- 1Division of Human Genetics, Department of Pathology, University of Cape Town, Cape Town, South Africa
- 2Research Laboratory, Botswana Harvard AIDS Institute Partnership, Gaborone, Botswana
The interesting history of Southern Africa has put the region in the spotlight for population medical genetics. Major events including the Bantu expansion and European colonialism have imprinted unique genetic signatures within autochthonous populations of Southern Africa, this resulting in differential allele frequencies across the region. This genetic structure has potential implications on susceptibility and resistance to infectious diseases such as human immunodeficiency virus (HIV) infection. Southern Africa is the region affected worst by HIV. Here, we discuss advances made in genome-wide association studies (GWAS) of HIV-1 in the past 12 years and dissect population diversity within Southern Africa. Our findings accentuate that a plethora of factors such as migration, language and culture, admixture, and natural selection have profiled the genetics of the people of Southern Africa. Genetic structure has been observed among the Khoe-San, among Bantu speakers, and between the Khoe-San, Coloureds, and Bantu speakers. Moreover, Southern African populations have complex admixture scenarios. Few GWAS of HIV-1 have been conducted in Southern Africa, with only one of these identifying two novel variants (HCG22rs2535307 and CCNG1kgp22385164) significantly associated with HIV-1 acquisition and progression. High genetic diversity, multi-wave genetic mixture and low linkage disequilibrium of Southern African populations constitute a challenge in identifying genetic variants with modest risk or protective effect against HIV-1. We therefore posit that it is compelling to assess genome-wide contribution of ancestry to HIV-1 infection. We further suggest robust methods that can pin-point population-specific variants that may contribute to the control of HIV-1 in Southern Africa.
Introduction
Southern Africa extends across a 2.7 million km2 land in the southernmost part of Africa and is the home to about 66 million people (Worldometers, 2019). The region comprises 10 mainland countries: Angola, Botswana, Lesotho, Malawi, Mozambique, Namibia, South Africa, eSwatini, Zambia, and Zimbabwe (Marks, 2014). The region carries the highest burden of human immunodeficiency virus/acquired immune deficiency syndrome (HIV/AIDS). Around 37 million people live with HIV globally, and over half of these live in Southern and eastern Africa (UNAIDS, 2017). Despite high exposure to HIV, some people stay uninfected (Fowke et al., 1996), while those infected exhibit heterogeneous clinical outcomes of HIV infection and to an extent also differential antiretroviral drug metabolism (Carr et al., 2017). This heterogeneity is partly due to underlying diversity in host genetics (Telenti and Goldstein, 2006).
Genetic diversity within and between human populations can be inferred from the observed distributions of genetic allele frequencies in populations, a concept also known as population (genetic) structure (Wright, 1950; Underhill and Kivisild, 2007). The classic measure of genetic population structure is Wright’s fixation index, FST, a type of ANOVA statistic (Tishkoff and Williams, 2002; Jakobsson et al., 2013). The value ranges from zero to one, with FST scores close to one indicating a high degree of population divergence (i.e., most genetic variation between populations), and FST scores close to zero indicating strong population similarity and gene flow (i.e., most genetic variation within populations) (Tishkoff and Williams, 2002). Geographic isolation, migration, population bottleneck, admixture, language and culture, and natural selection have played significant roles in contributing to variations in allele frequencies and the genetic landscape of Southern Africa (Campbell and Tishkoff, 2008; Campbell and Tishkoff, 2010; Botigue et al., 2013; Breton et al., 2014; Gurdasani et al., 2014; Li et al., 2014; Macholdt et al., 2014; Chimusa et al., 2015) as illustrated in Figure 1.
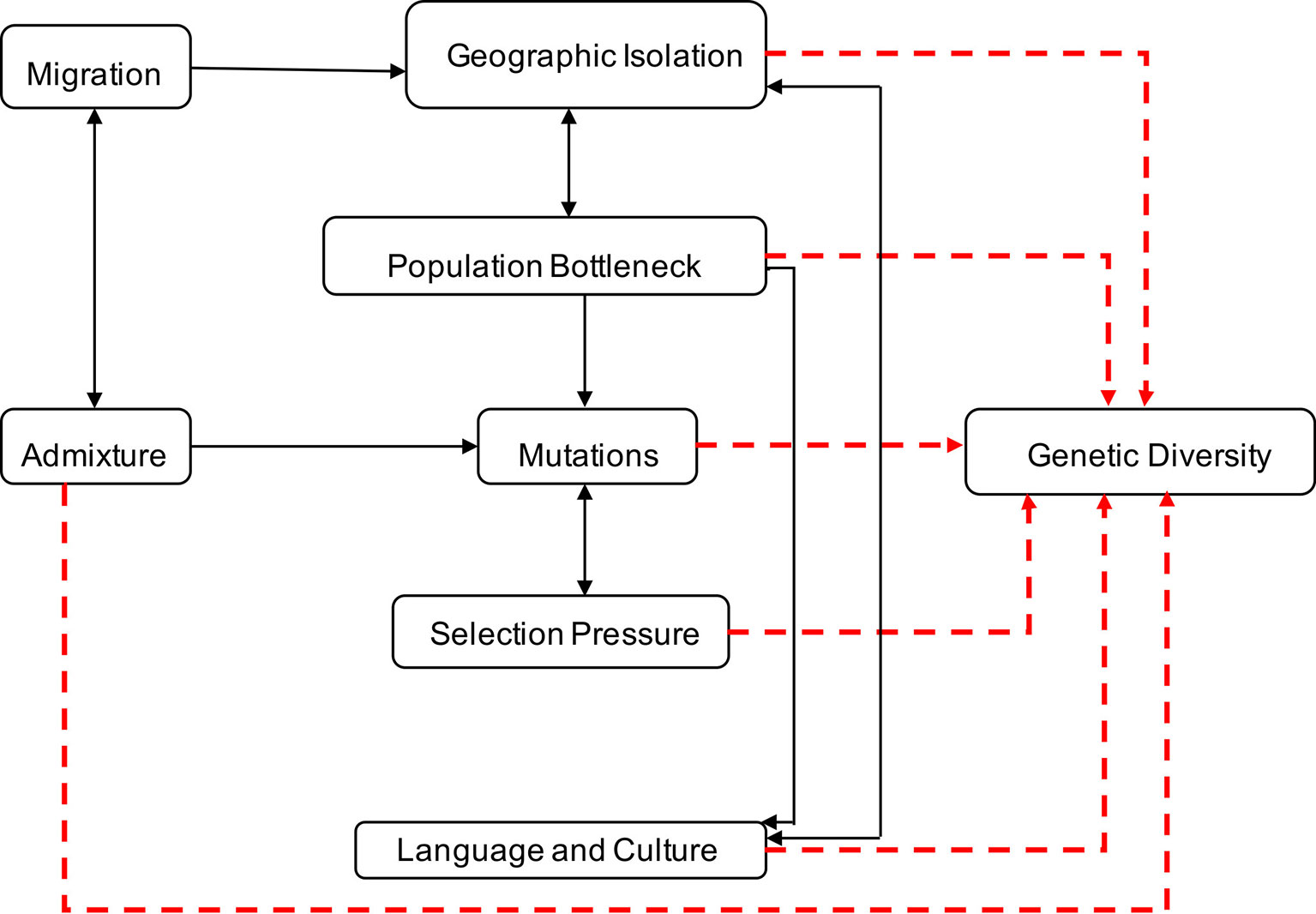
Figure 1 Factors contributing to human genetic diversity within Southern Africa. Although it is complex to represent the interplay between geographic isolation, migration, population bottleneck, admixture, language, culture, and natural selection on the genetic structure of Southern Africa, this figure provides a basic illustration of some of the factors that shaped the genetics of the people of Southern Africa. Geographic distribution and migration into Southern Africa have influenced population admixture and geographic isolation, yielding to population bottleneck, mutation, and selection, that shaped the genetics, culture, and language diversity in Southern Africa.
Genome-wide association studies (GWAS) are approaches of scanning the genome to identify in silico variants that confer susceptibility or resistance to a particular disease through statistical association models (Hirschhorn and Daly, 2005; Hutcheson et al., 2008). Unlike candidate gene-based methods that require a priori knowledge of suspected genes, GWAS have the potential to discover novel genomic loci (Telenti and Goldstein, 2006). Although GWAS are powerful techniques, the variants discovered through these methods (Purcell et al., 2007; Kang et al., 2010; Yang et al., 2011; Wen et al., 2018) have not accounted for all of the variability in viral load (Fellay et al., 2007; Fellay et al., 2009; Pereyra et al., 2010). The overall heritability of set point viral load in populations of European ancestry measured through GWAS was estimated to be 24.6%. Common variants contributed largely to this estimate of heritability (McLaren et al., 2015; Tough and McLaren, 2019). Like other complex traits, this highlights the importance of solving the missing heritability of HIV-1 infection phenotypes which might be uncovered by discovering factors such as rare variants, structural variants, and gene-gene and gene-environment interactions responsible for inter-host variability of viral load (Verma and Ritchie, 2018).
Confounders such as population structure can affect GWAS results. These have to be controlled to avoid spurious results (Hirschhorn and Daly, 2005; Price et al., 2006; Tishkoff et al., 2009; McLaren and Carrington, 2015). Moreover, characterizing genetic structure is crucial for reconstruction of human population history (Tishkoff et al., 2009). In general, African populations have the highest genetic variation and lower linkage disequilibrium (LD) among loci (Campbell and Tishkoff, 2008; The 1000 Genomes Project Consortium, 2010; Choudhury et al., 2018); therefore, not all tag-single nucleotide polymorphism (SNPs) selected from other populations can be used as proxies in African populations. Risk alleles can be structured in populations due to multiple demographic factors and genetic ancestry contributions (Botigue et al., 2013; Gurdasani et al., 2014; Chimusa et al., 2015; Skoglund et al., 2017). The people of Southern Africa are culturally, linguistically, and genetically diverse; the region has been underrepresented in previous genetic diversity studies (Awany et al., 2018; Choudhury et al., 2017; Sirugo et al., 2019).
Most GWAS were performed in non-African populations (Awany et al., 2018; Sirugo et al., 2019) in which HIV-1B is the prevalent subtype. It is possible that the genetics underlying the control of HIV-1 in Southern African is different from these other populations. Considering these genetic differences between African and other populations, and due to the enormous burden of HIV within Southern Africa, it is imperative to dissect human genetic diversity and investigate the role of genetic landscape on HIV acquisition and progression within the region. Deducing a comprehensive architecture of HIV host genetics in Southern Africa will assist in the development of population-specific interventions against HIV. Hence, this review aims to present a comprehensive discussion of the advances made in the GWAS of HIV-1 and document common variants within Southern Africa associated with HIV-1 infection.
We used PubMed search engine to retrieve HIV-1 GWAS studies which have been published in the past 12 years (2007–2019); species was restricted to the human species. The specific search terms were the following:((“genome”[MeSH Terms] OR “genome”[All Fields]) AND wide[All Fields] AND (“association”[MeSH Terms] OR “association”[All Fields]) AND (“hiv-1”[MeSH Terms] OR “hiv-1”[All Fields])) AND (“2007/01/01”[PDat]: “2019/04/30”[PDat] AND “humans”[MeSH Terms]). Ninety-eight items were retrieved; articles relevant to Southern Africa were used in the review. Cited studies which were not in the search results were directly searched for. To review population structure and admixture in Southern Africa, a relaxed search of the terms (population structure and Southern Africa; human genetic diversity and Southern Africa; admixture and Southern Africa) was performed in PubMed, and relevant articles were selected for this review. SNP annotations were confirmed on dbSNP (Sherry et al., 2001). A map of migration routes (refer to the Migration Into Southern Africa section) was created using maps package in R and edited using MacOS Preview software. We conclude with a discussion of research areas where further work on GWAS of HIV-1 is needed.
Migration Into Southern Africa
Demic diffusion has been shown to have a paramount role in genetic drift and gene flow, the two most important events that can trigger population structure. The major expansions of humans date as far back as 100,000 years ago (the Out of Africa (OOA) model) (Campbell and Tishkoff, 2008). One of the major human expansions that occurred in relatively recent years is the Bantu expansion that happened 3,000–5000 years ago. The Bantu dispersed from western Africa, in the region known as the proto-Bantu homeland in eastern Nigeria and western Cameroon (Cavalli-Sforza et al., 1993; Soodyall, 2006; de Filippo et al., 2010; Li et al., 2014).
Migration routes of the Bantu populations have been well described (Li et al., 2014). According to archeological, linguistics, and genetics evidence, two main theories of the split of Bantu speakers from their western African homeland have been postulated: a) migration of the eastern Bantu speakers directly from western Africa to eastern Africa, and thereafter the ancestors of Nguni and Sotho-Tswana speakers moved between 1,200 and 1,000 years into Southern Africa through Great Zimbabwe (Soodyall, 2006; Li et al., 2014) and b) migration of western Bantu speakers through southern Cameroon directly to Southern Africa. A hypothesis supported by Bayesian tree methods stated that the eastern and western Bantu speakers might have split later after passing through the central African rainforest (Li et al., 2014).
In more recent history, Southern Africa has seen an influx of migrants from other continents such as Europe and Asia. By 1750 the Dutch and Portuguese had colonized Southern Africa on the southwest and east coasts, respectively (Hall, 1993). These colonial powers brought slaves and exiles from Indonesia, Malaysia, east coast of Africa, Madagascar and India, to provide labor in wine and wheat farms in Cape Town, the then Cape of Good Hope (Hall, 1993; de Wit et al., 2010). Intermarriages and liaison between these populations resulted in progeny who are now termed “Coloureds” (de Wit et al., 2010). The major migration routes into Southern Africa are depicted in Figure 2.
