- 1Department of Intervention Research in Exercise Training, German Sport University Cologne, Cologne, Germany
- 2Department of Sports Science, Bielefeld University, Bielefeld, Germany
This network meta-analysis aimed at evaluating the effectiveness of different velocity-based (VBT) and traditional 1RM-based resistance training (TRT) interventions on strength and power indices in healthy participants. The research was conducted until December 2021 using the online electronic databases PubMed, Web of Science, PsycNet, and SPORTDiscus for studies with the following inclusion criteria: 1) controlled VBT trials, 2) strength and/or jump and/or sprint parameters as outcomes (c), participants aged between 18 and 40 years, and 4) peer-reviewed and published in English. Standardized mean differences (SMD) using a random effects models were calculated. Fourteen studies with 311 healthy participants were selected and 3 networks (strength, jump, and sprint) were achieved. VBT, TRT, repetitions in reserve (RIR), low velocity loss (lowVL), and high velocity loss (highVL) were ranked for each network. Based on P-score rankings, lowVL (P-score ≥ 0.59; SMD ≥ 0.33) and highVL (P-score ≥ 0.50; SMD ≥ 0.12) revealed favorable effects on strength, jump, and sprint performance compared to VBT (P-score ≤ 0.47; SMD ≤0.01), TRT (P-score ≤0.46; SMD ≤ 0.00), and RIR (P-score ≤ 0.46; SMD ≤ 0.12). In conclusion, lowVL and highVL showed notable effects on strength, jump, and sprint performance. In particular for jump performance, lowVL induced favorable improvements compared to all other resistance training approaches.
Introduction
Resistance training is considered an important part of an athlete’s weekly training schedule in both individual and team sports (Healy et al., 2019; Drury et al., 2021). Besides morphological and functional adaptations at the muscular level (Grgic et al., 2021), relevant performance abilities such as strength, jumping, and sprinting ability relevantly benefit from adequate resistance training (Suchomel et al., 2016). Traditional resistance training mainly relies on training approaches based on a certain percentage of the one repetition maximum (1RM) or autoregulative (perceived exertion or reps in reserve) (Zourdos et al., 2016).
Within the last decades, velocity-based resistance training (VBT) as an alternative strength training approach has gained increasing attention (González-Badillo and Sánchez-Medina, 2010). During VBT the training intensity is controlled by monitoring the mean concentric velocity (MCV) of each repetition (González-Badillo and Sánchez-Medina, 2010) using inertial sensors (Held et al., 2021b) or linear position transducers (Held et al., 2021a). In general, the level of fatigue increases gradually as a function of increasing effort during a training set (Sánchez-Medina and González-Badillo, 2011). Therefore, a decreasing MCV across repetitions within a set provides a feasible, simple, and promising tool to clearly objectify levels of fatigue (Sánchez-Medina and González-Badillo, 2011). Hence, VBT allows the application of a homogeneous stimulus across individuals (Pareja-Blanco et al., 2020) and resistance training control without excessive exhaustion (Padulo et al., 2012). Further, employing the “two-point method” (García-Ramos et al., 2018), the load-velocity relationship enables an athlete’s 1RM prediction without applying maximum loads. Compared to traditional 1RM-based strength training with large within subject day-to-day variability (Kiely, 2012; Jovanović and Flanagan, 2014), this relationship has been reported to be load- and exercise-specific (Beck et al., 2020), but robust over long-term training progress (González-Badillo and Sánchez-Medina, 2010).
The term VBT covers a variety of approaches (Weakley et al., 2021), with the two main approaches employing velocity zones (i.e., completing a set of repetitions within a pre-defined individual or generic velocity range) or velocity loss thresholds (i.e., performing repetitions within a set until the velocity drops below a predefined threshold) (Orange et al., 2022). In this context, qualitative assessment of the literature in two recently published systematic reviews (Włodarczyk et al., 2021; Baena-Marín et al., 2022) indicates superior adaptational potential in terms of 1RM, sprinting, and jumping performance for VBT approaches employing a velocity loss threshold of 10–20%. Therefore, combining different VBT approaches (e.g., employing velocity zones and velocity loss thresholds) to allow comparison with traditional strength training methods in the context of a meta-analysis (Liao et al., 2021; Orange et al., 2022) may impede well-informed decision making for trainers, athletes, and practitioners in the field of velocity-based strength training due to the partial heterogeneity of the studies (Liao et al., 2021).
Hence, a network meta-analysis (NMA) that enables effect size estimation based on both direct and indirect study comparison can serve as a proper alternative to synthesize available evidence in the field of velocity-based strength training. Specifically, this method allows estimating comparative effects of treatment arms that have not been directly compared in randomized trials (Caldwell et al., 2005). Thus, employing a NMA allows the comparison of VBT approaches with different velocity loss thresholds or velocity zones against traditional strength training methods. Therefore, the present network meta-analysis aimed at examining and comparing the effects of different strength training interventions by distinguishing between traditional and velocity-based training approaches based on maximal and explosive performance indices. We hypothesized that the VBT approaches in general might lead to superior effects on relevant performance surrogates compared to traditional 1RM-based training. We furthermore assume that limiting the loss of MCV to a lower limit will improve speed strength performance adaptations. The overall results of this NMA might help coaches and researchers to better select their training regime based on the intended training adaptations.
