- 1International Crops Research Institute for the Semi-Arid Tropics (ICRISAT), Hyderabad, India
- 2All India Coordinated Research Project on Pearl Millet (AICRP-PM), Indian Council of Agricultural Research (ICAR), Jodhpur, India
- 3Institute of Biological, Environmental & Rural Sciences (IBERS), Aberystwyth University, Gogerddan, United Kingdom
Pearl millet is a climate-resilient, drought-tolerant crop capable of growing in marginal environments of arid and semi-arid regions globally. Pearl millet is a staple food for more than 90 million people living in poverty and can address the triple burden of malnutrition substantially. It remained a neglected crop until the turn of the 21st century, and much emphasis has been placed since then on the development of various genetic and genomic resources for whole-genome scan studies, such as the genome-wide association studies (GWAS) and genomic selection (GS). This was facilitated by the advent of sequencing-based genotyping, such as genotyping-by-sequencing (GBS), RAD-sequencing, and whole-genome re-sequencing (WGRS) in pearl millet. To carry out GWAS and GS, a world association mapping panel called the Pearl Millet inbred Germplasm Association Panel (PMiGAP) was developed at ICRISAT in partnership with Aberystwyth University. This panel consisted of germplasm lines, landraces, and breeding lines from 27 countries and was re-sequenced using the WGRS approach. It has a repository of circa 29 million genome-wide SNPs. PMiGAP has been used to map traits related to drought tolerance, grain Fe and Zn content, nitrogen use efficiency, components of endosperm starch, grain yield, etc. Genomic selection in pearl millet was jump-started recently by WGRS, RAD, and tGBS (tunable genotyping-by-sequencing) approaches for the PMiGAP and hybrid parental lines. Using multi-environment phenotyping of various training populations, initial attempts have been made to develop genomic selection models. This mini review discusses advances and prospects in GWAS and GS for pearl millet.
Introduction
Pearl millet (Pennisetum glaucum (L) R. Br., syn. Cenchrus americanus (L.) Morrone) is an important C4 small-grained field crop of traditional smallholder farming systems that belongs to the grass family Poaceae and subfamily Panicoideae. An archaeological survey indicates that pearl millet was initially domesticated at the southern edge of the Sahara Desert in West Africa about 2500 BC (Manning et al., 2011). Pearl millet is a diploid (2n = 2x = 14), cross-pollinated warm-season crop with tremendous photosynthetic potential and high biomass production capacity. It is highly tillering, polymorphic, has a short life cycle, a large genome size (1.76 Gb), and an outbreeding nature (Bennett et al., 2000, Varshney et al., 2017). Climate-adaptive phenotypic, physiological, and reproductive attributes of pearl millet make this crop well-suited to grow in marginal conditions, such as poor soil fertility, limited soil water content, high salinity, extreme soil pH ranges, high soil Al3+ saturation, high temperatures, and scant rainfall. Pearl millet can thrive and produce a substantial amount of grain in drought-prone areas that receiving average annual precipitation <250 mm, whereas other cereal crops, such as maize, rice, sorghum, bread wheat, and barley, are likely to fail to give economic returns (Nambiar et al., 2011). Pearl millet is cultivated over ~27 million hectares in arid and semi-arid areas of Asia and Sub-Saharan Africa and is the primary food source for about 90 million resource-poor populations residing in marginal areas globally. Remarkably, the natural attribute of this crop to withstand ambient temperatures up to 42°C at the reproductive phase makes it suited for growth via irrigation in the extremely hot summers in north-western parts of India (Gupta et al., 2015).
Pearl millet has several nutritional properties compared to other staple cereal grains, and it is an excellent source of organic as well as inorganic nutrients and a cost-effective source of energy (Kumar et al., 2016). Pearl millet grains are rich in fibers (1.2 g/100 g), α-amylose, amino acids, proteins (8–19%), and low starch, mineral nutrients including phosphorus, magnesium, iron, and zinc. Owing to having such nutritional values, pearl millet ensures food and nutritional security for farmers living in poverty (Nambiar et al., 2011, Kanatti et al., 2014). Pearl millet is a rich source of several polyphenols, and other biologically important ingredients make it suited to play a role in reducing the rate of fat absorption, the lowering of glycemic indices, as well as in overcoming the risk of cardiac diseases, diabetes, and other medical problems. Overall, pearl millet has the capacity to combat micronutrient deficiency across developing countries (Rai et al., 2012) since it contributes 30–40% of inorganic nutrients and provides affordable staple food with an adequate level of iron and zinc in its cultivating areas (Rao et al., 2006).
An alternative approach to the QTL mapping is the genome-wide association study (GWAS) or association mapping (AM) approach (Gómez et al., 2011) based on the principle of a linkage disequilibrium (LD) to detect a substantial association between DNA marker and target trait (Gupta et al., 2005). Genetic linkage is found through extensive genotyping of a panel of germplasm or breeding populations showing contrasting phenotypes across variable environments. It has an immense power in identifying specific genes controlling the expression of the desired traits (Kraakman et al., 2004). The potential advantage of association mapping is the likelihood of a superior resolution mapping utilizing mass recombination events from numerous meiotic events throughout the germplasm evolutionary history. It has the power to evaluate and characterize several alleles concurrently in diploid (Zhao et al., 2007) as well as in polyploid crops (Breseghello and Sorrells, 2006). Association mapping offers many benefits over linkage mapping since it provides better mapping resolution due to historical mutations and recombinations in genetic lineages, which leads to the identification of markers in the vicinity of governing genes (Liu et al., 2016). Genetic polymorphisms having strong linkage with a genomic locus leading to phenotypic differences is expected to be substantially associated with a target trait across the panel of germplasm.
The analysis of QTL effects for minor QTLs using linkage mapping and genome-wide association mapping is often biased. Therefore, scientific groups have for years been trying to solve the issue of how to tackle these complex traits and outcomes in terms of genomic selection (GS). Genomic selection is a breeding approach exploiting high-density DNA markers distributed across the genome to facilitate the rapid selection of the best candidates and offers opportunities to enhance genetic gains (Meuwissen et al., 2001). GS uses different prediction models by combining the genotyping and phenotyping datasets of the training population (TP), which is subsequently used to determine genomic-estimated breeding values (GEBVs) for every genotype of breeding population (BP) from their genotyping scores. These GEBVs permit breeders to envisage superior genotypes that would be suitable either as a parent in hybridization or for next-generation advancement of the breeding program. The basic principle is that the information derived from several markers widely distributed over the genome, having the potential to reveal genetic variations in the genome, can evaluate breeding values without prior information of where the selected genes are located (Crossa et al., 2017).
In this paper, we review the advances made in the development of genetic and genomic resources for their use in genome-wide association studies (GWAS) and genomic selection (GS) in pearl millet.
