- 1 Institute for Advanced Biosciences, Keio University, Tsuruoka, Yamagata, Japan
- 2 Environment and Health Department, Istituto Superiore di Sanitá, Rome, Italy
It is breathtaking each time to observe the effects of simple social organization of complex systems. Whether watching the display of patterns formed by shoal of fish in an aquarium, or walking down the tropical jungle to witness the synchronized flashing of fireflies, life surrounding us inspires our thinking on the possible mechanisms required to achieve self assembly. Hence, for a long time, mankind has been curious about the mystery of self-organizations.
Noticeably, over the years, there have been a large number of works studying the self-organized behavior in biology. The formation of bio-films by bacteria for survival to environmental changes (Smith and Romesberg, 2007) and the synchronization of neural cells for cognition (Hipp et al., 2011) are good macroscopic examples of collective behaviors. How can one witness such coordination in the realm of molecular biology?
One essential feature for self-organized system is to display structure emerging from localized interactions. Obviously, using the traditional approach of monitoring a few intracellular molecules over time does not entail us to notice the existence of patterns or structures. On the other hand, the development of high throughput methodologies has been instrumental in observing the behavior of large number of molecules. We investigated the whole genome expression (consisting of 22,690 different ORFs from the Affymetrix standard platform) of the innate immune response to the Toll-like receptor (TLR) 4 stimulation (Tsuchiya et al., 2009a). The TLRs, with 10 known members, are “intruder” pattern recognizing proteins found mostly on immune cell surfaces (Kawai and Akira, 2010). The TLR 4, in particular, recognizes lipopolysaccharide (LPS) and triggers the MyD88- and TRIF-dependent pathways (Figure 1A). Hence, the MyD88 and TRIF are crucial for the proper induction of proinflammatory response. Note that the actual TLR 4 pathways are highly complex with several feedback mechanisms, such as the NF-κB regulatory loops and autocrine signaling (Hoffmann et al., 2002; Liu et al., 2008).
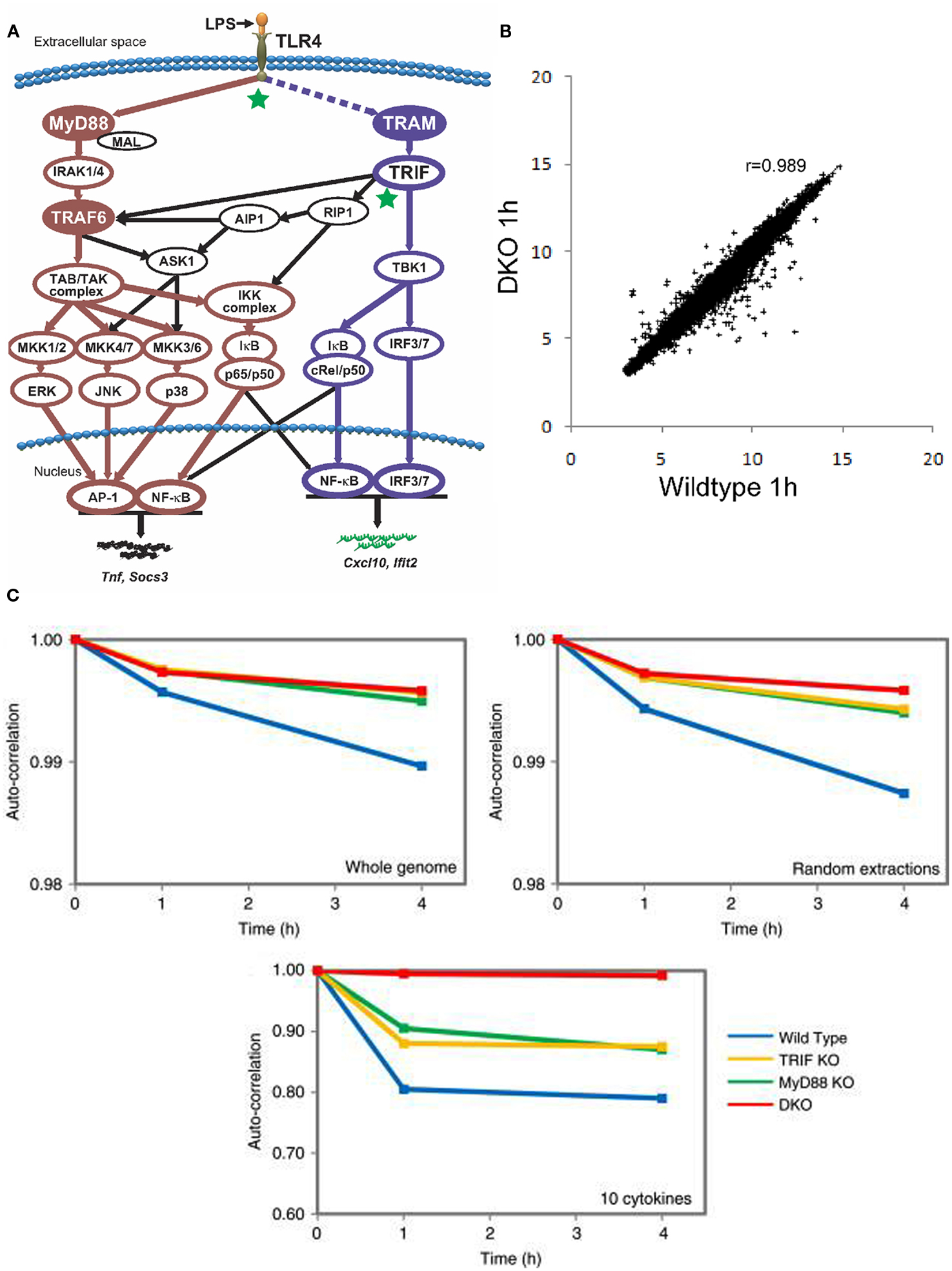
