- 1RAPID Facility, Department of Civil & Environmental Engineering, University of Washington, Seattle, WA, United States
- 2Evans School of Public Policy & Governance, University of Washington, Seattle, WA, United States
- 3Department of Human-Centered Design and Engineering, University of Washington, Seattle, WA, United States
- 4Geomatics Lab, School of Civil and Construction Engineering, Oregon State University, Corvallis, OR, United States
- 5Department of Civil and Coastal Engineering, University of Florida, Gainesville, FL, United States
- 6Center for Coastal Studies and Department of Civil & Environmental Engineering, Virginia Tech, Blacksburg, VA, United States
- 7Applied Physics Laboratory, University of Washington, Seattle, WA, United States
- 8Formerly, RAPID Facility, Department of Civil & Environmental Engineering, University of Washington, Seattle, WA, United States
Natural hazards and disaster reconnaissance investigations have provided many lessons for the research and practice communities and have greatly improved our scientific understanding of extreme events. Yet, many challenges remain for these communities, including improving our ability to model hazards, make decisions in the face of uncertainty, enhance community resilience, and mitigate risk. State-of-the-art instrumentation and mobile data collection applications have significantly advanced the ability of field investigation teams to capture quickly perishable data in post-disaster settings. The NHERI RAPID Facility convened a community workshop of experts in the professional, government, and academic sectors to determine reconnaissance data needs and opportunities, and to identify the broader challenges facing the reconnaissance community that hinder data collection and use. Participants highlighted that field teams face many practical and operational challenges before and during reconnaissance investigations, including logistics concerns, safety issues, emotional trauma, and after-returning, issues with data processing and analysis. Field teams have executed many effective missions. Among the factors contributing to successful reconnaissance are having local contacts, effective teamwork, and pre-event training. Continued progress in natural hazard reconnaissance requires adaptation of new, strategic approaches that acquire and integrate data over a range of temporal, spatial, and social scales across disciplines.
Introduction
Natural hazards and disaster reconnaissance investigations have led to important discoveries that have greatly improved our scientific understanding of hazards and their physical, social, and environmental consequences. For example, findings from one of the earliest field reconnaissance missions in the United States, the Lawson and Reid (1908) investigation of the 1906 ∼M7.9 San Francisco earthquake, led to the development of the landmark theory of elastic rebound (Reid, 1910), among other significant scientific and engineering advancements (Ellsworth, 1990). More recently, post-event reconnaissance investigations have provided new, fundamental knowledge essential for the development of computational models to simulate the physical and socioeconomic impacts of natural hazards, and for identifying ways that communities can restore their infrastructure, rebuild their built environment, and recover their socioeconomic capital (e.g., Xiao and Van Zandt, 2012; Xiao and Peacock, 2014; Cong et al., 2018; Kang et al., 2018; Nejat et al., 2019). Far from an uncaring or indifferent data-gathering exercise in the face of tragedy, reconnaissance campaigns are at their core “a humanitarian mission in the broadest sense” (Kaplan, 2010).
Natural hazards, such as wind events (i.e., tornadoes and coastal storms, including wind-generated waves and surges), earthquakes (and secondary effects such as shaking-induced damage to buildings and infrastructure, soil liquefaction and co-seismic landslides, and tsunamis), landslides, and volcanic eruptions, produce an extraordinary volume and quality of data that can inform our preparation and response to future events (Nature Geoscience, 2017). Such data are often highly ephemeral or “perishable” since they may be altered or removed during rescue and recovery activities, or by natural agents such as precipitation or wind following an event. Therefore, reconnaissance data must be collected soon after an event occurs. These data are also unique because they inherently include the real-world complexities (e.g., the interplay between natural, human, and built systems) that allow us to better understand and quantify the socio-technical dimensions related to damage, restoration, and resiliency of the built environment; such data are difficult to duplicate in a traditional laboratory setting. Reconnaissance data, once collected, processed, curated, and archived (Rathje et al., 2017), may be used and reused for a range of purposes, including (i) making discoveries and gaining fresh insights, (ii) testing and verifying models, (iii) reducing uncertainties in probabilistic models, and (iv) inspiring new simulation models, including new data-driven methods (e.g., Loggins et al., 2019).
In the past, reconnaissance investigators collected data and documented field observations using conventional recording and measurement tools, such as photography, note-taking, and surveying (Geotechnical Extreme Events Reconnaissance [GEER], 2014). Today, the availability of state-of-the-art instrumentation, mobile data collection technologies (e.g., RApp; Miles and Tanner, 2018; Berman et al., in press), training, and field support services, such as those provided by the Natural Hazards Engineering Research Infrastructure (NHERI) Natural Hazards Reconnaissance Facility (known as the RAPID) (Wartman et al., 2018; Berman et al., in press), has significantly advanced the ability of field investigation teams to capture perishable data in post-disaster settings.
This article briefly reviews the current state of natural hazards and disaster reconnaissance, including highlights from recent missions, difficulties teams face, and opportunities for progress. It then examines the grand challenges facing the natural hazards community and presents new approaches to meet these challenges through the strategic design, planning, and execution of reconnaissance campaigns. Many of the ideas presented in the article were developed with input from key stakeholders, including participants of a 2-day reconnaissance workshop, previous and current users of RAPID facility instrumentation, and other disciplinary experts in the professional, government, and academic sectors.
Natural Hazards and Disaster Reconnaissance
The history of natural hazard and disaster investigations spans many centuries. Interest in natural hazards, frequently by religious scholars, gathered momentum during the Renaissance and Reformation (14th to 16th centuries) when authorities began systematically cataloging earthquakes and other rare events such as plagues (Schenk, 2007; Tülüveli, 2015). Scholars often used these data in an attempt to reconcile extreme events with spiritual beliefs and religious concepts. Lawson and Reid (1908) comprehensive, two-volume report on the San Francisco, California earthquake (Figure 1) is one of the first rigorous scientific field studies of a major natural hazard (Ellsworth, 1990). A decade later, Prince (1920) conducted one of the first social sciences investigations of an extreme event, the Halifax, Nova Scotia, Canada explosion of a munitions ship in the city harbor. Social sciences studies of disasters became more systematic and formalized in the 1940s through the 1960s, largely due to work at the Disaster Research Center (Ohio State University), which was initially supported by the U.S. Office of Civil Defense to inform cold war civil defense efforts (e.g., Knowles, 2012). Earthquake Engineering Research Institute [EERI] (1971) conducted one of the first in-depth multidisciplinary investigations of a natural hazard event, the San Fernando, California earthquake.
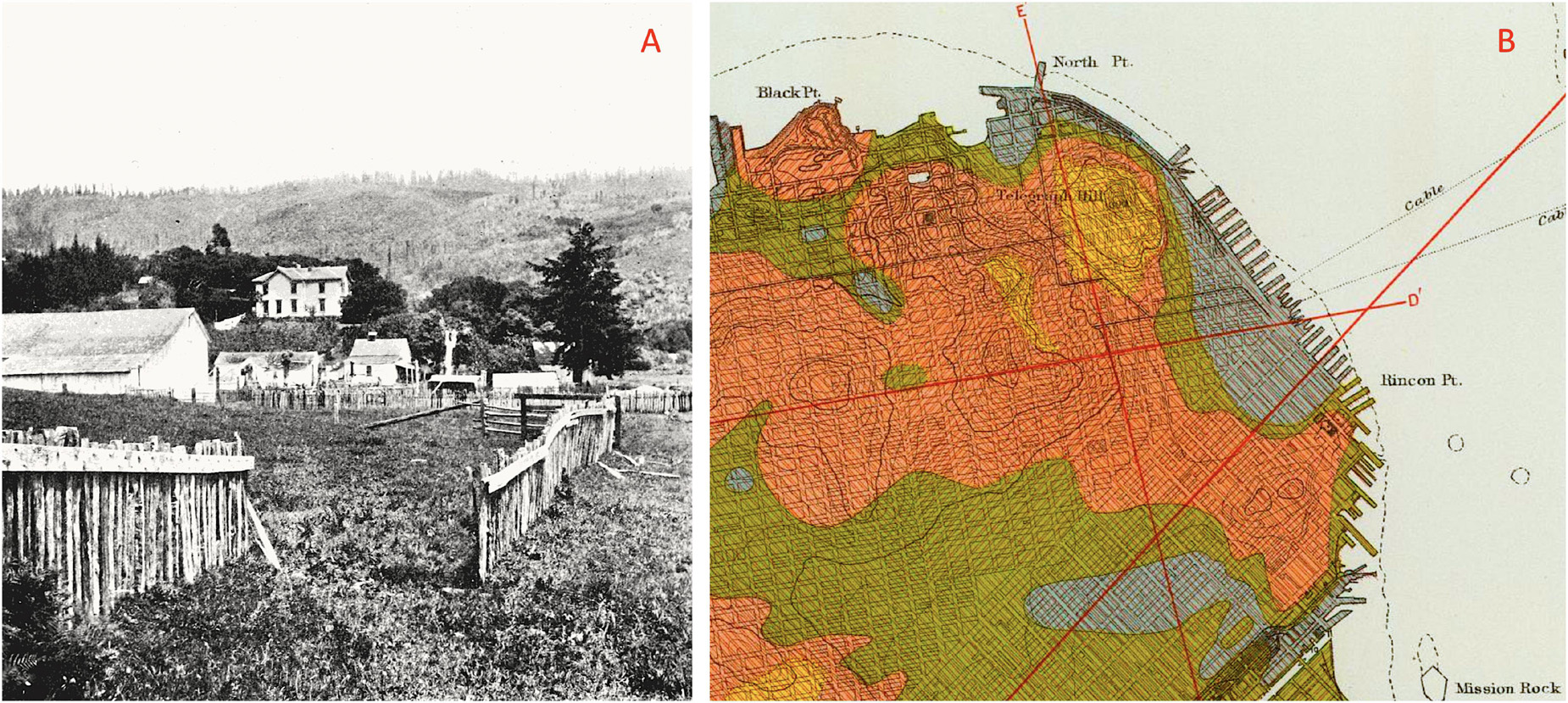
Figure 1. The Lawson and Reid (1908) reconnaissance investigation of the 1906 San Francisco earthquake led to significant scientific and engineering advancements. (A) Reconnaissance photograph showing fence offset by earthquake surface fault rupture near Bolinas, Marin County, CA, United States. This observation led to the development of the theory of elastic rebound (Reid, 1910). (B) Excerpt of “Map of San Francisco showing apparent intensity of the earthquake shock” (Lawson and Reid, 1908) showing area of high intensity shaking revealing the modern engineering concept of non-linear site response and effects (Gray and green tones depict areas of highest local shaking intensity). Both images are reproduced from Lawson and Reid (1908).
The EERI was one of the first professional organizations to formalize regular reconnaissance investigations of major seismic events by establishing the Learning from Earthquakes (LFE) program in 1973. Largely multidisciplinary in its approach, the LFE program deploys teams of geoscientists, engineers, and social scientists to investigate and observe the damaging effects of significant earthquakes worldwide. Recently, the LFE program has expanded to include a virtual earthquake reconnaissance teams, or “VERT,” that conduct rapid “virtual” (i.e., non-field based) assessments within 48 h of an earthquake (Fischer and Hakhamaneshi, 2019).
With the support of the U.S. National Science Foundation (NSF), the Geotechnical Extreme Events Reconnaissance (GEER) Association was formed in 1999 to conduct reconnaissance investigations of the geotechnical aspects of significant earthquakes in the U.S. and abroad (Bray et al., 2019). In 2011, GEER’s scope was expanded to include the study of the geotechnical aspects of other natural hazard events such as hurricanes, floods, and landslides (e.g., Dashti et al., 2014; Wartman et al., 2016; Hughes and Morales Vélez, 2017; Gallant et al., 2020; Montgomery et al., 2020). GEER authorizes research missions based upon (i) the opportunity to learn about new scientific hypotheses or engineering models, (ii) the availability of additional field data (e.g., ground motion recordings) to supplementary data gathered in the reconnaissance, and (iii), for international (non-U.S.) events, the potential for a similar event to occur in the future in the U.S (Geotechnical Extreme Events Reconnaissance [GEER], 2014). During the past several years, NSF began supporting other similar “extreme event reconnaissance (or research),” or EER, organizations including StEER (Structural Extreme Events Reconnaissance), OSEEER (Operations and Systems Engineering Extreme Events Research), SSEER (Social Science Extreme Events Research), ISEEER (Interdisciplinary Science and Engineering Extreme Events Research), NEER (Nearshore Extreme Events Reconnaissance), and SUstainable Material Management Extreme Events Reconnaissance (SUMMEER). These EER organizations are coordinated by CONVERGE (Peek et al., 2020), which seeks to advance ethically-grounded (Gaillard and Peek, 2019), scientifically rigorous, disciplinary, and interdisciplinary extreme events research.
There are other natural hazards reconnaissance organizations based at professional societies worldwide. The Earthquake Engineering Field Investigation Team (EEFIT), based in the United Kingdom, supports earthquake reconnaissance missions with the goals of making technical assessments, collecting geological and seismological data, assessing the effectiveness of earthquake protection systems, and investigating disaster management procedures and socioeconomic impacts (Stone et al., 2017). Italy hosts two organizations that have organized earthquake reconnaissance missions and conducted follow-on seismic policy analyses (e.g., Mazzoni et al., 2018), the Italian Network of University Laboratories for Earthquake Engineering (ReLUIS), and the European Centre for Training and Research in Earthquake Engineering (Eucentre). Elsewhere, the New Zealand Society for Earthquake Engineering (NZSEE) has supported reconnaissance investigations of earthquakes and major tsunamis worldwide for six decades (Wood P. R. et al., 2017). In Asia, the Asian Technical Committee (ATC3) “Geotechnology for Natural Hazards” has conducted reconnaissance missions following natural hazard events. Other organizations, such as the Nepalese Engineering Society, the Building Research Institute of Japan, among others, also conduct investigations in the region. Similarly, the American Society of Civil Engineers (ASCE) has supported reconnaissance missions in the U.S. and abroad through the primary society (e.g., Silva-Tulla and Nicholson, 2007) or its disciplinary institutes (e.g., Wartman et al., 2013).