Development of Genetic Resources
Genetic resources are the fundamental materials that play a pivotal role in plant genomic and phenomic studies to boost major scientific discoveries in advanced agriculture systems. Fortunately, genetic recourses have been collected and preserved by many national and international gene banks around the world. Pearl millet accessions have been collected and conserved by 97 gene banks (66,682 accessions) globally, in which ICRISAT has the largest collection (~21,594 pearl millet accessions from 51countries) (Singh and Upadhyaya, 2016). More importantly, core and mini core collections have been developed at ICRISAT and serve as essential resources for allele mining studies for the identification of agronomic studies, and they are also used for the development of tolerant lines for both abiotic and biotic stresses. Likewise, one more genotype-based reference set has been developed, and it comprises 300 pearl millet accessions (Upadhyaya et al., 2011). At ICRISAT, most of the accessions were evaluated for several agronomical traits, and these show the extent of genetic diversity and phenotypic variance for most of the qualitative and quantitative traits (Singh and Upadhyaya 2016). It is evident that vast genetic variability is the determining factor for the identification of promising germplasm for the desired trait (Upadhyaya et al., 2007). In addition to ICRISAT, major germplasm are preserved at the Institute of Research for Development (IRD, France), in which 3,968 accessions are maintained from 16 countries, and 3,821 accessions of cultivated P. glaucum and related species maintained at the Canadian Genetic Resources (Saskatoon, Canada). Additionally, there are 1,283 active collections of pearl millet accessions collected and preserved at the US Germplasm Resource Information Network (GRIN) (Yadav et al., 2007). For conducting AM studies, diverse genetic resources are the essential inputs, and pearl millet genetic resources are found to have enormous genetic diversity. For this reason, performing AM studies for desired traits in pearl millet crops is imperative and will provide immense genomic resources for future studies. Over the last five years, significant work has been carried out on pearl millet related AM studies, and this gives information about genetic diversity and linkage disequilibrium (LD). To get over this problem, ICRISAT, in association with AU, developed a world association mapping panel called the Pearl Millet inbred Germplasm Association Panel (PMiGAP). This panel comprises 346 lines consisting of germplasm lines, landraces, and breeding lines representing global pearl millet diversity. These lines were generated by repeated rounds of selfing (S0 through S11) from 1,000 accessions representing diverse cultivars, landraces, and mapping population parents of 27 countries. Thus, PMiGAP may be considered an excellent genetic resource for GWAS studies into pearl millet crop. By the year 2015, out of 346 PMiGAP lines, Sehgal used 250 lines for AM studies and evaluated these for drought-related traits under field conditions. Similarly, during another study on AM, in which 500 pearl millet lines included 252 global accessions and 248 Senegalese landraces, they found extant genetic diversity between global and Senegalese accessions (Hu et al., 2015). In addition to the above studies, several RIL (recombinant inbred line) populations were also developed for biotic and abiotic stresses, quality, as well as yield and yield-related traits. Rajaram et al. (2013) constructed pearl millet consensus maps by using four RIL populations (ICMB 841-P3 × 863B-P2 (RIP A), H 77/833-2 × PRLT 2/89-33 (RIP B), 81B-P6 × ICMP 451-P8 (RIP C), and PT 732B-P2 × P1449-2-P1 (RIP D). In other studies, iron- and zinc-related QTLs were identified in ICMB 841-P3 × 863B-P2 (144 progenies) and ICMS 8511-S1-17-2-1-1-B-P03 × AIMP 92901-S1-183-2-2-B-08 (317 progenies) RIL populations, respectively (Kumar et al., 2016; Kumar et al., 2018). In a recent study, Chelpuri et al. (2019) identified QTLs with resistance to major pathotype isolates of the downy mildew pathogen in the pearl millet RIL population, ICMB 89111-P6 × ICMB 90111-P6 (187 progenies). Therefore, there is a good opportunity for pearl millet researchers who can access these useful genetic resources to meet their research needs.
Development of Genomic Resources and Trait Mapping
Molecular or DNA-based markers, genetic linkage maps, and genomic sequence data are important genomic resources to perform a genetic evaluation and marker-assisted breeding in any plant species. Over the last decade, several types of molecular markers, genomic tools, and genetic linkage maps have been developed and deployed in millets (Serba and Yadav, 2016). Several DNA-based molecular markers, including restriction fragment length polymorphism [RFLP; (Liu et al., 1994)], amplified fragment length polymorphism [AFLP; (Devos et al., 1995)], random amplified polymorphic DNA (RAPD), expressed sequence tags-derived simple sequence repeats [EST-SSRs; (Senthilvel et al., 2008; Rajaram et al., 2013)] markers, sequence-tagged sites [STSs; (Allouis et al., 2001)], genomic simple sequence repeat [gSSRs; (Qi et al., 2004)], DArT array Technology [DArTs; (Senthilvel et al., 2010; Supriya et al., 2011)], conserved intron specific primers [CISP; (Sehgal et al., 2012)], single-stranded conformation polymorphism-SNP [SSCP-SNP; (Bertin et al., 2005)], and single nucleotide polymorphisms [SNPs; (Sehgal et al., 2012)] have been developed and exploited in genetic diversity, QTLs/genes identification, and marker-aided breeding for faster pearl millet breeding (Table 1). Molecular markers facilitate in analyzing genetic variations existed within the germplasm collections for precise selection of breeding parents in crossing programs, estimating population structure, and identification of QTLs for stress tolerance. Pearl millet has a wide range of DNA polymorphisms even in elite inbred parental lines of popular hybrids (Vadez et al., 2012).
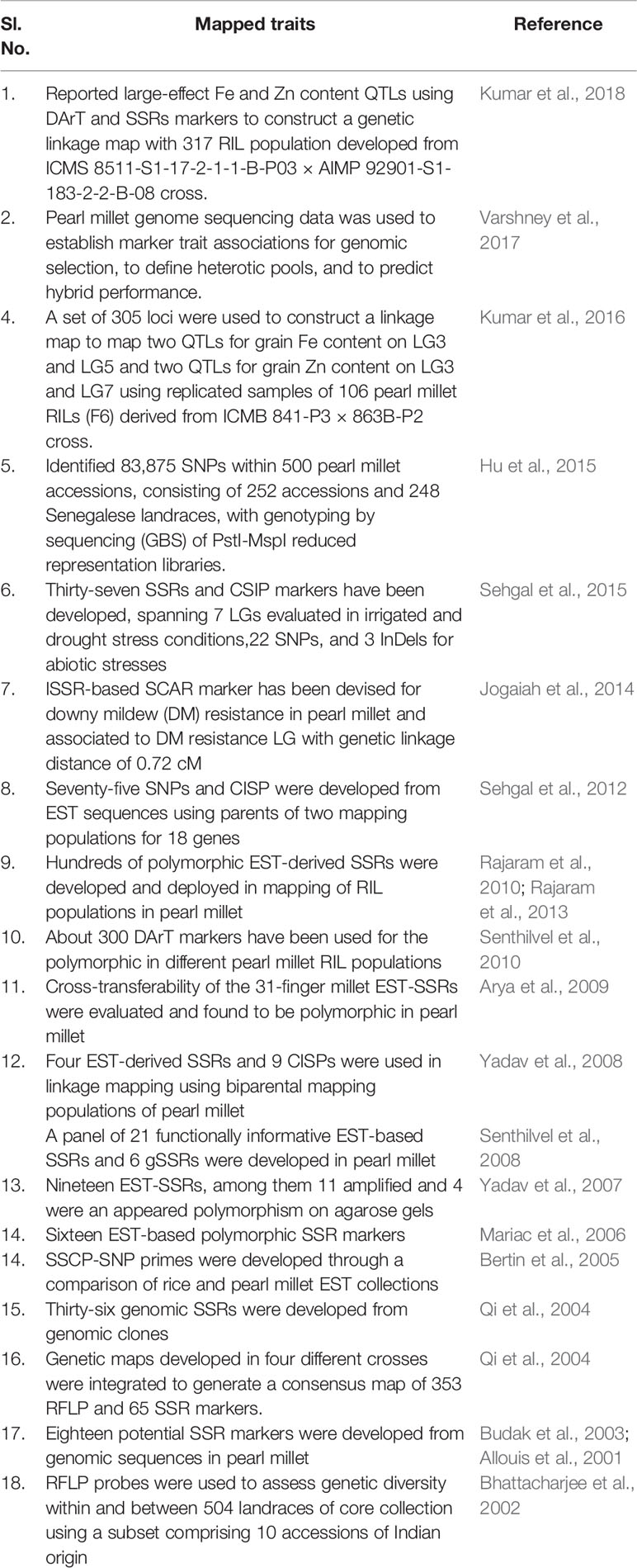
Table 1 Details of mapped traits and genomic resources developed in pearl millet, related to grain quality, yield, fodder, biomass, and biotic and abiotic stresses.
