Nodal Global Efficiency in Front-Parietal Lobe Mediated Periventricular White Matter Hyperintensity (PWMH)-Related Cognitive Impairment
- 1Department of Neurology, Drum Tower Hospital, Medical School and The State Key Laboratory of Pharmaceutical Biotechnology, Institute of Brain Science, Nanjing University, Nanjing, China
- 2Jiangsu Key Laboratory of Molecular Medicine, Medical School of Nanjing University, Nanjing, China
- 3Jiangsu Province Stroke Center for Diagnosis and Therapy, Nanjing, China
- 4Nanjing Neuropsychiatry Clinic Medical Center, Nanjing, China
- 5Department of Radiology, Affiliated Drum Tower Hospital of Nanjing University Medical School, Nanjing, China
White matter hyperintensity (WMH) is widely observed in the elderly population and serves as a key indicator of cognitive impairment (CI). However, the underlying mechanism remains to be elucidated. Herein, we investigated the topological patterns of resting state functional networks in WMH subjects and the relationship between the topological measures and CI. A graph theory-based analysis was employed in the resting-state functional magnetic resonance scans of 112 subjects (38 WMH subjects with cognitive impairment without dementia (CIND), 36 WMH subjects with normal cognition and 38 healthy controls (HCs), and we found that WMH-CIND subjects displayed decreased global efficiency at the levels of the whole brain, specific subnetworks [fronto-parietal network (FPN) and cingulo-opercular network (CON)] and certain nodes located in the FPN and CON, as well as decreased local efficiency in subnetworks. Our results demonstrated that nodal global efficiency in frontal and parietal regions mediated the impairment of information processing speed related to periventricular WMH (PWMH). Additionally, we performed support vector machine (SVM) analysis and found that altered functional efficiency can identify WMH-CIND subjects with high accuracy, sensitivity and specificity. These findings suggest impaired functional networks in WMH-CIND individuals and that decreased functional efficiency may be a feature of CI in WMH subjects.
Introduction
Cerebral small vessel disease (CSVD) is a group of clinical syndromes arising from impaired blood circulation in cerebral arterioles, small perforating arteries, capillaries and small veins in the brain. CSVD accounts for approximately 10–30% of ischaemic strokes worldwide (Patel and Markus, 2011; Xu et al., 2018) and is a leading vascular contributor to cognitive deficits and dementia, mainly involving global cognitive function, information processing speed, and executive function (Jokinen et al., 2009; Debette and Markus, 2010; Wu et al., 2016; Liu et al., 2019). The early prediction of cognitive impairment (CI) due to CSVD is of great value because managing vascular risk factors early, e.g., diabetes, hypertension, hyperlipidaemia and smoking, may decrease the risk of developing CI in individuals with CSVD (Gorelick et al., 2011; Gu et al., 2019).
White matter hyperintensities (WMH), subcortical/lacunar infarcts, an enlarged perivascular space and brain microbleeds have been perceived as radiological manifestations of CSVD (Ter Telgte et al., 2018). Many studies have investigated the association between these image representations and CI in CSVD subjects, and conflicting results have been shown. WMH is thought to be detected in 72–96% of the population over 60 years old (De Leeuw et al., 2001; Zhuang et al., 2018; Lampe et al., 2019). Increasing evidences have confirmed that WMH burden can lead to CI in executive function and information processing speed and associated with increased risk of dementia (Van Dijk et al., 2008; Sudo et al., 2013). However, WMH is very common in elderly subjects with normal cognition, and a portion of these individuals do not develop CI (Lampe et al., 2019). Similarly, although a link between subcortical/lacunar infarcts and CI has been shown by neuropathological studies (Raman et al., 2014), subcortical/lacunar infarcts are also commonly observed in elderly subjects without CI or with non-vascular CI (Brundel et al., 2012). The association between brain microbleeds and CI varies according to the counts and location of the lesions (Banerjee et al., 2016), but some studies have reported no association (Brundel et al., 2014; Heringa et al., 2014). Finally, the association between enlarged perivascular space and cognition in CSVD is also conflicting (Hurford et al., 2014; Yao et al., 2014). These findings suggest that the structural lesions of CSVD are not always associated with CI. Exploring the functional mechanisms underlying CI in CSVD would contribute to the understanding of the onset of CI in CSVD.
In the last two decades, a graph theory-based analysis method has been developed to explore brain functional connectivity (FC) networks in both resting or task states, and these networks can be constructed with functional neuroimaging data retrieved from fMRI blood-oxygen-level-dependent (BOLD) time series. Related studies have demonstrated that human brain large-scale functional networks are composed of brain regions highly correlated at the level of neural activity, partly reflecting the underlying structural connectivity architecture confirmed by diffusion tensor imaging scans (Smith et al., 2009; Van Den Heuvel et al., 2009). CSVD exerts its action on connectivity between structural and functional networks, thereby disrupting efficient communication in the brain network (Ter Telgte et al., 2018). A number of studies have investigated structural network connectivity derived from the integrity of white matter tracts in CSVD and the association between alterations in structural network connectivity and CI in CSVD. The network efficiency of the structural network was found to be decreased in CSVD subjects and correlated better with CI than traditional radiological features, e.g., WMH burden (Lawrence et al., 2014; Kim et al., 2015); in addition, lower network efficiency predicted conversion to dementia in CSVD patients (Tuladhar et al., 2016). In contrast, topological patterns of large-scale functional networks have rarely been studied in populations with CSVD. Functional brain alterations have been thought to arise prior to structural changes and clinical symptoms in subjects with non-vascular CI (Sheline and Raichle, 2013). Functional abnormalities can even be detected in healthy subjects at risk for CI (Nichols et al., 2012; Heise et al., 2014). Considering the potential link between functional networks and structural networks, we hypothesized that subjects with WMH, one of the most common images found in CSVD, would display altered topological patterns in large-scale functional networks, and these functional alterations would be associated with CI in subjects with WMH.
White matter hyperintensity subjects with cognitive impairment without dementia (CIND), WMH subjects with normal cognition and healthy subjects were recruited for the present study. All subjects underwent standard neuropsychological tests and multimodal MRI scans. We aimed to investigate topological patterns of resting state functional networks in WMH subjects with and without CI and the relationship between topological measures and CI.
Materials and Methods
Participants
The present investigation is a hospital-based cross-sectional study (Clinical Trial: ChiCTR-OOC-17010562) that consists of 112 right-handed participants (74 subjects with moderate-to-severe WMH and 38 matched healthy controls) between 50 and 80 years old. Subjects with moderate-to-severe WMH were divided into WMH-normal cognition (WMH-NC, n = 36) and WMH-CI (WMH-CIND n = 38) based on the Beijing version of the Montreal Cognitive Assessment (MoCA-BJ). In order to avoid the level of education affecting the results of this scale, the optimal cutoff points are determined according to education level (or years of education). For subjects with no formal education, the MoCA-BJ cutoff was 13/14; for subjects with 1–6 years of education, the MoCA-BJ cutoff was 19/20; and for subjects with seven or more years of education, it was 24/25 (Lu et al., 2011; Yu et al., 2012; Liu et al., 2019). Moderate-to-severe WMH was defined by neuroimaging evidence: Fazekas visual rating scales of grade 2 or 3. Exclusion criteria included cerebral hemorrhage, non-vascular-related WMH mimics (e.g., brain irradiation and multiple sclerosis), dementia (Clinical Dementia Rating Scale ≥1), Mini-Mental State Examination (MMSE) ≤23 and psychiatric disorders. This study was approved by the Ethics Committee of Nanjing Drum Tower Hospital, and each participant provided written informed consent.
MRI Scanning
All of the participants were examined on a Philips 3.0-T scanner (3.0 T Ingenia (32-channel head coil), Philips, Eindhoven, Netherlands) The examination protocol included a high-resolution T1-weighted turbo gradient echo sequence [repetition time (TR) = 9.8 ms, flip angle (FA) = 8°, echo time (TE) = 4.6 ms, FOV = 250 × 250 mm2, number of slices = 192, acquisition matrix = 256 × 256, thickness = 1.0 mm], a FLAIR sequence [TR = 4500 ms, TE = 333 ms, time interval (TI) = 1600 ms, number of slices = 200, voxel size = 0.95 × 0.95 × 0.95 mm3, acquisition matrix = 270 × 260] and a gradient-recalled echo planar imaging sequence (TR = 2000 ms, FA = 90°, TE = 30 ms, number of slices = 35, acquisition matrix = 64 × 64, FOV = 240 × 240 mm2, thickness = 4 mm). The Wisconsin White Matter Hyperintensities Segmentation Toolbox1 was used to automatically determine the total WMH volume, including deep-WMH (DWMH) and periventricular-WMH (PWMH), based on T1-weighted and FLAIR images. The total brain volume was automatically obtained using Statistical Parametric Mapping (SPM8)2. Given the skewed distribution of the WMH volumes, the values were first normalized by the corresponding total brain volume and then log-transformed (base 10).
Neuropsychological Measurement
All subjects underwent a standardized neuropsychological test protocol, including global cognitive assessments and multiple cognitive domain examinations. Global cognitive function was evaluated by MMSE and MoCA-BJ. The raw test scores were converted to Z-scores, which were used to calculate the compound cognitive index. Episodic memory was calculated as the mean of the Z-scores from the Wechsler Memory Scale-Visual Reproduction-delayed recall and Auditory Verbal Learning Test-delayed recall scores. Visuospatial function is a compound score that includes the mean of the Z-scores of the Clock Drawing Test and Visual Reproduction–copy test. Information processing speed was calculated as the average Z-scores of the Trail Making Test-A (TMT-A) and the Stroop Color and Word Tests A and B (Stroop A and B). The language function consisted of the Category Verbal Fluency test and Boston Naming Test. Executive function is a compound score of the average Z-scores of the Digit Span Test-backward, TMT-B and Stroop C.
Image Pre-processing
Resting-state fMRI data were pre-processed by the Graph Theoretical Network Analysis Toolbox3. The first ten volumes were discarded before slice time correction and realignment to the first volume to correct for head motion. No participant performed a displacement >2 mm or an angular rotation >2° in any direction. Next, the obtained images were spatially normalized to the Montreal Neurological Institute space and resampled with a resolution of 3 × 3 × 3 mm voxels. Subsequently, filtering was performed using a bandpass filter (0.01–0.1 Hz), and linear trends were also removed. Finally, several nuisance variables, including the Friston-24 parameters and white matter and cerebrospinal fluid signals, were removed by multiple linear regression analysis.
