with Classifier (Sortal, Container, and Default) as a within-subject factor and LanguageGroup (Chinese, Non-Chinese) as a between-subjects factor. There was a significant effect of LanguageGroup, F(1, 66) = 88.11, p < 0.001, = 0.57, with Chinese speakers showing longer fixation times to target AOIs than control participants. We observed also a significant Classifier * LanguageGroup interaction F(2, 66) = 10.0, p < 0.001, = 0.13.
Post-hoc multiple comparisons (with Bonferroni corrections) showed that the Chinese speakers fixated overall longer on the Target AOIs in Sortal trials compared to the Default trials, t(1, 19) = 2, 969, p =0.024, and in Container trials compared to the Default trials, t(1, 19) = 2,662, p =0.045. On the contrary, the Non-Chinese speakers looked at the Target AOIs longer in the Default trials compared to the Container trials, t(1, 19) = 2,559, p =0.042 (Figure 4). No other comparisons reached significance (all p > 0.07).
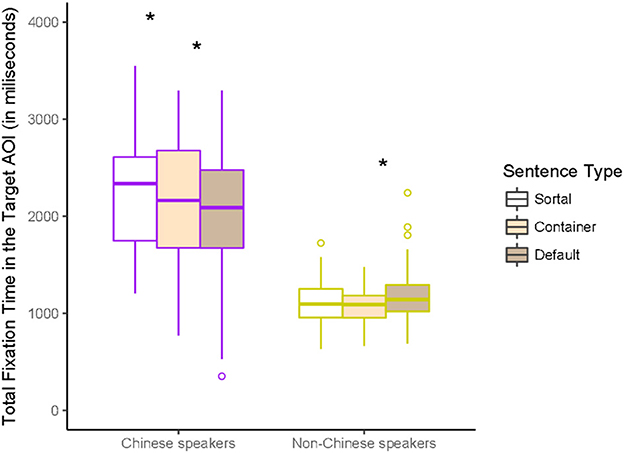
Figure 4. Dwell times in TargetAOIs in the trials with sentences containing sortal, container or default classifiers separately for the Chinese speakers and non-Chinese Speakers. The X-axis represents the LanguageGroup and the y-axis represents the total fixation time in the Target AOI throughout the stimulus presentation. *p ≤ 0.05.
Finally, we investigated when these differences in the fixation times in different Conditions emerged as the sentence was unfolding. For this purpose, we calculated the time frames for the classifiers and nouns in each of the sentences. The time frame was defined as the time (in miliseconds) from the onset to the offset of the classifier or the noun. The Audacity audio-software was used for identifying the onset and the offset of the classifiers and the nouns based on the sentences' waveforms.
In 13 out of the 120 sentences (10.8%) there was a double articulation in the transition from one phoneme to another (for details, see Supplementary material). In such cases, the time point of the disclosure of the target noun identity was not analyzed as part of the classifier time window; instead, we assigned phonetic characteristics that could identify the initial phoneme of the target noun to the noun's time frame.
We compared the average length of the calculated time frames for the Sortal, Container and Default trials (see mean lengths of the classifiers and the nouns in Table 3). We first ran a one-way ANOVA with Condition (Sortal, Container, Default) as a main factor on the classifier time frames (in miliseconds). The analysis revealed a significant effect of the Condition, F(2, 113) = 3.45, p = 0.035 (Figure 5). Post-hoc comparisons (with Bonferroni correction) revealed that the time frames for the Default classifier (M = 335.6, SD = 64.4) were on average shorter than the time frames for the Container classifier (M = 371.6, SD = 58.5). No other comparisons reached significance (all p > 0.57). Second, we ran a similar one-way ANOVA with Condition (Sortal, Container, Default) as a main factor on the noun time frames (in miliseconds). We again observed a main effect of Condition, F(2, 113) = 23.17, p < 0.001 (Figure 6). Post-hoc comparisons (with Bonferroni corrections) showed that the time frames for the nouns preceded by Sortal classifiers (M = 598.7, SD = 182.8) were significantly shorter than those preceded by Container classifiers (M = 714.4, SD = 158.9) and Default Classifiers (M = 882.14, SD = 203.9); in addition, the noun time frames preceded by Container classifiers were signifincantly shorter compared to those preceded by the Default classifiers (all p < 0.016). These variations in length could not be avoided during stimuli construction because semantic and grammatical compatibility were the primary criteria; however potential differences that arise from this variation in the length will be considered in the Discussion.
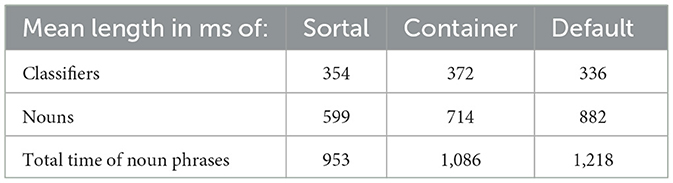
Table 3. Mean length of classifiers and nouns measured in ms from onset of classifier (sortal, container and default).
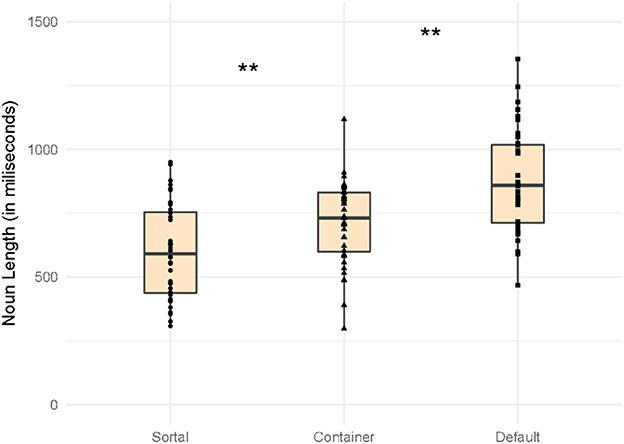
Figure 6. Mean length in ms of nouns classified by sortal, container and default classifiers. **p ≤ 0.01.