Initially, RFLP-derived DNA markers were devised and used to map about 180 loci ranged approximately 350 cM under seven linkage groups in pearl millet (Liu et al., 1992; Liu et al., 1994). Later, these markers were exploited in QTL mapping for downy mildew resistance in pearl millet (Jones et al., 1995). A subset of 21 polymorphic EST-SSRs and 6 genomic SSR markers were developed using sequence information from 3,520 expressed sequence tags (ESTs) and used in genome mapping of different pearl millet mapping populations (Senthilvel et al., 2008). Subsequently, these potentially developed EST-SSRs were deployed in marker-aided breeding for yield and drought stress resistance in pearl millet at the International Crops Research Institute for the Semi-Arid Tropics (ICRISAT). The development of a panel of 277 polymorphic DArT markers was reported from 6,900 DNA array-dart technology (DArT) clones using a PstI/BanII complexity reduction in a pearl millet RIL population (Senthilvel et al., 2010). Separately, 574 potential DArT markers were detected from 7,000 DArT clones obtained from 95 diverse genotypes using a PstI/BanII complexity reduction in genetically diverse inbred lines of pearl millet (Supriya et al., 2011). The mapping of 208 DArT markers along with 305 SSRs detected seven linkage groups covering 1,749 cM with an average intermarker distance of 5.73 cM and two co-localized QTLs for iron and zinc content on LG 3 were identified in pearl millet (Kumar et al., 2016). Using DArT markers, comparative mapping and genome organization analysis may easily be performed, and the price of marker-aided backcrossing (MABC) is also cheap relative to other markers systems.
Pearl millet EST resources were used to develop quality SNPs and CISP markers, and they were deployed to identify candidate genes related to a major QTL for drought tolerance using diverse (H 77/833-2, PRLT 2/89-33, ICMR 01029, and ICMR 01004) genotypes that represented mapping populations parents (Sehgal et al. (2012). Later, 83,875 SNP markers were identified using genotyping-by-sequencing (GBS) of PstI-MspI reduced representation libraries in pearl millet lines, represented by 252 world germplasm accessions and 248 landraces from Senegal, which revealed wide genetic variability in comparison to other germplasm collection in Africa and Asia (Hu et al., 2015). Moreover, ISSR-based sequence characterized amplified region (SCAR) markers were devised to examine genetic variations between two (ICMR 01007 and ICMR 01004) genotypes of pearl millet and a contrast mapping population for downy mildew resistance. A polymorphic locus (1.4 kb size) was found in the ICMR 01004 genotype, and further PCR amplification of these polymorphic loci was produced to be closely associated with downy mildew resistant LG with a genetic distance of 0.72 cM. An identified SCAR marker was eventually validated using diverse pearl millet genotypes belonging to Asia and Africa, and the outcomes demonstrate that the marker was linked to downy mildew disease-resistant genotypes only (Jogaiah et al., 2014). The development of a linkage map was reported to integrate 256 DArT markers and 70 SSR markers and used to identify QTLs on LG1 with LOD score of 27 for rust resistance in 168 F7 pearl millet RILs derived from cross 81B-P6 × ICMP 451-P8 (Ambawat et al., 2016). Using a total of 106 pearl millet RILs (F6) derived from ICMB 841-P3 × 863B-P2 cross and 305 (96 SSRs and 208 DArT) markers, a linkage map was generated to map QTLs for grain iron and zinc content (Kumar et al., 2016). Recently, Kumar et al. (2018) reported a large-effect Fe and Zn content quantitative trait loci (QTLs) linked with DArT and SSR markers to construct a genetic linkage map using 317 RIL population derived from the (ICMS 8511-S1-17-2-1-1-B-P03 × AIMP 92901-S1-183-2-2-B-08) cross (Table 1).
Case Studies for GWAS in Pearl Millet
The advent of the recently decoded pearl millet genome has opened prodigious possibilities to discern several QTLs and the functions of its associated candidate genes governing diverse traits (Varshney et al., 2017). The genome size of pearl millet ~1.79 Gb, representing 38,579 genes, 88,256 SSRs, and 4,50,000 SNPs, will certainly be a valuable resource for constructing precision genetic maps (Varshney et al., 2017). Genetic mapping can be constructed in two different ways; one way is through QTL-mapping/interval mapping (IM) and the other is by using the association mapping (AM)/LD-mapping approach. The major difference in these two mapping strategies is based on the presumed idea over recombination events causative for the phenotypic variations (Myles et al., 2009). In general, QTL-mapping/IM can be done by developing various mapping populations viz., F2, and recombinant inbred line (RIL), near-isogenic line (NIL), back cross (BC), and doubled haploid (DH)-derived populations in which one can assume a clear cut degree of relatedness for the recombination events between the two contrasting parents for the trait of interest (Abdurakhmonov and Abdukarimov, 2008). Genetic mapping in this type of controlled population size results in the limited attainability of meiotic events and the products in the form of QTLs will be localized with lower resolution (10 to 20 cM intervals), and it is also an expensive approach to maintain a large number of populations (Jannink and Walsh, 2002; Flint-Garcia et al., 2003; Holland, 2007).
On the other hand, in AM there is no requirement for developing hybridization-based mapping populations; rather, it needs diverse germplasm accessions, including collections of different land-races, varieties, and a breeding material termed as a ‘panel’ where relatedness for the recombination events are not under control because of numerous meiotic recombinations across the diverse germplasm (Verdeprado et al., 2018). The principle of AM relies on the linkage disequilibrium (LD), a non-random association between two genes/markers/QTLs at different loci; however, a non-random association between these components in the same loci results in increased linkage disequilibrium levels (Flint-Garcia et al., 2003; Álvarez et al., 2014). Taking the advantage of multiple historic recombination events within the diverse accessions since their domestication, the AM approach can be best suited for the identification of genes or QTLs with high resolution (100–1000 Kb), and these are tightly linked to a broad range of phenotypic traits (Mackay et al., 2009). The potential of identifying promising QTLs, and also in detecting causal polymorphisms at the gene level, has made association mapping a powerful approach to develop marker-trait associations (MTAs) with great precision (Meuwissen and Goddard, 2000; Palaisa et al., 2003).