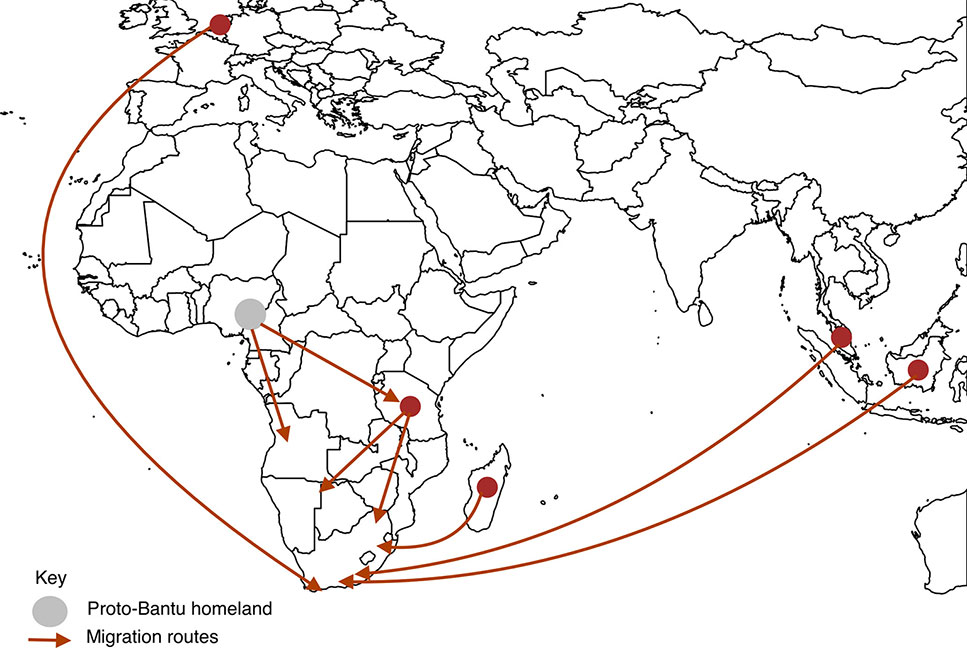
Figure 2 Migration routes into Southern Africa. We present here the main routes of pre-historic migration of the ancestors of the Southern African inhabitants. The circles represent places of origin, the gray circle being the proto-Bantu land and dark red circles being other places of origin. The arrows represent movement of people from places of origin into Southern Africa.
Language and Cultural Diversity in Southern Africa
The inhabitants of Southern Africa could be distinctly classified according to ways of subsistence and food production: hunters and gatherers (and fishers) and the agro-pastoralists (Phillipson, 1977). It has been suggested that the distribution of languages in Southern Africa is an effect of demic diffusion rather than language diffusion alone (Huffman, 1970; Li et al., 2014). Southern Africa was previously occupied by hunter-gatherers who had their own unique culture. Food production was a major drive in human expansions as evidenced in the spread of farming from the cradle in western Africa (Neumann et al., 2012; Russell et al., 2014). Farming was able to support higher population densities (population explosion) than hunting and gathering, which gave farmers an edge over the indigenous inhabitants. As such the culture and languages of hunter-gatherers have been replaced by that of early farmers (Diamond and Bellwood, 2003).
In general, African populations can be classified into four main ethnolinguistic groups: Niger-Kordofanian, Afroasiatic, Nilo-Saharan, and Khoisan (Tishkoff et al., 2009). Over 200 million sub-Saharan inhabitants speak a collective of related languages known as Bantu languages. Bantu speakers are a sub-group of the Niger-Kordofanian ethnolinguistic group (Heine and Nurse, 2000; Reed and Tishkoff, 2006; Campbell and Tishkoff, 2010; Li et al., 2014; Veeramah and Hammer, 2014). Majority of Southern African inhabitants are Bantu speakers and Khoisan-speakers. Over 50 Bantu languages are spoken in Southern Africa Eberhard et al. (2019), these include but are not limited to Tswana, Nama, Xhosa, Zulu, Ndebele, Swati, Sotho, Shona, Bemba, and Makua (Heine and Nurse, 2000; Soodyall, 2006).
Khoisan language families include Khoe, !Xun (Ju), Ta’a (including !Xo), !Wi, and Southwestern or Cape /Xam. The Khoe and the San intermixed resulting in linguistic affinities and diffusion of culture between the two groups; this led to them collectively being referred to as the Khoe-San (Schlebusch, 2010; Schlebusch et al., 2016). The present-day Khoe-San inhabit the central Kalahari Desert and Okavango swamps of Botswana, much of Namibia, Angola, Zimbabwe, Cape Province, and some parts of Lesotho. It is believed that the Khoe-San occupied most of Southern Africa before the arrival of farmers who displaced the Khoe-San to the desert (Barnard, 1992; Li et al., 2014). The Khoe-San are the indigenous inhabitants of Southern Africa. Analysis of genome-wide data and the lactase (LCT) region has shown that the Khoe originated from the assimilation of the San into eastern African Bantu-speaking populations (Schlebusch et al., 2012; Breton et al., 2014; Sadr, 2015; Schlebusch et al., 2016).
Using autosomal SNPs, the Pygmy (a Central Africa population), the Hadza, and Sandawe clustered together with Southern African Khoe-Sans. This suggested that the Pygmy, Southern African Khoe-San, Hadza, and Sandawe populations are a remnant of a proto-Khoe-San-Pygmy population (Tishkoff et al., 2009). Although there have been postulations of the San also originating in East Africa (Soodyall, 2006; Campbell and Tishkoff, 2008; Gonder et al., 2007; Barbieri et al., 2013a; Morris et al., 2014), the origin of the San in Southern Africa cannot be ruled out. In fact, Nurse et al. (1985) suggests that by the 17th century the San lived in most parts of Southern Africa. Before the arrival of Bantu speakers, the Southern San inhabited the foothills of the Drakensberg mountains, the northern, eastern, and western Cape provinces of South Africa, and the southmost parts of Botswana and Namibia [Supplementary information (Schlebusch et al., 2016)]. The rock paintings in Tsodilo Hills in northwestern Botswana show that the Northern San had been living around Tsodilo Hills by the first millennium AD before the arrival of the Khoe and the Bantu speakers.
The Southern African Khoe-San underwent a population bottleneck due to barriers such culture, language, and geographic isolation (i.e., the Kalahari Desert, which could not allow their interaction and contact with other populations). The Khoe-San also underwent genocide at the hands of Bantu speakers and European settlers. This stemmed from conflicts over land which the colonists had invaded while the Khoe-San also hunted the colonists’ cattle, as they used to hunt antelopes and elands (Adhikari, 2010; Schlebusch et al., 2016). The population decline was also due to the Khoe-San succumbing to diseases such as smallpox which were introduced by the settlers (Chimusa et al., 2015). It is speculated that smallpox might have been introduced into the Cape through passengers from India and then spread to European settlers who might have passed it to the Khoe-San. Since the Khoe-San had less resistance to the disease, this drastically reduced their population numbers. The acquisition of Khoe-San women as wives by the European settlers due to the small number of European women also diminished the gene pool of the Khoe-San, this further exacerbating the bottleneck of the Khoe-San population (Nurse et al., 1985).
Bantu speakers were agro-pastoralists, while the Khoe were pastoralists and the San were hunter-gatherers (Breton et al., 2014). There has been some cultural (Breton et al., 2014) and language diffusion within Southern Africa. Through interaction, the San acquired the pastoralism lifestyle from the Bantu and Khoe populations (Breton et al., 2014). Although the click consonants have been connected to the Khoe-San, these have been borrowed into some of the Bantu groups such as the Zulu and Xhosa populations (Barbieri et al., 2013a; Marks et al., 2015). Interactions and trade among Southern African populations has resulted in cultural diffusion which has driven intermixing of the different populations; this has in turn shaped the genetics of Southern African populations.
Population Genetic Diversity in Southern Africa
The gene pool of Southern Africa is a mosaic of alleles from multiple ancestries (Montinaro et al., 2017). Past historical events such as geographical isolation, colonialism, and the Bantu expansion have helped to shape the genetic landscape of Southern Africa (Choudhury et al., 2017). When determining variation between the Khoe and San, using two genetic marker systems, mitochondrial DNA (mtDNA) and Y-chromosome, Soodyall and colleagues could not unambiguously distinguish the Khoe from the San (Soodyall et al., 2008). According to the authors, the Khoe and the San share a common gene pool, which suggests that they branched off from a common ancestry.
Conversely, genome-wide studies analyzing millions of SNPs revealed that the Khoe and the San are a genetically diverse group (Schlebusch et al., 2012). Schlebusch et al. (2012) analyzed 2.3 million SNPs of autosomal chromosomes from 220 individuals representing 11 populations from Southern Africa. These populations were Ju/’hoansi, !Xun, /Gui and //Gana, Karretjie, ‡Khomani, Nama, Khwe, “Coloured” (Colesberg), “Coloured” (Wellington), Herero, and Bantu speakers (South Africa). In their report, there was a distinct stratification in the Khoe-San group; there was a separation between the Ju speakers (!Xun and Ju/’hoansi) who are the San, and the Nama representing Khoe speakers. This is consistent with the findings of a study by Uren et al. (2016) who found fine-scale structure between the ‡Khomani and Nama of South Africa.
The Khoe-San are genetically distinct from the Bantu populations (Table 1). In the Schlebusch et al. (2012) study, a variant associated with “fast-twitching” muscles and elite athletic performance had greater frequencies (>90%) in the Khoe-San groups than in other Southern African populations. Moreover, a strong signal of selection was found around the human leukocyte antigen (HLA) complex in several genes that are known to protect against infectious diseases in the ‡Khomani and Karretjie, this effect probably owing to early and extensive contact with European colonists and novel infectious diseases such as smallpox (Schlebusch et al., 2012). Likewise, Tau and colleagues also reported significant genetic differences between the Khoe-San and Bantu-speaking groups through analysis of short tandem repeats (STRs) (Tau et al., 2017).
In another study, SNPs associated with lactase persistence (LP) from eight Khoe-San and seven Bantu-speaking groups from Botswana, Namibia, and Zambia were characterized (Macholdt et al., 2014). LP is a distinctive trait of pastoralism. LP alleles were first identified in eastern Africa (Tishkoff et al., 2007) where the alleles have faced a strong positive selection. In the study conducted by Macholdt et al. (2014), -14010*C was the most frequent SNP with a collective higher prevalence in pastoralists (20.2%) than in foragers (6.7%) or agriculturalists (1.3%). Within the pastoralists group, -14010*C was observed at the highest frequency of 36% in the Nama population. This further shows the implication of migration and gene flow from the east of Africa into Southern Africa through Khoe speakers.
Y-STR analysis of Southern African populations revealed regional variation between the populations of Botswana, Zimbabwe, Namibia, and South Africa (Tau et al., 2015). Whole genome sequences of Southern African populations also revealed genetic variation among the Bantu of South Africa and among Khoe-San groups. Principal component analysis and structure analysis revealed significant genetic differentiation (p < 10-6) between the Xhosa and Sotho groups (Choudhury et al., 2017). FST analysis revealed genomic regions with high divergence between the two groups (Average FST ≥ 0.3) (Choudhury et al., 2017; Supplementary Table 11). There have been reports of a weak clustering of Bantu-speaking populations that might be underpinned by admixture (May et al., 2013; Retshabile et al., 2018). Southern (and eastern) African populations have greater genetic diversity than other populations closer to the western African origin. This might be due to admixture that arose from the mixing of eastern and Southern African Bantu populations with indigenous populations of the regions (Schlebusch et al., 2012; Chan et al., 2015). Admixture between genetically isolated and differentiated populations can result in a mosaic of population-specific chromosomal blocks (Daya et al., 2014; Sanderson et al., 2015; Chimusa et al., 2018).
Patterns of Admixture in Southern Africa
Bantu groups reached Southern Africa 1,200–2,000 years ago (Ramsay et al., 1996; Soodyall, 2006; Barbieri et al., 2014) and reached the southeastern Cape 1,300 years ago (Breton et al., 2014). Arriving in Southern Africa, Bantu groups did not replace indigenous populations entirely; instead, there have been assimilations of the indigenous populations into the Bantu groups through intermixing and intermarriages especially between migrant men and Khoe-San women (Nurse et al., 1985; Diamond and Bellwood, 2003; Barbieri et al., 2014; Li et al., 2014).
L0d and L0k mtDNA haplogroups have been previously shown to be localized in the Khoe-San (Behar et al., 2008; Barbieri et al., 2013b; Schlebusch et al., 2013). Barbieri et al. (2014) examined mtDNA haplogroups L0d and L0k to measure admixture between the Khoe-San and Bantu speakers. The presence of these mtDNA lineages in Bantu-speaking populations may suggest very ancient admixture with the Khoe-San (Barbieri et al., 2013b). The authors showed that these haplogroups were shared with the Khoe-San, with a group such as the Kgalagadi of southern Botswana harboring 53% frequency of the haplogroups (Barbieri et al., 2014). A considerable amount of admixture was also observed between the Bantu speakers of South Africa (Zulu and Xhosa populations) through elucidation of Y-chromosome haplogroups (Naidoo et al., 2010). Consistent with this finding, Gurdasani et al. (2014) found hunter-gatherers admixture across African populations, the greatest level of admixture being in Zulu and Xhosa populations.
