- Building Materials, Department of Civil, Environmental and Natural Resources Engineering, Luleå University of Technology, Luleå, Sweden
Digital transformation of concrete technology is one of the current “hot topics” tackled by both academia and industry. The final goal is to fully integrate the already existing advanced concrete technologies with novel sensors, virtual reality, or Internet of things to create self-learning and highly automated platforms controlling design, production, and long-term usage and maintenance of concrete and concrete structures. The digital transformation should ultimately enhance sustainability, elongate service life, and increase technological and cost efficiencies. This review article focuses on up-to-date developments. It explores current pathways and directions seen in research and industrial practices. It indicates benefits, challenges, and possible opportunities related to the digital transformation of concrete technology.
Introduction
Digitization refers to transfer of data stored in traditional documents to binary forms, while digital transformation is defined as a process of changing existing methods and models by utilizing latest IT technologies to produce real-time information for fast decision making (Parusheva, 2019; Zeltser et al., 2019; Daniotti et al., 2020; Papadonikolaki et al., 2020). For cement and concrete industries, it facilitates the process of data acquisition, their analysis, and utilization (Walther, 2018). Production of concrete starts with material characterization, mix design, and actual mixing followed by its transportation to a building site (Tomek, 2017). A significant amount of data created can be digitalized and used to control that process (Rasmussen and Beliatis, 2019). The digital transformation is expected to produce a more efficient process, improving the working environment and sustainability of concrete products (Phang et al., 2020). However, a number of challenges still need to be addressed, for example, methods for reliable prediction of early-age properties, modeling of hardening processes, and development of strength or durability (Wangler et al., 2019).
Concrete structures can be cast directly on a building site or prefabricated in advanced in a factory. The cast-on-site technique is preferable for monolithic, large-size structures including foundations, beams, columns, slabs, retaining walls, tunnels, and bridges (Liu et al., 2020). Concrete is transported from a ready-mix plant to the building site and then placed using pumps or dumpers. In the case of precast technology, concrete elements are cast in production halls and after achieving sufficient strength, transported to the building site. The cast-in-place technology offers more flexibility and adaptability (Simonsson and Emborg, 2009). Weakness includes sensitivity to weather, that is, extreme temperatures, wind, and precipitation. The current industrialization degree of concrete technology is relatively high, but it still requires several improvements in the quality of work, optimization of the process, and enhanced sustainability. It is foreseen that there is a possibility to expedite the process using the latest digitalization techniques and technological advancements (Wangler et al., 2016). Self-compacting concrete (SCC) is increasingly used, especially for the cast-in-place technique, which, due to the exclusion of vibration, offers a faster construction process and better working conditions (Ouchi, 2000). The main advantages include high casting rate and passability in congested reinforcement (De Schutter et al., 2008). The main challenge while using SCC is a need to use a new casting technology (Ferrara et al., 2007).
The digitalization process starts by merging material properties and construction techniques into an integrated digital environment. It includes digitalizing of fresh concrete properties, hardening processes, strength development, and durability using data collected from either manual measurements or installed sensors. The integration of measured parameters and digital technology enables to enhance the quality of concrete. However, it requires a strengthened collaboration between research and industry (Courard et al., 2014). Data collected from sensors can be integrated into a monitoring system, building information models, and controlling software. This process is expected to introduce a safer and error-free process and improve the productivity. The site supervisor has real-time access to data, which should facilitate the decision-making process related to, for example, the optimum casting speed, safe demolding time, or the required curing routine.
Research has been on going in the field of digital concrete, which refers to the digital fabrication of concrete, for example, 3D printing and robotics in digital fabrication (Wangler et al., 2016; Wangler et al., 2019; Van Damme, 2020). Those studies have explored the methods of fabrication and construction. The basic properties, mix design parameters, and their associated information need to be addressed. Commonly, these data are obtained in the laboratory, and the question remains open about the possibility of transforming the information acquisition into a digital process. This article reviews previous research dealing with digital transformation in concrete technology, and it focuses on latest developments with a special emphasis on disadvantages and limitations. It also indicates areas that need further improvements. This article is part of a project where attempts are made to develop a system that can help integrate all the available technologies into one smart decision-making system that enables engineers to foresee and expect the outcome of the mix design based on the inputs of material properties either physically or chemically related.
Material Characterizations and Mix Design
Advanced technologies such as virtual reality, 3D printing, Internet of things, smart sensors, and autonomous robots and vehicles have already been used in various industries. However, the concrete industry is clearly behind due to the lack of acceptance, related cost, current regulations, and new required expertise. Concrete itself has gone a tremendous development path over the past few decades. Cement has been partially or fully replaced with several types of by-products to enhance some properties and to increase its sustainability. At the same time, casting technology has remained rather unchanged (Ferrara et al., 2007).