Network Construction
To define the network nodes, Dosenbach’s atlas was used to parcellate the whole brain into 160 functionally segregated regions of interest (ROIs, radius = 5 mm). These 160 brain regions could be partitioned into six subnetworks: the default mode network (DMN), the fronto-parietal network (FPN), the cingulo-opercular network (CON), the sensorimotor network (SMN), the occipital network (ON), and the cerebellum network (CN). To define the network edge, we calculated Pearson correlation coefficients for each pair of 160 ROIs between the regional mean time series. A Fisher Z-transformation was used to improve the normality of the correlation coefficients. We applied a set of sparsity thresholds (ranging from 0.1 to 0.4, with steps of 0.01) to generate a binary undirected network. This range of sparsity thresholds was chosen because networks were not fully connected at lower sparsity thresholds and were less likely to maintain small-world architecture at higher sparsity thresholds.
Graph Theory Analysis
We used the Brain Connectivity Toolbox4 to calculate two graph theoretical measures including global and local efficiency at the network and node levels, respectively. Functional efficiency measures how efficiently information is exchanged over the distributed brain regions. Human brain networks are seen as systems that are both globally and locally efficient. The detailed descriptions of these metrics are given below.
Global efficiency measures the parallel information transfer ability of the network, which can be computed as follows:
where N is the number of nodes in the network G, and dij is the shortest path length between node i and j in the network.
Local efficiency measures the information exchanging ability among a sub-graph with locally interconnected nodes; it reflects system redundancy and tolerance to attack, which can be computed as follows:
where N is the number of nodes in the network G, and Gi is the sub-graph consisting of node i and its local neighbors.
To determine the nodal properties of the networks, we computed the nodal global efficiency and nodal local efficiency. The nodal efficiency quantifies the importance of the nodes for information communication within the network.
The nodal global efficiency measures the average shortest path length between a given node i and all of the other nodes in the network, which can be computed as follows:
where N is the number of nodes in the network G, and dij is the shortest path length between node i and j in the network.
The nodal local efficiency measures the global efficiency of the sub-graph formed by this given node’s neighbors, which can be computed as follows:
where Gi is the sub-graph consisting of node i and its local neighbors.
Moreover, we also calculated the area under the curve (AUC) for each efficiency metric over the range of sparsity (0.1∼0.4), which provided a summarized scalar for the brain topological characterization independent of single threshold selection.
Statistical Analysis
Differences across HC, WMH-NC and WMH-CIND groups in demographic and cognitive performance were assessed using chi-squared (χ2) tests or one-way analysis of variance (ANOVA) using Statistical Package for Social Sciences (SPSS) version 22 (IBM Corp., Armonk, NY, United States). WMH volumes were compared in SPSS by Kruskal-Wallis one-way ANOVA. The significance level was set at P < 0.05.
The AUC of the global and local efficiency over a wide range of thresholds of the network were analyzed with ANOVA to detect significant differences (with Bonferroni-corrected post hoc t-tests, P = 0.05/3). Then, to determine the brain regions with significantly altered nodal global and local efficiency, ANOVA was performed on the AUC of nodal efficiency with false-discovery rate (FDR) correction (q = 0.05).
We performed multiple linear regression analyses to investigate the relationships among log-transformed normalized WMH volume, functional efficiency metrics and cognition, adjusted for potential confounders (age, gender, and education).
Furthermore, we conducted mediation analyses to test the primary hypothesis of whether functional efficiency metrics mediated the relationships between WMH volumes and cognition, controlling for covariates (age, gender, and education). The primary estimates of interest were the degree of the changes in the direct path between WMH volumes and cognition, labeled c in the bi-variate models and c’ in the full mediating models, and the indirect path from WMH volumes to cognition through functional efficiency metrics: the product of paths a and b. We computed the bias-corrected 95% confidence intervals (CI) for the size of the mediating effects with bootstrapping (k = 1000 samples). The mediating effect is said to be present if the 95% CI does not contain zero. Mediation analyses were conducted in PROCESS for the SPSS framework.
Support Vector Machine
Support vector machine (SVM) analysis is extensively applied in disease classification. Generally, the SVM procedure involves three stages: feature selection, classifier training and prediction. SVM starts with feature selection as the basis for classification to form a high dimensional space. In this study, statistically significant features, like global and local efficiency, nodal global and local efficiency, between groups were selected for SVM. Then, SVM conducts the classifier training to construct a hyperplane that optimally separates the classes. Last, the classifier is used to predict the class label when a new sample is added into the classifier. In this study, SVM analysis was performed on the functional efficiency data of group differences using a toolkit named LIBSVM5. Due to the small sample size, we used the leave-one-out cross-validation test to evaluate the mean accuracy rate differentiating WMH-CIND from WMH-NC. The performance of a classifier can also be quantified using sensitivity, specificity and the area under the receiver operating characteristic curve (ROC) according to the results of cross-validation. Note that the specificity represents the proportion of WMH-NC subjects correctly predicted, while the sensitivity represents the proportion of WMH-CIND subjects correctly predicted.
Results
Demographic and Clinical Characteristics
Demographic and clinical variables for the HC, WMH-NC and WMH-CIND groups are summarized in Table 1. There were no significant differences in age, gender or years of education among the three groups. WMH, PWMH, and DWMH volumes in WMH-NC and WMH-CIND subjects were significantly higher than those in HCs (P < 0.001). The WMH-CIND group exhibited poorer performance on the MMSE (P < 0.001) and MoCA-BJ (P < 0.001) tests and showed worse episodic memory (P < 0.001), visuospatial function (P = 0.005), information processing speed (P < 0.001), language function (P < 0.001) and executive function (P < 0.001) than the HC and WMH-NC groups (details of cognitive performance in Table 1).
Alterations in Network-Level Efficiency
First, we compared the global and local efficiency of the whole brain among the three groups. We found significantly decreased global efficiency in WMH-CIND subjects compared with HCs at sparsities of 0.06∼0.35 (P < 0.05, uncorrected) and WMH-NC subjects at sparsities of 0.06∼0.35 (P < 0.05, uncorrected), but no significant differences were found between HCs and WMH-NC subjects. Furthermore, the AUC of global efficiency in the WMH-CIND group was significantly lower than that in the HC and WMH-NC groups (ANOVA, P < 0.001; Bonferroni-corrected, P < 0.05/3) (Figure 1A). Although the local efficiency of the three groups in some sparsities was significantly altered, no significant differences in the AUC of local efficiency were found (ANOVA, P = 0.471) (Figure 1B).
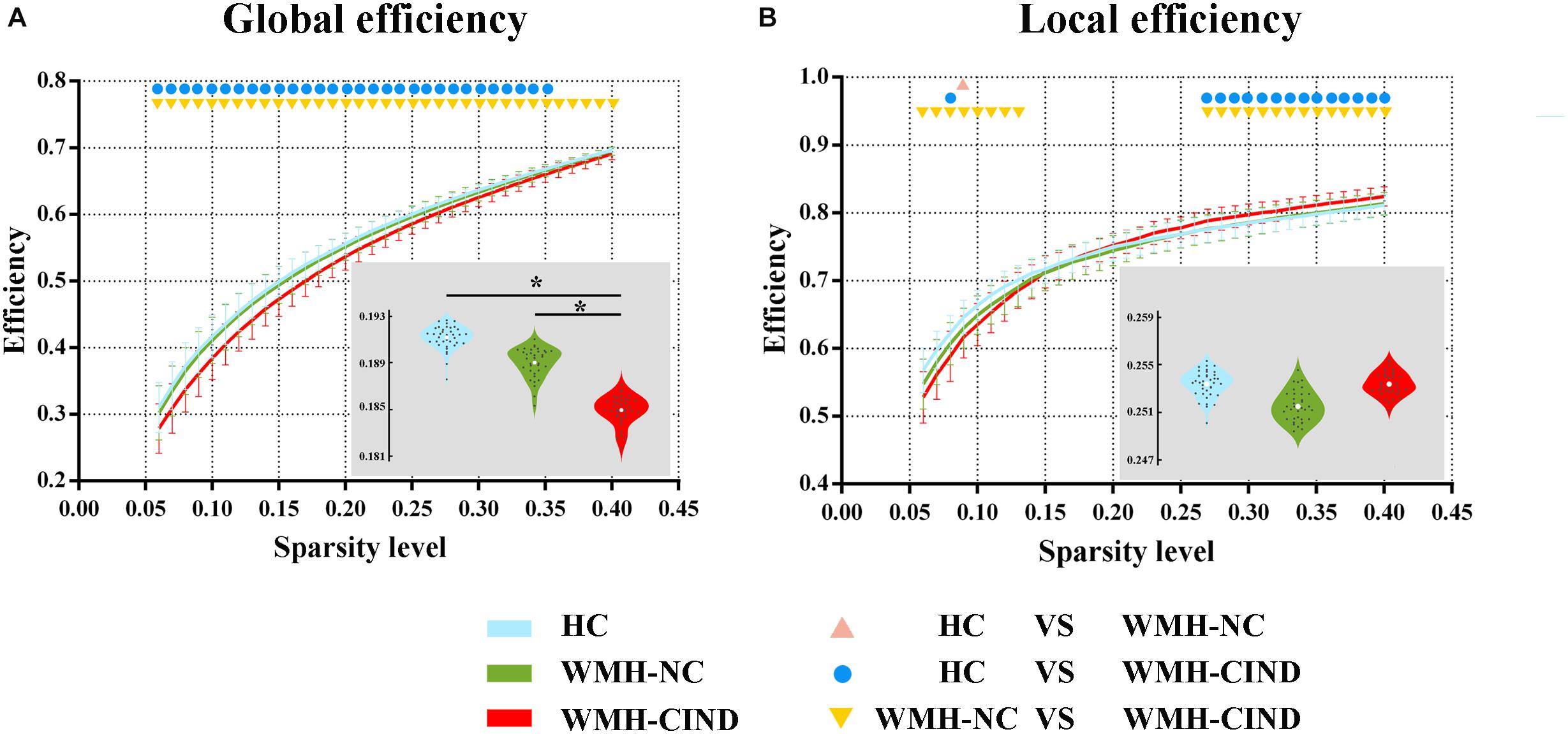
Figure 1. Global and local efficiency of the whole brain compared between HC, WMH-NC and WMH-CIND groups. (A) AUC of global efficiency in WMH-CIND subjects was significantly decreased compared with that of HCs and WMH-NC subjects (ANOVA, P < 0.001; Bonferroni-corrected, P < 0.05/3). (B) No significant differences in the AUC of local efficiency between the three groups were found (ANOVA, P = 0.471). Abbreviations: HC, healthy control; NC, normal cognition; CIND, cognitive impairment without dementia; WMH, white matter hyperintensities; AUC, area under the curve. ∗ indicates a statistical difference between groups.