Materials and methods
Search and screening procedures
This review was conducted in accordance with Preferred Reporting Items for Systematic Reviews and Meta-Analyses for Network Meta-Analyses (PRISMA-NMA) (Hutton et al., 2015). The literature search and screening were independently conducted by two researchers (KS and HB). Four health-related, biomedical, and psychological databases (PubMed, Web of Science, PsycNet and SPORTDiscus) were screened from inception of the respective journal until December 6, 2021. Relevant search terms (operators) were combined with Boolean conjunctions (OR/AND) and applied on two search levels (Table1).
The researchers also tracked cited articles and hand searched relevant primary articles and reviews. Duplicates were removed and the remaining studies underwent a manual screening. They were gradually screened using 1) the titles, 2) abstracts, and 3) full-texts of the potentially eligible articles. The final decision for inclusion or exclusion was made by two independent researchers (KS and SH). The following inclusion criteria based on the PICOS approach [population (P), intervention (I), comparators (C), main outcome (O), and study design (S)] were used: Full-text article published in English in a peer-reviewed journal; participants were healthy adults between 18 and 40 years (P), without any cognitive, neurological, orthopedic, and/or cardiac conditions that could affect physical testing and training; velocity-based training served as the interventional strategy of interest (I); active control group(s) that performed non velocity or velocity loss based resistance training and/or inactive control group(s) that did not receive any intervention served as a comparator (C); at least one outcome of strength (1RM), jump (CMJ or SJ), and sprint (10–30 m) performance had to be evaluated in the study (O); and prospective two- or multi-armed controlled intervention study with pre- and post-testing and more than 7 days duration (S). The exclusion criteria were: No adequate control conditions, which made integration into the network impossible.
Assessment of the methodological quality of included studies
The methodological quality (including risk of bias) of the included studies was independently rated using the PEDro (Physiotherapy Evidence Database) scale (Maher et al., 2003). The PEDro scale contains 11 dichotomous (yes or no) items, in which the criteria 2–9 rate randomization and internal validity and the criteria 10–11 rate the presence of statistically replicable results. Criterion 1 relates to external validity, but will not be used to calculate the PEDro score. Studies were rated independently by two non-blinded reviewers (KS and HB) who needed to obtain consensus on every item. Discordant study ratings were discussed point to point by two reviewers (KS and SH) who then came to a decision. To represent a high-quality study, the PEDro score had to be ≥6 on a scale from 0 to 10 (Maher et al., 2003).
Data extraction
Relevant data (required for calculating effect sizes) were extracted independently by two researchers (KS and SH) using a standardized extraction Excel spreadsheet adapted from the Cochrane Collaboration (Higgins et al., 2022). Means and standard deviations of pre-test and post-test scores on all strength, jump, and sprint related tests were extracted, along with the number of participants assessed in each group. If these point and variability measures were not reported in the full-text article, either the means and pooled within-group standard deviations of change scores were entered in an electronic spreadsheet or the authors of the article were contacted, and missing values were requested three times over the course of 2 months. If studies only presented means and standard deviations in figures, the WebPlotDigitizer Version 4 (Free Software Foundation, Boston, MA, United States) was used to extract means with standard deviations. For studies with multiple outcomes for a single neuromuscular test, the condition with the highest demand (as determined by two researchers) on the respective domain was extracted. In the case of multiple relevant neuromuscular tests, effect sizes and standard errors were pooled. Subsequently, all neuromuscular tests and outcomes were categorized into strength, jump, and sprint. In addition to these outcomes, relevant study information regarding author, year, number of participants, interventional data (weeks, frequency, duration per session, type of intervention), control condition, and PEDro scale scores were also recorded. For the simplification of the networks, similar treatments have been summarized into low velocity loss (LowVL), high velocity loss (HighVL), repetitions in reserve-based training (RIR), velocity-based resistance training (VBT), and traditional 1RM based resistance training (TRT). Thereby, LowVL and HighVL were defined as velocity losses of ≤20% and >20%, respectively.
Statistics
The standardized mean difference (SMD) and its 95% confidence intervals (95CI) were calculated for all the interventional treatments as a measure of treatment effectiveness. Thereby, SMDs were calculated as differences between means divided by the pooled standard deviations (trivial: SMD <0.2, small: 0.2 ≤ SMD <0.5, moderate: 0.5 ≤ SMD <0.8, large SMD ≥0.8) (Cohen, 1988). Subsequently, three separate network models for strength, sprint, and jump were computed. In order to visualize the networks, a network graph was created for each of the three networks. The estimations of treatment effects were calculated based on a random effects model (Senn, 2007). TRT was defined as reference treatment for the following network meta-analytical procedure. A ranking was created based on the P-score of the individual treatments. The P-score calculation following the surface under cumulative ranking (SUCRA) protocol of a treatment is obtained from estimating the effect sizes of pairwise treatment comparisons and presuming their point estimates are normally distributed. P-scores range from 0 to 100%, with 0 or 1 being the theoretically worst and best treatment, respectively, (Rücker and Schwarzer, 2015). P-scores in a frequentist NMA are analogous to the SUCRA (Mbuagbaw et al., 2017) values found in Bayesian NMA (Rücker and Schwarzer, 2015). Additionally, a forest plot was created to further visualize the ranking and effects of the treatments. The decomposed Q-statistics (within and between designs) were used to assess potential heterogeneity and inconsistency. Heterogeneity and inconsistency were further quantified by I2 (Higgins and Thompson, 2002). Funnel plots were created to check potential publication bias, whereby Egger’s test for asymmetry of the funnel plot (Egger et al., 1997) was used. R software (version 4.1.1; The R Foundation for Statistical Computing) and the package “netmeta” (Rücker et al., 2021) was used for all calculations and figures.
