- 1HudsonAlpha Institute for Biotechnology, Hunstville, AL, United States
- 2Department of Biotechnology Science and Engineering, University of Alabama in Huntsville, Hunstville, AL, United States
- 3Division of Geriatric Medicine, Department of Medicine, University of Pittsburgh School of Medicine, Pittsburgh, PA, United States
- 4Institute on Aging of UPMC, University of Pittsburgh School of Medicine, Pittsburgh, PA, United States
- 5Department of Biostatistics, University of Pittsburgh Graduate School of Public Health, Pittsburgh, PA, United States
- 6Department of Microbiology and Molecular Genetics, University of Pittsburgh School of Medicine, Pittsburgh, PA, United States
- 7UPMC Hillman Cancer Center, Pittsburgh, PA, United States
Background: Recent studies investigating longevity have revealed very few convincing genetic associations with increased lifespan. This is, in part, due to the complexity of biological aging, as well as the limited power of genome-wide association studies, which assay common single nucleotide polymorphisms (SNPs) and require several thousand subjects to achieve statistical significance. To overcome such barriers, we performed comprehensive DNA sequencing of a panel of 20 genes previously associated with phenotypic aging in a cohort of 200 individuals, half of whom were clinically defined by an “early aging” phenotype, and half of whom were clinically defined by a “late aging” phenotype based on age (65–75 years) and the ability to walk up a flight of stairs or walk for 15 min without resting. A validation cohort of 511 late agers was used to verify our results.
Results: We found early agers were not enriched for more total variants in these 20 aging-related genes than late agers. Using machine learning methods, we identified the most predictive model of aging status, both in our discovery and validation cohorts, to be a random forest model incorporating damaging exon variants [Combined Annotation-Dependent Depletion (CADD) > 15]. The most heavily weighted variants in the model were within poly(ADP-ribose) polymerase 1 (PARP1) and excision repair cross complementation group 5 (ERCC5), both of which are involved in a canonical aging pathway, DNA damage repair.
Conclusion: Overall, this study implemented a framework to apply machine learning to identify sequencing variants associated with complex phenotypes such as aging. While the small sample size making up our cohort inhibits our ability to make definitive conclusions about the ability of these genes to accurately predict aging, this study offers a unique method for exploring polygenic associations with complex phenotypes.
Introduction
Exceptional longevity is influenced by a combination of environmental and genetic factors, and previous twin studies report that the heritability of human longevity is approximately 25% (Herskind et al., 1996). Family studies have suggested that exceptional aging tends to run in families, yet the search for genetic determinants of longevity has produced inconsistent results (Sebastiani et al., 2012; Sebastiani et al., 2013; Pilling et al., 2017). Several genome-wide association (GWA) studies have attempted to pinpoint genetic influences of healthy aging or longevity, yet only two loci, TOMM40/APOE/APOC and FOXO3A, have repeatedly reached genome-wide significance (Deelen et al., 2014; Broer et al., 2015). Thus, an alternative approach to understanding genetic factors underlying a complex phenotype like exceptional aging is warranted.
In our analysis, we utilized a comprehensive targeted sequencing approach designed to interrogate rare and common variants in both coding and non-coding regions within 20 key genes that are strongly associated with involvement in aging related processes and have utilized traditional statistical and machine learning approaches to explore aging-related genetic variants. The 20 genes were chosen because they have previously been associated with various molecular functions involved in aging, such as DNA damage response and repair, telomere maintenance, metabolism, cellular senescence, and stress resistance. There is ample evidence suggesting a causal role of DNA damage in aging and age-related diseases; for example most progeroid syndromes, including Werner syndrome, Cockayne syndrome (CS), and Fanconi anemia, are characterized by accelerated aging, possibly as a result of hypersensitivity to genotoxins predominantly due to problems with DNA repair and genome maintenance (Gensler and Bernstein, 1981; Hoeijmakers, 2009; Behrens et al., 2014; Vermeij et al., 2016).
Several lines of evidence also suggest that levels of DNA damage increase with age, whereas DNA repair capacity in mammals reduces with age (Niedernhofer et al., 2018). Comparative studies in mammals further indicate that species longevity positively correlates with DNA repair efficiency (Hart and Setlow, 1974; Tian et al., 2017; Ma and Gladyshev, 2017). Long-lived species such as the naked mole rat, Heterocephalus glaber, and bowhead whale, Balaena mysticetus, have a higher copy number of genes associated with DNA repair, possibly allowing for decreased susceptibility to age-accumulated DNA damage (Macrae et al., 2015; Tian et al., 2019). Therefore, we hypothesized that variants associated with DNA repair, telomere maintenance, and genomic stability could be predictive of phenotypic age.
Single variant association tests, such as linear regression, have been the statistical tools of choice for large GWA studies. In fact, many longevity-targeted GWA studies have taken this approach (Newman et al., 2010; Broer et al., 2015). However, such univariate models leave out epistatic effects that may be predictive of heterogeneous diseases, such as aging, resulting in frequent preclusion of the actual number of genetic factors contributing to or predictive of polygenic diseases (Stephan et al., 2015). More complex statistical approaches would account for genetic factors that alone have little association, but when considered in a multiplex manner, hold great predictive power. Random forest and support vector machines (SVMs) are just two of a multitude of ensemble learning methods capable of analyzing large data sets such as those obtained in GWA studies. Random forest couples bootstrap sampling and conditional inference trees for determining the importance of variables for classifying data (Lunetta et al., 2004). We sought to use random forest in our analysis of phenotypic aging, as it is capable of handling sizable data sets, considers the interactions between variables, and provides importance measures for predictors. On the other hand, SVM is a type of supervised learning that not only supports high dimensional data, but is robust against noise and sparsity in the data (Furey et al., 2000). SVM functions by taking a set of input features or data and defining an optimal decision boundary or hyperplane that most accurately separates the input space based on assigned binary classifiers. These factors allow for better determination of genetic predictors in polygenic diseases that might be due to nonlinear interactions in both common and rare variants, and was thus also implemented in our analysis (Lunetta et al., 2004).
For this study, we sequenced a panel of 20 aging-related genes with a targeted sequencing method previously developed by our lab in a cohort of 200 individuals selected from the University of Pittsburgh Claude D. Pepper Older Americans Independence Center (Day et al., 2014). Half of the cohort was labeled as phenotypic “early” agers, as determined by age (65–75 years old) and the inability to either walk up a flight of stairs or walk for 15 min without resting. The other half of the cohort was labeled as phenotypic “late” agers defined by age (>75 years old) and the ability to pass the walking tests performed on the early agers. We point out that regardless of grouping, all patients were ambulatory. In addition to gait, we also assessed multiple parameters of function, mental status, strength, and activity and number of diseases (comorbidity index).
After applying univariate and multivariate analyses to the sequencing data, we show that a decision tree-based method, random forest, trained on genetic markers in the discovery cohort shows promise in predicting phenotypic age. Despite the fact that a sample set of 200 is small for this genomic study, we show that our exploratory analysis to determine genetic predictors of aging provides a useful and novel mechanistic approach for investigating the association of polygenic risk variants with complex diseases. Further analyses with larger cohorts would find this approach valuable for determining a set of genetic variants, which when considered alone do not hold predictive value, but in combination are highly predictive of phenotypic aging. A predictive model of early phenotypic aging would not only give insight into key biological processes of this complex phenotype, but could potentially be used in a clinical setting as a diagnostic tool to indicate patients who may be at risk for early onset of age-related diseases.
Materials and Methods
Cohort Characteristics
Discovery Set UPMC (University of Pittsburgh Medical Center Cohort) Participants
Participants were recruited through the University of Pittsburgh Claude D. Pepper Older Americans Independence Center, which maintains a registry of more than 2,500 older adults who live in the greater Pittsburgh area and are interested in participating in clinical research. Print and radio ads were also used to recruit additional patients. Respondents were screened with a standardized phone interview. All study participants were community-dwelling and medically-stable volunteers who were independently mobile. Most respondents (~90%) were of self-reported Caucasian ethnic background (Figures S1 and S2).
Assessments
● Demographic information: Age, gender, level of education, and smoking status.
● Body composition: Height, weight, and dual x-ray absorptiometry (DXA) to measure total fat and lean body mass.
● Cognitive function: Montreal Cognitive Assessment (MOCA) and Digit Symbol Substitution Test (DSST). Higher scores indicate better cognitive function.
● General health: Comorbidities were assessed using a comorbidity index (Rigler et al., 2002); a higher score suggests a greater number of comorbidities and poorer health (Sangha et al., 2003). The SF-36 measured patients' self-reported health and wellness; higher scores indicate better health (Ware and Sherbourne, 1992). Finally, participants were characterized as frail, prefrail, or robust using the five-item Fried Frailty Index; higher scores indicate frailty (Figure S3) (Abellan van Kan et al., 2008).
● Function and activity: We used the Community Healthy Activities Model Program for Seniors (CHAMPS) Physical Activity Questionnaire to assess the frequency of activity and estimate calories per week involved in the activity (Stewart et al., 2001). We assessed grip strength with a standard dynamometer. The short physical performance battery (SPPB) was used, which provides an integrated physical assessment based on several measures, including gait speed, chair stand, and balance; a higher score indicates better performance (Vasunilashorn et al., 2009).
Validation Set (Wellderly Cohort)
The Wellderly Cohort consists of individuals of at least 80 years of age with no chronic disease or need for chronic medications. Sample collection and processing for whole genome sequencing (WGS) as well as variant calling are as previously described (Erikson et al., 2016). Individuals used in this study had an average age of 86 and consisted of less males (n = 195) than females (n = 316). Comparison of overlapping clinical features in the discovery and validation cohorts were assessed to ensure a similar population distribution (Figures S4A, B). Furthermore, the cohort contains no enrichment for longevity variants.
Participant Group Determination
We sought to maximize the signal with respect to any genetic differences between the groups. Because there is no standard operational criterion for defining early and late agers, we used self-reported and performance-based measures of mobility (Abellan van Kan et al., 2008), strongly associated with incident functional decline, disability, and mortality in the elderly (Perera et al., 2016). As such, we operationally defined “early aged” participants as those 65–75 years of age who could not walk up a flight of stairs or walk for 15 min without resting; “late aged” were those age 75 years and older who could walk up a flight of stairs or walk for 15 min without resting. The age cut-off of 75 years was chosen as it has been utilized in numerous phenotypical aging studies in older adults (Boonen et al., 2006; Boonen et al., 2010; McClung et al., 2012). We excluded participants with a history of a major cancer. Table 1 depicts the differences in participant characteristics between groups.
Variant Genotyping
Clone adapted template capture hybridization sequencing (CATCH-Seq) was used as an alternative to other sequencing methods due to the low cost and high coverage ability of both coding and noncoding genomic regions (Day et al., 2014). CATCH-Seq yield is comparable to WGS (89 versus 98% at 100x) but at a fraction of the cost, allowing for more samples to be included in a study when only a small set of genes are under investigation, as is the case in this investigation. CATCH-Seq probes were designed to capture ~150–200 kilobase (kb) regions around each of the 20 target genes (Table 2). Standard Illumina sequencing libraries were hybridized to the CATCH-Seq probes, and the target-enriched libraries were subjected to 2 x 100 base pair (bp) paired-end sequencing on HiSeq 2500 sequencers. The resulting sequence data was aligned to the human reference genome (GRCh37) with Burrows-Wheeler Aligner (BWA) (Li and Durbin, 2009), and variants were called using GATK v2 (McKenna et al., 2010) with exclusion filters for variants with low mapping quality (mapq < 20) and low genotype quality (q < 30).