However, due to the high level of heterogeneity and heterozygosity in most of the germplasm accessions of pearl millet, very few association mapping strategies were delivered (Kannan et al., 2014); herein they are discussed and these detailed approaches may expand the scope of AM studies of pearl millet in future. A generalized workflow for the pearl millet genome-wide association studies (GWAS) pipeline is presented in Figures 1A, B. Pearl millet crop adaptation to various agro-climatic conditions is an important subject of study to explore the underlying genetics associated with this important nutri-cereal. Association studies made by Saïdou et al. (2009) on this aspect reveals the genetic factors responsible for the variations in flowering time at the phytochrome C (PHYC) (866 bp) locus, which is one of the key trait involved in crop adaptation. A total of 90 inbred and 598 pearl millet varieties from India, East, and West Africa were used for generating phenotypic data; followed by genotyping with 27 SSR and 6 AFLP markers. An LMM (linear mixed model) was used to identify a significant association between the phenotypic trait and genetic variations. With an aim to identify the best candidate gene loci associated with the flowering time, Saïdou et al. (2014) further explored an extra 100bp region surrounding the PHYC gene and performed an association study, MCMC method (Markov chain Monte Carlo method), to identify the tightly linked markers (75 SNPs and INDELS) surrounding the PHYC (6 Kb) genomic region and also to show the extent of LD to confer PHYC gene as the best candidate gene. By integrating the genome scan approach with association mapping, Mariac et al. (2011) identified the PgMADS11 gene, a MADS-box gene family member which plays a key role during somatic and reproductive phase development respective of different climatic conditions. Phenotyping data for the targeted traits from the 90 inbred lines viz., flowering time (FT), stem diameter (SD), plant height (PH), spikelet length (SpL), and spikelet density (SpD) are used for the association analysis; and the significant identified association of PgMADS11 alleles with a varied flowering time that deciphers the role of PgMADS11 in the plant adaptation process towards climatic change. Association studies of the selective SSR markers with the flowering time, plant height, panicle length, stover and grain yield were deciphered by Kannan et al. (2014).
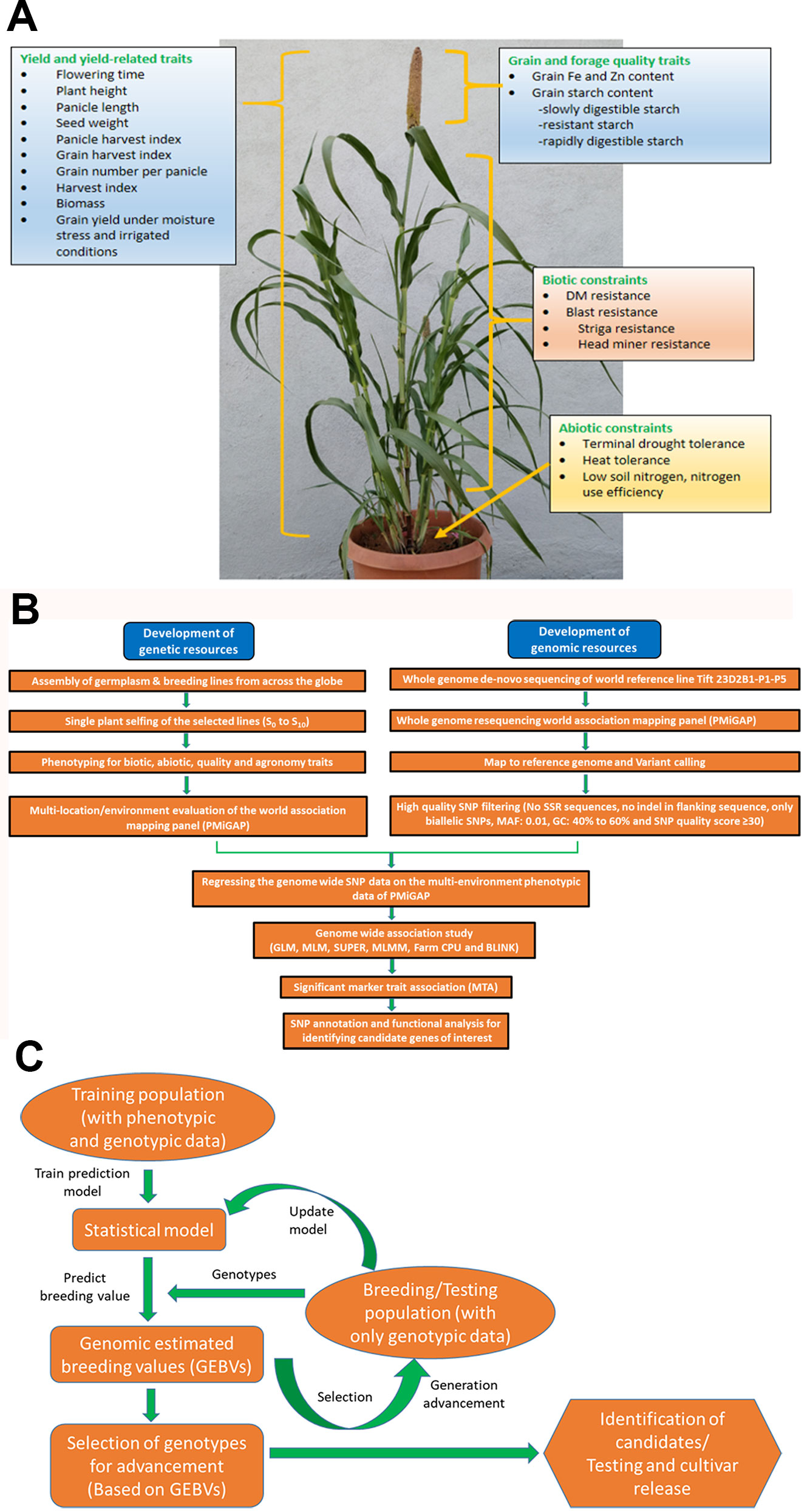
Figure 1 (A) Depiction of traits for which genome-wide association studies (GWAS) and genomic selection (GS) is being attempted at ICRISAT, Patancheru. (B) Workflow for genome-wide association studies (GWAS) pipeline. (C) Workflow for genomic selection pipeline.
A set of 250 full-sib progenies and 34 SSR markers were used for GWAS analysis, and results revealed the strong association of the Xpsmp2248_162 marker allele at linkage group 6 (LG6) with earlier flowering time and reduced plant height. Marker allele, Xpsmp2224_157 on LG7 was strongly associated with the plant height. For panicle length, Xpsmp2077_136, Xpsmp2233_260, and Xpsmp2224_157 were strongly associated with LG2, LG5, and LG7, respectively, whereas the Xpsmp2237_230 marker allele showed strong positive association on LG7 with grain yield. For stover dry matter yield, the Xicmp3058 193 marker allele showed strong positive correlation on LG6. There is a pressing need for information on genes associated with low phosphorus tolerance, especially in the regions of West Africa. Gemenet et al. (2015) made the first-ever reported association analysis of the available 285 DArT markers with the phenotypic data generated from 151 PMiGAP lines from West Africa across six environs under high and low P conditions. Results showed that the PgPb11603 DArT marker showed stable association with the flowering time, and the PgPb12954 marker showed a significant association with the grain yield.