Next, we looked at the cumulative fixation hits to the Target AOIs from the onset of the classifier over time, as we predicted that overt attention would be guided toward the Target noun objects in the Sortal earlier than any other object type. We calculated the proportion of the fixations to the Target AOI from the Classifier onset over fifteen 100 millisecond time windows per participant per trial. We ran a 15 × 3 × 2 ANOVA with TimeWindow (the 15 separate time windows) and Condition (Sortal, Container, Default) as within-subject factor and LanguageGroup (Chinese, Non-Chinese) as between-subject factor. We observed main effects of TimeWindow, F(2, 66) = 541.99, p < 0.001, = 0.89, Condition, F(2, 66) = 24.36, p < 0.001, = 0.27, and LanguageGroup, F(2, 66) = 83.00, p < 0.001, = 0.56. Most importantly, we also observed a significant TimeWindow * Condition * LanguageGroup interaction, F(2, 66) = 29.17, p < 0.001, = 0.31. Post-hoc comparisons (with Bonferroni corrections) showed that the proportion of the fixation hits to the Target AOIs started to diverge between the Chinese and Non-Chinese speakers at 500 ms from the classifier onset in the Sortal trials (p = 0.045; Figure 7), at 600 ms in the Container trials (p = 0.045; Figure 8) and at 1,100 ms in the Default trials (p = 0.015; Figure 9). This suggests that, in accordance with our predictions, the Chinese speakers looked at the target objects in the picture fastest after hearing the Sortal classifier, and 100 ms slower (on average) after hearing the Container classifier when compared to the baseline cumulative fixations to the Target AOI from the Control group. The Chinese speakers took longest time to move their gaze to the target object in the picture after hearing the Default classifier.
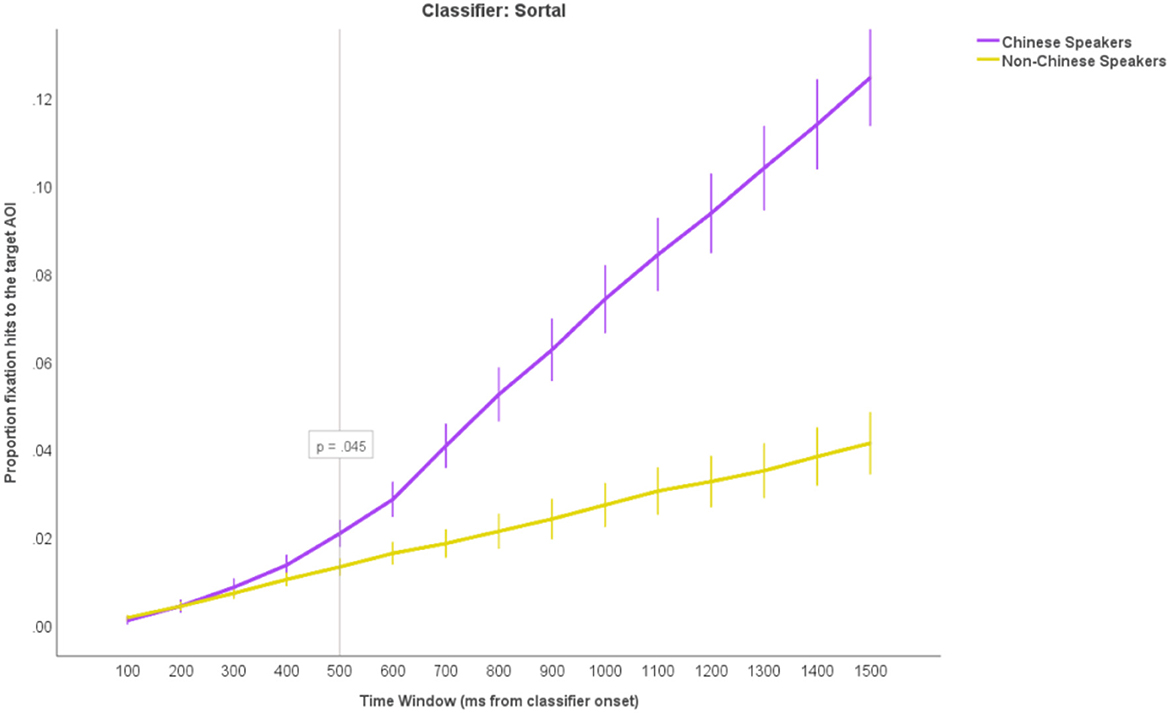
Figure 7. The cumulative proportions of fixation hits to the Target AOIs in fifteen 100-millisecond time windows from the classifier onset in the Sortal trials. The purple upper line represents the proportion of fixation hits in the Chinese group and the lower yellow line represents the controls (non-Chinese speakers). The reference line tagged with a p-value shows at what time point the Chinese start to diverge significantly from Controls. The error bars represent +/−2 SEM.