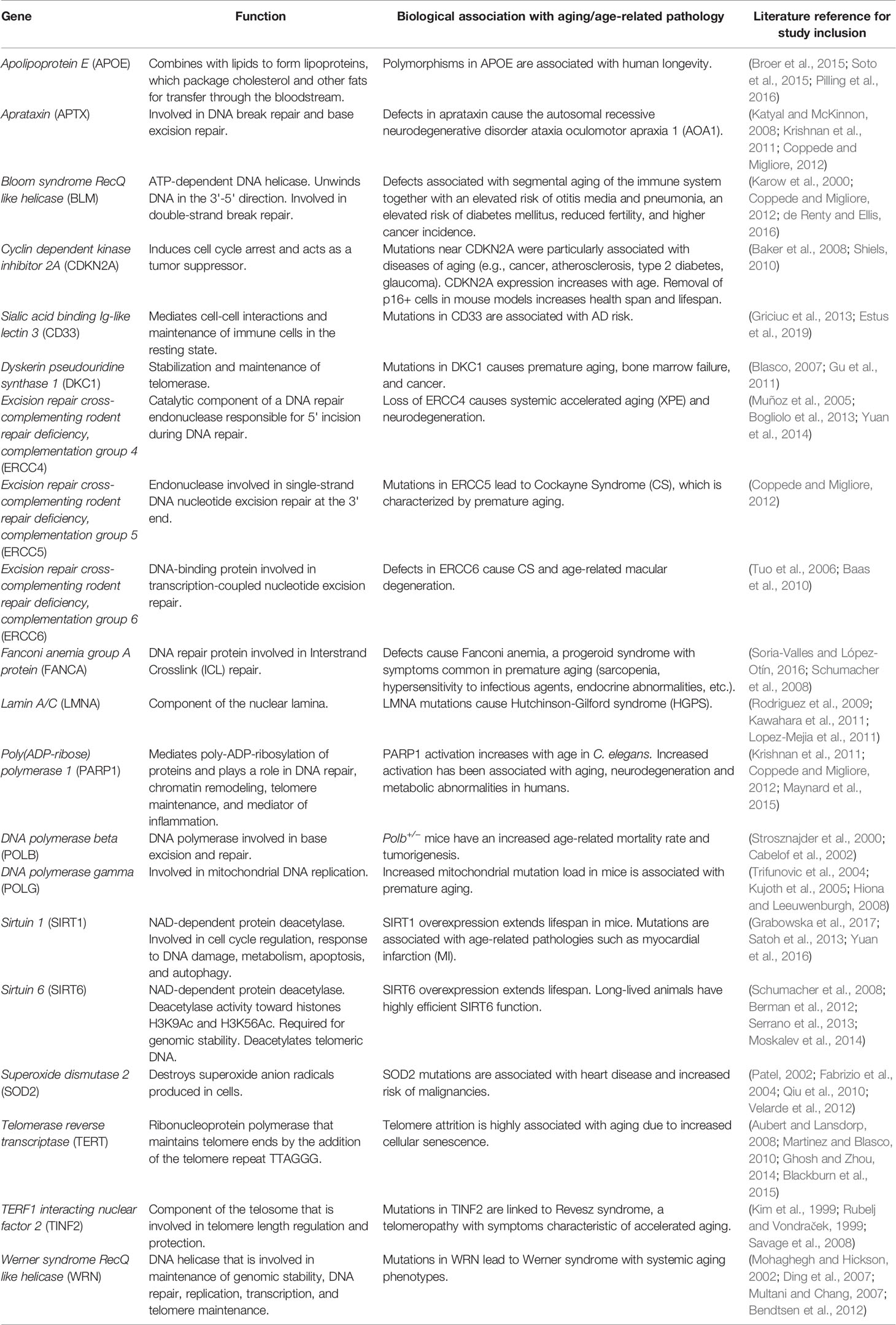
Table 2 Names, biological function, and literature references for aging association of the 20 genes sequenced.
Quality Control
Variant Inclusion Criteria
The initial datasets consisted of 25,273 variants in the discovery cohort and 8,018 variants in the validation cohort (Table S1). Rare variants, or those with less than eight alleles observed in the discovery cohort, and those with variants with over 10% missing data, were excluded. Variants not covered in both the discovery and validation cohort were also excluded. Variants were then imputed across individual genes +/− 50 kb using K-nearest neighbor imputation via the impute package in R (Hastie et al., 2001). A total of 5,896 variants was selected for further analysis.
Statistics
Total Variance Analysis
The sum of all variance between groups was analyzed using a Wilcoxon rank-sum test to determine whether “early” agers had more or less genetic variance in the target genes compared to “late” agers.
Single Variant Association
Logistic regression was utilized to assess the association of any single variant to the age group phenotype. A quantile-quantile (QQ) plot was used for evaluation of the distribution of p-values.
Gene Association
Wilcoxon rank-sum tests were used to compare the distribution of Combined Annotation-Dependent Depletion (CADD) scores of non-reference alleles near target genes (+/− 50 kb) between early and late agers. P-values were adjusted for multiple hypothesis via the Bonferroni method.
Predictive Modeling
Four-fold cross-validation with four different seeds using a random forest regression model via the RandomForest package in R as well as SVM classification via the e1071 package in R were conducted for predictive modeling of the aging phenotype (Liaw and Wiener, 2002; Dimitriadou et al., 2005). Default settings for number of trees grown (n = 500) and number of variables tried at each split (mtry = 6) were used for each random forest model. An SVM model was tuned using a range of costs (c = 0.1, 1.0, 10.0, 100.0) and gamma values (gamma = 0.5, 1, 2). Both random forest and SVM modeling were performed on 28 different stratifications of the data in addition to a control data set (Table S2) resulting in 928 models in total. Most of the data subsets consisted of different groups of genomic spaces within the sequenced data as well as filters for frequency and deleteriousness. The first subsets of the data contained all sequence variants in addition to groups with different filters, including a subset of rare variants (tAF < 0.1), very rare variants (tAF < 0.01), mildly deleterious, and highly deleterious variants as defined by the CADD score (CADD > 10 and CADD > 15, respectively). We then took subsets of only the variants within the start and end site of the target genes and then applied the same filters as the first to analyze rare (tAF < 0.1), very rare (tAF < 0.01), mildly (CADD > 10), and highly (CADD > 15) deleterious variants. The next set of subsections contained target gene variants plus 50 kb up- and down-stream of the transcription start and end sites to capture regulatory genomic space within the analysis. Once again, the same cutoffs for allele frequency and CADD score were applied. The last genomic space stratification included variants within exons of the target gene isoforms, thus eliminating intronic space from the models. Allele frequency and CADD score cutoffs further stratified the exonic variant subset. In addition to stratifications of the genomic space, publicly available databases such as the Genome-Wide Repository of Associations Between Phenotypes (GRASP), the single nucleotide polymorphisms (SNP) and copy number annotation (SCAN) database, and software such as SIFT (sorting intolerant from tolerant) were utilized for grouping the data based on variant effect (Ng and Henikoff, 2003; Gamazon et al., 2010; Lonsdale et al., 2013; Leslie et al., 2014). For this, we analyzed known versus unknown variant models, SIFT deleterious variants vs. SIFT tolerated variants, variants effecting expression, and GWAS variants. We also included a control set, which was made by randomly shuffling all of the variants. We did not adjust for age in any of the models tested, because age should not affect this analysis of SNPs.
We assessed the performance of each model using receiver-operating characteristics (ROCs). Additionally, we used Bayesian Classifier to determine the optimal cut-off between early and late agers in the random forest regression analysis. Top performing SVM and random forest models were tested on the validation (Wellderly) cohort of late agers, and the misclassification percentage, based on the optimal cut-off, was used to rank each model rather than ROC-area under the curve (AUC), since the cohort comprises a single class (late agers) rather than the binary class available in the discovery cohort. Top classifiers in the best performing random forest model were determined by analyzing the Gini importance measures (Gini coefficient) for each split in the top models, which gives a measure of variable importance. In other words, the higher the Gini coefficient, the better the classifier is at accurately splitting the data between two classes.
Enrichment Analysis
Enrichment for specific genomic domains and functions within the top variants was determined using a variety of tools. Enrichment of rare or severely deleterious variants was analyzed by assessing allele frequency and CADD scores of the top variants. We utilized the UCSC Genome Browser for determination of the specific location of each variant for analysis of intronic or exonic SNP enrichment (Kent et al., 2002). GRASP was used to discover whether the top classifying SNPs have been previously associated with specific phenotypes (Leslie et al., 2014). The Roadmap Epigenomics Project database was used to ascertain how many top variants were within regulatory regions via data from the HepG2 hepatocellular carcinoma cell line as well as GM12878 lymphoblastoid cells (Chadwick, 2012). Lastly, enrichment for transcription factor binding sites within the top 50 variants was assessed using data from the ENCODE database (ENCODE Project Consortium, 2012).
Results
Clinical Characteristics
As expected by design, and despite its older age, the late aging group had better scores for gait speed, chair rise time, SPPB, physical function, self-perceived health, bodily pain, social function, mental health, and vitality (all p < 0.05). The late aging group was also less likely to suffer from comorbidity or frailty (p < 0.05), expended more calories from all activity per week and from moderate activity per week, and displayed a higher frequency of all and moderate activity. However, cognitive scores were similar between the two groups.
Logistic Regression
To identify high-impact aging-related variants, variants were tested for association with the aging group using logistic regression. Top variants were within intronic and upstream regions of lamin A (LMNA) (rs915180, p value = 0.0015) and Werner syndrome ReqQ like helicase (WRN) (rs6989940, p value = 0.0017), however none of the top hits reached significance beyond what would be expected by chance given the number of individual variant tests. A QQ plot of the logistic regression p-values indicated deflation as a result of a lack of power owing to the small sample size in this study (Figure 1A and Table S3).