Association studies reveal that Xibmsp11/AP6.1, an SNP marker on an acetyl CoA carboxylase gene, is strongly associated with GY, GHI (grain harvest index), and PY (panicle yield) under both treatments; whereas InDel markers viz., Xibmcp09/AP10.1 & Xibmcp09/AP10.2 of a chlorophyll a/b binding protein gene are associated with GY and stay-green traits. Using association mapping, key alleles for grain iron and zinc were demonstrated by Anuradha et al. (2017). Developing MTAs (Marker Trait Associations) between 250 SSR and 17 genic markers with grain iron and zinc content for 130 diversified lines across different environs revealed that the Xicmp3092 marker had a strong association with grain iron content on LG 7, and markers Xpsmp2086 & Xpsmp2213 and Xipes0224 showed association with grain zinc content on LG 4 and LG 6, respectively; conserved association for grain iron and zinc, however, was exhibited by Xipes0180, Xpsmp2261, and Xipes0096 on LG 3, LG 5, and LG 7, respectively. Another association study by Varshney et al. (2017) delivered key findings while establishing MTAs. Using whole-genome SNP data, a total of 3,117,056 SNPs were selected for GWAS analysis, and the phenotypic data for 20 agro-morphological traits was generated from 288 TCH (testcross hybrids) under two-stage (early & late) drought stress conditions along with respective controls. A significant association of the markers with the desired trait GNP (grain number per panicle) was exhibited on pseudomolecules Pg1 and Pg5. Genetic and genomic sequence information is now readily available for pearl millet. As AM will purvey a high-resolution power with the species exhibiting genotypic diversity across the germplasm (Álvarez et al., 2014), expanding AM studies in pearl millet will be increasingly fruitful for further crop improvement programs.
Case Studies for Genomic Selection (GS) in Pearl Millet
Genomic (or genome-wide) selection (GS) is a promising strategy that has huge potential to explore and increase the genetic gain per selection in a breeding scheme per unit timeline and, thus, speed and efficacy in breeding programs (Spindel et al., 2015). GS has proven to be an economical and viable alternative to marker-assisted selection (MAS) and phenotypic selection (PS) for quantitative traits and accelerated crop improvement programs in cereals and several other crops (Heffner et al., 2009; Zhong et al., 2009; Crossa et al., 2010; Ornella et al., 2012; Poland et al., 2012; Spindel et al., 2015; Muleta et al., 2019). By developing efficient training population (having both genotypic and phenotypic data) designs, it predicts the genomic estimated breeding values (GEBV) of the testing population (having only genotypic data) by utilizing genome-wide high throughput DNA markers that are in linkage disequilibrium (LD) with QTL, and predicted GEBVs are used for selection (Meuwissen et al., 2001). One of the key advantages of GS is that decisions on selections can be taken during the off-season, leading to improvements in genetic gain on an annual basis (Heffner et al., 2009). Advancement and application of GS in pearl millet breeding programs facilitate precise prediction of hybrid performance along with ideal resource allocation. In ICRISAT, efforts are being made to exploit the available whole-genome resequencing (WGRS) data of PMiGAP lines along with phenotyping data for different traits for GWAS and GS. Building on the various target traits using GWAS (Figures 1A, B), various whole-genome prediction/genomic selection models are being developed and optimized in pearl millet. A generalized workflow for the pearl millet genomic selection pipeline is presented in Figure 1C.
Varshney and his group (Varshney et al., 2017) applied WGRS data for genomic selection by ridge regression best linear unbiased prediction (RR-BLUP) to predict grain yield for test crosses in four scenarios viz., the performance of grain yield in control, early stress, late stress, and across environments and observed high prediction accuracies for the performance of across environments. It was also reported that by using GS strategy (additive and dominance effects) the hybrid performance was also predicted by analyzing grain yield data with 302,110 SNPs, and 170 promising hybrid combinations were found, of which 11 hybrid combinations were already utilized for hybrid production with better performance and the remaining 159 hybrid combinations could be potential candidates for developing high yielding hybrids. A hierarchical clustering analysis of possible single cross combinations (167910) revealed two sets of lines with a higher hybrid performance by 8% by crossing each other. These hybrids could be a potential nucleus for establishing high-yielding heterotic gene pools for developing pearl millet hybrids with higher yield potential (Varshney et al., 2017). In a recent study, Liang et al. (2018) assessed two potential genotyping strategies viz., RAD-seq and tGBS, to characterize a set of ICRISAT-developed inbred pearl millet lines and evaluated the utility of genomic selection/prediction. By utilizing the projected hybrids from both (RADseq and tGBS) techniques and four genomic prediction schemes in pearl millet and assessed for each phenotype, 20 random rounds of five-fold cross-validation were performed for a tested SNP set. It was reported that, by utilizing hybrid data, the genomic prediction scheme (RR-BLUP) generated median prediction ranges (in parentheses) for different traits viz., 1,000 grain weight (0.73–0.74); days to flowering (0.87–0.89); grain yield (0.48–0.51); and plant height (0.72–0.73), respectively. Other traits with less/no heterosis, only hybrid, and hybrid/inbred schemes were also performed equivalently. It was also reported that hybrid GEBVs can be moderately improved by incorporating inbred phenotypic data sets, once inbred, and hybrid trait values relative to the mean trait values of that population. It was also well demonstrated that guileless integration of historical inbred phenotypic data into hybrid breeding programs could reduce the prediction accuracy of traits exhibiting heterosis. However, controlling the heterosis effects within the inbred genotype and trait data could improve the accuracy of GEBVs for hybrids, which, in turn, strengthens pearl millet hybrid breeding programs.
Challenges in Using GWAS and GS for Pearl Millet
Being a poor man’s crop, pearl millet has attracted relatively less attention from various governments and policymakers in terms of support for the development of upstream science. This is particularly noted in areas such as GWAS and GS. The funding issues for carrying out this basic work in genomics has always remained an issue in pearl millet.
On the crop side, the high outcrossing rates, heterozygous nature, presence of inbreeding depression, and residual heterozygosity pose bottlenecks in inbred line development programs for the development of association mapping panels and for parental line/cultivar development were used in the training sets for GS. The presence of rapid linkage disequilibrium decay (LDD) warrants a relatively high number of markers for carrying out GWAS and GS. High rates of segregation distortions in specific populations may also pose serious challenges in GWAS and for getting high prediction accuracies for robust GS model development. Single-cross hybrids occupy a major market share in India, while top-cross and three-way hybrids are important for Africa. The development of GS models for hybrid parental lines resulting in heterotic combinations is quite challenging. These warrant precise estimation of the general combining ability (GCA) and specific combining ability (SCA) for specific agro-ecologies and their precise genotype-by-environment (G × E) interactions.
Conclusions and Way Forward
Pearl millet is a nutritious, climate change ready crop capable of yielding economic return in marginal conditions where other cereals may fail. In recent years, pearl millet has seen an enormous increase in terms of various genetic and genomic tools at the disposal of pearl millet workers worldwide. Whole-genome sequencing of the pearl millet genome and resequencing efforts resulting in the generation of millions of genome-wide SNPs have facilitated efforts to map various yield and yield-related, key biotic and abiotic stress tolerance, and nutritionally important traits globally. These genomic resources have also facilitated taking up of the whole-genome prediction model development and validation efforts. There is a need to further validate the loci linked to various traits of interest and move from “loci” to “genes.” There is an enormous opportunity to apply these learnings in the development of robust whole-genome prediction models with special emphasis on combining ability and heterotic gene pool studies for the development of heterotic hybrids.
