- 1UMR CNRS 6015-INSERM U1083, MitoVasc Institute, Angers University, Angers, France
- 2Biochemistry and Genetics Department, Angers Hospital, Angers, France
The development of next generation sequencing (NGS) has greatly enhanced the diagnosis of mitochondrial disorders, with a systematic analysis of the whole mitochondrial DNA (mtDNA) sequence and better detection sensitivity. However, the exponential growth of sequencing data renders complex the interpretation of the identified variants, thereby posing new challenges for the molecular diagnosis of mitochondrial diseases. Indeed, mtDNA sequencing by NGS requires specific bioinformatics tools and the adaptation of those developed for nuclear DNA, for the detection and quantification of mtDNA variants from sequence alignment to the calling steps, in order to manage the specific features of the mitochondrial genome including heteroplasmy, i.e., coexistence of mutant and wildtype mtDNA copies. The prioritization of mtDNA variants remains difficult, relying on a limited number of specific resources: population and clinical databases, and in silico tools providing a prediction of the variant pathogenicity. An evaluation of the most prominent bioinformatics tools showed that their ability to predict the pathogenicity was highly variable indicating that special efforts should be directed at developing new bioinformatics tools dedicated to the mitochondrial genome. In addition, massive parallel sequencing raised several issues related to the interpretation of very low mtDNA mutational loads, discovery of variants of unknown significance, and mutations unrelated to patient phenotype or the co-occurrence of mtDNA variants. This review provides an overview of the current strategies and bioinformatics tools for accurate annotation, prioritization and reporting of mtDNA variations from NGS data, in order to carry out accurate genetic counseling in individuals with primary mitochondrial diseases.
Key Points for in silico Prioritization and Interpretation of mtDNA Variants
• Query dedicated mtDNA databases which are regularly updated (e.g., Mitomap, HmtDB).
• Consider the variant's frequency both within the general population and specific haplogroup.
• Detection and interpretation of low heteroplasmy levels should be carefully evaluated.
• Integrate additional information that might modulate the clinical penetrance (e.g., mitochondrial haplogroup, synergistic or helper mtDNA variants, nuclear variants).
• Evaluate inter-species and primates amino-acid or nucleotide conservation.
• Favor in silico prediction tools dedicated to mtDNA (e.g., APOGEE, Mitotip, Mtool Box).
Introduction
The prevalence of mitochondrial diseases primarily affecting oxidative phosphorylation (OXPHOS) is estimated at about 1 in 4,300 (Gorman et al., 2015). As mitochondrial proteins are encoded both by the nuclear genome and their own genome (mtDNA), their clinical presentation are highly heterogeneous. Human mtDNA is a 16,569 bp circular double-stranded molecule, encoding for 13 polypeptides involved in the oxidative phosphorylation (OXPHOS), together with 2 ribosomal RNAs and 22 tRNAs supporting the translational machinery (Wallace et al., 2010). Pathogenic variants of the mitochondrial genome can affect either the protein coding genes (Wallace et al., 2013), tRNAs (Tang et al., 2013; Gorman et al., 2015) and rRNA genes (Smith et al., 2014; Elson et al., 2015). Genetic defects in the mitochondrial genome can be identified in a variable proportion of patients with mitochondrial respiratory disorders, reaching up to 20% of patients (Thorburn et al., 2004). Hundreds of pathogenic mtDNA variants implicated in a variety of human diseases (Lott et al., 2013) have now been reported in the continuously updated Human Mitochondrial Genome Database—the Mitomap (Ruiz-Pesini et al., 2007; Lott et al., 2013) but as of today (July 2018) only 84 variants have a confirmed status, whereas a total of 595 other variants classified as reported, awaiting a final confirmation of pathogenicity (Figure 1). These mtDNA variants lead to a broad spectrum of maternally-inherited diseases, ranging from lethal neonatal syndromes to multisystemic disorders, with high variable clinical phenotypes and penetrance, mainly resulting from shifts and differences in the mutant load (Wallace et al., 2010). Indeed, due to stochastic segregation of mtDNA, the percentage of mutant and normal mtDNAs may drift during cellular divisions, and the percentage of the mutation load may vary drastically among the different tissues and organs, from 100% mutant load, defining homoplasmy, to the coexistence of mutant and wildtype copies, defining heteroplasmy. As the percentage of heteroplasmy increases, the energy production declines until the energy output falls below the minimum necessary for the physiological maintenance of cellular functions, causing the appearance of symptoms (Rossignol et al., 2003).
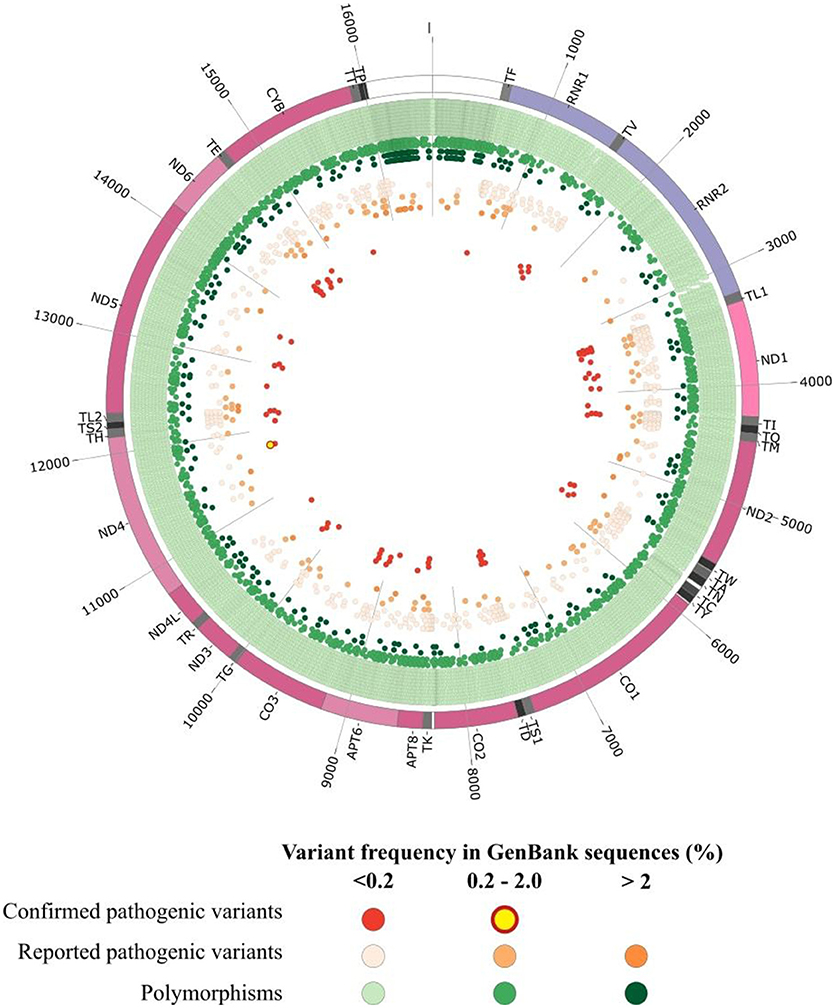
Figure 1. Graphical representation of human mitochondrial DNA variations. The outer circle depicts the mitochondrial genome with annotated tRNAs (gray), rRNAs (purple), protein-coding genes (Bentley et al., 2008), and non-coding regions (white). In the inner circles each point represent an mtDNA variant reported in GenBank sequences collected from Mitomap according to the variant status reported in Mitomap (polymorphisms in green, reported pathogenic variants in orange, confirmed pathogenic variants in red) and variant frequency in GenBank (< 0.2%, light color; 0.2–2.0%, medium color; >2.0%, dark color).