Concrete consists of binder, coarse and fine aggregate, water, admixtures, and various types of dry and wet additives. These materials are characterized by chemical composition, surface area, shape, texture, and amount of intermixed fine and coarse aggregates. These properties affect the mix design and behavior of concrete during mixing and casting and later determine hardened state properties and, often, also durability (Polat, 2013). The following sections will review currently used methods which are/or could be used to digitalize the properties of concrete ingredients.
Aggregates
Aggregates used in concrete include gravel, crushed stone, sand, slag, recycled concrete, and geosynthetic aggregates. They occupy up of 70–80 vol.% of concrete mix and affect most of its physical and mechanical properties. Aggregates should be clean, hard, and free of chemical and biological contaminants (Babu, 2014). Their quality and properties are quantified by several indicators, including shape, texture, air content, particle size distribution, water content, specific gravity, or density. Some of these indicators have already been successfully digitalized. For example, volume, angularity, and gradation have been determined using analysis of images obtained from video cameras. The obtained results have been in good agreement with manual measurements (Rao and Tutumluer, 2000). 3D mathematical analysis of particle shape has been successfully combined with X-ray tomography and spherical harmonics to determine particle shapes (Garboczi, 2002). Others used the same technique but supplemented it with a virtual reality modeling language. This approach enabled to obtain 3D images of aggregate particles (Erdogan et al., 2006). The surface texture has been determined using imaging techniques coupled with wavelet analysis of grey images. Unfortunately, results were strongly affected by the angularity and form of aggregates (Al-Rousan et al., 2007). The shape index and morphological features of coarse aggregates have been assessed by a digital processing approach, which established a correlation between the shape of aggregate and mechanical properties of asphalt concrete (Arasan et al., 2011). The shape of aggregates affected the required cement content, as well as the mechanical properties and durability of the produced concrete. Content of air voids in aggregates can be directly linked to the observed water demand. It has been determined by a feed-forward neural network with the error back-propagation algorithm using artificial neural networks (ANNs) and multiple linear regression with specific toolkits such as NTR2003 and WEKA (Zavrtanik et al., 2016). Digitalization of other properties, that is, water content, specific gravity, and density, appears to be still at a very early stage. A summary of research related to the digitalization of aggregate properties is shown in Table 1.
Cement
Selection of cement type and its amount must ensure achieving the targeted fresh and hardened state properties. The decision-making process is usually strongly regulated and depends, for example, on the exposure conditions or planned service life of the structure. Potentially, it could be automated through digitalization by utilizing research data collected over the last few decades combined with regulations and practical observations. As it will be shown later, most methods used in the current practice provide digital data which could be implemented into IT platforms. For example, Hughes et al. (1995) used Fourier-transform infrared (FTIR) spectroscopy to determine the cement composition, while Hamza et al. (2017) established the impact of the cement type on the resistance of concrete to sulfate attack. Suryani et al. (2020) determined the structural and optical properties of cement with the aid of X-ray diffraction (XRD). It included crystal size, microstrain, energy deformation, and stress.
The specific surface area of cement is a crucial parameter when selecting the cement type. Larger surface enhances the hydration process (Neville and Brooks, 1987). This parameter has been determined by various techniques, for example, neutron scattering, gas sorption, small-angle scattering, nuclear magnetic resonance imaging, X-ray scattering, and mercury intrusion porosimetry (Winslow and Diamond, 1974; Olek et al., 1990; Thomas et al., 1998). Unfortunately, none is digitalized and require additional manual work to transform collected data into a usable digital format (Thomas et al., 1999). Ferraris and Garboczi (2013) measured the particle size and specific surface area by laser diffraction X-ray computed microtomography, which enabled to determine particles as small as 45 μm. Another method is laser diffraction spectrometry, which determines the particle size by spreading the light around the particle’s contours (Hackley, 2004). It is able to detect particles having diameters in the range between 10 μm and 1 mm (Bowen, 2002). Erdogan (2010) used the X-ray microcomputed tomography technique incorporated with spherical harmonic analysis to determine the 3D shape of cement particles for characterizing cement, based on particle shape and chemical composition. In that case, the used spherical harmonic analysis enabled to determine the particle length, width, and thickness. The average shape of cement particles has been correlated with the volume fraction of belite and alite. A summary of digitalization of cement properties is given in Table 2.