Y-chromosome and mtDNA analyses of Southern African populations suggest patterns of sex-biased migration and admixture (Underhill and Kivisild, 2007). The Bantu expansion was possibly a male-dominated event which led to Khoe-San females being assimilated into Bantu-speaking populations (Bajic et al., 2018). Henn et al. found that approximately 35% of paternal lineages in the Khoe-San group were either of Bantu or European origin (Henn et al., 2011). This sex-biased interaction between migrant men and Khoe-San females has led to asymmetrical patterns of admixture in the region (Table 1). A common pattern is the flow of Y-chromosome from migrant men into Khoe-San populations or the flow of mtDNA from Khoe-San women into migrant populations (Choudhury et al., 2017; Bajic et al., 2018; Oliveira et al., 2019).
Precolonial admixture of indigenous Southern African populations with populations of west Eurasian ancestry has also been documented (Pickrell et al., 2014). Due to the age of this admixture and suggestive linguistic, archeological, and genetic evidences of migration into Southern Africa from eastern Africa, Pickrell et al. (2014) concluded that the precolonial west Eurasian ancestry in Southern Africa is due to the indirect gene flow through eastern Africa. Signatures of admixture in Khoe-San groups have also been observed. In their study, Pickrell and colleagues detected ∼6% non-Khoe-San ancestry in the Ju’|hoan North (Pickrell et al., 2012). Moreover, a genetic component common in Southeastern Bantu speakers was observed in most Khoe-San groups from Botswana, Lesotho, and South Africa (Montinaro et al., 2017).
The “Coloured” of South Africa (SAC) are another popular, well-studied population in Southern Africa (Table 1). The SAC show the highest level of intercontinental admixture, with high levels of Southern African Khoisan, Niger-Khodofanian, Indian, and European ancestries and low levels of Asian and Cushitic ancestries (Tishkoff et al., 2009). Consistent to this insight, a multi-faceted admixture scenario (five-way admixture) has been documented in a study that sought to develop a method (PROXYANC) that could detect the best proxy ancestry in admixture in the SAC population (Chimusa et al., 2013a). The results of this study suggested the admixture to be a combination of Europeans (16%), Xhosa (33%), Gujarati (12%), Chinese (7%), and ‡Khomani (31%) ancestries.
Recent direct admixture involving Eurasian groups has been detected using GLOBETROTTER, an R program, (Hellenthal et al., 2014) in the ‡Khomani and Karretjie of South Africa, dating back to five generations (220 years ago) which aligns with the period of European settlers’ arrival in Southern Africa (Busby et al., 2016). Congruent with this, in a recent study Chimusa et al. (2018) dated admixture in the SAC population to approximately 4 ± 1 generations ago, which likely reflects the wave of admixture that occurred during the era of colonialism in Southern Africa.
Admixture mapping is increasingly becoming an indispensable tool for predicting diseases in admixed populations. Admixture mapping is a method of measuring the contribution of ancestry to a phenotype (Smith et al., 2004; Chimusa et al., 2015; Chi et al., 2019). In Southern Africa, a GWAS of tuberculosis (TB) in the SAC population revealed a link between San ancestry and susceptibility to Mycobacterium tuberculosis (Chimusa et al., 2013b; Daya et al., 2014).
History of HIV in Southern Africa
AIDS was first recognized as a new disease in 1981 when multiple young homosexual men in Los Angeles and New York presented with opportunistic infections and a rare form of Kaposi’s Sarcoma (Greene, 2007; Sharp and Hahn, 2011). HIV was later confirmed as the causative agent of AIDS (Gallo et al., 1984). There are two types of HIV: HIV-1 and HIV-2. HIV-1 is classified into three groups; Main group (M), Outlier group (O), and non-M-non-O group (N). Due to high mutation and recombination rates of HIV-1, the pandemic group, M, is further divided into genetic subtypes A–D, F–H, J–K, and intersubtype recombinants, as well as circulating recombinant forms (CRFs) and unique recombinant forms (URFs). Over half of global HIV infections are caused by HIV-1. HIV-1 group M has a worldwide distribution; subtype A is more prevalent in eastern Europe, subtype B in North America, South America, and western Europe, and subtype C in Southern Africa and India. HIV-2, which is more prevalent in western Africa, is divided into subtypes A–H and is less prevalent and less pathogenic than HIV-1 (Hemelaar et al., 2011; Eberle and Gurtler, 2012; Wilkinson et al., 2015).
Southern Africa has the highest HIV prevalence globally with countries such as eSwatini leading with 27.4% [Figure 3, (Taha, 2011; Vermund et al., 2015)]. The predominant subtype in the region is HIV-1C (Wilkinson et al., 2015). Using coalescence and molecular clock methods, HIV-1C was traced to the late 1930s in the Democratic Republic of Congo (Wilkinson et al., 2015). This strain is believed to have later spread to Southern and eastern Africa (Hemelaar et al., 2011; Faria et al., 2014; Wilkinson et al., 2015). Although the first samples of HIV-1 were sampled in South Africa in 1985, the origin of HIV-1C epidemic in Southern Africa was placed around 1960 [95% highest posterior density (HPD) 1956–1964] (Wilkinson et al., 2015).
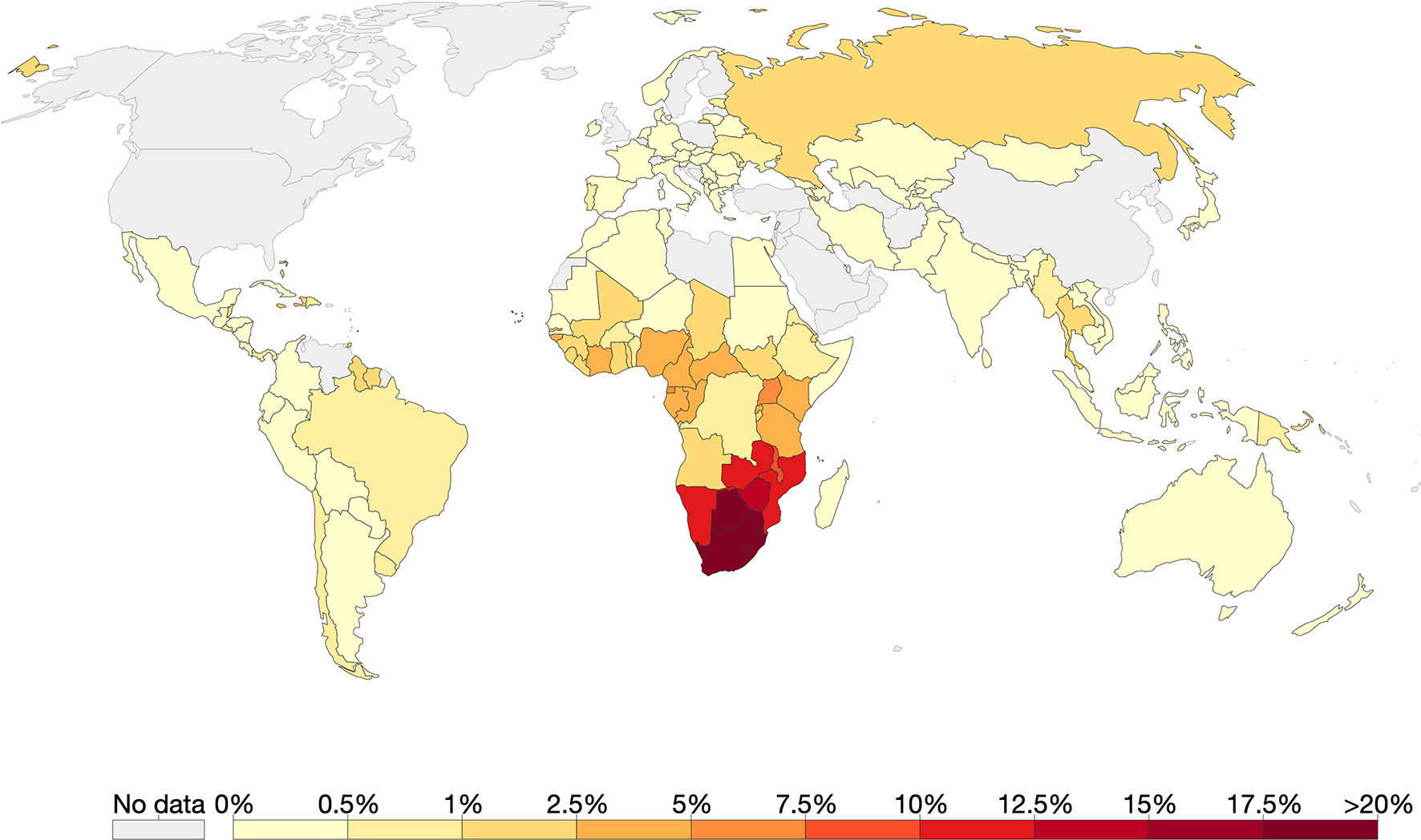
Figure 3 The global prevalence of HIV as of 2017. Of all the countries that had data, Southern Africa had the highest HIV prevalence. Source: Our World in Data; data provided by UNAIDS.
The first anti-HIV drug, azidothymidine (AZT) also known as zidovudine, was approved in 1987. This was an anti-cancer drug that was also found to inhibit reverse transcriptase (Greene, 2007). AZT and subsequent monotherapies were short-lived due to limitations including low genetic barrier to resistance and toxicity. This led to the introduction of combination therapy of multiple antiretrovirals [cART or highly active antiretroviral therapy (HAART)] with better tolerability and potency (Arts and Hazuda, 2012; Vella et al., 2012). In the early 1990s, there was high morbidity and mortality as a result of HIV in most Southern African countries (Kagaayi and Serwadda, 2016). The region has since seen a steep decline of 30% in HIV-related mortality between 2010 and 2017 due to rapid scale-up of antiretroviral therapy (UNAIDS, 2018). However, these drugs only suppress HIV to undetectable levels; they do not eliminate HIV from cellular reservoirs (McLaren and Carrington, 2015). While current therapeutic measures are geared towards elimination of HIV in cellular reservoirs and the use of broadly neutralizing antibodies against HIV, effective vaccination against HIV remains a hurdle due to ineffective immune responses against HIV and high variability of HIV sequence (Halper-Stromberg and Nussenzweig, 2016; Siliciano and Siliciano, 2016; Hsu and O’Connell, 2017).
Southern African countries concomitantly bear the brunt of both HIV and TB (Corbett et al., 2003; John et al., 2007; Abdool Karim et al., 2009; Dokubo et al., 2014; Tafuma et al., 2014). In 2017 an estimate of 10 million people developed active TB, and 72% of these were HIV-infected people living in Africa (WHO, 2018). HIV is the greatest predictor of development of active TB; susceptibility to active TB development increases 20-fold in people infected with HIV (Pawlowski et al., 2012). HIV/TB coinfection is the leading cause of mortality in Southern Africa even in people receiving antitubercular and antiretroviral therapy (Bisson et al., 2015; Campa et al., 2017). In a HIV/TB coinfection scenario, TB has a negative impact on immune response to HIV, thus exacerbating progression to AIDS (Bruchfeld et al., 2015). Another complication associated with HIV infection is the manifestation of adverse restoration of immune responses, known as immune reconstitution inflammatory syndrome (IRIS), which occurs during the initial months of antiretroviral treatment (Lawn et al., 2005). Although IRIS unmasks a spectrum of pre-existing opportunistic infections, it is commonly associated with mycobacterial infections (Lawn et al., 2005; Meintjes et al., 2008). TB-IRIS is a major health challenge in the developing world (Bruchfeld et al., 2015; Walker et al., 2018).
Pre-GWAS of HIV-1: Candidate AIDS Restriction Genes
One of the striking characteristics of HIV infection is the highly heterogeneous viral set point across infected individuals. Set point is the viral load of an individual that fluctuates around a steady point during the asymptomatic phase of HIV infection (Fraser et al., 2007). The difference in viral set point between individuals can be as high as 1,000-fold (Mellors et al., 1996; Fellay et al., 2007; Fraser et al., 2007). Some of this variability is underpinned by host genetic factors. Following the discovery that HIV infected cells by binding to CD4 cells and CCR5, the first AIDS restriction gene (ARG) to be discovered was CCR5-Δ32 (Dean et al., 1996). Subsequently, a number of SNPs associated with HIV-1 were identified by candidate gene analysis in other ARGs (O’Brien and Nelson, 2004; Hutcheson et al., 2008; Winkler, 2008). These ARGs include those encoding HLA class I antigen presentation molecules (Carrington et al., 1999; Gao et al., 2001; Carrington and O’Brien, 2003; Welzel et al., 2007); chemokines and chemokine receptor genes involved in HIV-1 cell entry such as CCR2, CXCR6, and CCL5 (RANTES) (Smith et al., 1997; An et al., 2002; Duggal et al., 2003); natural killer cell immunoglobin-like receptors (KIR) genes (Martin et al., 2002); cytokines (Shin et al., 2000); and the intrinsic viral restriction factors TRIM5 (Javanbakht et al., 2006) and APOBEC3G (An et al., 2004). A detailed discussion of these SNPs and harboring genes can be found in these reviews (O’Brien and Nelson, 2004; Hutcheson et al., 2008).
Candidate gene methods have been pivotal in revealing genes that may be used as targets for drug development (van Manen et al., 2011). For instance, CCR5 variants have enabled the development of HIV-1 entry inhibitor drugs (Troyer et al., 2011). Maraviroc, which is a CCR5 antagonist, is currently the only chemokine receptor in clinical use (Henrich and Kuritzkes, 2013). Through the identification of CCR5-Δ32, to date two people have maintained complete HIV-1 remission after a CCR5-Δ32 stem transplant (Hutter et al., 2009; UNAIDS, 2019). The ARGs identified by candidate gene analysis explained about 10% of the variability in HIV-1 infection (O’Brien and Nelson, 2004; Hutcheson et al., 2008). HapMap annotation of about 3 million human SNPs has facilitated the development of high-density arrays with 500,000 to 1,000,000 variants that could be utilized in the screening of variants associated with any disease (The International HapMap Consortium, 2003; Hutcheson et al., 2008; Winkler, 2008); this set the stage for HIV-1 GWAS.