Until the development of next generation sequencing (NGS), molecular diagnosis of mitochondrial disorders was based on a combination of several techniques, including targeted Sanger sequencing for the detection of mutations, long-range polymerase chain reaction (PCR) and Southern blotting, for the detection of mtDNA rearrangements and depletions, whereas fluorescent PCR restriction fragment length polymorphism (RFLP) and pyrosequencing were used for the quantification of mtDNA variants and rearrangements (Moraes et al., 2003; Bannwarth et al., 2005; Wong and Boles, 2005). These techniques are still useful as confirmatory and independent tools to ascertain the presence of a given mtDNA variant identified by NGS. The development of massive parallel sequencing techniques now allows the systematic screening of the whole mitochondrial genome thus increasing the efficacy of the workflow with increased sample throughput and greater sensitivity in the detection of mtDNA variants (Vancampenhout et al., 2014; Ye et al., 2014; Seneca et al., 2015). However, massive parallel sequencing of the whole mitochondrial genome, with the increasing quantity and complexity of mtDNA data, led to difficulties to appreciate the variants identified, thereby posing new challenges in the molecular diagnosis of mitochondrial diseases. Indeed, several issues related to the interpretation of very low mutational loads (Guo et al., 2013), the discovery of variants of unknown significance (van der Walt et al., 2012), and mutations unrelated to the patient phenotype, generate difficulties in prioritizing the variants, and as a consequence different interpretations of mtDNA variants in the diagnostic process. This review provides an overview of the current strategies, databases and bioinformatics tools for an accurate annotation, prioritization and report of mtDNA variations coming from NGS, in order to carry out fast and accurate genetic counseling in patients with primary mitochondrial disease.
mtDNA Variant Annotation
Careful mtDNA annotation of mtDNA variants is a prerequisite for accurate prioritization, addressed by several pipelines and online tools (Table 1A), like MSeqDR mvTool (Shen et al., 2018), Mitomaster (Lott et al., 2013), mtDNA-Server (Weissensteiner et al., 2016a), MitoTool (Fan and Yao, 2011), or SG-ADVISER (Rueda and Torkamani, 2017). Then, the general workflow for mtDNA variants prioritization could parallel that of the nuclear genome and rely on an accurate standardized annotation, based on consensus databases and in silico prediction tools.
Population and Clinical Databases
Whereas mtDNA data are available from exome and genome sequencing data (Griffin et al., 2014; Patowary et al., 2017), the frequency of mtDNA variants in the general population is not reported in the databases such as GnomAD (Lek et al., 2016) or MARRVEL (Wang et al., 2017). The number of dedicated databases focusing on mtDNA with an active curation is limited (Table 1B), only three being available online: Mitomap (Kogelnik et al., 1996; Lott et al., 2013), HmtDB (Clima et al., 2017) and HmtVar (Preste et al., 2018). Together these resources gathered for instance more than 45,000 whole mtDNA sequences and over 70,000 mtDNA control region sequences for the Mitomap database. However, the interpretation of the variant frequency in the general population is difficult given that databases include patient data and because of the peculiarities of mtDNA genetics (incomplete penetrance, heteroplasmy level, influence of mitochondrial haplogroup background). For example, the m.3460G>A primary LHON mutation is reported 20 times in GenBank (Bentley et al., 2008) as of July 10, 2018 (Mitomap database), the pathogenic and unquestionable variant being reported both in patients, and also from phylogenetic studies. There is currently no consensus threshold to consider, often mtDNA variant is frequent in the population, thresholds between 0.2 and 0.5% being arbitrarily chosen in several studies (Wang et al., 2012; Lieber, 2013). However, they do not consider the variant frequency within a peculiar haplogroup which can lead to misinterpretation as some haplogroups are underrepresented in databases. For instance, Asian and African lineages represent only 21 and 13% of the Mitomap GB dataset. To overcome this problem, the Mitomap database warns if a variant is identified at >1% in at least one of the macro-lineages or over 10% in the major haplogroups for tRNA variants (Sonney et al., 2017). For the first step of prioritization, forensic databases such as EMPOP (Parson and Dur, 2007) are useful.
Clinical databases combining mtDNA variants and clinical files are available (Table 1C). General databases such as CLINVAR (Landrum et al., 2016), CLINVAR Miner (Henrie et al., 2018), or OMIM (Amberger et al., 2015) carrying information on mtDNA in addition to nDNA variants, can be distinguished from databases specifically dedicated to mtDNA such as Mitomap, HmtVar or HmtDB which are more exhaustive. In the latter, more than 10,000 variants are gathered throughout the whole mtDNA (Figure 1). For example in MT-ND1 gene only 42 variations are reported in CLINVAR, whereas 693 variants are available in Mitomap (Lott et al., 2013), and among them, 62 are reported associated with a clinical phenotype. MtDNA-specific information, such as heteroplasmy and haplogroup frequency are not systematically reported in each database, hence questioning the value of pipelines collecting information from multiple resources in order to provide a complete description of the variant. Other specialized databases are also helpful for variant prioritization: Mamit-tRNA database which compiles information on mammalian mitochondrial tRNA genes (Putz et al., 2007) or the Mitobreak database focusing on mtDNA rearrangements (Damas et al., 2014).
Even if the prevalence of mitochondrial diseases is in the order of 1/4,300 (Gorman et al., 2015), it was shown that at least 1 in 200 newborn cord bloods carry one of the ten most common pathogenic mtDNA mutations, which is much more frequent than expected (Elliott et al., 2008). Many of these mutations will probably be lost through stochastic segregation, but there is still a chance that these mutations will be transmitted to the offspring and causing mtDNA diseases in next generations. As mtDNA variations can be obtained from WES (Griffin et al., 2014) or WGS data, the identification of pathogenic variants unrelated to the patient's phenotype poses a challenge for the interpretation and report of the variant. Selected mtDNA confirmed variants, such as LHON (e.g., m.11778G>A, m.14484T>C, m.3460T>C or other rare LHON mutations as classified in the top 19 mutations, see mitomap) or deafness pathogenic variants such as the m.1555G>A, should be considered as secondary and actionable findings. As defined by the American College of Medical Genetics and Genomics which claimed that “the results of a deliberate search for pathogenic alterations in genes that are not apparently relevant to a diagnostic indication for which the sequencing test was ordered but which may nonetheless be of medical value or utility to the ordering physician and the patient” (Green et al., 2013; Kalia et al., 2017). As an example, we discovered in our clinical center the m.1555G>A variant in the MT-RNR1, responsible for the amino-glycoside-induced and non-syndromic hearing loss (Bitner-Glindzicz et al., 2009; Vandebona et al., 2009) in a family with isolated optic atrophy but without hearing impairment. The discovery of a pathogenic variant should be considered as “actionable,” i.e., pathogenic variants whose penetrance would result in medical recommendations and health care prevention. Indeed, the presence of the m.1555G>A may modify patient management, audiometric follow-up and hearing protection, prompting appropriate genetic counseling and recommendations with the avoidance of the use of specific drugs such as aminoglycosides (Estivill et al., 1998). Other mtDNA variants with unrelated or unclear relation to the clinical phenotype have been identified in the general population, such as primary LHON mutations found in patients, but unaccompanied by any ophthalmological sign (Inagaki et al., 2006; Yang et al., 2016). Incomplete penetrance is a hallmark of optic neuropathies, starting with LHON, and several parameters such as modifier genes or environmental factors such as tobacco smoking or the consumption of alcohol or administration of ethambutol, have been identified as risk factors for asymptomatic carriers of LHON mutations (Yu-Wai-Man et al., 2016; Caporali et al., 2017). Thus, some of the mtDNA pathogenic variants, such as LHON or deafness pathogenic variants should be carefully treated as “actionable mutations” and call for genetic counseling, especially to avoid exposure to risk factors and for a clinical follow up.
In silico Prediction Tools and Pathogenicity Scores
The systematic mtDNA screening by NGS revealed a large number of novel variants of unknown significance (Lieber, 2013; McCormick et al., 2013), as exemplified by the identification of 11 patients among 71 from a pediatric cohort, harboring a novel variant of unknown significance (VUS) (van der Walt et al., 2012). In our diagnostic laboratory setting, about 6.5% of the patients carried a VUS. The interpretation of the clinical significance of mtDNA VUS is more complicated than for the nuclear VUS, in part due to the mtDNA characteristics, as heteroplasmy and high mutation rate (Marcelino and Thilly, 1999), which are not considered in the classical prioritization algorithms, and because of the limited guidelines for the mtDNA compared to those provided by the American College of Medical Genetics for nuclear VUS (Richards et al., 2015). Special efforts are currently underway with an initiative from an international group of mitochondrial researchers and clinicians to revise the ACMG guidelines specifically for mtDNA variants (Procaccio, personal communication).
In silico prediction tools, which evaluate the functional impact of variations using approaches based on interspecies sequence conservation and/or structure analysis, are currently the last step of variant prioritization. As prediction tools are specific for a type of variations, we will distinguish those dedicated to coding regions to those dedicated to other mtDNA regions.