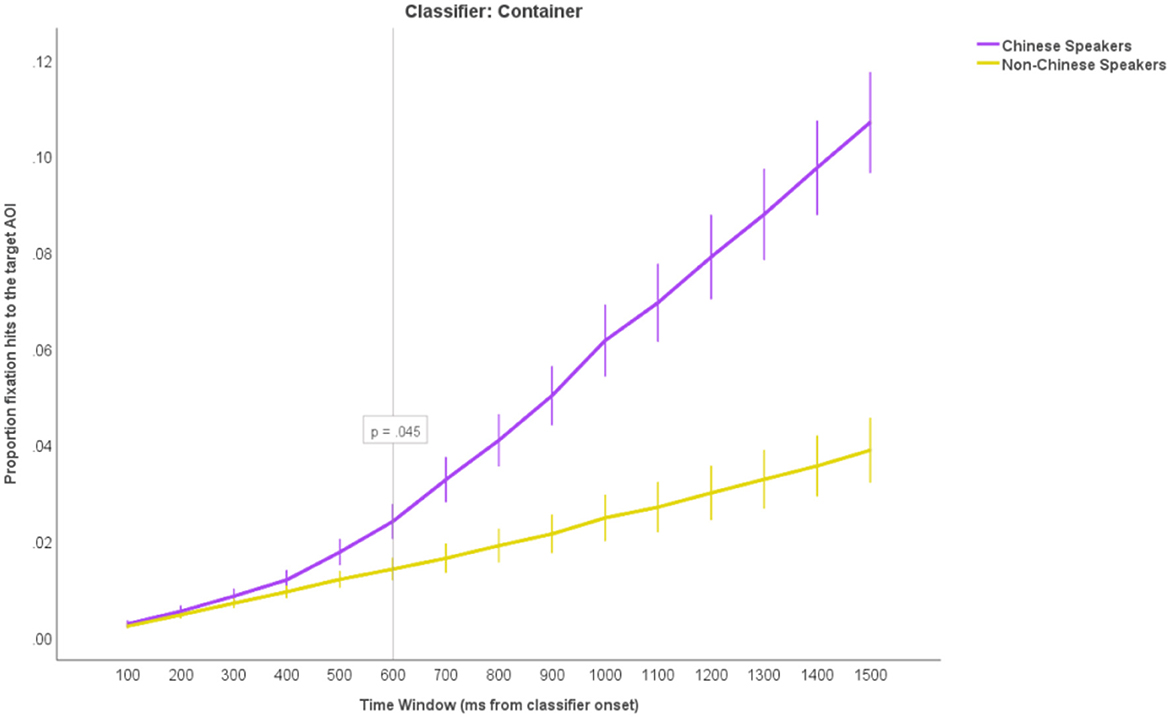
Figure 8. The cumulative proportions of fixation hits to the Target AOIs in fifteen 100-millisecond time windows from the classifier onset in the Container trials. The purple upper line represents the proportion of fixation hits in the Chinese group and the lower yellow line represents the controls (non-Chinese speakers). The reference line tagged with a p-value shows at what time point the Chinese start to diverge significantly from Controls. The error bars represent +/−2 SEM.
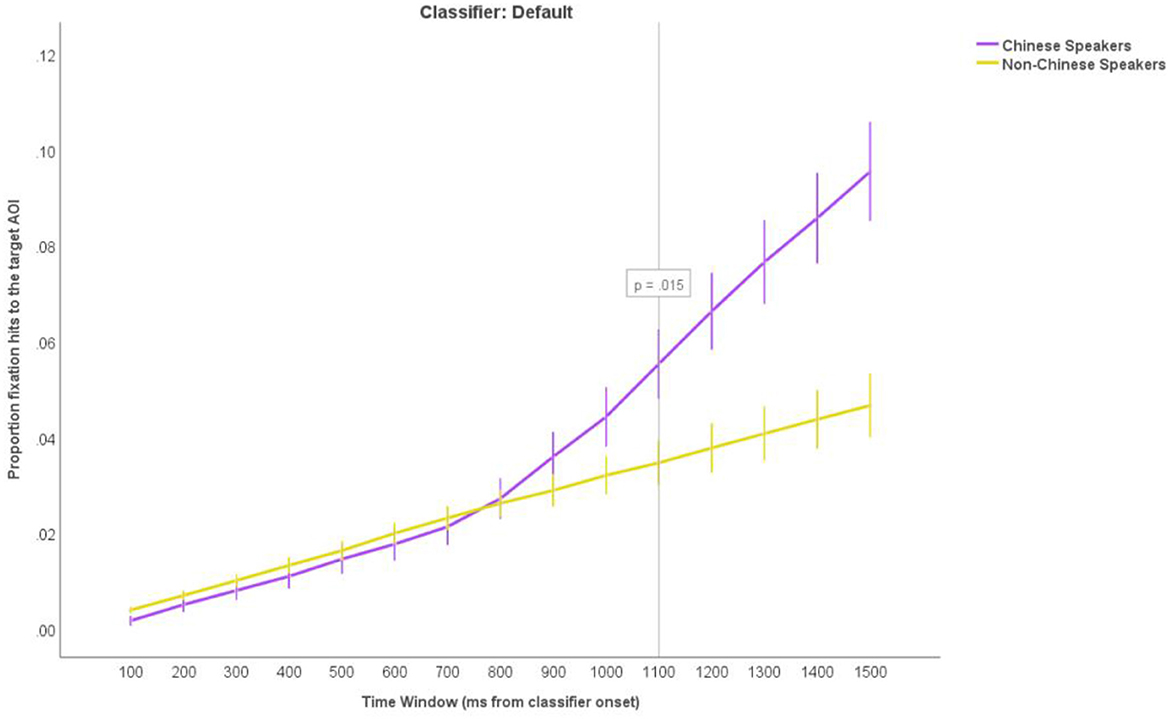
Figure 9. The cumulative proportions of fixation hits to the Target AOIs in fifteen 100-millisecond time windows from the classifier onset in the Default trials. The purple upper line represents the proportion of fixation hits in the Chinese group and the lower yellow line represents the controls (non-Chinese speakers). The reference line tagged with a p-value shows at what time point the Chinese start to diverge significantly from Controls. The error bars represent +/−2 SEM.
4. Discussion
In the present study, we monitored gaze behavior that was mediated by the linguistic devices in Chinese corresponding to classifiers. The findings supported our hypothesis that classifiers guided efficiently the eye-movements of the Chinese speakers to the relevant referents in the visual scene. As predicted, Chinese speakers behaved very differently from controls in terms of oculomotor behaviors toward the Target AOI vs. to Other AOIs, also depending on the classifier types.
Firstly, since the Chinese speakers looked significantly longer at Target AOIs than at Other-AOIs than controls, this suggests that the classifier-noun pairs indeed visually guided Chinese speakers to the reference object as a function of the language they spoke, without being explicitly instructed to do so. Since this behavior was absent in the control participants (who did not understand Chinese), we can rule out that non-linguistic artifacts in the visual stimuli would have caused these behaviors. Morever, the controls' gaze behavior could be used as a baseline to compare measurements with the Chinese participants.
Secondly, we predicted that classifier types would cause specific differences in dwell times, i.e., the time spent looking at the Target objects. Within-subject comparisons confirmed that the Chinese speakers looked significantly longer at the sortal than the container target AOIs as well as at the container than the default target AOIs, thus revealing a stepwise relationship between semantic overlap to nouns and looking times. We also observed a difference between the dwell times in the default and container trials in the control group. However, there these differences pointed in the opposite direction, with longest dwell times to the default target AOIs. Again, this excludes the possibility that other factors, such as visually salient features, guided fixations.