In addition to these organizations, self-organized teams sometimes form in the aftermath of an event, often with a focused hypothesis-driven research question or inquiry, to collect data. Table 1 summarizes the objectives and outcomes of recent reconnaissance investigations of several representative natural hazard events. Figures 2 through 5 present field data collected during several of the missions highlighted in Table 1.
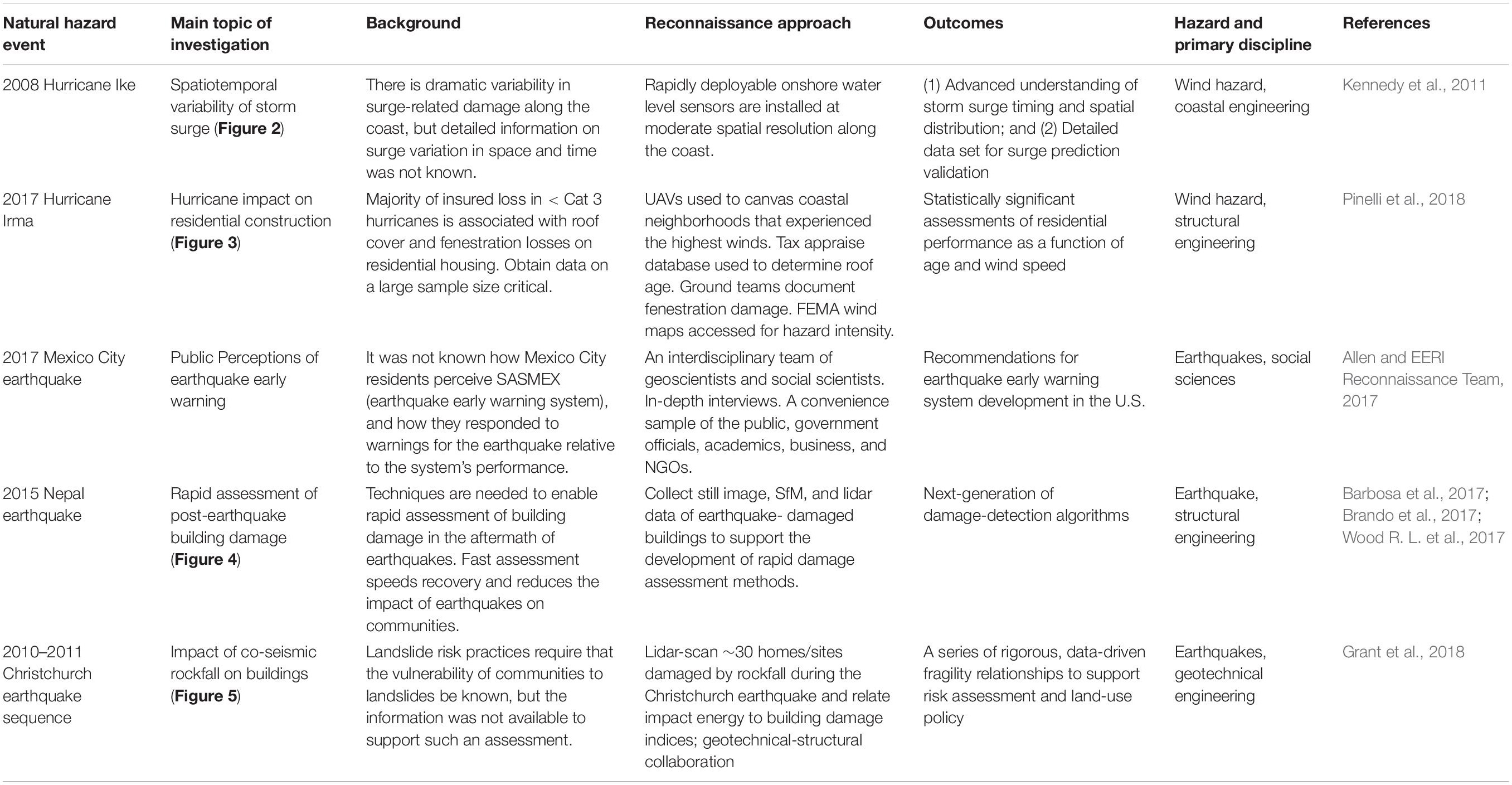
Table 1. Examples of reconnaissance approach, objectives, and outcomes from several recent earthquake and wind hazard missions (Figure 2).
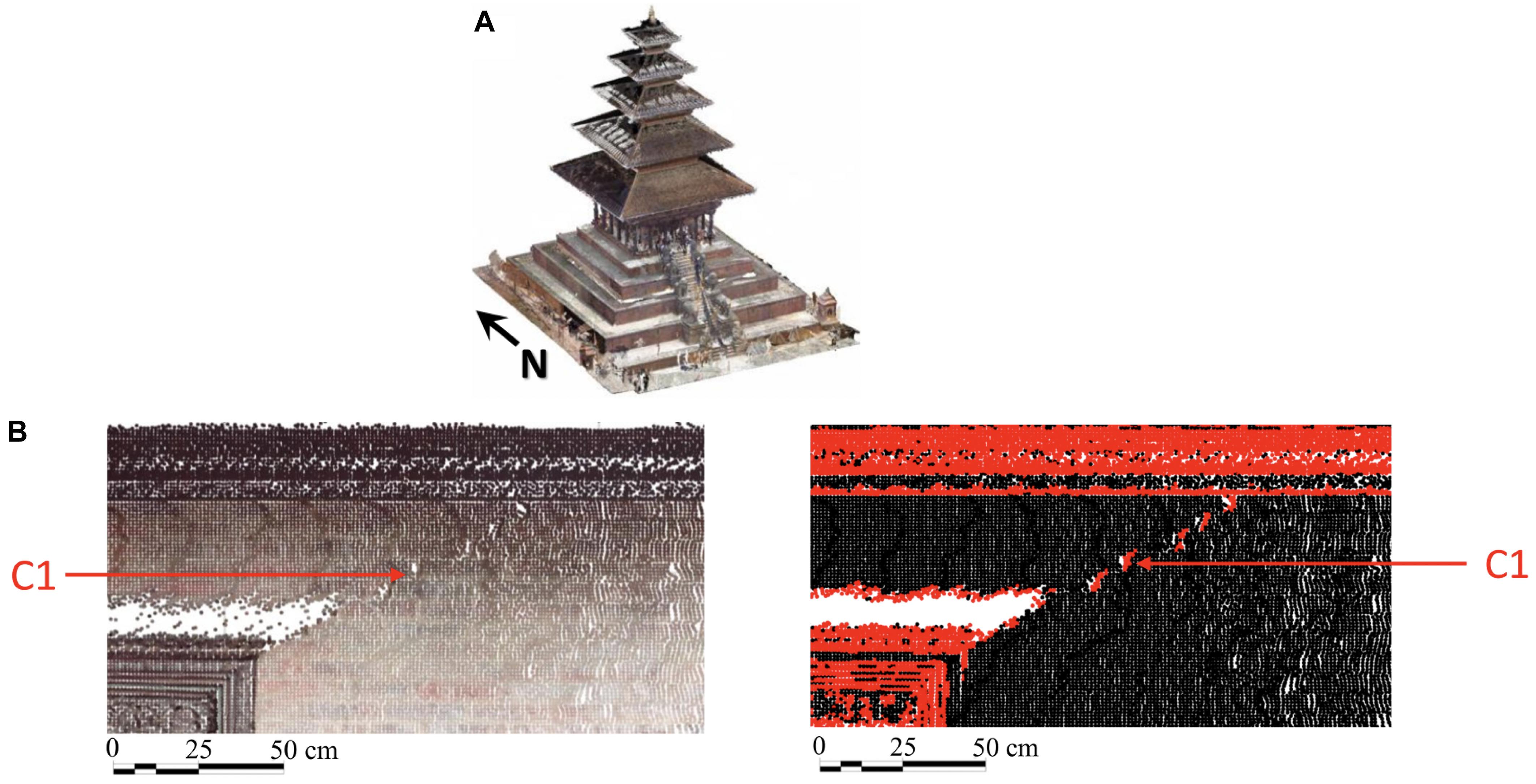
Figure 2. Assessing the performance of buildings using lidar data collected during reconnaissance. (A) Lidar-derived 3D model of Nyatapola Temple following the 2015 Ghorka Nepal Earthquake (B) Earthquake-induced crack (designated as “C1”) seen in a color point cloud (left) and detected defects shown in red (right). Reproduced from Wood P. R. et al. (2017).
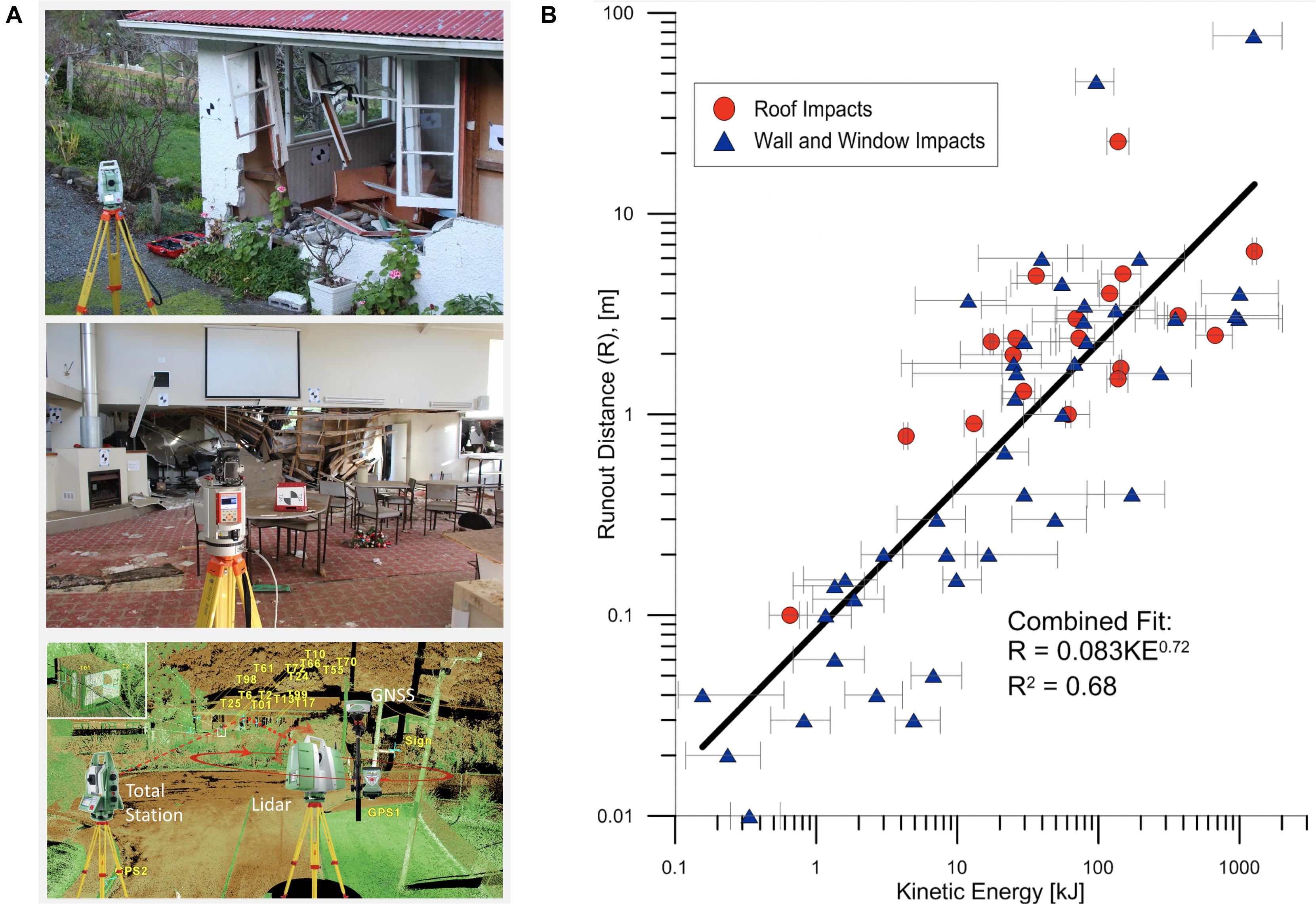
Figure 3. Reconnaissance investigation of the impact of rockfalls on dwellings during the 2011 Christchurch, New Zealand, earthquakes. (A) Lidar data was collected inside and outside buildings, geo-registered, then fusing into a single 3D model. (B) Field data reveals a direct correlation between rockfall impact energy and rock penetration into buildings. Modified from Grant et al. (2018).