A Global Perspective of GWAS of HIV-1
GWAS are useful tools for identifying genetic contributions towards predisposition to infection, progression to disease, and differential drug metabolism (Adebamowo et al., 2017). GWAS offer an unbiased opportunity to screen the entire genome and pinpoint variants implicated in a given phenotype of interest. Here and in the subsequent section, we present an account of GWAS of HIV-1 as illustrated in Table 2.
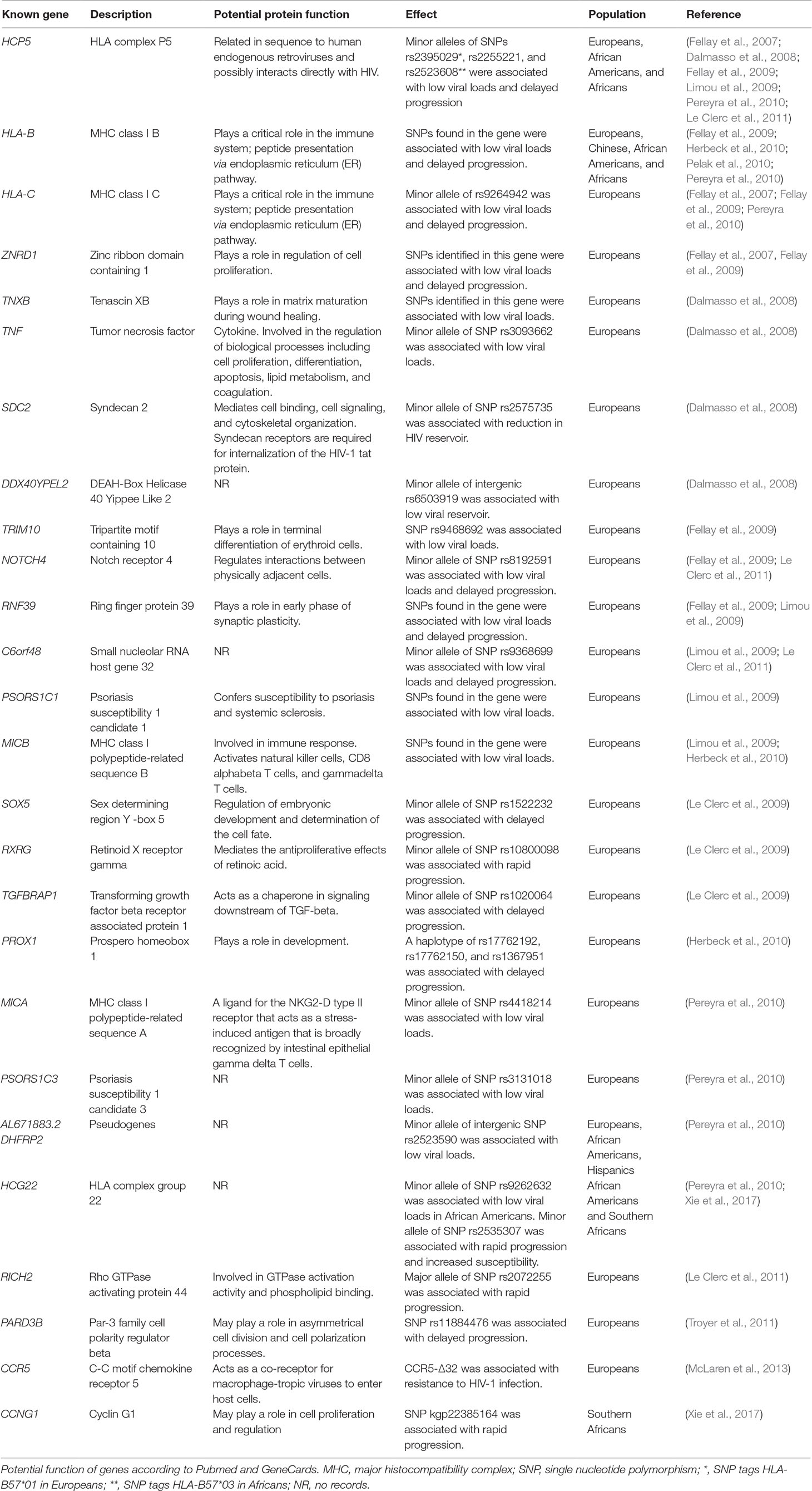
Table 2 GWAS significant genes associated with HIV-1 acquisition, viral load set point, and progression.
The first HIV GWAS report unraveled genome-wide contributions of host genetic markers in the control of HIV infection in Europeans and Caucasians (Fellay et al., 2007). Consistent with previous candidate gene studies, in the Fellay et al. (2007) study, the major genetic determinants of HIV set point were in HLA B and C loci. These findings were replicated in subsequent GWAS of HIV-1 in European/Caucasian populations, with additional loci being identified (Dalmasso et al., 2008; Fellay et al., 2009; Le Clerc et al., 2009; Limou et al., 2009; Herbeck et al., 2010; Pereyra et al., 2010; Le Clerc et al., 2011). In most studies, the HIV-1 phenotypes of interest were viral set point and progression to disease (Fellay et al., 2007; Dalmasso et al., 2008; Fellay et al., 2009; Le Clerc et al., 2009; Limou et al., 2009; Herbeck et al., 2010; Pereyra et al., 2010; Le Clerc et al., 2011; Troyer et al., 2011; van Manen et al., 2011; Bartha et al., 2013).
The effects of the following SNPs were consistently replicated in European samples: HCP5 rs2395029, HLA-C rs9264942, ZNRD1 rs9261174, NOTCH4 rs8192591, and C6orf48 rs9368699. The most replicated SNP was HCP5 rs2395029 (which tags HLA-B*5701). The minor allele G of HCP5 rs2395029 led to a reduction in viral load and delayed progression (Fellay et al., 2007; Dalmasso et al., 2008; Fellay et al., 2009; Limou et al., 2009; Pereyra et al., 2010; Le Clerc et al., 2011; Bartha et al., 2013). All these SNPs are located within the HLA region in chromosome 6. The HLA region is attributed by a long pattern of LD which makes it challenging to pinpoint the causal variant within this region. It has been observed that the minor allele of HCP5 rs2395029 is often observed with the controlling C allele HLA-Crs9264942, both leading to a protective effect (Fellay et al., 2007; Fellay et al., 2009). At gene level, besides HCP5, HLA-B, and HLA-C, the following were also overrepresented: TNXB, ZNRD1, RNF39, and MICB. The role of these genes was delayed progression [ZNRD1, RNF39 (Fellay et al., 2007; Fellay et al., 2009; Limou et al., 2009)] and reduction in viral load [TNXB and MICB (Dalmasso et al., 2008; Limou et al., 2009; Herbeck et al., 2010)]. Collectively, discovered SNPs within the European populations explained not more than 20% of the variability in viral load. More details on the identified SNPs can be found in the original publications and Table 2.
One of the major caveats of GWAS is the stringent threshold (p-value = 5.0 × 10-8) which accounts for multiple testing of about one million SNPs. As a result of this, SNPs with small effect sizes are not detected by GWAS and thousands of samples are required to effectively perform GWAS. In a study of progression in 404 Europeans, no SNP reached genome-wide significance (van Manen et al., 2011). The first GWAS of HIV-1 acquisition in European populations detected 11 SNPs which were genome-wide significant. Despite the large sample size, over 6,000 participants in each case/control category, these effects were disqualified due to frailty bias. Nonetheless, the study revealed a genome-wide association significance between imputed CCR5-Δ32 and HIV-1 acquisition [Supplementary Table 1 (McLaren et al., 2013)]. The outcomes of these studies also show that the defining and selection of GWAS phenotypes should be handled with care (van Manen et al., 2011; McLaren et al., 2013).
GWAS of HIV-1 have also been performed in other populations, African Americans, Hispanics, Chinese, and Africans (Pelak et al., 2010; Pereyra et al., 2010; Petrovski et al., 2011; Wei et al., 2015; Xie et al., 2017). Pereyra et al. identified an intergenic SNP rs2523590 in Hispanics, Europeans, and Americans. The minor allele of this SNP was associated with low viral loads (Pereyra et al., 2010). Two GWAS of African Americans identified the SNP rs2523608 which tags the HLA-B*5703 allele in people of African descent. Having the minor allele of the SNP leads to a reduction in viral load (Pelak et al., 2010; Pereyra et al., 2010). When considered alone, HLA-B*5703 had the strongest signal and accounted for about 10% of the variation in viral load set point. Participants who had the HLA-B*5703 allele (absent in Europeans) showed improved viral control of the same magnitude with that observed in Europeans carrying the HLA-B*5701 allele (Pelak et al., 2010). The HLA-B*5701 allele was present in African Americans because of admixture at 0.3% and had little contribution to HIV-1 control. The implications of different alleles of HLA-B*57 in two populations of different ancestries emphasizes the importance of investigating rare variants that might affect HIV infection distinctly in different populations.
The first and only HIV-1 GWAS in the Chinese population was reported in 2015 (Wei et al., 2015). Several novel SNPs that correlated with HIV-1 viral load set point were identified, albeit not genome-wide significant. The top signals such as rs2442719 were observed in the HLA region. The authors highlighted that they observed no significant association for the highest peaks identified in Caucasians and African Americans (HCP5 rs2395029 and HLA-B rs2523608 respectively), this indicating ethnic specificity of the variant associations [Supplementary Table 1 (Wei et al., 2015)].
The CCR5-Δ32 variant that is associated with resistance to HIV infection in people of European ancestry is rare in African populations (Fowke et al., 1996; Joubert et al., 2010; Lingappa et al., 2011; Petrovski et al., 2011). Since CCR5 variants were the only confirmed genetic markers that could influence HIV-1 acquisition in people of non-African ancestry, few studies were conducted to discover host genetics underlying HIV-1 acquisition in African populations (Kenya, Uganda and Tanzania, South Africa and Botswana (Lingappa et al., 2011), and Malawi (Petrovski et al., 2011). Lingappa et al. additionally investigated host genetics of viral control. These studies failed to detect signals of association between genetic variants and susceptibility to HIV-1 nor viral control. The authors postulated several arguments to explain the failure to detect signals: (1) susceptibility to HIV-1 might be due to non-genetic factors such as mode of transmission and viral sequence variability, (2) common or rare variants in an African populations might have not been represented in the chip used for genotyping, or (3) these weak signals may be due to the authors’ insufficient sample size (Lingappa et al., 2011; Petrovski et al., 2011).
The most recent HIV-1 GWAS was also conducted in an African (Botswana) population (Xie et al., 2017). This is the first GWAS to reveal significant variants associated with HIV-1 acquisition and progression in an African population. Moreover, two SNPs which had never been reported to associate with HIV infection nor progression were identified. These were SNPs rs2535307 and kgp22385164 located near HCG22 (immune regulatory gene within chromosome 6) and CCNG1 (encodes a cyclin that controls cell cycle; within chromosome 5) genes, respectively. The minor alleles of both SNPs led to lower CD4 T-cell counts and high viral loads. HCG22 was associated with progression and acquisition of HIV1-C, while CCNG1 was associated with progression only in the Botswana cohort [Table 2 (Xie et al., 2017)]. A validation test was done in data from three independent American cohorts, and no significant association was observed around the two genes (HCG22and CCNG1). SNPs that were found to associate with HIV infection and progression in previous studies did not appear in the top 100,000 p-values in this study (Xie et al., 2017).
Most of the SNPs that were identified in GWAS of HIV-1 are located within genes that play a role in immune response (Table 2 and Figure 4). We present in Figure 4, a pathway interaction network of host candidate genes from GWAS of HIV-1 (Table 2) using GeneMANIA software (Warde-Farley et al., 2010). Out of the 28 genes in Table 2, GeneMANIA was able to predict the functions of 23 genes. Other genes that GeneMANIA deemed biologically and/or transcriptionally similar to the HIV-1 candidate genes from GWAS are also added to the network. According to databases accessed by GeneMANIA, the interactive genes are co-expressed with identified genes from GWAS of HIV-1 (Table 2). This suggests that more robust GWAS methods may pinpoint novel HIV-associated variants within genes from this broad gene-based network.