Results
Trial flow
In total 12,206 potentially relevant articles were initially found (Figure 1). After removing duplicates, 9,574 article titles and abstracts were carefully screened for relevance. The full-texts of the remaining 138 potentially relevant articles were thoroughly studied. Altogether, 125 papers were excluded as they did not meet the inclusion criteria or fulfilled the exclusion criteria. Consequently, quantitative and qualitative data were extracted from a final set of 13 articles (Table 2).
Study characteristics and quality
Included trials (311 healthy adults) consisted of 24 ± 11 participants per study (range 10 to 55) with an average age of 23.0 ± 1.7 years (range 19.6 to 26.0 years). The average study quality was high as indicated by a PEDro score of 5.9 ± 0.3 (range 5 to 6; Figure 2). Apart from one three armed design (Rodríguez-Rosell et al., 2021), most studies employed a two-armed design (Pareja-Blanco et al., 2017b; Pérez-Castilla et al., 2018; Dorrell et al., 2020; Galiano et al., 2020; Pareja-Blanco et al., 2020; Rodríguez-Rosell et al., 2020; Shattock and Tee, 2020; Held et al., 2021a; Andersen et al., 2021; Banyard et al., 2021; Jiménez-Reyes et al., 2021; Montalvo-Pérez et al., 2021).
Strength, jump, and sprint network
The strength, jump, and sprint networks revealed low heterogeneity and non-significant heterogeneity inconsistency (I2 and Q statistics; Figure 3). The funnel plot evaluations and non-significant Egger’s tests revealed no risk of bias for the strength, jump, and sprint network (Figure 4). Figures 2, 3 visualize the ranking of treatments and the pairwise comparison, respectively. In the strength network (Figure 3A), data from 13 studies (276 participants) representing 15 (pairwise comparison) effect sizes were included. The most common comparison was LowVL vs. HighVL (n = 7), followed by VBT vs. TRT (n = 4), and LowVL vs. TRT (n = 2). The jump network (Figure 3B) is based on 10 studies (220 participants) representing 10 (pairwise comparison) effect sizes. The most common comparison was LowVL vs. HighVL (n = 6), followed by VBT vs. TRT (n = 3). The sprint network (Figure 3C) contained data from 9 studies (204 participants), representing 9 (pairwise comparison) effect sizes. The most common comparison was LowVL vs. HighVL (n = 6), followed by VBT vs. TRT (n = 2).
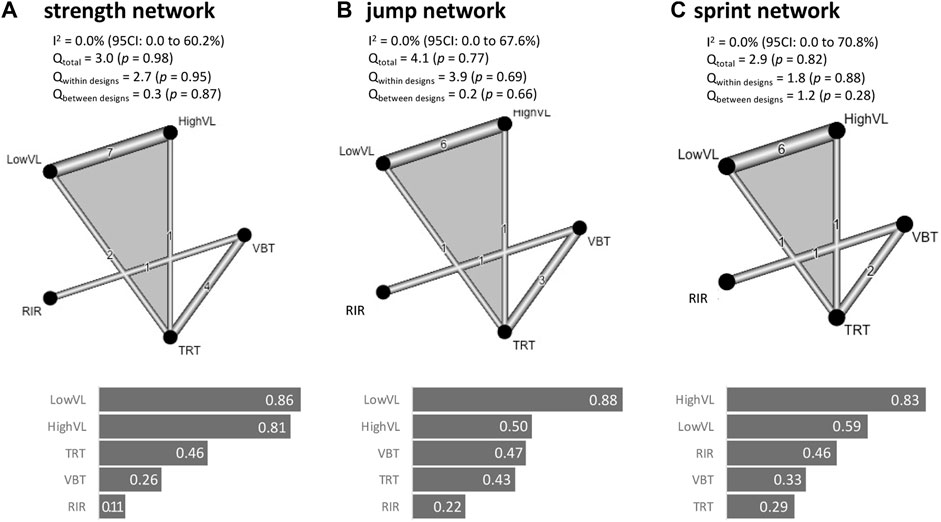
FIGURE 3. Network plots of the strength (A), jump (B), and sprint (C) network. In addition, I2, Q statistic, and P-score rankings are given. LowVL: low velocity loss (≤20%); HighVL: high velocity loss (>20%); RIR: repetitions in reserve-based training; VBT: velocity-based resistance training; TRT: traditional 1RM based resistance training (TRT).