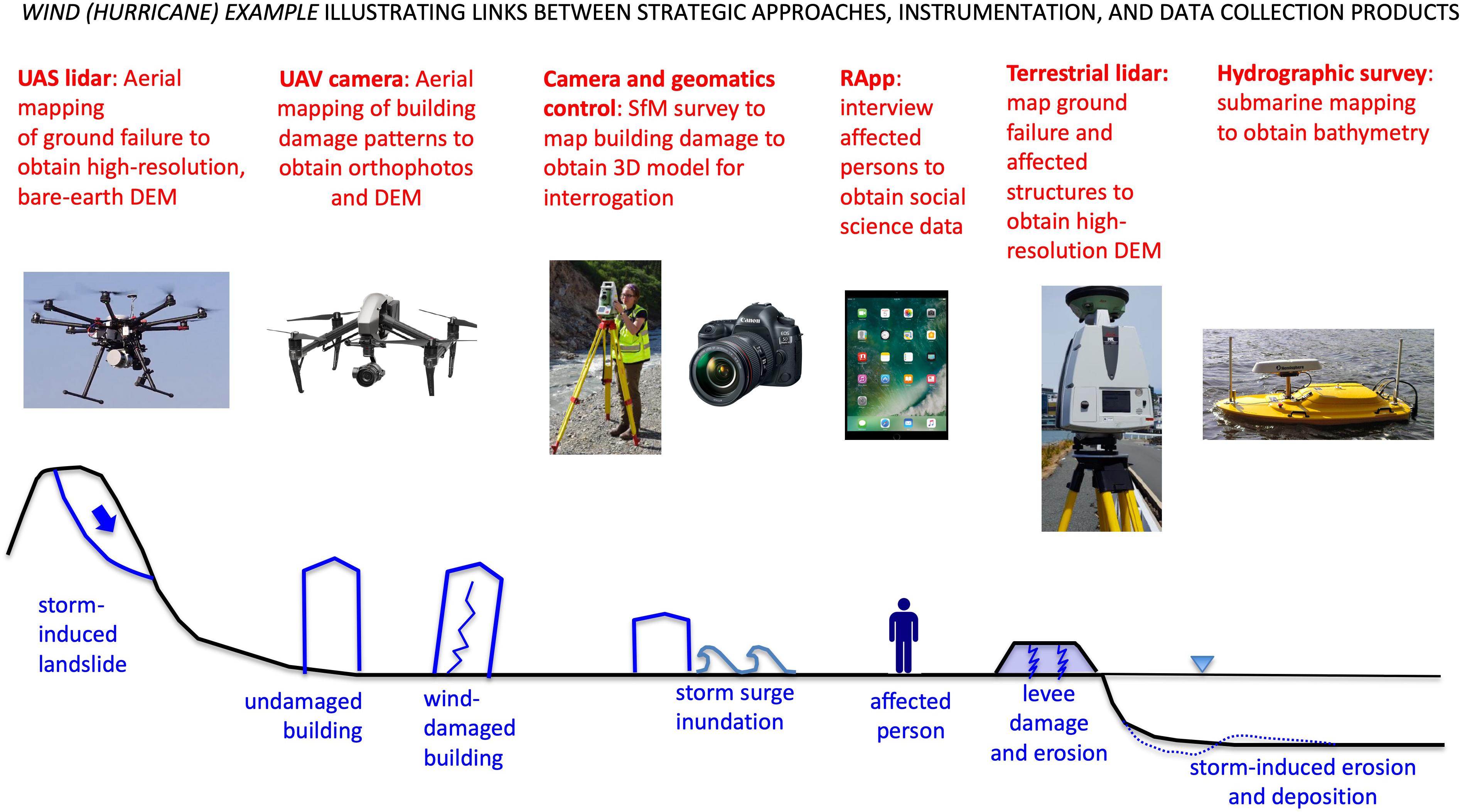
Figure 4. Diagram depicting damage features, secondary effects, and human and societal impacts that commonly result from an extreme wind event. The diagram is similar to Figure 7, illustrating the commonalities between seismic and wind natural hazard events. Diagrams and inset images are as noted in Figure 7.
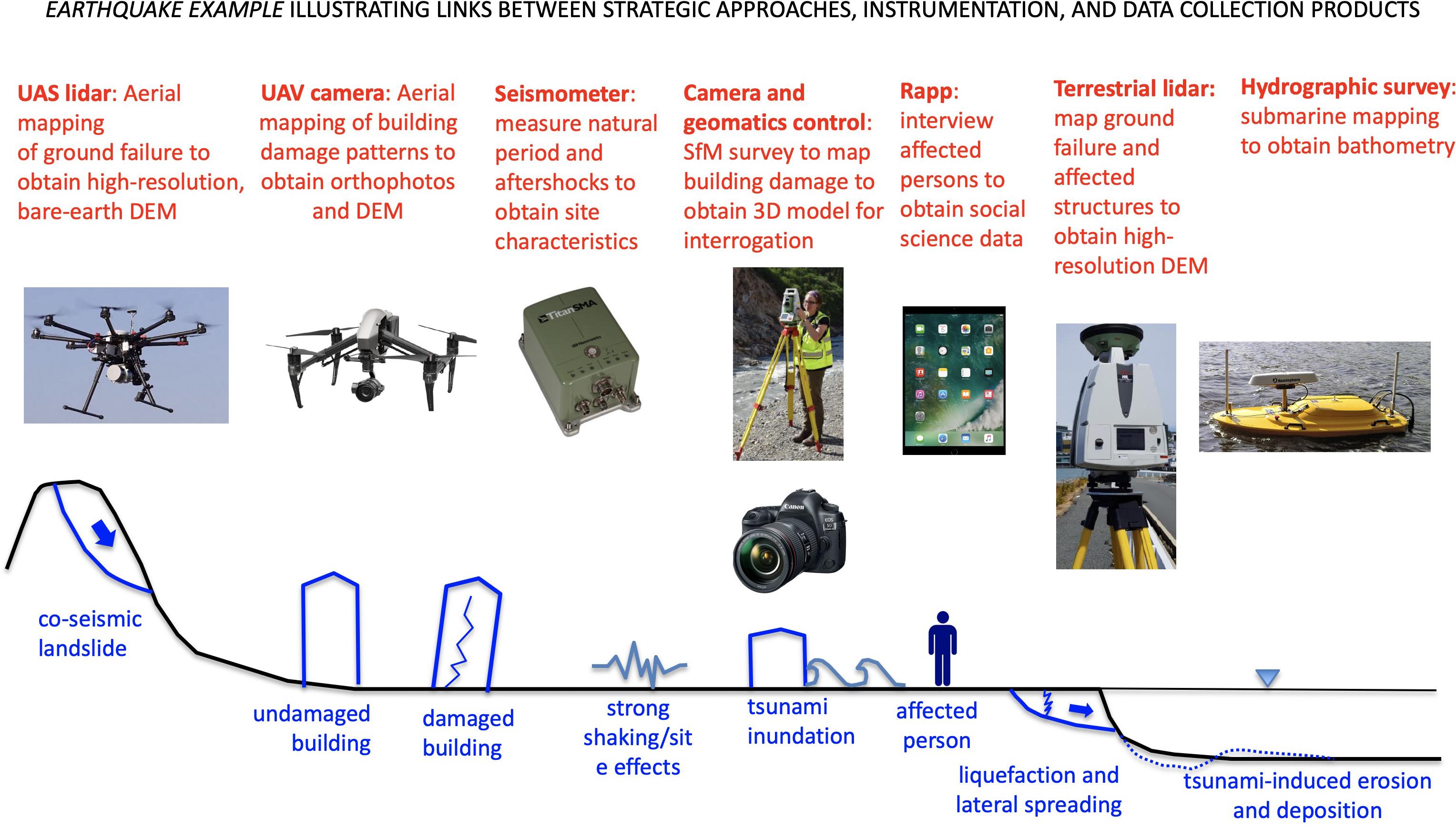
Figure 5. Diagram illustrating damage features, secondary effects, and human and societal impacts that often result from a significant earthquake (blue illustrations and accompanying text). Superimposed above this hypothetical post-event landscape are annotations linking instrumentation (shown with inset photographs) and data collections activities and products (shown in red) to event features.
Reconnaissance Instrumentation and Natural Hazard Simulation
By enabling the prompt collection of high-resolution data sets, advanced reconnaissance instrumentation now plays a central role in providing the academic, research, and professional communities with an unprecedented volume of high-quality, open-source, engineering, geophysical, social, and behavioral data. In addition, new software and cyberinfrastructure tools allow complex data sets to be archived, integrated, explored, and visualized (Rathje et al., 2017). These computational resources facilitate collaboration among experts across different fields to support advancements at the intersections of the natural hazards specialty disciplines. A unique aspect of the RAPID Facility is its portfolio of geospatial, image-centric data collection instrumentation. High-resolution georeferenced laser, image, and video data collected from full fields of view (i.e., top to bottom; inside and outside) of infrastructure within affected regions support the development of 3D post-event models (Berman et al., in press). Such models can be safely interrogated to extensive detail by geographically distributed research teams—an aspect that allows investigators the time and vision to collaboratively continue to discover new and important aspects of the impact of the surveyed event (Olsen and Kayen, 2013; Olsen et al., 2015). These types of terrestrial data sets are increasingly being fused with broader scale satellite imagery to appreciate the regional context for damage at a specific site (e.g., Yamazaki and Matsuoka, 2007; Eguchi et al., 2008; Rathje and Franke, 2016; Gallant et al., 2020).
Modeling and simulation lie at the center of the natural hazard community’s broader goal to understand, simulate, and predict the performance of built, natural, and social systems during and after natural hazards events (Edge et al., 2020). Over the past decade, a portfolio of highly sophisticated natural hazards models has significantly improved our ability to simulate the effects of extreme events across a wide range of spatial and temporal scales (e.g., Roelvink et al., 2009; Dietrich et al., 2011; LeVeque et al., 2011; Pita et al., 2013; Mandli and Dawson, 2014; Yim et al., 2014; Baradaranshoraka et al., 2019). These natural hazards models have become increasingly data-driven, requiring comprehensive data sets to capture complex, system-level responses. Examples of such models include performance-based earthquake engineering (PBEE) design methods and resilience-based design methods (e.g., FEMA, 2018; McAllister et al., 2019), which require fragility data to relate structural, non-structural, and infrastructure systems performance to engineering demand parameters, and stochastic wind hazard loss models (Hamid et al., 2011; Pita et al., 2015) that require field data to better calibrate and validate the hazard, infrastructure vulnerability, costing components, and economic impacts of preparedness and mitigation policies.
The RAPID Facility’s principal scientific goal is to inform natural hazards computational simulation models, infrastructure performance assessment, and economic impact analysis by supporting the collection, development, and assessment of high-quality disaster data sets (Figure 6). These data sets help advance our fundamental understanding of natural hazards and their impacts. Examples of reconnaissance data collection required to improve the natural hazards modeling and simulation include the following:
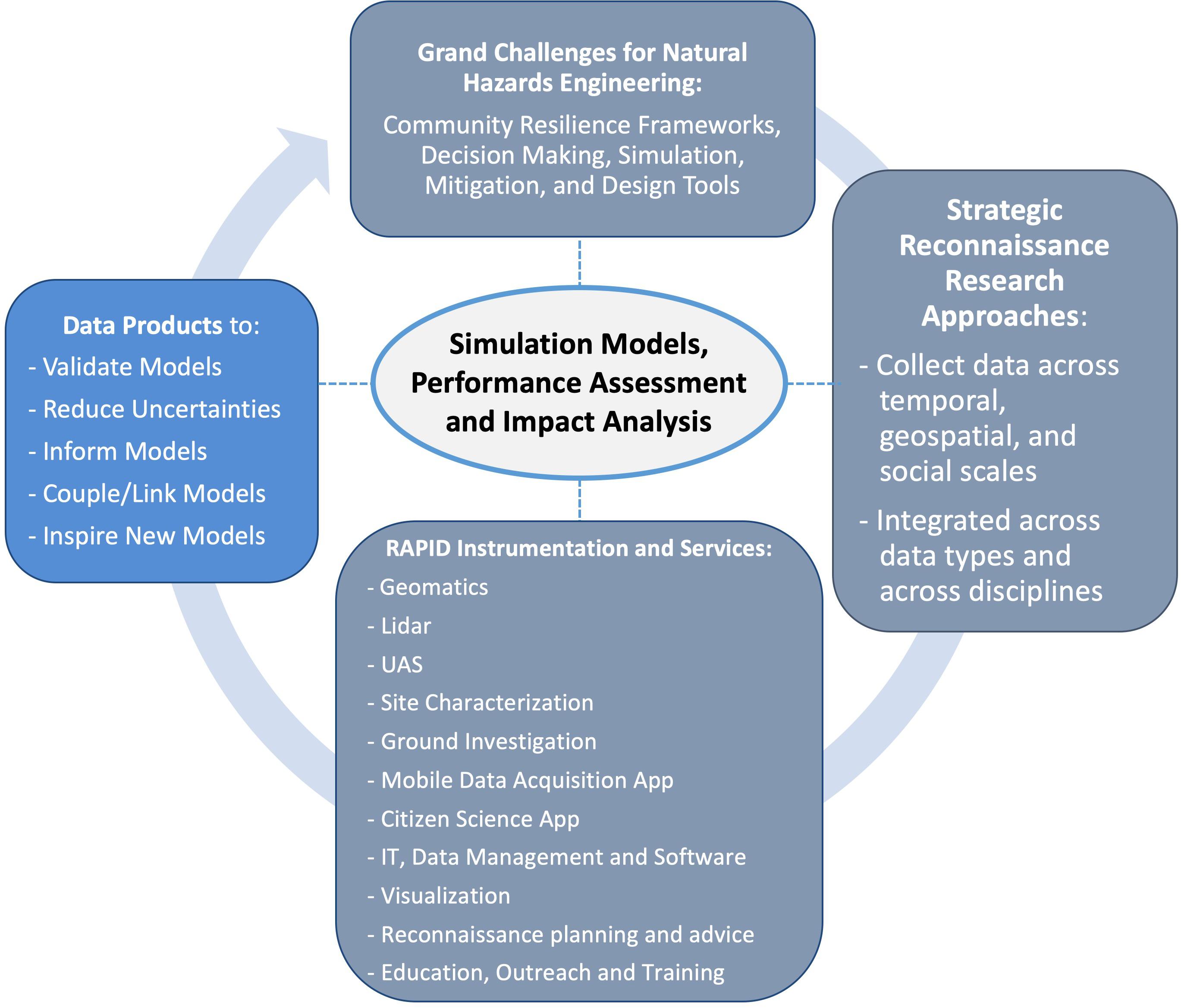
Figure 6. Grand challenges for the natural hazards community require new strategic approaches for reconnaissance data collection utilizing RAPID instrumentation and services. This data collection will produce data products that are needed to meet grand challenges. Central to this cycle is the RAPID Facility’s scientific goal of informing natural hazards computational simulation models, infrastructure performance assessment, and socioeconomic impact analysis by supporting the collection, development, and assessment of high-quality data sets [digital elevation model (DEM)].