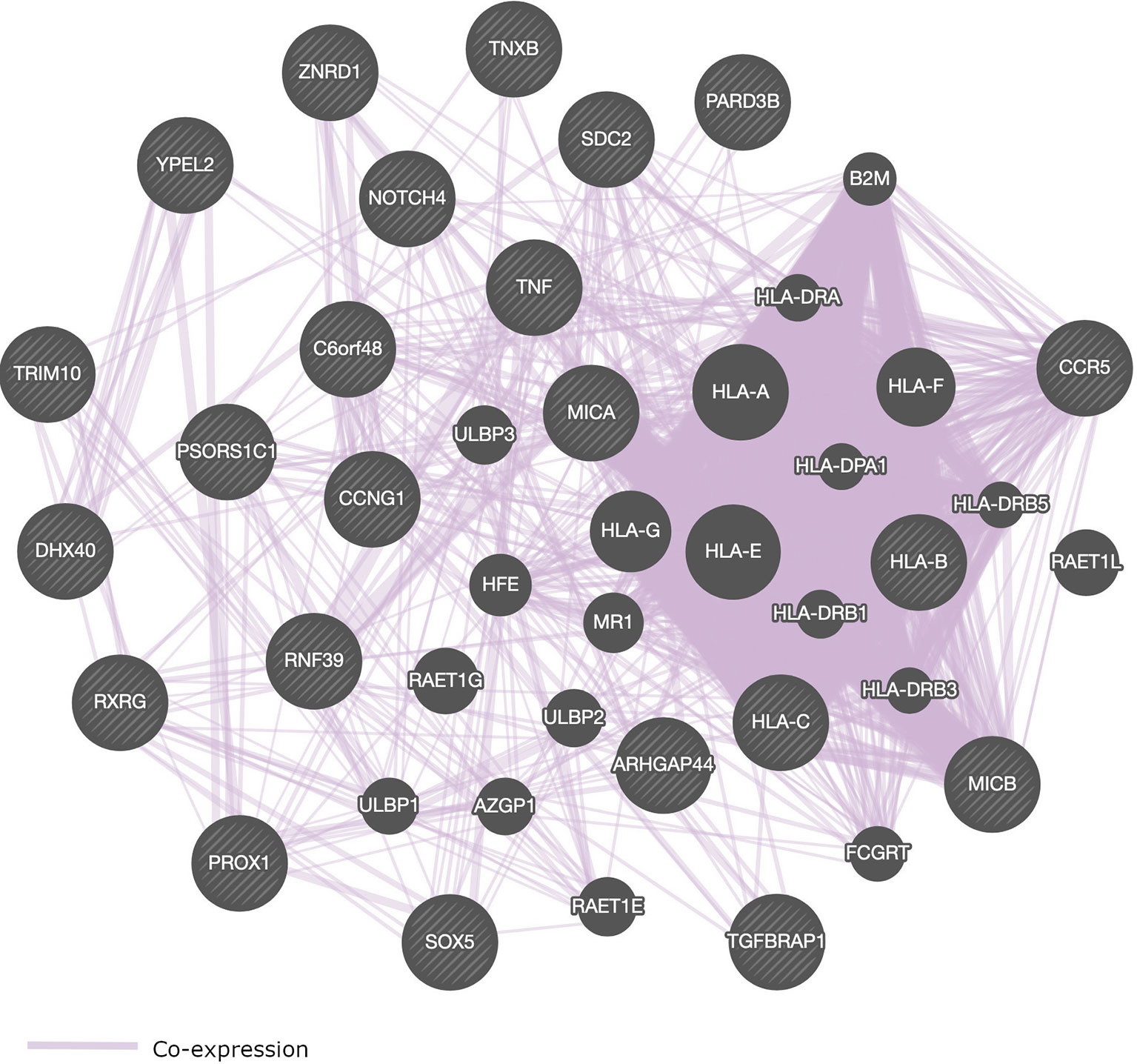
Figure 4 Pathway interaction network of genome-wide significant genes that control HIV-1 phenotypes. SNPs within or close to these genes are associated with the control of HIV-1. Striped circles indicate genes which have been identified from GWAS of HIV-1. Circles without stripes indicate genes putatively involved in the same pathway with genes implicated in HIV-1 phenotypes via GWAS. Purple lines represent gene co-expressions. The following genes could not be recognized by GeneMANIA software: HCP5, PSORS1C3, AL671883.2, DHFRP2, and HCG22.
GWAS of HIV-1 in Southern Africa
Only three GWAS of adult African populations have been published to date (Lingappa et al., 2011; Petrovski et al., 2011; Xie et al., 2017). These studies investigated common variants in Southern African populations of Malawi, Botswana, and South Africa. No variant reached genome-wide significance in two of these studies (Lingappa et al., 2011; Petrovski et al., 2011), while only two SNPs that had never been associated with HIV-1 acquisition and progression before were reported in the Botswana cohort (Xie et al., 2017). This lack of significant GWAS results is likely due to (1) lack of power from both sample size and statistical approaches used in both studies, (2) that the variants on microarrays could not tag the causal variants due to different LD patterns and different haplotype block structures between populations, or (3) that populations of Southern Africa use different mechanisms to control HIV-1C as a result of genetic diversity compared to previously studied populations.
Conclusions and Perspectives
Southern Africa has a unique genetic architecture with evident structure between the populations within the region. The haplotype data of Khoe-San and Southern Bantu ethnolinguistic groups are currently absent from existing haplotype reference panels such as 1000 Genomes Project. Studies of population genetics and ancient DNA in Southern Africa are likely to provide new opportunities to discover novel disease susceptibility loci and refine gene-disease association signals. Southern Africans harbor ancient genetic diversity, as well as historical admixture, which can lead to complexities in (a) the design of studies assessing the genetic determinants of diseases and human variation, and (b) approaches for reconstructing DNA segments (sequence alignment) and variant discovery.
This review provided a broad discussion of genetic diversity in Southern Africa and its implication in the genetic architecture of HIV-1 phenotypes. In addition, we posited the advances made in HIV-1 GWAS. Few GWAS of HIV have been conducted using adult populations from Southern Africa. This is not enough considering that the region has the highest social and economic burden of HIV. Despite the insufficient sample sizes, HIV-1 GWA studies have reaffirmed the role of HLA in controlling HIV infection. HLA-B, HLA-C, ZNRD1, RFN39, and HCP5 genes continue to be the most influential players in the outcome of HIV infection. However, a lot of implicated genes in GWAS reached significance by virtue of LD between the putative genes and the discovered variants. It is worthy to note that current findings from HIV-1 GWAS have not yet had a major impact on therapeutic optimization.
Critically, there has been a considerable amount of failure to replicate HIV-1 GWAS results across studies with some of the reasons being small sample sizes, different LD patterns between populations (causal SNPs probably not in the same haplotype block as tag-SNPs), and different criteria of phenotypic stratification. Nonetheless, many studies revealed novel variants which were not reported in previous studies. Two novel SNPs associated with HIV progression and acquisition were discovered in a Southern African population. This might imply that different networks of genes control HIV-1 in different regions. Given this, we hypothesize that functional categories of the genome may contribute disproportionately to the predisposition and resistance to HIV-1.
High genetic diversity, multi-wave genetic mixture, and low LD in the case of Southern African populations constitute a challenge in identifying genetic variants with modest risk or protective effect against HIV-1. Current GWAS of HIV-1 in Southern Africa were performed mostly in homogeneous populations; Malawi GWAS had a total of 1,532 Chichewa-speaking individuals, while the Botswana GWAS had a total of 556 individuals of undisclosed ethnicity. The Lingappa et al. study had 798 in total including 191participants of undisclosed ethnicity from Southern Africa. These sample sizes are not well powered to detect genome-wide significant SNPs of intermediate and small effect. Furthermore, considering the high genetic diversity and diverse patterns of admixture within Southern Africa, the populations used in the two GWAS of HIV-1 do not represent the diversity in the region. Thus, the findings of these studies cannot be effectively applied in other populations within the region. Hence, we need an inclusion of other populations in GWAS of HIV-1 in Southern Africa. More studies of admixture mapping should also be done to investigate the genetic role of different ancestries in HIV-1 phenotypes.
Moreover, factors such as host microbiome, viral genomics, epigenetics, social factors, and environmental factors can further contribute to HIV-1 acquisition, transmission, and progression. However, these components are not accounted for in current developed GWAS approaches that are mostly tailored for populations with long range of LD and haplotypes of European populations. These current GWAS approaches may limit the power of detecting possible associated variants and the ability to predict HIV-1 predisposition/resistance in the African context, in particular, Southern Africa. Therefore, failure to leverage these characteristics in modeling their joint contributions will be an obstacle to fully understand HIV-1 phenotype variability for an efficient and effective personalized therapy.
To date, GWAS of HIV-1 used Eurocentric microarrays for genotyping. Like other complex traits, HIV-1 phenotypes are polygenic; these traits are influenced by many rare variants of small effect sizes that microarray-based GWAS may fail to detect. However, other unbiased methods such as whole-genome or whole-exome sequences have the power to identify not only rare genetic variants but can also uncover more novel variants. Additionally, GWAS of Southern Africa may use SNP arrays that have a better coverage and representation of African genotypes and LD structures such as the recently developed Human Heredity and Health in Africa Consortium (H3Africa) SNP array. Other cost-effective approaches would be to develop or optimize targeted sequencing approaches such as the recently developed single primer enrichment technology. The advantage of target enrichment approaches is that they offer scalability and avoid the ascertainment bias that is common with microarray genotyping.
Exploring information from GWAS summary statistics provides a new paradigm to GWAS and might enable a complete characterization of genetic susceptibility/resistance to a disease. To account for the missing heritability of HIV-1, we suggest to further (a) examine whole genome or whole exome sequences of Southern African populations to uncover population-specific variants, (b) investigate functional categories of the genome and cell type-specific elements to estimate their enrichment and polygenic contribution to heritability of HIV-1, (c) perform a meta-analysis of previous HIV-1 GWAS results of African populations, (d) predict HIV-1 polygenic risk score, and (e) use robust methods for aggregation of GWAS signals to augment the identification of implicated genes and pathways or sub-networks. Importantly, it is worthy to note that HIV-1 polygenic risk scores based on European GWAS results may likely be poor predictors in Southern African populations because of differences in haplotype structure, patterns of LD (i.e., LD-tagging), and population-specific variation.
Changes in the host gene expression (or regulation) are a crucial stage in biological mechanisms underlying resistance and predisposition to HIV-1, and yet our current understanding of gene regulation is still limited. Without deep understanding of host gene regulation and the paucity in available tools for examining regulation, the transition from GWAS results to biological insights (biological mechanisms, genes involved, and the direction of causality) will remain a challenge.
Studies of different populations (including sub-Saharan Africa) have revealed that women with diverse vaginal microbiota have an increased risk of acquiring and transmitting HIV. However, these findings were not correlated with host genetics. Most studies investigating HIV and the gut microbiome were focused in non-African populations. It is known that the gut microbiome of populations differ geographically; therefore, it will be challenging to translate data from developed countries to populations in developing countries. Given the influences of (1) host genetics on the microbiome (gut, oral, genital, rectal, and lung), (2) environmental factors on microbiome profile, (3) HIV-1 infection on the microbiome, and (4) microbiome on host phenotypes, it is apparent that integrating the microbiome in host GWAS will reveal important insights and launch the first steps towards establishing causality in HIV-1 GWAS. The integrative analysis will be critical to comprehend the role and mechanisms of host genetics, the microbiome, and environment in the manifestation of HIV-1 infections.
Author Contributions
PT and EC conceived, structured, and wrote the content of the manuscript.
Funding
This work was supported through the sub-Saharan African Network for TB/HIV Research Excellence (SANTHE), a DELTAS Africa Initiative [grant # DEL-15-006]. The DELTAS Africa Initiative is an independent funding scheme of the African Academy of Sciences (AAS)’s Alliance for Accelerating Excellence in Science in Africa (AESA) and supported by the New Partnership for Africa’s Development Planning and Coordinating Agency (NEPAD Agency) with funding from the Wellcome Trust [grant # 107752/Z/15/Z] and the UK government. The views expressed in this publication are those of the authors and not necessarily those of AAS, NEPAD Agency, Wellcome Trust, or the UK government. The authors would also like to thank the National Research Foundation of South Africa for funding (NRF) [grant # RA171111285157/119056].
Conflict of Interest
The authors declare that the research was conducted in the absence of any commercial or financial relationships that could be construed as a potential conflict of interest.
Acknowledgement
We would like to thank Simani Gaseitsiwe, PhD; Vlad Novitsky, PhD; Melvin Leteane, PhD for their support and guidance; Denis Awany for the useful discussions and The Centre for High-Performance Computing (CHPC, www.chpc.ac.za) for computing resources.
