- 1Laboratorio de Genética de la Conservación, Jardín Botánico, Instituto de Biología, Universidad Nacional Autónoma de México, Ciudad Universitaria, Coyoacán, Mexico
- 2CONACYT/CONABIO, Comisión Nacional para el Conocimiento y Uso de la Biodiversidad, Tlalpan, Mexico
- 3Departamento de Ecología Evolutiva, Instituto de Ecología, Universidad Nacional Autónoma de México, Ciudad Universitaria, Coyoacán, Mexico
In Mexico's territory, the center of origin and domestication of maize (Zea mays), there is a large phenotypic diversity of this crop. This diversity has been classified into “landraces.” Previous studies have reported that genomic variation in Mexican maize is better explained by environmental factors, particularly those related with altitude, than by landrace. Still, landraces are extensively used by agronomists, who recognize them as stable and discriminatory categories for the classification of samples. In order to investigate the genomic foundation of maize landraces, we analyzed genomic data (35,909 SNPs from Illumina MaizeSNP50 BeadChip) obtained from 50 samples representing five maize landraces (Comiteco, Conejo, Tehua, Zapalote Grande, and Zapalote Chico), and searched for markers suitable for landrace assignment. Landrace clusters could not be identified taking all the genomic information, but they become manifest taking only a subset of SNPs with high FST among landraces. Discriminant analysis of principal components was conducted to classify samples using SNP data. Two classification analyses were done, first classifying samples by landrace and then by altitude category. Through this classification method, we identified 20 landrace-informative SNPs and 14 altitude-informative SNPs, with only 6 SNPs in common for both analyses. These results show that Mexican maize phenotypic diversity can be classified in landraces using a small number of genomic markers, given the fact that landrace genomic diversity is influenced by environmental factors as well as artificial selection due to bio-cultural practices.
1. Introduction
Maize (Zea mays) is grown in most of Mexico under contrasting environmental conditions, from tropical rainforests to arid semideserts, and by farmers from a variety of cultural backgrounds (Boege, 2008; Ruiz Corral et al., 2013). Therefore, the phenotypic and genetic diversity of maize landraces is influenced by a multiplicity of environmental and bio-cultural factors. Environmental factors are mainly correlated to temperature and rainfall, while bio-cultural variables relate to socioeconomic factors and the distribution of ethnic diversity (Boege, 2008; Perales and Golicher, 2014). As a consequence, there is a large diversity within and among landraces. This generates doubts about the genomic basis for landrace classification, even though maize racial categories are widely used by agronomists. Here, we aim to distinguish between natural and artificial selection as drivers of genomic variation among landraces. This is a challenge rarely addressed, but necessary for safeguarding maize diversity considering the factors affecting maize genetic diversity, as well as for rationally guiding crop improvement in the face of changing environmental conditions.
Maize landraces can be defined as dynamic populations with a historical origin and distinct identity, and which are often genetically diverse, locally adapted, and associated with a set of farmers' practices of seed selection and field management as well as with traditional knowledge (Camacho-Villa et al., 2015). Mexican maize diversity was described and classified into landraces during the twentieth century. Nowadays, CONABIO (Mexican National Commission for the Knowledge and use of Biodiversity) recognizes 64 landraces existing in Mexico, clustered in seven racial groups or complexes (CONABIO, 2011).
Recent studies on Mexican maize genomic diversity have found that the distribution of genetic variation is better explained by environmental variables governed by altitude and latitude than by landrace identity (van Heerwaarden et al., 2011; Breña Ochoa, 2013; Arteaga et al., 2015; Romero Navarro et al., 2017). Maize is grown throughout Mexico along an altitude gradient spanning 0–2,700 masl. This wide range in altitude correlates with a substantial range in temperature and moisture gradients, which are associated with local adaptation of maize populations growing at different altitudes (Pressoir and Berthaud, 2004; Mercer et al., 2008; Ruiz-Corral et al., 2008; Romero Navarro et al., 2017). Agronomically, local adaptation implies high levels of genotype-by-environment interactions, making it difficult to grow landraces in altitudes different from their original ones (Perales et al., 2003; Mercer et al., 2008; Lorant et al., 2017). Another factor influencing altitudinal differentiation is gene flow between domestic maize and populations of its wild ancestors (teosintes Z. m. ssp. mexicana and Z. m. ssp. parviglumis). Teosinte subspecies have allopatric distributions, Z. m. ssp. mexicana growing in highlands and Z. m. ssp. parviglumis growing in lowlands. Highland maize populations' genomic constitution is notably influenced by teosinte mexicana growing sympatrically, as there are high levels of gene flow among them (van Heerwaarden et al., 2011; Hufford et al., 2013; Lorant et al., 2017; Romero Navarro et al., 2017). Taken together, these facts account for the correlation between genetic variation and altitude.
Morphologically different populations of maize present low levels of genotypic differentiation, revealing that phenotypic and genetic variation do not correlate (Pressoir and Berthaud, 2004; Arteaga et al., 2015). This fact suggests that phenotypic diversity in maize is the result of a complex process and cannot be explained only by genetic factors. Genetic homogeneity among maize populations is explained by the fact that these populations are open systems with high levels of gene flow through open pollination and seed exchange by farmers (Dyer and Taylor, 2008; Orozco-Ramírez et al., 2016). Still, the high degree of variability within and among landraces is associated with the selection by smallholders of different parts of the plant, the different environmental conditions in which it is grown, and in general the different agroecological practices people growing maize have (Bellon et al., 2009; Orozco-Ramírez et al., 2016). Despite the complex genetic constitution of maize populations, landraces are phenotypically distinguishable, suggesting that there must be a genetic basis underlying phenotypic diversity. This apparent paradox could be explained by farmers selecting for genes with major and pleiotropic effects (Pressoir and Berthaud, 2004; Bouchet et al., 2017). Therefore, identifying the genetic variations underlying landrace phenotypic differences has proved a complicated task.
Currently, smallholder cultivators growing maize select seeds year by year to meet quality and variety desires according to their diet and traditions (Ortega Paczka, 2003; Boege, 2008; Orozco-Ramírez et al., 2016). This has been done for over 9,000 years by Mexican indigenous groups and mestizo subsistence producers (Yamasaki et al., 2005; van Heerwaarden et al., 2011). Nowadays, Mexican maize landraces are still grown mostly by smallholders, typically in parcels of land <5 ha in marginal environments, but together accounting for 85% of Mexico's productive land (SIAP, 2008; Arteaga et al., 2015). Because of this joint extent of farmland, and because maize landraces often equal or surpass the yield production of breeding lines in marginal environments (Perales et al., 2003), maize landraces are crucial for Mexican food security (Bellon et al., 2011; CONABIO, 2011). Maize landraces have also historically been used as donor material for breeding efforts at a global scale, making them important reservoirs of genetic diversity for further improvement (Troyer, 2004; Smith et al., 2015; Dwivedi et al., 2016).
