- 1Holsworth Research Initiative, La Trobe Rural Health School, La Trobe University, Bendigo, VIC, Australia
- 2School of Psychology and Public Health, La Trobe University, Melbourne, VIC, Australia
- 3John Ochsner Heart and Vascular Institute, Ochsner Clinical School-The University of Queensland School of Medicine, New Orleans, LA, United States
- 4Cardiology Department, Bendigo Health, Bendigo, VIC, Australia
- 5Department of Exercise Sciences, University of Auckland, Auckland, New Zealand
Regular physical activity is important for cardiovascular health. However, high-volume endurance exercise has been associated with increased number of electrocardiogram (ECG) abnormalities, including disturbances in cardiac rhythm (arrhythmias) and abnormalities in ECG pattern. The aim of this study was to assess if heart rate variability (HRV) is associated with ECG abnormalities. Fifteen participants with previous cycling experience completed a 21-day high-volume endurance exercise cycle over 3,515 km. Participants wore a 5-lead Holter monitor for 24 h pre- and post-exercise, which was used to quantify ECG abnormalities and export sinus R-to-R intervals (NN) used to calculate HRV characteristics. As noise is prevalent in 24-h HRV recordings, both 24-h and heart rate collected during stable periods of time (i.e., deep sleep) were examined. Participants experienced significantly more arrhythmias post high-volume endurance exercise (median = 35) compared to pre (median = 12; p = 0.041). All 24-h and deep sleep HRV outcomes were not different pre-to-post high-volume endurance exercise (p > 0.05). Strong and significant associations with arrhythmia number post-exercise were found for total arrhythmia (total arrhythmia number pre-exercise, ρ = 0.79; age, ρ = 0.73), supraventricular arrhythmia (supraventricular arrhythmia number pre-exercise: ρ = 0.74; age: ρ = 0.66), and ventricular arrhythmia (age: ρ = 0.54). As a result, age and arrhythmia number pre-exercise were controlled for in hierarchical regression, which revealed that only deep sleep derived low frequency to high frequency (LF/HF) ratio post high-volume endurance exercise predicted post total arrhythmia number (B = 0.63, R2Δ = 34%, p = 0.013) and supraventricular arrhythmia number (B = 0.77, R2Δ = 69%, p < 0.001). In this study of recreationally active people, only deep sleep derived LF/HF ratio was associated with more total and supraventricular arrhythmias after high-volume endurance exercise. This finding suggests that measurement of sympathovagal balance during deep sleep might be useful to monitor arrhythmia risk after prolonged high-volume endurance exercise performance.
Introduction
Regular aerobic exercise is important for cardiovascular (CV) health (Hower et al., 2018) and decreases the risk of CVdiseases (CVD), such as myocardial infarction and heart failure (Channon, 2020). It also leads to positive chronic structural and functional adaptations to the heart (Sharma, 2003) which are more apparent if the recommended volume of exercise per week is exceeded (George et al., 2012). This has led to an increasing proportion of the general population performing aerobic exercise on a weekly basis for periods of time that greatly exceed exercise recommendations for health (Piercy et al., 2018) (termed high-volume endurance exercise). However, links between high-volume endurance exercise and adverse CV changes are increasingly being reported (Williams and Thompson, 2014; Elliott and La Gerche, 2015), such as sudden cardiac death (Tsuji et al., 1996) and electrocardiogram (ECG) abnormalities (Ector et al., 2007; Abdulla and Nielsen, 2009; Wundersitz et al., 2019). ECG abnormalities include disturbances in the cardiac rhythm (arrhythmias) and abnormalities in the ECG pattern. Arrhythmias can be further classified as those originating in the ventricles (ventricular arrhythmias) or in the atria and other regions of the heart above the ventricles (supraventricular arrhythmias). For example, a 10-fold increase in cardiac arrhythmias was found in adults pre-to-post high-volume endurance exercise performance previously. High-volume endurance exercise is also being performed by people with varied training histories and across the lifespan, which may also be of concern for older adults performing this form of exercise as age is related to ECG abnormality (Zorzi et al., 2018b; Mannina et al., 2021). An ability to understand when the heart is acutely under excess stress from the chronic performance of high-volume endurance exercise would be beneficial for safe high-volume endurance exercise performance for those in the general population, as well as reducing heart injury risks that can be severe and potentially life threatening.
Imbalances in autonomic control are increasingly being linked to chronic ECG abnormality in the literature (Task Force of the European Society of Cardiology and the North American Society of Pacing and Electrophysiology, 1996; Sareban et al., 2020), especially in clinical populations (e.g., Smith, 1982; Binkley et al., 1991; Bigger et al., 1992; Algra et al., 1993; Kocovic et al., 1993; Hartikainen et al., 1996; de Oliveira et al., 2014). A relatively simple and non-invasive method that has shown promise for monitoring autonomic control is through heart rate variability (HRV) assessment, which is used to measure changes in intervals between successive heartbeats and is a non-invasive reflection of the health of the cardiac automatic nervous system (ANS) (Rajendra Acharya et al., 2006). A healthy ANS normally displays variability in heart rate and a stressed system is usually less variable (Kleiger et al., 1987; Nolan et al., 1998; La Rovere et al., 2003). Although, elevations in atrial fibrillation (AF) is strongly associated with increased risk of mortality (Bigger et al., 1992) and arrhythmias like AF can lead to elevations in HRV (e.g., low frequency to high frequency [LF/HF] ratio Lombardi et al., 2001).
It has been previously demonstrated that high-volume endurance exercise changes HRV in athletes (Zehender et al., 1990; Carter et al., 2003; Hynynen et al., 2006; Swanson, 2006; Seiler et al., 2007; Seidl and Asplund, 2014). For example, Seiler et al., 2007 found that highly trained athletes displayed changes in HRV, lasting 90 min after performing 60 to 120 min of exercise, and Hynynen et al. (2006) found chronic overtraining in athletes lead to significantly decreased HRV characteristics. Although, HRV analysis appears to provide a useful evaluation of autonomic control in athletes, reflecting the hearts ability to recover and respond to changing exercise-related stimuli (Acharya et al., 2007) a potential limitation of this method is that any activity during waking hours, such as physical movement (Moody, 1992; Saponznikov et al., 1992; Tobaldini et al., 2013) can introduce errors or noise within the signal. Hence, potentially important changes in ANS control might be missed. A more reliable method of assessing HRV, is to section and analyze data for periods where participants heart rate is stable, such as during deep sleep (Clifford et al., 2005; Herzig et al., 2017a,b; Kim and Shin, 2017). Deep sleep refers to sleep periods where there is regular breathing, high delta wave activity and low variation in HRV characteristics (Herzig et al., 2017b). Previous authors have reported that deep sleep HRV is more time accurate and reliable when compared to waking hours and other restful periods (Catcheside et al., 2001).
Deep sleep HRV has been assessed in several studies of athletes, but only shortly after an acute bout of exercise (Al Haddad et al., 2009; Myllymaki et al., 2012; Herzig et al., 2018). For example, Al Haddad et al., 2009 assessed the effect of supramaximal intermittent exercise to exhaustion on deep sleep HRV and found post-exercise vagal-related HRV characteristics significantly changed immediately and 12 h after exercise (i.e., LF/HF ratio expressed as a percentage significantly higher, mean RR significantly lower), but had returned to normal after 36 h. To date, however, no study has assessed the effect of high-volume endurance exercise on deep sleep HRV or investigated if changes in HRV are related to ECG abnormalities in response to high-volume endurance exercise. Therefore, the aim of this study was to assess the effect of high-volume endurance exercise in recreationally active people on HRV characteristics and if changes in HRV characteristics are related to ECG abnormalities in response to high-volume endurance exercise.
Materials and Methods
Participants and Protocol
This study performed a secondary analysis on previously published data (Wundersitz et al., 2019). The study was approved by the Bendigo Health Care Group Human Research Ethics Committee and the La Trobe University Human Ethics Committee (HREC/17/BHCG/9). Participants or legal guardians of participants aged under 18 years provided written informed consent prior to participating. Fifteen (14 male) recreationally active people (mean ± SD: age, 34.7 ± 19.3 years; height, 1.79 ± 0.08 m; body mass, 78.3 ± 17.6 kg) with 8.4 ± 12.8 years of recreational cycling experience took part in the ‘Make a difference, change our world’ charity bicycle ride (MADRIDE)1. The ride covered 3,515 km from Bunbury in Western Australia to Bendigo in Victoria (similar in distance to the Tour De France) (Marijon et al., 2013). Prior to completing the ride, participants completed screening (Balady et al., 1998) and training history questionnaires. Participants reported training for 8.7 (95%CI 4.9 – 12.5) months and covering 3.6 (3.1 – 4.2) sessions per week. On average during each session they trained for 2.9 (2.1 – 3.6) hours and covered 78 (63.5 – 92.5) km. Participants cycled for 21 consecutive days, rode for 6.4 (5.8 – 6.9) hours a day and on average covered 167 (150.9 – 183.8) km/day at 26.3 (25.2 – 27.4) km/h.