A plethora of in silico bioinformatics prediction tools exists for the prioritization of nuclear DNA coding variants. A thorough analysis of the main tools commonly used to evaluate the pathogenicity of variants demonstrated that the performances vary drastically when variants of the mtDNA-encoded proteins were tested. A set of 38 confirmed pathogenic variants (M) and 224 variants considered to be polymorphisms (P) according to Mitomap, were assessed with a set of 19 different prediction tools gathered in MitImpact2 (Castellana et al., 2015; Figure 2). Variants of the mitochondrial genome were subdivided into four categories based on the prediction of pathogenicity, i.e., benign, medium, damaging, and no prediction. The performances of the prediction of pathogenic mtDNA variants differed significantly between the different bioinformatics tools. For instance, more than 70% of the confirmed pathogenic mutations were predicted to be benign with SIFT, whereas about 15% of the pathogenic variants were not predicted as such by Polyphen2, highlighting that the tools developed for nDNA are barely suitable for mtDNA. Conversely, recent tools developed for mtDNA using machine learning based approaches (Table 1D) show better performances (Figure 2), as MToolBox (Calabrese et al., 2014), the meta-predictor APOGEE (Castellana et al., 2017), or Mitoclass.1 (Martin-Navarro et al., 2017), confirming the need to pursue the development of tools dedicated to mitochondrial genetics.
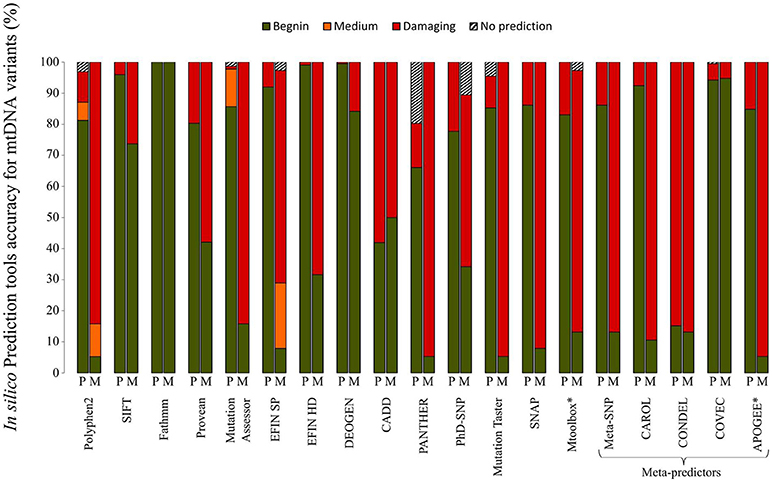
Figure 2. Performances of in silico prediction tools for non-synonymous mtDNA variants. A set of 38 confirmed pathogenic variants (M) and 224 non-synonymous variants classified as mtDNA polymorphisms (P) according to Mitomap, were assessed with 19 different prediction tools. Information about the different in silico tools is available at MitImpact2 website (http://mitimpact.css-mendel.it/). Variants were classified into 4 categories: benign (green), medium (orange), damaging (red) and no prediction (hatched) according to the tool prediction. Results are expressed as percentages. *In silico tools developed for mtDNA.
Few tools are dedicated to mitochondrial tRNAs, accounting for nearly 50% of the mtDNA alterations identified in patients (Schaefer et al., 2008; Gorman et al., 2015). PON-mt-RNA is a multifactorial score associating 12 features including evolutionary conservation, primary to tertiary structures, and functional assays including biochemistry and histochemistry (Niroula and Vihinen, 2016). All the precomputed data are downloadable at http://structure.bmc.lu.se/PON-mt-tRNA/datasets.html/. MITOTIP, the most recent tool available through Mitomap combines conservation data, structural analogies with other tRNA variants and secondary structure information (Sonney et al., 2017), giving the best prediction performances in terms of sensitivity and specificity. However, specific tools for the interpretation of VUS in MT-RNR1 and MT-RNR2 combining conservational information with functional and structural data are helpful to better interpretation of ribosomal variants (Smith et al., 2014; Elson et al., 2015).
Thus, many bioinformatics tools are useful for predicting the functional impact of mtDNA variants, nevertheless results should still be considered with caution, due to the high rate of false negative and false positive predictions as demonstrated in Figure 2. To overcome this problem and improve the prioritization of mtDNA VUS, several teams have developed scoring approaches (McFarland et al., 2004; Mitchell et al., 2006; Wong, 2007). These scores which combined algorithms similar to those of in silico prediction tools (i.e., structure, conservation) and functional in vivo and in vitro evaluation show better performances, but their use is still limited, because functional studies are time-consuming and tissues such as fibroblasts, cybrids, or muscle samples, are not always available for assessing the consequences of the variants on mitochondrial physiology. Given the increasing number of variants of unknown significance identified by NGS, it would be interesting to regularly re-evaluate these pathogenicity scores based on new information.
Heteroplasmy Level Interpretation
The development of NGS techniques and dedicated bioinformatics pipelines (Calabrese et al., 2014; Weissensteiner et al., 2016a; Marquis et al., 2017) has widely improved the detection of low-level mtDNA variations. While the major drawback of Sanger sequencing is its lack of sensitivity for detecting DNA mutant loads lower than 20% (Procaccio et al., 2006; Wong, 2010), the limit of detection (LOD) of NGS strategies is considerably lower for the major NGS technologies with an LOD close to 5% for pyrosequencing methods (Zaragoza et al., 2010; Sosa et al., 2012) and semiconductor technology (Huang, 2011; McElhoe et al., 2014; Vancampenhout et al., 2014; Seneca et al., 2015), and close to 1% for reversible terminated chemistry (Huang, 2011; Zhang et al., 2012). Recently, the development of duplex sequencing further improved the power to detect low-level of heteroplasmy down to 0.01% (Schmitt et al., 2012; Ahn et al., 2015).
One of the first pitfalls to this gain of sensitivity is the difficulty to confirm very low heteroplasmy mtDNA variations, eliminating possible sequencing artifacts, which could impair the diagnosis accuracy and prevent sound genetic counseling. Several sensitive technics have been developed such as fluorescent PCR-RFLP (Procaccio et al., 2006; Bannwarth et al., 2013), PNA clamp PCR (Urata et al., 2004), digital or real-time PCR (He et al., 2002; Grady et al., 2014). Thus nowadays, although no consensus threshold has yet been defined, previous studies establishing the detection limit from 1 to 10% according to the technology used (Cui et al., 2013; Wong, 2013; Seneca et al., 2015). The detection of low mutation loads improves the diagnosis of mitochondrial diseases and the quality of genetic counseling, particularly for mutation carriers. However, the clinical relevance is sometimes difficult to interpret in probands, with the risk of false conclusion of the implication of mtDNA in the disease, instead of a nuclear gene variant. For example, a report described a patient presenting the Alper's syndrome, carrying both nuclear and mtDNA mutations: two pathogenic variants in POLG and also the m.3243A>G at 8% mutation load in blood (Tang et al., 2013). The mtDNA mutation was probably not responsible for the phenotype, considered as secondary finding, but may potentially modulate the clinical phenotype as described (Tang et al., 2013). Indeed, it is commonly accepted that mtDNA mutations have clinical consequences only over a certain heteroplasmy level, also called threshold effect (Rossignol et al., 2003). It was recently shown that clinical phenotypes are associated with low heteroplasmic mtDNA pathogenic variants (Ng et al., 2018) or deletions (Leung et al., 2018), and additional low-level heteroplasmic variants can explain the phenotypic variability of mtDNA homoplasmic mutations (Ballana et al., 2008). Unfortunately, mtDNA databases do not specify the level of heteroplasmy leading to clinical phenotype in patient, with the exception of Mitomap (Kogelnik et al., 1996; Lott et al., 2013), which provides partial information mentioning the homoplasmic or heteroplasmic nature of pathogenic variants. So, there are different situations to consider depending on the mtDNA variation and the tissue analyzed in the proband. When the mutation has been identified from a blood sample, or from the analysis of more relevant tissues, such as muscle or uroepithelial cells (McDonnell et al., 2004; Blackwood et al., 2010; Liu et al., 2013; Fayssoil et al., 2017; Grady et al., 2018), the presence of the heteroplasmic mutation can be linked to the phenotype of the patient. Indeed, due to the stochastic segregation of mtDNA, mutation loads can drastically vary in-between and within tissues, and several mutations may undergo selection in blood cells, as for example the m.3243A>G, for which heteroplasmy decreases by 1.4% per year in blood (Rahman et al., 2001). Recently, a new algorithm was developed to estimate the m.3243A>G mutation heteroplasmy in muscle based on the quantification in blood or uroepithelial cells (Grady et al., 2018). This tool available through an online webserver (https://newcastle-mito-apps.shinyapps.io/m3243ag_heteroplasmy_tool/) is then helpful for the clinicians for the interpretation of low-level of the m.3243A>G mutation identified in peripheral tissues. Coupling the mtDNA copy number with mutant load quantification is another argument to assess the clinical variability (Frey et al., 2017; Emperador et al., 2018). Indeed, an increase of the mtDNA copy number in a heteroplasmic situation will modify the absolute value of the wild type mtDNA copies, even if the mutant load remains unchanged and therefore may explain the variability of the clinical phenotypes of mtDNA-related disorders, as shown for LHON (Giordano et al., 2014) or MELAS syndrome (Liu et al., 2013; Grady et al., 2018). The gain of sensitivity enabled by massive parallel sequencing also allows identifying high level of heteroplasmy, in samples that were initially considered as homoplasmic (Genasetti et al., 2007; Ballana et al., 2008; Carrasco Salas et al., 2016). The detection of heteroplasmy has always been considered as strong argument for the variant scoring pathogenicity (McFarland et al., 2004; Mitchell et al., 2006).