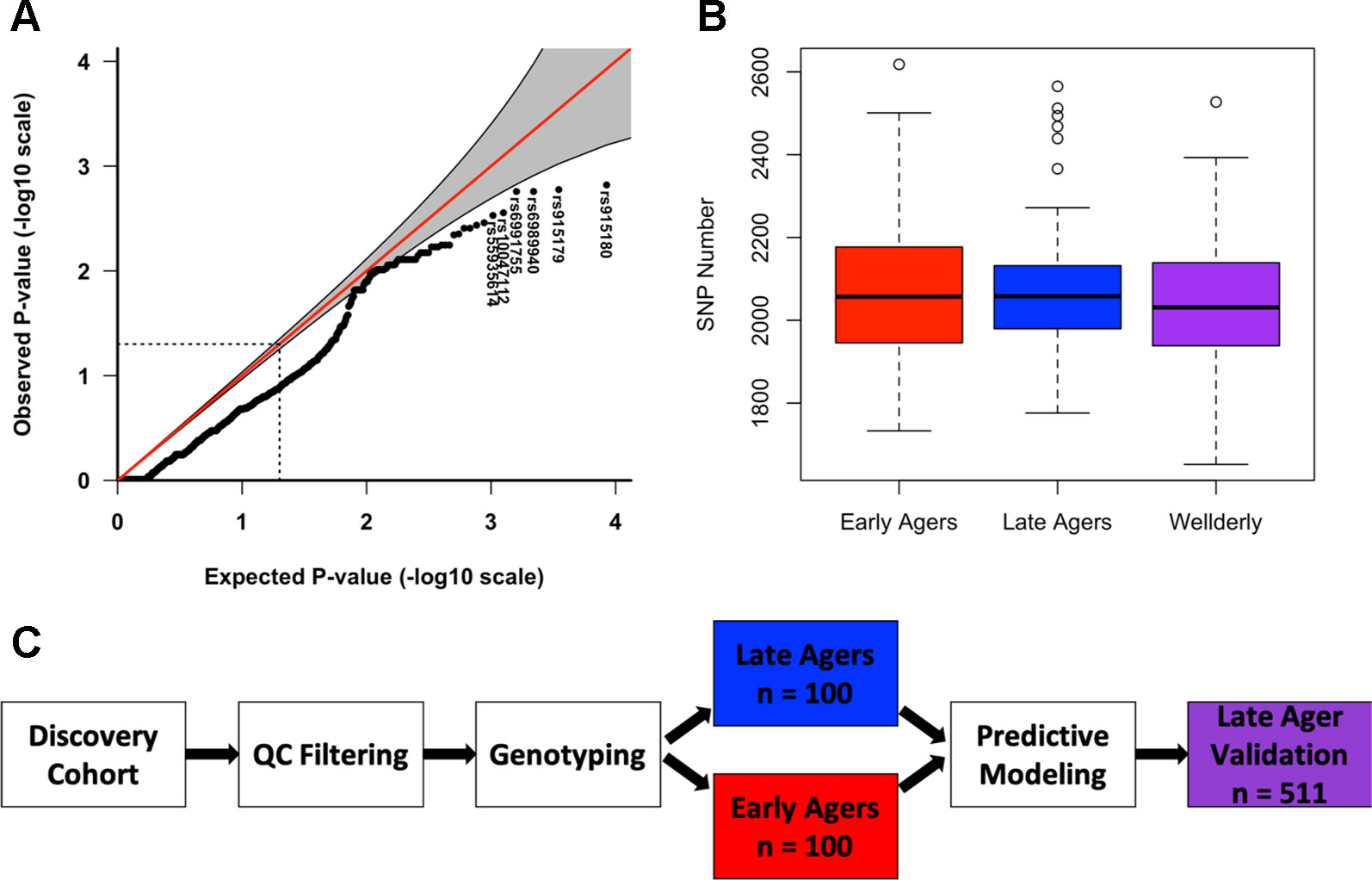
Figure 1 Logistic regression and variant burden reveal lack of association with early aging. (A) Quantile-quantile plot of logistic regression p-values. (B) Box plot of total number of variants in the discovery early aged group (red), discovery late ager group (blue), and the validation late ager group (purple). (C) Diagram of predictive modeling analysis study design.
Variant Burden
We combined the number of alternate alleles among all 20 genes in each subject following simple inclusion criteria of the variants for quality control to determine if early agers had a larger variant burden in aging-related genes compared to late agers, finding no significant difference (Wilcoxon p value = 0.75) (Figure 1B). This method was then repeated for each individual gene, for which we compared the total amount of non-reference alleles in early agers compared to late agers in order to test whether the variant burden in that gene differed between groups. There was little difference in total non-reference allele count per target gene between early and late agers for most of the genes analyzed (Figures S5A, B). However, LMNA approached the Bonferroni corrected p-value of 0.003 according to a Wilcoxon rank-sum test (p-value = 0.006, FDR = 0.1).
Machine Learning
Since neither the univariate nor the gene-based multivariate analyses yielded statistically significant associations with aging group, we moved on to a computational approach geared at determining the predictive power of our sequencing data for aging status. Both random forest and SVM were applied to the variant data to determine the best genetic predictors of late aging. The ability of both the random forest algorithm and SVM to outperform other non-parametric classification methods led to our use of these predictive modeling approaches in this study (Furey et al., 2000; Lunetta et al., 2004). As depicted in Figure 1C, the training cohorts were divided into early and late agers for random forest model training, and top performing models according to the ROC-AUC were then tested for prediction of aging status in the validation cohort. Various stratifications of the data were fed into each algorithm to determine the best subset of predictors. These subsets included: variants of both low and high allele frequencies, variants that are known to effect expression (eQTL) defined by the SCAN database, variants previously associated with aging as determined by the GRASP database, functional variants determined by ENCODE, variants with low and high levels of deleteriousness as defined by the CADD scores, and variants near or within the target genes. Four-fold cross-validated random forest at four different seeds was performed on these various filters of the variant data as previously described, resulting in a total of 16 models per filter, or 464 total models.
The distribution of ROC-AUCs, a measure of model sensitivity and specificity, was compared to identify the top performing models (Table S4, Figure S9). Random forest performed on the non-reference alleles within the exons of the 20 target genes having a CADD score greater than 15 showed the greatest performance (mean ROC-AUC = 0.62) among random forest models, while the model trained on non-reference alleles within TFBSs proved to have the highest performance among all SVM models (Figures 2A–D), but failed to outperform the top random forest model. Furthermore, the top random forest model outperformed the random forest model trained on the control shuffled data set (Wilcoxon p-value = 1.5x10−4), demonstrating that despite having a mean AUC of 0.62, the model performs significantly better than the control model. This model also proved to outperform that of all sequenced variants (mean ROC-AUC = 0.51) (Wilcoxon p-value = 9.5x10−5). For analysis of model predictive power in an independent cohort, we tested the ability of the top random forest model to correctly identify the validation (Wellderly) cohort as late agers. As previously stated, because this cohort lacked any early agers, we used percent misclassification rather than ROC-AUC to assess prediction accuracy as ROC-AUC assessment requires two groups. This analysis revealed that the top model performed well on the model validation (Wellderly) cohort (median misclassification = 0.02) (Figure 3A). Additionally, smoking status, which is known to affect aging, was tested as a predictor of age group for comparison of genomic data to environment in predicting aging status, revealing that our model built on high CADD exon variants in aging-related genes performed comparably (Figure 3B) (Valdes et al., 2005; Csiszar et al., 2009; Astuti et al., 2017). Because there is a significant difference in BMI between early and late agers (p = 5.6 x 10−8), we tested the correlation between the predictor value and BMI in the discovery cohort for the top performing model, which revealed little correlation between age group prediction and BMI (Spearman Rho = 0.07) (Figure S6). Furthermore, a scatterplot of the predicted age group from the best model (mean ROC-AUC = 0.62) versus BMI in both cohorts details a lack of trend between the two values, further supporting that this is a model predictive of early versus late aging rather than BMI (Figure S7).
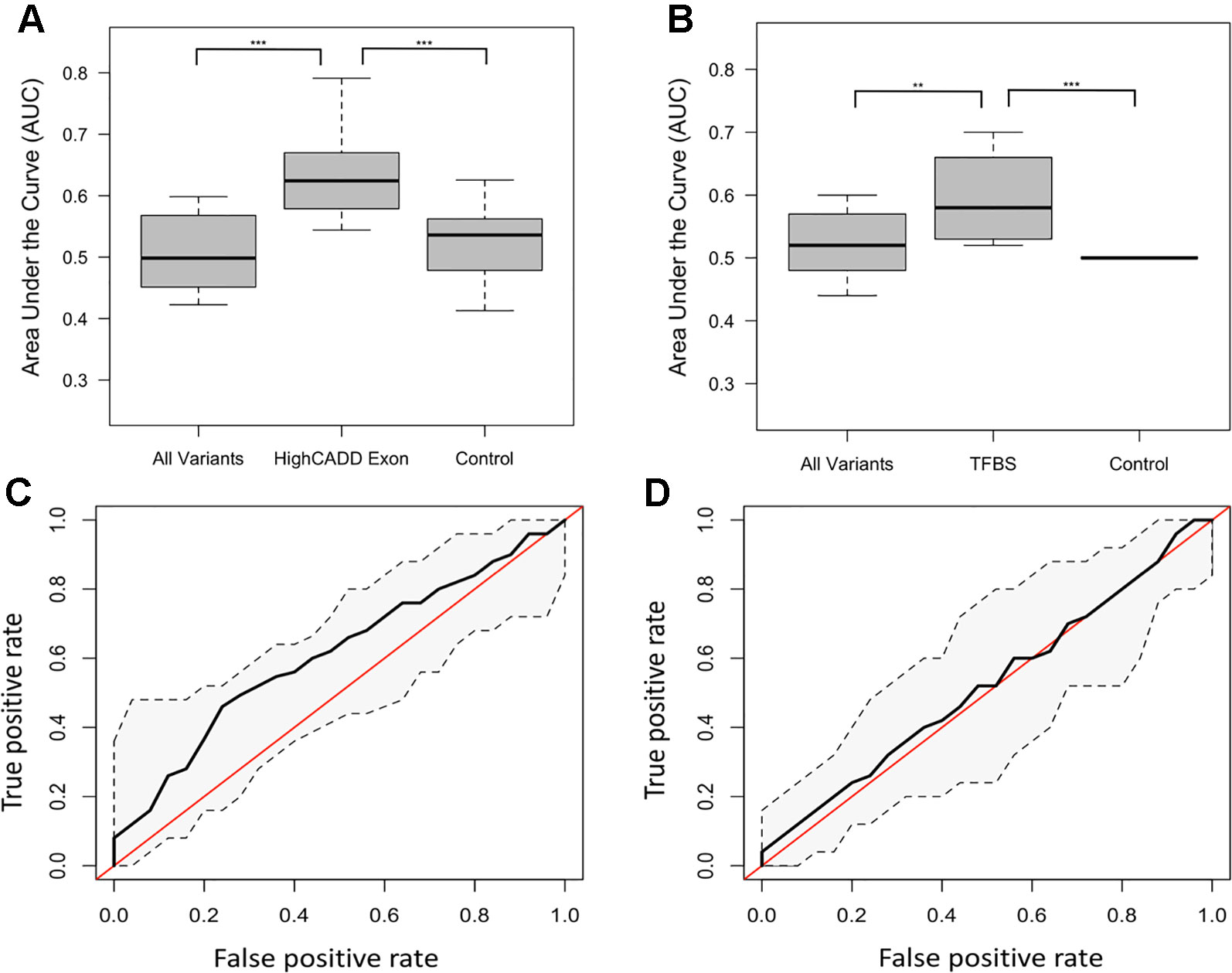
Figure 2 Different subsets of variants defined as top predictive models using random forest and support vector machine (SVM) learning methods. (A) Boxplots of the random forest model area under the curve (AUCs) for the all variant, high Combined Annotation-Dependent Depletion (CADD) exon and control subsets of the variant data. P-values between groups determined by performing a Kruskal-Wallis test. **** = p < 0.0001, *** = p < 0.001. (B) Boxplots of the SVM model AUCs for the all variant, transcription factor binding site (TFBS), and control subsets of the variant data. P-values between groups determined by performing a Kruskal-Wallis test. (C) Receiver-operating characteristic (ROC) curve of the mean high CADD exon random forest model with confidence intervals. The red line represents the null AUC (0.5). (D) ROC curve of the mean TFBS SVM model with confidence intervals. The red line represents the null AUC (0.5).