To determine HRV characteristics and ECG abnormality number, a 5-lead Holter monitor (SEER Light; GE Healthcare, Horten, Norway) was placed on each participants chest by a cardiac technician and worn for 24 h (9.2 ± 3.2 days pre-exercise). Whilst wearing the Holter monitor, participants were instructed to avoid exercising and to limit intense activities of daily living during this time. Participants again wore the same Holter monitor after completing the 21-days of high-volume endurance exercise (3.0 ± 1.8 days post-exercise). The availability of Holter monitors for research in a public hospital setting limited the ability to test all participants immediately post multi-day high-volume endurance exercise and was added as a covariate to analyses. Holter monitoring was chosen as it is the gold standard method of HRV measurement (Akintola et al., 2016; Georgiou et al., 2018).
Data Analysis
Manufacturer software (version 8, MARS Holter Analysis System; GE Healthcare, Horten, Norway) was used to process raw Holter monitor data throughout both 24-h wear periods. Holter data were screened to identify ECG abnormalities by an experienced cardiac technician and verified by a cardiologist who were both blinded to participant identity and measurement time point. R-to-R intervals between QRS complexes (RR intervals) were then exported using QRSDK software (MARS Holter Analysis System version 8; GE Healthcare, Horten, Norway) to allow further analyses. Abnormal RR intervals (non-sinus rhythm) were excluded from the analysis and those normal intervals between QRS complexes not excluded (NN intervals) were saved for further analyses in LabView software (version 2016; National Instruments, United Kingdom). Specifically, the NN signal was visually displayed in LabView and then deep sleep periods were identified and exported. To be classified as a deep sleep period, a 10-min duration where NN intervals were stationary was required and this inactive period was considered to be when the NN interval had less than a 1% change in slope (Brandenberger et al., 2005). The middle 5-min within this 10-min window was then used to calculate time and frequency domain HRV outcomes. Specifically, time domain variables included: heart rate (HR) mean, NN mean, standard deviation of the NN intervals (NNSD), proportion derived by dividing NN50 by the total number of NN intervals (PNN50) and square root of the mean squared difference of successive NN intervals (RMMSD). Frequency domain variables included: Total power (the sum of the four spectral densities; low-frequency component [LF], high-frequency component [HF], ultra-low frequency [ULF] and very-low frequency component [VLF]), normalized power in the low frequency (LF norm), normalized power in the high frequency (HF norm) and LF/HF ratio. Time and frequency domain variables were analyzed according to recommendations by Malik et al. (1996) In addition, Poincaré SD1 (length of the transverse line of the Poincaré plot area) was calculated to quantify changes in parasympathetic nervous system activity and Poincaré SD2 (length of the longitudinal line of the Poincaré plot area) was calculated to quantify changes in sympathetic nervous system activity (Brennan et al., 2001). Then the ratio of the two (SD1/SD2) was calculated to represent the balance between parasympathetic and sympathetic nervous system activity. Poincaré analyses can be used to detect irregularities that may otherwise be difficult to determine with conventional time and frequency domain variables (Laitio et al., 2000).
Statistical Analyses
SPSS for Windows (version 25; IBM Corporation, Armonk, NY, United States) was used to perform all statistical analyses. Shapiro-Wilks tests were performed to assess normality of data. If data were not-normally distributed, the data were log transformed. To assess the effect of 21-days of high-volume endurance exercise on HRV, one-way analysis of variance (ANOVAs - within factor: measurement time) were performed. Mauchly’s test was consulted for all ANOVA’s and Greenhouse Geisser corrected p-values are presented when the assumption of sphericity was violated. If significant main effects were found, pairwise comparisons with Bonferroni correction were performed. To explore the effect ‘participant age’ and the ‘measurement time point post cycling’ that data were captured, both were added as a covariates and additional ANCOVAs performed if significant Pearson’s correlation coefficients were identified between the covariate and dependent variable (Kim, 2018). Data were conveyed as the mean and 95% confidence interval unless data were not normally distributed (then the data were presented as median and interquartile range). Effect sizes (partial eta-squared statistic; η2p) are presented and statistical significance was set as p < 0.05.
To identify if there were factors that might predict post high-volume endurance exercise arrhythmia response, associations between participant characteristics (i.e., age, pre-exercise arrhythmia, training history, weekly training distance, measurement time point, gender) and all 24 h and deep sleep HRV pre/post characteristics were assessed with Spearman Rho correlations (ρ). Next, a two-step hierarchical regression was performed to identify which HRV characteristics were most associated with arrhythmia response (total arrhythmia, as well as supraventricular and ventricular arrhythmia responses) post multi-day endurance exercise. For the arrhythmia data, three (1 pre, 2 post) scores were 1.96 standard deviations above the mean and positively skewed the variables. Given that outlier adjustment is preferable to whole variable transformation in samples with 1 or 2 outliers, we winsorized (Rivest, 1994) the outliers whereby scores were adjusted down, while maintaining their rank. The pre and post multi-day endurance exercise arrhythmia data were normally distributed after winsorizing.
Results
ECG abnormalities that were not classified as arrhythmias were noted in seven participants, three pre (2nd degree AV block [two] and ST wave elevation) and four post (1st degree AV block, 2nd degree AV block, and ST-T wave changes [two]). In addition, single beat abnormalities were noted in three participants (Pre: bundle branch block, non-conducted atrial ectopic and ventricular escape beat; Post: junctional escape beat). Participants experienced significantly less total arrhythmias pre high-volume endurance exercise (median = 12, interquartile range = 4 – 62) compared to post (median = 35, interquartile range = 4 – 231; F1, 14 = 5.038, p = 0.041, η2p = 0.265). However, the differences pre to post high-volume endurance exercise in supraventricular (pre: median = 37, interquartile range = 3 – 40; post: median = 53, interquartile range = 4 – 57; F1, 14 = 0.903, p = 0.358, η2p = 0.061) and ventricular (pre: median = 6, interquartile range = 0 – 6; Post: median = 20, interquartile range = 0 – 20; F1, 14 = 1.060, p = 0.321, η2p = 0.07) arrhythmias did not reach significance.
24 h and Deep Sleep HRV Pre and Post High-Volume Endurance Exercise
There was an average of 5.8 (95%CI: 4.7 to 6.9) deep sleep periods per person identified at pre and 5.9 (95%CI: 4.8 to 6.9) identified post high-volume endurance exercise (F1, 14 = 0.006, p = 0.938, η2p < 0.001). 24-h and deep sleep HRV characteristics pre and post high-volume exercise are shown in Tables 1, 2. There was no statistically significant effect of measurement time on 24-h (p > 0.05, η2p ≤ 0.139) or deep sleep (p > 0.05, η2p ≤ 0.110) HRV characteristics measured.