Second, we calculated the global and local efficiency of the six subnetworks. As displayed in Figure 2, WMH-CIND subjects showed lower global efficiency in the FPN than HCs and WMH-NC subjects (ANOVA, P = 0.004; Bonferroni-corrected, P < 0.05/3). In addition, the global efficiency of the CON in WMH-CIND subjects was significantly lower than that in HCs (ANOVA, P = 0.003; Bonferroni-corrected, P < 0.05/3). As shown in Figure 3, we found significantly decreased local efficiency in the FPN in WMH-CIND subjects compared with WMH-NC subjects (ANOVA, P = 0.014; Bonferroni-corrected, P < 0.05/3). Furthermore, HCs showed higher local efficiency in the CON than WMH-NC and WMH-CIND subjects (ANOVA, P = 0.004; Bonferroni-corrected, P < 0.05/3). No significant group differences in global and local efficiency were found in other subnetworks.
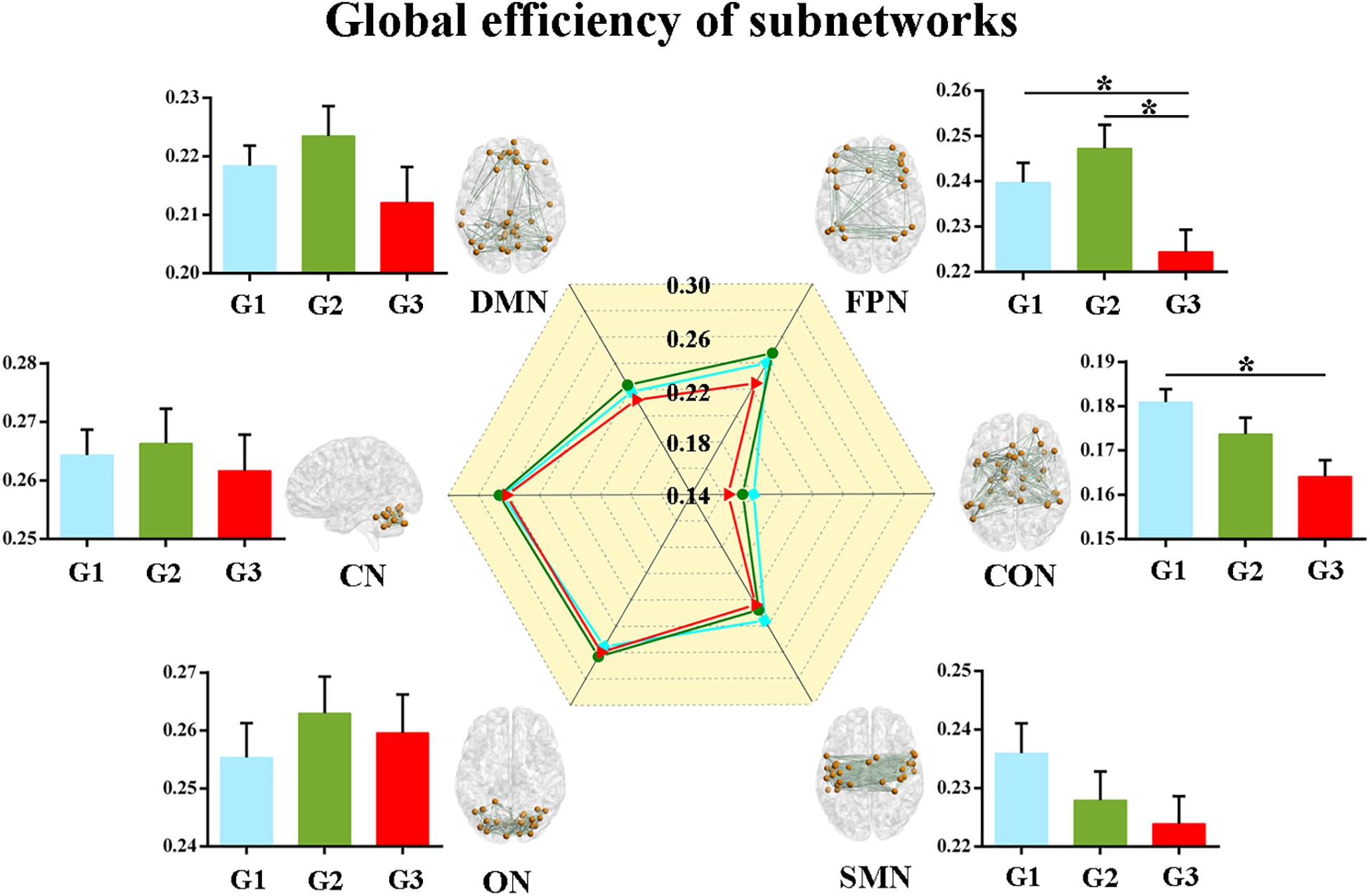
Figure 2. Global efficiency of the six subnetworks. WMH-CIND subjects showed lower global efficiency in the FPN than HCs and WMH-NC subjects (ANOVA, P = 0.004; Bonferroni-corrected, P < 0.05/3). The global efficiency of the CON in WMH-CIND subjects was significantly lower than that in HCs (ANOVA, P = 0.003; Bonferroni-corrected, P < 0.05/3). Abbreviations: HC, healthy control; NC, normal cognition; CIND, cognitive impairment without dementia; WMH, white matter hyperintensities; AUC, area under the curve; DMN, default mode network; FPN, fronto-parietal network; CON, cingulo-opercular network; SMN, sensorimotor network; ON, occipital network; CN, cerebellum network; G1 represents the HC group; G2 represents the WMH-NC group; and G3 represents the WMH-CIND group. ∗ indicates a statistical difference between groups.
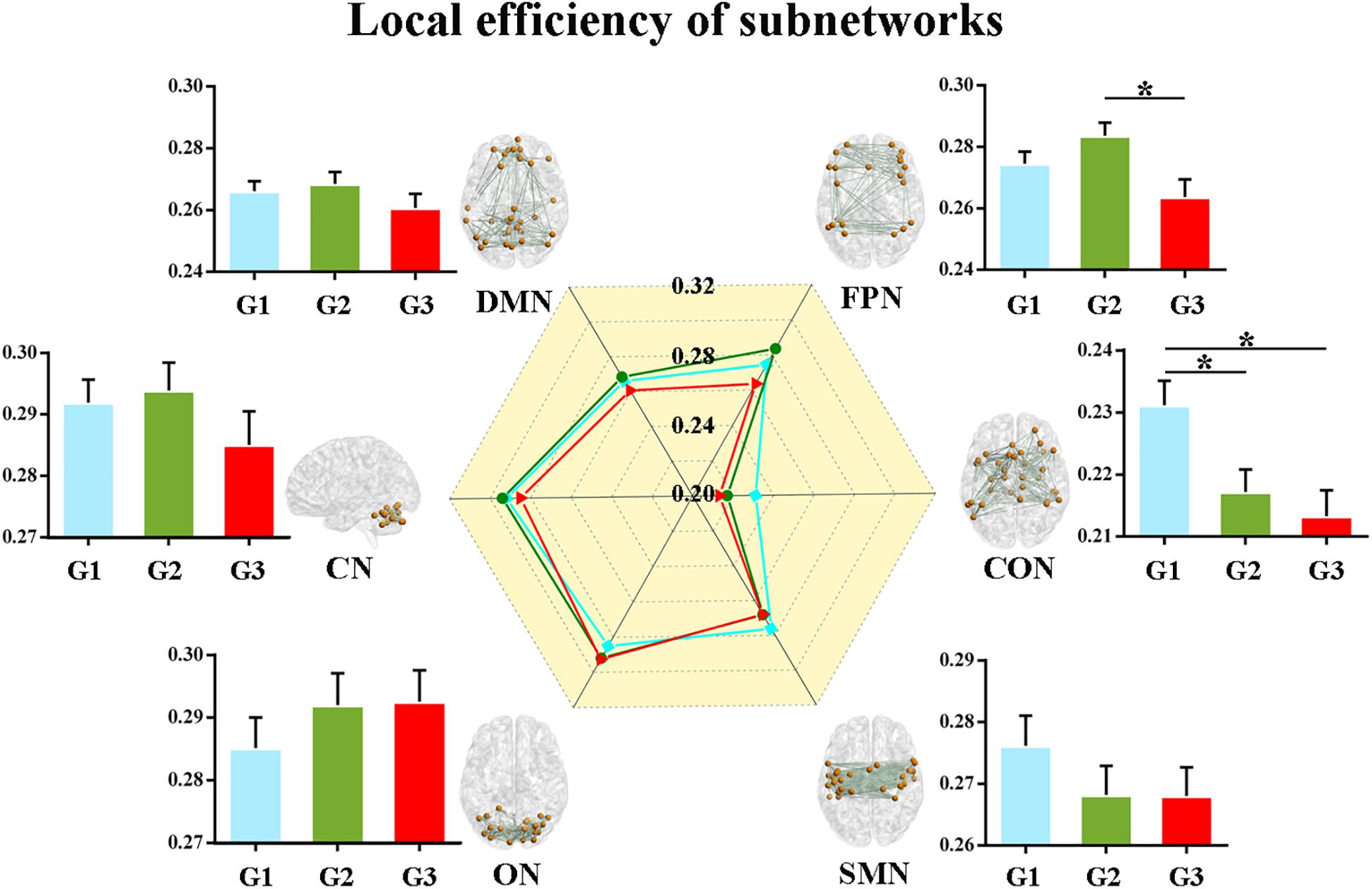
Figure 3. Local efficiency of the six subnetworks. Significantly decreased local efficiency in the FPN in WMH-CIND subjects compared with WMH-NC subjects was found (ANOVA, P = 0.014; Bonferroni-corrected, P < 0.05/3). HCs showed higher local efficiency in the CON than WMH-NC and WMH-CIND subjects (ANOVA, P = 0.004; Bonferroni-corrected, P < 0.05/3). Abbreviations: HC, healthy control; NC, normal cognition; CIND, cognitive impairment without dementia; WMH, white matter hyperintensities; AUC, area under the curve; DMN, default mode network; FPN, fronto-parietal network; CON, cingulo-opercular network; SMN, sensorimotor network; ON, occipital network; CN, cerebellum network; G1 represents the HC group; G2 represents the WMH-NC group; and G3 represents the WMH-CIND group. ∗ indicates a statistical difference between groups.