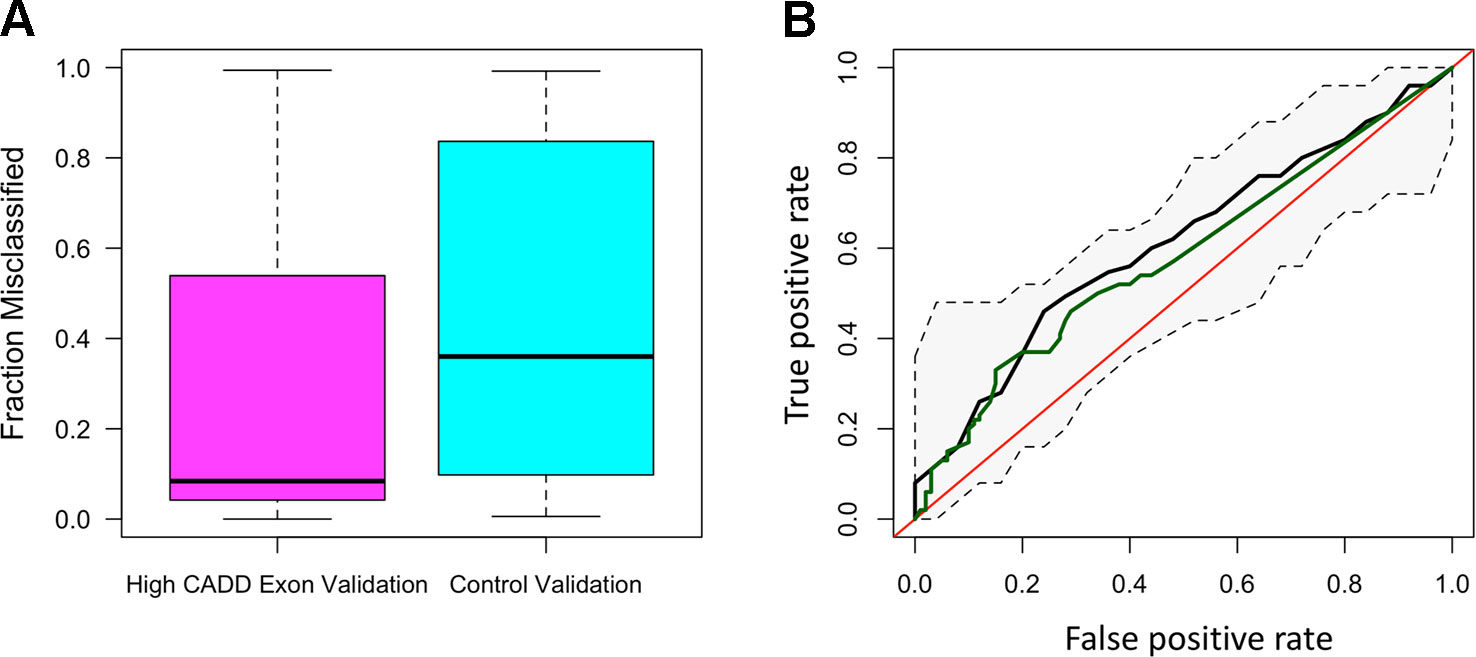
Figure 3 The random forest high Combined Annotation-Dependent Depletion (CADD) exon model is predictive of late aging status in the validation cohort and outperforms smoking as a predictor of aging. (A) Boxplots of the fraction of misclassified patient samples based on the random forest high CADD exon model (magenta) and the control random forest model (shuffled dataset) (teal). (B) Receiver-operating characteristic curve of the mean area under the curve (AUC) resulting from the random forest high CADD exon model (black) with confidence intervals in the discovery cohort and the AUC resulting from smoking status as a sole predictor of early versus late aging (green).
One of the most advantageous aspects of the random forest, especially when predicting phenotypes, is that it returns importance scores for each predictor in the model, allowing for the ranking of classifiers within the dataset and associations between predictors and phenotypes. Classifiers in the top performing model were ordered by their Gini coefficient, a measure of how well the classifier contributed to accurately separating the classes. We found that the predictors within the top performing model (high CADD exon variants) were nonsynonymous mutations within 9 of the 20 genes (APTX, BLM, ERCC4, ERCC5, ERCC6, LMNA, PARP1, POLG, and WRN).
Enrichment Analysis
Top variants were determined by averaging the Gini coefficients across the 16 models performed on the highly deleterious target gene exon data set. Enrichment analysis was then conducted on these variants in regard to gene and variant effect. We found that a majority of the top variants were located within excision repair cross complementation group 4 (ERCC4), ERCC5, LMNA, and PARP1 (Figure 4A and Table S5). Furthermore, 6 of the predictor's regions have previously been associated with more than 15 different phenotypes in the GRASP database (Table S6). Enrichment analysis of variant consequence effect revealed that predictors are enriched for those that cause a nonsynonymous change as well as a stop gain, or premature termination codon (p < 0.001) and depleted for synonymous mutations (Figure 4B).
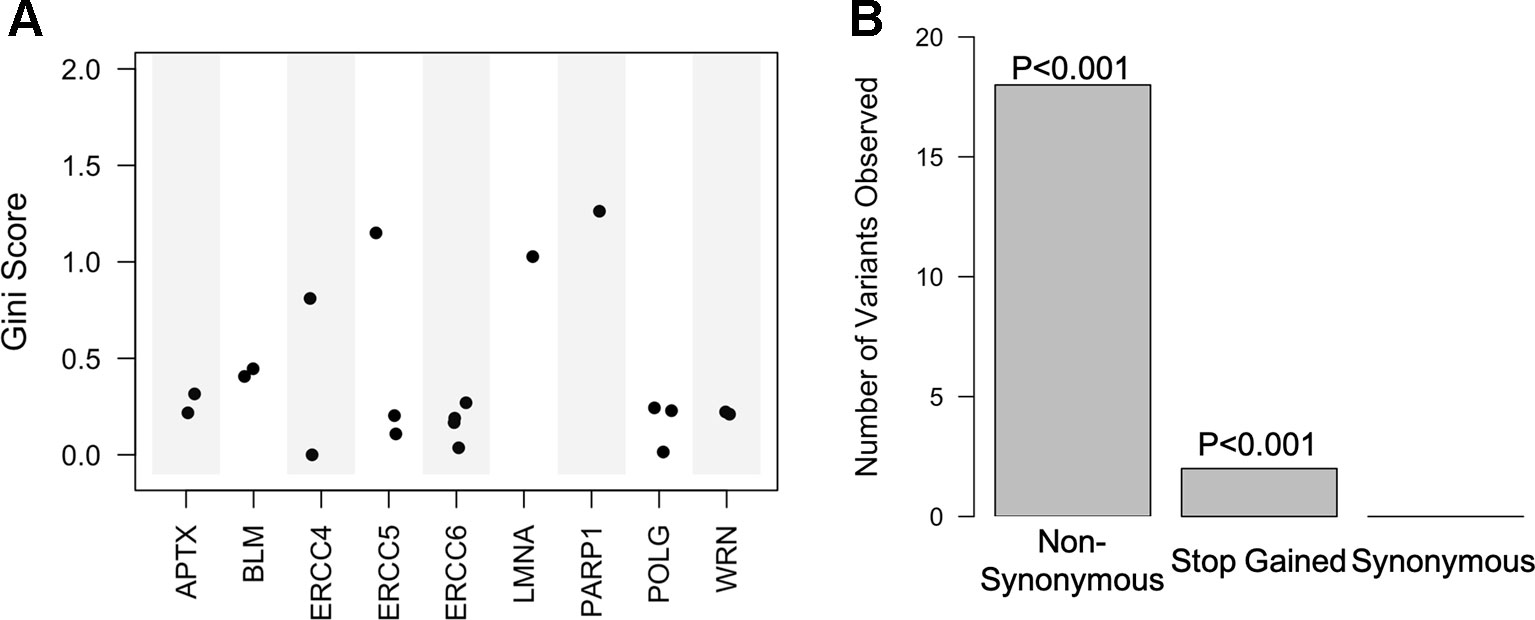
Figure 4 Random forest high Combined Annotation-Dependent Depletion exon predictive variants are within 9 of the 20 genes and mostly non-synonymous. (A) Scatter plot of the Gini score for each of the predictive variants based on corresponding gene. (B) Bar plot of the variant consequence type within the predictors with corresponding empirical p-values.
Discussion
Although aging is highly dependent on environmental, behavioral, and social interactions, studies have shown that a quarter of the variance explaining longevity is heritable (Herskind et al., 1996; Perls et al., 2002; van den Berg et al., 2018; van den Berg et al., 2019). Yet, only a handful of genetic determinants explaining a small portion of the heritability have been discovered to date. Hampering additional discovery are the complexity of the biology of aging as well as the rarity of the longevity phenotype. Analysis of late aging rather than longevity allows for larger cohort sizes, as late agers are more common in the general population than long-lived individuals (>100 years old); however, the lack of a clear definition for “healthy” or late aging makes genetic analysis and cross-study interpretation of this phenotype extremely difficult. Recently, Reed et al., defined “healthy” aging as living to the age of 70 in the absence of coronary surgery, heart attack, stroke, diabetes, or prostate cancer, finding an approximate 50% heritability of the defined phenotype in a cohort of male twins (Reed et al., 2004). Several other late aging cohorts exist, characterized by various definitions and resulting in inconsistent heritability percentages and gene associations (Walter et al., 2011; Brooks-Wilson, 2013; Erikson et al., 2016). Furthermore, large-scale aging GWA studies to date have failed to identify recurrent specific genomic regions that statistically associate with the longevity or late aging phenotypes, though combined analysis of SNPs have identified pathways and multi-allele signatures associated with aging phenotypes, indicating that these studies should include polygenic or epistatic associations in addition to the more traditional analysis of single gene associations to more successfully discover genetic determinants of aging phenotypes (Brooks-Wilson 2013). This observation led us to design a unique approach for determining genetic predictors of phenotypic aging by conducting targeted sequencing of 20 previously determined aging-related genes in a cohort of “early” and “late” agers. This approach allowed for the identification of a set of genetic variants associated with various aspects of genomic integrity as possible predictors of late aging. While we emphasize that our small discovery cohort (n = 200) is not ideal for a genomic association study, our process of combining targeted sequencing and machine learning to identify a set of genetic factors that together act as predictive determinants for a complex disease will be useful in further genetic association studies of complex phenotypes for which individual variant association is insufficient.