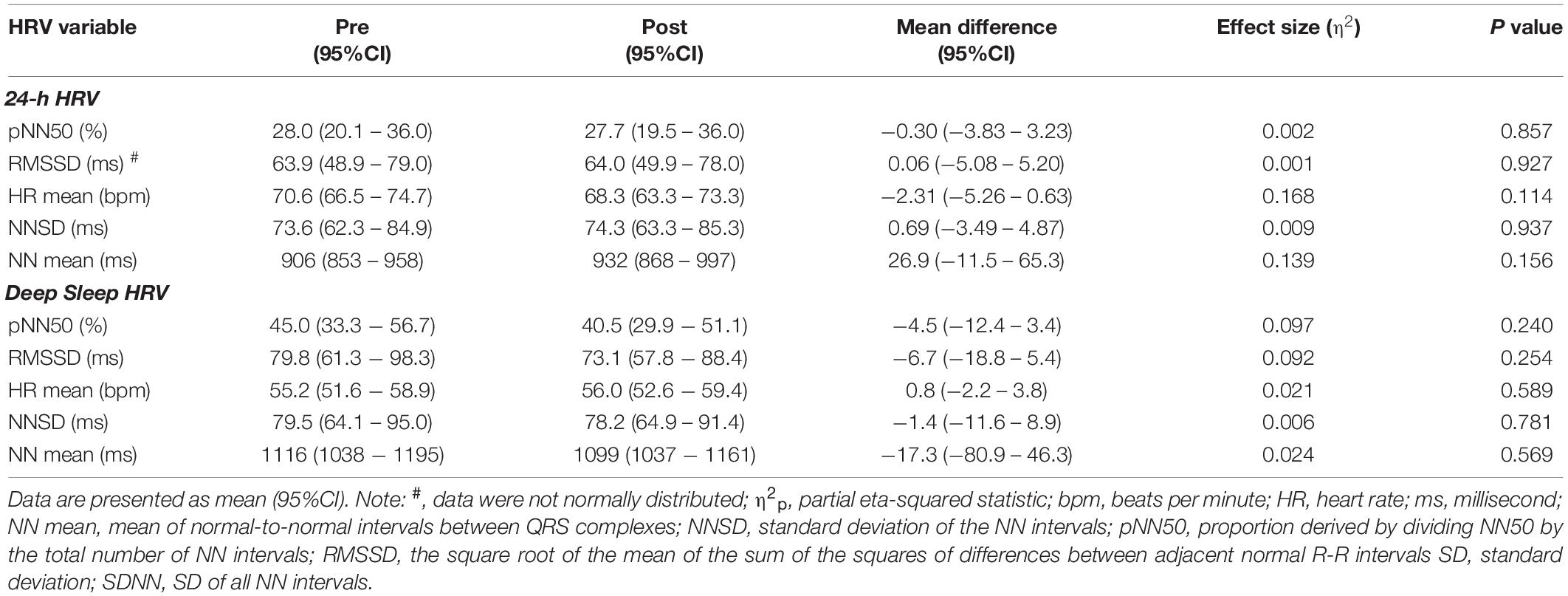
Table 1. Time domain HRV characteristics calculated pre and post high-volume endurance exercise (n = 15).
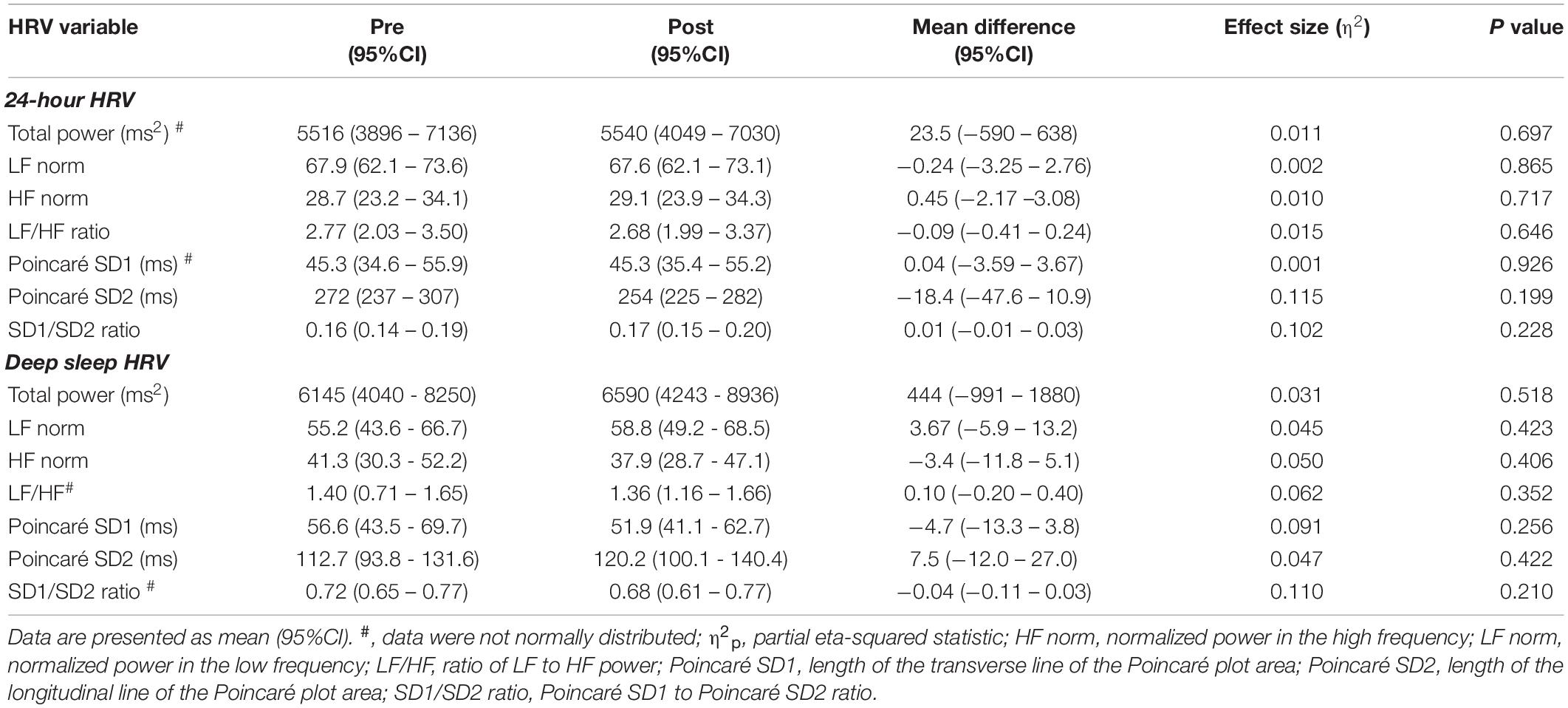
Table 2. Frequency domain HRV characteristics calculated pre and post high-volume endurance exercise (n = 15).
Factors Associated With Arrhythmia Response Post High-Volume Endurance Exercise
Age was the only factor to strongly correlate with total, supraventricular and ventricular arrhythmia post high-volume endurance exercise (ρ ≥ 0.54, p < 0.05). Arrhythmia pre strongly correlated with total and supraventricular arrhythmia post high-volume endurance exercise (ρ ≥ 0.66, p < 0.01), but not ventricular arrhythmia (ρ = 0.27, p < 0.05). All other factors were not significant (p > 0.05) and displayed moderate or lower correlations (ρ ≤ 0.43) with arrhythmia response post-exercise (Table 3). Deep sleep and 24-h HRV correlations are provided in Supplementary Data.