Perspectives: Toward an Integrative Analysis of the Mitochondrial Genome
With the development of NGS, we have now access to the entire mtDNA sequencing information. Therefore, additional information such as mitochondrial haplogroups, identification of helper or synergistic mutations and co-occurrences of variants should be incorporated in clinical diagnostic settings, as they are thought to modulate the phenotypic expression of mtDNA pathogenic variants.
Influence of Mitochondrial Haplogroups
Mitochondrial haplogroups, i.e., clusters of nucleotide polymorphisms accumulated in mtDNA during human evolution and transmitted through maternal lineage, play a role in modulating the penetrance of mitochondrial diseases (Ghelli et al., 2009; Gomez-Duran et al., 2012), or in age-related disorders (van der Walt et al., 2004; Wolf et al., 2010; Hudson et al., 2013). Haplogroups are defined by ancient sequence polymorphisms that occur at the base of a particular branch of the mtDNA phylogenetic tree (Ingman et al., 2000). For example, the higher prevalence of specific subclades of haplogroup J have been shown to modify the pathogenicity and penetrance of LHON (Brown et al., 2002; Ghelli et al., 2009; Caporali et al., 2017). Computing mitochondrial haplogroups from NGS data is relatively easy, as many bioinformatics tools (Tables 1C,D) have been developed based on the PhyloTree data (van Oven and Kayser, 2009) such as HaploGrep2 (Weissensteiner et al., 2016b), Mitomaster (Lott et al., 2013), or HmtDB (Clima et al., 2017) available on a web-server, or integrated into an all-in-one pipeline as in MToolBox bioinformatics suite (Calabrese et al., 2014), MseqDR mvTool (Shen et al., 2018), mit-o-matic (Vellarikkal et al., 2015). Conversely, the Phy-Mer software allows the classification of haplogroups from the FASTQ files, i.e., without prior alignment, avoiding mistakes caused by artifactual sequencing variants (Navarro-Gomez et al., 2015). However, few databases provide information about the prevalence of a variant in a specific haplogroup. Mitomaster allows a quick overview of the variant distribution in the different haplogroups, while HmtDB through multiple queries gives the frequency of a pathogenic variant within the haplogroup.
Co-occurrence of mtDNA Variants
Even with well-characterized mitochondrial phenotypes such as LHON or MELAS, shown to be associated with confirmed mtDNA variants, such as the m.11778G>A or m.3243A>G, respectively, mitochondrial whole genome screening may provide additional information with the co-occurrences of mtDNA variants that may modulate the phenotype (El Meziane et al., 1998; Khan et al., 2013). For example, the presence of the heteroplasmic m.12300G>A variant in MT-TL2, with a mutant load of about 10%, was shown to suppress the mitochondrial dysfunction in transmitochondrial cybrid cells carrying the m.3243A>G mutation with 99% mutated mtDNA, emphasizing the need for a complete mtDNA screening (El Meziane et al., 1998). A large study analyzing the distribution of known disease-causing mutations in a set of more than 30,000 mtDNA sequences has recently suggested that the mtDNA background influences the development of mtDNA mutagenesis with the acquisition of recurrent mtDNA variants (Wei et al., 2017). In addition, it was recently shown that, apart from any pathogenic mtDNA variants, the combination of rare non-synonymous polymorphisms could lead to LHON, as exemplified by both combinations of variants m.14258G>A in the MT-ND6 gene (p.Pro139Leu) and m.14582A> G (p.Val31Ala); m.14258G> A, m.10680G> A in the MT-ND4L gene and m.12033A> G in the MT-ND4 gene (Caporali et al., 2018). Functional studies of cybrid cells carrying both variant combinations revealed that the biochemical deficiency was transferred to mutant cybrids. Unfortunately, currently databases and bioinformatic pipelines do not allow identifying rare co-occurrences of variants, and further developments of these databases are needed to implement a searchable function of possible combinations of mtDNA variants.
Influence of the Nuclear Genome
As mitochondria are driven by two genomes, several studies have demonstrated that nuclear variants may modulate the phenotypic expression of mtDNA pathogenic variants (Davidson et al., 2009; Jiang et al., 2016). For example, it has recently been suggested that the c.572G> T variant (p.Gly191Val) in YARS2, a gene coding for mitochondrial tyrosyl-tRNA synthetase was associated with a mitochondrial protein translation defect, worsening mitochondrial respiratory chain deficiency in patients carrying the m.11778G>A LHON mutation (Jiang et al., 2016). Thus, YARS2 appeared as a nuclear modifier, capable of triggering optic atrophy in individuals carrying the m.11778G>A mutation, and would explain the incomplete penetrance of LHON, in addition to other parameters or environmental factors (Dimitriadis et al., 2014; Giordano et al., 2015). Unfortunately, major databases such as Mitomap, HmtDB, and HmtVar do not currently allow the search of the co-occurrence of mtDNA variants or in combination with nuclear variants. As the mtDNA data can be extracted from exome or genome sequencing data (Griffin et al., 2014), these information could be integrated in general databases such as GnomAD.
The integration of additional information in mitochondrial databases or in the filtering and prioritization process of bioinformatics pipelines, such as haplogroups, co-occurrences of mtDNA or nuclear variants shown to modulate the phenotype should be very helpful to assess the pathogenicity of a given variant for a better interpretation and as a possible explanation for incomplete penetrance or phenotypic variability. Special efforts should be directed at developing bioinformatics tools dedicated to the mitochondrial genome such as MseqDR.
Conclusion
Due to NGS technologies the amount of mtDNA is now constantly increasing and special efforts from the mitochondrial and scientific community have to be made to collect and organize the large quantity of generated information. In addition, the complexity of mtDNA interpretation is increasing in an exponential manner, requiring better and specific prediction tools to assess mtDNA variant pathogenicity or to assess the co-occurrence of variants.
Author Contributions
CB and VP decided on the content and structure of the first version of the manuscript. CB, DG, VD-D, EC, PA-B, and MC collected the information. VP and CB drafted the first and final version of the manuscript while DG, GL, DB, and PR revised the manuscript.
Funding
This work was founded by grants from: Institut National de la Santé et de la Recherche Médicale (INSERM), Centre National de la Recherche Scientifique (CNRS), University Hospital of Angers. This work was also supported by the following patients' foundations: Association Contre les Maladies Mitochondriales and Association Française Myopathies-Telethon, Fondation VISIO, Ouvrir les Yeux, Union Nationale des Aveugles et Déficients Visuels.
Conflict of Interest Statement
The authors declare that the research was conducted in the absence of any commercial or financial relationships that could be construed as a potential conflict of interest.