Dwell times, or the percentage of time a viewer spends looking at a specific AOI (Holmqvist et al., 2011), provide other interesting implications for cognitive processing (Mahanama et al., 2022). One is that it correlates with successful object selection success rate and fewer object selection corrections (Paulus and Remijn, 2021). In other words, when participants look longer at something, this is an indication that they are certain of their choice. Longer dwell times also indicate higher as opposed to lower information processing (Tullis and Albert, 2013). Thus, since dwell time may indicate higher informativeness, this can be used as an index of the amount of meaningful information that the visual token carries for the viewer (Suvorov, 2015). This is compatible with findings that longer dwell times correlate with motivation and level interest in the AOI (Fisher et al., 2017). Furthermore, long dwell times testify to the level of top-down attention, i.e., the attention driven by what the participant already knows. Language, and specifically the semantics of classifier phrases, represents one kind of knowledge that can drive top-down attention (Baluch and Itti, 2011; Chen et al., 2021). Finally, we think the relevance of dwell times is particularly apparent in a Visual World Paradigm experiment, since they correlate with situational awareness (Hauland and Duijm, 2002), and indicate that participants refrain from looking at contextually irrelevant stimuli (Mohanty and Sussman, 2013). In sum, dwell times on the object (referent) may reveal what the listener is thinking of and understands.
Finally, sortal, container and default classifiers differed with respect to the time point, after classifier onset, in which the frequency of fixations to Target AOIs diverged significantly in the Chinese speakers' group from the controls. Chinese speakers directed significantly more looks to the Target AOI the earliest in the Sortal classifier condition at 500 ms after classifier onset; later in the Container condition at 600 ms, and the latest in the default condition at 1,100 after classifier onset.
Using the visual world paradigm with naturalistic photographs, we aimed to show that a cognitive divide exists between different classifier types. On the one hand, sortal classifiers semantically overlap with their congruent nouns; on the other hand, a mensural classifier type called container is thematically but not intrinsically attached to the semantics of the nouns. Additionally, we expected that the default classifier would trigger a spontaneous visual search while also guiding gaze less efficiently to a referent object, due to semantic unrelatedness between classifier and noun.
Of particular interest to our study was the hypothesis that “increased attention afforded to conceptually related items is proportional to the degree of conceptual overlap”, as originally proposed by Huettig and Altmann (2005). Given the assumption that “language-mediated eye movements are a sensitive measure” to establish such a cognitive relationship between world and language (Tanenhaus et al., 1995; Spivey et al., 2002; Huettig and Altmann, 2005), we monitored the moment-by-moment changes in participants' gaze behavior while listening to sentences with either Sortal, Container or Default classifiers.
The average length of the classifiers and nouns in each condition was essential to how these results could be interpreted. However, due to the speed at which the brain processes semantic knowledge, the actual time point when semantics is comprehended may not be equivalent to the number of milliseconds calculated from classifier onset to offset.
Recent neurolinguistic research suggest that there might be a difference in the speed at which “coarse” as opposed to “detailed” semantic information is processed in the brain in the anterior temporal lobe (ATL), a brain region believed by many to be a semantic processing center or “hub” (e.g., Ralph et al., 2017). Specifically, domain-level semantic distinctions may be available earlier than detailed semantic information. Whereas coarse semantic distinctions are reported accessible at 120 ms post stimulus onset, finer semantics are activated only from 250 ms post stimulus onset (Ralph et al., 2017), although some researchers report 150 ms as the earliest time point for discriminating coarse-grained, category-level information, such as knowing that an object is an animal or a vehicle (VanRullen and Thorpe, 2001; Kirchner and Thorpe, 2006; Liu et al., 2009). This difference in semantic processing of an earlier time window for coarse as opposed to a later time window for fine-grained semantics is supported by several neuroscientific studies (e.g., VanRullen and Thorpe, 2001; Kirchner and Thorpe, 2006; Martinovic et al., 2007; Liu et al., 2009; Crouzet et al., 2010; Clarke et al., 2011, 2012; Jackson et al., 2015). In general, category-sensitive responses to visually presented images from superordinate categories tend to appear pre-200 ms poststimulus onset and fine-grained effects appear at 200–300 ms (Clarke et al., 2012). This difference in coarse vs. detailed information processing parallels semantic differences in classifier and noun semantics. Typically, classifier systems have classifiers for animals and vehicles and other items at the domain-general level (Aikhenvald, 2000), as for Chinese. While classifier semantics is schematic, in the sense that it's meaning can be described in terms of the relatively few semantic features that all or most of the nouns it classifies have in common, the nouns they classify embellish rich, distinctive, and fine-grained semantics with specific individual associations and usages; especially when a classifier is semantically structured around a semantic core (radial categories; Lakoff, 1986).
Based on the above evidence we suggest the following interpretation of the effects that the different classifier types had on the timing of gaze behavior. All classifiers were fully pronounced between 300 and 400 ms post stimuli onset. Thus, processing of the default classifier was completed significantly earlier than the two other classifier types (at 336 ms). The latency from the completion of the default classifier to the time point when Chinese diverge from controls was 764 ms. The corresponding duration for the sortal classifier was 146 ms, and for the container classifier 228 ms. This means that the sortal classifier may be within the earliest limit for coarse semantic processing at 150 ms and the container may be slightly outside the more lenient pre-200 ms window. The default classifier, by contrast, diverged significantly only past the time estimated even for detailed semantic knowledge, which occurs at 400 ms. This suggests that for the default classifier, additional information about noun phonology was necessary to hit at the referenced object.
One conclusion to draw from the above is that the semantic content of sortal classifiers facilitates directing the Chinese speakers' gaze toward the classified object. The container classifier likewise influences the direction of the speakers' gaze; however, this takes place about 100 ms later than the sortal classifiers, as estimated from averaged classifier lengths. This time point is slightly outside the time estimated for early coarse semantic processing, which might indicate that container classifiers are less tied to the nouns they classify.