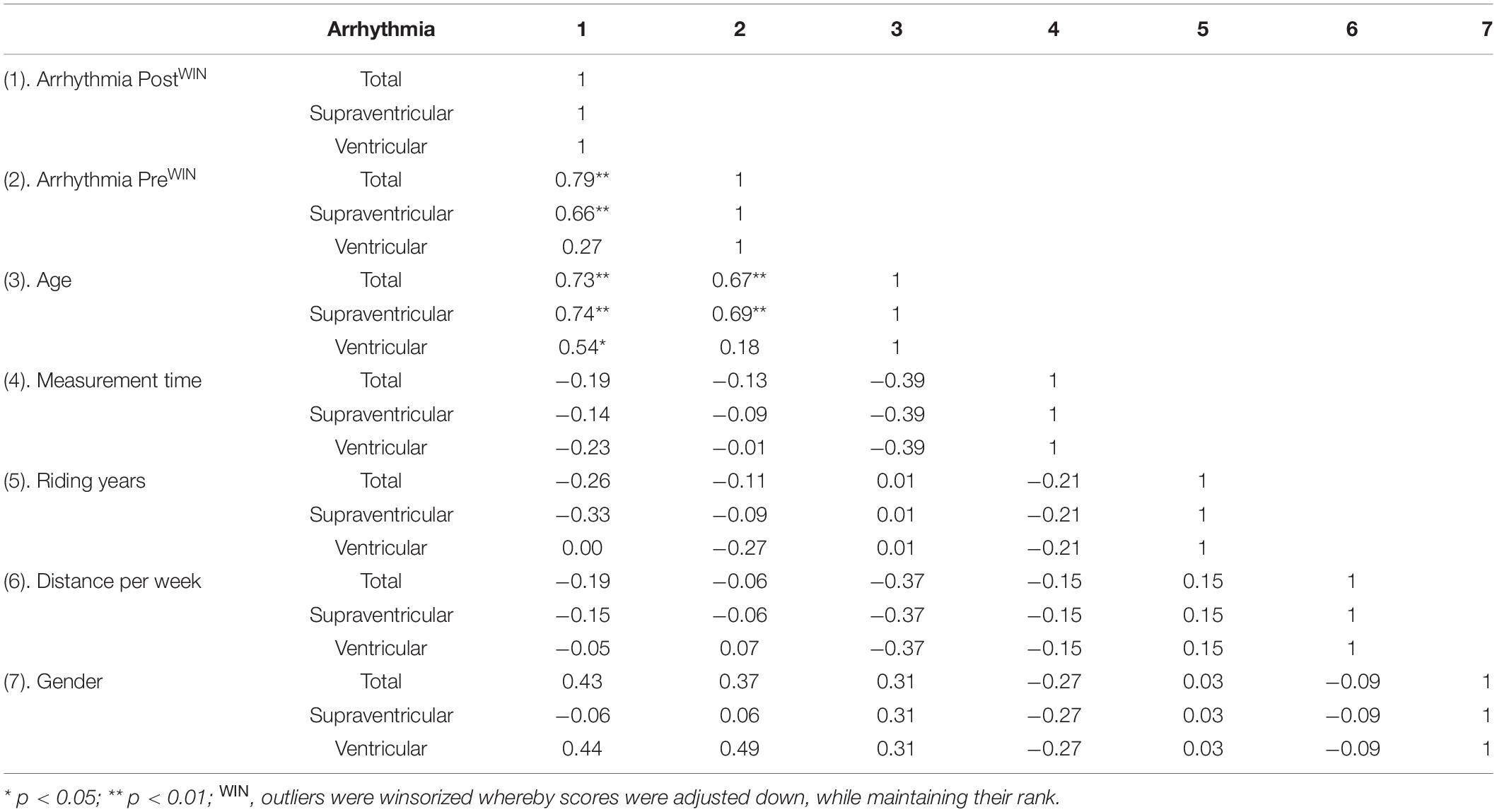
Table 3. Correlations comparing arrhythmia, age, days post-ride measurement performed, riding years and weekly training distance.
Does HRV Predict Post High-Volume Endurance Exercise ECG Predict Total Arrhythmia, Supraventricular and Ventricular Number
In the final hierarchal regression model that used the stepwise entry method at step 2, only deep sleep derived LF/HF ratio post high-volume endurance exercise was included in the final model for total arrhythmia and supraventricular arrhythmia analyses after controlling for age and pre-exercise arrhythmia number (Table 4). Specifically, higher LF/HF ratio post high-volume endurance exercise was strongly associated with higher counts of arrhythmia (total arrhythmia: B = 0.80, p = 0.012). The full model with all variables included was also a significant predictor of post-exercise total arrhythmia, R2 = 0.64, F(3, 10) = 6.01, p = 0.013 and supraventricular arrhythmia R2 = 0.69, F(3, 10) = 22.13, p < 0.001. Post exercise ventricular arrhythmia during deep sleep and the 24-h data (total arrhythmia, supraventricular and ventricular arrhythmia post exercise), were not associated with participant age or arrhythmia pre-exercise and no additional variables were entered at step 2.
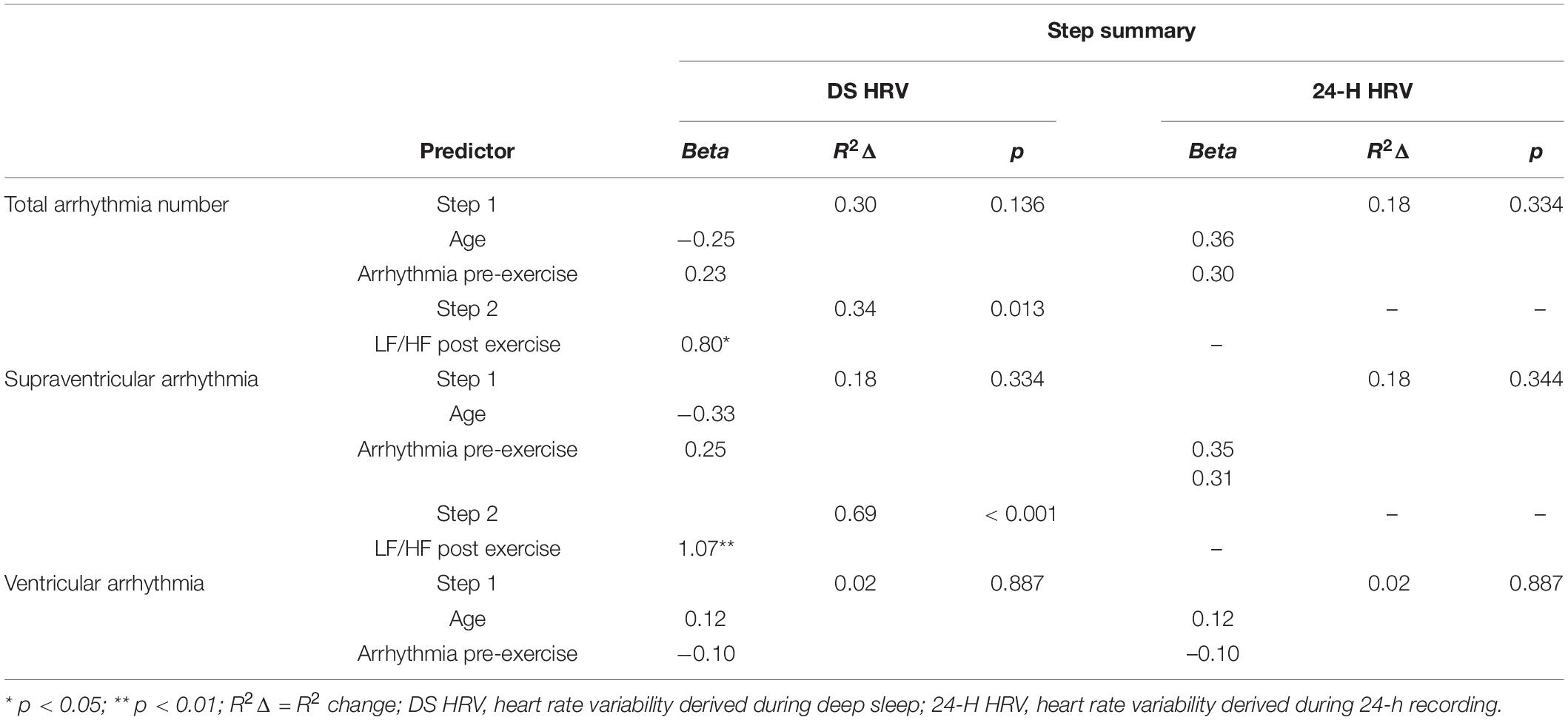
Table 4. Hierarchical regression with a Stepwise solution after step 1 assessing if measures of 24-h and deep sleep HRV are associated with post exercise ECG abnormality and arrhythmia after controlling for age and pre-exercise abnormality (n = 14).
Additional Findings
We removed the one female participant from the regression analyses as controlling for gender with such an imbalance is not appropriate. However, to confirm if the trends observed would remain with the additional female participant, we re-ran the analysis and controlled for gender. In this model, the findings mirrored those from total arrhythmia, with higher LF/HF post-exercise only associated with all post-exercise ECG abnormality (B = 0.80, p = 0.012) and supraventricular arrhythmia (B = 1.07, p < 0.001) during deep sleep.
Discussion
This was the first study to assess the relationship between deep sleep HRV characteristics, using a controlled measurement technique, and number of ECG abnormality in a recreational population of cyclists after performing multi-day high-volume endurance exercise. The main finding was that during deep sleep LF/HF ratio was a strong predictor of post-exercise total and supraventricular arrhythmia after controlling for age and arrhythmia number pre-exercise. We also confirmed that baseline arrhythmia number, in particular total and supraventricular arrhythmia number, and age were strongly correlated with post-exercise arrhythmia response, and that HRV characteristics were not significantly changed after performing multi-day high-volume endurance exercise.
Results from the current study suggest that post multi-day high-volume endurance exercise total arrhythmia and supraventricular number were related to baseline arrhythmia number and participant age. This result supports the widespread understanding that age is related to ECG abnormality, both in athletes (Andersen et al., 2013; Zorzi et al., 2018a,b) and the general population (Furberg et al., 1994; Lok and Lau, 1996; Mannina et al., 2021). This result is likely influenced by cardiovascular system deterioration with increasing age (Hatch et al., 2011) and accumulated demand on the heart from cumulative years of exercise training (Myrstad et al., 2014). The association between baseline total arrhythmia number with post-exercise total arrhythmia response suggests that multi-day high-volume endurance exercise is not likely to influence ECG abnormality in people with baseline arrhythmias.