1. Lifelines and other elements of the built environments are ultimately socio-technical systems (Miles et al., 2014). That is, there are core social, economic, and behavioral components to the development, operation, and maintenance of all engineered systems. There is a crucial need for research to better unpack and quantify the socio-technical dimensions related to damage, restoration, and reconstruction of elements of the built environment. This research is needed to advance existing socio-technical loss (e.g., Kircher et al., 2006) and recovery models (Miles and Chang, 2011), as well as to develop new ones. Most socio-technical modeling efforts to date have focused on modeling losses.
2. Development of high-resolution, geocoded data sets, such as aerial photography, lidar, and ground-based documentation of post-event damage (e.g., Gurley and Masters, 2011; Lombardo et al., 2015), to reduce uncertainties in stochastic models characterizing the vulnerability of infrastructure to wind and earthquake damage. Modern catastrophe risk models ultimately seek to project damage, loss, and recovery time at the whole-building, infrastructure system, or regional scale; examples modeling tools include FEMA (2018) as well as the community and regional resilience modeling tools such as OpenQuake (Pagani et al., 2014) and those being developed by the Center for Risk-Based Community Resilience Planning (van de Lindt et al., 2015) and the NHERI SimCenter. These tools predict building performance through the aggregation of component failures (e.g., FEMA, 2018 for earthquake hazard and Pita et al., 2015 for wind hazard) or based on building-level models such as those incorporated in FEMA HAZUS-MH (Kircher et al., 2006). These simulation tools include numerous assumptions regarding probabilistic structural component capacities, load paths, the influence of aging, and cascading damage from neighboring structures. Thus, they benefit substantially from refinements to these assumptions informed by detailed geocoded field data stratified by building code and localized hazard intensity.
Provision of appropriate data to test, verify, and calibrate co-seismic landslide displacement models [e.g., the popular and practice-oriented Newmark et al. (1965) sliding model, as well as more advanced coupled (e.g., Rathje and Bray, 2000) or finite element formulations]. Specifically, advanced geomatics technologies such as lidar could capture intricate ground deformation patterns and landslide morphological features, eroded quickly after an event. There are relatively few high-quality case histories of co-seismic landslide displacement, which represents a pressing research need in the field of geotechnical earthquake engineering (Harp et al., 2011).
1. Provision of the appropriate data to quantify underlying physical phenomena and to develop, validate, improve, and reduce uncertainty in physics-based, computational modeling of wind, waves, storm surge, tsunami inundation, sediment transport, morphological change, and other related processes representing the inter-related, destructive forcing mechanisms of natural hazards (Kennedy et al., 2020b and references therein). Specifically, modern reconnaissance instrumentation can capture rare, but critical, perishable data during and following natural hazards, including the quantification of inundation extent, flow speeds, flow depth, wave conditions, wind speeds, soil properties, erosion and accretion, and inundation-related damage to civil infrastructure and the natural environment (Kennedy et al., 2020a). These data help improve understanding of, for example, (a) the interplay between the natural landscape (land cover, topographic features), the built environment (critical infrastructure, homes), and hydrodynamics and (b) how and when concurrent multi-hazard components (e.g., wind vs. surge) lead to the functional failure of critical infrastructure—ultimately leading to more resilient communities (e.g., Baradaranshoraka et al., 2017).
2. Simulation of structural response to ground shaking is validated mainly through comparison with data from experiments in controlled laboratory environments and with data collected from reconnaissance following earthquakes. The structural models may be focused on component behaviors, building behaviors, or even the behavior of entire classes of buildings through the development of fragility functions. Recent examples of field data informing advances in local structural behavior models include Kanvinde et al. (2015), who investigated fracture of eccentrically braced frame links during the 2011 Christchurch earthquake and used collected field data helped to validate newly developed fracture models employed in detailed finite element analyses. At the macro-level, fragility functions derived from reconnaissance data on the performance of wood-frame buildings have resulted in large-scale loss estimations for San Francisco arising from the soft-story collapse of wood-frame structures and spurred public policy to encourage retrofit (FEMA, 2012). Such observation-based fragility data are also critical to loss estimation software such as FEMA (2018), FEMA HAZUS-MH (Kircher et al., 2006) and OpenQuake (Pagani et al., 2014), and the regional loss estimation tools being developed by the Center for Risk-Based Community Resilience Planning and the NHERI SimCenter.
Grand Challenges for the Natural Hazards and Disaster Research Communities
In 2011, the National Research Council convened a community workshop to identify grand challenges for earthquake engineering. These challenges served to guide research after the conclusion of the George E. Brown, Jr. Network for Earthquake Engineering Simulation operations (National Research Council [NRC], 2011). While the title of the workshop highlighted earthquake engineering, the NRC steering committee noted that the identified grand challenges (community resilience, decision making, simulation, mitigation, design tools) were broad and also pertained to other natural and anthropogenic hazards. These grand challenges are adopted here as an overarching framework for identifying reconnaissance research opportunities for natural hazards and disaster research communities.
Community Resilience
To better understand the direct and indirect impacts of natural hazards events, a framework is needed to measure, monitor, and evaluate community-level resilience. The lack of historical data on community impacts and recovery following past disasters presents a significant impediment to meeting this goal (National Research Council [NRC], 2011). Advanced reconnaissance instrumentation helps address this challenge by enabling the systematic collection and archiving integrated, interdisciplinary data pertinent to engineering and the natural and social sciences. This knowledge is necessary to evaluate the utility and validity of the range of community resilience frameworks—a significant gap in the state-of-the-art in disaster science and engineering (Miles, 2015).
Hazard and Impact Simulation and Decision Making
Computational simulation and forecasting of the timing and regional distribution of the hazard itself (e.g., Frankel et al., 2018), as well as its physical and social impacts and recovery, are essential for decision making, planning, and mitigation. Such simulations—which span a range of temporal scales, including both short-term (e.g., informing electricity restoration with expected damage patterns) and long-term time frames (e.g., identifying local vulnerabilities for risk reduction policy-making)—present a challenge to the professional community (National Research Council [NRC], 2011). New, high-performance computing and software platforms such as the NHERI DesignSafe-CI and SimCenter (Blain et al., 2020) create the opportunity to make significant progress with this challenge. However, such simulations are highly complex and require extensive hypervariable data sets for model development and testing. Since many of these models are inherently data-driven, they also require high-quality data (e.g., initial and boundary conditions) to provide reliable forecasts.
Mitigation
Renewal and retrofit strategies are essential to mitigate hazards posed to infrastructure systems and communities (e.g., water and wastewater supply and distribution systems, power and energy systems, at-risk buildings, and coastal communities) (National Research Council [NRC], 2011). The development of effective mitigation strategies requires computational models (see above), design methods, and construction standards that, when harmonized, are capable of identifying critical vulnerabilities and quantifying the impacts of risk reduction measures. In addition, post-event data are needed to evaluate loss estimation methodologies, such as HAZUS-MH, investigate the efficacy of mitigation approaches (e.g., Gurley and Masters, 2011), and provide feedback on state-mandated insurance incentives for homeowners who employ mitigation. New multiscale data collection tools provide the means to address these needs. For example, terrestrial lidar and building survey equipment could be used to collect data on the seismic performance of retrofitted buildings. Similarly, lidar or structure from motion (SfM)/multi view stereo photogrammetry (Eltner et al., 2016; Özyeşil et al., 2017) technology can be used in coastal communities after hurricanes to quantify morphological changes, civil infrastructure damage, and ecological damage in detail and on a large scale. Importantly, all of these data sources can be integrated and overlaid with imagery to develop three-dimensional models of impacted regions or damage-affected infrastructure.
Design Tools
Improved capability to characterize uncertainty in the predictive ability of design tools is essential to exploit newer, more sustainable, and resilient building materials. Improved design tools are also needed to capitalize on innovative structural concepts (e.g., self- centering structural systems with replaceable fuses) (National Research Council [NRC], 2011). Performance-based design provides the framework for addressing this challenge, but such design relies on high-quality performance data to define model relationships (e.g., fragility functions). Advanced instrumentation offers a means to meet this challenge. For example, sensors could be installed on structures and earth systems to monitor response to aftershocks (Geli et al., 1988; Zhou et al., 2013), and aerial imagery could be used to validate the performance of wind-resistant roof covers.
In 2017, the Network Coordination Office (Johnson et al., 2020) of NHERI convened a task group to prepare a network-wide science plan to guide future research and to focus investigators on keeping the communities and the built environment safe from natural hazards. The NCO’s NHERI network science plan was first published in July 2017 (Smith et al., 2017) and reflected many of the principals of the National Research Council Grand Challenges report (National Research Council [NRC], 2011). The NHERI network science plan highlights the need to (1) identify and quantify the characteristics of natural hazards that are damaging to civil infrastructure and disruptive to communities, (2) evaluate the physical vulnerability of civil infrastructure and the social vulnerability of populations in communities exposed to natural hazards, and (3) create the technologies and engineering tools to design, construct, retrofit, and operate multi-hazard resilient and sustainable infrastructure. The network issued a revised science plan in January 2020 (Edge et al., 2020) that reflects the potential role of several new, rapidly advancing technologies (e.g., advanced computational methods, information science, bio-inspired design, convergence science) in improving community resilience to natural hazards. The revised science plan identifies three grand challenges for the community. These include (1) identifying and quantifying the characteristics of earthquake, windstorm, and associated hazards that are damaging to civil infrastructure and disruptive to communities, (2) assessing the physical vulnerability of civil infrastructure and the social vulnerability of populations in communities, and (3) creating the technologies and engineering tools to design, construct, retrofit, and operate a multi-hazard resilient and sustainable infrastructure. In addition to the NRC workshop report and the NHERI network science plans, other reports suggest specific research activities and tasks to help meet challenges in the fields of earthquake hazard reduction (National Earthquake Hazards Reduction Program, 2008), resilience (National Research Council [NRC], 2011), windstorm and coastal inundation impact reduction (Coulbourne et al., 2014), and disaster risk reduction (Aitsi-Selmi et al., 2015).
Research Needs, Challenges, and Opportunities for Natural Hazards Reconnaissance
Methodology
In January 2017, the RAPID Facility convened a 2-day workshop to determine natural hazards and disaster reconnaissance data needs and opportunities and identify the broader challenges facing the reconnaissance community that encumber data collection and use. The workshop attendees—individuals having expertise across a range of natural hazards (e.g., wind events, earthquakes, and their secondary effects) and disciplines (engineering, and the natural and social sciences)—participated in three types of activities (also see Supplementary Material).
(1) Informational presentations to provide background material to help stimulate later discussion in activity groups
(2) Guided “brainstorming-type” small group activities
(3) Responding to open-ended questions posed on poster boards placed in the break area during the first day of the workshop
During the brainstorming activities, participants were asked to first reflect on questions individually and later to share, discuss, and synthesize their ideas in small, pre-assigned groups. For some disciplinary-focused activities, groups were organized by specialty, while in other activities, groups were intentionally interdisciplinary to allow cross-fertilization of ideas between sciences and engineering domains. The ideas developed during the individual sessions and group discussions were attached to poster boards using sticky notes. Each group reported the general themes to all of the workshop participants. Over 1,600 ideas, comments, and replies recorded on sticky notes during the workshop. After the workshop, each of these notes was assigned a unique identifier code, cataloged, and then read and transcribed to a comprehensive database, which is included as Supplementary Material to this article. The workshop organizers then synthesized and analyzed the database of workshop comments and transcriptions to identify significant themes on needs, challenges, and opportunities for natural hazards and disaster reconnaissance.
Findings
Many of the workshop participants were seasoned reconnaissance investigators with the collective experience of dozens of reconnaissance missions following natural hazard events and other disasters—both natural and anthropogenic in origin. The participants responded to questions about the practical and operational challenges they have faced before, during, and after reconnaissance investigations. They also provided feedback about what went well (i.e., their “successes”) during reconnaissance missions. As noted in Table 2, major challenges before deploying for reconnaissance mainly involve logistics in the compressed time frames intrinsic to extreme event investigations. During field missions, many of the challenges relate to the on-the-ground realities of working in a disaster zone, including safety concerns and emotional trauma. The difficulties after reconnaissance missions primarily pertain to data processing, analysis, and archiving. The participants reported a range of common themes about pre- and during deployment successes (Table 3), including having previously established local contacts in the affected region, teamwork and camaraderie, and prior training on instrumentation reconnaissance methods, and safety. Successes after reconnaissance missions mainly pertain to the production of unique data products, improved fundamental knowledge, and positive impacts on policy and practice.