The default classifier by contrast, did not contribute to guiding attention. This may depend on the phonological cues of the nouns. Although nouns classified by the default classifier were not fully pronounced by the time of significant divergence, they nearly doubled the time necessary for processing detailed semantic information and phonological clues would be sufficient to choose between alternate distractors and targets. If the default classifier played a role in directing attention, we should have seen a divergence at least between 536 and 636, which allows 200–300 ms for coarse semantic processing after the default classifier was fully pronounced. However, such a threshold was never approached. Note also that the default nouns took considerably longer to pronounce and allowed for more time to differentiate between targets as the auditory stimulus unfolds.
Keeping in mind that the mean durations of the sortal classifiers were not shorter than that of the container classifiers, we are led to conclude that sortal classifiers, arriving at a significant difference 100 ms earlier than the container classifiers, are the least semantically ambiguous and hence the most efficient reference trackers. Most remarkably, although the default classifiers were significantly shorter than the container and sortal classifiers, gazes were not directed toward default objects until 500 ms later than the container phrases, at 1,100 ms.
Looking at these results from a linguistic point of view, several functions of classifiers could hint at their attention-guiding role. At a first glance, what may seem puzzling from a cognitive perspective is the non-economical nature of classifiers, given that they either convey eminently redundant information or even no sematic information at all. The apparently spurious role of these proliferate linguistic devices is surprising considering how frequently they appear in world's languages and how important it is to spare cognitive resources. In fact, there exist worldwide at least five hundred classifier languages (Vajda, 2002), some spoken by very large populations of speakers (e.g., Chinese or Japanese), that require classifiers.
Despite their apparently non-economical role, the present findings show that numeral classifiers and, possibly other noun classification devices, might serve basic cognitive functions. A prominent function, we propose, is being controlling the listeners' attention to the referent of an unfolding linguistic expression. As some linguists have already proposed, classifiers possess a reference tracking function and a more general deictic function (seen when classifiers develop into anaphora), thus binding larger texts via memory traces retrieved from semantic memory, as well as a construal function.
Classifiers are used as deixis in communication. When someone runs away with a bag of peanuts and one wants to direct bystanders' attention, shouting 挡住那只猴子! Dǎngzhù nà zhı̄ hóuzi! “Stop that animal monkey!” instead of 挡住那个扒手! Dǎngzhù nà gè páshǒu! “Stop that (default classifier) thief!” is far more efficient when it comes to catching the culprit. Second, sortal classifiers serve as pre-established memory anchors, in a top-down function. When searching for a specific term that one has temporarily forgotten, the retrieval of some fragments of the memory trace may render more likely to recall the broader category than the specific item. Consider Gurr-goni (Australia), where a speaker tries to remember a plant name and uses the class 3 prefix mu- for the “vegetable food” class (which also contains plant names) with the indefinite/interrogative (Aikhenvald, 2000: 55).
(4) mu-njatbu muwu-me-nji awurr-ni-Ø
3.CLASS.3-whatsit 3AUG.A.3CL3:O-get-PRECONT 3AUGS-be-PRECONT
“What's that CLASS.3 thing (vegetable food) they're getting?”
Third, salience and construal reflect attentive processes. If the communicative purpose is to single out an object from several other objects, any property that is both salient and unique will do. Referents that are not considered salient may even be left unclassified (Daley, 1996, p. 136; Aikhenvald, 2000, p. 334). Situational as well as established uses of classifiers invoke the listeners' attention. Often, a speaker can choose between several congruent classifiers to characterize a noun, in which case there is an opportunity of highlighting certain characteristics that call on the speaker's attention in certain situations, cf. example (5) from Chinese,
(5) The noun 总统 zǒngtǒng “president (of a country)”: (a) 一个总统 Yı̄ ge zǒngtǒng (neutral, uses the regular classifier for people); (b) 一名总统 Yı̄ míng zǒngtǒng (emphasizes the profession/status of a person with classifier 名 míng); (c) 一位总统 Yı̄ wèi zǒngtǒng (emphasizes the honorific status of humans with classifier 位 wèi); (d) 一届总统 Yı̄ jiè zǒngtǒng (emphasizes the president's public office by using a classifier for events, meetings, elections).
Construal is used to draw attention to semantic nuances, so that classifiers can highlight selected semantic properties of the same noun. The allowed variation in classifier use rests on the semantic link between a sortal classifier and its noun, but even in creative usages the classifier serves as an attention catcher.
A parallel deployment to attention in communication is observed for sortal classifiers in improving textual clarity and coherence in written texts. Classifiers may develop functions that resemble those of pronouns in non-classifier languages, e.g., the anaphoric use. Anaphors are proforms that refer to any contextual entity; they can be demonstratives (that, this), adverbs (such, so), or typically, pronouns. Pronouns point back to a noun that has been introduced in a text or discourse. According to Aikhenvald (2000, p. 329), all kinds of classifiers can occur as anaphoric and participant-tracing devices. Note that these functions are widespread geographically as well as across nominal classification devices. Although anaphoric use is not found in Chinese, these functions are closely related and deserve mention. Anaphoric use of numeral classifiers is documented in Japanese (Downing, 1986), Burmese (Becker, 1975), Vietnamese (Daley, 1996), and Malay (Hopper, 1986). Noun class markers used as participant trackers are widespread in Papuan and Australian languages, for example Ungarinjin (Rumsey, 1982, p. 37), in Yimas (Foley, 1986, p. 88) and Dyirbal (Dixon, 1982, p. 71). Anaphoric classifiers are especially useful when long intervals between the antecedent nouns and subsequent mentions of the referent occur, or if other personas intervene and cause potential ambiguity as to which referent is intended (Downing, 1986). However, while smaller pronoun systems such as the one that one finds in English only carry three semantic distinctions (he, she, it), the classifier systems can potentially distinguish between dozens of semantic classes. This provides a resource of high semantic specificity.