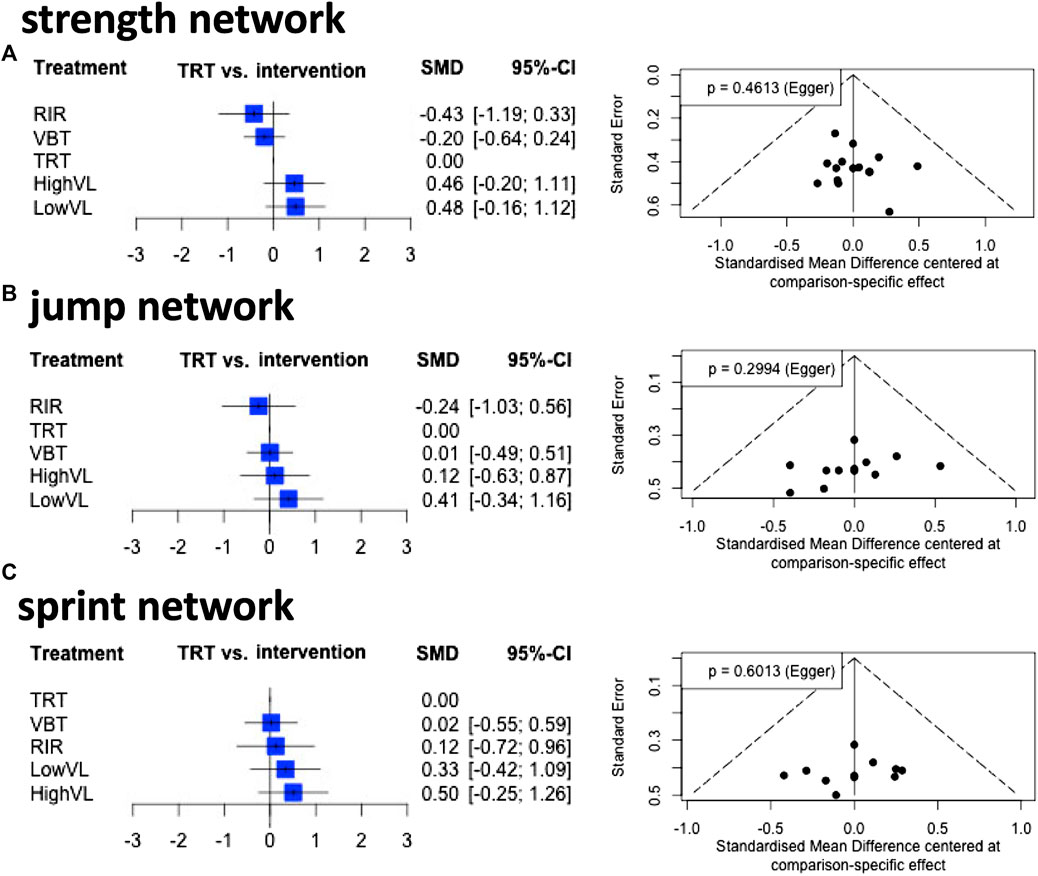
FIGURE 4. Forest and funnel plots for the strength (A), jump (B), and sprint (C) network. In addition, Egger´s p scores are given. LowVL: low velocity loss (≤20%); HighVL: high velocity loss (>20%); RIR: repetitions in reserve-based training; VBT: velocity-based resistance training; TRT: traditional 1RM based resistance training (TRT).
Discussion
This is the first network meta-analytical review that investigated and compared the effects of different velocity-based resistance training approaches with traditional 1RM-based resistance training settings. Interestingly, (high and low) velocity loss based resistance training approaches ranked (based on P-scores) consistently at the top two for the strength, jump, and sprint network, respectively. All three networks revealed small to moderate positive effects for both high and low velocity-loss-based resistance training approaches compared to a traditional resistance training approach. Other velocity based resistance training approaches ranked consistently between third and fourth place according to the P-score ranking of the strength, jump, and sprint network. Thereby, these other velocity based resistance training approaches revealed small negative to trivial positive effects on strength, jump, and sprint performance. Traditional 1RM and repetitions in reserve based resistance training approaches ranked between third and fifth, with moderate negative to trivial positive effects on strength, jump, and sprint performance.
A recent meta-analytical review (Liao et al., 2021) revealed similar effects of VBT and TRF on strength performance (MD = 3.03 kg; 95% CI: −3.55, 9.61; I2 = 0%), despite lower training volume during VBT approaches. Based on P-scores our findings revealed favorable effects of both high and low velocity loss based resistance training approaches (on strength performance) compared to traditional 1RM or RIR based resistance training approaches. Overall, traditional 1RM based resistance training to failure does not necessarily lead to higher strength gains (Izquierdo-Gabarren et al., 2009). In addition, recent findings revealed reduced training induced stress and less need of recovery via low velocity loss based resistance training (Held et al., 2021a). This finding has been confirmed by other scholars (Sánchez-Medina and González-Badillo, 2011), who observed a high correlation between increasing velocity loss and mechanical or metabolic stress. Furthermore, neuromuscular performance recovers faster post low velocity loss resistance training rather than post high velocity loss approaches (Pareja-Blanco et al., 2019).
In line with a previous systematic review (Włodarczyk et al., 2021), we observed (based on P-scores) favorable effects of low velocity loss approaches on jump performance adaptations. In this context, high velocity loss approaches seem to have negative effects on the type IIX muscle fibers (Pareja-Blanco et al., 2017a). While 8 weeks of low velocity loss based resistance training did not induce a reduction in type IIX fiber content (of the m. vastus lateralis), high velocity loss based resistance training produced a significant reduction in type IIX content (Pareja-Blanco et al., 2017a). In particular, the rate of force development dependent jump performance (McLellan et al., 2011; Laffaye et al., 2014; Maffiuletti et al., 2016) has been attributed at the proportion of IIX fibers (Andersen et al., 2010). Confirming these previous findings, Martinez-Canton and colleagues (Martinez-Canton et al., 2021) revealed increased CAMKII (calmodulin kinase II) activity via high velocity loss training, which has been linked to a decrease in IIX isoforms through a change in calcium handling (Tavi and Westerblad, 2011; Summermatter et al., 2012; Gehlert et al., 2015). Therefore, Pareja-Blanco and colleagues (Pareja-Blanco et al., 2017b) concluded in line with our findings that an optimal velocity loss ranges from 10 to 20% for jump performance developments.