Concrete Mix Design
The concrete mix design establishes the proportions and type of its constituents, that is, binder or binders, aggregates, fillers, water, chemical additives, admixtures, and possible fibers. The concrete mix design along with other factors, especially including, casting technology, curing procedure, and environmental conditions, determines the ultimate workability, strength, or durability of concrete. The concept of digitalizing the concrete mix design has been used for a relatively long time already. For example, the water-to-cement ratio has been determined using a near-field microwave technique with an open-ended rectangular waveguide probe radiating into OPC materials at 5 GHz (G-band) and 10 GHz (X-band) (Bois et al., 1998). The same concept has been also applied to determine the coarse aggregate-to-cement (ca/c) ratio (Bois et al., 2000). A real-time, on-site evaluation of the water-to-cement ratio (w/c) used microwave non-destructive testing (Mubarak et al., 2001). A monopole antenna probe, operating at 3 GHz with a reflectometer, has been also used to efficiently determine the w/c ratio (Providakis et al., 2011). The concrete mix design has been also optimized by artificial neural networks (ANNs) using various input data, for example, workability or compressive strength (Ji-Zong et al., 1999; Yeh, 1999; Ji et al., 2006; Ziolkowski and Niedostatkiewicz, 2019). The method enabled estimation of dosage of materials, choice of the type of cement, and effects of chemical and mineral admixtures (Ji-Zong et al., 1999). The same concept but with different design algorithms has been used to estimate nominal and equivalent w/c ratios, fly ash (FA)-to-binder ratio, and aggregate size (Ji et al., 2006). Others used a set of concrete recipes to optimize the mix design based on maximum aggregate size, slump, fineness modulus, and compressive strength by incorporating an adaptive neural fuzzy inference system (Neshat, 2012). Recently, a machine learning algorithm has been used to optimize the mix (Ziolkowski and Niedostatkiewicz, 2019). Concrete mixes for 3D printing were designed to obtain the required extrudability, buildability, workability, and open time (Lediga and Kruger, 2017). A summary of digitalized methods and tools used in the concrete mix design is shown in Table 3.
Concrete Properties
Concrete Temperature
The temperature of fresh concrete and the ambient temperature are very important parameters while designing concrete mix composition, or planning, transporting, casting, and curing (Shoukry et al., 2011). Generally, high temperature accelerates the hydration process, which might require addition of retarders, decreasing the amount of cement, or addition of certain secondary cementitious materials (SCMs) (Gamil et al., 2019). On the contrary, a lower temperature slows down the hydration process and delays strength development (Ma et al., 2015). To counteract these effects, accelerators can be used in combination with, for example, rapid hardening cement and heat curing (Alhozaimy, 2009; Fang et al., 2018). Most standards limit the maximum concrete temperature to prevent cracking, lower strength, and delayed ettringite formation (Hale et al., 2005).
Digitalization of concrete temperature measurement is rather advanced (Wong et al., 2007; Norris et al., 2008; Barroca et al., 2013; Chen and Wu, 2015; Kim et al., 2015; Liu et al., 2017). State-of-the-art technologies with embedded sensors have been used. One common technology used to monitor the temperature is thermal imaging using infrared thermography. This technology is non-destructive, but it is applicable only to concrete not exposed to sunlight (Tran et al., 2017). Other techniques include, for example, fiber Bragg grating sensors, which are used to monitor temperature and shrinkage at the same time (Wong et al., 2007). Embedded nanotechnology/microelectromechanical systems (MEMS) sensors have been used to monitor moisture and temperature of concrete at the same time. Unfortunately, issues with repeatability and signal processing have been faced (Norris et al., 2008). Embedded thermal sensors have been used for temperature monitoring, but the thermography sensors must be in visual contact with the monitored concrete. It might be difficult to achieve due to, for example, form covers or other materials present on the concrete surface (Azenha et al., 2011). To overcome this drawback, automatic wireless sensors were used, but a 5 °C discrepancy was observed between actual and experimental values (Barroca et al., 2013). Another example is the so-called passive wireless surface acoustic wave (SAW) sensor combined with orthogonal frequency coding (OFC). The main constraints were related to the effect of propagation loss and isotropic radiation loss (Kim et al., 2015). Sensors utilizing passive radio frequency identification (RFID) and radio frequency integrated circuit (RFIC) (Chen and Wu, 2015; Liu et al., 2017) enabled short-range remote sensing and achieved the detection resolution of 0.25 °C (Chen and Wu, 2015; Liu et al., 2017). Their main shortcoming was the signal instability and a lack of electronic protection (Chang and Hung, 2012). A summary of methods and tool for digitalization of concrete temperature monitoring is given in Table 4.