We believe that careful examination of genomic variation between landraces is a way to test for evidence of the influence of bio-cultural processes (human selection of desired characters based on cultural preferences) that may not have been detected by less comprehensive approaches of earlier studies. This could also grant the distinction of loci influenced by human selection from those influenced by natural selection (given by environmental conditions). In the present study, we used SNP genomic information from 50 samples of five Mexican maize landraces to create a classification model based on landrace identity. This allowed the identification of informative SNPs for sample landrace classification. SNPs influenced by environmental factors derived from altitude differences were also identified. In this way, the influence of environmental influence could be discriminated from landrace-informative SNPs, thus allowing to pinpoint probable artificially selected loci distinguishing landraces.
2. Materials and Methods
2.1. Sampling and SNP Genotyping
The 59 Mexican landraces defined by CONABIO (2011) (http://www.biodiversidad.gob.mx/usos/maices/razas2012.html) were examined to select five (Zapalote Chico, Conejo, Zapalote Grande, Tehua, and Comiteco) that show contrasting phenotypic characteristics (Figure 1 and Table 1). These landraces are represented by 37 accessions from the Germplasm Bank of the International Maize and Wheat Improvement Center (CIMMYT) that were genotyped in this study (see below), plus 13 accessions from a previous study that used the same extraction protocol and SNP data generation (Arteaga et al., 2015). Our sample size for each landrace was 8–14 accessions, collected between 1946 and 2010 and covering most of the potential distribution of each landrace (see Supplementary Table 1 and Figure 2). Potential distributions were taken from Perales and Golicher (2011).
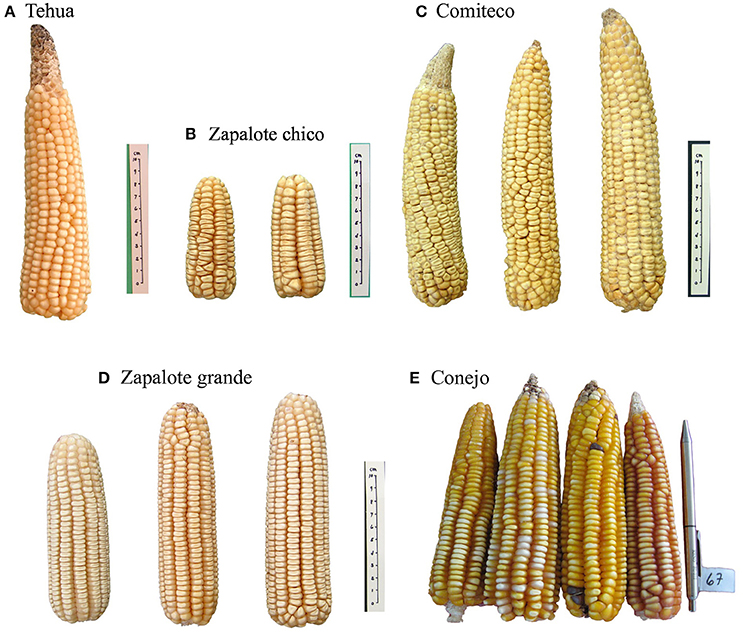
Figure 1. Representative images of each of the landraces used in this study, note the difference in cob size among them. Images credit: Instituto Nacional de Investigaciones Forestales Agrcolas y Pecuarias (INIFAP) and Global Maize Project (CONABIO, 2011).
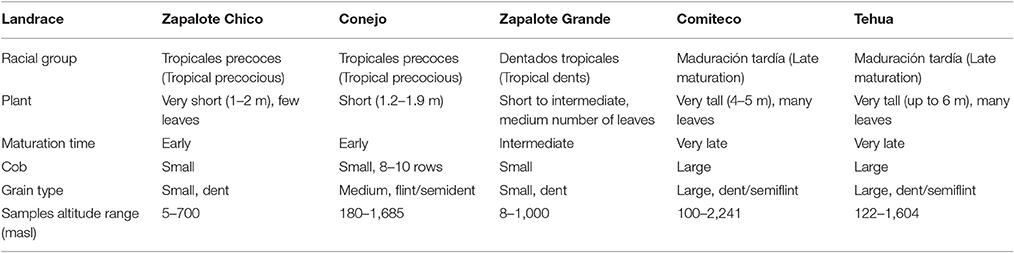
Table 1. Main phenotypic characteristics of the analyzed landraces according to CONABIO (2011).
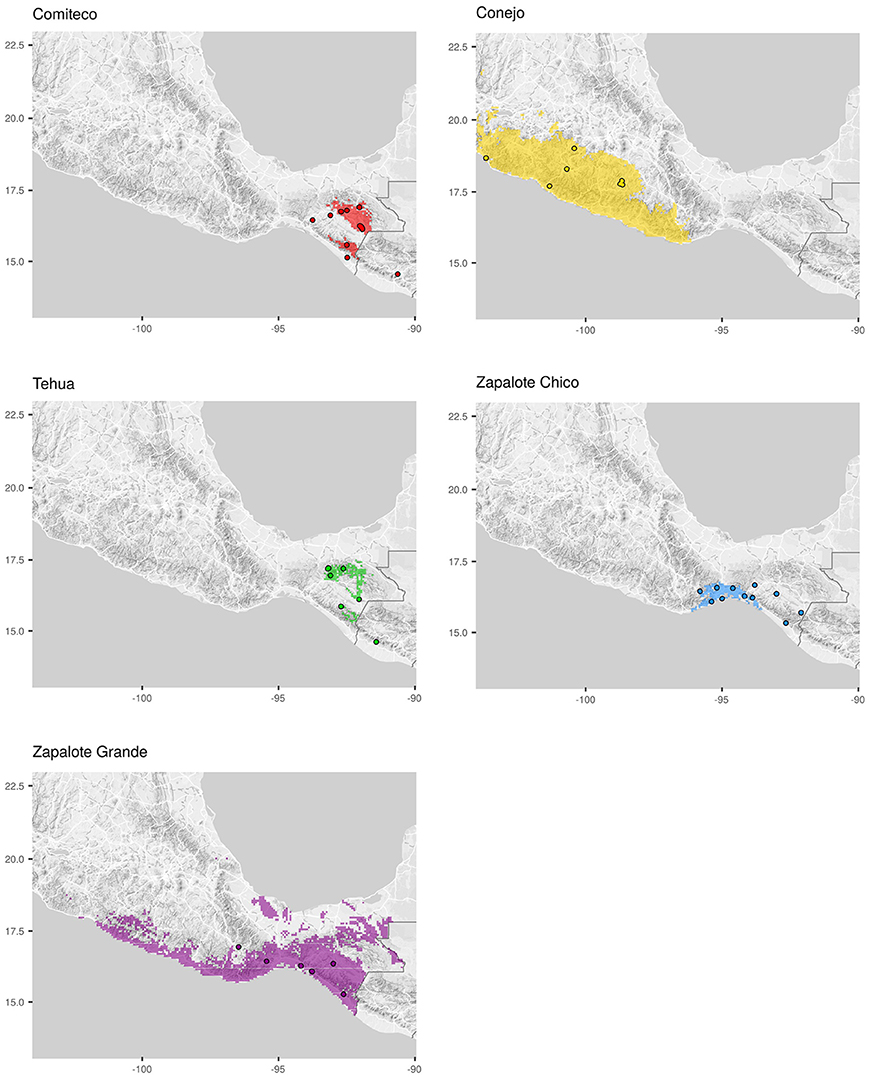
Figure 2. Sample collection sites. Each map shows the potential distribution of the maize landrace (shadowed area) and the location where the genotyped accessions of each landrace were collected (dots). Landrace potential distributions were taken from Perales and Golicher (2011).