While Pareja-Blanco and colleagues (Pareja-Blanco et al., 2017b) emphasize the benefits of low VL for sprint performance adaptations, a recent meta-analytic review (Liao et al., 2021) indicates comparable sprint performance adaptations at low and high velocity loss approaches (MD = 0.01 s; 95% CI: -0.06, 0.07; I2 = 0%). In contrast, the high velocity loss approach scores best in our P-score ranking. However, there is a large overlap in the variances of the low and high velocity loss approaches, which makes it difficult to clearly distinguish between them in terms of effectiveness. Nevertheless, our ranking shows a substantial gap between both low and high velocity loss approaches and RIR, VBT, and TRT, which again highlights the value of velocity loss based resistance training.
Apart from high P-scores, the low and the high velocity loss approaches revealed only small positive mean effects compared to a traditional resistance training approach. Furthermore, 95% confidence intervals revealed small negative (−0.42) to large positive (+1.26) strength, jump and sprint adaptation effects compared to a traditional resistance training approach. Nevertheless, considering the lower loads and the resulting reduced needs for recovery associated with lower velocity loss training (Sánchez-Medina and González-Badillo, 2011; Pareja-Blanco et al., 2019; Held et al., 2021a), these small effects are relevant for athletes and coaches in a short and long term perspectives on the training process. However, a corresponding sample size estimation for a randomized crossover trail (paired t-test; SMD = 0.41; α error = 0.05; power (1-β error) = 0.90). using G*Power (Version 3.1.9.6) (Faul et al., 2007) revealed a required sample size ≥50 participants, which is substantially above the mean sample size of the included studies of this network meta-analyses. Therefore, future research on velocity loss approaches should consider larger sample sizes or individualized approaches with more frequent repeated measures (Hecksteden et al., 2015, 2018). based on single-subject designs (Hecksteden et al., 2015, 2018) and/or Bayesian statistics using informative priors (Hecksteden et al., 2022) could tackle these sample sized related limitations (Hecksteden et al., 2021).
A limitation of the current network meta-analysis is that only 14 studies could be identified that met our inclusion criteria. These stringent inclusion criteria were necessary to achieve homogeneous networks. Furthermore, this decision is confirmed by the finding that our network models show no evidence of heterogeneity or inconsistency. Nevertheless, the used network meta-analytical approach enables effect size estimation based on both direct and indirect study comparison (Rücker and Schwarzer, 2015). Therefore, our results are in line with previous reviews and meta-analyses (Liao et al., 2021; Włodarczyk et al., 2021), despite the aforementioned limitations.
This network meta-analytical review revealed that (high and low) velocity loss based resistance training approaches revealed favorable effects on strength, jump, and sprint performance compared to other velocity based, traditional 1RM, and repetitions in reserve based resistance training approaches. In particular for jump performance, low velocity loss resistance training approaches induced favorable improvements compared to all other resistance training approaches. Overall, these findings indicate that the choice of resistance training approach and in particular the level of velocity loss has an impact on the magnitude of the effects and should, therefore, be carefully considered by coaches and athletes. In particular, velocity loss based approaches seem beneficial for increasing strength, jump and sprint performances. In particular, low velocity loss within a resistance training seems to be beneficial for strength and jump performance adaptations. In contrast, sprint performance seems to benefit from both low and high velocity loss based resistance training. In this context, future research should focus on the optimal amount of this velocity loss.
Data availability statement
The original contributions presented in the study are included in the article/Supplementary Material, further inquiries can be directed to the corresponding author.
Author contributions
SH and LD contributed to conception and design of the study. KS conducted the literature search. SH and KS organized the database. SH and LR performed the statistical analysis. SH wrote the first draft of the manuscript. LD, PW, KS, and LR wrote sections of the manuscript. PW copyedited the draft for content, language, and format, and organized the submission and revision/resubmission process. All authors contributed to manuscript revision, read, and approved the submitted version.
Funding
This study was financially supported by the German Federal Institute of Sports Science (BISp) under Grant (ZMVI4-070513/ 19-20). Further, we acknowledge the financial support of the German Research Foundation (DFG) and the Open Access Publication Fund of Bielefeld University for the article processing charge.
Acknowledgments
We acknowledge Hagen Bierlich (HB) for his help during the study selection process.
Conflict of interest
The authors declare that the research was conducted in the absence of any commercial or financial relationships that could be construed as a potential conflict of interest.
Publisher’s note
All claims expressed in this article are solely those of the authors and do not necessarily represent those of their affiliated organizations, or those of the publisher, the editors and the reviewers. Any product that may be evaluated in this article, or claim that may be made by its manufacturer, is not guaranteed or endorsed by the publisher.