Alterations in Node-Level Efficiency
As we previously did for network-level efficiency, the AUC of nodal global and local efficiency were compared for each node. Out of the 160 ROIs, nine nodes showed significant group differences in nodal global efficiency, while no nodes were found to be significantly different in terms of nodal local efficiency (P < 0.05, FDR corrected). These nine nodes included the left dorsolateral prefrontal cortex (DLPFC), left ventral frontal cortex, left basal ganglia, left precentral gyrus, left angular gyrus, right dorsal anterior cingulate cortex, right anterior insular cortex, right precentral gyrus and right intraparietal sulcus (Figure 4).
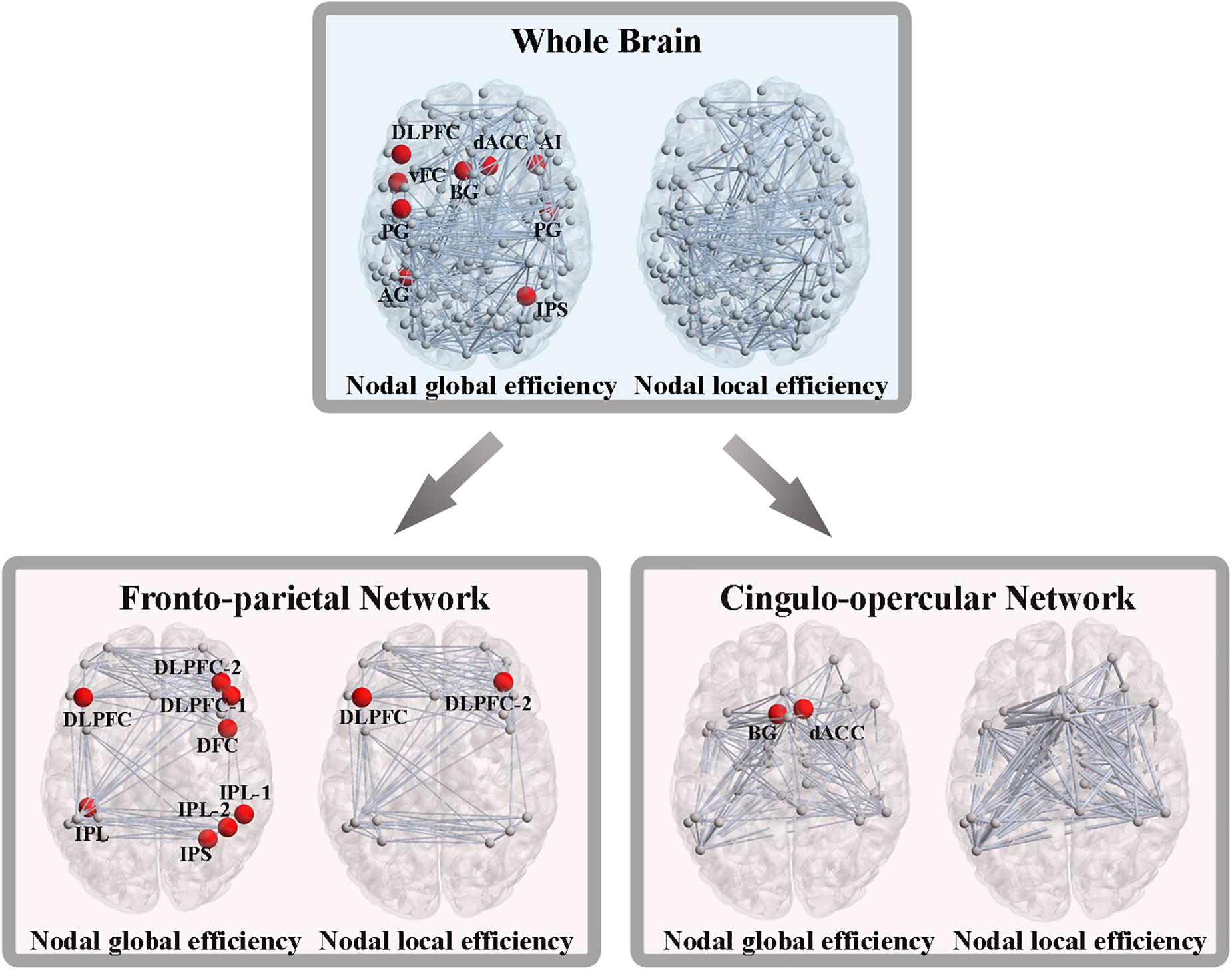
Figure 4. Altered nodal global and local efficiency. Abbreviations: DLPFC, dorsolateral prefrontal cortex; vFC, ventral frontal cortex; BG, basal ganglia; PG, precentral gyrus; AG, angular gyrus; dACC, dorsal anterior cingulate cortex; AI, anterior insular cortex; IPS, intraparietal sulcus; IPL, inferior parietal lobule; DFC, dorsal frontal cortex.
From the perspective of the subnetworks, we have distinguished the significantly altered global and local efficiency of the FPN and CON. Next, at the node level of the FPN, eight nodes exhibited significant group differences in nodal global efficiency, and two nodes showed significant differences in nodal local efficiency (P < 0.05, FDR corrected) (Figure 4). At the node level of the CON, two nodes showed significant alterations in nodal global efficiency, while no nodes were found to be different in terms of nodal local efficiency (P < 0.05, FDR corrected) (Figure 4).
Relationship Among WMH Volumes, Functional Efficiency Metrics, and Cognition
We performed multiple linear regression analyses to investigate the relationships among WMH volumes, functional efficiency metrics and cognition, adjusted for potential confounders (age, gender and education) in WMH-CIND subjects. First, we found that higher total WMH volumes and PWMH volumes were associated with lower scores for executive function (β = −0.398, P = 0.011; β = −0.353, P = 0.017, respectively) and information processing speed (β = −0.397, P = 0.017; β = −0.471, P = 0.002, respectively) (more details in Supplementary Table S1). Second, higher PWMH volumes were associated with lower nodal global efficiency in the left inferior parietal lobule (IPL) (β = −0.489, P = 0.006) and right dorsal frontal cortex (DFC) (β = −0.371, P = 0.039) (more details in Supplementary Table S2). Third, functional efficiency metrics were associated with cognitive scores for information processing speed (nodal global efficiency of the left DLPFC: β = 0.501, P = 0.003; nodal global efficiency of the left IPL in FPN: β = 0.419, P = 0.003; nodal global efficiency of the right DFC in FPN: β = 0.438, P = 0.004) (more details in Supplementary Table S3).
Additionally, we conducted mediation analyses to explore whether functional efficiency metrics mediated the relationships between WMH volumes and cognition in WMH-CIND subjects, adjusting for age, gender and education. We found that the association between PWMH volume and the TMT-A score was significantly mediated by nodal global efficiency in the right DFC in the FPN (indirect effect: −0.26; 95% CI: −0.74, −0.01) (Figure 5A). A multiple mediator model demonstrated that the indirect effect of PWMH on the Stroop B test was significantly mediated by nodal global efficiency in the left IPL in the FPN and in the left DLPFC (total indirect effect: −0.42; 95% CI: −1.12, −0.05) (Figure 5B). The nodal global efficiency of the left IPL and right DFC in the FPN and the left DLPFC mediated the association between PWMH volume and Stroop A performance (total indirect effect: −0.52; 95% CI: −1.15, −0.08) and information processing speed (total indirect effect: −0.36; 95% CI: −0.96, −0.003) Figures 5C,D).