Figure 1. (A) A highly simplified schematic of TLR4 signaling depicting the primary MyD88-dependent and TRIF/TRAM-dependent pathways (Selvarajoo et al, 2008). (B) Invariance in whole genome Pearson correlation between wildtype 1 h (x-axis) and DKO 1 h (y-axis) murine macrophages. Each point in the plot represents the expression of a single ORF (Tsuchiya et al., 2009a). (C) Auto correlations analysis for whole genome (upper left), random extractions of ORFs (upper right), and 10 important cytokines genes (tnf, il1b, il12, il6, il8, ccl3, ccl4, socs3, socs1, and cxc10; lower panel) after LPS stimulation (Tsuchiya et al., 2009b). x-axis represents time (in hours), and y-axis represents the correlation coefficient with t = 0.
Our dataset on LPS stimulation of murine macrophages referred to 12 experimental readouts (i.e., four genotypes at three time points): wildtype, MyD88 knock-out (KO), TRIF-KO, and MyD88/TRIF Double KO (DKO) at 0, 1, and 4 h. The common experience of any experimentalist dealing with whole transcriptome data is the fact that any two independent samples of the same cell kind when correlated over 20,000 different gene products will more or less display a near to unity correlation. Our correlation analyses revealed a strong organization, spanning four-order of magnitudes of gene expression levels and encompassing tens of thousands of gene products across all 12 readouts, notwithstanding the huge phenotype macroscopic differences between different samples (e.g., the DKO have their phenotypic immune response abolished; Figure 1B). This is a very remarkable fact of nature calling for an explanation and clearly supporting the crucial importance of a thorough investigation of its origin from a statistical mechanics perspective (Conti et al., 2007; Censi et al., 2010).
The strong invariance of the transcriptome profiles is a consequence of the existence of very few “attractors” in the gene expression space correspondent to different cell types. The theoretically transfinite number of different transcription profiles supported by more than 20,000 different genes each varying over four-order of magnitudes of expression levels drastically collapses to around 200–300 tissue types present in the metazoans (Lima de Faria, 1988). This constrained behavior implies a strongly connected network of gene expression levels endowed with a very few “energy minima” or “allowed states” correspondent to different tissues. The response to acute and transient stimuli (like in our case the response to LPS stimulation by macrophages) does not alter the global attractor organization (Tsuchiya et al., 2009a), on the contrary the response implies a transient dramatic change of very few “responder genes” (growth factors, cytokines in our case), the system then comes back to its “stable state” thanks to the internal constraints between different genes.