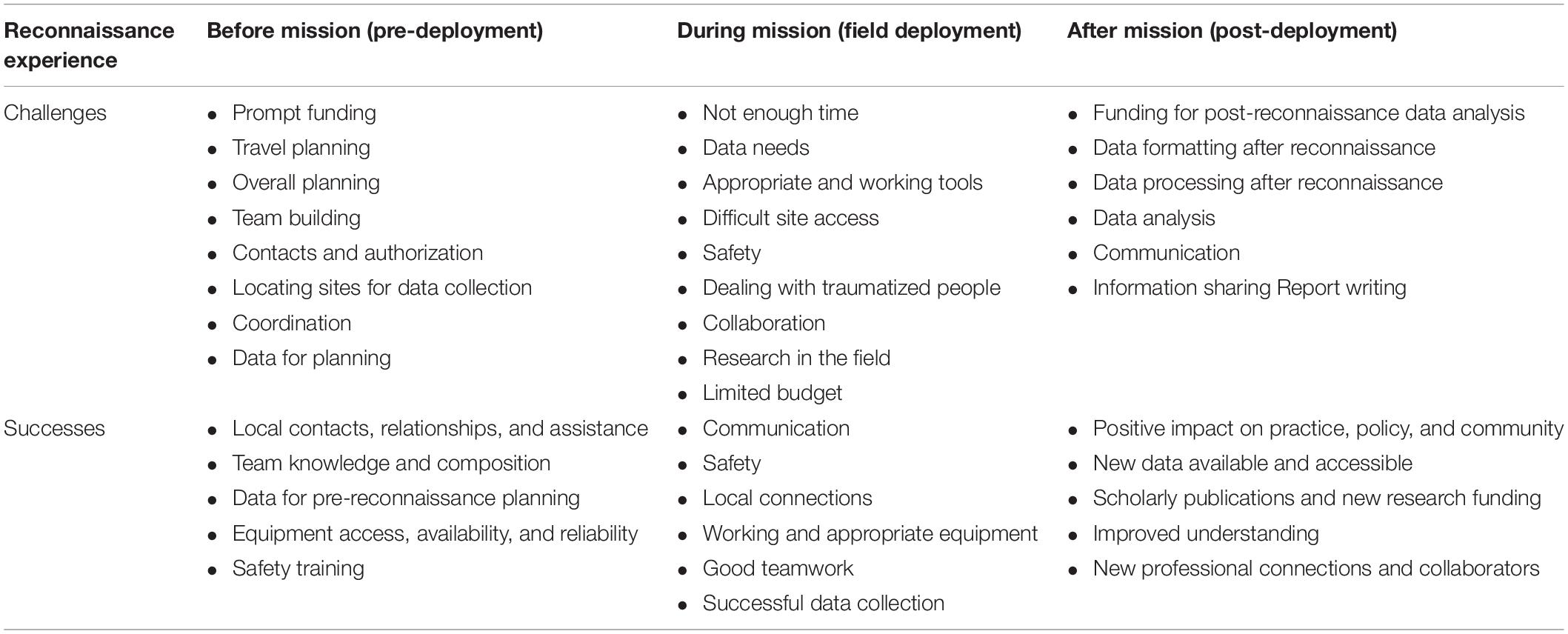
Table 2. Synthesis of key themes in workshop participant responses to questions about challenges and successes before, during, and after reconnaissance missions (see Supplementary Material for complete list).
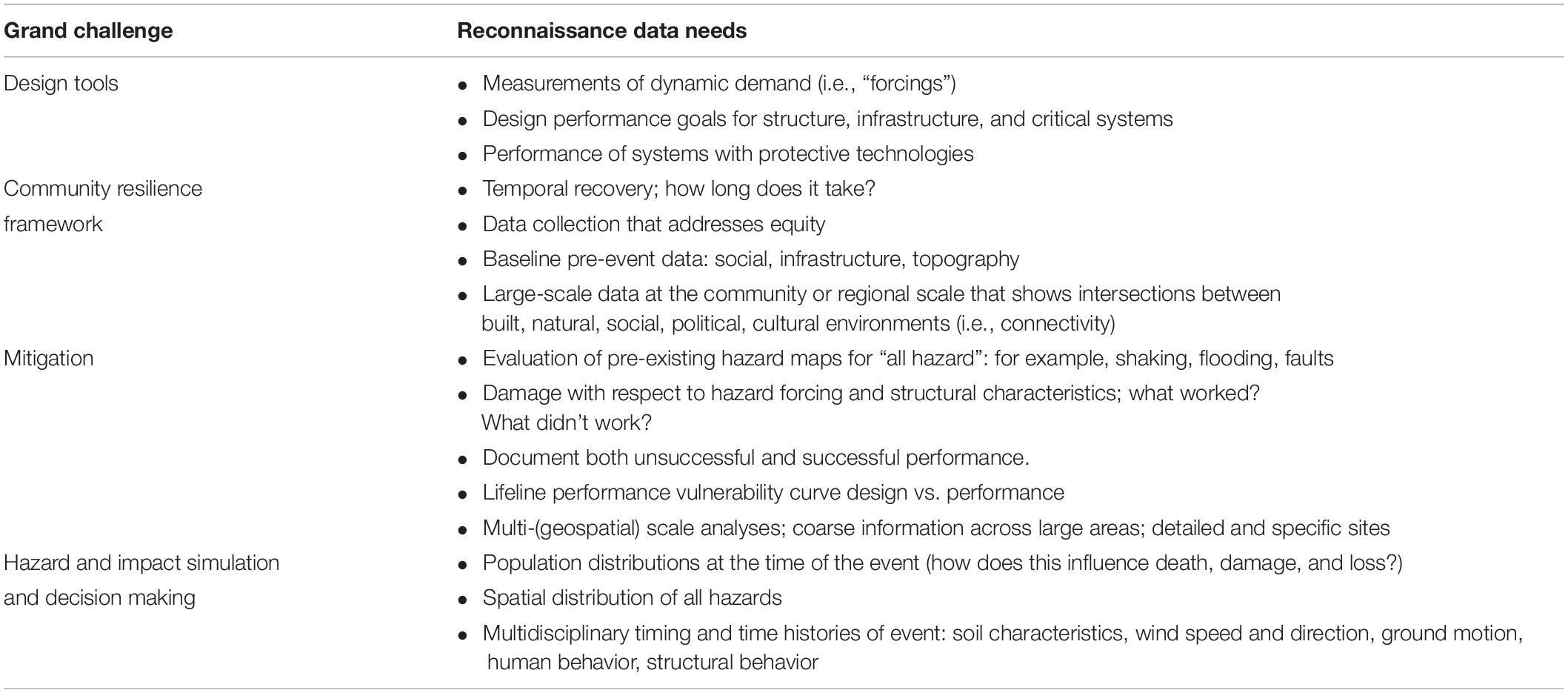
Table 3. Synthesis of High Priority Reconnaissance Data Needs to address Grand Challenges for the natural hazards and disaster research communities (see Supplementary Material for complete list).
The workshop participants were also asked to identify reconnaissance data needed to support the four National Research Council [NRC] (2011) grand challenges (i.e., community resilience framework, hazard and impact simulation and decision making, mitigation, and design tools). As indicated in Table 3, the responses, which form the basis of our recommended strategic approaches for natural hazards and disaster reconnaissance, are broadly themed on concepts of cross-scale, multidisciplinary data collection.
Strategic Approaches for Natural Hazards and Disaster Reconnaissance
Post-disaster reconnaissance investigations have historically often involved the collection and development of data sets by disciplinary teams following natural hazard events. These data sets have usually been collected over limited geospatial scales (e.g., at the site or neighborhood scales) with little supporting metadata. As a result, such data sets can be challenging, if not impossible, to integrate. Meeting community challenges and accomplishing the scientific goal of improving simulation models requires new strategic approaches for reconnaissance investigations that acquire and integrate data over a range of temporal, spatial, and social scales across disciplines. Figures 7, 8 illustrate links between the strategic approaches for natural hazard reconnaissance data collection, instrumentation, and resulting data products, for a hypothetical earthquake and wind event, respectively.
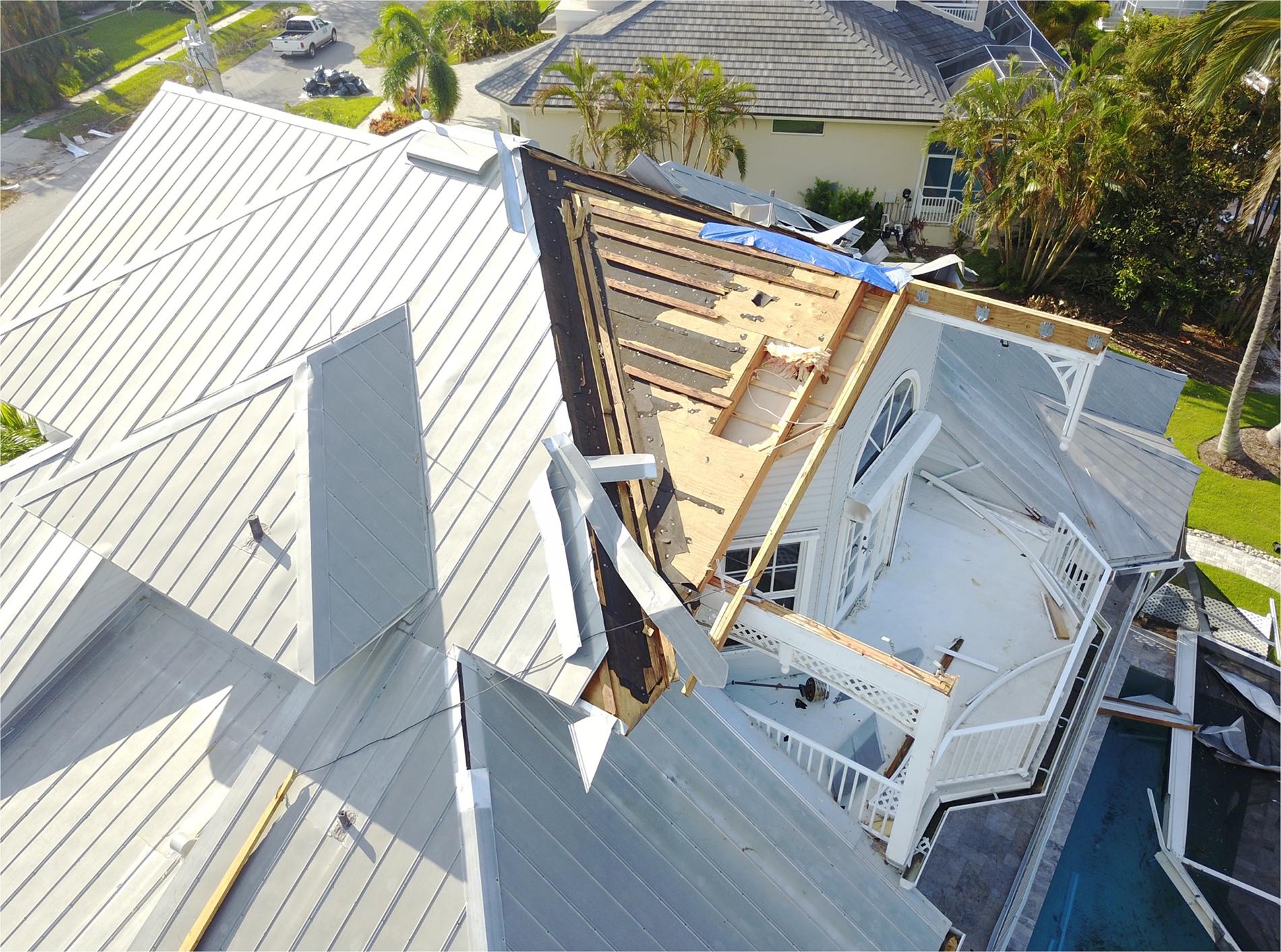
Figure 7. UAVs with high-resolution cameras are well-suited capture perishable data (e.g., roof cover damage, debris field), and provide complementary datasets for ground-based damage surveys. The areal perspective of UAVs reveals structural damage that is hidden from the view of ground-based damage surveyors. Photograph by Kwasi Oerry was acquired under sponsorship from the Florida Building Commission.