Our initial analysis of overall variant burden and individual variant association with early versus late aging failed to produce any variant with statistically significant association. While this is typical of GWA studies, especially those with a complex phenotype or small sample sizes, single variant association does prove useful for prioritizing variants by p-value. In our analysis, two intronic variants within LMNA had the strongest association (rs915180 and rs915179) and were also the most predictive variants in the unfiltered data set random forest models (Figure S8). These variants have also been previously associated with longevity (Table S3). In fact, rs915179 is part of a haplotype within LMNA that is specifically associated with longevity (Conneely et al., 2012). Sebastiani et al., used rs915179 as part of a “genetic signature” of exceptional longevity and later confirmed this variant in a meta-analysis of longevity (p = 0.0001) (Sebastiani et al., 2012). LMNA encodes lamins A and C, which are nuclear envelope proteins. These proteins are associated with Hutchinson-Gilford progeria syndrome (HGPS), an extremely rare disease causing premature aging and with a life expectancy of about 13 years (Conneely et al., 2012). Interestingly, defective forms of LMNA are produced in small amounts within cells of healthy individuals, and there is evidence that this amount increases with age (Rodriguez et al., 2009). This variant was also one of the first to be associated with Alzheimer's disease in GWA studies, indicating that it may play a pivotal role in cognitive function, which is known to decline with increasing age. In GWA studies, rs915180 has been associated with suicide attempts in patients with mood disorders, as well as with cardiomyopathy, chronic kidney disease, and birth weight (Perlis et al., 2010; Köttgen et al., 2010; Horikoshi et al., 2013). Since this association failed to reach genome-wide significance, future studies involving larger cohorts are needed to further assess the association of rs915179 with late aging.
Because the individual variant association proved inadequate for determining variants within our data that are predictive of aging status, we next focused our analysis on machine learning. Random forest and SVM were performed on various stratifications of the data, and assessment of the resulting ROC-AUC and misclassification percentages revealed that the random forest model built using variants with a CADD score over 15 (high CADD) proved to be the best performing predictor of aging status. As previously noted, one of the benefits of using random forest is that it ranks predictors based on how well they add to the purity of the model (Gini coefficient). The mean coefficient for each predictor in all trials of the high CADD exon variants was used as a metric with which to rank variants (Figure 4A). The variant with the highest predictive power (rs1136410) in our top performing model of aging status is located in PARP1 and causes an A > G alteration in the 17th exon (mean Gini = 1.26). PARP1 is responsible for posttranslational modification of nuclear proteins in response to various types of DNA damage as well as oxidative stress (Muiras et al., 1998; Beneke and Bürkle, 2007). With an essential role in base excision repair (BER) and double strand break (DSB) repair, PARP1 has been known as the “sensor of nicks” within DNA (Mao et al., 2011; Czarny et al., 2017). Comparative studies among 13 mammalian species found that the enzymatic activity of PARP1 positively correlates with maximum lifespan in various mammals, including humans (Bürkle et al., 1992; Muiras et al., 1998; Piskunova et al., 2008; Noren Hooten et al., 2012). Additionally, this variant has previously been associated with survival in patients with early stage non-small-cell lung cancer, depression, and baseline hippocampal volume loss in apolipoprotein E genotypeε4 (APOE4) (Nho et al., 2013).
The next strongest predictor in the top performing model is located within ERCC5/XPG (mean Gini = 1.15), located on chromosome 13q22–33 which causes a G > C (His1104Asp) change in the last (15th) exon of the gene (rs17655) (Zhao et al., 2018). ERCC5 is an excision repair gene that is responsible for forming the 3' incision during nucleotide excision repair (NER) and is known to be extremely polymorphic (Zhao et al., 2018). The variant is located within the C-terminal of the gene and inhibits interactions of ERCC5 with other DNA repair proteins (Xu et al., 2016). Damaging variants in this gene can lead to deficiencies in the NER pathway, causing xeroderma pigmentosum (XP) and Cockayne syndrome (CS), both of which result in symptoms shared with phenotypic aging (O'Donovan et al., 1994; Barnhoorn et al., 2014). Additionally, this specific variant, rs17655, is well-studied for its association with cancer risk, especially in gastric and colon cancer (Zhao et al., 2018). The well-established relationship between accelerated aging and deficient DNA damage repair (Gensler and Bernstein, 1981), in addition to the high importance this variant has in our top performing model, leads to the hypothesis that ERCC5 is important for attenuating the aging process.
The next most important variant in the predictors is within LMNA (rs513043), which causes a missense mutation (G > A) in the 2nd codon and has a CADD score of 18.44, indicating a high degree of deleteriousness (mean Gini = 1.03). LMNA encodes nuclear proteins lamins A and C for which mutations in this gene are associated with numerous diseases including cardiomyopathies, lipodystrophy, muscular dystrophies, and progeroid (early aging) syndromes, such as HGPS. Again, the nuclear lamina has been repeatedly linked to aging; in fact, Sebastiani et al., used numerous LMNA variants to build a “genetic signature” of longevity (Sebastiani et al., 2012).
Lastly, a variant in ERCC4 (rs1800067) was also one of the top predictors in the best predictive model (mean Gini = 0.81). This variant causes a missense mutation (G > A) in the 8th exon, has a CADD score of 36, indicating a very high degree of deleteriousness within the gene, and has been associated with HDL cholesterol and risk of glioma and lung cancer. ERCC4 is an excision repair gene that forms a heterodimer with excision repair cross complementation group 1 (ERCC1) for NER. Reduced expression of ERCC4-ERCC1 leads to XPF-ERCC1 (XPE) progeria in humans that is characterized by systemic accelerated aging (Niedernhofer et al., 2006). Moreover, other studies examining genes under positive selection in the longest-lived mammalian species, the bowhead whale, identified ERCC1 as a top hit, suggesting that this pathway may promote maintenance of health (Keane et al., 2015). Jorgensen et al., showed that this variant is significantly associated with benign breast disease (BBD), especially in patients with a family history of breast cancer (Jorgensen et al., 2009).
Like many genomic studies of longevity and late aging, several limitations of this study warrant comment. First, in the absence of field-wide consensus regarding the definition of early versus late aging, we relied on physical function to differentiate the two groups. The parameters used to differentiate them—the ability to walk 15 min without stopping and to climb a flight of stairs—are well-validated (Abellan van Kan et al., 2008; Perera et al., 2014; Perera et al., 2016) and can be viewed as integrative, i.e., incorporating the impact of both physiological decline and diseases. The advantage of using such standardized assessments of function is the ability to differentiate participants into non-overlapping groups. The disadvantage is that impaired function may reflect the effect of not only early aging, but also comorbidity. However, because aging is characterized by both constriction of physiological reserve and the accumulation of diseases, it is difficult to disentangle the impact of early aging and disease. It is possible that subtle effects of genes or alleles on aging were masked by the impact of superimposed diseases, but testing this hypothesis will require a study large enough to identify a sufficient number of participants who qualify as early agers in the absence of disease. It is also possible that conditions such as comorbidity, obesity, and frailty lie in the causal pathway from any genetic predispositions to functional outcomes. Therefore, efforts to control for them would attenuate any associations between genetics and the function-based group definition. Another limitation of this study, which is common among many genomic studies, is the cross-sectional design; future studies are needed to examine longitudinal trajectories. Lastly, a validation cohort consisting of both early and late agers would improve our confidence in the constructiveness of this model for both early and late aging phenotypes.
Conclusion
In conclusion, the two assessments regarding walking and stair climbing helped to identify a group of phenotypically late agers who had a better gait speed, higher activity and greater activity as well as physical function compared to a group of phenotypic early agers. This study found that more complex statistical analyses encompassing epistatic effects rather than traditional single gene association tests are useful for interpretation of rare genomic data generated using deep sequencing methods. Random forest provided information complementary to more traditional statistical analyses, including the ability to correctly classify the validation cohort of late agers 90% of the time. Predictors in the model were within genes that are involved in DNA repair and stability. We recognize that there are many genes and possibly intergenic regions of the genome engaged with genome stability and the biology of aging that were not included in this study; however, the genes chosen for analysis here are those with which the authors have had the greatest familiarity and sequence knowledge. Additionally, while we did not account for admixture in our analysis, we believe this would not drastically alter our results, as most of our discovery cohort and the entire validation cohort were of self-reported European American descent. While we realize that the training set has a low number of patients to achieve statistical certainty, we propose that holistic analysis of rare variant data may have promise in a larger cohort. Thus, targeted sequencing of genes involved in aging in combination with machine learning should be considered as a method to determine predictors of complex phenotypes.
Data Availability Statement
The raw sequencing data in this manuscript is available at NCBI (SubmissionID: SUB6523418 BioProject ID: PRJNA589212).
Ethics Statement
Patient samples were collected with written consent and in compliance with the University of the University of Pittsburgh Institutional Review Board IRB#: REN17120030/PRO14010101.
Author Contributions
SG, NR, SP, and AL conducted the experimental design. SG and NR performed patient/sample collection. SP and MB performed data analysis with the guidance of DA. MB wrote the original draft of the manuscript. All authors reviewed and helped edit the manuscript.
Funding
There was no commercial affiliation. Funds were provided by the National Institutes of Health grant for the University of Pittsburgh Pepper Older Americans Independence Center (P30AG024827) and by discretionary monies from the Office of the Senior Vice Chancellor for the Health Sciences, University of Pittsburgh, a non-profit entity. Neither funding source played any role in the study design, data collection and analysis, decision to publish, or preparation of the manuscript.
Conflict of Interest
The authors declare that the research was conducted in the absence of any commercial or financial relationships that could be construed as a potential conflict of interest.
Acknowledgments
We thank the University of Pittsburgh Pepper Older Americans Independence Center for support of recruitment and patient evaluation (P30AG024827). We also thank Ryne C. Ramaker for his advice regarding statistical analysis and assistance in drafting the written results in this study.
Abbreviations
SVM, support vector machine; TFBS, transcription factor binding site; BMI, body mass index; CADD, Combined Annotation Dependent Depletion; SIFT, sorting intolerant from tolerant; GWAS, genome wide association study; ROC, receiver operating characteristic; AUC, area under the curve.