References
Ahn, E. H., Hirohata, K., Kohrn, B. F., Fox, E. J., Chang, C. C., and Loeb, L. A. (2015). Detection of ultra-rare mitochondrial mutations in breast stem cells by duplex sequencing. PLoS ONE 10:e0136216. doi: 10.1371/journal.pone.0136216
Amberger, J. S., Bocchini, C. A., Schiettecatte, F., Scott, A. F., and Hamosh, A. (2015). OMIM.org: Online Mendelian Inheritance in Man [OMIM(R)], an online catalog of human genes and genetic disorders. Nucleic Acids Res. 43, D789–D798. doi: 10.1093/nar/gku1205
Ballana, E., Govea, N., de Cid, R., Garcia, C., Arribas, C., Rosell, J., et al. (2008). Detection of unrecognized low-level mtDNA heteroplasmy may explain the variable phenotypic expressivity of apparently homoplasmic mtDNA mutations. Hum. Mutat. 29, 248–257. doi: 10.1002/humu.20639
Bannwarth, S., Procaccio, V., Lebre, A. S., Jardel, C., Chaussenot, A., Hoarau, C., et al. (2013). Prevalence of rare mitochondrial DNA mutations in mitochondrial disorders. J. Med. Genet. 50, 704–714. doi: 10.1136/jmedgenet-2013-101604
Bannwarth, S., Procaccio, V., and Paquis-Flucklinger, V. (2005). Surveyor nuclease: a new strategy for a rapid identification of heteroplasmic mitochondrial DNA mutations in patients with respiratory chain defects. Hum. Mutat. 25, 575–582. doi: 10.1002/humu.20177
Bentley, D. R., Balasubramanian, S., Swerdlow, H. P., Smith, G. P., Milton, J., Brown, C. G., et al. (2008). Accurate whole human genome sequencing using reversible terminator chemistry. Nature 456, 53–59. doi: 10.1038/nature07517
Bitner-Glindzicz, M., Pembrey, M., Duncan, A., Heron, J., Ring, S. M., Hall, A., et al. (2009). Prevalence of mitochondrial 1555A–>G mutation in European children. N. Engl. J. Med. 360, 640–642. doi: 10.1056/NEJMc0806396
Blackwood, J. K., Whittaker, R. G., Blakely, E. L., Alston, C. L., Turnbull, D. M., and Taylor, R. W. (2010). The investigation and diagnosis of pathogenic mitochondrial DNA mutations in human urothelial cells. Biochem. Biophys. Res. Commun. 393, 740–745. doi: 10.1016/j.bbrc.2010.02.072
Brown, M. D., Starikovskaya, E., Derbeneva, O., Hosseini, S., Allen, J. C., Mikhailovskaya, I. E., et al. (2002). The role of mtDNA background in disease expression: a new primary LHON mutation associated with Western Eurasian haplogroup. J. Hum. Genet. 110, 130–138. doi: 10.1007/s00439-001-0660-8
Calabrese, C., Simone, D., Diroma, M. A., Santorsola, M., Gutta, C., Gasparre, G., et al. (2014). MToolBox: a highly automated pipeline for heteroplasmy annotation and prioritization analysis of human mitochondrial variants in high-throughput sequencing. Bioinformatics 30, 3115–3117. doi: 10.1093/bioinformatics/btu483
Caporali, L., Iommarini, L., La Morgia, C., Olivieri, A., Achilli, A., Maresca, A., et al. (2018). Peculiar combinations of individually non-pathogenic missense mitochondrial DNA variants cause low penetrance Leber's hereditary optic neuropathy. PLoS Genet. 14:e1007210. doi: 10.1371/journal.pgen.1007210
Caporali, L., Maresca, A., Capristo, M., Del Dotto, V., Tagliavini, F., Valentino, M. L., et al. (2017). Incomplete penetrance in mitochondrial optic neuropathies. Mitochondrion 36, 130–137. doi: 10.1016/j.mito.2017.07.004
Carrasco Salas, P., Palma Milla, C., Lopez Montiel, J., Benito, C., Franco Freire, S., and Lopez Siles, J. (2016). Leber hereditary optic neuropathy: usefulness of next generation sequencing to study mitochondrial mutations on apparent homoplasmy. Med. Clin. 146, 163–166. doi: 10.1016/j.medcli.2015.10.015
Castellana, S., Fusilli, C., Mazzoccoli, G., Biagini, T., Capocefalo, D., Carella, M., et al. (2017). High-confidence assessment of functional impact of human mitochondrial non-synonymous genome variations by APOGEE. PLoS Comput. Biol. 13:e1005628. doi: 10.1371/journal.pcbi.1005628
Castellana, S., Ronai, J., and Mazza, T. (2015). MitImpact: an exhaustive collection of pre-computed pathogenicity predictions of human mitochondrial non-synonymous variants. Hum. Mutat. 36, E2413–E2422. doi: 10.1002/humu.22720
Clima, R., Preste, R., Calabrese, C., Diroma, M. A., Santorsola, M., Scioscia, G., et al. (2017). HmtDB 2016: data update, a better performing query system and human mitochondrial DNA haplogroup predictor. Nucleic Acids Res. 45, D698–D706. doi: 10.1093/nar/gkw1066
Cui, H., Li, F., Chen, D., Wang, G., Truong, C. K., Enns, G. M., et al. (2013). Comprehensive next-generation sequence analyses of the entire mitochondrial genome reveal new insights into the molecular diagnosis of mitochondrial DNA disorders. Genet. Med. 15, 388–394. doi: 10.1038/gim.2012.144
Damas, J., Carneiro, J., Amorim, A., and Pereira, F. (2014). MitoBreak: the mitochondrial DNA breakpoints database. Nucleic Acids Res. 42, D1261–D1268. doi: 10.1093/nar/gkt982
Davidson, M. M., Walker, W. F., Hernandez-Rosa, E., and Nesti, C. (2009). Evidence for nuclear modifier gene in mitochondrial cardiomyopathy. J. Mol. Cell. Cardiol. 46, 936–942. doi: 10.1016/j.yjmcc.2009.02.011
Dimitriadis, K., Leonhardt, M., Yu-Wai-Man, P., Kirkman, M. A., Korsten, A., De Coo, I. F., et al. (2014). Leber's hereditary optic neuropathy with late disease onset: clinical and molecular characteristics of 20 patients. J. Rare Dis. 9:158. doi: 10.1186/s13023-014-0158-9
El Meziane, A., Lehtinen, S. K., Hance, N., Nijtmans, L. G., Dunbar, D., Holt, I. J., et al. (1998). A tRNA suppressor mutation in human mitochondria. Nat. Genet. 18, 350–353. doi: 10.1038/ng0498-350
Elliott, H. R., Samuels, D. C., Eden, J. A., Relton, C. L., and Chinnery, P. F. (2008). Pathogenic mitochondrial DNA mutations are common in the general population. Am. J. Hum. Genet. 83, 254–260. doi: 10.1016/j.ajhg.2008.07.004
Elson, J. L., Smith, P. M., Greaves, L. C., Lightowlers, R. N., Chrzanowska-Lightowlers, Z. M., Taylor, R. W., et al. (2015). The presence of highly disruptive 16S rRNA mutations in clinical samples indicates a wider role for mutations of the mitochondrial ribosome in human disease. Mitochondrion 25, 17–27. doi: 10.1016/j.mito.2015.08.004
Emperador, S., Vidal, M., Hernández-Ainsa, C., Ruiz-Ruiz, C., Woods, D., Morales-Becerra, A., et al. (2018). The decrease in mitochondrial DNA mutation load parallels visual recovery in a leber hereditary optic neuropathy patient. Front. Neurosci. 12:61. doi: 10.3389/fnins.2018.00061
Estivill, X., Govea, N., Barcelo, E., Badenas, C., Romero, E., Moral, L., et al. (1998). Familial progressive sensorineural deafness is mainly due to the mtDNA A1555G mutation and is enhanced by treatment of aminoglycosides. Am. J. Hum. Genet. 62, 27–35. doi: 10.1086/301676
Fan, L., and Yao, Y. G. (2011). MitoTool: a web server for the analysis and retrieval of human mitochondrial DNA sequence variations. Mitochondrion 11, 351–356. doi: 10.1016/j.mito.2010.09.013