References
Andersen L. L., Andersen J. L., Zebis M. K., Aagaard P. (2010). Early and late rate of force development: Differential adaptive responses to resistance training? Scand. J. Med. Sci. Sports 20, e162–e169. doi:10.1111/j.1600-0838.2009.00933.x
Andersen V., Paulsen G., Stien N., Baarholm M., Seynnes O., Saeterbakken A. H., et al. (2021). Resistance training with different velocity loss thresholds induce similar changes in strengh and hypertrophy. J. Strength Cond. Res. Publish Ahead of Print. doi:10.1519/JSC.0000000000004067
Baena-Marín M., Rojas-Jaramillo A., González-Santamaría J., Rodríguez-Rosell D., Petro J. L., Kreider R. B., et al. (2022). Velocity-based resistance training on 1-RM, jump and sprint performance: A systematic review of clinical trials. Sports (Basel) 10, 8. doi:10.3390/sports10010008
Banyard H. G., Tufano J. J., Weakley J. J. S., Wu S., Jukic I., Nosaka K., et al. (2021). Superior changes in jump, sprint, and change-of-direction performance but not maximal strength following 6 Weeks of velocity-based training compared with 1-repetition-maximum percentage-based training. Int. J. Sports Physiol. Perform. 16, 232–242. doi:10.1123/ijspp.2019-0999
Beck M., Varner W., LeVault L., Boring J., Fahs C. A. (2020). Decline in unintentional lifting velocity is both load and exercise specific. J. Strength Cond. Res. 34, 2709–2714. doi:10.1519/JSC.0000000000003786
Caldwell D. M., Ades A. E., Higgins J. P. T. (2005). Simultaneous comparison of multiple treatments: Combining direct and indirect evidence. BMJ 331, 897–900. doi:10.1136/bmj.331.7521.897
Cohen J. (1988). Statistical power analysis for the behavioral sciences. New York, NY, USA: Routledge.
Dorrell H. F., Smith M. F., Gee T. I. (2020). Comparison of velocity-based and traditional percentage-based loading methods on maximal strength and power adaptations. J. Strength Cond. Res. 34, 46–53. doi:10.1519/JSC.0000000000003089
Drury B., Clarke H., Moran J., Fernandes J. F. T., Henry G., Behm D. G., et al. (2021). Eccentric resistance training in youth: A survey of perceptions and current practices by strength and conditioning coaches. J. Funct. Morphol. Kinesiol. 6, 21. doi:10.3390/jfmk6010021
Egger M., Smith G. D., Schneider M., Minder C. (1997). Bias in meta-analysis detected by a simple, graphical test. BMJ 315, 629–634. doi:10.1136/bmj.315.7109.629
Faul F., Erdfelder E., Lang A.-G., Buchner A. (2007). G*Power 3: A flexible statistical power analysis program for the social, behavioral, and biomedical sciences. Behav. Res. Methods 39, 175–191. doi:10.3758/bf03193146
Galiano C., Pareja-Blanco F., Hidalgo de Mora J., Sáez de Villarreal E. (2020). Low-velocity loss induces similar strength gains to moderate-velocity loss during resistance training. J. Strength Cond. Res. 36, 340–345. Ahead of Print. doi:10.1519/JSC.0000000000003487
García-Ramos A., Haff G. G., Pestaña-Melero F. L., Pérez-Castilla A., Rojas F. J., Balsalobre-Fernández C., et al. (2018). Feasibility of the 2-point method for determining the 1-repetition maximum in the bench press exercise. Int. J. Sports Physiol. Perform. 13, 474–481. doi:10.1123/ijspp.2017-0374
Gehlert S., Bloch W., Suhr F. (2015). Ca2+-Dependent regulations and signaling in skeletal muscle: From electro-mechanical coupling to adaptation. Int. J. Mol. Sci. 16, 1066–1095. doi:10.3390/ijms16011066
González-Badillo J. J., Sánchez-Medina L. (2010). Movement velocity as a measure of loading intensity in resistance training. Int. J. Sports Med. 31, 347–352. doi:10.1055/s-0030-1248333
Grgic J., Schoenfeld B. J., Orazem J., Sabol F. (2021). Effects of resistance training performed to repetition failure or non-failure on muscular strength and hypertrophy: A systematic review and meta-analysis. J. Sport Health Sci. 11, 202–211. S2095254621000077. doi:10.1016/j.jshs.2021.01.007
Healy R., Kenny I. C., Harrison A. J. (2019). Resistance training practices of sprint coaches. J. Strength Cond. Res. 35, 1939–1948. doi:10.1519/JSC.0000000000002992
Hecksteden A., Forster S., Egger F., Buder F., Kellner R., Meyer T., et al. (2022). Dwarfs on the shoulders of giants: Bayesian analysis with informative priors in elite sports research and decision making. Front. Sports Act. Living 4, 793603. doi:10.3389/fspor.2022.793603
Hecksteden A., Kellner R., Donath L. (2021). Dealing with small samples in football research. Sci. Med. Footb. 0, 1–9. doi:10.1080/24733938.2021.1978106
Hecksteden A., Kraushaar J., Scharhag-Rosenberger F., Theisen D., Senn S., Meyer T., et al. (2015). Individual response to exercise training - a statistical perspective. J. Appl. Physiol. 118, 1450–1459. doi:10.1152/japplphysiol.00714.2014
Hecksteden A., Pitsch W., Rosenberger F., Meyer T. (2018). Repeated testing for the assessment of individual response to exercise training. J. Appl. Physiol. 124, 1567–1579. doi:10.1152/japplphysiol.00896.2017
Held S., Hecksteden A., Meyer T., Donath L. (2021a). Improved strength and recovery after velocity-based training: A randomized controlled trial. Int. J. Sports Physiol. Perform. 16, 1185–1193. doi:10.1123/ijspp.2020-0451
Held S., Rappelt L., Deutsch J.-P., Donath L. (2021b). Valid and reliable barbell velocity estimation using an inertial measurement unit. Int. J. Environ. Res. Public Health 18, 9170. doi:10.3390/ijerph18179170
Higgins J. P. T., Thompson S. G. (2002). Quantifying heterogeneity in a meta-analysis. Stat. Med. 21, 1539–1558. doi:10.1002/sim.1186
Higgins J. P. T., Thomas J., Chandler J., Cumpston M., Li T., Page M. J., et al. (Editors) (2022). Cochrane handbook for systematic reviews of interventions version 6.3. Available at: www.training.cochrane.org/handbook.