Supplementary Material
The Supplementary Material for this article can be found online at: https://www.frontiersin.org/articles/10.3389/fgene.2019.01277/full#supplementary-material
References
Abellan van Kan, G., Rolland, Y., Bergman, H., Morley, J. E., Kritchevsky, S. B., Vellas, B. (2008). The I.A.N.A Task Force on frailty assessment of older people in clinical practice. J. Nutr. Health Aging 12, 29–37. doi: 10.1007/BF02982161
Astuti, Y., Wardhana, A., Watkins, J., Wulaningsih, W., PILAR Research Network (2017). Cigarette smoking and telomere length: a systematic review of 84 studies and meta-analysis. Environ. Res. 158, 480–489. doi: 10.1016/j.envres.2017.06.038
Aubert, G., Lansdorp, P. M. (2008). Telomeres and aging. Physiol. Rev. 88, 557–579. doi: 10.1152/physrev.000262007
Baas, D. C., Despriet, D. D., Gorgels, T. G. M. F., Bergeron-Sawitzke, J., Uitterlinden, A. G., Hofman, A., et al. (2010). The ERCC6 gene and age-related macular degeneration. PloS One 5, e13786. doi: 10.1371/journal.pone.0013786
Baker, D. J., Jin, F., Van Deursen, J. M. (2008). The yin and yang of the Cdkn2a locus in senescence and aging. Cell Cycle 7, 2795–2802. doi: 10.4161/cc.7.186687
Barnhoorn, S., Uittenboogaard, L. M., Jaarsma, D., Vermeij, W. P., Tresini, M., Weymaere, M., et al. (2014). Cell-autonomous progeroid changes in conditional mouse models for repair endonuclease XPG deficiency. PloS Genet. 10, 7–9. doi: 10.1371/journal.pgen.1004686
Behrens, A., van Deursen, J. M., Rudolph, K. L., Schumacher, B. (2014). Impact of genomic damage and ageing on stem cell function. Nat. Cell Biol. 16, 201–207. doi: 10.1038/ncb2928
Bendtsen, K. M., Juul, J., Trusina, A. (2012). Fragile DNA repair mechanism reduces ageing in multicellular model. PloS One 7, e36018. doi: 10.1371/journal.pone.0036018
Beneke, S., Bürkle, A. (2007). Survey and summary: poly(ADP-ribosyl)ation in mammalian ageing. Nucleic Acids Res. 35, 7456–7465. doi: 10.1093/nar/gkm735
Berman, A. E., Leontieva, O. V., Natarajan, V., McCubrey, J. A., Demidenko, Z. N., Nikiforov, M. A. (2012). Recent progress in genetics of aging, senescence and longevity: focusing on cancer-related genes. Oncotarget 3, 1522–1532. doi: 10.18632/oncotarget.889
Blackburn, E. H., Epel, E. S., Lin, J. (2015). Human telomere biology: a contributory and interactive factor in aging, disease risks, and protection. Sci. (Eighty-. ). 350, 1193–1198. doi: 10.1126/science.aab3389
Blasco, M. A. (2007). Telomere length, stem cells and aging. Nat. Chem. Biol. 3, 640–649. doi: 10.1038/nchembio.2007.38
Bogliolo, M., Schuster, B., Stoepker, C., Derkunt, B., Su, Y., Raams, A., et al. (2013). Mutations in ERCC4, encoding the DNA-repair endonuclease XPF, cause Fanconi anemia. Am. J. Hum. Genet. 92, 800–806. doi: 10.1016/j.ajhg.2013.04.002
Boonen, S., Marin, F., Mellstrom, D., Xie, L., Desaiah, D., Krege, J. H., et al. (2006). Safety and efficacy of teriparatide in elderly women with established osteoporosis: bone anabolic therapy from a geriatric perspective. J. Am. Geriatr. Soc. 54, 782–789. doi: 10.1111/j.1532-5415.2006.00695.x
Boonen, S., Black, D. M., Colón-Emeric, C. S., Eastell, R., Magaziner, J. S., Eriksen, E. F., et al. (2010). Efficacy and safety of a once-yearly intravenous zoledronic acid 5 mg for fracture prevention in elderly postmenopausal women with osteoporosis aged 75 and older. J. Am. Geriatr. Soc. 58, 292–299. doi: 10.1111/j.1532-5415.2009.02673.x
Broer, L., Buchman, A. S., Deelen, J., Evans, D. S., Faul, J. D., Lunetta, K. L., et al. (2015). GWAS of longevity in CHARGE consortium confirms APOE and FOXO3 candidacy. J. Gerontol. - Ser. A. Biol. Sci. Med. Sci. 70, 110–118. doi: 10.1093/gerona/glu166
Brooks-Wilson, A. R. (2013). Genetics of healthy aging and longevity. Hum. Genet. 132, 1323–1338. doi: 10.1007/s00439-013-1342-z
Bürkle, A., Grube, K., Küpper, J. H. (1992). Poly(ADP-ribosyl)ation: its role in inducible DNA amplification, and its correlation with the longevity of mammalian species. Exp. Clin. Immunogenet. 9, 230–240.
Cabelof, D. C., Raffoul, J. J., Yanamadala, S., Ganir, C., Guo, Z., Heydari, A. R. (2002). Attenuation of DNA polymerase beta-dependent base excision repair and increased DMS-induced mutagenicity in aged mice. Mutat. Res. 500, 135–145. doi: 10.1016/s0027-5107(02)00003-9
Chadwick, L. H. (2012). The NIH roadmap epigenomics program data resource. Epigenomics 4, 317–324. doi: 10.2217/epi.12.18
Conneely, K. N., Capell, B. C., Erdos, M. R., Sebastiani, P., Solovieff, N., Swift, A. J., et al. (2012). Human longevity and common variations in the LMNA gene: a meta-analysis. Aging Cell 11, 475–481. doi: 10.1111/j.1474-9726.2012.00808.x
Coppede, F., Migliore, L. (2012). DNA repair in premature aging disorders and neurodegeneration. Curr. Aging Sci. 3, 3–19. doi: 10.2174/1874612811003010003
Csiszar, A., Podlutsky, A., Wolin, M. S., Losonczy, G., Pacher, P., Ungvari, Z. (2009). Oxidative stress and accelerated vascular aging: implications for cigarette smoking. Front. Biosci. (Landmark Ed. 14, 3128–3144. doi: 10.2741/3440
Czarny, P., Kwiatkowski, D., Toma, M., Kubiak, J., Sliwinska, A., Talarowska, M., et al. (2017). Impact of single nucleotide polymorphisms of base excision repair genes on DNA damage and efficiency of DNA repair in recurrent depression disorder. Mol. Neurobiol. 54, 4150–4159. doi: 10.1007/s12035-016-9971-6
Day, K., Song, J., Absher, D. (2014). Targeted sequencing of large genomic regions with CATCH-Seq. PloS One 9, 111756. doi: 10.1371/journal.pone.0111756
de Renty, C., Ellis, N. A. (2016). Bloom's syndrome: why not premature aging?: a comparison of the BLM and WRN helicases. Ageing Res. Rev. 33, 36–51. doi: 10.1016/j.arr.2016.05.010
Deelen, J., Beekman, M., Uh, H. W., Broer, L., Ayers, K. L., Tan, Q., et al. (2014). Genome-wide association meta-analysis of human longevity identifies a novel locus conferring survival beyond 90 years of age. Hum. Mol. Genet. 23, 4420–4432. doi: 10.1093/hmg/ddu139
Dimitriadou, A. E., Hornik, K., Leisch, F., Meyer, D., Weingessel, A., Friedrichleischcituwienacat, M. F. L. (2005). The e1071 Package, Misc Functions of Department of Statistics. Available at: https://cran.r-project.org/web/packages/e1071/index.html.
Ding, S., Yu, J.-C., Chen, S.-T., Hsu, G.-C., Shen, C.-Y. (2007). Genetic variation in the premature aging gene WRN: a case-control study on breast cancer susceptibility. Cancer Epidemiol. Biomarkers Prev. 16, 263–269. doi: 10.1158/1055-9965.EPI-06-0678
ENCODE Project Consortium. (2012). An integrated encyclopedia of DNA elements in the human genome. Nature 489, 57–74. doi: 10.1038/nature11247
Erikson, G. A., Bodian, D. L., Rueda, M., Niederhuber, J. E., Topol, E. J., Torkamani, A., et al. (2016). Whole-genome sequencing of a healthy aging resource whole-genome sequencing of a healthy aging cohort. Cell 165, 1002–1011. doi: 10.1016/j.cell.2016.03.022
Estus, S., Shaw, B. C., Devanney, N., Katsumata, Y., Press, E. E., Fardo, D. W. (2019). Evaluation of CD33 as a genetic risk factor for Alzheimer's disease. Acta Neuropathol. 138 (2), 189–199. doi: 10.1007/s00401-019-02000-4
Fabrizio, P., Pletcher, S. D., Minois, N., Vaupel, J. W., Longo, V. D. (2004). Chronological aging-independent replicative life span regulation by Msn2/Msn4 and Sod2 in Saccharomyces cerevisiae. FEBS Lett. 557, 136–142. doi: 10.1016/S0014-5793(03)01462-5
Furey, T. S., Cristianini, N., Duffy, N., David, W. (2000). Support vector machine classification and validation of cancer tissue samples using microarray expression data. Bioinformatics 16, 906–914.