By contrast, mensural classifiers like the container classifier in Chinese are used primarily as unitizers. While a speaker's choice of sortal classifiers is determined by the inherent and time-stable semantic characteristics of the nouns, mensural classifiers are selected based on temporary states, as are a certain arrangement and measured quantities. Thus, mensural classifiers differ from sortal classifiers in their semantics (Aikhenvald, 2000, p. 115). As a consequence, there is freedom in choice of classifiers available for each noun (Berlin, 1968, p. 175; Aikhenvald, 2000, p. 115). For example, in the Chinese noun for “water”, 水 shuǐ with seven possible mensural classifiers.
(3) 水 shuǐ ‘water': 杯 bēi ‘cup/glass', 瓶 píng ‘bottle', 壶 hú ‘pot', 桶 tǒng ‘bucket', 罐 guàn ‘can', 滩 tān ‘puddle', 滴 dı̄ ‘drop' etc.
Clearly, such freedom weakens the association between noun and classifier by virtue of less stable combinations in the everyday use of classifiers and a frailer semantic bonding to the nouns. In other classifier languages than Chinese, mensural classifiers can be grammatically and semantically more detached from the nouns they classify. Whereas sortal classifiers commonly use animacy (i.e., a time-stable quality) as a semantic basis, very few languages use animacy as a semantic basis in mensural classifiers (Aikhenvald, 2000, p. 293).
To conclude, although from a grammatical perspective, classifiers may seem utterly redundant, they appear to be functional by providing an efficient visual guidance in conversation. It is unlikely that semantics per se can guide attention efficiently as the simpler visual features are able to (Wolfe and Horowitz, 2004; see also Hagen and Laeng, 2016). Yet, it is plausible to suggest that once a semantic domain is selected, this would narrow down the number of pertinent perceptual attributes that apply to the domain and, therefore, optimize the windowing of attention onto the target noun's referent. The ability of classifiers in drawing attention to the correct objects appears to depend on the type of relationship that exists between classifier and nouns. Thus, semantic overlap gives the clearest clue to the top-down mechanisms selecting attributes for visual attention. That is, a pragmatic relationship based on worldly knowledge of how substances can be contained may also offer a fair lead. Finally, we saw that the unspecified content of the default classifier moderated the participants' gaze toward the target objects only long after the noun's pronunciation had been initiated.
Despite the internal differences highlighted here, it should be stressed that classifiers form a coherent grammatical class that differs from related grammatical devices like quantifiers (Aikhenvald, 2000, p. 115–120). In Chinese there are no formal grammatical differences between sortal and mensural classifiers, which support the idea that these types be basically one category, with at best a gradient distinction between the two suptypes. Nevertheless, our results are consistent with a semantic distinction between the two. This seems to be mirrored in the Chinese speakers' minds, as signaled by the gaze behavior of the Chinese speakers in the present study.
5. Conclusion
In this study we investigated how Chinese numeral classifiers play a relevant role in guiding visual attention and assisting the online comprehension of utterances. We discovered that the greater the semantic relatedness was between classifier and congruent noun, the more efficiently the gaze of Chinese speakers was directed to the referenced objects. This facilitation played out as earlier as well as longer looking times toward classified objects that were more semantically related to their classifier, in an incremental fashion. Compared to controls who did not understand Chinese, the Chinese speakers revealed a behavior consistent with our predictions on classifier-noun semantic relationship. Referents of nouns following the sortal classifiers, where classifier-noun semantic fields overlap, were targeted the earliest and looked at the longest. In contrast, the referents of nouns following the container classifiers were looked at later than the sortal classifiers. Finally, the referents of default classifier had the shortest looking times and directed the Chinese speakers to the referenced objects the latest. Hence, sortal classifiers are likely processed in an early time window, whereas container classifiers are processed at a slightly later window of processing for coarse categorical information.
Data availability statement
The raw data supporting the conclusions of this article will be made available by the authors, without undue reservation.
Ethics statement
The studies involving humans were approved by NSD—Norsk Senter for Forskningsdata. The studies were conducted in accordance with the local legislation and institutional requirements. The participants provided their written informed consent to participate in this study. No potentially identifiable images or data are presented in this study.
Author contributions
ML, BL, and AB conceived the idea and use of method, planned the experimental design, and took and prepared the photographs for the experiment. HE made the Chinese stimuli and was in charge of Chinese-specific matters. AB analyzed the data supported by BL. AB made the figures. ML wrote up the manuscript. All authors contributed to the article and approved the submitted version.
Funding
The study received funding from the Norwegian Research Council, grant number 222415/F10.
Acknowledgments
We wish to thank Xiuyi Dong who conscientiously read the stimuli onto sound files for us as well as to our research assistant, Fredrik Svartdal Færevåg, for collecting these data.
Conflict of interest
The authors declare that the research was conducted in the absence of any commercial or financial relationships that could be construed as a potential conflict of interest.
Publisher's note
All claims expressed in this article are solely those of the authors and do not necessarily represent those of their affiliated organizations, or those of the publisher, the editors and the reviewers. Any product that may be evaluated in this article, or claim that may be made by its manufacturer, is not guaranteed or endorsed by the publisher.
Supplementary material
The Supplementary Material for this article can be found online at: https://www.frontiersin.org/articles/10.3389/flang.2023.1222982/full#supplementary-material
References
Aikhenvald, A. Y. (2000). Classifiers: A typology of Noun Categorization Devices: A Typology of Noun Categorization Devices. Oxford: Oxford University Press Oxford.
Aikhenvald, A. Y. (2021). One of a kind: on the utility of specific classifiers. Cognit. Semant. 7, 232–257. doi: 10.1163/23526416-07020001
Aikhenvald, A. Y., and Green, D. (1998). Palikur and the typology of classifiers. Anthropol. Linguist. 40: 429–480.