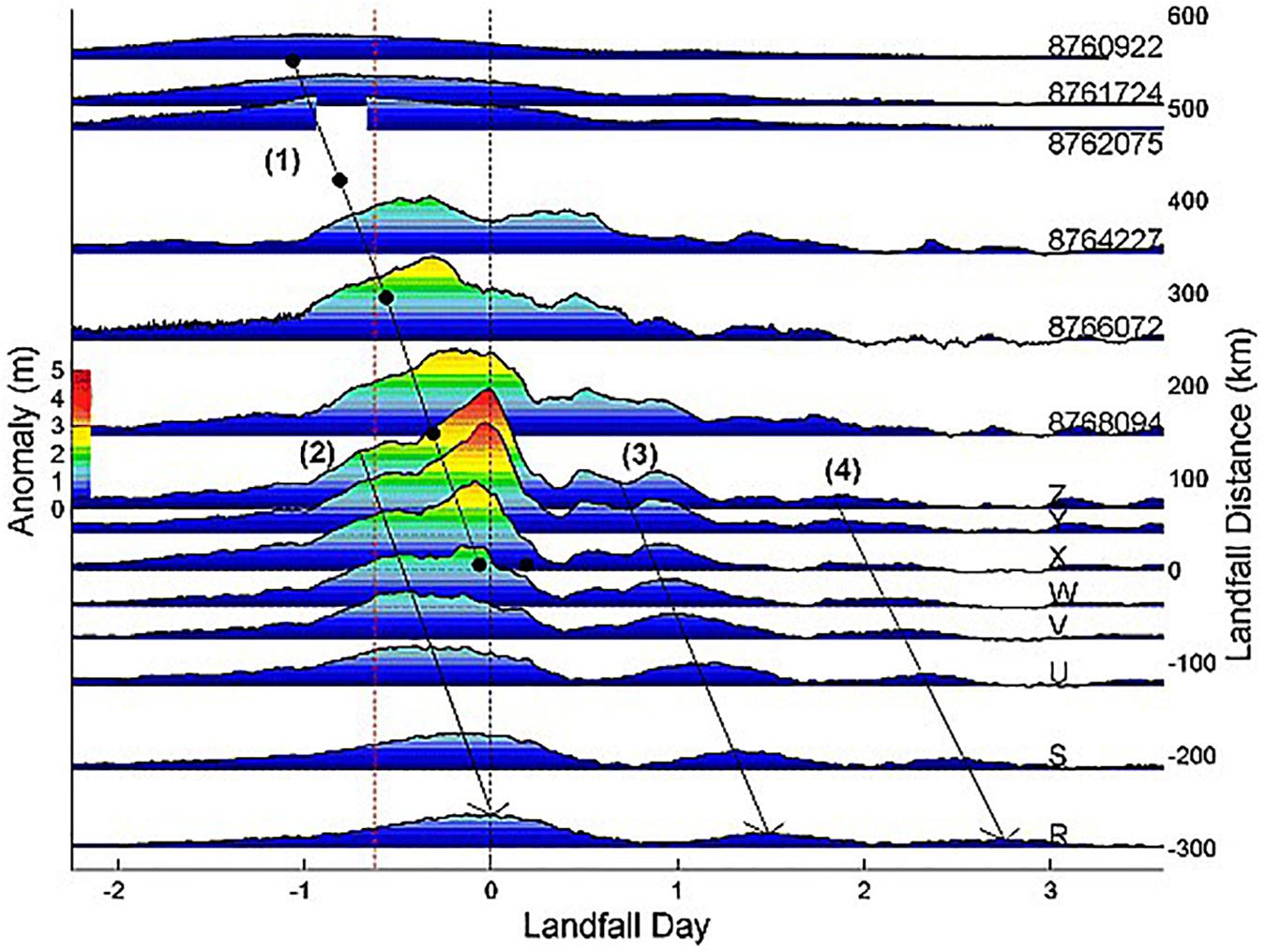
Figure 8. Observed time series of water level anomaly during Hurricane Ike (2008) along the open coasts of Louisiana and Texas (top-to-bottom show easternmost locations to westernmost locations). Data shown include rapidly installed pressure sensors (R-Z) by A. Kennedy (University of Notre Dame) and NOAA stations (8760922, 8761724, 8762075, 8764227, 8766072, 8768094). Line 1 shows the location of Hurricane Ike, while Line 2 shows the propagation of the forerunner wave. Reproduced from Kennedy et al. (2011) with permission of the publisher.
Temporal Scales
Resilience is the central, unifying goal of the natural hazards and disaster research communities (e.g., National Research Council [NRC], 2011; National Research Council, 2012). The term refers to an impacted community’s ability to resist, absorb, accommodate, adapt to, transform and ultimately recover and move on from the effects of a hazard in a timely and efficient manner (United Nations, 2017). A path toward better upstanding, assessing, and improving resilience involves collecting and analyzing data over time frames representing conditions and states before, during, and after significant natural hazard events. Data on pre-event or “before” conditions are essential for understanding the pre-existing factors that influence, shape, and define a community’s response to a natural hazard event. With its emphasis on post-event response, the collection of pre-event data is largely outside the scope of the traditional reconnaissance community; however, much of this data currently exists or is being collected by governmental agencies and authorities, non- governmental organizations, and the private sector.
Moreover, there exists an opportunity for the natural hazards and disaster communities to lead organized efforts to catalog, organize, and synthesize such data, making it possible to link them with reconnaissance data. Data on the direct impacts of an event (“during event data”) are the traditional focus of reconnaissance investigations. These data provide critical information on the character of the loadings and the consequent physical response of the built environment, the immediate social, economic and public health impacts on communities, and interactions between these. These data also represent the starting point for recovery from natural hazard events. After an event, data collected are critical for understanding the response, recovery, and evolution of communities following events. Collecting data representing during and after events conditions requires both traditional rapid response reconnaissance investigations and follow-up data-gathering efforts. These longer-term data gathering investigations may span periods of weeks, months, or years, depending on the nature of the event and the characteristics of the affected communities.
Geospatial Scales
Natural hazard events often impact areas spanning 100s-to-1000s of square kilometers. Their widespread geographic distribution makes them, by definition, regional-scale events. The resulting damage and impact patterns reflect the fundamental nature of the hazard and the characteristics of the communities and built systems within affected regions. Over the past several decades, the ability to analyze the effects of natural hazards at the site- and building-scales has significantly improved, leading to better modeling tools, new building technologies, and robust building codes. In recent years the natural hazards and disaster communities have shown a growing interest in regional-scale impact modeling. A key advantage of regional-scale models is their ability to forecast the distribution of hazard impacts and thus capture system-level performance and propagation of risk across a region. Such models are particularly important when considering the impact of hazards on geographically distributed critical infrastructure systems.
Improving our understanding of hazard impacts and advancing regional scale modeling requires collection and synthesis of data over spatial scales spanning multiple orders of magnitude (i.e., from the site-specific to the regional scales; ∼m2 to ∼km2). This necessitates a portfolio of instrumentation that can facilitate the acquisition of fine-grained, high-resolution “site-specific” data and also support the collection in a practical manner of data from a much broader area. This also requires reconnaissance investigations to be conducted at both local and regional scales. Acquiring multiscale data enables the local impacts of a hazard to be understood in the broader context of regional-scale loading patterns and community characteristics. Equally important, this data can support the information necessary to bridge site-specific and regional scale models, which improves the ability to simulate the consequences of an extreme event across a vast region.
Social Scales
Natural hazard events can have immensely varying impacts and consequences at all social scales, from individuals and households to neighborhoods and communities; organizations, businesses, and governments; and up to and including countries, cultures, and global consequences (e.g., Oliver-Smith, 1996; Paton and Johnston, 2001; Quarantelli, 2003; Boon et al., 2012). As the Covid-19 pandemic wreaks havoc on individual lives, senior centers, vulnerable communities, nations, and the global economy, inequities and the heterogeneity of hazard effects at different social scales have commanded renewed attention (e.g., Adams-Prassl et al., 2020). Differences in natural and built environments contribute to potentially predictable variation in hazard impacts on society and individuals and can interact with societal responses (Paton and Johnston, 2001). Infrastructure damages can hinder immediate and longer-term responses, including emergency responses, evacuation, and sheltering, but also communications and governance. Direct hazard effects on the physical environment, such as flooding, landslides, and fire, are not only potentially deadly to individuals but can also cause longer-term mental harm and disrupt social and economic activities at multiple scales. However, the lack of population-representative fine-scaled data on damages and human exposures for natural hazards and disasters continues to be called out (e.g., Bakkensen and Mendelsohn, 2016).
Assessing hazard exposures and consequences across these domains and social scales requires instrumentation and data collection sensitive to and associated with social, built, and natural environmental conditions, as well as temporal and spatial scales. Data collection processes that are multi-scalar and consider the social processes that can make people hard to reach will have a better chance of representing minority populations most likely to be among the most vulnerable to the majority of hazard events (Shaghaghi et al., 2011). Data can be contextualized with the appropriate metadata, but also improved by designing direct data collections—such as observations, interviews, and surveys—to address these contextual factors and link geophysical, engineering, and social data. Social scientists have long acknowledged interactions across social scales (e.g., Bronfenbrenner and Morris, 2006). New technologies, analytical approaches, and data sources—such as biophysical and EEG (electroencephalogram) measurement tools (e.g., Bailey et al., 2017), crowdsourcing (e.g., Cobb et al., 2014), social media (e.g., Chae et al., 2014; Spence et al., 2016; Wang and Taylor, 2018), and satellite observations of night lights and other forms of evidence of human activities and interventions at larger scales (e.g., Ehrlich et al., 2009; Ceola et al., 2014)—can enable researchers to examine these interactions in new ways. They can also support insights into and simulations of how individual responses and behaviors contribute to or are shaped by responses and events at larger social scales.
Multidisciplinary Data Sets
A disaster is a severe disruption of the functioning of a community or a society at any scale due to hazardous events interacting with conditions of exposure, vulnerability, and capacity leading to human, material, economic and environmental losses and/or impacts (United Nations, 2020). A better understanding of the complicated relationship between hazards, the built environment, and communities requires that the physical and socioeconomic factors leading to disasters be untangled. Accomplishing this requires the reconnaissance community to collect and synthesize multidisciplinary data sets. In addition to improving our fundamental understanding of disasters, these data can play a critical role in establishing relationships between hazards and their broad consequences, ultimately leading to an improved ability to model, manage, and mitigate risk to communities.
Conclusion
Natural hazard events provide extraordinary opportunities to improve our fundamental understanding of disasters and their consequences. This understanding is critical for reducing the growing human and capital losses arising from extreme events (e.g., Coronese et al., 2019). To minimize losses, the natural hazard and disaster research and practice communities must meet several key challenges related to improving modeling and design making, community resilience, and hazard mitigation (e.g., National Research Council [NRC], 2011). Reconnaissance data, which captures real-world complexities of events (e.g., the interplay between natural, human, and built systems), plays an increasingly important role in meeting these challenges. The recent availability of state-of-the-art instrumentation and mobile data collection applications has dramatically improved the quality and increased the quantity of disaster data, paving the way toward a new era of natural hazards reconnaissance. However, to fully realize the potential of these advancements, we must employ new strategic approaches for natural hazards reconnaissance that acquire and integrate data over a range of temporal, spatial, and social scales across disciplines. Specifically, this involves the following.
(1) Data collection over time frames representing conditions and states before, during, and after significant natural hazard events.
(2) The collection and synthesis of data over spatial scales spanning multiple orders of magnitude (i.e., from the site-specific to the regional scales; ∼m2 to ∼km2).
(3) Data collection is sensitive to and associated with social, built, and natural environmental conditions, and considers the social processes that can make populations hard to reach.
(4) The collection and synthesis of multidisciplinary data sets to establish relationships between hazard events, their antecedents, and their broad consequences, ultimately leading to an improved ability to model, manage, and mitigate disaster risk to communities.
Data Availability Statement
All datasets generated for this study are included in the article/Supplementary Material.
Author Contributions
JW, JB, AB, SM, MO, KG, JI, LL, TT, and JD developed the RAPID Facility science plan reported in this article. SM and AB designed the community workshop and later worked in close collaboration with JW and JB to interpret the result and develop findings. MG, AL, and JP work with members of the reconnaissance community users to implement the science plan into field missions. All authors contributed to manuscript drafting and revision, and read and approved the submitted version.
Funding
The U.S. National Science Foundation supported this work under grant number 1611820. Any opinions, findings, and conclusions or recommendations expressed in this material are those of the authors and do not necessarily reflect the views of the National Science Foundation.
Conflict of Interest
The authors declare that the research was conducted in the absence of any commercial or financial relationships that could be construed as a potential conflict of interest.
Acknowledgments
We thank the many experts (see the list in Supplementary Material) who participated in the January 2017 reconnaissance workshop in Seattle and engaged in thoughtful discussions that led to many of the ideas expressed in this article. A portion of the content if this manuscript has been published as part of the RAPID Facility Science Plan (RAPID Facility, 2017).
Supplementary Material
The Supplementary Material for this article can be found online at: https://www.frontiersin.org/articles/10.3389/fbuil.2020.573068/full#supplementary-material
References
Adams-Prassl, A., Boneva, T., Golin, M., and Rauh, C. (2020). Inequality in the Impact of the Coronavirus Shock: Evidence from Real Time Surveys. IZA Discussion Papers, No. 13183. Bonn: Institute of Labor Economics (IZA).