Gamazon, E. R., Zhang, W., Konkashbaev, A., Duan, S., Kistner, E. O., Nicolae, D. L., et al. (2010). SCAN: SNP and copy number annotation Bioinformatics 26, 259–262. doi: 10.1093/bioinformatics/btp644
Gensler, H. L., Bernstein, H. (1981). DNA damage as the primary cause of aging. Q. Rev. Biol. 56, 279–303. doi: 10.1086/412317
Ghosh, S., Zhou, Z. (2014). Genetics of aging, progeria and lamin disorders. Curr. Opin. Genet. Dev. 26, 41–46. doi: 10.1016/j.gde.2014.05.003
Grabowska, W., Sikora, E., Bielak-Zmijewska, A. (2017). Sirtuins, a promising target in slowing down the ageing process. Biogerontology 18, 447–476. doi: 10.1007/s10522-017-9685-9
Griciuc, A., Serrano-Pozo, A., Parrado, A. R., Lesinski, A. N., Asselin, C. N., Mullin, K., et al. (2013). Alzheimer's disease risk gene CD33 inhibits microglial uptake of amyloid beta. Neuron 78, 631–643. doi: 10.1016/j.neuron.2013.04.014
Gu, B.-W., Fan, J.-M., Bessler, M., Mason, P. J. (2011). Accelerated hematopoietic stem cell aging in a mouse model of dyskeratosis congenita responds to antioxidant treatment. Aging Cell 10, 338–348. doi: 10.1111/j.1474-9726.2011.00674.x
Hart, R. W., Setlow, R. B. (1974). Correlation between deoxyribonucleic acid excision-repair and life-span in a number of mammalian species. Proc. Natl. Acad. Sci. U.S.A. 71, 2169–2173. doi: 10.1073/pnas.71.62169
Hastie, T., Tibshirani, R., Narasimhan, B., Gilbert, C. (2001). Impute: imputation for microarray data. Bioinformatics 17, 520–525. doi: 10.18129/B9.bioc.impute
Herskind, A. M., McGue, M., Holm, N. V., Sorensen, T. I. A., Harvald, B., Vaupel, J. W. (1996). The heritability of human longevity: a population-based study of 2872 Danish twin pairs born 1870-1900. Hum. Genet. 97, 319–323. doi: 10.1007/bf02185763
Hiona, A., Leeuwenburgh, C. (2008). The role of mitochondrial DNA mutations in aging and sarcopenia. Exp. Gerontol. 43, 24–33. doi: 10.1016/j.exger.2007.10.001
Hoeijmakers, J. H. J. (2009). DNA damage, aging, and cancer. N. Engl. J. Med. 361, 1475–1485. doi: 10.1056/NEJMra0804615
Horikoshi, M., Yaghootkar, H., Mook-Kanamori, D., Sovio, U., Taal, H., Hennig, B., et al. (2013). New loci associated with birth weight identify genetic links between intrauterine growth and adult height and metabolism. Nat. Genet. 45, 76. doi: 10.2337/db13-dd08
Jorgensen, T. J., Helzlsouer, K. J., Clipp, S. C., Bolton, J. H., Crum, R. M., Visvanathan, K. (2009). DNA repair gene variants associated with benign breast disease in high cancer risk women. Cancer Epidemiol. Biomarkers Prev. 18, 346–350. doi: 10.1158/1055-9965.EPI-08-0659
Köttgen, A., Pattaro, C., Böger, C. A., Fuchsberger, C., Olden, M., Glazer, N. L., et al. (2010). Multiple loci associated with indices of renal function and chronic kidney disease. Nat. Genet. 42, 376–384. doi: 10.1038/ng.568.Multiple
Karow, J. K., Wu, L., Hickson, I. D. (2000). RecQ family helicases: roles in cancer and aging. Curr. Opin. Genet. Dev. 10, 32–38. doi: 10.1016/S0959-437X(99)00039-8
Katyal, S., McKinnon, P. J. (2008). DNA strand breaks, neurodegeneration and aging in the brain. Mech. Ageing Dev. 129, 483–491. doi: 10.1016/j.mad.2008.03.008
Kawahara, T. L. A., Rapicavoli, N. A., Wu, A. R., Qu, K., Quake, S. R., Chang, H. Y. (2011). Dynamic chromatin localization of sirt6 shapes stress- and aging-related transcriptional networks. PloS Genet. 7, 1–12. doi: 10.1371/journal.pgen.1002153
Keane, M., Semeiks, J., Webb, A. E., Li, Y. I., Quesada, V., Craig, T., et al. (2015). Insights into the evolution of longevity from the bowhead whale genome. Cell Rep. 10, 112–122. doi: 10.1016/j.celrep.2014.12.008
Kent, W. J., Sugnet, C. W., Furey, T. S., Roskin, K. M., Pringle, T. H., Zahler, A. M., et al. (2002). The human genome browser at UCSC. Genome Res. 12, 996–1006. doi: 10.1101/gr.229102
Kim, S., Kaminker, P., Campisi, J. (1999). TIN2, a new regulator of telomere length in human cells. Nat. Genet. 23, 405–412. doi: 10.1038/70508
Krishnan, V., Liu, B., Zhou, Z. (2011). DNA repair, human diseases and aging. Intech 13, 3–46. doi: http://dx.doi.org/10.5772/57353
Kujoth, G. C., Hiona, A., Pugh, T. D., Someya, S., Panzer, K., Wohlgemuth, S. E., et al. (2005). Mitochondrial DNA mutations, oxidative stress, and apoptosis in mammalian aging. Science 309, 481–484. doi: 10.1126/science.1112125
Leslie, R., O'Donnell, C. J., Johnson, A. D. (2014). GRASP: analysis of genotype-phenotype results from 1390 genome-wide association studies and corresponding open access database. Bioinformatics 30, 185–194. doi: 10.1093/bioinformatics/btu273
Li, H., Durbin, R. (2009). Fast and accurate short read alignment with Burrows-Wheeler transform. Bioinformatics 25, 1754–1760. doi: 10.1093/bioinformatics/btp324
Liaw, A., Wiener, M. (2002). Classification and Regression by randomForest. Newsl. R. Proj. 2 (3), 18–22.
Lonsdale, J., Thomas, J., Salvatore, M., Phillips, R., Lo, E., Shad, S., et al. (2013). The Genotype-Tissue Expression (GTEx) project. Nat. Genet. 45, 580–585. doi: 10.1038/ng2653
Lopez-Mejia, I. C., Vautrot, V., De toledo, M., Behm-ansmant, I., Bourgeois, C. F., Navarro, C. L., et al. (2011). A conserved splicing mechanism of the LMNA gene controls premature aging. Hum. Mol. Genet. 20, 4540–4555. doi: 10.1093/hmg/ddr385
Lunetta, K. L., Hayward, L. B., Segal, J., Van Eerdewegh, P. (2004). Screening large-scale association study data: exploiting interactions using random forests. BMC Genet. 5, 32. doi: 10.1186/1471-2156-5-32
Ma, S., Gladyshev, V. N. (2017). Molecular signatures of longevity: insights from cross-species comparative studies. Semin. Cell Dev. Biol. 70, 190–203. doi: 10.1016/j.semcdb.2017.08.007
Macrae, S. L., Zhang, Q., Lemetre, C., Seim, I., Calder, R. B., Hoeijmakers, J., et al. (2015). Comparative analysis of genome maintenance genes in naked mole rat, mouse, and human. Aging Cell 14, 288–291. doi: 10.1111/acel.12314
Mao, Z., Hine, C., TIan, X., Van Meter, M., Au, M., Vaidya, A., et al. (2011). SIRT6 promotes DNA repair under stress by activating PARP1 Zhiyong. Sci. (Eighty-. ). 332, 1443–1446. doi: 10.1126/science.1202723
Martinez, P., Blasco, M. A. (2010). Role of shelterin in cancer and aging. Aging Cell 9, 653–666. doi: 10.1111/j.1474-9726.2010.00596.x
Maynard, S., Fang, E. F., Scheibye-Knudsen, M., Croteau, D. L., Bohr, V. A. (2015). DNA damage, DNA repair, aging, and neurodegeneration. Cold Spring Harb. Perspect. Med. 5, a025130. doi: 10.1101/cshperspect.a025130
McClung, M. R., Boonen, S., Törring, O., Roux, C., Rizzoli, R., Bone, H. G., et al. (2012). Effect of denosumab treatment on the risk of fractures in subgroups of women with postmenopausal osteoporosis. J. Bone Miner. Res. 27, 211–218. doi: 10.1002/jbmr.536
McKenna, A., Hanna, M., Banks, E., Sivachenko, A., Cibulksis, K., Kernytsky, A., et al. (2010). The genome analysis toolkit: a MapReduce framework for analyzing next-generation DNA sequencing data. Genome Res. 20, 1297–1303. doi: 10.1101/gr.107524.110.20
Mohaghegh, P., Hickson, I. D. (2002). Premature aging in RecQ helicase-deficient human syndromes. Int. J. Biochem. Cell Biol. 34, 1496–1501. doi: 10.1016/S1357-2725(02)00039-0
Moskalev, A. A., Aliper, A. M., Smit-McBride, Z., Buzdin, A., Zhavoronkov, A. (2014). Genetics and epigenetics of aging and longevity. Cell Cycle 13, 1063–1077. doi: 10.4161/cc.28433
Muñoz, P., Blanco, R., Flores, J. M., Blasco, M. A. (2005). XPF nuclease-dependent telomere loss and increased DNA damage in mice overexpressing TRF2 result in premature aging and cancer. Nat. Genet. 37, 1063–1071. doi: 10.1038/ng1633
Muiras, M. L., Müller, M., Schächter, F., Bürkle, A. (1998). Increased poly(ADP-ribose) polymerase activity in lymphoblastoid cell lines from centenarians. J. Mol. Med. (Berl). 76, 346–354. doi: 10.1007/s001090050226
Multani, A. S., Chang, S. (2007). WRN at telomeres: implications for aging and cancer. J. Cell Sci. 120, 713–721. doi: 10.1242/jcs.03397
Newman, A. B., Walter, S., Lunetta, K. L., Garcia, M. E., Slagboom, P. E., Christensen, K., et al. (2010). A meta-analysis of four genome-wide association studies of survival to age 90 years or older: the cohorts for heart and aging research in genomic epidemiology consortium. J. Gerontol. - Ser. A. Biol. Sci. Med. Sci. 65 A, 478–487. doi: 10.1093/gerona/glq028
Ng, P. C., Henikoff, S. (2003). SIFT: predicting amino acid changes that affect protein function. Nucleic Acids Res. 31, 3812–3814. doi: 10.1093/nar/gkg509
Nho, K., Corneveaux, J. J., Kim, S., Lin, H., Risacher, S. L., Shen, L., et al. (2013). Whole-exome sequencing and imaging genetics identify functional variants for rate of change in hippocampal volume in mild cognitive impairment. Mol. Psychiatry 18, 781–787. doi: 10.1038/mp.2013.24
Niedernhofer, L. J., Garinis, G. A., Raams, A., Lalai, A. S., Robinson, A. R., Appeldoorn, E., et al. (2006). A new progeroid syndrome reveals that genotoxic stress suppresses the somatotroph axis. Nature 444, 1038–1043. doi: 10.1038/nature05456
Niedernhofer, L. J., Gurkar, A. U., Wang, Y., Vijg, J., Hoeijmakers, J. H. J., Robbins, P. D. (2018). Nuclear genomic instability and aging. Annu. Rev. Biochem. 87, 295–322. doi: 10.1146/annurev-biochem-062917-012239
Noren Hooten, N., Fitzpatrick, M., Kompaniez, K., Jacob, K. D., Moore, B. R., Nagle, J., et al. (2012). Coordination of DNA repair by NEIL1 and PARP-1: A possible link to aging. Aging (Albany. NY). 4, 674–685. doi: 10.18632/aging.100492
O'Donovan, A., Davies, A. A., Moggs, J. G., West, S. C., Wood, R. D. (1994). XPG endonuclease makes the 3′ Incision in human DNA nucleotide excision repair. Nature 371, 432–435. doi: 10.1038/371432a0
Patel, M. N. (2002). Oxidative stress, mitochondrial dysfunction, and epilepsy. Free Radic. Res. 36, 1139–1146. doi: 10.1080/1071576021000016391
Perera, S., Studenski, S., Newman, A., Simonsick, E., Harris, T., Schwartz, A., et al. (2014). Are estimates of meaningful decline in mobility performance consistent among clinically important subgroups? (Health ABC study). J. Gerontol. - Ser. A. Biol. Sci. Med. Sci. 69, 1260–1268. doi: 10.1093/gerona/glu033