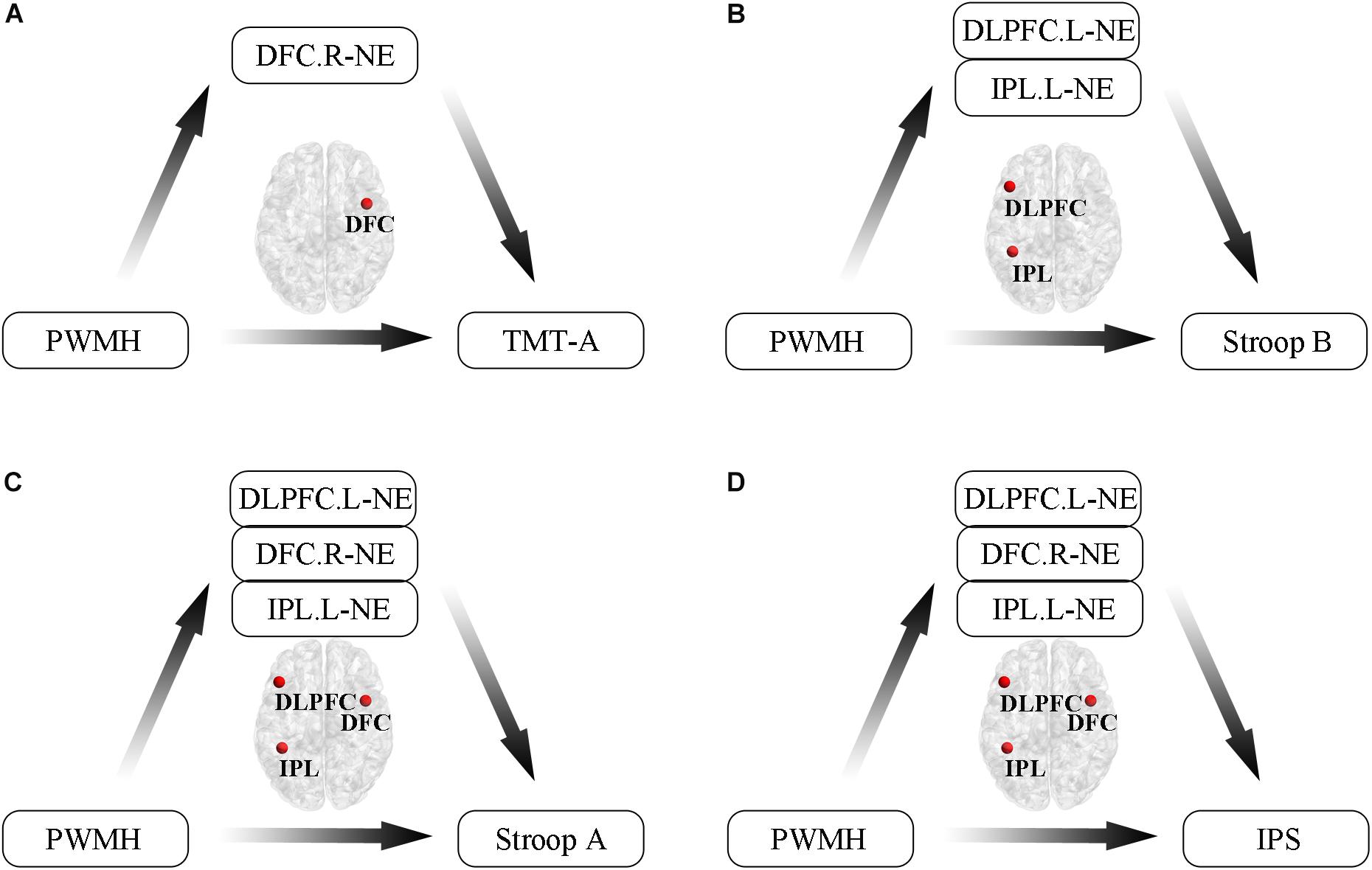
Figure 5. Mediation analyses to explore relationships between WMH volumes, functional efficiency metrics and cognition in WMH-CIND subjects. (A) Association between PWMH volume and TMT-A score was significantly mediated by nodal global efficiency in the right DFC in the FPN (indirect effect: –0.26; 95% CI: –0.74, –0.01). (B) Indirect effect of PWMH on Stroop B performance was significantly mediated by nodal global efficiency in the left IPL in the FPN and the left DLPFC (total indirect effect: –0.42; 95% CI: –1.12, –0.05). (C,D) The nodal global efficiency in the left IPL and the right DFC in the FPN and the left DLPFC mediated the association between PWMH volume and Stroop A performance (total indirect effect: –0.52; 95% CI: –1.15, –0.08) and information processing speed (total indirect effect: –0.36; 95% CI: –0.96, –0.003). Abbreviation: CIND, cognitive impairment without dementia; WMH, white matter hyperintensities; PWMH, periventricular-white matter hyperintensities; DLPFC.L, left dorsolateral prefrontal cortex; DFC.R, right dorsal frontal cortex; IPL.L, left inferior parietal lobule; NE, nodal global efficiency; TMT-A, trail making test-A; Stroop B, Stroop Color and Word Tests B; IPS, information processing speed.
Discriminative Analysis Based on Functional Efficiency Metrics
Using the classifier trained on differential functional efficiency metrics (Figures 1–4) and the leave-one-out cross-validation, SVM-based multivariate pattern analysis was able to classify the WMH-CIND subjects from subjects with only WMH with an accuracy of 81.08%, a sensitivity of 76.32% and a specificity of 86.11%. The ROC curve of the classifier was yielded, as shown in Figure 6. The area under the ROC curve was 0.86, indicating good classification power.
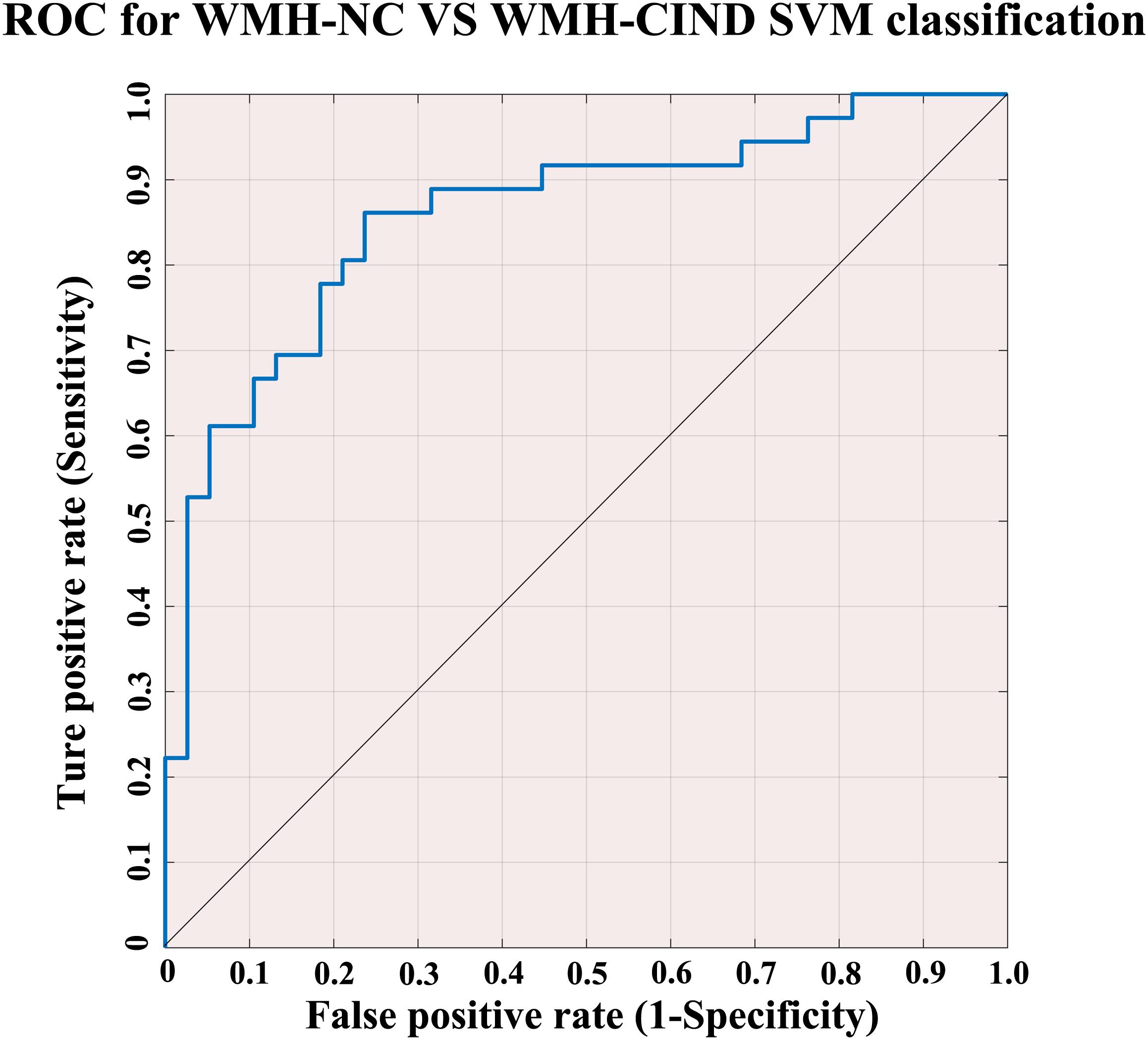
Figure 6. ROC for WMH-NC group vs. WMH-CIND group SVM classification. SVM analysis to classify the WMH-CIND subjects from subjects with only WMH with an accuracy of 81.08%, a sensitivity of 76.32% and a specificity of 86.11%. The area under the ROC curve was 0.86. Abbreviations: NC, normal cognition; CIND, cognitive impairment without dementia; WMH, white matter hyperintensities; SVM, support vector machine; ROC, receiver operating characteristic curve.
Discussion
The present study first investigated topological patterns of resting state functional networks in WMH subjects with and without CI using a graph theory-based analysis method. The results showed that (i) WMH-CIND subjects displayed significant decreases in global efficiency at the levels of the whole brain, specific subnetworks (the FPN and CON) and certain nodes (mainly located in the FPN and CON), as well as decreased local efficiency in the abovementioned subnetworks and nodes; (ii) periventricular white matter hyperintensity (PWMH)-related impairment of information processing speed is mediated by a reduction in nodal global efficiency (brain regions involved: DLPFC. L, DFC. R, and IPL); and (iii) alterations in brain functional efficiency served as a useful neuroimaging marker for the identification of WMH-CIND subjects. Our findings added to the growing notion that alterations in large-scale brain functional networks might contribute to the onset of vascular CI.
Significant Decreases in Connectivity Efficiency at Different Levels in WMH-CIND Subjects
As a direct topological measure of functional integration, global efficiency represents the capacity for parallel processing from distributed brain regions, while functional segregation refers to specialized processing within densely interconnected groups (the so called “modules”) and can be quantified by local efficiency (Rubinov and Sporns, 2010). As shown in Figures 1–4, the significant reduction in global efficiency in WMH-CIND subjects suggested potential damage to long association fibers that, in turn, impaired communication between crucial neural networks, especially those responsible for executive function and information processing speed (Ds, 2004; Sun et al., 2011; Schaefer et al., 2014). This damage finally gave rise to an impaired integration ability in WMH-CIND subjects’ brains. Similar results found in local efficiency reflect damage to neighborhood relationships between different nodes in the same modules, leading to a decrease in the level of specialized processing capacity. Specifically, involved brain regions were mainly located in the FPN and CON, and the alterations in both global efficiency and local efficiency revealed strong positive correlations with certain neuropsychological test scores (TMT-A, Stroop A/B and information processing speed tests). The FPN maintains and promotes homeostatic balance across multiple functional networks and serves as a flexible hub according to task demands (Cole et al., 2014). Previous findings showed that both the FPN and CON are related to information processing speed, executive function and attention (Niendam et al., 2012; Posner et al., 2016). The present findings confirmed the association between CI and functional alterations in large-scale brain networks in WMH subjects.