Supplementary Material
The Supplementary Material for this article can be found online at: https://www.frontiersin.org/articles/10.3389/fgene.2019.00905/full#supplementary-material
References
Abdool Karim, S. S., Churchyard, G. J., Karim, Q. A., Lawn, S. D. (2009). HIV infection and tuberculosis in South Africa: an urgent need to escalate the public health response. Lancet (London, England) 374, 921–933. doi: 10.1016/S0140-6736(09)60916-8
Adebamowo, S. N., Tekola-Ayele, F., Adeyemo, A. A., Rotimi, C. N. (2017). Genomics of cardiometabolic disorders in sub-Saharan Africa. Public Health Genomics 20, 9–26. doi: 10.1159/000468535
Adhikari, M. (2010). A total extinction confidently hoped for: the destruction of Cape San society under Dutch colonial rule, 1700–1795. J. Genocide Res. 12, 19–44. doi: 10.1080/14623528.2010.508274
An, P., Bleiber, G., Duggal, P., Nelson, G., May, M., Mangeat, B., et al. (2004). APOBEC3G genetic variants and their influence on the progression to AIDS. J. Virol. 78, 11070–11076. doi: 10.1128/JVI.78.20.11070-11076.2004
An, P., Nelson, G. W., Wang, L., Donfield, S., Goedert, J. J., Phair, J., et al. (2002). Modulating influence on HIV/AIDS by interacting RANTES gene variants. Proc. Natl. Acad. Sci. U. S. A. 99, 10002–10007. doi: 10.1073/pnas.142313799
Arts, E. J., Hazuda, D. J. (2012). HIV-1 antiretroviral drug therapy. Cold Spring Harb. Perspect. Med. 2, a007161. doi: 10.1101/cshperspect.a007161
Awany, D., Allali, I., Dalvie, S., Hemmings, S., Mwaikono, K. S., Thomford, N. E., et al. (2018). Host and microbiome genome-wide association studies: current state and challenges. Front. Genet. 9, 637. doi: 10.3389/fgene.2018.00637
Bajic, V., Barbieri, C., Hubner, A., Guldemann, T., Naumann, C., Gerlach, L., et al. (2018). Genetic structure and sex-biased gene flow in the history of Southern African populations. Am. J. Phys. Anthropol. 167, 656–671. doi: 10.1002/ajpa.23694
Barbieri, C., Butthof, A., Bostoen, K., Pakendorf, B. (2013a). Genetic perspectives on the origin of clicks in Bantu languages from southwestern Zambia. Eur. J. Hum. Genet. 21, 430–436. doi: 10.1038/ejhg.2012.192
Barbieri, C., Vicente, M., Oliveira, S., Bostoen, K., Rocha, J., Stoneking, M., et al. (2014). Migration and interaction in a contact zone: mtDNA variation among Bantu-speakers in Southern Africa. PLoS One 9, e99117. doi: 10.1371/journal.pone.0099117
Barbieri, C., Vicente, M., Rocha, J., Mpoloka, S. W., Stoneking, M., Pakendorf, B. (2013b). Ancient substructure in early mtDNA lineages of Southern Africa. Am. J. Hum. Genet. 92, 285–292. doi: 10.1016/j.ajhg.2012.12.010
Barnard, A. (1992). Hunters and herders of Southern Africa: a comparative ethnography of the Khoisan peoples. Cambridge, UK: Cambridge University Press. doi: 10.1017/CBO9781139166508
Bartha, I., Carlson, J. M., Brumme, C. J., McLaren, P. J., Brumme, Z. L., John, M., et al. (2013). A genome-to-genome analysis of associations between human genetic variation, HIV-1 sequence diversity, and viral control. Elife 2, e01123. doi: 10.7554/eLife.01123
Behar, D. M., Villems, R., Soodyall, H., Blue-Smith, J., Pereira, L., Metspalu, E., et al. (2008). The dawn of human matrilineal diversity. Am. J. Hum. Genet. 82, 1130–1140. doi: 10.1016/j.ajhg.2008.04.002
Bisson, G. P., Zetola, N., Collman, R. G. (2015). Persistent high mortality in advanced HIV/TB despite appropriate antiretroviral and antitubercular therapy: an emerging challenge. Curr. HIV/AIDS Rep. 12, 107–116. doi: 10.1007/s11904-015-0256-x
Botigue, L. R., Henn, B. M., Gravel, S., Maples, B. K., Gignoux, C. R., Corona, E., et al. (2013). Gene flow from North Africa contributes to differential human genetic diversity in southern Europe. Proc. Natl. Acad. Sci. 110, 11791–11796. doi: 10.1073/pnas.1306223110
Breton, G., Schlebusch, C. M., Lombard, M., Sjodin, P., Soodyall, H., Jakobsson, M. (2014). Lactase persistence alleles reveal partial East African ancestry of Southern African Khoe pastoralists. Curr. Biol. 24, 852–858. doi: 10.1016/j.cub.2014.02.041
Bruchfeld, J., Correia-Neves, M., Källenius, G. (2015). Tuberculosis and HIV coinfection. Cold Spring Harb. Perspect. Med. 5, a017871. doi: 10.1101/cshperspect.a017871
Busby, G. B., Band, G., Si Le, Q., Jallow, M., Bougama, E., Mangano, V. D., et al. (2016). Admixture into and within sub-Saharan Africa. Elife 5, e15266. doi: 10.7554/eLife.15266
Campa, A., Baum, M. K., Bussmann, H., Martinez, S. S., Farahani, M., van Widenfelt, E., et al. (2017). The effect of micronutrient supplementation on active TB incidence early in HIV infection in Botswana. Nutr. Diet. Suppl. 2017, 37–45. doi: 10.2147/NDS.S123545
Campbell, M. C., Tishkoff, S. A. (2008). African genetic diversity: implications for human demographic history, modern human origins, and complex disease mapping. Annu. Rev. Genomics Hum. Genet. 9, 403–433. doi: 10.1146/annurev.genom.9.081307.164258
Campbell, M. C., Tishkoff, S. A. (2010). The evolution of human genetic and phenotypic variation in Africa. Curr. Biol. 20, R166–R173. doi: 10.1016/j.cub.2009.11.050
Carr, D. F., Bourgeois, S., Chaponda, M., Takeshita, L. Y., Morris, A. P., Cornejo Castro, E. M., et al. (2017). Genome-wide association study of nevirapine hypersensitivity in a sub-Saharan African HIV-infected population. J. Antimicrob. Chemother. 72, 1152–1162. doi: 10.1093/jac/dkw545
Carrington, M., Nelson, G. W., Martin, M. P., Kissner, T., Vlahov, D., Goedert, J. J., et al. (1999). HLA and HIV-1: heterozygote advantage and B*35-Cw*04 disadvantage. Science 283, 1748–1752. doi: 10.1126/science.283.5408.1748
Carrington, M., O’Brien, S. J. (2003). The influence of HLA genotype on AIDS. Annu. Rev. Med. 54, 535–551. doi: 10.1146/annurev.med.54.101601.152346
Cavalli-Sforza, L. L., Menozzi, P., Piazza, A. (1993). Demic expansions and human evolution. Science (80-.) 259, 639–646. doi: 10.1126/science.8430313
Chan, E. K. F., Hardie, R.-A., Petersen, D. C., Beeson, K., Bornman, R. M. S., Smith, A. B., et al. (2015). Revised timeline and distribution of the earliest diverged human maternal lineages in Southern Africa. PLoS One 10, e0121223. doi: 10.1371/journal.pone.0121223
Chi, C., Shao, X., Rhead, B., Gonzales, E., Smith, J. B., Xiang, A. H., et al. (2019). Admixture mapping reveals evidence of differential multiple sclerosis risk by genetic ancestry. PLoS Genet. 15, 1–27. doi: 10.1371/journal.pgen.1007808
Chimusa, E. R., Daya, M., Moller, M., Ramesar, R., Henn, B. M., van Helden, P. D., et al. (2013a). Determining ancestry proportions in complex admixture scenarios in South Africa using a novel proxy ancestry selection method. PLoS One 8, e73971. doi: 10.1371/journal.pone.0073971
Chimusa, E. R., Defo, J., Thami, P. K., Awany, D., Mulisa, D. D., Allali, I., et al. (2018). Dating admixture events is unsolved problem in multi-way admixed populations. Brief. Bioinform. doi: 10.1093/bib/bby112
Chimusa, E. R., Meintjies, A., Tchanga, M., Mulder, N., Seoighe, C., Soodyall, H., et al. (2015). A genomic portrait of haplotype diversity and signatures of selection in indigenous Southern African populations. PLoS Genet. 11, e1005052. doi: 10.1371/journal.pgen.1005052
Chimusa, E. R., Zaitlen, N., Daya, M., Moller, M., van Helden, P. D., Mulder, N. J., et al. (2013b). Genome-wide association study of ancestry-specific TB risk in the South African Coloured population. Hum. Mol. Genet. 23, 796–809. doi: 10.1093/hmg/ddt462
Choudhury, A., Aron, S., Sengupta, D., Hazelhurst, S., Ramsay, M. (2018). African genetic diversity provides novel insights into evolutionary history and local adaptations. Hum. Mol. Genet. 27, R209–R218. doi: 10.1093/hmg/ddy161
Choudhury, A., Ramsay, M., Hazelhurst, S., Aron, S., Bardien, S., Botha, G., et al. (2017). Whole-genome sequencing for an enhanced understanding of genetic variation among South Africans. Nat. Commun. 8, 1–12. doi: 10.1038/s41467-017-00663-9
Corbett, E. L., Watt, C. J., Walker, N., Maher, D., Williams, B. G., Raviglione, M. C., et al. (2003). The growing burden of tuberculosis: global trends and interactions with the HIV epidemic. Arch. Intern. Med. 163, 1009–1021. doi: 10.1001/archinte.163.9.1009
Dalmasso, C., Carpentier, W., Meyer, L., Rouzioux, C., Goujard, C., Chaix, M.-L., et al. (2008). Distinct genetic loci control plasma HIV-RNA and cellular HIV-DNA levels in HIV-1 infection: the ANRS Genome Wide Association 01 study. PLoS One 3, e3907. doi: 10.1371/journal.pone.0003907
Daya, M., van der Merwe, L., Gignoux, C. R., van Helden, P. D., Moller, M., Hoal, E. G. (2014). Using multi-way admixture mapping to elucidate TB susceptibility in the South African Coloured population. BMC Genomics 15, 1021. doi: 10.1186/1471-2164-15-1021
de Filippo, C., Heyn, P., Barham, L., Stoneking, M., Pakendorf, B. (2010). Genetic perspectives on forager-farmer interaction in the Luangwa valley of Zambia. Am. J. Phys. Anthropol. 141, 382–394. doi: 10.1002/ajpa.21155
de Wit, E., Delport, W., Rugamika, C. E., Meintjes, A., Moller, M., van Helden, P. D., et al. (2010). Genome-wide analysis of the structure of the South African Coloured Population in the Western Cape. Hum. Genet. 128, 145–153. doi: 10.1007/s00439-010-0836-1
Dean, M., Carrington, M., Winkler, C., Huttley, G. A., Smith, M. W., Allikmets, R., et al. (1996). Genetic restriction of HIV-1 infection and progression to AIDS by a deletion allele of the CKR5 structural gene. Hemophilia Growth and Development Study, Multicenter AIDS Cohort Study, Multicenter Hemophilia Cohort Study, San Francisco City Cohort, ALIVE. Science 273, 1856–1862. doi: 10.1126/science.273.5283.1856
Diamond, J., Bellwood, P. (2003). Farmers and their languages: the first expansions. Science (80-.) 300, 597–603. doi: 10.1126/science.1078208
Dokubo, E. K., Baddeley, A., Pathmanathan, I., Coggin, W., Firth, J., Getahun, H., et al. (2014). Provision of antiretroviral therapy for HIV-positive TB patients—19 countries, sub-Saharan Africa, 2009–2013. MMWR. Morb. Mortal. Wkly. Rep. 63, 1104–1107.
Duggal, P., An, P., Beaty, T. H., Strathdee, S. A., Farzadegan, H., Markham, R. B., et al. (2003). Genetic influence of CXCR6 chemokine receptor alleles on PCP-mediated AIDS progression among African Americans. Genes Immun. 4, 245–250. doi: 10.1038/sj.gene.6363950
Eberhard, D. M., Simons, G. F., Fennig, C. D. Ethnologue: Languages of the World. Ethnologue. Available at: https://www.ethnologue.com/region/saf [Accessed February 10, 2019].
Eberle, J., Gurtler, L. (2012). HIV types, groups, subtypes and recombinant forms: errors in replication, selection pressure and quasispecies. Intervirology 55, 79–83. doi: 10.1159/000331993
Faria, N. R., Rambaut, A., Suchard, M. A., Baele, G., Bedford, T., Ward, M. J., et al. (2014). HIV epidemiology. The early spread and epidemic ignition of HIV-1 in human populations. Science 346, 56–61. doi: 10.1126/science.1256739
Fellay, J., Ge, D., Shianna, K. V., Colombo, S., Ledergerber, B., Cirulli, E. T., et al. (2009). Common genetic variation and the control of HIV-1 in humans. PLoS Genet. 5, e1000791. doi: 10.1371/journal.pgen.1000791
Fellay, J., Shianna, K. V., Ge, D., Colombo, S., Ledergerber, B., Weale, M., et al. (2007). A whole-genome association study of major determinants for host control of HIV-1. Science (80-.) 317, 944–947. doi: 10.1126/science.1143767
Fowke, K. R., Nagelkerke, N. J., Kimani, J., Simonsen, J. N., Anzala, A. O., Bwayo, J. J., et al. (1996). Resistance to HIV-1 infection among persistently seronegative prostitutes in Nairobi, Kenya. Lancet (London, England) 348, 1347–1351. doi: 10.1016/S0140-6736(95)12269-2
Fraser, C., Hollingsworth, T. D., Chapman, R., de Wolf, F., Hanage, W. P. (2007). Variation in HIV-1 set-point viral load: epidemiological analysis and an evolutionary hypothesis. Proc. Natl. Acad. Sci. U. S. A. 104, 17441–17446. doi: 10.1073/pnas.0708559104
Gallo, R. C., Salahuddin, S. Z., Popovic, M., Shearer, G. M., Kaplan, M., Haynes, B. F., et al. (1984). Frequent detection and isolation of cytopathic retroviruses (HTLV-III) from patients with AIDS and at risk for AIDS. Science 224, 500–503. doi: 10.1126/science.6200936
Gao, X., Nelson, G. W., Karacki, P., Martin, M. P., Phair, J., Kaslow, R., et al. (2001). Effect of a single amino acid change in MHC class I molecules on the rate of progression to AIDS. N. Engl. J. Med. 344, 1668–1675. doi: 10.1056/NEJM200105313442203
GeneCards. (2019). GeneCards—human gene database. https://www.genecards.org/ [Accessed 26, July 2019].
Gonder, M. K., Mortensen, H. M., Reed, F. A., de Sousa, A., Tishkoff, S. A. (2007). Whole-mtDNA genome sequence analysis of ancient African lineages. Mol. Biol. Evol. 24, 757–768. doi: 10.1093/molbev/msl209
Greene, W. C. (2007). A history of AIDS: looking back to see ahead. Eur. J. Immunol. 37 , S94–102. doi: 10.1002/eji.200737441
Gurdasani, D., Carstensen, T., Tekola-Ayele, F., Pagani, L., Tachmazidou, I., Hatzikotoulas, K., et al. (2014). The African Genome Variation Project shapes medical genetics in Africa. Nature 517, 327–332. doi: 10.1038/nature13997
Hall, M. (1993). The archaeology of colonial settlement in Southern Africa. Annu. Rev. Anthropol. 22, 177–200. doi: 10.1146/annurev.an.22.100193.001141
Halper-Stromberg, A., Nussenzweig, M. C. (2016). Towards HIV-1 remission: potential roles for broadly neutralizing antibodies. J. Clin. Invest. 126, 415–423. doi: 10.1172/JCI80561
Heine, B., Nurse, D. (2000). African languages: An introduction. 1st ed. Cambridge: Cambridge University Press.