Turning to temporal correlation analysis, Figure 1C reports the auto-correlation distribution in time for all the four genotypes relative to the above described choices of genes. In the case of the entire genome (top left), we observe a major departure from unit correlation correspondent to a greater response, as expected, in the case of wildtype. The three mutated genotypes all displayed a very minor, albeit reliable, and monotonically related to time, departure from unity correlation pointing to the “global sensing” of LPS stimulation. The presence of a strong attractor-like structure constraining the genome-wide expression at the cell population level into a sharply defined configuration spanning the entire set of gene expression values allows for only minor departures from unit of the auto-correlation in time, despite the fact that the TLR 4 signaling possesses numerous feedback regulations (Hoffmann et al., 2002; Liu et al., 2008). Shifting to a random choice of 100 genes we observed exactly the same pattern displayed by the entire genome basis (Figure 1C, top right). We iterated many times these random choices and observed a strongly invariant picture starting from a minimal choice of around 80 genes (Tsuchiya et al., 2009a). This is a confirmation of the “scalable” character of gene expression network, or fractality, constituting a strongly connected set whose general connectivity can be appreciated starting from a minimum sample of elements.
To summarize, the macroscopic view of temporal gene expressions reveals two distinct mode of innate immune response: (i) the local motion of specific genes responsible for the acute innate immune effect of LPS stimulation is registered by the cytokine choice and, (ii) the global motion of the entire gene regulation network as a connected system. The first is the primarily investigated proinflammatory response that can be modeled using linear response demonstrating the equilibrium state (Selvarajoo et al., 2008; Selvarajoo, 2011). The latter shows the rest of genome, which would otherwise be considered unrelated, is in fact correlated by the complex causality network linking different gene expressions and thus demonstrating self-organized behavior. This emergent pattern does not seem to be dependent of key molecular parameters such as MyD88 and TRIF or in their combined effect, but a signature from global non-equilibrium state of the entire network (Bak and Paczuski, 1995). Notably, the latter state may be crucial for the ability of macrophages to perform its secondary role of coordinated phagocytosis, that is, the removal of necrotic debris of infected cells (Brouckaert et al., 2004).
In another relevant work by Nilsson et al. (2006) on TLR4 stimulation, the tracking of 2892 genes over longer periods (up to 24 h) in wildtype macrophages revealed coordinated dynamics among specific clusters of genes that became active at different times. The subnetworks of genes are connected with master or “hub” genes, comprising mainly the well-known transcriptional factors of diverse cellular processes (e.g., ATF-3, NRF-2, ETS) into a scale-free topology, providing means for genomic order of TLR4 response. Pondering deeper into genome character, it has been recently shown for hematopoietic progenitor cell differentiation that gene coregulation move from ordered to disordered and then return to ordered entropy state over several days, through the self-organizing lineage-specific chromosomal networks (Rajapakse et al., 2009).
Overall, viewing the whole genome response in entirety and investigating the response of thousands of gene expressions in correlation matrix offers a simple, yet powerful tool to observe and interpret the complex self-organizing nature of living systems. We believe future studies using non-linear approaches and the concept of chaos may elucidate the presence of self-organized criticality to infer “avalanches” of our immune system. As for now, we stress how the traditional distinction between “house-keeping” and “modulated” genes is untenable when in presence of an integrated whole of relations supporting a self-organized behavior.
References
Bak, P., and Paczuski, M. (1995). Complexity, contingency, and criticality. Proc. Natl. Acad. Sci. U.S.A. 92, 6689–6696.