Supraventricular arrhythmia number post high-volume endurance exercise was also related to baseline supraventricular number. This result is not unexpected, considering that high volume endurance exercise is accepted as a factor contributing to the development of supraventricular arrhythmias (D’Souza et al., 2019), including bradyarrhythmia, atrioventricular block (Baldesberger et al., 2008) and atrial fibrillation (Andersen et al., 2013; Myrstad et al., 2014). Further, supraventricular arrhythmias can have serious quality of life and sport participation consequences in athletes (D’Souza et al., 2019), although in the general population it has been suggested that they are common and rarely life threatening (Blomstrom-Lundqvist et al., 2003). The current findings highlight the importance of screening programs being performed prior to high-volume endurance exercise to identify unknown and potentially serious cardiac issues (Corrado et al., 2006; Mont et al., 2020).
Controlling for age and baseline arrhythmia number, the current results showed that higher LF/HF ratio post-exercise, when recorded during deep sleep, was a strong predictor of post-exercise total arrhythmia and supraventricular arrhythmia number. This finding was not seen when LF/HF ratio data were analyzed over a 24-h period or when ventricular arrhythmias were assessed. To our knowledge, researchers have not previously assessed the effect of endurance exercise in humans on HRV measures (during deep sleep or 24 h) and used these to predict ECG abnormality response. There has been HRV and ECG abnormality research in one study of horses performing endurance exercise (Flethøj et al., 2016), as well as animal and clinical populations performing single (e.g., Guiraud et al., 2013; Broux et al., 2017; Frick et al., 2019) or multiple (training studies; e.g., Deligiannis et al., 1999; Andrade et al., 2017) sessions of short duration exercise. In these studies, mixed findings were found between LF/HF ratio and ECG abnormality. For example, similar to the current study Guiraud et al. (2013), found an association between ECG abnormality and LF/HF ratio, with a decrease in ECG abnormality strongly related to a decrease in LF/HF ratio in people with chronic heart failure performing high intensity exercise (r = 0.66; p < 0.01). However Broux et al. (2017), found that LF/HF ratio was significantly lower at rest and during exercise (lunging test) in horses with atrial fibrillation (p < 0.001). Although few exercise studies are available, there is an abundance of research in clinical populations demonstrating that either a higher (Lombardi et al., 2001; Rizzo et al., 2015) or lower (Nortamo et al., 2018; Sagnard et al., 2020) LF/HF ratio is associated with ECG abnormality. There are a number of potential factors alone or in combination that might contribute to inconsistent findings in the literature, such as the duration over which HRV was assessed (Furlan et al., 1990), as well as the population (i.e., animal or healthy/clinical human) and type of ECG abnormality (Lombardi et al., 2001) under investigation. For example, mechanisms responsible for heart rate control are not likely to, and cannot, be considered stable with longer term recordings, such as over 24-h (Furlan et al., 1990). Performing moderate physical activity (Moody, 1992; Saponznikov et al., 1992; Tobaldini et al., 2013), emotional circumstances/mental stress (Task Force of the European Society of Cardiology and the North American Society of Pacing and Electrophysiology, 1996), and changing respiration (Malliani et al., 1991) or blood pressure (i.e., hypotension) can all induce changes in LF or HF power, hence LF/HF ratio, across longer term recordings. These should be controlled for and the results of the current study support this assumption, suggesting that measurement of HRV during deep sleep are more sensitive to detect ECG abnormalities than those calculated from 24-h recordings.
Regardless of the inconsistencies in the literature, the results of the current study suggest that there is potential to use HRV measures, such as LF/HF ratio, to acutely monitor responses to high-volume endurance exercise. If LF/HF ratio increases post high-volume endurance exercise, this could be used to indicate liability to increased ECG arrhythmia number. Further wearable devices (i.e., phones, fitness tracking watches, etc.) offer a cost-effective way to monitor exercise within 24-h of performance (i.e., whenever they go to sleep) and non-invasively as long as they produce the HRV measure LF/HF ratio during sleep. Future research should continue to assess the relationship between LF/HF ratio and ECG abnormality number after performing high-volume endurance exercise in a large cohort of people, especially those who perform exercise for health benefit. Future studies should endeavor to identify a potential threshold value where any increase in LF/HF ratio above this is of concern.
HRV measures assessed during deep sleep were unchanged as a result of high-volume endurance exercise. Recovery from acute, shorter duration exercise is normally rapid (e.g., Kaikkonen et al., 2007; Michael et al., 2016) and complete recovery might take at least 48 h after high-intensity exercise (Stanley et al., 2013). Our results extend this work and confirm that after 21-days of high-volume endurance exercise participants displayed no differences when measured 72 h after.
Strengths and Limitations
A strength of this study was that a controlled measurement technique (i.e., 5-lead Holter monitor) was used to quantify outcomes in the study and HRV measures were measured during deep sleep (and over 24-h) rather than averaged over the 24-h monitoring period. There are, however, a number of limitations that should be acknowledged. First, as data were only captured pre- and post-high-volume endurance exercise, application of HRV measures during exercise as a potential predictor of endurance exercise produced ECG abnormality are not known. What is known is that HRV characteristics are substantially reduced during exercise (Michael et al., 2017), so any change in LF/HF ratio might or might not be related to ECG abnormality in this situation and more research is needed. Second, LF/HF ratio is a non-invasive measure that is typically used as an indirect measure of sympathovagal balance (Task Force of the European Society of Cardiology and the North American Society of Pacing and Electrophysiology, 1996), however, this is controversial in the literature (Billman, 2013; Michael et al., 2017). Higher LF/HF ratio post-exercise being a strong predictor of post-exercise ECG arrhythmia number suggest that high-volume endurance exercise was associated with low vagal activation and sympathetic predominance (Eckberg, 1997) leading to increased ECG arrhythmia number. Sympathetic predominance is typically seen in people under chronic life stress (e.g., Lucini et al., 2005) suggesting that high-volume endurance exercise can lead to a similar situation. However, measures of stress were not captured in this study, so it was not possible to assess the influence of this potential confounding variable.
Conclusion
High-volume endurance exercise is growing in popularity across the lifespan due to perceived health benefits. However, there is a body of evidence in professional athletes suggesting that exercise might have negative CV consequences when the amount of exercise performed greatly exceeds recommendations. Results from the current study in recreationally active people found that baseline ECG arrhythmia number, in particular supraventricular arrhythmia number, was associated with post high-volume endurance exercise ECG arrhythmia number. After controlling for baseline ECG arrhythmia number, the HRV characteristic LF/HF ratio measured during deep sleep was shown to be strongly associated with ECG arrhythmia number after performing high-volume endurance exercise for 21 consecutive days. LF/HF ratio offers promise as a non-invasive measure associated with high-volume endurance exercise-induced ECG abnormality.
Data Availability Statement
The original contributions presented in the study are available online (doi: 10.26181/1914974) included in Supplementary Material, further inquiries can be directed to the corresponding author.
Ethics Statement
The studies involving human participants were reviewed and approved by Bendigo Health Care Group Human Research Ethics Committee and La Trobe University Human Ethics Committee (HREC/17/BHCG/9). Written informed consent to participate in this study was provided by the participants’ legal guardian/next of kin.
Author Contributions
MK, BG, and CL conceptualized the project. DW and KN performed data collection. DW, KN, and SP analyzed the data. DW, MK, and BW statistically analyzed the data. SP, DW, and BG drafted the first draft. DW, MK, CL, and BG adapted the draft to the final manuscript. All authors contributed to the article and approved the submitted version.
Conflict of Interest
The authors declare that the research was conducted in the absence of any commercial or financial relationships that could be construed as a potential conflict of interest.
Publisher’s Note
All claims expressed in this article are solely those of the authors and do not necessarily represent those of their affiliated organizations, or those of the publisher, the editors and the reviewers. Any product that may be evaluated in this article, or claim that may be made by its manufacturer, is not guaranteed or endorsed by the publisher.
Acknowledgments
We extend their gratitude to the participants for their contribution to this research. We also acknowledge the Bendigo Tertiary Education Anniversary Foundation, Holsworth Research Initiative’s support of Wundersitz, and Professor Kingsley’s research.