Altmann, G. T., and Kamide, Y. (2007). The real-time mediation of visual attention by language and world knowledge: linking anticipatory (and other) eye movements to linguistic processing. J. Mem. Lang. 57, 502–518. doi: 10.1016/j.jml.2006.12.004
Baluch, F., and Itti, L. (2011). Mechanisms of top-down attention. Trends Neurosci. 34, 210–224. doi: 10.1016/j.tins.2011.02.003
Becker, A. J. (1975). A linguistic image of nature: the Burmese numerative classifier system. Linguistics 165, 109–121 doi: 10.1515/ling.1975.13.165.109
Berlin, B. (1968). Tzeltal Numeral Classifiers: A Study in Ethnographic Semantics. The Hague: Mouton. doi: 10.1515/9783111584232
Chen, Y., Lu, W., Mottini, A., Li, L. E., Droppo, J., Du, Z., et al. (2021). “Top-down attention in end-to-end spoken language understanding,” in ICASSP 2021-2021 IEEE International Conference on Acoustics, Speech and Signal Processing (ICASSP). Toronto: IEEE, 6199–6203. doi: 10.1109/ICASSP39728.2021.9414313
Cheung, C. C. H. (2016). “Chinese: Parts of speech,” in The Routledge Encyclopedia of the Chinese Language, 242–294.
Clarke, A., Taylor, K. I., Devereux, B., Randall, B., and Tyler, L. K. (2012). From perception to conception: how meaningful objects are processed over time. Cerebral Cortex. 23, 187–197. doi: 10.1093/cercor/bhs002
Clarke, A., Taylor, K. I., and Tyler, L. K. (2011). The evolution of meaning: spatio-temporal dynamics of visual object recognition. J. Cognitive Neurosci. 23, 1887–1899. doi: 10.1162/jocn.2010.21544
Conklin, N. F. (1981). The Semantics and Syntax of Numeral Classification in Tai and Austronesian. Clarivate: ProQUest.
Cooper, R. M. (1974). The control of eye fixation by the meaning of spoken language: a new methodology for the real-time investigation of speech perception, memory, and language processing. Cogn. Psychol. 6, 84–107. doi: 10.1016/0010-0285(74)90005-X
Crouzet, S. M., Kirchner, H., and Thorpe, S. J. (2010). Fast saccades toward faces: face detection in just 100 ms. J. Vis. 10, 16–16. doi: 10.1167/10.4.16
Daley, K. A. C. (1996). The Use of Classifiers in Vietnamese Narrative Texts. Arlington: University of Texas at Arlington.
Denny, J. P. (1979). “The “extendedness” variable in classifier semantics: universal semantic features and cultural variation,” Ethnology: Boas, Sapir, and Whorf Revisited, Mathiot, M. The Hague: Mouton, 97–119. doi: 10.1515/9783110804157-006
Denny, J. P., and Creider, C. A. (1986). “The semantics of noun classes in Proto Bantu,” in Noun Classes and Categorization, ed C. G. Craig (Amsterdam: John Benjamins Publishing Company), 217–240. Available online at: http://digital.casalini.it/9789027279170
Dixon, R. M. W. (1982). Where Have All the Adjectives Gone? And Other Essays in Semantics and Syntax. Berlin: Mouton doi: 10.1515/9783110822939
Downing, P. (1986). “The anaphoric use of classifiers in Japanese,” in Noun Classes and Categorization, ed C. G. Craig (Amsterdam: John Benjamins Publishing Company), 345–375. Available online at: http://digital.casalini.it/9789027279170
Enfield, J. (2004). Nominal classification in Lao: a sketch. STUF-Lang. Typol. Univer. 57, 117–143. doi: 10.1524/stuf.2004.57.23.117
Erbaugh, M. S. (1986). “Taking stock: The development of chinese noun classifiers historically and in young children,” in Noun Classes and Categorization, ed C. G. Craig (Amsterdam: John Benjamins Publishing Company), 399–436. Available online at: http://digital.casalini.it/9789027279170
Fisher, D. F., Monty, R. A., and Senders, J. W. (2017). Eye Movements: Cognition and Visual Perception. London: Routledge.
Hagen, T., and Laeng, B. (2016). The change detection advantage for animals: an effect of ancestral priorities or progeny of experimental design? i-Perception. 7, 1–17. doi: 10.1177/2041669516651366
Hauland, G., and Duijm, N. (2002). “Eye movement based measures of team situation awareness (Tsa),” in Japan-halden MMS Workshop (Kyoto, Japan: Kyoto University), 82–85.
Heath, J. (1983). “Referential tracking in Nunggubuyu,” in Switch-Reference and Universal Grammar, Munro, P., and Haiman, J. (eds.). Amsterdam/Philadelphia: John Benjamins, 129–149. doi: 10.1075/tsl.2.09hea
Holmqvist, K., Nyström, M., Andersson, R., Dewhurst, R., Jarodzka, H., and Van de Weijer, J. (2011). Eye Tracking: A Comprehensive Guide to Methods and Measures. Oxford: OUP Oxford.
Hopper, P. J. (1986). “Some discourse functions of classifiers in malay,” in Noun Classes and Categorization, ed C. G. Craig (Amsterdam: John Benjamins Publishing Company), 309-326. Available online at: http://digital.casalini.it/9789027279170
Huettig, F., and Altmann, G. T. (2005). Word meaning and the control of eye fixation: semantic competitor effects and the visual world paradigm. Cognition 96, B23–B32. doi: 10.1016/j.cognition.2004.10.003
Huettig, F., Quinlan, P. T., McDonald, S. A., and Altmann, G. T. (2006). Models of high-dimensional semantic space predict language-mediated eye movements in the visual world. Acta psychologica. 121, 65–80. doi: 10.1016/j.actpsy.2005.06.002
Huettig, F., Rommers, J., and Meyer, A. S. (2011). Using the visual world paradigm to study language processing: a review and critical evaluation. Acta Psychol. 137, 151–171. doi: 10.1016/j.actpsy.2010.11.003
Jackson, R. L., Lambon Ralph, M. A., and Pobric, G. (2015). The timing of anterior temporal lobe involvement in semantic processing. J. Cognit. Neurosci. 27, 1388–1396. doi: 10.1162/jocn_a_00788
Kirchner, H., and Thorpe, S. J. (2006). Ultra-rapid object detection with saccadic eye movements: visual processing speed revisited. Vision Res. 46, 1762–1776. doi: 10.1016/j.visres.2005.10.002
Liu, H., Agam, Y., Madsen, J. R., and Kreiman, G. (2009). Timing, timing, timing: fast decoding of object information from intracranial field potentials in human visual cortex. Neuron. 62, 281–290. doi: 10.1016/j.neuron.2009.02.025
Lobben, M., Bochynska, A., Tanggaard, S., and Laeng, B. (2020). Classifiers in non-European languages and semantic impairments in western neurological patients have a common cognitive structure. Lingua 245, 102929. doi: 10.1016/j.lingua.2020.102929
Löbel, E. (2000). “Classifiers vs. genders and noun classes: a case study in Vietnamese,” in Gender in Grammar and Cognition, Unterbeck, et al. Berlin: Mouton de Gruyter, 259–319.