Author Contributions
RKS and RG planned and coordinated this study. RKS, RG, RY, TC, RBS, SB, MP, and VP contributed to this work and drafted the manuscript. RKS and RG edited the manuscript for publication.
Conflict of Interest
The authors declare that the research was conducted in the absence of any commercial or financial relationships that could be construed as a potential conflict of interest.
Acknowledgments
Funding support from the Department of Biotechnology (DBT), Government of India, the Biotechnology and Biological Sciences Research Council (BBSRC), United Kingdom, and the CGIAR Research Program on Grain Legumes and Dryland Cereals (CRP-GLDC) is gratefully acknowledged. This work has been published as part of the CRP-GLDC.
References
Abdurakhmonov, I. Y., Abdukarimov, A. (2008). Application of association mapping to understanding the genetic diversity of plant germplasm resources. Int. J. Plant Genomics 2008, 1–18. doi: 10.1155/2008/574927
Allouis, S., Qi, X., Lindup, S., Gale, M. D., Devos, K. M. (2001). Construction of a BAC library of pearl millet. Pennisetum Glaucum. Theor. Appl. Genet. 102, 1200–1205. doi: 10.1007/s001220100559
Álvarez, M. F., Mosquera, T., Blair, M. W. (2014). The use of association genetics approaches in plant breeding. Plant Breed. Rev. 38, 17–68. doi: 10.1002/9781118916865.ch02
Ambawat, S., Senthilvel, S., Hash, C. T., Nepolean, T., Rajaram, V., Eshwar, K., et al. (2016). QTL mapping of pearl millet rust resistance using an integrated DArT-and SSR-based linkage map. Euphytica 209, 461–476. doi: 10.1007/s10681-016-1671-9
Anuradha, N., Satyavathi, C. T., Bharadwaj, C., Nepolean, T., Sankar, S. M., Singh, S. P., et al. (2017). Deciphering genomic regions for high grain iron and zinc content using association mapping in pearl millet. Front. Plant Sci. 8, 412. doi: 10.3389/fpls.2017.00412
Arya, L., Verma, M., Gupta, V. K., Karihaloo, J. L. (2009). Development of EST SSRs in finger millet (Eleusine coracana ssp coracana) and their transferability to pearl millet (Pennisetum glaucum). J. Plant Biochem. Biotechnol. 18, 97–100.
Bennett, M. D., Bhandol, P., Leitch, I. J. (2000). Nuclear DNA amounts in angiosperms and their modern uses—807 new estimates. Annals Bot. 86, 859–909. doi.org/10.1006/anbo.2000.1253
Bertin, I., Zhu, J. H., Gale, M. D. (2005). SSCP-SNP in pearl millet-a new marker system for comparative genetics. Theor. Appl. Genet. 110, 1467–1472. doi: 10.1007/s00122-005-1981-0
Bhattacharjee, R., Bramel, P., Hash, C., Kolesnikova-Allen, M., Khairwal, I. (2002). Assessment of genetic diversity within and between pearl millet landraces. Theor. Appl. Genet. 105, 666–673. doi: 10.1007/s00122-002-0917-1
Breseghello, F., Sorrells, M. E. (2006). Association mapping of kernel size and milling quality in wheat (Triticum aestivum L.) cultivars. Genetics 172, 1165–1177. doi: 10.1534/genetics.105.044586
Budak, H., Pedraza, F., Cregan, P. B., Baenziger, P. S., Dweikat, I. (2003). Development and utilization of SSRs to estimate the degree of genetic relationships in a collection of pearl millet germplasm. Crop Sci. 43, 2284–2290.
Chelpuri, D., Sharma, R., Durga, K. K., Katiyar, P., Mahendrakar, M. D., Singh, R. B., et al. (2019). Mapping quantitative trait loci (QTLs) associated with resistance to major pathotype-isolates of pearl millet downy mildew pathogen. Eur. J. Plant Pathol., 1–12. doi: 10.1007/s10658-019-01718-x
Crossa, J., Gustavo de, L. C., Paulino, P., Daniel, G., Juan, B., José, L. A., et al. (2010). Prediction of genetic values of quantitative traits in plant breeding using pedigree and molecular markers. Genetics 186, 713–724. doi: 10.1534/genetics.110.118521
Crossa, J., Pérez-Rodríguez, P., Cuevas, J., Montesinos-López, O., Jarquín, D., de los Campos, G., et al. (2017). Genomic selection in plant breeding: methods, models, and perspectives. Trends Plant Sci. 22, 961–975. doi: 10.1016/j.tplants.2017.08.011
Devos, K. M., Pittaway, T. S., Busso, C. S., Gale, M. D., Witcombe, J. R., Hash, C. T. (1995). Molecular tools for the pearl millet nuclear genome. Int. Sorghum Millets Newslett. 36, 64–66.
Flint-Garcia, S. A., Thornsberry, J. M., Buckler, E. S., IV (2003). Structure of linkage disequilibrium in plants. Annu. Rev. Plant Boil. 54, 357–374. doi: 10.1146/annurev.arplant.54.031902.134907
Gemenet, D. C., Hash, C. T., Sanogo, M. D., Sy, O., Zangre, R. G., Leiser, W. L. (2015). Phosphorus uptake and utilization efficiency in West African pearl millet inbred lines. Field Crops Res. 171, 54–66. doi: 10.1016/j.fcr.2014.11.001
Gómez, G., Álvarez, M. F., Mosquera, T. (2011). Association mapping, a method to detect quantitative trait loci: statistical bases. Agron. Colomb. 29, 367–376.
Gupta, P. K., Rustgi, S., Kulwal, P. L. (2005). Linkage disequilibrium and association studies in higher plants: present status and future prospects. Plant Mol. Biol. 57, 461–485. doi: 10.1007/s11103-005-0257-z
Gupta, S. K., Rai, K. N., Singh, P., Ameta, V. L., Gupta, S. K., Jayalekha, A. K., et al. (2015). Seed set variability under high temperatures during flowering period in pearl millet (Pennisetum glaucum L.(R.) Br.). Field Crops Res. 171, 41–53. doi: 10.1016/j.fcr.2014.11.005
Heffner, E. L., Sorrells, M. E., Jannink, J.-L. (2009). Genomic selection for crop improvement. Crop Sci. 49 (1), 1–12. doi: 10.2135/cropsci2008.08.0512
Holland, J. B. (2007). Genetic architecture of complex traits in plants. Curr. Opin.in Plant Boil. 10, 156–161. doi: 10.1016/j.pbi.2007.01.003
Hu, Z., Mbacké, B., Perumal, R., Guèye, M. C., Sy, O., Bouchet, S., et al. (2015). Population genomics of pearl millet (Pennisetum glaucum (L.) R. Br.): comparative analysis of global accessions and Senegalese landraces. BMC Genomics 16, 1048. doi: 10.1186/s12864-015-2255-0
Jogaiah, S., Sharathchandra, R. G., Raj, N., Vedamurthy, A. B., Shetty, H. S. (2014). Development of SCAR marker associated with downy mildew disease resistance in pearl millet (Pennisetum glaucum L.). Mol. Biol. Rep. 41, 7815–7824. doi: 10.1007/s11033-014-3675-7
Jannink, J. L., Walsh, B. (2002). Association mapping in plant populations. Quant. Genet. Genomics Plant Breed., 59–68. doi: 10.1079/9780851996011.0059
Jones, E. S., Liu, C. J., Gale, M. D., Hash, C. T., Witcombe, J. R. (1995). Mapping quantitative trait loci for downy mildew resistance in pearl millet. Theor. Appl. Genet. 94, 448–456.