Supplementary Material
The Supplementary Material for this article can be found online at: https://www.frontiersin.org/articles/10.3389/fphys.2022.848174/full#supplementary-material
Footnotes
References
Abdulla, J., and Nielsen, J. R. (2009). Is the risk of atrial fibrillation higher in athletes than in the general population? A systematic review and meta-analysis. Europace 11, 1156–1159. doi: 10.1093/europace/eup197
Acharya, U. R., Suri, J., Spaan, J. A. E., and Krishnan, S. M. (2007). Advances in Cardiac Signal Processing. Berlin: Springer.
Akintola, A. A., van de Pol, V., Bimmel, D., Maan, A. C., and van Heemst, D. (2016). Comparative analysis of the equivital EQ02 lifemonitor with holter ambulatory ECG device for continuous measurement of ECG, heart rate, and heart rate variability: a validation study for precision and accuracy. Front. Physiol. 7:391. doi: 10.3389/fphys.2016.00391
Al Haddad, H., Laursen, P. B., Ahmaidi, S., and Buchheit, M. (2009). Nocturnal heart rate variability following supramaximal intermittent exercise. Int. J. Sports Physiol. Perform. 4, 435–447. doi: 10.1123/ijspp.4.4.435
Algra, A., Tijssen, J. G., Roelandt, J. R., Pool, J., and Lubsen, J. (1993). Heart rate variability from 24-hour electrocardiography and the 2-year risk for sudden death. Circulation 88, 180–185. doi: 10.1161/01.CIR.88.1.180
Andersen, K., Farahmand, B., Ahlbom, A., Held, C., Ljunghall, S., Michaëlsson, K., et al. (2013). Risk of arrhythmias in 52 755 long-distance cross-country skiers: a cohort study. Eur. Heart J. 34, 3624–3631. doi: 10.1093/eurheartj/eht188
Andrade, D. C., Arce-Alvarez, A., Toledo, C., Díaz, H. S., Lucero, C., Schultz, H. D., et al. (2017). Exercise training improves cardiac autonomic control, cardiac function, and arrhythmogenesis in rats with preserved-ejection fraction heart failure. J. Appl. Physiol. 123, 567–577. doi: 10.1152/japplphysiol.00189.2017
Balady, G. J., Chaitman, B., Driscoll, D., Foster, C., Froelicher, E., Gordon, N., et al. (1998). Recommendations for cardiovascular screening, staffing, and emergency policies at health/fitness facilities. Circulation 97, 2283–2293.
Baldesberger, S., Bauersfeld, U., Candinas, R., Seifert, B., Zuber, M., Ritter, M., et al. (2008). Sinus node disease and arrhythmias in the long-term follow-up of former professional cyclists. Eur. Heart J. 29, 71–78. doi: 10.1093/eurheartj/ehm555
Bigger, J. T., Fleiss, J. L., Steinman, R. C., Rolnitzky, L. M., Kleiger, R. E., and Rottman, J. N. (1992). Correlations among time and frequency domain measures of heart period variability two weeks after acute myocardial infarction. Am. J. Cardiol. 69, 891–898. doi: 10.1016/0002-9149(92)90788-Z
Billman, G. (2013). The LF/HF ratio does not accurately measure cardiac sympatho-vagal balance. Front. Physiol. 4:26. doi: 10.3389/fphys.2013.00026
Binkley, P. F., Nunziata, E., Haas, G. J., Nelson, S. D., and Cody, R. J. (1991). Parasympathetic withdrawal is an integral component of autonomic imbalance in congestive heart failure: demonstration in human subjects and verification in a paced canine model of ventricular failure. J. Am. College Cardiol. 18, 464–472. doi: 10.1016/0735-1097(91)90602-6
Blomstrom-Lundqvist, C., Scheinman, M. M., Aliot, E. M., Alpert, J. S., Calkins, H., Camm, A. J., et al. (2003). ACC/AHA/ESC guidelines for the management of patients with supraventricular arrhythmias–executive summary. a report of the American college of cardiology/American heart association task force on practice guidelines and the European society of cardiology committee for practice guidelines (writing committee to develop guidelines for the management of patients with supraventricular arrhythmias) developed in collaboration with NASPE-Heart Rhythm Society. J. Am. Coll. Cardiol. 42, 1493–1531. doi: 10.1016/j.jacc.2003.08.013
Brandenberger, G., Buchheit, M., Ehrhart, J., Simon, C., and Piquard, F. (2005). Is slow wave sleep an appropriate recording condition for heart rate variability analysis? Auton. Neurosci. 121, 81–86. doi: 10.1016/j.autneu.2005.06.002
Brennan, M., Palaniswami, M., and Kamen, P. (2001). Do existing measures of Poincare plot geometry reflect nonlinear features of heart rate variability? IEEE Trans. Biomed. Eng. 48, 1342–1347. doi: 10.1109/10.959330
Broux, B., De Clercq, D., Decloedt, A., Ven, S., Vera, L., van Steenkiste, G., et al. (2017). Heart rate variability parameters in horses distinguish atrial fibrillation from sinus rhythm before and after successful electrical cardioversion. Equine Vet. J. 49, 723–728. doi: 10.1111/evj.12684
Carter, J. B., Banister, E. W., and Blaber, A. P. (2003). Effect of endurance exercise on autonomic control of heart rate. Sports Med. 33, 33–46.
Catcheside, P. G., Chiong, S. C., Orr, R. S., Mercer, J., Saunders, N. A., and McEvoy, R. D. (2001). Acute cardiovascular responses to arousal from non-REM sleep during normoxia and hypoxia. Sleep 24, 895–902. doi: 10.1093/sleep/24.8.895
Channon, K. M. (2020). Exercise and cardiovascular health: new routes to reap more rewards. Cardiovasc. Res. 116, e56–e58. doi: 10.1093/cvr/cvz264
Clifford, G. D. Z. L., Janz, B. A., Mietus, J. E., Young, C. Y., and Mark, R. G. (2005). Segmentation of 24-hour cardiovascular activity using ECG- based sleep/sedation and noise metrics. Comput. Cardiol. 32, 595–598.