Hellenthal, G., Busby, G. B. J., Band, G., Wilson, J. F., Capelli, C., Falush, D., et al. (2014). A genetic atlas of human admixture history. Science (80-.) 343, 747–751. doi: 10.1126/science.1243518
Hemelaar, J., Gouws, E., Ghys, P. D., Osmanov, S. (2011). Global trends in molecular epidemiology of HIV-1 during 2000–2007. AIDS 25, 679–689. doi: 10.1097/QAD.0b013e328342ff93
Henn, B. M., Gignoux, C. R., Jobin, M., Granka, J. M., Macpherson, J. M., Kidd, J. M., et al. (2011). Hunter-gatherer genomic diversity suggests a Southern African origin for modern humans. Proc. Natl. Acad. Sci. U. S. A. 108, 5154–5162. doi: 10.1073/pnas.1017511108
Henrich, T. J., Kuritzkes, D. R. (2013). HIV-1 entry inhibitors: recent development and clinical use. Curr. Opin. Virol. 3, 51–57. doi: 10.1016/j.coviro.2012.12.002
Herbeck, J. T., Gottlieb, G. S., Winkler, C. A., Nelson, G. W., An, P., Maust, B. S., et al. (2010). Multistage genomewide association study identifies a locus at 1q41 associated with rate of HIV-1 disease progression to clinical AIDS. J. Infect. Dis. 201, 618–626. doi: 10.1086/649842
Hirschhorn, J. N., Daly, M. J. (2005). Genome-wide association studies for common diseases and complex traits. Nat. Rev. Genet. 6, 95–108. doi: 10.1038/nrg1521
Hsu, D. C., O’Connell, R. J. (2017). Progress in HIV vaccine development. Hum. Vaccin. Immunother. 13, 1018–1030. doi: 10.1080/21645515.2016.1276138
Huffman, T. N. (1970). The early Iron Age and the spread of the Bantu. S. Afr. Archaeol. Bull. 25, 3–21. doi: 10.2307/3888762
Hutcheson, H. B., Lautenberger, J. A., Nelson, G. W., Pontius, J. U., Kessing, B. D., Winkler, C. A., et al. (2008). Detecting AIDS restriction genes: from candidate genes to genome-wide association discovery. Vaccine 26, 2951–2965. doi: 10.1016/j.vaccine.2007.12.054
Hutter, G., Nowak, D., Mossner, M., Ganepola, S., Mussig, A., Allers, K., et al. (2009). Long-term control of HIV by CCR5 Delta32/Delta32 stem-cell transplantation. N. Engl. J. Med. 360, 692–698. doi: 10.1056/NEJMoa0802905
Jakobsson, M., Edge, M. D., Rosenberg, N. A. (2013). The relationship between F(ST) and the frequency of the most frequent allele. Genetics 193, 515–528. doi: 10.1534/genetics.112.144758
Jakobsson, M., Scholz, S. W., Scheet, P., Gibbs, J. R., VanLiere, J. M., Fung, H.-C., et al. (2008). Genotype, haplotype and copy-number variation in worldwide human populations. Nature 451, 998–1003. doi: 10.1038/nature06742
Javanbakht, H., An, P., Gold, B., Petersen, D. C., O’Huigin, C., Nelson, G. W., et al. (2006). Effects of human TRIM5alpha polymorphisms on antiretroviral function and susceptibility to human immunodeficiency virus infection. Virology 354, 15–27. doi: 10.1016/j.virol.2006.06.031
John, M.-A., Menezes, C. N., Chita, G., Sanne, I., Grobusch, M. P. (2007). High tuberculosis and HIV coinfection rate, Johannesburg. Emerg. Infect. Dis. 13, 795–796. doi: 10.3201/eid1305.060908
Joubert, B. R., Lange, E. M., Franceschini, N., Mwapasa, V., North, K. E., Meshnick, S. R. (2010). A whole genome association study of mother-to-child transmission of HIV in Malawi. Genome Med. 2, 17. doi: 10.1186/gm138
Kagaayi, J., Serwadda, D. (2016). The history of the HIV/AIDS epidemic in Africa. Curr. HIV/AIDS Rep. 13, 187–193. doi: 10.1007/s11904-016-0318-8
Kang, H. M., Sul, J. H., Service, S. K., Zaitlen, N. A., Kong, S.-Y., Freimer, N. B., et al. (2010). Variance component model to account for sample structure in genome-wide association studies. Nat. Genet. 42, 348–354. doi: 10.1038/ng.548
Lawn, S. D., Bekker, L.-G., Miller, R. F. (2005). Immune reconstitution disease associated with mycobacterial infections in HIV-infected individuals receiving antiretrovirals. Lancet. Infect. Dis. 5, 361–373. doi: 10.1016/S1473-3099(05)70140-7
Le Clerc, S., Coulonges, C., Delaneau, O., Van Manen, D., Herbeck, J. T., Limou, S., et al. (2011). Screening low-frequency SNPS from genome-wide association study reveals a new risk allele for progression to AIDS. J. Acquir. Immune Defic. Syndr. 56, 279–284. doi: 10.1097/QAI.0b013e318204982b
Le Clerc, S., Limou, S., Coulonges, C., Carpentier, W., Dina, C., Taing, L., et al. (2009). Genomewide association study of a rapid progression cohort identifies new susceptibility alleles for AIDS (ANRS Genomewide Association Study 03). J. Infect. Dis. 200, 1194–1201. doi: 10.1086/605892
Li, J. Z., Absher, D. M., Tang, H., Southwick, A. M., Casto, A. M., Ramachandran, S., et al. (2008). Worldwide human relationships inferred from genome-wide patterns of variation. Science (80-.) 319, 1100–1104. doi: 10.1126/science.1153717
Li, S., Schlebusch, C., Jakobsson, M. (2014). Genetic variation reveals large-scale population expansion and migration during the expansion of Bantu-speaking peoples. Proceedings. Biol. Sci. 281. doi: 10.1098/rspb.2014.1448
Limou, S., Le Clerc, S., Coulonges, C., Carpentier, W., Dina, C., Delaneau, O., et al. (2009). Genomewide association study of an AIDS-nonprogression cohort emphasizes the role played by HLA genes (ANRS Genomewide Association Study 02). J. Infect. Dis. 199, 419–426. doi: 10.1086/596067
Lingappa, J. R., Petrovski, S., Kahle, E., Fellay, J., Shianna, K., McElrath, M. J., et al. (2011). Genomewide association study for determinants of HIV-1 acquisition and viral set point in HIV-1 serodiscordant couples with quantified virus exposure. PLoS One 6, e28632. doi: 10.1371/journal.pone.0028632
Macholdt, E., Lede, V., Barbieri, C., Mpoloka, S. W., Chen, H., Slatkin, M., et al. (2014). Tracing pastoralist migrations to Southern Africa with lactase persistence alleles. Curr. Biol. 24, 875–879. doi: 10.1016/j.cub.2014.03.027
Marks, S. E. (2014). Southern Africa. https://www.britannica.com/place/Southern-Africa [Accessed 20 January 2019].
Marks, S. J., Montinaro, F., Levy, H., Brisighelli, F., Ferri, G., Bertoncini, S., et al. (2015). Static and moving frontiers: the genetic landscape of Southern African Bantu-speaking populations. Mol. Biol. Evol. 32, 29–43. doi: 10.1093/molbev/msu263
Martin, M. P., Gao, X., Lee, J.-H., Nelson, G. W., Detels, R., Goedert, J. J., et al. (2002). Epistatic interaction between KIR3DS1 and HLA-B delays the progression to AIDS. Nat. Genet. 31, 429–434. doi: 10.1038/ng934
May, A., Hazelhurst, S., Li, Y., Norris, S. A., Govind, N., Tikly, M., et al. (2013). Genetic diversity in black South Africans from Soweto. 14, 644. doi: 10.1186/1471-2164-14-644
McLaren, P. J., Carrington, M. (2015). The impact of host genetic variation on infection with HIV-1. Nat. Immunol. 16, 577–583. doi: 10.1038/ni.3147
McLaren, P. J., Coulonges, C., Bartha, I., Lenz, T. L., Deutsch, A. J., Bashirova, A., et al. (2015). Polymorphisms of large effect explain the majority of the host genetic contribution to variation of HIV-1 virus load. Proc. Natl. Acad. Sci. 112, 14658–14663. doi: 10.1073/pnas.1514867112
McLaren, P. J., Coulonges, C., Ripke, S., van den Berg, L., Buchbinder, S., Carrington, M., et al. (2013). Association study of common genetic variants and HIV-1 acquisition in 6,300 infected cases and 7,200 controls. PLoS Pathog. 9, e1003515. doi: 10.1371/journal.ppat.1003515
Meintjes, G., Lawn, S. D., Scano, F., Maartens, G., French, M. A., Worodria, W., et al. (2008). Tuberculosis-associated immune reconstitution inflammatory syndrome: case definitions for use in resource-limited settings. Lancet. Infect. Dis. 8, 516–523. doi: 10.1016/S1473-3099(08)70184-1
Mellors, J. W., Rinaldo, C. R. J., Gupta, P., White, R. M., Todd, J. A., Kingsley, L. A. (1996). Prognosis in HIV-1 infection predicted by the quantity of virus in plasma. Science 272, 1167–1170. doi: 10.1126/science.272.5265.1167
Montinaro, F., Busby, G. B. J., Gonzalez-Santos, M., Oosthuitzen, O., Oosthuitzen, E., Anagnostou, P., et al. (2017). Complex ancient genetic structure and cultural transitions in Southern African populations. Genetics 205, 303–316. doi:10.1534/genetics.116.189209.
Morris, A. G., Heinze, A., Chan, E. K. F., Smith, A. B., Hayes, V. M. (2014). First ancient mitochondrial human genome from a prepastoralist Southern African. Genome Biol. Evol. 6, 2647–2653. doi: 10.1093/gbe/evu202
Naidoo, T., Schlebusch, C. M., Makkan, H., Patel, P., Mahabeer, R., Erasmus, J. C., et al. (2010). Development of a single base extension method to resolve Y chromosome haplogroups in sub-Saharan African populations. Investig. Genet. 1, 6. doi: 10.1186/2041-2223-1-6
Neumann, K., Bostoen, K., Höhn, A., Kahlheber, S., Ngomanda, A., Tchiengué, B. (2012). First farmers in the central African rainforest: a view from southern Cameroon. Quat. Int. 249, 53–62. doi: 10.1016/j.quaint.2011.03.024
Nurse, G. T., Weiner, J. S., Jenkins, T., et al., (1985). The peoples of Southern Africa and their affinities. New York: Oxford University Press.
O’Brien, S. J., Nelson, G. W. (2004). Human genes that limit AIDS. Nat. Genet. 36, 565–574. doi: 10.1038/ng1369
Oliveira, S., Hubner, A., Fehn, A.-M., Aco, T., Lages, F., Pakendorf, B., et al. (2019). The role of matrilineality in shaping patterns of Y chromosome and mtDNA sequence variation in southwestern Angola. Eur. J. Hum. Genet. 27, 475–483. doi: 10.1038/s41431-018-0304-2
Pawlowski, A., Jansson, M., Sköld, M., Rottenberg, M. E., Källenius, G. (2012). Tuberculosis and HIV co-infection. PLoS Pathog. 8, e1002464. doi: 10.1371/journal.ppat.1002464
Pelak, K., Goldstein, D. B., Walley, N. M., Fellay, J., Ge, D., Shianna, K. V., et al. (2010). Host determinants of HIV-1 control in African Americans. J. Infect. Dis. 201, 1141–1149. doi: 10.1086/651382
Pereyra, F., Jia, X., McLaren, P. J., Telenti, A., de Bakker, P. I. W., Walker, B. D., et al. (2010). The major genetic determinants of HIV-1 control affect HLA class I peptide presentation. Science 330, 1551–1557. doi: 10.1126/science.1195271
Petrovski, S., Fellay, J., Shianna, K. V., Carpenetti, N., Kumwenda, J., Kamanga, G., et al. (2011). Common human genetic variants and HIV-1 susceptibility: a genome-wide survey in a homogeneous African population. AIDS 25, 513–518. doi: 10.1097/QAD.0b013e328343817b
Phillipson, D. W. (1977). The later prehistory of Eastern and Southern Africa. London: Heinemann. Available at: https://books.google.co.za/books?id=pRC1AAAAIAAJ.
Pickrell, J. K., Patterson, N., Barbieri, C., Berthold, F., Gerlach, L., Güldemann, T., et al. (2012). The genetic prehistory of Southern Africa. Nat. Commun. 3, 1143. doi: 10.1038/ncomms2140
Pickrell, J. K., Patterson, N., Loh, P.-R., Lipson, M., Berger, B., Stoneking, M., et al. (2014). Ancient west Eurasian ancestry in Southern and Eastern Africa. Proc. Natl. Acad. Sci. U. S. A. 111, 2632–2637. doi: 10.1073/pnas.1313787111
Price, A. L., Patterson, N. J., Plenge, R. M., Weinblatt, M. E., Shadick, N. A., Reich, D. (2006). Principal components analysis corrects for stratification in genome-wide association studies. Nat. Genet. 38, 904–909. doi: 10.1038/ng1847
Purcell, S., Neale, B., Todd-Brown, K., Thomas, L., Ferreira, M. A. R., Bender, D., et al. (2007). PLINK: a tool set for whole-genome association and population-based linkage analyses. Am. J. Hum. Genet. 81, 559–575. doi: 10.1086/519795
Ramsay, J., Morton, B., Mgadla, T., (1996). Building a nation: a history of Botswana from 1800 to 1910. Gaborone: Longman Botswana.