From CIMMYT's accessions, one seed per accession was germinated in greenhouse conditions. DNA was extracted from leaf tips collected from 3-week-old seedlings with Thermo Fisher Scientific Genomic DNA Purification Kit (K0512) (Thermo-Fisher Scientific, Waltham, MA, USA). DNA quality was determined by agarose gel electrophoresis and with a Thermo-Fisher Scientific Nanodrop Spectrophotometer 2000 (Thermo-Fisher Scientific, Waltham, MA, USA). Accessions with a 260/280 ratio below 1.6 were re-extracted.
Single nucleotide polymorphisms were genotyped at INMEGEN (Instituto Nacional de Medicina Genómica) with the Illumina MaizeSNP50 BeadChip on an Infinium HD assay (Illumina, San Diego, Ca, USA). Automated allele calling was done in GenomeStudio 2010.1 (Genotyping module 1.7.4; Illumina); loci with GenTrain score <0.3 and with more than 30% missing data were excluded. Data was exported to PLINK format (Purcell et al., 2007). A maximum of 10% missing data per individual was allowed. Data from the 13 accessions obtained from Arteaga et al. (2015) was added to this dataset. Only SNPs present in both groups of accessions were retained.
2.2. Subsetting SNPs into Candidate Loci of Artificial Selection
In order to guide the search for landrace-distinguishing SNPs, three sets of SNPs were defined: (1) all the SNPs available (35,909), (2) SNPs of domestication and improvement (974 SNPs), and (3) SNPs with high FST (435 SNPs). Set one refers to all the SNPs retained after filtering and joining our dataset with Arteaga's. The SNPs of domestication and improvement were determined using the maize domestication and improvement genes reported by Hufford et al. (2012) and Meyer and Purugganan (2013). To do this we used the Panzea Genotype Search Tool (http://cbsuss05.tc.cornell.edu/hdf5/hdf5.asp) to search which of our 35,909 SNPs were located in loci reported by Hufford et al. (2012) and Meyer and Purugganan (2013). SNPs with high FST were defined after calculating the value of FST among landraces for every SNP using the SNPStats package (Clayton, 2015) in R (R Core Team, 2008) and taking the 1% of SNPs with the highest FST.
2.3. Identifying Loci to Distinguish Landraces
We used the three previously defined set of SNPs to examine if they could be useful for distinguishing the landraces in a principal components analysis (PCA) and discriminant analysis of principal components (DPCA). The PCA was performed for each set using the package SNPRelate (Zheng et al., 2012). The first two components were used for plotting, coloring accessions by landrace. A K-means clustering analysis was performed to the three sets of SNPs to identify which set better recovered the landrace pattern using the packages adegenet (Jombart, 2008) and ape (Paradis et al., 2004). The DAPC (Jombart et al., 2010) was carried out to classify samples according to their landrace. This analysis was performed with the set of SNPs with high FST using the R package adegenet. The contribution of each SNP to the DAPC classification model was calculated. The SNP distribution showed a long-tailed distribution, the top contributing SNPs (~2%) were identified as landrace-informative SNPs.
2.4. Identifying Loci Associated to Altitude
To discard the possible confounding effect of altitude in the SNPs identified as landrace-informative, we repeated the previous analyses but grouping the accessions by altitude instead of landrace. For this, first the accessions were grouped according to their altitude in two groups: high altitude (>750 masl) and low altitude (0-750 masl). The limit between the altitudinal groups was defined following the altitudinal distribution of the landraces included, separating the interquartile range of the landraces typically growing at low altitude (Zapalote Chico and Zapalote Grande) from the interquantile range of the landraces growing at high altitude (Tehua and Comiteco). Still, all landraces except Zapalote Chico had accessions from both altitude categories. All Zapalote Chico accessions belonged to the low altitude group (see Table 1).
FST index of each SNP between these two altitude groups was calculated using the SNPStats R package, and a subset of the 1% of SNPs with highest FST was extracted to continue the analysis. Using this group of SNPs, a DAPC was performed as earlier, but so as to have a model that classifies the samples according to their altitude group. The contribution of each SNP to the classification model was obtained, and the SNPs with the highest contribution were identified as altitude-informative SNPs. The groups of landrace informative SNPs and altitude associated SNPs were compared to identify which landrace-informative SNPs are independent from altitude.
2.5. Biological Annotation of Obtained SNPs
SNPs identified as landrace- or altitude-informative were reviewed in order to obtain their genomic position and biological annotation. This was accomplished by searching for the corresponding loci through the locus lookup tool at MaizeGDB (www.maizegdb.org/locus_lookup; Andorf et al., 2010). Further information about genes associated with the obtained SNPs was recovered through literature review.
2.6. Data and Code Accessibility
All SNP data and R scripts used for this study is available in the Dryad online repository: http://dx.doi.org/10.5061/dryad.j2n8q.
3. Results
3.1. Subsetting SNPs into Candidate Loci of Artificial Selection
After data filtering, the final SNPs data set consisted of 35,909 SNPs. The domestication and improvement SNP set consists of 974 SNPs located within the loci associated with domestication and improvement reported by Hufford et al. (2012) and Meyer and Purugganan (2013). A total of 84 SNPs were located in the loci reported by Meyer and Purugganan. In the loci reported by Hufford et al. 501 SNPs were located in loci associated with improvement and 389 SNPs in loci associated with domestication.
Values of FST among landraces for every SNP ranged from 0 to 0.597 (mean = 0.056, SD = 0.055). Most SNPs present FST values close to 0, indicating that they are poorly differentiated among landraces. The high FST SNP set consists of 435 SNPs with FST values higher than 0.2283, representing 1% of SNPs with highest FST (Figure 3). Most domestication and improvement SNPs have relatively small values of FST, and only 6 SNPs are common between the two sets.
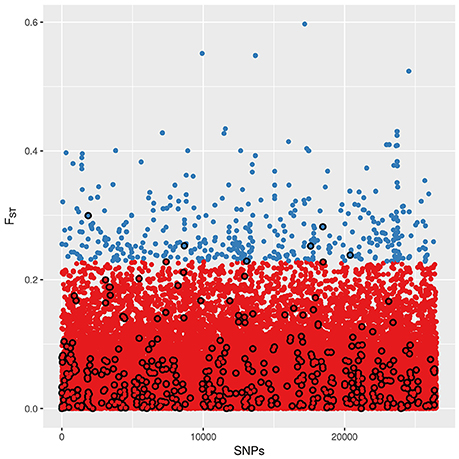
Figure 3. Distribution of SNPs according to their FST value among landraces. Blue: SNPs set of high FST SNPs. Black circle line: SNPs of the domestication and improvement. There are only six SNPs belonging to both SNP sets.