Fayssoil, A., Laforet, P., Bougouin, W., Jardel, C., Lombes, A., Becane, H. M., et al. (2017). Prediction of long-term prognosis by heteroplasmy levels of the m.3243A>G mutation in patients with the mitochondrial encephalomyopathy, lactic acidosis and stroke-like episodes syndrome. Eur. J. Neurol. 24, 255–261. doi: 10.1111/ene.13176
Frey, S., Geffroy, G., Desquiret-Dumas, V., Gueguen, N., Bris, C., Belal, S., et al. (2017). The addition of ketone bodies alleviates mitochondrial dysfunction by restoring complex I assembly in a MELAS cellular model. Biochim. Biophys. Acta 1863, 284–291. doi: 10.1016/j.bbadis.2016.10.028
Genasetti, A., Valentino, M. L., Carelli, V., Vigetti, D., Viola, M., Karousou, E. G., et al. (2007). Assessing heteroplasmic load in Leber's hereditary optic neuropathy mutation 3460G->A/MT-ND1 with a real-time PCR quantitative approach. J. Mol. Diagn. 9, 538–545. doi: 10.2353/jmoldx.2007.060183
Ghelli, A., Porcelli, A. M., Zanna, C., Vidoni, S., Mattioli, S., Barbieri, A., et al. (2009). The background of mitochondrial DNA haplogroup J increases the sensitivity of Leber's hereditary optic neuropathy cells to 2,5-hexanedione toxicity. PLoS ONE 4:e7922. doi: 10.1371/journal.pone.0007922
Giordano, C., Iommarini, L., Giordano, L., Maresca, A., Pisano, A., Valentino, M. L., et al. (2014). Efficient mitochondrial biogenesis drives incomplete penetrance in Leber's hereditary optic neuropathy. Brain 137(Pt 2), 335–353. doi: 10.1093/brain/awt343
Giordano, L., Deceglie, S., d'Adamo, P., Valentino, M. L., La Morgia, C., Fracasso, F., et al. (2015). Cigarette toxicity triggers Leber's hereditary optic neuropathy by affecting mtDNA copy number, oxidative phosphorylation and ROS detoxification pathways. Cell Death Dis. 6:e2021. doi: 10.1038/cddis.2015.364
Gomez-Duran, A., Pacheu-Grau, D., Martinez-Romero, I., Lopez-Gallardo, E., Lopez-Perez, M. J., Montoya, J., et al. (2012). Oxidative phosphorylation differences between mitochondrial DNA haplogroups modify the risk of Leber's hereditary optic neuropathy. Biochim. Biophys. Acta 1822, 1216–1222. doi: 10.1016/j.bbadis.2012.04.014
Gorman, G. S., Schaefer, A. M., Ng, Y., Gomez, N., Blakely, E. L., Alston, C. L., et al. (2015). Prevalence of nuclear and mitochondrial DNA mutations related to adult mitochondrial disease. Ann. Neurol. 77, 753–759. doi: 10.1002/ana.24362
Grady, J. P., Murphy, J. L., Blakely, E. L., Haller, R. G., Taylor, R. W., Turnbull, D. M., et al. (2014). Accurate measurement of mitochondrial DNA deletion level and copy number differences in human skeletal muscle. PLoS ONE 9:e114462. doi: 10.1371/journal.pone.0114462
Grady, J. P., Pickett, S. J., Ng, Y. S., Alston, C. L., Blakely, E. L., Hardy, S. A., et al. (2018). mtDNA heteroplasmy level and copy number indicate disease burden in m.3243A>G mitochondrial disease. EMBO Mol. Med. 10:e8262. doi: 10.15252/emmm.201708262
Green, R. C., Berg, J. S., Grody, W. W., Kalia, S. S., Korf, B. R., Martin, C. L., et al. (2013). ACMG recommendations for reporting of incidental findings in clinical exome and genome sequencing. Genet. Med. 15, 565–574. doi: 10.1038/gim.2013.73
Griffin, H. R., Pyle, A., Blakely, E. L., Alston, C. L., Duff, J., Hudson, G., et al. (2014). Accurate mitochondrial DNA sequencing using off-target reads provides a single test to identify pathogenic point mutations. Genet. Med. 16, 962–971. doi: 10.1038/gim.2014.66
Guo, Y., Li, C.-I., Sheng, Q., Winther, J. F., Cai, Q., Boice, J. D., et al. (2013). Very low-level heteroplasmy mtDNA variations are inherited in humans. J. Genet. Genomics 40, 607–615. doi: 10.1016/j.jgg.2013.10.003
He, L., Chinnery, P. F., Durham, S. E., Blakely, E. L., Wardell, T. M., Borthwick, G. M., et al. (2002). Detection and quantification of mitochondrial DNA deletions in individual cells by real-time PCR. Nucleic Acids Res. 30:e68. doi: 10.1093/nar/gnf067
Henrie, A. H., Hemphill, S. E., Ruiz-Schultz, N., Cushman, B., DiStefano, M. T., Azzariti, D., et al. (2018). ClinVar Miner: demonstrating utility of a web-based tool for viewing and filtering ClinVar data. Hum. Mutat. 39, 1051–1060. doi: 10.1002/humu.23555
Huang, T. (2011). Next generation sequencing to characterize mitochondrial genomic DNA heteroplasmy. Curr. Protoc. Hum. Genet. Chapter 19:Unit19.8. doi: 10.1002/0471142905.hg1908s71
Hudson, G., Nalls, M., Evans, J. R., Breen, D. P., Winder-Rhodes, S., Morrison, K. E., et al. (2013). Two-stage association study and meta-analysis of mitochondrial DNA variants in Parkinson disease. Neurology 80, 2042–2048. doi: 10.1212/WNL.0b013e318294b434
Inagaki, Y., Mashima, Y., Fuse, N., Ohtake, Y., Fujimaki, T., and Fukuchi, T. (2006). Mitochondrial DNA mutations with Leber's hereditary optic neuropathy in Japanese patients with open-angle glaucoma. Jpn. J. Ophthalmol. 50, 128–134. doi: 10.1007/s10384-005-0290-0
Ingman, M., Kaessmann, H., Paabo, S., and Gyllensten, U. (2000). Mitochondrial genome variation and the origin of modern humans. Nature 408, 708–713. doi: 10.1038/35047064
Jiang, P., Jin, X., Peng, Y., Wang, M., Liu, H., and Liu, X. (2016). The exome sequencing identified the mutation in YARS2 encoding the mitochondrial tyrosyl-tRNA synthetase as a nuclear modifier for the phenotypic manifestation of Leber's hereditary optic neuropathy-associated mitochondrial DNA mutation. Hum. Mol. Genet. 25, 584–596. doi: 10.1093/hmg/ddv498
Kalia, S. S., Adelman, K., Bale, S. J., Chung, W. K., Eng, C., Evans, J. P., et al. (2017). Recommendations for reporting of secondary findings in clinical exome and genome sequencing, 2016 update (ACMG SF v2.0): a policy statement of the American College of Medical Genetics and Genomics. Genet. Med. 19, 249–255. doi: 10.1038/gim.2016.190
Khan, N. A., Govindaraj, P., Jyothi, V., Meena, A. K., and Thangaraj, K. (2013). Co-occurrence of m.1555A>G and m.11778G>A mitochondrial DNA mutations in two Indian families with strikingly different clinical penetrance of Leber hereditary optic neuropathy. Mol. Vis. 19, 1282–1289.
Kogelnik, A. M., Lott, M. T., Brown, M. D., Navathe, S. B., and Wallace, D. C. (1996). MITOMAP: a human mitochondrial genome database. Nucleic Acids Res. 24, 177–179. doi: 10.1093/nar/24.1.177
Landrum, M. J., Lee, J. M., Benson, M., Brown, G., Chao, C., Chitipiralla, S., et al. (2016). ClinVar: public archive of interpretations of clinically relevant variants. Nucleic Acids Res. 44, D862–D868. doi: 10.1093/nar/gkv1222
Lek, M., Karczewski, K. J., Minikel, E. V., Samocha, K. E., Banks, E., Fennell, T., et al. (2016). Analysis of protein-coding genetic variation in 60,706 humans. Nature 536, 285–291. doi: 10.1038/nature19057
Leung, D. G., Cohen, J. S., Michelle, E. H., Bai, R., Mammen, A. L., and Christopher-Stine, L. (2018). Mitochondrial DNA deletions with low-level heteroplasmy in adult-onset myopathy. J. Clin. Neuromuscul. Dis. 19, 117–123. doi: 10.1097/CND.0000000000000200
Lieber, D. S. (2013). Computational and Experimental Approaches for Evaluating the Genetic Basis of Mitochondrial Disorders. Harvard University.