Aitsi-Selmi, A., Egawa, S., Sasaki, H., Wannous, C., and Murray, V. (2015). The sendai framework for disaster risk reduction: renewing the global commitment to people’s resilience, health, and well-being. Int. J. Disast. Risk Sci. 6, 164–176. doi: 10.1007/s13753-015-0050-9
Allen, R. M., and EERI Reconnaissance Team (2017). Quake warnings, seismic culture. Science 358:1111. doi: 10.1126/science.aar4640
Bailey, A. W., Johann, J., and Kang, H. (2017). Cognitive and physiological impacts of adventure activities: beyond self-report data. J. Exper. Educ. 40, 153–169. doi: 10.1177/1053825917701250
Bakkensen, L. A., and Mendelsohn, R. O. (2016). Risk and adaptation: evidence from global hurricane damages and fatalities. J. Assoc. Environ. Resour. Econ. 3, 555–587. doi: 10.1086/685908
Baradaranshoraka, M., Pinelli, J.-P., Gurley, K., Peng, X., and Zhao, M. (2017). Hurricane wind versus storm surge damage in the context of a risk prediction model. ASCE J Struct. Eng. 143:04017103. doi: 10.1061/(ASCE)ST.1943-541X.0001824
Baradaranshoraka, M., Pinelli, J.-P., Gurley, K., Zhao, M., Peng, X., and Paleo-Torres, A. (2019). Characterization of coastal flood damage states for residential buildings. ASCE ASME J. Risk Uncertain. Eng. Syst. 5:04019001. doi: 10.1061/AJRUA6.0001006
Barbosa, A. R., Fahnestock, L. A., Fick, D. R., Gautam, D., Soti, R., Wood, R., et al. (2017). Performance of medium-to-high rise reinforced concrete frame buildings with masonry infill in the 2015 Gorkha, Nepal, earthquake. Earthq. Spec. 33, 197–218. doi: 10.1193/051017eqs087m
Berman, J. W., Wartman, J., Olsen, M. J., Irish, J., Miles, S., Tanner, T., et al. (in press). Natural hazards reconnaissance with the NHERI RAPID facility. Front. Built Environ.
Blain, C., Bobet, A., Browning, J., Edge, B., Holmes, W., Johnson, D., et al. (2020). The Network coordination office of NHERI (Natural hazards engineering research infrastructure). Front. Built Environ. 6:108.
Boon, H., Cottrell, A., King, D., Stevenson, R., and Millar, J. (2012). Bronfenbrenner’s bioecological theory for modelling community resilience to natural disasters. Nat. Hazards 60, 381–408. doi: 10.1007/s11069-011-0021-24
Brando, G., Rapone, D., Spacone, E., Matt, S. O., and Olsen, M. J. (2017). Damage reconnaissance of unreinforced masonry bearing wall buildings after the 2015 Gorkha, Nepal, earthquake. Earthq. Spec. 33, 243–273. doi: 10.1193/010817eqs009m
Bray, J. D., Frost, J. D., Rathje, E. M., and Garcia, F. E. (2019). Recent advances in geotechnical post-earthquake reconnaissance. Front. Built Environ. 5:5. doi: 10.3389/fbuil.2019.00005
Bronfenbrenner, U., and Morris, P. (2006). “The bioecological model of human development,” in Handbook of Child Psychology: Theoretical Models of Human Development, 6th Edn, eds R. M. Lerner and W. Damon (Washington, DC: John Wiley & Sons Inc), 793–828.
Ceola, S., Laio, F., and Montanari, A. (2014). Satellite nighttime lights reveal increasing human exposure to floods worldwide. Geophys. Res. Lett. 41, 7184–7190. doi: 10.1002/2014GL061859
Chae, J., Thom, D., Jang, Y., Kim, S., Ertl, T., and Ebert, D. S. (2014). Public behavior response analysis in disaster events utilizing visual analytics of microblog data. Comput. Graph. 38, 51–60. doi: 10.1016/j.cag.2013.10.008
Cobb, C., Mccarthy, T., Perkins, A., Bharadwaj, A., Comis, J., Do, B., et al. (2014). “Designing for the deluge: understanding & supporting the distributed, collaborative work of crisis volunteers,” in Proceedings of the 17th ACM Conference on Computer Supported Cooperative Work & Social Computing, New York, NY.
Cong, Z., Nejat, A., Liang, D., Pei, Y., and Javid, R. J. (2018). Individual relocation decisions after tornadoes: a multi-level analysis. Disasters 42, 233–250. doi: 10.1111/disa.12241
Coronese, M., Lamperti, F., Keller, K., Chiaromonte, F., and Roventini, A. (2019). Evidence for sharp increase in the economic damages of extreme natural disasters. Proc. Natl. Acad. Sci. U.S.A. 116, 21450–21455. doi: 10.1073/pnas.1907826116
Coulbourne, W., Galsworthy, J., Hangan, H., Jones, C., Letchford, C., Smith, T., et al. (2014). Measurement Science R&D Roadmap for wIndstorm and Coastal Inundation Impact Reduction. Grant/Contract Reports (NISTGCR)-14-973-13. Available online at: www.nist.gov/publications/measurement-science-rd-roadmap-windstorm-and-coastal-inundation-impact-reduction (accessed October 9, 2020).
Dashti, S., Palen, L., Heris, M. P., Anderson, K. M., Anderson, T. J., and Anderson, S. (2014). “Supporting disaster reconnaissance with social media data: A design-oriented case study of the 2013 Colorado floods,” in Proceedings of the 11th International Conference on Information Systems for Crisis Response and Management (ISCRAM), University Park, PA.
Dietrich, J. C., Zijlema, M., Westerink, J. J., Holthuijsen, L. H., Dawson, C., Luettich, R. A., et al. (2011). Modeling hurricane waves and storm surge using integrally-coupled, scalable computations. Coast. Eng. 58, 45–65. doi: 10.1016/j.coastaleng.2010.08.001
Earthquake Engineering Research Institute [EERI] (1971). Earthquake Investigation Committee Los Angeles Earthquake of February 9, 1971., ed. Moran, D., Meehan, J. F., Pinkham, C. W., Brugger, W. A., Allen, C., Duke, C. M., Housner, G. W., Degenkolb, H. J., Crandall, L. Available online at: https://www.eeri.org/1971/02/san-fernando/ (accessed June 12, 2020).
Edge, B., Ramirez, J., Peek, L., Bobet, A., Holmes, W., Robertson, I., et al. (2020). Natural Hazards Engineering Research Infrastructure, 5-Year Science Plan, Multi-Hazard Research To Make a More Resilient World, Second Edition. DesignSafe-CI. Available online at: https://www.designsafe-ci.org/data/browser/public/designsafe.storage.published/PRJ-2731 (accessed September 25, 2020).
Eguchi, R., Huyck, C., Ghosh, S., and Adams, B. (2008). “The application of remote sensing technologies for disaster management,” in Proceedings of the 14th World Conference on Earthquake Engineering, Beijing.
Ehrlich, D., Guo, H. D., Molch, K., Ma, J. W., and Pesaresi, M. (2009). Identifying damage caused by the 2008 Wenchuan earthquake from VHR remote sensing data. Int. J. Digit. Earth 2, 309–326. doi: 10.1080/17538940902767401
Ellsworth, W. L. (1990). “Earthquake history 1769-1989,” in The San Andreas Fault System, ed. R. E. Wallace (California: U. S. Geological Survey), 153–178.
Eltner, A., Kaiser, A., Castillo, C., Rock, G., Neugirg, F., Abellá, L., et al. (2016). Image-based surface reconstruction in geomorphometry – merits, limits and developments. Earth Surf. Dynam. 4, 359–389. doi: 10.5194/esurf-4-359-2016
FEMA (2012). “Seismic evaluation and retrofit of multi-unit wood-frame buildings with weak first stories,” in FEMA P-807 (Washington, DC: Federal Emergency Management Agency).
FEMA (2018). Seismic Performance Assessment of Buildings: FEMA P-58-1. Washington, DC: Federal Emergency Management Agency.
Fischer, E. C., and Hakhamaneshi, M. (2019). The New Paradigm of Post-Disaster Reconnaissance. Geotechnics Of Extreme Events. Available online at: https://www.readgeo.com/geostrata/may_jun_2019/MobilePagedArticle.action?articleId=1489220#articleId1489220 (accessed June 12, 2020).
Frankel, A., Wirth, E., Marafi, N., Vidale, J., and Stephenson, W. (2018). Broadband synthetic seismograms for magnitude 9 Earthquakes on the Cascadia megathrust Based on 3D simulations and stochastic synthetics, Part 1: methodology and overall results. Bull. Seismol. Soc. Am. 108, 2347–2369. doi: 10.1785/0120180034
Gaillard, J. C., and Peek, L. (2019). Disaster-zone research needs a code of conduct. Nature 575, 440–442. doi: 10.1038/d41586-019-03534-z
Gallant, A. P., Montgomery, J., Mason, H. B., Hutabarat, D., Reed, A. N., Wartman, J., et al. (2020). The sibalaya flowslide initiated by the 28 September 2018 MW 7.5 Palu-Donggala, Indonesia earthquake. Landslides 17, 1925–1934. doi: 10.1007/s10346-020-01354-1
Geli, L., Bard, P.-Y., and Jullien, B. (1988). The effect of topography on earthquake ground motion: a review and new results. Bull. Seismol. Soc. Am. 78, 42–63. doi: 10.1016/0148-9062(88)90024-1
Geotechnical Extreme Events Reconnaissance [GEER] (2014). Manual for GEER Reconnaissance Teams, Ver 4., ed.Kayen, R. Available online at: http://www.geerassociation.org/media/files/Important%20Docs/GEER_Recon_Team_Manual_2014_v4.pdf (accessed June 12 2020).
Grant, A., Wartman, J., Massey, C., Olsen, M., O’Banion, M., and Motley, M. (2018). The impact of rockfalls on dwellings during the 2011 Christchurch, New Zealand, earthquakes. Landslides 15, 31–42. doi: 10.1007/s10346-017-0855-852
Gurley, K. R., and Masters, F. J. (2011). Post-2004 hurricane field survey of residential building performance. ASCE Nat. Haz. Rev. 12, 177–183. doi: 10.1061/(ASCE)NH.1527-6996.0000044
Hamid, S. S., Pinelli, J.-P., Chen, S.-C., and Gurley, K. (2011). Catastrophe model-based assessment of hurricane risk and estimates of potential insured losses for the State of Florida. ASCE Nat. Haz. Rev. 12, 171–176. doi: 10.1061/(ASCE)NH.1527-6996.0000050
Harp, E., Keefer, D., Hiroshi, S., and Yagi, H. (2011). Landslide inventories: The essential part of seismic landslide hazard analyses. Eng. Geol. 122, 9–21. doi: 10.1016/j.enggeo.2010.06.013
Hughes, K. S., and Morales Vélez, A. C. (2017). “Characterization of landslide sites in Puerto Rico after Hurricanes Irma and Maria,” in Proceedings of the American Geophysical Union, Fall Meeting 2017, Abstract #NH23E-2859, Washington, DC.
Johnson, D. R., Blain, C. A., Bobet, A., Browning, J., Edge, B., Holmes, B., et al. (2020). The Network coordination office of NHERI (Natural hazards engineering research infrastructure). Front. Built Environ. 6:108. doi: 10.3389/fbuil.2020.00108
Kang, H., Burton, H. V., and Miao, H. (2018). Replicating the recovery following the 2014 south napa earthquake using stochastic process models. Earthq. Spect. 34, 1247–1266. doi: 10.1193/012917EQS020M
Kanvinde, A. M., Marshall, K. S., Grilli, D. A., and Bomba, G. (2015). Forensic analysis of link fractures in eccentrically braced frames during the February 2011 Christchurch Earthquake: testing and simulation. J. Struct. Eng. 141:5. doi: 10.1061/(ASCE)ST.1943-541X.0001043
Kaplan, K. (2010). Mining Destruction for Data to Help Others. Los Angeles Times, February 1. Available online at: https://web.archive.org/web/20151011135150/http://articles.latimes.com/2010/feb/01/science/la-sci-disaster-research1-2010feb01/2 (accessed September 25, 2020).
Kennedy, A., Copp, A., Florence, M., Gradel, A., Gurley, K., Janssen, M., et al. (2020a). Hurricane Michael (2018) in the area of Mexico Beach, Florida. J. Water Port. C. 146:05020004. doi: 10.1061/(ASCE)WW.1943-5460.0000590
Kennedy, A., Cox, D., Irish, J., Kaihatu, J., Lynett, P., and Tomiczek, T. (2020b). “Envisioning the future coast: coastal engineering research in the coming decades,” in Proceedings of the A report from the Coastal Engineering Research Framework Workshop, November 13–14, 2018, Arlington, VA.
Kennedy, A. B., Gravois, U., Zachry, B. C., Westerink, J. J., Hope, M. E., Dietrich, J. C., et al. (2011). Origin of the Hurricane Ike forerunner surge. Geophys. Res. Lett. 38:L08608. doi: 10.1029/2011GL047090
Kircher, C. A., Whitman, R. V., and Holmes, W. T. (2006). HAZUS Earthquake Loss Estimation. Methods Nat. Hazards Rev. 7:45. doi: 10.1061/(ASCE)1527-6988
Knowles, S. G. (2012). The Disaster Experts: Mastering Risk in Modern America. Philadelphia: University of Pennsylvania Press.
Lawson, A. C., and Reid, H. F. (1908). The California Earthquake of April 18, 1906. Report of the State Earthquake Investigation Commission. Washington, DC: Carnegie Institution of Washington.
LeVeque, R. J., George, D. L., and Berger, M. J. (2011). Tsunami modelling with adaptively refined finite volume methods. Acta Numer. 20, 211–289. doi: 10.1017/S0962492911000043
Loggins, R., Little, R., Mitchell, J., Sharkey, T., and Wallace, W. (2019). CRISIS: modeling the restoration of interdependent civil and social infrastructure systems following an extreme event. Nat. Hazards Rev. 20:326. doi: 10.1061/(ASCE)NH.1527-6996.0000326
Lombardo, F. T., Roueche, D. B., and Prevatt, D. O. (2015). Comparison of two methods of near-surface wind speed estimation in the 22 May, 2011 Joplin, Missouri Tornado. J. Wind Eng. Indust. Aerodyn. 138, 87–97. doi: 10.1016/j.jweia.2014.12.007
Mandli, K. T., and Dawson, C. N. (2014). Adaptive mesh refinement for storm surge. Ocean Model. 75, 36–50. doi: 10.1016/j.ocemod.2014.01.002
Mazzoni, S., Castori, G., Galasso, C., Calvi, P., Dreyer, R., Fischer, E., et al. (2018). 2016–2017 Central Italy earthquake sequence: seismic retrofit policy and effectiveness. Earthq. Spec. 34, 1671–1691. doi: 10.1193/100717EQS197M
McAllister, T., Clavin, C., Ellingwood, B., van de Lindt, J. W., Mizzen, D., and Lavelle, F. (2019). Data, Information, and Tools Needed for Community Resilience Planning and Decision Making. Gaithersburg, MD: National Institute of Standards and Technology.