Interestingly, we found that there was no remarkable difference in the local efficiency between the WMH-CIND group, the WMH-NC group and the HC group at the whole brain level. A possible explanation is that some nodes may have intergroup differential local efficiency with statistical significance while others do not, which is confirmed by the following nodal efficiency analysis. Since global local efficiency is the average value of nodal local efficiency, a “neutralization reaction” may occur in the process of mean calculation. The more nodes involved, the more complicated the “neutralization” effect will be, eventually leading to an unpredictable or variable local efficiency value at the integral level. Moreover, compared to healthy subjects, WMH-NC subjects did not display altered functional connectivity patterns in our study, possibly suggesting a compensatory or maladaptive mechanism to maintain cognitive performance in those with mild pathological changes and symptoms (Prins and Scheltens, 2015). In addition, we did not observe the expected alterations in functional connectivity efficiency in the default mode network (DMN), which has been reported in several studies (Spreng et al., 2013; Dey et al., 2016). This inconsistency may result from different analytical methods. Voxel-wise FC analyses were performed in previous studies, while the present study focused on ROI-wise FC patterns.
Mediation of the Association Between PWMH and Information Processing Speed Deficits by Nodal Global Efficiency
Previous studies have demonstrated that a disrupted topological organization of nodes is strongly correlated with CI or dementia, but the potential mechanisms are still unclear (Wen et al., 2005; Tuladhar et al., 2015; Yi et al., 2015). Here, we conduct a mediation analysis to further investigate the effect of nodal efficiency on the positive correlation between WMH burden and CI. We found that PWMH-related impairment of information processing speed is mediated by the reduction in nodal global efficiency, with corresponding brain regions (DLPFC. L, DFC. R, and IPL. L) involved (De Simoni et al., 2018). Similar studies elaborate on the relevance of information processing speed and injury to the above regions. DLPFC was considered an important control area to modulate the processing of social cognition and emotional cues (Weissman et al., 2008; Vanderhasselt et al., 2013), which can preserve cognitive tasks when emotional disturbances occur (Wessa et al., 2013). In addition, transcranial direct current stimulation to the left DLPFC can significantly increase impaired cognitive control in depression, which further illustrates its importance in information processing speed (Plewnia et al., 2015). The role of the IPL in meaning processing was confirmed by parametric manipulation (Chou et al., 2009), while the dorsal portion of the right inferior frontal cortex, especially the inferior frontal junction, is associated with specific attentional function, which is a major facet of information processing (Sebastian et al., 2016). Information processing speed, an important subdomain of human cognitive ability, is now commonly used as a behavioral indicator of the integrity of cognitive function and is specifically cited as a key component within the diagnostic framework for neurocognitive disorders in the DSM-5 (Li et al., 2010; Torrens-Burton et al., 2017). Reduced information processing speed is regarded as a highly robust feature of CI (Salthouse and Ferrer-Caja, 2003), and their relationship has been widely underpinned by numerous studies linking measured alterations in information processing speed to brain structure lesions (Wulfing et al., 2005; Jacobs et al., 2013; Hong et al., 2015). In addition, the structural network analysis also demonstrated that white matter microstructural damages were associated with the impaired information processing speed and the network global efficiency was shown to mediate the relationship between the white matter damage and cognitive dysfunction (Lawrence et al., 2014). From the functional network perspective, our study not only demonstrated that functional network efficiency played the “mediator” role, but also emphasized the importance of left dorsolateral prefrontal cortex, right dorsal frontal cortex, and left inferior parietal lobule in the mediation analysis.
Information processing speed is commonly impaired in subjects with WMH, and the mediation effects by nodal efficiency may describe the potential mechanisms underlying the link. The mediation effects of nodal efficiency were mainly found in frontal and parietal regions, the core nodes in the FPN. As PWMH burden increased, the nodal efficiency in the frontal and parietal regions decreased, suggesting that the FPN was disrupted. Then, the FPN could not integrate ongoing information across multiple functional networks very efficiently. Thus, information processing speed is commonly impaired in subjects with high PWMH burden.
Compared to previous studies, we set relatively tight inclusion criteria to eliminate the influence of irrelevant variables such as non-vascular factors, dementia and psychiatric symptoms as much as possible, ensuring, to a large extent, homogeneity across samples from the same group. Meanwhile, we also explored the internal relationships between diverse WMH burden levels and specific cognitive domains in a spatially oriented manner by applying the visual rating of WMH according to Scheltens et al. (Xu et al., 2019), which improves the relative accuracy of our study to a degree.
Clustering Analysis Based on Support Vector Machine and Automatic Classification of WMH Patients
The early identification of WMH-CIND plays a vital role in the prevention of the onset and progression of CI and dementia. Several diagnostic approaches and potential detection methods have been developed (Van Maurik et al., 2017), including standard neuropsychological tests (Bai et al., 2010; Xu et al., 2010), neuroimaging markers (structural MRI markers, including those for hippocampal atrophy, ventricular volume and whole brain atrophy) and PET-CT markers (Nettiksimmons et al., 2010; Arbizu et al., 2018; Caminiti et al., 2018), electrophysiological tests (Imperatori et al., 2019) and biomarkers in cerebrospinal fluid (P-tau, tau, Aβ peptides, and Aβ42/P-tau ratio) (Van Harten et al., 2011; Llorens et al., 2016), with their respective strengths and weaknesses, as well as different prognostic accuracies and specificities. In recent years, resting-state fMRI (rs-fMRI) and diffusion MR markers have been increasingly used in the investigation of new markers of CI (Franzmeier et al., 2017). Based on our findings of intergroup FC differences, we evaluated their impact on the classification of WMH-CIND and WMH-NC by applying a machine learning algorithm called SVM analysis. Recently, Zhu et al. (2019) applied the altered whole-brain functional connections as the features to identify the WMH-CIND. From the “network property” perspective in our study, the differential functional efficiency metrics shows its unique value with an accuracy/sensitivity/specificity of 80.3%/ 76.5%/ 84.4%, respectively, highlighting the superior classificatory role of fMRI-SVM based automatic identification of WMH-CIND patients. To some extent, the topological properties may provide more information reflecting neural communication. I think the two approaches were complementary and both need to be applied in the future research. This strategy may have potential application value for clinical diagnosis.
Limitations
Several limitations in this study need to be further addressed. First, the sample size was relatively small, which might lead to insufficient statistical power. Second, the topological organization of brain networks is affected by different parcellation strategies. The Dosenbach’s atlas used in this study was derived from a series of meta-analyses of task-related fMRI studies. Recently, two novel brain-wide graphs (264 ROIs reported in Power et al. (2011) and 333 cortical surface parcels reported in Gordon et al. (2016) may be used to further assess the suitability in the identification of WMH-CIND subjects. Third, a multicenter longitudinal study should be designed instead of simple cross-sectional research considering inevitable individual variation, and an individualized evaluation system for disease progression in WMH patients will ultimately be formulated in the future. Lastly, in this study, we only compared WMH with CIND and WMH without CIND, but didn’t involve CIND without WMH. We would add those subjects in the further studies.
Conclusion
Our findings demonstrated that decreased global efficiency and local efficiency, especially in the FPN and CON, were prominently related to CI in WMH subjects, and decreased nodal global efficiency also mediated the association between WMH burden and CI. Discriminative analysis based on functional efficiency metrics further highlighted the superior classificatory role of large-scale functional networks in the identification of CI in WMH subjects. These findings provide novel insights into the altered large-scale brain functional networks of WMH subjects and may contribute to the investigation of markers of CI in the population.
Data Availability Statement
The datasets generated for this study are available on request to the corresponding author.
Ethics Statement
The studies involving human participants were reviewed and approved by the Ethics Committee of Nanjing Drum Tower Hospital. The patients/participants provided their written informed consent to participate in this study. Written informed consent was obtained from the individual(s) for the publication of any potentially identifiable images or data included in this article.
Author Contributions
YX conceived and designed the experiments. HC, LH, DY, and QY performed the experiments. HC and LH analyzed the data. HC, LH, and YX drafted the manuscript. YX revised the manuscript. HC, LH, DY, QY, MG, RQ, CL, ML, LY, BZ, and YX collected the data and contributed the materials and analysis tools.
Funding
This work was supported by the National Key Research and Development Program of China (2016YFC1300504 and 2016YFC0901004), the National Natural Science Foundation of China (81920108017 and 81630028), the Key Research and Development Program of Jiangsu Province of China (BE2016610), and Jiangsu Province Key Medical Discipline (ZDXKA2016020).
Conflict of Interest
The authors declare that the research was conducted in the absence of any commercial or financial relationships that could be construed as a potential conflict of interest.