3.2. Identifying Loci to Distinguish Landraces
PCA using each of the three sets of SNPs reflects that the genomic information each set carries is structured differently. Figure 4 shows the different distribution patterns of the first two principal component among the three SNP sets. The percent of variance explained by the first three PCs of the high FST SNP set is 20.91, 6.30, and 5.67, respectively, which is higher than the variance explained by the first three PCs of the set of all SNPs (4.39, 3.12, 2.89) and the domestication and improvement SNPs (5.04, 3.80, 3.60).
The utility of the three sets of SNPs for grouping the accessions according to their landrace was assessed by a k-means clustering analysis, testing from one to five clusters and determining the appropriate number of clusters through the Bayesian information criterion (BIC) (Figure 5). For the all SNPS and domestication and improvement SNPs sets, the best clustering groups all the accessions into a single cluster. The best clustering for the high FST SNP set identifies three groups: one group containing only accessions of Zapalote Chico, a second group with accessions of landraces Zapalote Grande and Conejo, and a third group with accessions of landraces Tehua and Comiteco. Since the only SNP set capable of identifying different clusters in the accessions was the high FST SNP set, we continued the analysis only with these SNPs.
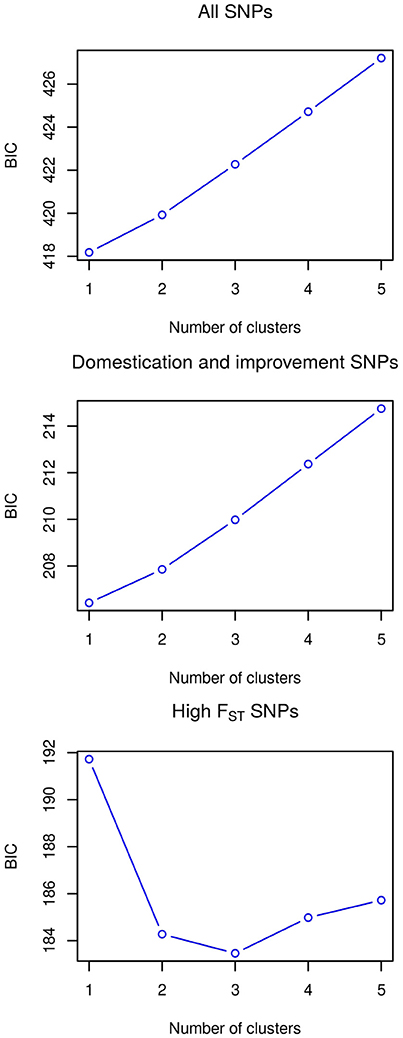
Figure 5. Clustering analysis with the three SNP sets, the lower value of BIC indicates optimal clustering.
The classification model from the DAPC analysis used the first four principal components of the high FST SNP set and was able to classify the accessions using two discriminant functions (Figure 6A). The first discriminant function (horizontal axis) recovers an altitude distribution pattern for the accessions, sorting them successively from the landrace growing in the lowest altitudes (Zapalote Chico) to the landrace growing in the highest places (Comiteco). The second discriminant function separates races growing in similar altitudes. The two discriminant functions are able to separate the clusters of accessions according to their landrace.
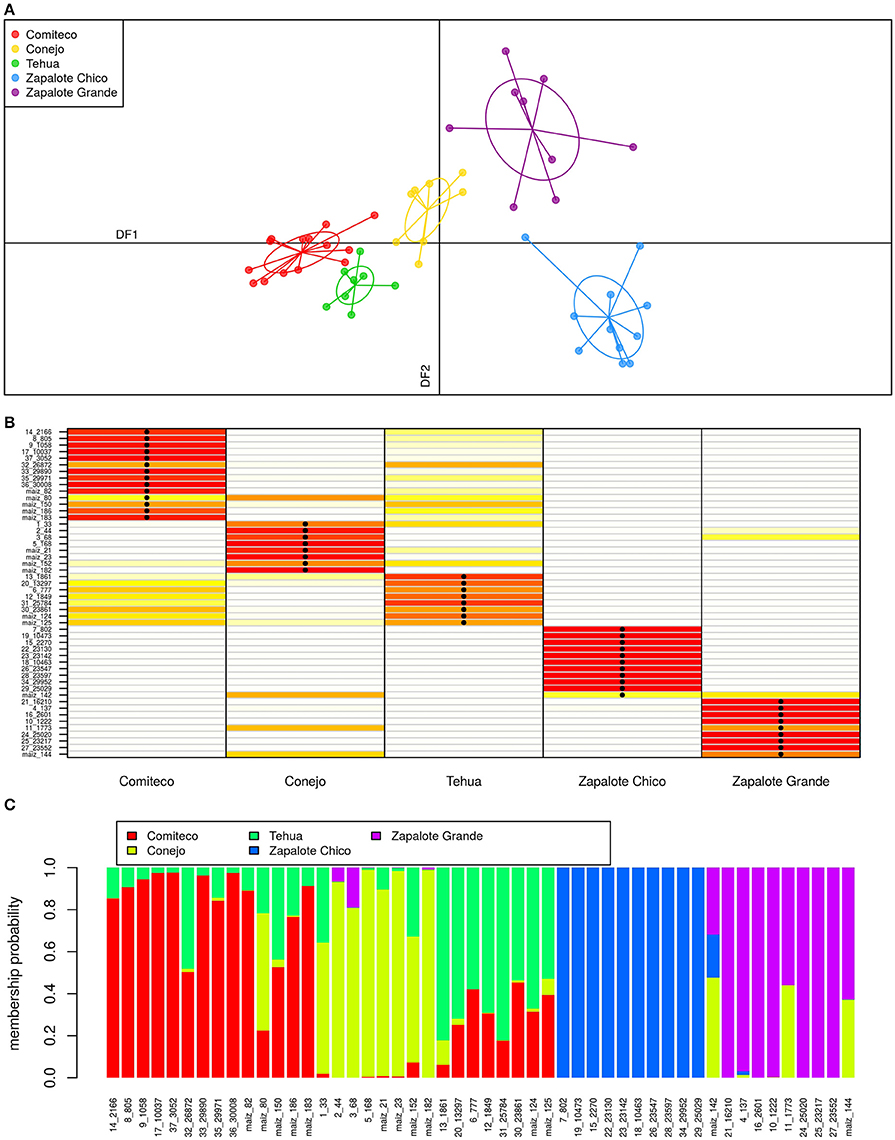
Figure 6. Landrace DAPC. (A) Classification plot: here the two discriminant functions of the DAPC are plotted for each sample. (B) Assignment plot: this plot shows the accuracy of the model to recover samples landrace. The color of each cell points out the probability of assigning a given sample (row) to the corresponding landrace (column) with red colors meaning high probability of assignment. The black dots in cells show the assigned landrace of the sample, so when a dot falls on a red cell means a correct landrace classification. (C) Structure plot: this admixture-like plot describes, for each sample, the membership probability to the different landraces according to the model.