Mahanama, B., Jayawardana, Y., Rengarajan, S., Jayawardena, G., Chukoskie, L., Snider, J., et al. (2022). Eye movement and pupil measures: a review. Front. Comp. Sci. 3, 733531. doi: 10.3389/fcomp.2021.733531
Martinovic, J., Gruber, T., and Müller, M. M. (2007). Induced gamma band responses predict recognition delays during object identification. J. Cognitive Neurosci. 19, 921–934. doi: 10.1162/jocn.2007.19.6.921
Martins, S. A. (1994). Análise da morfosintaxe da língua Dâw (Maku-Kamã) e sua classificação tipológica. Florianópolis: Universidade Federal de Santa Catarina.
Mohanty, A., and Sussman, T. J. (2013). Top-down modulation of attention by emotion. Front. Hum. Neurosci. 7, 102. doi: 10.3389/fnhum.2013.00102
Moore, C., and Dunham, P. (1995). Joint Attention: Its Origins and Role in Development. Mahwah, NJ: Lawrence Erlbaum Associates.
Paulus, Y. T., and Remijn, G. B. (2021). Usability of various dwell times for eye-gaze-based object selection with eye tracking. Displays 67, 101997. doi: 10.1016/j.displa.2021.101997
Porretta, V., Kyröläinen, A., van Rij, J., and Järvikivi, J. (2018). “Visual world paradigm data: from preprocessing to nonlinear time-course analysis” in Smart Innovation, Systems and Technologies, Czarnowski, I., Howlett, R. J., and Jain, L. C. (eds.). Cham: Springer International Publishing, 268–277.
Ralph, M. A. L., Jefferies, E., Patterson, K., and Rogers, T. T. (2017). The neural and computational bases of semantic cognition. Nat. Rev. Neurosci. 18, 42–55. doi: 10.1038/nrn.2016.150
Rumsey, A. (1982). An Intrasentence Grammar of Ungarinjin, North-Western Australia. Canberra: Pacific Linguistics.
Salverda, A. P., and Michael, K. (2017). “Chapter 5—The Visual World Paradigm,” in Research Methods in Psycholinguistics and the Neurobiology of Language: A Practical Guide, De Groot, A. M. B., and Hagoort, P. (eds.). Oxford: Wiley.
Spivey, M. J., Tanenhaus, M. K., Eberhard, K. M., and Sedivy, J. C. (2002). Eye movements and spoken language comprehension: Effects of visual context on syntactic ambiguity resolution. Cognitive Psychol. 45, 447–481. doi: 10.1016/S0010-0285(02)00503-0
Stocker, K., and Laeng, B. (2017). Analog and digital windowing of attention in language, visual perception, and the brain. Cognitive Semantics 3, 158–118. doi: 10.1163/23526416-00302002
Strube, M., and Ponzetto, S. P. (2006). WikiRelate! Computing semantic relatedness using Wikipedia. AAAI 6, 1419–1424.
Suvorov, R. (2015). The use of eye tracking in research on video-based second language (L2) listening assessment: a comparison of context videos and content videos. Lang. Test. 32, 463–483. doi: 10.1177/0265532214562099
Tanenhaus, M. K., Spivey-Knowlton, M. J., Eberhard, K. M., and Sedivy, J. C. (1995). Integration of visual and linguistic information in spoken language comprehension. Science 268, 1632–1634. doi: 10.1126/science.7777863
Tomasello, M., and Farrar, J. (1986). Joint attention and early language. Child Dev. 57, 1454–1463. doi: 10.2307/1130423
Tse, S. K., Li, H., and Leung, S. O. (2007). The acquisition of Cantonese classifiers by preschool children in Hong Kong. J. Child Lang. 34, 495–517. doi: 10.1017/S0305000906007975
Vajda, E. (2002). Alexandra Y. Aikhenvald, classifiers: a typology of noun categorization devices. J. Linguist. 38, 137–172. doi: 10.1017/S0022226702211378
VanRullen, R., and Thorpe, S. J. (2001). The time course of visual processing: from early perception to decision-making. J. Cognit. Neurosci. 13, 454–461. doi: 10.1162/08989290152001880
Wolfe, J. M., and Horowitz, T. S. (2004). What attributes guide the deployment of visual attention and how do they do it?. Nature Rev. Neurosci. 5, 495. doi: 10.1038/nrn1411
Keywords: semantic relatedness, visual world paradigm, coarse vs. fine neural semantic processing, eye tracking, numeral classifiers, Chinese, reference trackers
Citation: Lobben M, Bochynska A, Eifring H and Laeng B (2023) Tracking semantic relatedness: numeral classifiers guide gaze to visual world objects. Front. Lang. Sci. 2:1222982. doi: 10.3389/flang.2023.1222982
Received: 15 May 2023; Accepted: 31 July 2023;
Published: 06 September 2023.
Edited by:
Moreno I. Coco, Sapienza University of Rome, ItalyReviewed by:
Nikole Patson, Ohio State University, Marion, United StatesJaklin Kornfilt, Syracuse University, United States
Copyright © 2023 Lobben, Bochynska, Eifring and Laeng. This is an open-access article distributed under the terms of the Creative Commons Attribution License (CC BY). The use, distribution or reproduction in other forums is permitted, provided the original author(s) and the copyright owner(s) are credited and that the original publication in this journal is cited, in accordance with accepted academic practice. No use, distribution or reproduction is permitted which does not comply with these terms.
*Correspondence: Halvor Eifring, aGFsdm9yZUB1aW8ubm8=