Perera, S., Patel, K. V., Rosano, C., Rubin, S. M., Satterfield, S., Harris, T., et al. (2016). Gait speed predicts incident disability: a pooled analysis. J. Gerontol. Ser. A. Biol. Sci. Med. Sci. 71, 63–71. doi: 10.1093/gerona/glv126
Perlis, R. H., Huang, J., Purcell, S., Fava, M., Rush, A. J., Sullivan, P. F., et al. (2010). Genome-wide association study of suicide attempts in mood disorder patients. Am. J. Psychiatry 167, 1499–1507. doi: 10.1176/appi.ajp.2010.10040541
Perls, T. T., Wilmoth, J., Levenson, R., Drinkwater, M., Cohen, M., Bogan, H., et al. (2002). Life-long sustained mortality advantage of siblings of centenarians. Proc. Natl. Acad. Sci. U. S. A. 99, 8442–8447. doi: 10.1073/pnas.122587599
Pilling, L. C., Atkins, J. L., Bowman, K., Jones, S. E., Tyrrell, J., Beaumont, R. N., et al. (2016). Human longevity is influenced by many genetic variants: evidence from 75,000 UK Biobank participants. Aging (Albany. NY). 8, 1–24. doi: 10.1101/038430
Pilling, L. C., Kuo, C. L., Sicinski, K., Tamosauskaite, J., Kuchel, G. A., Harries, L. W., et al. (2017). Human longevity: 25 genetic loci associated in 389,166 UK biobank participants. Aging (Albany. NY). 9, 2504–2520. doi: 10.18632/aging.101334
Piskunova, T. S., Yurova, M. N., Ovsyannikov, A. I., Semenchenko, A. V., Zabezhinski, M. A., Popovich, I. G., et al. (2008). Deficiency in Poly(ADP-ribose) Polymerase-1 (PARP-1) accelerates aging and spontaneous carcinogenesis in mice. Curr. Gerontol. Geriatr. Res. 2008, 1–11. doi: 10.1155/2008/754190
Qiu, X., Brown, K., Hirschey, M. D., Verdin, E., Chen, D. (2010). Calorie restriction reduces oxidative stress by SIRT3-mediated SOD2 activation. Cell Metab. 12, 662–667. doi: 10.1016/j.cmet.2010.11.015
Reed, T., Dick, D. M., Uniacke, S. K., Foroud, T., Nichols, W. C. (2004). Genome-wide scan for a healthy aging phenotype provides support for a locus near D4S1564 promoting healthy aging. J. Gerontol. A. Biol. Sci. Med. Sci. 59, 227–232. doi: 10.1093/gerona/59.3.b227
Rigler, S. K., Studenski, S., Wallace, D., Reker, D. M., Duncan, P. W. (2002). Co-morbidity adjustment for functional outcomes in community-dwelling older adults. Clin. Rehabil. 16, 420–428. doi: 10.1191/0269215502cr515oa
Rodriguez, S., Coppedè, F., Sagelius, H., Eriksson, M. (2009). Increased expression of the Hutchinson-Gilford progeria syndrome truncated lamin A transcript during cell aging. Eur. J. Hum. Genet. 17, 928–937. doi: 10.1038/ejhg.2008.270
Rubelj, I., Vondraček, Z. (1999). Stochastic mechanism of cellular aging - Abrupt telomere shortening as a model for stochastic nature of cellular aging. J. Theor. Biol. 197, 425–438. doi: 10.1006/jtbi.19980886
Sangha, O., Stucki, G., Liang, M. H., Fossel, A. H., Katz, J. N. (2003). The self-administered comorbidity questionnaire: a new method to assess comorbidity for clinical and health services research. Arthritis Rheumatol. 49, 156–163. doi: 10.1002/art.10993
Satoh, A., Brace, C. S., Rensing, N., Cliften, P., Wozniak, D. F., Herzog, E. D., et al. (2013). Sirt1 extends life span and delays aging in mice through the regulation of Nk2 Homeobox 1 in the DMH and LH. Cell Metab. 18, 416–430. doi: 10.1016/j.cmet.2013.07.013
Savage, S. A., Giri, N., Baerlocher, G. M., Orr, N., Lansdorp, P. M., Alter, B. P. (2008). TINF2, a component of the shelterin telomere protection complex, is mutated in dyskeratosis congenita. Am. J. Hum. Genet. 82, 501–509. doi: 10.1016/j.ajhg.2007.10.004
Schumacher, B., Garinis, G. A., Hoeijmakers, J. H. J. (2008). Age to survive: DNA damage and aging. Trends Genet. 24, 77–85. doi: 10.1016/j.tig.2007.11.004
Sebastiani, P., Solovieff, N., Dewan, A. T., Walsh, K. M., Puca, A., Hartley, S. W., et al. (2012). Genetic signatures of exceptional longevity in humans. PloS One 7, e29848. doi: 10.1371/journal.pone.0029848
Sebastiani, P., Bae, H., Sun, F. X., Andersen, S. L., Daw, E. W., Malovini, A., et al. (2013). Meta-analysis of genetic variants associated with human exceptional longevity. Aging (Albany. NY). 5, 653–661. doi: 10.18632/aging.100594
Serrano, M., López-Otín, C., Blasco, M. A., Kroemer, G., Partridge, L. (2013). The hallmarks of aging. Cell 153, 1194–1217. doi: 10.1016/j.cell.2013.05.039
Shiels, P. G. (2010). Improving precision in investigating aging: why telomeres can cause problems. J. Gerontol. - Ser. A. Biol. Sci. Med. Sci. 65 A, 789–791. doi: 10.1093/gerona/glq095
Soria-Valles, C., López-Otín, C. (2016). iPSCs: on the road to reprogramming aging. Trends Mol. Med. 22, 713–724. doi: 10.1016/j.molmed.2016.05.010
Soto, I., Graham, L. C., Richter, H. J., Simeone, S. N., Radell, J. E., Grabowska, W., et al. (2015). APOE stabilization by exercise prevents aging neurovascular dysfunction and complement induction. PloS Biol. 13, e1002279. doi: 10.1371/journal.pbio.1002279
Stephan, J., Stegle, O., Beyer, A. (2015). A random forest approach to capture genetic effects in the presence of population structure. Nat. Commun. 6, 1–10. doi: 10.1038/ncomms8432
Stewart, A. L., Mills, K. M., King, A. C., Haskell, W. L., Gillis, D., Ritter, P. L. (2001). CHAMPS physical activity questionnaire for older adults: outcomes for interventions. Med. Sci. Sports Exerc. 33, 1126–1141. doi: 10.1097/00005768-200107000-00010
Strosznajder, J. B., Jęśko, H., Strosznajder, R. P. (2000). Effect of amyloid beta peptide on poly(ADP-ribose) polymerase activity in adult and aged rat hippocampus. Acta Biochim. Pol. 47, 847–854.
Tian, X., Seluanov, A., Gorbunova, V. (2017). Molecular mechanisms determining lifespan in short- and long-lived species. Trends Endocrinol. Metab. 28, 722–734. doi: 10.1016/j.tem.2017.07.004
Tian, X., Firsanov, D., Zhang, Z., Cheng, Y., Luo, L., Tombline, G., et al. (2019). SIRT6 is responsible for more efficient DNA double-strand break repair in long-lived species. Cell 177, 622–638.e22. doi: 10.1016/j.cell.2019.03.043
Trifunovic, A., Wredenberg, A., Falkenberg, M., Spelbrink, J. N., Rovio, A. T., Bruder, C. E., et al. (2004). Premature ageing in mice expressing defective mitochondrial DNA polymerase. Nature 429, 417–423. doi: 10.1038/nature02517
Tuo, J., Ning, B., Bojanowski, C. M., Lin, Z.-N., Ross, R. J., Reed, G. F., et al. (2006). Synergic effect of polymorphisms in ERCC6 5' flanking region and complement factor H on age-related macular degeneration predisposition. Proc. Natl. Acad. Sci. U. S. A. 103, 9256–9261. doi: 10.1073/pnas.0603485103
Valdes, A. M., Andrew, T., Gardner, J. P., Kimura, M., Oelsner, E., Cherkas, L. F., et al. (2005). Obesity, cigarette smoking, and telomere length in women. Lancet 366, 662–664. doi: 10.1016/S0140-6736(05)66630-5
van den Berg, N., Rodríguez-Girondo, M., de Craen, A. J. M., Houwing-Duistermaat, J. J., Beekman, M., Slagboom, P. E. (2018). Longevity around the turn of the 20th century: life-long sustained survival advantage for parents of today's nonagenarians. J. Gerontol. Ser. A. 73, 1295–1302. doi: 10.1093/gerona/gly049
van den Berg, N., Rodríguez-Girondo, M., van Dijk, I. K., Mourits, R. J., Mandemakers, K., Janssens, A. A. P. O., et al. (2019). Longevity defined as top 10% survivors and beyond is transmitted as a quantitative genetic trait. Nat. Commun. 10, 35. doi: 10.1038/s41467-018-07925-0
Vasunilashorn, S., Coppin, A. K., Patel, K. V., Lauretani, F., Ferrucci, L., Bandinelli, S., et al. (2009). Use of the short physical performance battery score to predict loss of ability to walk 400 meters: analysis from the InCHIANTI study. J. Gerontol. - Ser. A. Biol. Sci. Med. Sci. 64, 223–229. doi: 10.1093/gerona/gln022
Velarde, M. C., Flynn, J. M., Day, N. U., Melov, S., Campisi, J. (2012). Mitochondrial oxidative stress caused by Sod2 deficiency promotes cellular senescence and aging phenotypes in the skin. Aging (Albany. NY). 4, 3–12. doi: 10.18632/aging.100423
Vermeij, W. P., Hoeijmakers, J. H. J., Pothof, J. (2016). Genome integrity in aging: human syndromes, mouse models, and therapeutic options. Annu. Rev. Pharmacol. Toxicol. 56, 427–445. doi: 10.1146/annurev-pharmtox-010814-124316
Walter, S., Atzmon, G., Demerath, E. W., Franceschini, N. (2011). A genome-wide association study of aging. Neurobiol. Aging 32, 1–21. doi: 10.1016/j.neurobiolaging.2011.05.026.A
Ware, J. E., Sherbourne, C. D. (1992). The MOS 36-item short-form healthy survey (SF-36). Med. Care 30, 473–483.
Xu, B. N., Tian, Y., Liu, S. Y., Zhao, X. T., Wang, Q., Tian, D. L., et al. (2016). The relationship between XPG rs17655 polymorphism and the risk of lung cancer. Int. J. Clin. Exp. Med. 9, 4620–4624.
Yuan, Q., Liu, J.-W., Xing, C.-Z., Yuan, Y. (2014). Associations of ERCC4 rs1800067 polymorphism with cancer risk: an updated meta-analysis. Asian Pacific J. Cancer Prev. 15, 7639–7644. doi: 10.7314/APJCP.2014.15.187639
Yuan, Y., Cruzat, V. F., Newshome, P., Cheng, J., Chen, Y., Lu, Y. (2016). Regulation of SIRT1 in aging: roles in mitochondrial function and biogenesis. Mech. Ageing Dev. 155, 10–21. doi: 10.1016/j.mad.2016.02.003
Keywords: machine learning, aging, genetics, bioinformatics, sequencing
Citation: Breitbach ME, Greenspan S, Resnick NM, Perera S, Gurkar AU, Absher D and Levine AS (2019) Exonic Variants in Aging-Related Genes Are Predictive of Phenotypic Aging Status. Front. Genet. 10:1277. doi: 10.3389/fgene.2019.01277
Received: 21 March 2019; Accepted: 19 November 2019;
Published: 19 December 2019.
Edited by:
Alexey Moskalev, Komi Scientific Center (RAS), RussiaReviewed by:
Jing Dong, Baylor College of Medicine, United StatesSvetlana Ukraintseva, Duke University, United States
Copyright © 2019 Breitbach, Greenspan, Resnick, Perera, Gurkar, Absher and Levine. This is an open-access article distributed under the terms of the Creative Commons Attribution License (CC BY). The use, distribution or reproduction in other forums is permitted, provided the original author(s) and the copyright owner(s) are credited and that the original publication in this journal is cited, in accordance with accepted academic practice. No use, distribution or reproduction is permitted which does not comply with these terms.
*Correspondence: Devin Absher, ZGFic2hlckBodWRzb25hbHBoYS5vcmc=
†These authors share senior authorship