This model has a high degree of accuracy in assigning the correct landrace to each sample, 94% of the samples are correctly classified (Figure 6B). This accuracy is achieved despite the high degree of admixture present among some samples (Figure 6C). Tehua and Comiteco are the two landraces with more admixture between them; and accordingly, the ones in which the landrace reassignment by the model is less discriminant. Samples from Conejo and Zapalote Grande show certain degree of admixture, but the model is able to reassign their accessions accurately. Zapalote Chico is the landrace with less admixture, forming a clearly separated cluster from the rest. This classification model gains more validity considering that the samples we analyzed are representative of each landrace's geographic distribution and their time of collection spans from 1946 to 2010.
The contribution of each SNP to each discriminant function was calculated. For both functions, the SNP contributions showed an exponential distribution, with most SNPs having very little contribution and a few SNPs with very high contribution (Supplementary Figure 1). The top contributing SNPs of each function were identified, giving a group of 11 informative SNPs for the first function and 9 informative SNPs for the second function (Supplementary Table 2). Together, these 20 SNPs are identified as the landrace-informative SNPs. Genomic position and biological annotation of these SNPs was obtained: 9/20 are associated to seven genes, four of which have not yet been characterized. The three characterized genes are a polygalacturonase enzyme, heat shock factor 30 (HSF30), and transcription factor IIIA (Supplementary Table 2).
3.3. Identifying Loci Associated with Altitude
A set of SNPs differentiated between the two altitude groups was identified using FST index values. FST values between altitude groups for all SNPs ranged from 0 to 0.5251 (mean = 0.0345, SD = 0.043). Data for the 1% of SNPs with the highest FST (above 0.1728) was extracted. The best k-means grouping using this group of SNPs resulted in two groups, which almost perfectly recovered the high and low altitude groups previously defined, clustering only one accession incorrectly. The DAPC was done using the first principal component and one discriminant function (Supplementary Figure 2). The classification model had an almost perfect performance, misclassifying only that same accession that was incorrectly clustered before (Figure 6C). The contribution of each SNP to the discriminant function followed an exponential distribution. The top 14 contributing SNPs were identified as altitude-informative SNPs (Supplementary Figure 3), and their genomic position and biological annotation was obtained (Supplementary Table 3). Of the 14 altitude associated SNPs, eight fall within loci of genes of which only three genes have been annotated. These genes correspond to the auxin responsive protein SAUR40, Transformer-2, and the heat shock factor 30 (HSF30).
Comparing the altitude-informative SNPs with the landrace-informative SNPs, there are five SNPs shared between the two sets (Supplementary Table 2), two of which are associated to an uncharacterized gene (GRMZM2G100103). Additionally, both analyses identified a SNP, albeit a different one each, part of a gene associated with the heat shock factor protein 30. So this locus was also considered to be shared between the analyses. In total, for the landrace analysis there are 14 uniquely informative SNPs and six SNPs shared with the altitude analysis. For the altitude analysis, there are eight unique SNPs that are not shared with the landrace analysis.
4. Discussion
We found that samples of Mexican maize can be classified by landrace using only a small number of SNPs. Furthermore, we could identify genomic loci differentiating the five landraces we studied, and we could distinguish them from loci correlated with altitude.
4.1. Clustering by Landrace is Achieved Only with High FST SNPs
Both the PCA and the k-means clustering analysis show that accession clustering depends on the set of SNPs used. In particular, the set of SNPs with high FST groups the samples according to their landrace while neither of the other two sets (“domestication and improvement SNPs” and “all SNPs”) does.
The k-means clustering analysis grouped all the samples in a single cluster when using all the SNPs, meaning that the whole-genomic distribution of variation is not structured by landrace. In other words, from a whole-genome perspective, these five landraces are not distinguishable among them. This is consistent with previous studies showing that maize genomic structure is not explained by landrace, and is better correlated with environmental factors (van Heerwaarden et al., 2011; Breña Ochoa, 2013; Arteaga et al., 2015). Clustering analysis with the domestication and improvement SNPs also failed in finding structure among the samples, grouping them all in one cluster. Domestication SNPs are defined as loci with differentiated between teosinte and maize, thus involved in maize domestication from its wild relative. In this sense, it is expected that domestication SNPs are not differentiated among maize landraces. On the other hand, the improvement SNPs we used were found as loci differentiated between maize landraces and modern improved lines (Hufford et al., 2012). Modern improved lines derive from distinct landrace founders and have been subject to subsequent selection for agronomic traits, which are not the same that constitute the differences among these landraces. Thus, genomic variation in modern lines not necessarily maintains the structure of variation found on landraces, as shown by the low values of FST found in most domestication and improvement SNPs (Figure 3) indicates that these loci are not differentiated among landraces and so do not explain morphological differentiation.
Using the set of high FST SNPs (435 SNPs, Figure 2) recovers the landrace assemblages as recognized by morphology. This suggests that this set of SNPs are under different selective pressures depending on the landrace, therefore varying in a different way from the rest of the genome. These selective pressures are probably a combination of environmental differences among the areas the landraces are grown, and those guided by the smallholder farmers that continuously select seed looking for certain characteristics of the crop: desired morphology, taste, compatibility with their agricultural practices, among others (Pressoir and Berthaud, 2004; Boege, 2008; Bellon et al., 2011).
We were able to classify samples by landrace using the high FST SNPs. Sample classification was achieved with high accuracy (94%) despite broad landrace admixture. Most samples show some degree of mixing among landraces (Figure 6C), this pattern is explained by the known high degree of gene flow among maize population. There is a high degree of gene flow in maize populations. It is caused both by open pollination of maize fields flowering at the same time, and by seed transportation and exchange by farmers (Dyer and Taylor, 2008; Orozco-Ramírez et al., 2016). Gene flow among landraces is also strengthened by the fact that 85.5% of the total average surface cultivated with maize in Mexico (covering an average of 8.4 million ha between 1996 and 2006) is cultivated by smallholders using landraces (SIAP, 2008, 2016) therefore creating a large and genetically diverse landscape for open pollination to occur. Seed manipulation performed by producers maintains a phenotypic differentiation of maize landraces, in spite of high levels of gene flow, both by pollen and seed exchange (Pressoir and Berthaud, 2004; Dyer and Taylor, 2008; Mercer et al., 2008; Orozco-Ramírez et al., 2016).
4.2. Distinguishing the Influence of Artificial from Natural Selection on the Genome
Accurate models to classify accessions by landraces and altitude categories were attained through DAPC, allowing for the further inference of informative markers for both models. Informative SNPs were identified by their high contribution to the classification models, being the most informative SNPs when trying to classify by race or by altitude. SNPs association to landrace and altitude is based on the idea that if the model found these marker to be the most useful when separating the landrace/altitude groups, it is because there are underlying differences among groups in these markers, probably due to selection. We identified 14 landrace-informative SNPs that are independent from altitude. Even though our altitude analysis does not account for all the environmental factors, previous studies establish altitude as the principal factor shaping maize genomic variation (van Heerwaarden et al., 2011; Breña Ochoa, 2013; Arteaga et al., 2015; Romero Navarro et al., 2017), thus making it a valid approximation of environmentally driven natural selection.