Supplementary Material
The Supplementary Material for this article can be found online at: https://www.frontiersin.org/articles/10.3389/fnagi.2019.00347/full#supplementary-material
Footnotes
- ^ https://sourceforge.net/projects/w2mhs
- ^ http://www.fil.ion.ucl.ac.uk/spm
- ^ http://www.nitrc.org/projects/gretna/
- ^ https://sites.google.com/site/bctnet/
- ^ http://www.csie.ntu.edu.tw/~cjlin/libsvm/
References
Arbizu, J., Festari, C., Altomare, D., Walker, Z., Bouwman, F., Rivolta, J., et al. (2018). Clinical utility of FDG-PET for the clinical diagnosis in MCI. Eur. J. Nucl. Med. Mol. Imaging 45, 1497–1508. doi: 10.1007/s00259-018-4039-7
Bai, L., Tian, J., Zhong, C., Xue, T., You, Y., Liu, Z., et al. (2010). Acupuncture modulates temporal neural responses in wide brain networks: evidence from fMRI study. Mol Pain 6:73. doi: 10.1186/1744-8069-6-73
Banerjee, G., Wilson, D., Jager, H. R., and Werring, D. J. (2016). Novel imaging techniques in cerebral small vessel diseases and vascular cognitive impairment. Biochim. Biophys. Acta 1862, 926–938. doi: 10.1016/j.bbadis.2015.12.010
Brundel, M., De Bresser, J., Van Dillen, J. J., Kappelle, L. J., and Biessels, G. J. (2012). Cerebral microinfarcts: a systematic review of neuropathological studies. J. Cereb. Blood Flow Metab. 32, 425–436. doi: 10.1038/jcbfm.2011.200
Brundel, M., Kwa, V. I., Bouvy, W. H., Algra, A., Kappelle, L. J., and Biessels, G. J. (2014). Cerebral microbleeds are not associated with long-term cognitive outcome in patients with transient ischemic attack or minor stroke. Cerebrovasc. Dis. 37, 195–202. doi: 10.1159/000358119
Caminiti, S. P., Ballarini, T., Sala, A., Cerami, C., Presotto, L., Santangelo, R., et al. (2018). FDG-PET and CSF biomarker accuracy in prediction of conversion to different dementias in a large multicentre MCI cohort. Neuroimage Clin. 18, 167–177. doi: 10.1016/j.nicl.2018.01.019
Chou, T. L., Chen, C. W., Wu, M. Y., and Booth, J. R. (2009). The role of inferior frontal gyrus and inferior parietal lobule in semantic processing of Chinese characters. Exp. Brain Res. 198, 465–475. doi: 10.1007/s00221-009-1942-y
Cole, M. W., Repovs, G., and Anticevic, A. (2014). The frontoparietal control system: a central role in mental health. Neuroscientist 20, 652–664. doi: 10.1177/1073858414525995
De Leeuw, F. E., De Groot, J. C., Achten, E., Oudkerk, M., Ramos, L. M., Heijboer, R., et al. (2001). Prevalence of cerebral white matter lesions in elderly people: a population based magnetic resonance imaging study. The rotterdam scan study. J. Neurol. Neurosurg. Psychiatry 70, 9–14. doi: 10.1136/jnnp.70.1.9
De Simoni, S., Jenkins, P. O., Bourke, N. J., Fleminger, J. J., Hellyer, P. J., Jolly, A. E., et al. (2018). Altered caudate connectivity is associated with executive dysfunction after traumatic brain injury. Brain 141, 148–164. doi: 10.1093/brain/awx309
Debette, S., and Markus, H. S. (2010). The clinical importance of white matter hyperintensities on brain magnetic resonance imaging: systematic review and meta-analysis. BMJ 341:c3666. doi: 10.1136/bmj.c3666
Dey, A. K., Stamenova, V., Turner, G., Black, S. E., and Levine, B. (2016). Pathoconnectomics of cognitive impairment in small vessel disease: a systematic review. Alzheimers Dement. 12, 831–845. doi: 10.1016/j.jalz.2016.01.007
Ds, C. (2004). Psychobiological mechanisms of resilience and vulnerability: implications for successful adaptation to extreme stress. Am. J. Psychiatry 161, 195–216. doi: 10.1176/appi.ajp.161.2.195
Franzmeier, N., Caballero, M. A. A., Taylor, A. N. W., Simon-Vermot, L., Buerger, K., Ertl-Wagner, B., et al. (2017). Resting-state global functional connectivity as a biomarker of cognitive reserve in mild cognitive impairment. Brain Imaging Behav. 11, 368–382. doi: 10.1007/s11682-016-9599-1
Gordon, E. M., Laumann, T. O., Adeyemo, B., Huckins, J. F., Kelley, W. M., and Petersen, S. E. (2016). Generation and evaluation of a cortical area parcellation from resting-state correlations. Cereb. Cortex 26, 288–303. doi: 10.1093/cercor/bhu239
Gorelick, P. B., Scuteri, A., Black, S. E., Decarli, C., Greenberg, S. M., Iadecola, C., et al. (2011). Vascular contributions to cognitive impairment and dementia: a statement for healthcare professionals from the american heart association/american stroke association. Stroke 42, 2672–2713. doi: 10.1161/str.0b013e3182299496
Gu, Y., Liu, R., Qin, R., Chen, X., Zou, J., Jiang, Y., et al. (2019). Characteristic changes in the default mode network in hypertensive patients with cognitive impairment. Hypertens. Res. 42, 530–540. doi: 10.1038/s41440-018-0176-4
Heise, V., Filippini, N., Trachtenberg, A. J., Suri, S., Ebmeier, K. P., and Mackay, C. E. (2014). Apolipoprotein E genotype, gender and age modulate connectivity of the hippocampus in healthy adults. Neuroimage 98, 23–30. doi: 10.1016/j.neuroimage.2014.04.081
Heringa, S. M., Reijmer, Y. D., Leemans, A., Koek, H. L., Kappelle, L. J., and Biessels, G. J. (2014). Multiple microbleeds are related to cerebral network disruptions in patients with early Alzheimer’s disease. J. Alzheimers Dis. 38, 211–221. doi: 10.3233/JAD-130542
Hong, Z., Ng, K. K., Sim, S. K., Ngeow, M. Y., Zheng, H., Lo, J. C., et al. (2015). Differential age-dependent associations of gray matter volume and white matter integrity with processing speed in healthy older adults. Neuroimage 123, 42–50. doi: 10.1016/j.neuroimage.2015.08.034
Hurford, R., Charidimou, A., Fox, Z., Cipolotti, L., Jager, R., and Werring, D. J. (2014). MRI-visible perivascular spaces: relationship to cognition and small vessel disease MRI markers in ischaemic stroke and TIA. J. Neurol. Neurosurg. Psychiatry 85, 522–525. doi: 10.1136/jnnp-2013-305815
Imperatori, C., Farina, B., Adenzato, M., Valenti, E. M., Murgia, C., Marca, G. D., et al. (2019). Default mode network alterations in individuals with high-trait-anxiety: an EEG functional connectivity study. J. Affect. Disord. 246, 611–618. doi: 10.1016/j.jad.2018.12.071
Jacobs, H. I., Leritz, E. C., Williams, V. J., Van Boxtel, M. P., Van Der Elst, W., Jolles, J., et al. (2013). Association between white matter microstructure, executive functions, and processing speed in older adults: the impact of vascular health. Hum. Brain Mapp. 34, 77–95. doi: 10.1002/hbm.21412
Jokinen, H., Kalska, H., Ylikoski, R., Madureira, S., Verdelho, A., Van Der Flier, W. M., et al. (2009). Longitudinal cognitive decline in subcortical ischemic vascular disease–the LADIS Study. Cerebrovasc. Dis. 27, 384–391. doi: 10.1159/000207442
Kim, H. J., Im, K., Kwon, H., Lee, J. M., Kim, C., Kim, Y. J., et al. (2015). Clinical effect of white matter network disruption related to amyloid and small vessel disease. Neurology 85, 63–70. doi: 10.1212/WNL.0000000000001705
Lampe, L., Kharabian-Masouleh, S., Kynast, J., Arelin, K., Steele, C. J., Loffler, M., et al. (2019). Lesion location matters: the relationships between white matter hyperintensities on cognition in the healthy elderly. J. Cereb. Blood Flow Metab. 39, 36–43. doi: 10.1177/0271678X17740501
Lawrence, A. J., Chung, A. W., Morris, R. G., Markus, H. S., and Barrick, T. R. (2014). Structural network efficiency is associated with cognitive impairment in small-vessel disease. Neurology 83, 304–311. doi: 10.1212/WNL.0000000000000612
Li, W., Prakash, R., Kelly-Cobbs, A. I., Ogbi, S., Kozak, A., El-Remessy, A. B., et al. (2010). Adaptive cerebral neovascularization in a model of type 2 diabetes: relevance to focal cerebral ischemia. Diabetes Metab. Res. Rev. 59, 228–235. doi: 10.2337/db09-0902
Liu, R., Chen, H., Qin, R., Gu, Y., Chen, X., Zou, J., et al. (2019). The altered reconfiguration pattern of brain modular architecture regulates cognitive function in cerebral small vessel disease. Front. Neurol. 10:324. doi: 10.3389/fneur.2019.00324
Llorens, F., Schmitz, M., Ferrer, I., and Zerr, I. (2016). CSF biomarkers in neurodegenerative and vascular dementias. Prog. Neurobiol. 13, 36–53. doi: 10.1016/j.pneurobio.2016.03.003
Lu, J., Li, D., Li, F., Zhou, A., Wang, F., Zuo, X., et al. (2011). Montreal cognitive assessment in detecting cognitive impairment in Chinese elderly individuals: a population-based study. J. Geriatr. Psychiatry Neurol. 24, 184–190. doi: 10.1177/0891988711422528
Nettiksimmons, J., Harvey, D., Brewer, J., Carmichael, O., Decarli, C., Jack, C. R., et al. (2010). Subtypes based on cerebrospinal fluid and magnetic resonance imaging markers in normal elderly predict cognitive decline. Neurobiol. Aging 31, 1419–1428. doi: 10.1016/j.neurobiolaging.2010.04.025
Nichols, L. M., Masdeu, J. C., Mattay, V. S., Kohn, P., Emery, M., Sambataro, F., et al. (2012). Interactive effect of apolipoprotein e genotype and age on hippocampal activation during memory processing in healthy adults. Arch. Gen. Psychiatry 69, 804–813. doi: 10.1001/archgenpsychiatry.2011.1893
Niendam, T. A., Laird, A. R., Ray, K. L., Dean, Y. M., Glahn, D. C., and Carter, C. S. (2012). Meta-analytic evidence for a superordinate cognitive control network subserving diverse executive functions. Cogn. Affect. Behav. Neurosci. 12, 241–268. doi: 10.3758/s13415-011-0083-5
Patel, B., and Markus, H. S. (2011). Magnetic resonance imaging in cerebral small vessel disease and its use as a surrogate disease marker. Int. J. Stroke 6, 47–59. doi: 10.1111/j.1747-4949.2010.00552.x
Plewnia, C., Schroeder, P. A., Kunze, R., Faehling, F., and Wolkenstein, L. (2015). Keep calm and carry on: improved frustration tolerance and processing speed by transcranial direct current stimulation (tDCS). PLoS One 10:e0122578. doi: 10.1371/journal.pone.0122578
Posner, M. I., Rothbart, M. K., and Voelker, P. (2016). Developing brain networks of attention. Curr. Opin. Pediatr. 28, 720–724. doi: 10.1097/mop.0000000000000413
Power, J. D., Cohen, A. L., Nelson, S. M., Wig, G. S., Barnes, K. A., Church, J. A., et al. (2011). Functional network organization of the human brain. Neuron 72, 665–678.