Izquierdo-Gabarren M., González de Txabarri Expósito R., García-Pallarés J., Sánchez-Medina L., Sáez de Villarreal E. S., Izquierdo M., et al. (2009). Concurrent endurance and strength training not to failure optimizes performance gains. Med. Sci. Sports Exerc. 42, 1191–1199. doi:10.1249/MSS.0b013e3181c67eec
Jiménez-Reyes P., Castaño-Zambudio A., Cuadrado-Peñafiel V., González-Hernández J. M., Capelo-Ramírez F., Martínez-Aranda L. M., et al. (2021). Differences between adjusted vs. non-adjusted loads in velocity-based training: Consequences for strength training control and programming. PeerJ 9, e10942. doi:10.7717/peerj.10942
Jovanović M., Flanagan D. E. P. (2014). Researched applications of velocity based strength training. 21, 12.
Kiely J. (2012). Periodization paradigms in the 21st century: Evidence-led or tradition-driven? Int. J. Sports Physiol. Perform. 7, 242–250. doi:10.1123/ijspp.7.3.242
Laffaye G., Wagner P. P., Tombleson T. I. L. (2014). Countermovement jump height: Gender and sport-specific differences in the force-time variables. J. Strength Cond. Res. 28, 1096–1105. doi:10.1519/JSC.0b013e3182a1db03
Liao K.-F., Wang X.-X., Han M.-Y., Li L.-L., Nassis G. P., Li Y.-M., et al. (2021). Effects of velocity based training vs. traditional 1RM percentage-based training on improving strength, jump, linear sprint and change of direction speed performance: A systematic review with meta-analysis. PLoS One 16, e0259790. doi:10.1371/journal.pone.0259790
Maffiuletti N. A., Aagaard P., Blazevich A. J., Folland J., Tillin N., Duchateau J., et al. (2016). Rate of force development: Physiological and methodological considerations. Eur. J. Appl. Physiol. 116, 1091–1116. doi:10.1007/s00421-016-3346-6
Maher C. G., Sherrington C., Herbert R. D., Moseley A. M., Elkins M. (2003). Reliability of the PEDro scale for rating quality of randomized controlled trials. Phys. Ther. 83, 713–721. doi:10.1093/ptj/83.8.713
Martinez‐Canton M., Gallego‐Selles A., Gelabert‐Rebato M., Martin‐Rincon M., Pareja‐Blanco F., Rodriguez‐Rosell D., et al. (2021). Role of CaMKII and sarcolipin in muscle adaptations to strength training with different levels of fatigue in the set. Scand. J. Med. Sci. Sports 31, 91–103. doi:10.1111/sms.13828
Mbuagbaw L., Rochwerg B., Jaeschke R., Heels-Andsell D., Alhazzani W., Thabane L., et al. (2017). Approaches to interpreting and choosing the best treatments in network meta-analyses. Syst. Rev. 6, 79. doi:10.1186/s13643-017-0473-z
McLellan C. P., Lovell D. I., Gass G. C. (2011). The role of rate of force development on vertical jump performance. J. Strength Cond. Res. 25, 379–385. doi:10.1519/JSC.0b013e3181be305c
Montalvo-Pérez A., Alejo L. B., Valenzuela P. L., Gil-Cabrera J., Talavera E., Lucia A., et al. (2021). Traditional versus velocity-based resistance training in competitive female cyclists: A randomized controlled trial. Front. Physiol. 12, 586113. doi:10.3389/fphys.2021.586113
Orange S. T., Hritz A., Pearson L., Jeffries O., Jones T. W., Steele J., et al. (2022). Comparison of the effects of velocity-based vs. traditional resistance training methods on adaptations in strength, power, and sprint speed: A systematic review, meta-analysis, and quality of evidence appraisal. J. Sports Sci. 0, 1–15. doi:10.1080/02640414.2022.2059320
Padulo J., Mignogna P., Mignardi S., Tonni F., D’Ottavio S. (2012). Effect of different pushing speeds on bench press. Int. J. Sports Med. 33, 376–380. doi:10.1055/s-0031-1299702
Pareja-Blanco F., Alcazar J., Sánchez-Valdepeñas J., Cornejo-Daza P. J., Piqueras-Sanchiz F., Mora-Vela R., et al. (2020). Velocity loss as a critical variable determining the adaptations to strength training. Med. Sci. Sports Exerc. 52, 1752–1762. doi:10.1249/MSS.0000000000002295
Pareja-Blanco F., Rodríguez-Rosell D., Sánchez-Medina L., Sanchis-Moysi J., Dorado C., Mora-Custodio R., et al. (2017a). Effects of velocity loss during resistance training on athletic performance, strength gains and muscle adaptations. Scand. J. Med. Sci. Sports 27, 724–735. doi:10.1111/sms.12678
Pareja-Blanco F., Sánchez-Medina L., Suárez-Arrones L., González-Badillo J. J. (2017b). Effects of velocity loss during resistance training on performance in professional soccer players. Int. J. Sports Physiol. Perform. 12, 512–519. doi:10.1123/ijspp.2016-0170