Reed, F. A., Tishkoff, S. A. (2006). African human diversity, origins and migrations. Curr. Opin. Genet. Dev. 16, 597–605. doi: 10.1016/j.gde.2006.10.008
Retshabile, G., Mlotshwa, B. C., Williams, L., Mwesigwa, S., Mboowa, G., Huang, Z., et al. (2018). Whole-exome sequencing reveals uncaptured variation and distinct ancestry in the Southern African population of Botswana. Am. J. Hum. Genet. 102, 731–743. doi: 10.1016/j.ajhg.2018.03.010
Roser, M., Ritchie, H. (2019). HIV / AIDS. Our World In Data. Available at: https://ourworldindata.org/hiv-aids [Accessed April 16, 2019].
Russell, T., Silva, F., Steele, J. (2014). Modelling the spread of farming in the Bantu-speaking regions of Africa: an archaeology-based phylogeography. PLoS One 9, e87854. doi: 10.1371/journal.pone.0087854
Sadr, K. (2015). Livestock first reached Southern Africa in two separate events. PLoS One 10, e0134215. doi: 10.1371/journal.pone.0134215
Sanderson, J., Sudoyo, H., Karafet, T. M., Hammer, M. F., Cox, M. P. (2015). Reconstructing past admixture processes from local genomic ancestry using wavelet transformation. Genetics 200, 469–481.
Schlebusch, C. (2010). Issues raised by use of ethnic-group names in genome study. Nature 464, 487. doi: 10.1038/464487a
Schlebusch, C. M., Lombard, M., Soodyall, H. (2013). MtDNA control region variation affirms diversity and deep sub-structure in populations from Southern Africa. BMC Evol. Biol. 13, 56. doi: 10.1186/1471-2148-13-56
Schlebusch, C. M., Prins, F., Lombard, M., Jakobsson, M., Soodyall, H. (2016). The disappearing San of southeastern Africa and their genetic affinities. Hum. Genet. 135, 1365–1373. doi: 10.1007/s00439-016-1729-8
Schlebusch, C. M., Skoglund, P., Sjodin, P., Gattepaille, L. M., Hernandez, D., Jay, F., et al. (2012). Genomic variation in seven Khoe-San groups reveals adaptation and complex African history. Science 338, 374–379. doi: 10.1126/science.1227721
Sharp, P. M., Hahn, B. H. (2011). Origins of HIV and the AIDS pandemic. Cold Spring Harb. Perspect. Med. 1, a006841. doi: 10.1101/cshperspect.a006841
Sherry, S. T., Ward, M. H., Kholodov, M., Baker, J., Phan, L., Smigielski, E. M., et al. (2001). dbSNP: the NCBI database of genetic variation. Nucleic Acids Res. 29, 308–311. doi: 10.1093/nar/29.1.308
Shin, H. D., Winkler, C., Stephens, J. C., Bream, J., Young, H., Goedert, J. J., et al. (2000). Genetic restriction of HIV-1 pathogenesis to AIDS by promoter alleles of IL10. Proc. Natl. Acad. Sci. U. S. A. 97, 14467–14472. doi: 10.1073/pnas.97.26.14467
Siliciano, J. D., Siliciano, R. F. (2016). Recent developments in the effort to cure HIV infection: going beyond N = 1. J. Clin. Invest. 126, 409–414. doi: 10.1172/JCI86047
Sirugo, G., Williams, S. M., Tishkoff, S. A. (2019). The missing diversity in human genetic studies. Cell 177. 26–31 doi: 10.1016/j.cell.2019.02.048
Skoglund, P., Thompson, J. C., Prendergast, M. E., Mittnik, A., Sirak, K., Hajdinjak, M., et al. (2017). Reconstructing prehistoric African population structure. Cell 171, 59–71.e21. doi: 10.1016/j.cell.2017.08.049
Smith, M. W., Dean, M., Carrington, M., Winkler, C., Huttley, G. A., Lomb, D. A., et al. (1997). Contrasting genetic influence of CCR2 and CCR5 variants on HIV-1 infection and disease progression. Hemophilia Growth and Development Study (HGDS), Multicenter AIDS Cohort Study (MACS), Multicenter Hemophilia Cohort Study (MHCS), San Francisco City Cohort. Science 277, 959–965. doi: 10.1126/science.277.5328.959
Smith, M. W., Patterson, N., Lautenberger, J. A., Truelove, A. L., McDonald, G. J., Waliszewska, A., et al. (2004). A high-density admixture map for disease gene discovery in African Americans. Am. J. Hum. Genet. 74, 1001–1013. doi: 10.1086/420856
Soodyall, H. (2006). The prehistory of Africa: tracing the lineage of modern man. Soodyall Jeppestown, H, editor. (South Africa: Jonathan Ball Publisher).
Soodyall, H., Makkan, H., Haycock, P., Naidoo, T. (2008). The genetic prehistory of the Khoe and San. S. Afr. Humanity 20, 37–48.
Tafuma, T. A., Burnett, R. J., and Huis In ‘t Veld, D. (2014). National guidelines not always followed when diagnosing smear-negative pulmonary tuberculosis in patients with HIV in Botswana. PLoS One 9, e88654. doi: 10.1371/journal.pone.0088654
Taha, T. E. (2011). Mother-to-child transmission of HIV-1 in sub-Saharan Africa: past, present and future challenges. Life Sci. 88, 917–921. doi: 10.1016/j.lfs.2010.09.031
Tau, T., Davison, S., D’Amato, M. E. (2015). Polymorphisms at 17 Y-STR loci in Botswana populations. Forensic Sci. Int. Genet. 17, 47–52. doi: 10.1016/j.fsigen.2015.03.001
Tau, T., Wally, A., Fanie, T. P., Ngono, G. L., Mpoloka, S. W., Davison, S., et al. (2017). Genetic variation and population structure of Botswana populations as identified with AmpFLSTR identifiler short tandem repeat (STR) loci. Sci. Rep. 7, 6768. doi: 10.1038/s41598-017-06365-y
Telenti, A., Goldstein, D. B. (2006). Genomics meets HIV-1. Nat. Rev. Microbiol. 4, 865–873. doi: 10.1038/nrmicro1532
The 1000 Genomes Project Consortium (2010). A map of human genome variation from population-scale sequencing. Nature 467, 1061–1073. doi: 10.1038/nature09534
The International HapMap Consortium (2003). The International HapMap Project. Nature 426, 789–796. doi: 10.1038/nature02168
Tishkoff, S. A., Reed, F. A., Friedlaender, F. R., Ehret, C., Ranciaro, A., Froment, A., et al. (2009). The genetic structure and history of Africans and African Americans. Science 324, 1035–1044. doi: 10.1126/science.1172257
Tishkoff, S. A., Reed, F. A., Ranciaro, A., Voight, B. F., Babbitt, C. C., Silverman, J. S., et al. (2007). Convergent adaptation of human lactase persistence in Africa and Europe. Nat. Genet. 39, 31–40. doi: 10.1038/ng1946
Tishkoff, S. A., Williams, S. M. (2002). Genetic analysis of African populations: human evolution and complex disease. Nat. Rev. Genet. 3, 611–621. doi: 10.1038/nrg865
Tough, R. H., McLaren, P. J. (2019). Interaction of the host and viral genome and their influence on HIV disease. Front. Genet. 9, 720. doi: 10.3389/fgene.2018.00720
Troyer, J. L., Nelson, G. W., Lautenberger, J. A., Chinn, L., McIntosh, C., Johnson, R. C., et al. (2011). Genome-wide association study implicates PARD3B-based AIDS restriction. J. Infect. Dis. 203, 1491–1502. doi: 10.1093/infdis/jir046
UNAIDS. (2017). UNAIDS data 2017. Jt. United Nations Program. HIV/AIDS, 1–248. doi: 978-92-9173-945-5
UNAIDS (2019). UNAIDS is greatly encouraged by news of a possible cure of an HIV-positive man | UNAIDS. Available at: http://www.unaids.org/en/resources/presscentre/pressreleaseandstatementarchive/2019/march/20190305_PS_cure [Accessed May 10, 2019].
Underhill, P. A., Kivisild, T. (2007). Use of Y chromosome and mitochondrial DNA population structure in tracing human migrations. Annu. Rev. Genet. 41, 539–564. doi: 10.1146/annurev.genet.41.110306.130407
Uren, C., Kim, M., Martin, A. R., Bobo, D., Gignoux, C. R., Van Helden, P. D., et al. (2016). Fine-scale human population structure in Southern Africa reflects ecogeographic boundaries. Genetics 204, 303–314. doi: 10.1534/genetics.116.187369
van Manen, D., Delaneau, O., Kootstra, N. A., Boeser-Nunnink, B. D., Limou, S., Bol, S. M., et al. (2011). Genome-wide association scan in HIV-1-infected individuals identifying variants influencing disease course. PLoS One 6, e22208. doi: 10.1371/journal.pone.0022208
Veeramah, K. R., Hammer, M. F. (2014). The impact of whole-genome sequencing on the reconstruction of human population history. Nat. Publ. Gr. 15, 149–162. doi: 10.1038/nrg3625
Vella, S., Schwartlander, B., Sow, S. P., Eholie, S. P., Murphy, R. L. (2012). The history of antiretroviral therapy and of its implementation in resource-limited areas of the world. AIDS 26, 1231–1241. doi: 10.1097/QAD.0b013e32835521a3
Verma, S. S., Ritchie, M. D. (2018). Another round of “Clue” to uncover the mystery of complex traits. Genes (Basel). 9, 61. doi: 10.3390/genes9020061
Vermund, S. H., Sheldon, E. K., Sidat, M. (2015). Southern Africa: the highest priority region for HIV prevention and care interventions. Curr. HIV/AIDS Rep. 12, 191–195. doi: 10.1007/s11904-015-0270-z
Walker, N. F., Stek, C., Wasserman, S., Wilkinson, R. J., Meintjes, G. (2018). The tuberculosis-associated immune reconstitution inflammatory syndrome: recent advances in clinical and pathogenesis research. Curr. Opin. HIV AIDS 13, 512–521. doi: 10.1097/COH.0000000000000502
Warde-Farley, D., Donaldson, S. L., Comes, O., Zuberi, K., Badrawi, R., Chao, P., et al. (2010). The GeneMANIA prediction server: biological network integration for gene prioritization and predicting gene function. Nucleic Acids Res. 38, W214–W220. doi: 10.1093/nar/gkq537
Wei, Z., Liu, Y., Xu, H., Tang, K., Wu, H., Lu, L., et al. (2015). Genome-wide association studies of HIV-1 host control in ethnically diverse Chinese populations. Sci. Rep. 5, 10879. doi: 10.1038/srep10879
Welzel, T. M., Gao, X., Pfeiffer, R. M., Martin, M. P., O’Brien, S. J., Goedert, J. J., et al. (2007). HLA-B Bw4 alleles and HIV-1 transmission in heterosexual couples. AIDS 21, 225–229. doi: 10.1097/QAD.0b013e3280123840
Wen, Y.-J., Zhang, H., Ni, Y.-L., Huang, B., Zhang, J., Feng, J.-Y., et al. (2018). Methodological implementation of mixed linear models in multi-locus genome-wide association studies. Brief. Bioinform. 19, 700–712. doi: 10.1093/bib/bbw145
Wilkinson, E., Engelbrecht, S., de Oliveira, T. (2015). History and origin of the HIV-1 subtype C epidemic in South Africa and the greater Southern African region. Sci. Rep. 5, 16897. doi: 10.1038/srep16897
Winkler, C. A. (2008). Identifying host targets for drug development with knowledge from genome-wide studies: lessons from HIV-AIDS. Cell Host Microbe 3, 203–205. doi: 10.1016/j.chom.2008.04.001
Xie, W., Agniel, D., Shevchenko, A., Malov, S. V., Svitin, A., Cherkasov, N., et al. (2017). Genome-wide analyses reveal gene influence on HIV disease progression and HIV-1C acquisition in Southern Africa. AIDS Res. Hum. Retroviruses 33, 597–609. doi: 10.1089/aid.2016.0017
Yang, J., Lee, S. H., Goddard, M. E., Visscher, P. M. (2011). GCTA: a tool for genome-wide complex trait analysis. Am. J. Hum. Genet. 88, 76–82. doi: 10.1016/j.ajhg.2010.11.011
Worldometers. (2019). Southern Africa population. Available at http://www.worldometers.info/world-population/southern-africa-population/ [Accessed 14 January 2019].
Keywords: population structure, diversity, genome-wide association studies (GWAS), host genetics, Southern Africa, HIV-1
Citation: Thami PK and Chimusa ER (2019) Population Structure and Implications on the Genetic Architecture of HIV-1 Phenotypes Within Southern Africa. Front. Genet. 10:905. doi: 10.3389/fgene.2019.00905
Received: 16 May 2019; Accepted: 26 August 2019;
Published: 27 September 2019.
Edited by:
William Scott Bush, Case Western Reserve University, United StatesReviewed by:
Fabio Marroni, University of Udine, ItalyMark Z. Kos, University of Texas Rio Grande Valley Edinburg, United States
Copyright © 2019 Thami and Chimusa. This is an open-access article distributed under the terms of the Creative Commons Attribution License (CC BY). The use, distribution or reproduction in other forums is permitted, provided the original author(s) and the copyright owner(s) are credited and that the original publication in this journal is cited, in accordance with accepted academic practice. No use, distribution or reproduction is permitted which does not comply with these terms.
*Correspondence: Emile R. Chimusa, ZW1pbGUuY2hpbXVzYUB1Y3QuYWMuemE=