A potential caveat of our analyses is that the genomic data used was obtained from the Illumina MaizeSNP50 BeadChip. This SNP chip was developed from the genome of maize B73 line to identify genomic sites of agronomic interest (Ganal et al., 2011). Therefore, it presents a bias toward identifying variation present in the B73 genome, and it is highly probable that there are other genomic regions differentiated among landraces that are not recognized by the SNP chip. In other words, our analysis may be missing variation at other alleles, which make up a substantial proportion of natural variation (Huang and Han, 2014).
The proposed landrace and altitude informative loci identified here represent an opportunity to further confirm if SNPs identified by genomic analyses can explain landraces phenotypic characteristics. This can be assessed by testing their direct influence in the plant phenotype, and analyzing their variation among other maize landraces. However, through searching for the functional annotation of associated genes, our approximation showed interesting results hinting functional importance of the identified loci.
One of the five annotated genes associated with the informative markers, the heat shock factor 30 HSF30, was shared between the landrace and the altitude analyses. HSF30 is part of the heat shock transcription factors that drive the cellular heat stress response in plants (Westerheide et al., 2012). HSF30 has not been functionally characterized in maize, but it is homologous to heat shock factor A2 present in other plant species where its function has been studied. For example, in Arabidopsis thaliana, HSF2A is the heat shock factor with highest expression under heat stress conditions, and acts as regulator of several stress-responsive genes (Schramm et al., 2006); and in Solanum lycopersicum, it has been shown that HSF2A transcription is strictly dependent on heat stress, and acts as a strong transcriptional activator for heat shock proteins (Nover et al., 2001). Considering the homology between HSF30 and HSF2A and the relationship between altitude and temperature, our results suggest that landraces hold a component of local adaptation to the altitudinal range where they are grown.
The other two annotated genes identified among the landrace-informative SNPs correspond to a polygalacturonase enzyme and transcription factor IIIA. An homologous gene of transcription factor IIIA has been characterized in rice, where its function was associated with plant development and tolerance to abiotic stress related to salinity, low temperatures, and drought (Huang et al., 2012). We consider transcription factor IIIA of special interest, because of the non-linear phenotypic effects a transcription factor can exert on a developing plant in the context of a transcription regulatory network (Davila-Velderrain and Alvarez-Buylla, 2014). Further maize research focusing on the effects of variation in this gene grown under different environmental conditions would be very interesting.
4.3. What Makes Zapalote Chico So Different and Homogeneous?
An interesting result in the classification model is that the samples of Zapalote Chico were the most distant cluster and presented a high homogeneity within the landrace (Figure 6). This has already been highlighted by a study focusing in the role of MuDR transposons, which are a characteristic of the Mutator maize line and are associated with a high rate of somatic mutations (Gutiérrez-Nava et al., 1998). Specifically, Gutierrez-Nava and collaborators reported that Zapalote Chico has an elevated rate of MuDR transposons, and that, when inter-crossed with germplasm from another landrace, the genomic elements present in Zapalote Chico cause somatic mutations, an effect known as hybrid dysgenesis. These mutations do not happen when two samples of Zapalote Chico are inter-crossed, showing that somehow the mutagenic activity of the transposons is repressed. This phenomenon explains Zapalote Chico's genetic isolation relative to other landraces. Interestingly, Zapotecs, the indigenous people growing this landrace, interpret this phenomenon in the form of a myth, which holds that when Zapalote Chico is crossed with another kind of maize it “kills" it (Gutiérrez-Nava et al., 1998).
4.4. Genetic Differences Are Not Enough to Explain the Landraces Existence
In this study, we focused on genomic differences among maize landraces; we did not consider other factors that cause phenotypic differences and that could have a strong influence on crop phenotypes. Notably, the manipulation of the agroecological system by the people that grow maize is an important source of variation that could explain part of the differences seen among landraces (Jardón Barbolla and Benítez, 2012). Nonetheless, landraces, characterized in terms of both local adaptation and satisfaction of cultural preferences, have an associated genomic variation underlying their differences (Bellon et al., 2009). Several tens of millions of rural populations in Mexico depend solely on their maize production. Mexican cultural richness is reflected in the wide differences of uses people give to maize, resulting in a great variety in terms of flavor, shape, color, texture, and other organoleptic characteristics (Boege, 2008). Although natural and artificial selection exert their influence in particular loci, the concerted action of whole genomic variation and external conditions during a plant's development determine its phenotype, and consequently, overall success for its further sowing by the people using it.
Mexican maize is grown in complex agroecological systems where biological (interspecies relations, genetic flow, pollinators activities, soil microbiome, etc.), social, and cultural (farming techniques, seed interchange, land tenure patterns, religious, and symbolic uses, etc.) factors interact. This demands the study of maize and the agroecosystems in which it grows, including the groups of small-scale maize producers, as a whole for the understanding of maize diversity.
Mexican maize landraces contain a high genomic diversity within and among them, which results from the underlying multiplicity of environmental and biocultural factors mediating their existence (Arteaga et al., 2015). These factors entail selective pressures, both natural/environmental and artificial/biocultural, acting on different genomic regions. In this study we found that, even though from a whole-genome perspective maize landraces are indistinguishable, it is possible to identify genomic regions differentiated among landraces. Furthermore, combining the landrace analysis with an environmental association analysis on the genomic data, it is possible to recognize loci influenced by natural or artificial selection. Our results are evidence of the genomic variation that exists in maize landraces and their importance for maize diversity conservation. Nevertheless, it is important to keep in mind that landraces are evolving entities, sustained through a process based on a great genomic, environmental and bio-cultural diversity. Furthermore, focusing on preserving landraces by monitoring their phenotypic diversity through a small number of representative samples may lead to losing the genetic diversity underlying local adaptation. Understanding the genomic foundation of landrace diversity and the processes influencing it is imperative for its maintenance. Given the different factors shaping maize biodiversity, a program aimed at conserving the genomic and phenotypic richness of maize requires the maintenance of the biocultural processes in which it is grown and used.
Author Contributions
AW, JC, and DP designed the study. AW coordinated the study. JC conducted lab work. JC and AM designed and conducted the analyses. All authors analyzed the results and wrote the manuscript.
Funding
Funding was provided by the Dirección General del Sector Primario y Recursos Naturales Renovables (DGSPRNR) of the Secretaría de Medio Ambiente y Recursos Naturales (SEMARNAT) through the grants 2009-1 and 2013 to the Comisión Nacional para el Conocimiento y Uso de la Biodiversidad (CONABIO).
Conflict of Interest Statement
The authors declare that the research was conducted in the absence of any commercial or financial relationships that could be construed as a potential conflict of interest.
The reviewer SR and handling Editor declared their shared affiliation, and the handling Editor states that the process nevertheless met the standards of a fair and objective review.