Pareja-Blanco F., Villalba-Fernández A., Cornejo-Daza P., Sánchez-Valdepeñas J., González-Badillo J. (2019). Time course of recovery following resistance exercise with different loading magnitudes and velocity loss in the set. Sports 7, 59. doi:10.3390/sports7030059
Pérez-Castilla A., García-Ramos A., Padial P., Morales-Artacho A. J., Feriche B. (2018). Effect of different velocity loss thresholds during a power-oriented resistance training program on the mechanical capacities of lower-body muscles. J. Sports Sci. 36, 1331–1339. doi:10.1080/02640414.2017.1376900
Rodríguez-Rosell D., Yáñez-García J. M., Sánchez-Medina L., Mora-Custodio R., González-Badillo J. J. (2020). Relationship between velocity loss and repetitions in reserve in the bench press and back squat exercises. J. Strength Cond. Res. 34, 2537–2547. doi:10.1519/JSC.0000000000002881
Rodríguez‐Rosell D., Yáñez‐García J. M., Mora‐Custodio R., Sánchez‐Medina L., Ribas‐Serna J., González‐Badillo J. J., et al. (2021). Effect of velocity loss during squat training on neuromuscular performance. Scand. J. Med. Sci. Sports 31, 1621–1635. doi:10.1111/sms.13967
Rücker G., Krahn U., König J., Efthimiou O., Schwarzer G. (2021). netmeta: Network meta-analysis using frequentist methods. Available at: https://cran.r-project.org/web/packages/netmeta/index.html.
Rücker G., Schwarzer G. (2015). Ranking treatments in frequentist network meta-analysis works without resampling methods. BMC Med. Res. Methodol. 15, 58. doi:10.1186/s12874-015-0060-8
Sánchez-Medina L., González-Badillo J. J. (2011). Velocity loss as an indicator of neuromuscular fatigue during resistance training. Med. Sci. Sports Exerc. 43, 1725–1734. doi:10.1249/MSS.0b013e318213f880
Senn S. (2007). Trying to be precise about vagueness. Stat. Med. 26, 1417–1430. doi:10.1002/sim.2639
Shattock K., Tee J. C. (2020). Autoregulation in Resistance Training: A Comparison of Subjective Versus Objective Methods, 8.
Suchomel T. J., Nimphius S., Stone M. H. (2016). The importance of muscular strength in athletic performance. Sports Med. 46, 1419–1449. doi:10.1007/s40279-016-0486-0
Summermatter S., Thurnheer R., Santos G., Mosca B., Baum O., Treves S., et al. (2012). Remodeling of calcium handling in skeletal muscle through PGC-1α: Impact on force, fatigability, and fiber type. Am. J. Physiol. Cell Physiol. 302, C88–C99. doi:10.1152/ajpcell.00190.2011
Tavi P., Westerblad H. (2011). The role of in vivo Ca 2+ signals acting on Ca 2+ -calmodulin-dependent proteins for skeletal muscle plasticity: Ca 2+ signals and Ca 2+ decoders in skeletal muscle. J. Physiol. 589, 5021–5031. doi:10.1113/jphysiol.2011.212860
Weakley J., Mann B., Banyard H., McLaren S., Scott T., Garcia-Ramos A., et al. (2021). Velocity-based training: From theory to application. Strength Cond. J. 43, 31–49. doi:10.1519/SSC.0000000000000560
Włodarczyk M., Adamus P., Zieliński J., Kantanista A. (2021). Effects of velocity-based training on strength and power in elite athletes—a systematic review. Int. J. Environ. Res. Public Health 18, 5257. doi:10.3390/ijerph18105257
Keywords: power-based training, maximum strength, counter movement jump, squat jump, mean concentric velocity
Citation: Held S, Speer K, Rappelt L, Wicker P and Donath L (2022) The effectiveness of traditional vs. velocity-based strength training on explosive and maximal strength performance: A network meta-analysis. Front. Physiol. 13:926972. doi: 10.3389/fphys.2022.926972
Received: 23 April 2022; Accepted: 11 July 2022;
Published: 10 August 2022.
Edited by:
Brennan J. Thompson, Utah State University, United StatesReviewed by:
Christos Paizis, Université de Bourgogne, FranceThierry Busso, Université Jean Monnet, France
Copyright © 2022 Held, Speer, Rappelt, Wicker and Donath. This is an open-access article distributed under the terms of the Creative Commons Attribution License (CC BY). The use, distribution or reproduction in other forums is permitted, provided the original author(s) and the copyright owner(s) are credited and that the original publication in this journal is cited, in accordance with accepted academic practice. No use, distribution or reproduction is permitted which does not comply with these terms.
*Correspondence: Pamela Wicker, cGFtZWxhLndpY2tlckB1bmktYmllbGVmZWxkLmRl