Prins, N. D., and Scheltens, P. (2015). White matter hyperintensities, cognitive impairment and dementia: an update. Nat. Rev. Neurol. 11, 157–165. doi: 10.1038/nrneurol.2015.10
Raman, M. R., Preboske, G. M., Przybelski, S. A., Gunter, J. L., Senjem, M. L., Vemuri, P., et al. (2014). Antemortem MRI findings associated with microinfarcts at autopsy. Neurology 82, 1951–1958. doi: 10.1212/WNL.0000000000000471
Rubinov, M., and Sporns, O. (2010). Complex network measures of brain connectivity: uses and interpretations. Neuroimage 52, 1059–1069. doi: 10.1016/j.neuroimage.2009.10.003
Salthouse, T. A., and Ferrer-Caja, E. (2003). What needs to be explained to account for age-related effects on multiple cognitive variables? Psychol. Aging 18, 91–110. doi: 10.1037/0882-7974.18.1.91
Schaefer, A., Quinque, E. M., Kipping, J. A., Arelin, K., Roggenhofer, E., Frisch, S., et al. (2014). Early small vessel disease affects frontoparietal and cerebellar hubs in close correlation with clinical symptoms–a resting-state fMRI study. J. Cereb. Blood Flow Metab. 34, 1091–1095. doi: 10.1038/jcbfm.2014.70
Sebastian, A., Jung, P., Neuhoff, J., Wibral, M., Fox, P. T., Lieb, K., et al. (2016). Dissociable attentional and inhibitory networks of dorsal and ventral areas of the right inferior frontal cortex: a combined task-specific and coordinate-based meta-analytic fMRI study. Brain Struct. Funct. 221, 1635–1651. doi: 10.1007/s00429-015-0994-y
Sheline, Y. I., and Raichle, M. E. (2013). Resting state functional connectivity in preclinical Alzheimer’s disease. Biol. Psychiatry 74, 340–347. doi: 10.1016/j.biopsych.2012.11.028
Smith, S. M., Fox, P. T., Miller, K. L., Glahn, D. C., Fox, P. M., Mackay, C. E., et al. (2009). Correspondence of the brain’s functional architecture during activation and rest. Proc. Natl. Acad. Sci. U.S.A. 106, 13040–13045. doi: 10.1073/pnas.0905267106
Spreng, R. N., Sepulcre, J., Turner, G. R., Stevens, W. D., and Schacter, D. L. (2013). Intrinsic architecture underlying the relations among the default, dorsal attention, and frontoparietal control networks of the human brain. J. Cogn. Neurosci. 25, 74–86. doi: 10.1162/jocn_a_00281
Sudo, F. K., Alves, C. E., Alves, G. S., Ericeira-Valente, L., Tiel, C., Moreira, D. M., et al. (2013). White matter hyperintensities, executive function and global cognitive performance in vascular mild cognitive impairment. Arq. Neuropsiquiatr. 71, 431–436. doi: 10.1590/0004-282X20130057
Sun, Y. W., Qin, L. D., Zhou, Y., Xu, Q., Qian, L. J., Tao, J., et al. (2011). Abnormal functional connectivity in patients with vascular cognitive impairment, no dementia: a resting-state functional magnetic resonance imaging study. Behav. Brain Res. 223, 388–394. doi: 10.1016/j.bbr.2011.05.006
Ter Telgte, A., Van Leijsen, E. M. C., Wiegertjes, K., Klijn, C. J. M., Tuladhar, A. M., and De Leeuw, F. E. (2018). Cerebral small vessel disease: from a focal to a global perspective. Nat. Rev. Neurol. 14, 387–398. doi: 10.1038/s41582-018-0014-y
Torrens-Burton, A., Basoudan, N., Bayer, A. J., and Tales, A. (2017). Perception and reality of cognitive function: information processing speed, perceived memory function, and perceived task difficulty in older adults. J. Alzheimers Dis. 60, 1601–1609. doi: 10.3233/JAD-170599
Tuladhar, A. M., Van Norden, A. G., De Laat, K. F., Zwiers, M. P., Van Dijk, E. J., Norris, D. G., et al. (2015). White matter integrity in small vessel disease is related to cognition. Neuroimage Clin. 7, 518–524. doi: 10.1016/j.nicl.2015.02.003
Tuladhar, A. M., Van Uden, I. W., Rutten-Jacobs, L. C., Lawrence, A., Van Der Holst, H., Van Norden, A., et al. (2016). Structural network efficiency predicts conversion to dementia. Neurology 86, 1112–1119. doi: 10.1212/wnl.0000000000002502
Van Den Heuvel, M. P., Mandl, R. C., Kahn, R. S., and Hulshoff Pol, H. E. (2009). Functionally linked resting-state networks reflect the underlying structural connectivity architecture of the human brain. Hum. Brain Mapp. 30, 3127–3141. doi: 10.1002/hbm.20737
Van Dijk, E. J., Prins, N. D., Vrooman, H. A., Hofman, A., Koudstaal, P. J., and Breteler, M. M. (2008). Progression of cerebral small vessel disease in relation to risk factors and cognitive consequences: rotterdam scan study. Stroke 39, 2712–2719. doi: 10.1161/STROKEAHA.107.513176
Van Harten, A. C., Kester, M. I., Visser, P. J., Blankenstein, M. A., Pijnenburg, Y. A., Van Der Flier, W. M., et al. (2011). Tau and p-tau as CSF biomarkers in dementia: a meta-analysis. Clin. Chem. Lab. Med. 49, 353–366. doi: 10.1515/CCLM.2011.086
Van Maurik, I. S., Zwan, M. D., Tijms, B. M., Bouwman, F. H., Teunissen, C. E., Scheltens, P., et al. (2017). Interpreting biomarker results in individual patients with mild cognitive impairment in the Alzheimer’s biomarkers in daily practice (ABIDE) project. JAMA Neurol. 74, 1481–1491. doi: 10.1001/jamaneurol.2017.2712
Vanderhasselt, M. A., Baeken, C., Van Schuerbeek, P., Luypaert, R., and De Raedt, R. (2013). Inter-individual differences in the habitual use of cognitive reappraisal and expressive suppression are associated with variations in prefrontal cognitive control for emotional information: an event related fMRI study. Biol. Psychol. 92, 433–439. doi: 10.1016/j.biopsycho.2012.03.005
Weissman, D. H., Perkins, A. S., and Woldorff, M. G. (2008). Cognitive control in social situations: a role for the dorsolateral prefrontal cortex. Neuroimage 40, 955–962. doi: 10.1016/j.neuroimage.2007.12.021
Wen, D. S., Wu, J. Z., Fu, S. M., Liu, B., Li, X. X., and Dong, J. J. (2005). [Effects of 17 beta-estradiol on the adhesion, invasion and motility potential of salivary mucoepidermoid carcinoma Mc3 cells]. Zhonghua Kou Qiang Yi Xue Za Zhi 40, 390–393.
Wessa, M., Heissler, J., Schonfelder, S., and Kanske, P. (2013). Goal-directed behavior under emotional distraction is preserved by enhanced task-specific activation. Soc. Cogn. Affect. Neurosci. 8, 305–312. doi: 10.1093/scan/nsr098
Wu, Y. F., Wu, W. B., Liu, Q. P., He, W. W., Ding, H., Nedelska, Z., et al. (2016). Presence of lacunar infarctions is associated with the spatial navigation impairment in patients with mild cognitive impairment: a DTI study. Oncotarget 7, 78310–78319. doi: 10.18632/oncotarget.13409
Wulfing, P., Gotte, M., Sonntag, B., Kersting, C., Schmidt, H., Wulfing, C., et al. (2005). Overexpression of Endothelin-A-receptor in breast cancer: regulation by estradiol and cobalt-chloride induced hypoxia. Int. J. Oncol. 26, 951–960.
Xu, G. Y., Wang, F., Jiang, X., and Tao, J. (2010). Aquaporin 1, a potential therapeutic target for migraine with aura. Mol. Pain 6:68. doi: 10.1186/1744-8069-6-68
Xu, J., Van Dam, N. T., Feng, C., Luo, Y., Ai, H., Gu, R., et al. (2019). Anxious brain networks: a coordinate-based activation likelihood estimation meta-analysis of resting-state functional connectivity studies in anxiety. Neurosci. Biobehav. Rev. 96, 21–30. doi: 10.1016/j.neubiorev.2018.11.005
Xu, X., Gao, Y., Liu, R., Qian, L., Chen, Y., Wang, X., et al. (2018). Progression of white matter hyperintensities contributes to lacunar infarction. Aging Dis. 9, 444–452. doi: 10.14336/AD.2017.0808
Yao, M., Herve, D., Jouvent, E., Duering, M., Reyes, S., Godin, O., et al. (2014). Dilated perivascular spaces in small-vessel disease: a study in CADASIL. Cerebrovasc. Dis. 37, 155–163. doi: 10.1159/000356982
Yi, L. Y., Liang, X., Liu, D. M., Sun, B., Ying, S., Yang, D. B., et al. (2015). Disrupted topological organization of resting-state functional brain network in subcortical vascular mild cognitive impairment. CNS Neurosci. Ther. 21, 846–854. doi: 10.1111/cns.12424
Yu, J., Li, J., and Huang, X. (2012). The beijing version of the montreal cognitive assessment as a brief screening tool for mild cognitive impairment: a community-based study. BMC Psychiatry 12:156. doi: 10.1186/1471-244X-12-156
Zhu, W., Huang, H., Yang, S., Luo, X., Zhu, W., Xu, S., et al. (2019). Dysfunctional Architecture Underlies White Matter Hyperintensities with and without Cognitive Impairment. J. Alzheimers Dis. 71, 461–476. doi: 10.3233/JAD-190174
Keywords: cognitive impairment, cingulo-opercular network, functional efficiency, fronto-parietal network, white matter hyperintensity
Citation: Chen H, Huang L, Yang D, Ye Q, Guo M, Qin R, Luo C, Li M, Ye L, Zhang B and Xu Y (2019) Nodal Global Efficiency in Front-Parietal Lobe Mediated Periventricular White Matter Hyperintensity (PWMH)-Related Cognitive Impairment. Front. Aging Neurosci. 11:347. doi: 10.3389/fnagi.2019.00347
Received: 15 September 2019; Accepted: 28 November 2019;
Published: 10 December 2019.
Edited by:
Marcelo L. Berthier, University of Málaga, SpainReviewed by:
Yong Liu, Institute of Automation (CAS), ChinaSeong Hye Choi, Inha University, South Korea
Copyright © 2019 Chen, Huang, Yang, Ye, Guo, Qin, Luo, Li, Ye, Zhang and Xu. This is an open-access article distributed under the terms of the Creative Commons Attribution License (CC BY). The use, distribution or reproduction in other forums is permitted, provided the original author(s) and the copyright owner(s) are credited and that the original publication in this journal is cited, in accordance with accepted academic practice. No use, distribution or reproduction is permitted which does not comply with these terms.
*Correspondence: Yun Xu, xuyun20042001@aliyun.com
†These authors have contributed equally to this work