Kanatti, A., Rai, K. N., Radhika, K., Govindaraj, M., Sahrawat, K. L., Srinivasu, K., et al. (2014). Relationship of grain iron and zinc content with grain yield in pearl millet hybrids. Crop Improv. 41, 91–96.
Kannan, B., Senapathy, S., Raj, B., Gajaraj, A., Chandra, S., Muthiah, A. (2014). Association analysis of SSR markers with phenology, grain, and stover-yield related traits in Pearl Millet (Pennisetum glaucum (L.) R. Br.). Sci. World J. 2014, 1–14. doi: 10.1155/2014/562327
Kraakman, A. T., Niks, R. E., Van den Berg, P. M., Stam, P., Van Eeuwijk, F. A. (2004). Linkage disequilibrium mapping of yield and yield stability in modern spring barley cultivars. Genetics 168, 435–446. doi: 10.1534/genetics.104.026831
Kumar, S., Hash, C. T., Thirunavukkarasu, N., Singh, G., Rajaram, V., Rathore, A., et al. (2016). Mapping quantitave trait loci controlling high iron and zinc in self and open pollinated grains of pearl millet [Pennisetum glaucum (L) R. Br.]. Front. Plant Sci. 7, 1636. doi: 10.3389/fpls.2016.01636
Kumar, S., Hash, C., Nepolean, T., Mahendrakar, M., Satyavathi, C., Singh, G., et al. (2018). Mapping grain iron and zinc content quantitative trait loci in an iniadi-derived immortal population of pearl millet. Genes 9, 248.
Liang, Z., Gupta, S. K., Yeh, C. T., Zhang, Y., Ngu, D. W., Kumar, R., et al. (2018). Phenotypic data from inbred parents can improve genomic prediction in pearl millet hybrids. G3: Genes Genomes Genet. 8, 7, 2513–2522. doi: 10.3390/genes9050248
Liu, C. J., Witcombe, J. R., Pittaway, T. S., Nash, M., Hash, C. T., Busso, C. S., et al. (1994). An RFLP-based genetic map of pearl millet (Pennisetum glaucum). Theor. Appl. Genet. 89, 481–487. doi: 10.1534/g3.118.200242
Liu, C. J., Witcombe, J. R., Pittaway, T. S., Nash, M., Hash, C. T., Gale, M. D. (1992). Restriction fragment length polymorphism in pearl millet, Pennisetum glaucum,”. Actes decolloque International. Eds. Mounolou, J. C, Sarr, A. (Paris: Bureau des Ressources Génétiques), 233–241.
Liu, N., Xue, Y., Guo, Z., Li, W., Tang, J. (2016). Genome-wide association study identifies candidate genes for starch content regulation in maize kernels. Front. Plant Sci. 7, 1046. doi: 10.1007/BF00225384
Mackay, T. F., Stone, E. A., Ayroles, J. F. (2009). The genetics of quantitative traits: challenges and prospects. Nat. Rev. Genet. 10, 565–577. doi: 10.2135/cropsci2011.09.0503
Manning, K., Pelling, R., Higham, T., Schwenniger, J. L., Fuller., D. Q. (2011). 4500-year old domesticated pearl millet (Pennisetum glaucum) from the Tilemsi Valley, Mali: new insights into an alternative cereal domestication pathway. J. Archaeol. Sci. 38, 312–322. doi: 10.1038/nrg2612
Mariac, C., Jehin, L., Saïdou, A. A., Thuillet, A. C., Couderc, M., Sire, P. (2011). Genetic basis of pearl millet adaptation along an environmental gradient investigated by a combination of genome scan and association mapping. Mol. Ecol. 20, 80–91. doi: 10.1016/j.jas.2010.09.007
Mariac, C., Luong, V., Kapran, I., Mamadou, A., Sagnard, F., Deu, M., et al (2006). Diversity of wild and cultivated pearl millet accessions (Pennisetum glaucum [L.] R. Br.) in Niger assessed by microsatellite markers. Theoret. Appl. Genet. 11, 49–58. doi: 10.1007/s00122-006-0409-9
Meuwissen, T. H. E., Goddard, M. E. (2000). Fine mapping of quantitative trait loci using linkage disequilibria with closely linked marker loci. Genetics 155, 421–430. doi: 10.1111/j.1365-294X.2010.04893.x
Meuwissen, T. H., Hayes, B. J., Goddard, M. E. (2001). Prediction of total genetic value using genome-wide dense marker maps. Genetics 157, 1819–1829.
Muleta, K. T., Pressoir, G., Morris, G. P. (2019). Optimizing genomic selection for a sorghum breeding program in Haiti: a simulation study. G3. Genes Genomes Genet. 9, 391–401. doi: 10.1534/g3.118.200932
Myles, S., Peiffer, J., Brown, P. J., Ersoz, E. S., Zhang, Z., Costich, D. E., et al. (2009). Association mapping: critical considerations shift from genotyping to experimental design. Plant Cell 212194-, 2202. doi: 10.1105/tpc.109.068437
Nambiar, V. S., Dhaduk, J. J., Sareen, N., Shahu, T., Desai, R. (2011). Potential functional implications of pearl millet (Pennisetum glaucum) in health and disease. J. Appl. Pharm. Sci. 01, 62–67. doi: 10.1105/tpc.109.068437
Ornella, L., Singh, S., Perez, P., Burgueño, J., Singh, R., Tapia, E., et al. (2012). Genomic prediction of genetic values for resistance to wheat rusts. Plant Genome 5, 136–148. doi: 10.3835/plantgenome2012.07.0017
Palaisa, K. A., Morgante, M., Williams, M., Rafalski, A. (2003). Contrasting effects of selection on sequence diversity and linkage disequilibrium at two phytoene synthase loci. Plan. Cell. 15, 1795–1806. doi: 10.1105/tpc.012526
Poland, J., Endelman, J., Dawson, J., Rutkoski, J., Wu, S., Manes, Y., et al. (2012). Genomic selection in wheat breeding using genotyping-by-sequencing. Plant Genome 5, 103–113. doi: 10.1105/tpc.012526
Qi, X., Pittaway, T. S., Lindup, S., Liu, H., Waterman, E., Padi, F. K., et al. (2004). An integrated genetic map and a new set of simple sequence repeat markers for pearl millet, Pennisetum glaucum. Theor. Appl. Genet. 109, 1485–1493. doi: 10.3835/plantgenome2012.06.0006
Rai, K. N., Govindaraj, M., Rao, A. S. (2012). Genetic enhancement of grain iron and zinc content in pearl millet. Crops Foods. 4, 119–125. doi: 10.1111/j.1757-837X.2012.00135.x.