Miles, S. B. (2015). Foundations of community disaster resilience: well-being, identity, services, and capitals. Environ. Hazards 14, 103–121. doi: 10.1080/17477891.2014.999018
Miles, S. B., and Chang, S. E. (2011). ResilUS: a community based disaster resilience model. Cartogr. Geogr. Inform. Sci. 38, 36–51. doi: 10.1559/1523040638136
Miles, S. B., Gallagher, H., and Huxford, C. J. (2014). Restoration and impacts from the September 8, 2011, San Diego Power Outage. J. Infrastruct. Syst. 20:176. doi: 10.1061/(ASCE)IS.1943-555X.0000176
Miles, S. B., and Tanner, T. (2018). “Designed a disaster reconnaissance field app with a user-centered approach,” in Proceedings of the 11th U.S. National Conference of Earthquake Engineering, Los Angeles, CA.
Montgomery, J., Candia, G., Lemnitzer, A., and Martinez, A. (2020). The September 19, 2017 Mw 7.1 Puebla-Mexico City earthquake: observed rockfall and landslide activity. Soil Dyn. Earthq. Eng. 130:105972. doi: 10.1016/j.soildyn.2019.105972
National Earthquake Hazards Reduction Program (2008). Strategic Plan for the National Earthquake Hazards Reduction Program, Fiscal Years 2009–2013.
National Research Council [NRC] (2011). Grand Challenges in Earthquake Engineering Research: A. (Community) Workshop Report. Washington DC: The National Academies Press.
National Research Council (2012). Disaster Resilience: A National Imperative. Washington, DC: The National Academies Press. doi: 10.17226/13457
Nejat, A., Moradi, S., and Ghosh, S. (2019). Anchors of social network awareness index: a key to modeling postdisaster housing recovery. J. Infrastruct. Syst. 25:04019004. doi: 10.1061/(asce)is.1943-555x.0000471
Newmark, N. M. (1965). Effects of earthquakes on dams and embankments. Geotechnique 15, 139–159. doi: 10.1680/geot.1965.15.2.139
Oliver-Smith, A. (1996). Anthropological research on hazards and disasters. Annu. Rev. Anthropol. 25, 303–328. doi: 10.1146/annurev.anthro.25.1.303
Olsen, M., and Kayen, R. (2013). “Post-Earthquake and Tsunami 3D laser scanning forensic investigations,” in Proceedings of the Sixth Congress on Forensic Engineering, San Francisco, CA.
Olsen, M. J., Gillins, D. T., Cubrinovski, M., Bradley, B. A., Price, C., and Chin, C. Y. (2015). “How can geomatics technologies benefit geotechnical studies?,” in Proceedings of the 6th International Conference on Earthquake Geotechnical Engineering, Christchurch.
Özyeşil, O., Voroninski, V., Basri, R., and Singer, A. (2017). A survey of structure from motion. Acta Numer. 26, 305–364. doi: 10.1017/S096249291700006X
Pagani, M., Monelli, D., Weatherill, G., Danciu, L., Crowley, H., Silva, V., et al. (2014). OpenQuake engine: an open hazard (and Risk) software for the global Earthquake model. Seismol. Res. Lett. 85, 692–702. doi: 10.1785/0220130087
Paton, D., and Johnston, D. (2001). Disasters and communities: vulnerability, resilience and preparedness. Disaster Prev. Mgmt. Intern. J. 10, 270–277. doi: 10.1108/EUM0000000005930
Peek, L., Tobin, J., Adams, R., Wu, H., and Mathews, M. (2020). A framework for convergence research in the hazards and DisasterField: the natural hazards engineering research infrastructure CONVERGE facility. Front. Built Environ. 6:110. doi: 10.3389/fbuil.2020.00110
Pinelli, J.-P., David, R., Kijewski-Correa, T., Fernando, P., David, P., Ioannis, Z., et al. (2018). “Overview of damage observed in regional construction during the passage of Hurricane Irma over the State of Florida,” in Proceedings of the Forging Forensic Frontiers, ASCE Forensic Engineering 8th Congress, Austin, TX.
Pita, G., Pinelli, J.-P., Gurley, K., and Mitrani-Reiser, J. (2015). State of the art of Hurricane vulnerability estimation methods: a review. ASCE Nat. Haz. Rev. 16:04014022. doi: 10.1061/(ASCE)NH.1527-6996.0000153
Pita, G. L., Pinelli, J.-P., Gurley, K. R., and Hamid, S. (2013). Hurricane vulnerability modeling: development and future trends. J. Wind Eng. Ind. Aerod. 114, 96–105. doi: 10.1016/j.jweia.2012.12.004
Prince, S. H. (1920). Catastrophe and Social Change Based Upon a Sociological Study of the Halifax Disaster. Chapel Hill: Project Gutenberg.
Quarantelli, E. (2003). A Half Century Of Social Science Disaster Research:Selected Major Findings And Their Applicability. University of Delaware Preliminary Paper No. 336. Newark, DE: Disaster Research Center.
RAPID Facility (2017). Science Plan. Available online at: https://rapid.designsafe-ci.org/media/filer_public/f5/a8/f5a875ed-6061-4795-89ab-eeb8f9c24d67/additional_materials.pdf (accessed June 19, 2020).
Rathje, E., and Franke, K. (2016). Remote sensing for geotechnical earthquake reconnaissance. Soil Dyn. Earthq. Eng. 91, 304–316. doi: 10.1016/j.soildyn.2016.09.016
Rathje, E. M., and Bray, J. D. (2000). Nonlinear coupled seismic sliding analysis of earth structures. J. Geotech. Geoenviron. Eng. 126, 1002–1014. doi: 10.1061/(ASCE)1090-0241(2000)126:11(1002)
Rathje, E. M., Dawson, C., Padgett, J. E., Pinelli, J.-P., Stanzione, D., Adair, A., et al. (2017). DesignSafe: new cyberinfrastructure for natural hazards engineering. Nat. Haz. Rev. 18:06017001. doi: 10.1061/(ASCE)NH.1527-6996.0000246
Reid, H. F. (1910). The Mechanics of the Earthquake, The California Earthquake of April 18, 1906; Report of the State Investigation Commission. Washington, DC: Carnegie Institution of Washington.
Roelvink, D., Reniers, A., van Dongeren, A., van Thiel de Vries, J. A., Mccall, R., and Lescinski, J. (2009). Modelling storm impacts on beaches, dunes and barrier islands. Coast. Eng. 56, 11–12. doi: 10.1016/j.coastaleng.2009.08.006
Schenk, G. J. (2007). Historical disaster research. state of research, concepts, methods and case studies. Histor. Soc. Res. 32:3.
Shaghaghi, A., Bhopal, R., and Sheikh, A. (2011). Approaches to recruiting ‘hard-to-reach’ populations into research: a review of the literature. Health Promot. Perspect. 1, 86–94. doi: 10.5681/hpp.2011.009
Silva-Tulla, F., and Nicholson, P. (2007). Embankments, Dams, and Slopes: Lessons From the New Orleans Levee Failures and Other Current Issues. Reston, VA: American Society of Civil Engineers.
Smith, T., Holmes, W., and Edge, B. (2017). Natural Hazards Engineering Research Infrastructure, Five-Year Science Plan, Multi-Hazard Research to Make a More Resilient World, 1st Edn, Arlington, VA: DesignSafe-CI.
Spence, P., Lachlan, K., and Rainear, A. (2016). Social media and crisis research: data collection and directions. Comput. Hum. Behav. 54, 667–672. doi: 10.1016/j.chb.2015.08.045
Stone, H., D’Ayala, D., and Wilkinson, S. (2017). The Use of Emerging Technology in Post- Disaster Reconnaissance Missions, Earthquake Engineering Field Investigation Team Report. Available online at: https://www.istructe.org/IStructE/media/Public/Resources/report-eefit-grant-emerging-technology-reconnaissance-20170131.pdf (accessed June 5, 2020).
Tülüveli, G. (2015). Historical seismicity in the Middle East: new insights from Ottoman primary sources (sixteenth to mid- eighteenth centuries). J. Seismol. 19, 1003–1008. doi: 10.1007/s10950-015-9499-7
United Nations (2017). United Nations Office for Disaster Reduction, Terminology. Available online at: www.undrr.org/terminology/resilience (accessed October 9, 2020).
United Nations (2020). United Nations Office for Disaster Reduction, Terminology. New York, NY: United Nations.
van de Lindt, J. W., Ellingwood, B., McAllister, T., Gardoni, P., Cox, D., Cutler, H., et al. (2015). “Computational environment for modeling and enhancing community resilience: Introducing the center for risk-based community resilience planning,” in Proceedings of the 2nd International Conference on Performance-based and Life-Cycle Structural Engineering (PLSE 2015), Gaithersburg, MD.
Wang, Y., and Taylor, J. (2018). Coupling sentiment and human mobility in natural disasters: a Twitter-based study of the 2014 South Napa Earthquake. Nat. Hazards 92, 907–925. doi: 10.1007/s11069-018-3231-1
Wartman, J., Berman, J., Olsen, M. J., Irish, J. L., Miles, S., Gurley, K., et al. (2018). “The NHERI RAPID facility; enabling the next-generation of natural hazards reconnaissance,” in Proceedings of the 11th U.S. National Conference of Earthquake Engineering, Los Angeles, CA.
Wartman, J., Dunham, L., Tiwari, B., and Pradel, D. (2013). Landslides in eastern Honshu induced by the 2011 off the Pacific Coast of Tohoku earthquake. Bull. Seismol. Soc. Am. 103, 1503–1521. doi: 10.1785/0120120128
Wartman, J., Montgomery, D. R., Anderson, S. A., Keaton, J. R., Benoît, J., Dela Chapelle, J., et al. (2016). The 22 March 2014 Oso landslide, Washington, USA. Geomorphology 253, 275–288. doi: 10.1016/j.geomorph.2015.10.022
Wood, P. R., Elwood, K., Brunsdon, D. R., and Horspool, N. A. (2017). “Learning from earthquakes; past, present, future,” in Proceedings of the 2017 NZSEE Annual Technical Conference, Wellington, NZ.
Wood, R. L., Mohammadi, M. E., Barbosa, A. R., Abdulrahman, L., Soti, R., Kawan, C. K., et al. (2017). Damage assessment and modeling of the five-tiered pagoda-style Nyatapola temple. Earthq. Spec. 33, 377–384. doi: 10.1193/121516eqs235m
Xiao, Y., and Peacock, W. G. (2014). Do hazard mitigation and preparedness reduce physical damage to businesses in disasters? Critical role of business disaster planning. ASCE Nat. Haz. Rev. 15:137. doi: 10.1061/(ASCE)NH.1527-6996.0000137
Xiao, Y., and Van Zandt, S. (2012). Building community resiliency: spatial links between household and business post-disaster return. Urban Stud. 49, 2523–2542. doi: 10.1177/0042098011428178
Yamazaki, F., and Matsuoka, M. (2007). Remote sensing technologies in post-disaster damage assessment. J. Earthq. Tsunami 1, 93–210. doi: 10.1142/S1793431107000122
Yim, S. C., Olsen, M. J., Cheung, K. F., and Azadbakht, M. (2014). Tsunami modeling, fluid load simulation, and validation using geospatial field data. J. Struct. Eng. ASCE Special Issue Comp. Sim. Struct. Eng. 140, 1–14. doi: 10.1061/(ASCE)ST.1943-541X.0000940
Keywords: natural hazard, disaster, reconnaissance, instrumentation, simulation, data
Citation: Wartman J, Berman JW, Bostrom A, Miles S, Olsen M, Gurley K, Irish J, Lowes L, Tanner T, Dafni J, Grilliot M, Lyda A and Peltier J (2020) Research Needs, Challenges, and Strategic Approaches for Natural Hazards and Disaster Reconnaissance. Front. Built Environ. 6:573068. doi: 10.3389/fbuil.2020.573068
Received: 16 June 2020; Accepted: 28 September 2020;
Published: 10 November 2020.
Edited by:
Tiziana Rossetto, University College London, United KingdomReviewed by:
Richard L. Wood, University of Nebraska–Lincoln, United StatesCarmine Galasso, University College London, United Kingdom
Copyright © 2020 Wartman, Berman, Bostrom, Miles, Olsen, Gurley, Irish, Lowes, Tanner, Dafni, Grilliot, Lyda and Peltier. This is an open-access article distributed under the terms of the Creative Commons Attribution License (CC BY). The use, distribution or reproduction in other forums is permitted, provided the original author(s) and the copyright owner(s) are credited and that the original publication in this journal is cited, in accordance with accepted academic practice. No use, distribution or reproduction is permitted which does not comply with these terms.
*Correspondence: Joseph Wartman, d2FydG1hbkB1dy5lZHU=