Acknowledgments
We thank the International Maize and Wheat Improvement Center (CIMMYT) for seed samples. Thanks to Jorge Nieto for insights on Zapalote Chico's uniqueness. Thanks to Karl Phillips and Ángel Kato for comments on an early version of this manuscript. Thanks to Francisca Acevedo, Oswaldo Oliveros, and CARB-CONABIO members for logistic support throughout the realization of this work. Thanks to the members of the Laboratorio de Genética de la Conservación for their comments and support. JC is a doctoral student from Programa de Doctorado en Ciencias Biomédicas, Universidad Nacional Autónoma de México (UNAM) and received fellowship 446988 from CONACYT. Special thanks to all the people that produce and consume totopos itsmeños, nicuatole, gorditas, elotes, tamales, tortillas, pozol, and the rest of delicious food made out of maize and mesoamerican genius.
Supplementary Material
The Supplementary Material for this article can be found online at: http://journal.frontiersin.org/article/10.3389/fgene.2017.00045/full#supplementary-material
References
Andorf, C. M., Lawrence, C. J., Harper, L. C., Schaeffer, M. L., Campbell, D. A., and Sen, T. Z. (2010). The locus lookup tool at maizegdb: identification of genomic regions in maize by integrating sequence information with physical and genetic maps. Bioinformatics 26, 434–436. doi: 10.1093/bioinformatics/btp556
Arteaga, M. C., Moreno-Letelier, A., Mastretta-Yanes, A., Vázquez-Lobo, A., Breña-Ochoa, A., Moreno-Estrada, A., et al. (2015). Genomic variation in recently collected maize landraces from Mexico. Genomics Data 7, 38–45. doi: 10.1016/j.gdata.2015.11.002
Bellon, M., Barrientos-Priego, A., Colunga-García, P., Perales, H., Reyes Aguero, J., Rosales Serna, R., et al. (2009). “Diversidad y conservación de recursos genéticos en plantas cultivadas,” in Capital Natural de México, Vol. II:Estado de Conservación y Tendencias de Cambio, (México: CONABIO), 355–382.
Bellon, M. R., Hodson, D., and Hellin, J. (2011). Assessing the vulnerability of traditional maize seed systems in mexico to climate change. Proc. Natl. Acad. Sci. U.A.S. 108, 13432–13437. doi: 10.1073/pnas.1103373108
Boege, E. (2008). El Patrimonio Biocultural de los Pueblos Indígenas de México: Hacia una Conservación In situ de la Biodiversidad y Agrodiversidad en los Territorios Indígenas. México: Instituto Nacional de Antropología e Historia y Comisión Nacional para el Desarrollo de los Pueblos Indígenas.
Bouchet, S., Bertin, P., Presterl, T., Jamin, P., Coubriche, D., Gouesnard, B., et al. (2017). Association mapping for phenology and plant architecture in maize shows higher power for developmental traits compared with growth influenced traits. Heredity 118, 249–259. doi: 10.1038/hdy.2016.88
Breña Ochoa, A. (2013). Estructura Genética Espacial Asociada a la Selección en Razas de maíz de México. México: Tesis Universidad Nacional Autónoma de México.
Camacho-Villa, T., Maxted, N., Scholten, M., and Ford-Lloyd, B. (2015). Defining and identifying crop landraces. Plant Genet. Resour. 3, 373–384. doi: 10.1079/PGR200591
CONABIO (2011). Proyecto Global de maíces Nativos: Recopilación, Generación, Actualización y análisis de Información Acerca de la Diversidad Genética de Maíces y sus Parientes Silvestres en méxico. Mexico: CONABIO. Available online at: http://www.biodiversidad.gob.mx/genes/pdf/proyecto/InformedeGestion_V1.pdf
Davila-Velderrain, J., and Alvarez-Buylla, E. R. (2014). Bridging the genotype and the phenotype: towards an epigenetic landscape approach to evolutionary systems biology. BioRxiv. doi: 10.1101/004218
Dwivedi, S. L., Ceccarelli, S., Blair, M. W., Upadhyaya, H. D., Are, A. K., and Ortiz, R. (2016). Landrace germplasm for improving yield and abiotic stress adaptation. Trends Plant Sci. 21, 31–42. doi: 10.1016/j.tplants.2015.10.012
Dyer, G. A., and Taylor, J. E. (2008). A crop population perspective on maize seed systems in Mexico. Proc. Natl. Acad. Sci. U.S.A. 105, 470–475. doi: 10.1073/pnas.0706321105
Ganal, M. W., Durstewitz, G., Polley, A., Bérard, A., Buckler, E. S., Charcosset, A., et al. (2011). A large maize (Zea mays L.) SNP genotyping array: development and germplasm genotyping, and genetic mapping to compare with the B73 reference genome. PLoS ONE 6:e28334. doi: 10.1371/journal.pone.0028334
Gutiérrez-Nava, M., Warren, C., León, P., and Walbot, V. (1998). Transcriptionally active MuDR, the regulatory element of the mutator transposable element family of Zea mays, is present in some accessions of the Mexican land race Zapalote chico. Genetics 149, 329–346.
Huang, J., Sun, S., Xu, D., Lan, H., Sun, H., Wang, Z., et al. (2012). A TFIIIA-type zinc finger protein confers multiple abiotic stress tolerances in transgenic rice (Oriza sativa L.). Plant Mol. Biol. 80, 337–350. doi: 10.1007/s11103-012-9955-5
Huang, X., and Han, B. (2014). Natural variations and genome-wide association studies in crop plants. Annu. Rev. Plant Biol. 65, 4.1–4.21. doi: 10.1146/annurev-arplant-050213-035715
Hufford, M. B., Lubinksy, P., Pyhäjärvi, T., Devengenzo, M. T., Ellstrand, N. C., and Ross-Ibarra, J. (2013). The genomic signature of crop-wild introgression in maize. PLoS Genet. 9:e1003477. doi: 10.1371/journal.pgen.1003477
Hufford, M., Xu, X., van Heerwaarden, J., Pyhäjärvi, T., Chia, J., Cartwright, R., et al. (2012). Comparative population genomics of maize domestication and improvement. Nat. Genet. 44, 808–811. doi: 10.1038/ng.2309
Jardón Barbolla, L., and Benítez, M. (2012). “La comunidad agroecológica como unidad ecológica, de domesticación y de conservación,” in Naturaleza, Ciencia y Sociedad: 40 años en la Facultad de Ciencias, ed F. Guerrero McManus (México: Facultad de Ciencias-CEIICH, UNAM), 1–16.