Rajaram, V., Nepolean, T., Senthilvel, S., Varshney, R. K., Vadez, V., Srivastava, R. K., et al. (2013). Pearl millet [Pennisetum glaucum (L.) R. Br.] consensus linkage map constructed using four RIL mapping populations and newly developed EST-SSRs. BMC Genomics 14, 159. doi: 10.1007/s00122-004-1765-y
Rajaram, V., Varshney, R. K., Vadez, V., Nepolean, T., Senthilvel, S., Kholova, J. (2010). Development of EST resources in pearl millet and their use in development and mapping of EST-SSRs in four RIL populations. Plant Anim. Gen. 18, 9–13.
Rao, P. P., Birthal, P. S., Reddy, B. V., Rai, K. N., Ramesh, S. (2006). Diagnostics of sorghum and pearl millet grains-based nutrition in India. International Sorghum and Millets News letter. 47, 93–96.
Saïdou, A. A., Mariac, C., Luong, V., Pham, J. L., Bezançon, G., Vigouroux, Y. (2009). Association studies identify natural variation at PHYC linked to flowering time and morphological variation in pearl millet. Genetics 182, 899–910. doi: 10.1186/1471-2164-14-159
Saïdou, A. A., Clotault, J., Couderc, M., Mariac, C., Devos, K. M., Thuillet, A. C. (2014). Association mapping, patterns of linkage disequilibrium and selection in the vicinity of the PHYTOCHROME C gene in pearl millet. Theor. Appl. Genet. 127, 19–32. doi: 10.1534/genetics.109.102756
Sehgal, D., Rajaram, V., Armstead, I. P., Vadez, V., Yadav, Y. P., Hash, C. T., et al. (2012). Integration of gene-based markers in a pearl millet genetic map for identification of candidate genes underlying drought tolerance quantitative trait loci. BMC Plant Biol. 12 (1), 9. doi: 10.1007/s00122-013-2197-3
Sehgal, D., Skot, L., Singh, R., Srivastava, R. K., Das, S. P., Taunk, J. (2015). Exploring potential of pearl millet germplasm association panel for association mapping of drought tolerance traits. PloS One 10, 1–28. doi: 10.1186/1471-2229-12-9
Senthilvel, S., Jayashree, B., Mahalakshmi, V., Kumar, P. S., Nakka, S., Nepolean, T., et al. (2008). Development and mapping of simple sequence repeat markers for pearl millet from data mining of expressed sequence tags. BMC Plant Biol. 8, 119. doi: 10.1371/journal.pone.0122165
Senthilvel, S., Nepolean, T., Supriya, A., Rajaram, V., Kumar, S., Hash, C. T., et al. (2010).Development of a molecular linkage map of pearl millet integrating DArT and SSR markers, in: Proc. Plant Animal Genome 18 Conference, San Diego, CA. pp. 9–13. doi: 10.1186/1471-2229-8-119
Serba, D. D., Yadav, R. S. (2016). Genomic tools in pearl millet breeding for drought tolerance: status and prospects. Front. Plant Sci. 7, 1724. doi: 10.3389/fpls.2016.01724
Singh, M., Upadhyaya, H. D. (2016). Genetic and genomic resources for grain cereals improvement. Academic Press. pp: 253–289. doi: 10.1016/B978-0-12-802000-5.00006-X
Spindel, J., Begum, H., Akdemir, D., Virk, P., Collard, B., Redona, E., et al. (2015). Genomic selection and association mapping in rice (Oryza sativa): effect of trait genetic architecture, training population composition, marker number and statistical model on accuracy of rice genomic selection in elite, tropical rice breeding lines. PloS Genet. 11, p.e1004982. doi: 10.1371/journal.pgen.1005350
Supriya, A., Senthilvel, S., Nepolean, T., Eshwar, K., Rajaram, V., Shaw, R., et al. (2011). Development of a molecular linkage map of pearl millet integrating DArT and SSR markers. Theor. Appl. Genet. 123, 239–250. doi: 10.1371/journal.pgen.1004982
Upadhyaya, H. D., Reddy, K. N., Gowda, C. L. L. (2007). Pearl millet germplasm at ICRISAT genebank-status and impact. J. SAT Agricul. Res. 3, 1–5.
Upadhyaya, H. D., Yadav, D., Reddy, K. N., Gowda, C. L. L., Singh, S. (2011). Development of pearl millet minicore collection for enhanced utilization of germplasm. Crop Sci. 5, 217–223.
Vadez, V., Hash, T., Bidinger, F. R., Kholova, J. (2012). Phenotyping pearl millet for adaptation to drought. Front. Physiol. 3, 1–386. doi: 10.3389/fphys.2012.00386
Varshney, R. K., Shi, C., Thudi, M., Mariac, C., Wallace, J., Qi, P., et al. (2017). Pearl millet genome sequence provides a resource to improve agronomic traits in arid environments. Nat. Biotechnol. 35, 969. doi: 10.1007/s00122-011-1580-1
Verdeprado, H., Kretzschmar, T., Begum, H., Raghavan, C., Joyce, P., Lakshmanan, P. (2018). Association mapping in rice: basic concepts and perspectives for molecular breeding. Plan. Produc. Sci. 21, 159–176. doi: 10.1038/nbt.3943
Yadav, O. P., Mitchell, S. E., Fulton, T. M., Kresovich, S. (2008). Transferring molecular markers from sorghum, rice and other cereals to pearl millet and identifying polymorphic markers. An Open Access Journal published by ICRISAT. 6, 1–4.
Yadav, O. P., Mitchell, S. E., Zamora, A., Fulton, T. M., Kresovich, S. (2007). Development of new simple sequence repeat markers for pearl millet. J. SAT Agricul. Res. 3, 1–34.
Zhao, K., Aranzana, M. J., Kim, S., Lister, C., Shindo, C., Tang, C., et al. (2007). An Arabidopsis example of association mapping in structured samples. PloS Genet. 3, e4. doi: 10.1080/1343943X.2018.1483205
Keywords: pearl millet, genetic resources, genomic resources, genomic selection, genome-wide association studies, molecular markers
Citation: Srivastava RK, Singh RB, Pujarula VL, Bollam S, Pusuluri M, Chellapilla TS, Yadav RS and Gupta R (2020) Genome-Wide Association Studies and Genomic Selection in Pearl Millet: Advances and Prospects. Front. Genet. 10:1389. doi: 10.3389/fgene.2019.01389
Received: 20 September 2019; Accepted: 19 December 2019;
Published: 28 February 2020.
Edited by:
Nunzio D’Agostino, Università degli Studi di Napoli Federico II, ItalyReviewed by:
Cheng Sun, Chinese Academy of Agricultural Sciences, ChinaVandana Jaiswal, Institute of Himalayan Bioresource Technology (CSIR), India
Zhenbin Hu, Kansas State University, United States
Copyright © 2020 Srivastava, Singh, Pujarula, Bollam, Pusuluri, Chellapilla, Yadav and Gupta. This is an open-access article distributed under the terms of the Creative Commons Attribution License (CC BY). The use, distribution or reproduction in other forums is permitted, provided the original author(s) and the copyright owner(s) are credited and that the original publication in this journal is cited, in accordance with accepted academic practice. No use, distribution or reproduction is permitted which does not comply with these terms.
*Correspondence: Rakesh K. Srivastava, r.k.srivastava@cgiar.org; Rajeev Gupta, g.rajeev@cgiar.org