Corrado, D., Basso, C., Pavei, A., Michieli, P., Schiavon, M., and Thiene, G. (2006). Trends in sudden cardiovascular death in young competitive athletes after implementation of a preparticipation screening program. JAMA 296, 1593–1601. doi: 10.1001/jama.296.13.1593
de Oliveira, C. A., de Brito Junior, H. L., Bastos, M. G., de Oliveira, F. G., Casali, T. G., Bignoto, T. C., et al. (2014). Depressed cardiac autonomic modulation in patients with chronic kidney disease. Brazil. J. Nephrol. 36, 155–162. doi: 10.5935/0101-2800.20140025
Deligiannis, A., Kouidi, E., and Tourkantonis, A. (1999). Effects of physical training on heart rate variability in patients on hemodialysis. Am. J. Cardiol. 84, 197–202. doi: 10.1016/S0002-9149(99)00234-9
D’Souza, A., Trussell, T., Morris, G. M., Dobrzynski, H., and Boyett, M. R. (2019). Supraventricular arrhythmias in athletes: basic mechanisms and new directions. Physiology 34, 314–326. doi: 10.1152/physiol.00009.2019
Ector, J., Ganame, J., van der Merwe, N., Adriaenssens, B., Pison, L., Willems, R., et al. (2007). Reduced right ventricular ejection fraction in endurance athletes presenting with ventricular arrhythmias: a quantitative angiographic assessment. Eur. Heart J. 28, 345–353. doi: 10.1093/eurheartj/ehl468
Elliott, A. D., and La Gerche, A. (2015). The right ventricle following prolonged endurance exercise: are we overlooking the more important side of the heart? A meta-analysis. Br. J. Sports Med. 49, 724–729. doi: 10.1136/bjsports-2014-093895
Flethøj, M., Kanters, J. K., Haugaard, M. M., Pedersen, P. J., Carstensen, H., Balling, J. D., et al. (2016). Changes in heart rate, arrhythmia frequency, and cardiac biomarker values in horses during recovery after a long-distance endurance ride. J. Am. Vet. Med. Assoc. 248, 1034–1042. doi: 10.2460/javma.248.9.1034
Frick, L., Schwarzwald, C. C., and Mitchell, K. J. (2019). The use of heart rate variability analysis to detect arrhythmias in horses undergoing a standard treadmill exercise test. J. Vet. Intern. Med. 33, 212–224. doi: 10.1111/jvim.15358
Furberg, C. D., Psaty, B. M., Manolio, T. A., Gardin, J. M., Smith, V. E., and Rautaharju, P. M. (1994). Prevalence of atrial fibrillation in elderly subjects (the Cardiovascular Health Study). Am. J. Cardiol. 74, 236–241. doi: 10.1016/0002-9149(94)90363-8
Furlan, R., Guzzetti, S., Crivellaro, W., Dassi, S., Tinelli, M., Baselli, G., et al. (1990). Continuous 24-hour assessment of the neural regulation of systemic arterial pressure and RR variabilities in ambulant subjects. Circulation 81, 537–547. doi: 10.1161/01.cir.81.2.537
George, K., Whyte, G. P., Green, D. J., Oxborough, D., Shave, R. E., Gaze, D., et al. (2012). The endurance athletes heart: acute stress and chronic adaptation. Br. J. Sports Med. 46, (Suppl. 1), i29–i36. doi: 10.1136/bjsports-2012-091141
Georgiou, K., Larentzakis, A. V., Khamis, N. N., Alsuhaibani, G. I., Alaska, Y. A., and Giallafos, E. J. (2018). Can wearable devices accurately measure heart rate variability? A systematic review. Folia Med. 60, 7–20. doi: 10.2478/folmed-2018-0012
Guiraud, T., Labrunee, M., Gaucher-Cazalis, K., Despas, F., Meyer, P., Bosquet, L., et al. (2013). High-intensity interval exercise improves vagal tone and decreases arrhythmias in chronic heart failure. Med. Sci. Sports Exerc. 45, 1861–1867. doi: 10.1249/MSS.0b013e3182967559
Hartikainen, J., Malik, M., Staunton, A., Poloniecki, J., and Camm, A. (1996). Distinction between arrhythmic and nonarrhythmic death after acute myocardial infarction based on heart rate variability, signal-averaged electrocardiogram, ventricular arrhythmias and left ventricular ejection fraction. J. Am. College Cardiol. 28, 296–304. doi: 10.1016/0735-1097(96)00169-6
Hatch, F., Lancaster, M. K., and Jones, S. A. (2011). Aging is a primary risk factor for cardiac arrhythmias: disruption of intracellular Ca2 + regulation as a key suspect. Expert Rev. Cardiovasc. Ther. 9, 1059–1067. doi: 10.1586/erc.11.112
Herzig, D., Asatryan, B., Brugger, N., Eser, P., and Wilhelm, M. (2018). The association between endurance training and heart rate variability: the confounding role of heart rate. Front. Physiol. 9:756. doi: 10.3389/fphys.2018.00756
Herzig, D., Eser, P., Omlin, X., Riener, R., Wilhelm, M., and Achermann, P. (2017a). Reproducibility of heart rate variability is parameter and sleep stage dependent. Front. Physiol. 8:1100. doi: 10.3389/fphys.2017.01100
Herzig, D., Testorelli, M., Olstad, D. S., Erlacher, D., Achermann, P., Eser, P., et al. (2017b). Heart-rate variability during deep sleep in world-class alpine skiers: a time-efficient alternative to morning supine measurements. Int. J. Sports Physiol. Perform. 12, 648–654. doi: 10.1123/ijspp.2016-0257
Hower, I. M., Harper, S. A., and Buford, T. W. (2018). Circadian rhythms, exercise, and cardiovascular health. J. Circ. Rhythms 16:7. doi: 10.5334/jcr.164
Hynynen, E., Uusitalo, A., Konttinen, N., and Rusko, H. (2006). Heart rate variability during night sleep and after awakening in overtrained athletes. Med. Sci. Sports Exerc. 38, 313–317. doi: 10.1249/01.mss.0000184631.27641.b5
Kaikkonen, P., Nummela, A., and Rusko, H. (2007). Heart rate variability dynamics during early recovery after different endurance exercises. Eur. J. Appl. Physiol. 102, 79–86. doi: 10.1007/s00421-007-0559-8
Kim, H.-Y. (2018). Statistical notes for clinical researchers: covariance and correlation. Restor. Dent. Endod. 43:e4. doi: 10.5395/rde.2018.43.e4
Kim, J. L. J., and Shin, M. (2017). Sleep stage classification based on noise-reducued fractual property of heart rate variability. Proc. Comput. Sci. 116, 435–440.
Kleiger, R. E., Miller, J. P., Bigger, J. T. Jr., and Moss, A. J. (1987). Decreased heart rate variability and its association with increased mortality after acute myocardial infarction. Am. J. Cardiol. 59, 256–262. doi: 10.1016/0002-9149(87)90795-8
Kocovic, D., Harada, T., Shea, J., Soroff, D., and Friedman, P. (1993). Alterations of heart rate and of heart rate variability after radiofrequency catheter ablation of supraventricular tachycardia. Delineation of parasympathetic pathways in the human heart. Circulation 88, 1671–1681. doi: 10.1161/01.CIR.88.4.1671
La Rovere, M. T., Pinna, G. D., Maestri, R., Mortara, A., Capomolla, S., Febo, O., et al. (2003). Short-term heart rate variability strongly predicts sudden cardiac death in chronic heart failure patients. Circulation 107, 565–570. doi: 10.1161/01.cir.0000047275.25795.17
Laitio, T. T., Huikuri, H. V., Kentala, E. S., Mäkikallio, T. H., Jalonen, J. R., Helenius, H., et al. (2000). Correlation properties and complexity of perioperative RR-interval dynamics in coronary artery bypass surgery patients. Anesthesiology 93, 69–80. doi: 10.1097/00000542-200007000-00015
Lok, N.-S., and Lau, C.-P. (1996). Prevalence of palpitations, cardiac arrhythmias and their associated risk factors in ambulant elderly. Int. J. Cardiol. 54, 231–236. doi: 10.1016/0167-5273(96)02601-0
Lombardi, F., Colombo, A., Basilico, B., Ravaglia, R., Garbin, M., Vergani, D., et al. (2001). Heart rate variability and early recurrence of atrial fibrillation after electrical cardioversion. J. Am. Coll. Cardiol. 37, 157–162. doi: 10.1016/S0735-1097(00)01039-1
Lucini, D., Fede, G. D., Parati, G., and Pagani, M. (2005). Impact of chronic psychosocial stress on autonomic cardiovascular regulation in otherwise healthy subjects. Hypertension 46, 1201–1206. doi: 10.1161/01.HYP.0000185147.32385.4b
Malik, M., Camm, A. J., Bigger, J. T., Breithardt, G., Cerutti, S., Cohen, R. J., et al. (1996). Heart rate variabilty. Standards of measumernent, physiological interpretation and clinical use. Eur. Heart J. 17, 354–381. doi: 10.1093/oxfordjournals.eurheartj.a014868
Malliani, A., Pagani, M., Lombardi, F., and Cerutti, S. (1991). Cardiovascular neural regulation explored in the frequency domain. Circulation 84, 482–492.