Liu, H., Ma, Y., Fang, F., Zhang, Y., Zou, L., Yang, Y., et al. (2013). Wild-type mitochondrial DNA copy number in urinary cells as a useful marker for diagnosing severity of the mitochondrial diseases. PLoS ONE 8:e67146. doi: 10.1371/journal.pone.0067146
Lott, M. T., Leipzig, J. N., Derbeneva, O., Xie, H. M., Chalkia, D., Sarmady, M., et al. (2013). mtDNA variation and analysis using mitomap and mitomaster. Curr. Protoc. Bioinformatics 44, 1 23.1–26. doi: 10.1002/0471250953.bi0123s44
Marcelino, L. A., and Thilly, W. G. (1999). Mitochondrial mutagenesis in human cells and tissues. Mutat. Res. 434, 177–203. doi: 10.1016/S0921-8777(99)00028-2
Marquis, J., Lefebvre, G., Kourmpetis, Y. A. I., Kassam, M., Ronga, F., De Marchi, U., et al. (2017). MitoRS, a method for high throughput, sensitive, and accurate detection of mitochondrial DNA heteroplasmy. BMC Genomics 18:326. doi: 10.1186/s12864-017-3695-5
Martin-Navarro, A., Gaudioso-Simon, A., Alvarez-Jarreta, J., Montoya, J., Mayordomo, E., and Ruiz-Pesini, E. (2017). Machine learning classifier for identification of damaging missense mutations exclusive to human mitochondrial DNA-encoded polypeptides. BMC Bioinformatics. 18:158. doi: 10.1186/s12859-017-1562-7
McCormick, E., Place, E., and Falk, M. J. (2013). Molecular genetic testing for mitochondrial disease: from one generation to the next. Neurotherapeutics 10, 251–261. doi: 10.1007/s13311-012-0174-1
McDonnell, M. T., Schaefer, A. M., Blakely, E. L., McFarland, R., Chinnery, P. F., Turnbull, D. M., et al. (2004). Noninvasive diagnosis of the 3243A > G mitochondrial DNA mutation using urinary epithelial cells. Eur. J. Hum. Genet. 12, 778–781. doi: 10.1038/sj.ejhg.5201216
McElhoe, J. A., Holland, M. M., Makova, K. D., Su, M. S., Paul, I. M., Baker, C. H., et al. (2014). Development and assessment of an optimized next-generation DNA sequencing approach for the mtgenome using the Illumina MiSeq. Forensic Sci. Int. Genet. 13:20–29. doi: 10.1016/j.fsigen.2014.05.007
McFarland, R., Elson, J. L., Taylor, R. W., Howell, N., and Turnbull, D. M. (2004). Assigning pathogenicity to mitochondrial tRNA mutations: when “definitely maybe” is not good enough. Trends Genet. 20, 591–596. doi: 10.1016/j.tig.2004.09.014
Mitchell, A. L., Elson, J. L., Howell, N., Taylor, R. W., and Turnbull, D. M. (2006). Sequence variation in mitochondrial complex I genes: mutation or polymorphism? J. Med. Genet. 43, 175–179. doi: 10.1136/jmg.2005.032474
Moraes, C. T., Atencio, D. P., Oca-Cossio, J., and Diaz, F. (2003). Techniques and pitfalls in the detection of pathogenic mitochondrial DNA mutations. J. Mol. Diagn. 5, 197–208. doi: 10.1016/S1525-1578(10)60474-6
Navarro-Gomez, D., Leipzig, J., Shen, L., Lott, M., Stassen, A. P., Wallace, D. C., et al. (2015). Phy-Mer: a novel alignment-free and reference-independent mitochondrial haplogroup classifier. Bioinformatics 31, 1310–1312. doi: 10.1093/bioinformatics/btu825
Ng, Y. S., Lax, N. Z., Maddison, P., Alston, C. L., Blakely, E. L., Hepplewhite, P. D., et al. (2018). MT-ND5 mutation exhibits highly variable neurological manifestations at low mutant load. EBioMedicine 30, 86–93. doi: 10.1016/j.ebiom.2018.02.010
Niroula, A., and Vihinen, M. (2016). PON-mt-tRNA: a multifactorial probability-based method for classification of mitochondrial tRNA variations. Nucleic Acids Res. 44, 2020–2027. doi: 10.1093/nar/gkw046
Parson, W., and Dur, A. (2007). EMPOP–a forensic mtDNA database. Forensic Sci. Int. Genet. 1, 88–92. doi: 10.1016/j.fsigen.2007.01.018
Patowary, A., Nesbitt, R., Archer, M., Bernier, R., and Brkanac, Z. (2017). Next generation sequencing mitochondrial DNA analysis in autism spectrum disorder. Autism Res. 10, 1338–1343. doi: 10.1002/aur.1792
Preste, R., Vitale, O., Clima, R., Gasparre, G., and Attimonelli, M. (2018). HmtVar: a new resource for human mitochondrial variations and pathogenicity data. Nucleic Acids Res. gky1024. doi: 10.1093/nar/gky1024
Procaccio, V., Neckelmann, N., Paquis-Flucklinger, V., Bannwarth, S., Jimenez, R., Davila, A., et al. (2006). Detection of low levels of the mitochondrial tRNALeu(UUR) 3243A>G mutation in blood derived from patients with diabetes. Mol. Diagn. Ther. 10, 381–389. doi: 10.1007/BF03256215
Putz, J., Dupuis B Fau - Sissler, M., Sissler M Fau - Florentz, C., and Florentz, C. (2007). Mamit-tRNA, a database of mammalian mitochondrial tRNA primary and secondary structures. RNA 13, 1184–1190.doi: 10.1261/rna.588407
Rahman, S., Poulton, J., Marchington, D., and Suomalainen, A. (2001). Decrease of 3243 A–>G mtDNA mutation from blood in MELAS syndrome: a longitudinal study. Am. J. Hum. Genet. 68, 238–240. doi: 10.1086/316930
Richards, S., Aziz, N., Bale, S., Bick, D., Das, S., Gastier-Foster, J., et al. (2015). Standards and guidelines for the interpretation of sequence variants: a joint consensus recommendation of the American College of Medical Genetics and Genomics and the Association for Molecular Pathology. Genet. Med. 17, 405–424. doi: 10.1038/gim.2015.30
Rossignol, R., Faustin, B., Rocher, C., Malgat, M., Mazat, J. P., and Letellier, T. (2003). Mitochondrial threshold effects. Biochem, J. 370(Pt 3), 751–762. doi: 10.1042/BJ20021594
Rueda, M., and Torkamani, A. (2017). SG-ADVISER mtDNA: a web server for mitochondrial DNA annotation with data from 200 samples of a healthy aging cohort. BMC Bioinformatics 18:373. doi: 10.1186/s12859-017-1778-6
Ruiz-Pesini, E., Lott, M. T., Procaccio, V., Poole, J. C., Brandon, M. C., Mishmar, D., et al. (2007). An enhanced MITOMAP with a global mtDNA mutational phylogeny. Nucleic Acids Res. 35, D823–D828. doi: 10.1093/nar/gkl927
Schaefer, A. M., McFarland, R., Blakely, E. L., He, L., Whittaker, R. G., Taylor, R. W., et al. (2008). Prevalence of mitochondrial DNA disease in adults. Ann. Neurol. 63, 35–39. doi: 10.1002/ana.21217
Schmitt, M. W., Kennedy, S. R., Salk, J. J., Fox, E. J., Hiatt, J. B., and Loeb, L. A. (2012). Detection of ultra-rare mutations by next-generation sequencing. Proc. Natl. Acad. Sci. U.S.A. 109, 14508–14513. doi: 10.1073/pnas.1208715109
Seneca, S., Vancampenhout, K., Van Coster, R., Smet, J., Lissens, W., Vanlander, A., et al. (2015). Analysis of the whole mitochondrial genome: translation of the Ion Torrent Personal Genome Machine system to the diagnostic bench? Eur. J. Hum. Genet. 23, 41–48. doi: 10.1038/ejhg.2014.49
Shen, L., Attimonelli, M., Bai, R., Lott, M. T., Wallace, D. C., Falk, M. J., et al. (2018). MSeqDR mvTool: a mitochondrial DNA Web and API resource for comprehensive variant annotation, universal nomenclature collation, and reference genome conversion. Hum. Mutat. 39, 806–810. doi: 10.1002/humu.23422
Smith, P. M., Elson, J. L., Greaves, L. C., Wortmann, S. B., Rodenburg, R. J., Lightowlers, R. N., et al. (2014). The role of the mitochondrial ribosome in human disease: searching for mutations in 12S mitochondrial rRNA with high disruptive potential. Hum. Mol. Genet. 23, 949–967. doi: 10.1093/hmg/ddt490
Sonney, S., Leipzig, J., Lott, M. T., Zhang, S., Procaccio, V., Wallace, D. C., et al. (2017). Predicting the pathogenicity of novel variants in mitochondrial tRNA with MitoTIP. PLoS Comput. Biol. 13:e1005867. doi: 10.1371/journal.pcbi.1005867
Sosa, M. X., Sivakumar, I. K., Maragh, S., Veeramachaneni, V., Hariharan, R., Parulekar, M., et al. (2012). Next-generation sequencing of human mitochondrial reference genomes uncovers high heteroplasmy frequency. PLoS Comput. Biol. 8:e1002737. doi: 10.1371/journal.pcbi.1002737