Brouckaert, G., Kalai, M., Krysko, D. V., Saelens, X., Vercammen, D., Ndlovu, M. N., Haegeman, G., D’Herde, K., and Vandenabeele, P. (2004). Phagocytosis of necrotic cells by macrophages is phosphatidylserine dependent and does not induce inflammatory cytokine production. Mol. Biol. Cell 15, 1089–1100.
Censi, F., Bartolini, P., Giuliani, A., and Calcagnini, G. (2010). A systems biology strategy on differential gene expression data discloses some biological features of atrial fibrillation. PLoS ONE 5, e13668. doi: 10.1371/journal.pone.0013668
Conti, F., Valerio, M. C., Zbilut, J. P., and Giuliani, A. (2007). Will systems biology offer new holistic paradigms to life sciences? Syst. Synth. Biol. 4, 161–165.
Hipp, J. F., Engel, A. K., and Siegel, M. (2011). Oscillatory synchronization in large-scale cortical networks predicts perception. Neuron 69, 387–396.
Hoffmann, A., Levchenko, A., Scott, M. L., and Baltimore, D. (2002). The IκB-NF-κB signaling module: temporal control and selective gene activation. Science 298, 1241–1245.
Kawai, T., and Akira, S. (2010). The role of pattern-recognition receptors in innate immunity: update on Toll-like receptors. Nat. Immunol. 11, 373–384.
Lima de Faria, A. (1988). Evolution Without Selection. Form and Function by Autoevolution. Amsterdam: Elsevier.
Liu, X., Zhang, Y., Yu, Y., Yang, X., and Cao, X. (2008). SOCS3 promotes TLR4 response in macrophages by feedback inhibiting TGF-β1/Smad3 signaling. Mol. Immunol. 45, 1405–1413.
Nilsson, R., Bajic, V. B., Suzuki, H., di Bernardo, D., Björkegren, J., Katayama, S., Reid, J. F., Sweet, M. J., Gariboldi, M., Carninci, P., Hayashizaki, Y., Hume, D. A., Tegner, J., and Ravasi, T. (2006). Transcriptional network dynamics in macrophage activation. Genomics 88, 133–142.
Rajapakse, I., Perlman, M. D., Scalzo, D., Kooperberg, C., Groudine, M., and Kosak, S. T. (2009). The emergence of lineage-specific chromosomal topologies from coordinate gene regulation. Proc. Natl. Acad. Sci. U.S.A. 106, 6679–6684.
Selvarajoo, K. (2011). Macroscopic law of conservation revealed in the population dynamics of toll-like receptor signaling. Cell Commun. Signal. 9, 9.
Selvarajoo, K., Takada, Y., Gohda, J., Helmy, M., Akira, S., Tomita, M., Tsuchiya, M., Inoue, J., and Matsuo, K. (2008). Signaling flux redistribution at toll-like receptor pathway junctions. PLoS ONE 3, e3430. doi: 10.1371/journal.pone.0003430
Smith, P. A., and Romesberg, F. E. (2007). Combating bacteria and drug resistance by inhibiting mechanisms of persistence and adaptation. Nat. Chem. Biol. 3, 549–556.
Tsuchiya, M., Piras, V., Choi, S., Akira, S., Tomita, M., Giuliani, A., and Selvarajoo, K. (2009a). Emergent genome-wide control in wildtype and genetically mutated lipopolysaccarides-stimulated macrophages. PLoS ONE 4, e4905. doi: 10.1371/journal.pone.0004905
Citation: Selvarajoo K and Giuliani A (2012) Finding self-organization from the dynamic gene expressions of innate immune responses. Front. Physio. 3:192. doi: 10.3389/fphys.2012.00192
Received: 22 March 2012; Accepted: 22 May 2012;
Published online: 11 June 2012.
Copyright: © 2012 Selvarajoo and Giuliani. This is an open-access article distributed under the terms of the Creative Commons Attribution Non Commercial License, which permits non-commercial use, distribution, and reproduction in other forums, provided the original authors and source are credited.
*Correspondence:YWxlc3NhbmRyby5naXVsaWFuaUBpc3MuaXQ=