Mannina, C., Jin, Z., Matsumoto, K., Ito, K., Biviano, A., Elkind, M. S. V., et al. (2021). Frequency of cardiac arrhythmias in older adults: findings from the subclinical atrial fibrillation and risk of ischemic stroke (SAFARIS) study. Int. J. Cardiol. 337, 64–70. doi: 10.1016/j.ijcard.2021.05.006
Marijon, E., Tafflet, M., Antero-Jacquemin, J., El Helou, N., Berthelot, G., Celermajer, D. S., et al. (2013). Mortality of French participants in the Tour de France (1947-2012). Eur. Heart J. 34, 3145–3150. doi: 10.1093/eurheartj/eht347
Michael, S., Graham, K., and Davis, G. (2017). Cardiac autonomic responses during exercise and post-exercise recovery using heart rate variability and systolic time intervals—a review. Front. Physiol. 8:301. doi: 10.3389/fphys.2017.00301
Michael, S., Jay, O., Halaki, M., Graham, K., and Davis, G. (2016). Submaximal exercise intensity modulates acute post-exercise heart rate variability. Eur. J. Appl. Physiol. 116, 697–706. doi: 10.1007/s00421-016-3327-9
Mont, L., Pelliccia, A., Sharma, S., Biffi, A., Borjesson, M., Brugada Terradellas, J., et al. (2020). Pre-participation cardiovascular evaluation for athletic participants to prevent sudden death: position paper from the EHRA and the EACPR, branches of the ESC. Endorsed by APHRS, HRS, and SOLAECE. Eur. J. Prevent. Cardiol. 24, 41–69. doi: 10.1177/2047487316676042
Myllymaki, T., Rusko, H., Syvaoja, H., Juuti, T., Kinnunen, M. L., and Kyrolainen, H. (2012). Effects of exercise intensity and duration on nocturnal heart rate variability and sleep quality. Eur. J. Appl. Physiol. 112, 801–809. doi: 10.1007/s00421-011-2034-9
Myrstad, M., Nystad, W., Graff-Iversen, S., Thelle, D. S., Stigum, H., Aarønæs, M., et al. (2014). Effect of years of endurance exercise on risk of atrial fibrillation and atrial flutter. Am. J. Cardiol. 114, 1229–1233. doi: 10.1016/j.amjcard.2014.07.047
Nolan, J., Batin, P. D., Andrews, R., Lindsay, S. J., Brooksby, P., Mullen, M., et al. (1998). Prospective study of heart rate variability and mortality in chronic heart failure: results of the United Kingdom heart failure evaluation and assessment of risk trial (UK-heart). Circulation 98, 1510–1516. doi: 10.1161/01.cir.98.15.1510
Nortamo, S., Ukkola, O., Kiviniemi, A., Tulppo, M., Huikuri, H., and Perkiömäki, J. S. (2018). Impaired cardiac autonomic regulation and long-term risk of atrial fibrillation in patients with coronary artery disease. Heart Rhythm 15, 334–340. doi: 10.1016/j.hrthm.2017.10.021
Piercy, K. L., Troiano, R. P., Ballard, R. M., Carlson, S. A., Fulton, J. E., Galuska, D. A., et al. (2018). The physical activity guidelines for Americans. JAMA 320, 2020–2028. doi: 10.1001/jama.2018.14854
Rajendra Acharya, U., Paul Joseph, K., Kannathal, N., Lim, C. M., and Suri, J. S. (2006). Heart rate variability: a review. Med. Biol. Eng. Comput. 44, 1031–1051. doi: 10.1007/s11517-006-0119-0
Rivest, L.-P. (1994). Statistical properties of Winsorized means for skewed distributions. Biometrika 81, 373–383. doi: 10.1093/biomet/81.2.373
Rizzo, M. R., Sasso, F. C., Marfella, R., Siniscalchi, M., Paolisso, P., Carbonara, O., et al. (2015). Autonomic dysfunction is associated with brief episodes of atrial fibrillation in type 2 diabetes. J. Diabetes Compl. 29, 88–92. doi: 10.1016/j.jdiacomp.2014.09.002
Sagnard, A., Guenancia, C., Mouhat, B., Maza, M., Fichot, M., Moreau, D., et al. (2020). Involvement of autonomic nervous system in new-onset atrial fibrillation during acute myocardial infarction. J. Clin. Med. 9:1481. doi: 10.3390/jcm9051481
Saponznikov, D., Luria, M. H., Mahler, Y., and Gotsman, M. S. (1992). Day vs night ECG and heart rate variability patterns in patients without obvious heart disease. J. Electrocardiol. 25, 175–184. doi: 10.1016/0022-0736(92)90002-H
Sareban, M., Guasch, E., Mont, L., and Niebauer, J. (2020). Endurance Exercise and Atrial Fibrillation–A State of the Art Review. German J. Sports Med. 71, 236–242. doi: 10.5960/dzsm.2020.462
Seidl, J., and Asplund, C. A. (2014). Effects of excessive endurance activity on the heart. Curr. Sports Med. Rep. 13, 361–364. doi: 10.1249/JSR.0000000000000097
Seiler, S., Haugen, O., and Kuffel, E. (2007). Autonomic recovery after exercise in trained athletes: intensity and duration effects. Med. Sci. Sports Exerc. 39, 1366–1373. doi: 10.1249/mss.0b013e318060f17d
Sharma, S. (2003). Athlete’s heart–effect of age, sex, ethnicity and sporting discipline. Exp. Physiol. 88, 665–669. doi: 10.1113/eph8802624
Smith, S. A. (1982). Reduced sinus arrhythmia in diabetic autonomic neuropathy: diagnostic value of an age-related normal range. Br. Med. J. 285, 1599–1601. doi: 10.1136/bmj.285.6355.1599
Stanley, J., Peake, J. M., and Buchheit, M. (2013). Cardiac parasympathetic reactivation following exercise: implications for training prescription. Sports Med. 43, 1259–1277. doi: 10.1007/s40279-013-0083-4
Swanson, D. R. (2006). Atrial fibrillation in athletes: implicit literature-based connections suggest that overtraining and subsequent inflammation may be a contributory mechanism. Med. Hypotheses. 66, 1085–1092. doi: 10.1016/j.mehy.2006.01.006
Task Force of the European Society of Cardiology and the North American Society of Pacing and Electrophysiology (1996). Heart rate variability. Circulation 93, 1043–1065. doi: 10.1161/01.CIR.93.5.1043
Tobaldini, E., Nobili, L., Strada, S., Casali, K. R., Braghiroli, A., and Montano, N. (2013). Heart rate variability in normal and pathological sleep. Front. Physiol. 4:294. doi: 10.3389/fphys.2013.00294
Tsuji, H., Larson, M. G., Venditti, F. J. Jr., Manders, E. S., Evans, J. C., Feldman, C. L., et al. (1996). Impact of reduced heart rate variability on risk for cardiac events. The Framingham Heart Study. Circulation 94, 2850–2855. doi: 10.1161/01.cir.94.11.2850
Williams, P. T., and Thompson, P. D. (2014). Increased cardiovascular disease mortality associated with excessive exercise in heart attack survivors. Mayo Clin. Proc. 89, 1187–1194. doi: 10.1016/j.mayocp.2014.05.006
Wundersitz, D., Williamson, J., Nadurata, V., Nolan, K., Lavie, C., and Kingsley, M. (2019). The impact of a 21-day ultra-endurance ride on the heart in young, adult and older adult recreational cyclists. Int. J. Cardiol. 286, 137–142. doi: 10.1016/j.ijcard.2019.03.016
Zehender, M., Meinertz, T., Keul, J., and Just, H. (1990). ECG variants and cardiac arrhythmias in athletes: clinical relevance and prognostic importance. Am. Heart J. 119, 1378–1391. doi: 10.1016/s0002-8703(05)80189-9
Zorzi, A., De Lazzari, M., Mastella, G., Niero, A., Trovato, D., Cipriani, A., et al. (2018a). Ventricular arrhythmias in young competitive athletes: prevalence, determinants, and underlying substrate. J. Am. Heart Assoc. 7:e009171. doi: 10.1161/jaha.118.009171
Zorzi, A., Mastella, G., Cipriani, A., Berton, G., Del Monte, A., Gusella, B., et al. (2018b). Burden of ventricular arrhythmias at 12-lead 24-hour ambulatory ECG monitoring in middle-aged endurance athletes versus sedentary controls. Eur. J. Prev. Cardiol. 25, 2003–2011. doi: 10.1177/2047487318797396
Keywords: sympathovagal balance, autonomic imbalance, cardiac, arrhythmia, endurance
Citation: Wundersitz DWT, Wright BJ, Gordon BA, Pompei S, Lavie CJ, Nadurata V, Nolan K and Kingsley MIC (2022) Sympathovagal Balance Is a Strong Predictor of Post High-Volume Endurance Exercise Cardiac Arrhythmia. Front. Physiol. 13:848174. doi: 10.3389/fphys.2022.848174
Received: 04 January 2022; Accepted: 14 February 2022;
Published: 21 March 2022.
Edited by:
Zbigniew Waśkiewicz, Jerzy Kukuczka Academy of Physical Education in Katowice, PolandReviewed by:
Robert Gajda, Grupa Gajda-Med. Medical Center, PolandKenichi Hashimoto, National Defense Medical College, Japan
Copyright © 2022 Wundersitz, Wright, Gordon, Pompei, Lavie, Nadurata, Nolan and Kingsley. This is an open-access article distributed under the terms of the Creative Commons Attribution License (CC BY). The use, distribution or reproduction in other forums is permitted, provided the original author(s) and the copyright owner(s) are credited and that the original publication in this journal is cited, in accordance with accepted academic practice. No use, distribution or reproduction is permitted which does not comply with these terms.
*Correspondence: Daniel W. T. Wundersitz, ZC53dW5kZXJzaXR6QGxhdHJvYmUuZWR1LmF1
†These authors have contributed equally to this work and share first authorship