Jombart, T. (2008). adegenet: a R package for the multivariate analysis of genetic markers. Bioinformatics 24, 1403–1405. doi: 10.1093/bioinformatics/btn129
Jombart, T., Devillard, S., and Balloux, F. (2010). Discriminant analysis of principal components: a new method for the analysis of genetically structured populations. BMC Genet. 11:94. doi: 10.1186/1471-2156-11-94
Lorant, A., Pedersen, S., Holst, I., Hufford, M. B., Winter, K., Piperno, D., et al. (2017). The potential role of genetic assimilation during maize domestication. BioRxiv. doi: 10.1101/105940
Mercer, K., Martínez-Vásquez, A., and Perales, H. (2008). Asymmetrical local adaptation of maize landraces along an altitudinal gradient. Evol. Appl. 1, 489–500. doi: 10.1111/j.1752-4571.2008.00038.x
Meyer, R. S., and Purugganan, M. D. (2013). Evolution of crop species: genetics of domestication and diversification. Nat. Rev. Genet. 14, 840–852. doi: 10.1038/nrg3605
Nover, L., Bharti, K., Döring, P., Mishra, S. K., Ganguli, A., and Scharf, K. D. (2001). Arabidopsis and the heat stress transcription factor world: how many heat stress transcription factors do we need? Cell Stress Chaperones 6, 177–189. doi: 10.1379/1466-1268(2001)006<0177:AATHST>2.0.CO;2
Orozco-Ramírez, Q., Ross-Ibarra, J., Santacruz-Varela, A., and Brush, S. (2016). Maize diversity associated with social origin and environmental variation in Southern Mexico. Heredity 116, 477–484. doi: 10.1038/hdy.2016.10
Ortega Paczka, R. (2003). “La diversidad del maíz en México,” in Sin Maíz No Hay País, eds G. Esteva and C. Marielle (México: CONACULTA), 123–154.
Paradis, E., Claude, J., and Strimmer, K. (2004). Ape: analyses of phylogenetics and evolution in r language. Bioinformatics 20, 289–290. doi: 10.1093/bioinformatics/btg412
Perales, H. R., Brush, S. B., and Qualset, C. O. (2003). Landraces of maize in central Mexico: an altitudinal transect. Econ. Bot. 57, 7–20. doi: 10.1663/0013-0001(2003)057[0007:LOMICM]2.0.CO;2
Perales, H., and Golicher, D. (2011). Modelos de Distribución para las Razas de Maíz en México y Propuesta de Centros de Diversidad y de Provincias Bioculturales. Available online at: http://www.biodiversidad.gob.mx/genes/pdf/proyecto/Informe%20completo_apendices.pdf.
Perales, H., and Golicher, D. (2014). Mapping the diversity of maize races in Mexico. PLoS ONE 9:e114657. doi: 10.1371/journal.pone.0114657
Pressoir, G., and Berthaud, J. (2004). Population structure and strong divergent selection shape phenotypic diversification in maize landraces. Heredity 92, 95–101. doi: 10.1038/sj.hdy.6800388
Purcell, S., Neale, B., Todd-Brown, K., Thomas, L., Ferreira, M., Bender, D., et al. (2007). PLINK: a tool set for whole-genome association and population-based linkage analyses. Am. J. Hum. Genet. 81, 559–575. doi: 10.1086/519795
R Core Team (2008). R: A Language and Environment for Statistical Computing. Vienna: R Foundation for Statistical Computing.
Romero Navarro, J. A., Wilcox, M., Burgueño, J., Romay, C., Swarts, K., Trachsel, S., et al. (2017). A study of allelic diversity underlying flowering-time adaptation in maize landraces. Nat. Genet. 49, 476–480. doi: 10.1038/ng.3784
Ruiz-Corral, J. A., Durán-Puga, N., Sánchez-González, J. J., Ron-Parra, J., González Eguiarte, D., Holland, J., et al. (2008). Climatic adaptation and ecological descriptors of 42 Mexican maize races. Crop Sci. 48, 1502–1512. doi: 10.2135/cropsci2007.09.0518
Ruiz Corral, J. A., Hernández Casillas, J., Sanchez, J., Corona Ortega, A., Ojeda Ramírez, G., Guerrero Herrera, M., et al. (2013). Ecología, Adaptación y Distribución Actual y Potencial de las Razas Mexicanas de Maíz. Mexico: INIFAP.
Schramm, F., Ganguli, A., Kiehlmann, E., Englich, G., Walch, D., and von Koskull-Dring, P. (2006). The heat stress transcription factor hsfa2 62 serves as a regulatory amplifier of a subset of genes in the heat stress response in arabidopsis. Plant Mol. Biol. 60, 759–772. doi: 10.1007/s11103-005-5750-x
SIAP (2008). Situación Actual y Perspectivas del Maíz en México 1996-2012. Mexico: Secretaría de Agricultura, Ganadería, Desarrollo Rural, Pesca y Alimentación, Servicio de Información Agroalimentaria y Pesquera.
SIAP (2016). Anuario Estadístico de la Producción Agrícola. Available online at: http://infosiap.siap.gob.mx/aagricola_siap_gb/icultivo/
Smith, S., Bubeck, D., Nelson, B., Stanek, J., and Gerke, J. (2015). “Genetic diversity and modern plant breeding,” in Genetic Diversity and Erosion in Plants, eds M. Ahuja and S. Jain (Cham: Springer International Publishing), 55–88.
Troyer, A. (2004). Background of US hybrid corn II. Crop Sci. 44, 370–380. doi: 10.2135/cropsci2004.3700
van Heerwaarden, J., Doebley, J., Briggs, W., Glaubitz, J., Goodman, M., Sanchez Gonzalez, J., et al. (2011). Genetic signals of origin, spread, and introgression in a large sample of maize landraces. Proc. Natl. Acad. Sci. U.S.A. 108, 1088–1092. doi: 10.1073/pnas.1013011108
Westerheide, S., Raynes, R., Powell, C., Xue, B., and Uversky, V. (2012). HSF transcription factor family, heat shock response, and protein intrinsic disorder. Curr. Protein Pept. Sci. 13, 86–103. doi: 10.2174/138920312799277956
Yamasaki, M., Tenaillon, M., Bi, V., Schroeder, S., Sanchez-Villeda, H., Doebley, J. F., et al. (2005). A large-scale screen for artificial selection in maize identifies candidate agronomic loci for domestication and crop improvement. Plant Cell 17, 2859–2872. doi: 10.1105/tpc.105.037242
Keywords: maize diversity, artificial selection, Maize SNP50K BeadChip, maize genomics
Citation: Caldu-Primo JL, Mastretta-Yanes A, Wegier A and Piñero D (2017) Finding a Needle in a Haystack: Distinguishing Mexican Maize Landraces Using a Small Number of SNPs. Front. Genet. 8:45. doi: 10.3389/fgene.2017.00045
Received: 11 January 2017; Accepted: 29 March 2017;
Published: 18 April 2017.
Edited by:
Paula Casati, CONICET, ArgentinaReviewed by:
Sebastian Pablo Rius, CONICET, ArgentinaMehboob-ur- Rahman, National Institute for Biotechnology and Genetic Engineering (NIBGE), Pakistan
Copyright © 2017 Caldu-Primo, Mastretta-Yanes, Wegier and Piñero. This is an open-access article distributed under the terms of the Creative Commons Attribution License (CC BY). The use, distribution or reproduction in other forums is permitted, provided the original author(s) or licensor are credited and that the original publication in this journal is cited, in accordance with accepted academic practice. No use, distribution or reproduction is permitted which does not comply with these terms.
*Correspondence: Ana Wegier, awegier@ib.unam.mx