Tang, S., Wang, J., Zhang, V. W., Li, F. Y., Landsverk, M., Cui, H., et al. (2013). Transition to next generation analysis of the whole mitochondrial genome: a summary of molecular defects. Hum. Mutat. 34, 882–893. doi: 10.1002/humu.22307
Thorburn, D. R., Sugiana, C., Salemi, R., Kirby, D. M., Worgan, L., Ohtake, A., et al. (2004). Biochemical and molecular diagnosis of mitochondrial respiratory chain disorders. Biochim. Biophys. Acta 1659, 121–128. doi: 10.1016/j.bbabio.2004.08.006
Urata, M., Wada, Y., Kim, S. H., Chumpia, W., Kayamori, Y., Hamasaki, N., et al. (2004). High-sensitivity detection of the A3243G mutation of mitochondrial DNA by a combination of allele-specific PCR and peptide nucleic acid-directed PCR clamping. Clin. Chem. 50, 2045–2051. doi: 10.1373/clinchem.2004.033761
van der Walt, E. M., Smuts, I., Taylor, R. W., Elson, J. L., Turnbull, D. M., Louw, R., et al. (2012). Characterization of mtDNA variation in a cohort of South African paediatric patients with mitochondrial disease. Eur. J. Hum. Genet. 20, 650–656. doi: 10.1038/ejhg.2011.262
van der Walt, J. M., Dementieva, Y. A., Martin, E. R., Scott, W. K., Nicodemus, K. K., Kroner, C. C., et al. (2004). Analysis of European mitochondrial haplogroups with Alzheimer disease risk. Neurosci. Lett. 365, 28–32. doi: 10.1016/j.neulet.2004.04.051
van Oven, M., and Kayser, M. (2009). Updated comprehensive phylogenetic tree of global human mitochondrial DNA variation. Hum. Mutat. 30, E386–E394. doi: 10.1002/humu.20921
Vancampenhout, K., Caljon, B., Spits, C., Stouffs, K., Jonckheere, A., De Meirleir, L., et al. (2014). A bumpy ride on the diagnostic bench of massive parallel sequencing, the case of the mitochondrial genome. PLoS ONE 9:e112950. doi: 10.1371/journal.pone.0112950
Vandebona, H., Mitchell, P., Manwaring, N., Griffiths, K., Gopinath, B., Wang, J. J., et al. (2009). Prevalence of mitochondrial 1555A–>G mutation in adults of European descent. N. Engl. J. Med. 360, 642–644. doi: 10.1056/NEJMc0806397
Vellarikkal, S. K., Dhiman, H., Joshi, K., Hasija, Y., Sivasubbu, S., and Scaria, V. (2015). mit-o-matic: a comprehensive computational pipeline for clinical evaluation of mitochondrial variations from next-generation sequencing datasets. Hum. Mutat. 36, 419–424. doi: 10.1002/humu.22767
Wallace, D. C., Fan, W., and Procaccio, V. (2010). Mitochondrial energetics and therapeutics. Annu. Rev. Pathol. 5, 297–348. doi: 10.1146/annurev.pathol.4.110807.092314
Wallace, D. C., Lott, M. T., and Procaccio, V. (2013). “Mitochondrial medicine: the mitochondrial biology and genetics of metabolic and degenerative diseases, cancer, and aging,” in Emery and Rimoin's Principle and Practice of Medical Genetics, 6th Edn. (Waltham, MA: Elsevier), 1–153.
Wang, J., Al-Ouran, R., Hu, Y., Kim, S. Y., Wan, Y. W., Wangler, M. F., et al. (2017). MARRVEL: integration of human and model organism genetic resources to facilitate functional annotation of the human genome. Am. J. Hum. Genet. 100, 843–853. doi: 10.1016/j.ajhg.2017.04.010
Wang, J., Schmitt, E. S., Landsverk, M. L., Zhang, V. W., Li, F. Y., Graham, B. H., et al. (2012). An integrated approach for classifying mitochondrial DNA variants: one clinical diagnostic laboratory's experience. Genet. Med. 14, 620–626. doi: 10.1038/gim.2012.4
Wei, W., Gomez-Duran, A., Hudson, G., and Chinnery, P. F. (2017). Background sequence characteristics influence the occurrence and severity of disease-causing mtDNA mutations. PLoS Genet. 13:e1007126. doi: 10.1371/journal.pgen.1007126
Weissensteiner, H., Forer, L., Fuchsberger, C., Schopf, B., Kloss-Brandstatter, A., Specht, G., et al. (2016a). mtDNA-Server: next-generation sequencing data analysis of human mitochondrial DNA in the cloud. Nucleic Acids Res. 44, W64–W69. doi: 10.1093/nar/gkw247
Weissensteiner, H., Pacher, D., Kloss-Brandstatter, A., Forer, L., Specht, G., Bandelt, H. J., et al. (2016b). HaploGrep 2: mitochondrial haplogroup classification in the era of high-throughput sequencing. Nucleic Acids Res. 44, W58–W63. doi: 10.1093/nar/gkw233
Wolf, C., Gramer, E., Muller-Myhsok, B., Pasutto, F., Wissinger, B., and Weisschuh, N. (2010). Mitochondrial haplogroup U is associated with a reduced risk to develop exfoliation glaucoma in the German population. BMC Genet. 11:8. doi: 10.1186/1471-2156-11-8
Wong, L. J. (2007). Pathogenic mitochondrial DNA mutations in protein-coding genes. Muscle Nerve. 36, 279–293. doi: 10.1002/mus.20807
Wong, L. J. (2010). Molecular genetics of mitochondrial disorders. Dev. Disabil. Res. Rev. 16, 154–162. doi: 10.1002/ddrr.104
Wong, L. J. (2013). Challenges of bringing next generation sequencing technologies to clinical molecular diagnostic laboratories. Neurotherapeutics 10, 262–272. doi: 10.1007/s13311-012-0170-5
Wong, L. J., and Boles, R. G. (2005). Mitochondrial DNA analysis in clinical laboratory diagnostics. Clin. Chim. Acta 354, 1–20. doi: 10.1016/j.cccn.2004.11.003
Yang, H., Liu, R., and Wang, C. C. (2016). Searching the co-occurrence of pathogenic mutations for Leber's hereditary optic neuropathy and hearing loss in more than 26,000 whole mitochondrial genomes. Mitochondrial DNA A DNA Mapp. Seq. Anal. 27, 3399–3402. doi: 10.3109/19401736.2015.1018239
Ye, F., Samuels, D. C., Clark, T., and Guo, Y. (2014). High-throughput sequencing in mitochondrial DNA research. Mitochondrion. 17, 157–163. doi: 10.1016/j.mito.2014.05.004
Yu-Wai-Man, P., Hudson, G., Klopstock, T., and Chinnery, P. F. (2016). Reply: parsing the differences in affected with LHON: genetic versus environmental triggers of disease conversion. Brain 139(Pt 3):e18. doi: 10.1093/brain/awv340
Zaragoza, M. V., Fass, J., Diegoli, M., Lin, D., and Arbustini, E. (2010). Mitochondrial DNA variant discovery and evaluation in human Cardiomyopathies through next-generation sequencing. PLoS ONE 5:e12295. doi: 10.1371/journal.pone.0012295
Keywords: mitochondria, mitochondrial diseases, mitochondrial DNA, next generation sequencing, bioinformatics, mtDNA variant interpretation
Citation: Bris C, Goudenege D, Desquiret-Dumas V, Charif M, Colin E, Bonneau D, Amati-Bonneau P, Lenaers G, Reynier P and Procaccio V (2018) Bioinformatics Tools and Databases to Assess the Pathogenicity of Mitochondrial DNA Variants in the Field of Next Generation Sequencing. Front. Genet. 9:632. doi: 10.3389/fgene.2018.00632
Received: 05 September 2018; Accepted: 27 November 2018;
Published: 11 December 2018.
Edited by:
Ivana Kurelac, Università degli Studi di Bologna, ItalyReviewed by:
Caterina Garone, University of Cambridge, United KingdomRoberto Preste, Università degli Studi di Bari, Italy
Copyright © 2018 Bris, Goudenege, Desquiret-Dumas, Charif, Colin, Bonneau, Amati-Bonneau, Lenaers, Reynier and Procaccio. This is an open-access article distributed under the terms of the Creative Commons Attribution License (CC BY). The use, distribution or reproduction in other forums is permitted, provided the original author(s) and the copyright owner(s) are credited and that the original publication in this journal is cited, in accordance with accepted academic practice. No use, distribution or reproduction is permitted which does not comply with these terms.
*Correspondence: Vincent Procaccio, dmlwcm9jYWNjaW9AY2h1LWFuZ2Vycy5mcg==