Workability
Workability is an essential technological property of concrete controlling the casting process and affecting the quality of produced concrete elements or structures. It can be measured, for example, by slump or slump flow combined with T50 time in the case of self-compacting concrete (Fares, 2015). A number of digitalizing solutions have been introduced, and artificial neural networks (ANNs) is one the examples (Bai, 2003; Yeh, 2006a; Oztas, 2006; Yeh, 2009; Kim and Park, 2018). They produce a more accurate prediction of workability than the non-linear regression analysis (Yeh, 2006a), and it has the ability to model the slump for any mix design (Yeh, 2009). Another example method is based on 3D depth sensors (Kim and Park, 2018). Rheological properties of concrete described by the yield stress and the plastic viscosity are crucial for designing self-compacting concrete mixes (Wallevik, 2003; Roussel, 2011) (Ferraris et al., 2012). An effective device called 4C-Rheometer was developed by the Danish Technological Institute (Danish Technological Institute and C.C, 2020). It enabled to determine rheology based on automated measurements of slump flow and flow time. A summary of digitalization of workability measurements is given in Table 5.
Setting Time and Hydration Rate
Initial and final setting times of cement are used to monitor the hardening rate. The initial setting time indicates how long concrete mix maintains its plasticity. It indicates the allowable time to cast the concrete. The final setting time indicates the time after which concrete loses its plasticity, and it is especially useful for planning surface finishing processes. Both times are related to the hydration process, which can be monitored using calorimetry and measuring the evolved heat (Mostafa and Brown, 2005; Xu, 2011; Gawlicki et al., 2010). Parameters affecting the degree of cement hydration are summarized in Figure 1 (Xu et al., 2010).
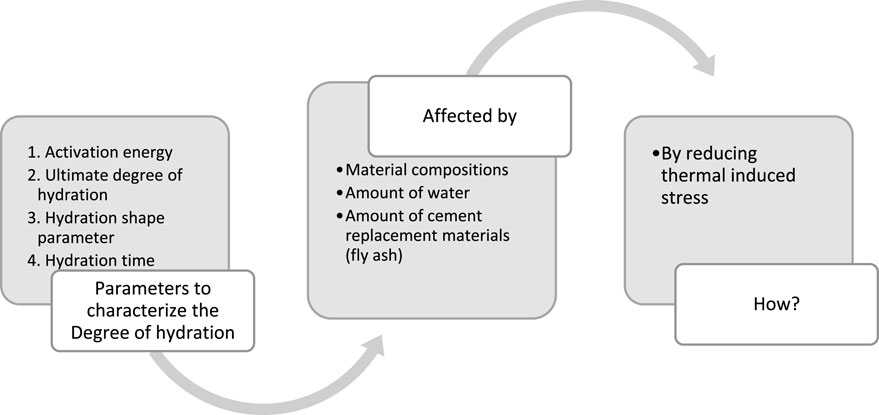
FIGURE 1. Parameters and process used to characterize cement hydration (summarized from the work of Xu et al., 2010).
Several attempts were made to digitalize the assessment of the setting time. For example, Rizzo et al. (2014) used a non-destructive setup measuring strength development by sensors detecting the propagation of highly non-linear solitary waves (HNSWs). The waves were reflected at the sensor interface and transmitted to the monitored concrete. The transmission time and the reflection from the interface were measured and compared with the hydration time. These parameters were then correlated with initial and final setting times measured by using the Vicat apparatus. The hydration rate has been also monitored using the Fabry–Perot fiber optic temperature sensor. The concrete temperature depended on the water-to-cement ratio (Zou et al., 2012). Yet another effective method to digitalize the hydration rate is the monitoring of the crack formation (Yang et al., 2010). The hydration degree was also assessed by the thermogravimetric analysis (Deboucha et al., 2017). The method estimated the ultimate amount of bound water, which was verified by isothermal calorimetry combined with the assessment of compressive strength. The differential thermal and thermogravimetric analysis was also used to estimate the degree of hydration. In that case, the degree of hydration was calculated using experimental results. A good agreement between results based on differential thermal and thermogravimetric analysis was observed (Monteagudo et al., 2014).
The hydration process can also be measured using other methods, including X-ray diffraction (XRD), scanning electron microscopy (SEM), thermogravimetric analysis (TGA), or non-contact impedance measurement (NCIM) (Tang et al., 2016). For example, XRD was combined with calorimetry to monitor the hydration of cement blended with fly ash for the first 44 h. It enabled estimating the effects of fly ash (FA) (Dittrich et al., 2014).
Concrete Maturity
Maturity is an indicator used to predict strength development depending on the curing temperature (Chengju, 1989; McCullough and Rasmussen, 1999; Topçu and Toprak, 2005; Zhang et al., 2008; Yikici and Chen, 2015). The required (Ballim and Graham, 2009; Lee and Hover, 2015) systems based on that concept have been developed. For example, high-performance concrete paving (HIPERPAV) software utilized temperature data and the maturity concept to estimate the concrete strength at an early age (Ruiz, 2001). Another system developed by Giatec Scientific Inc.is based on wireless temperature sensors integrated with a special smartphone application. It enables live monitoring, but the maximum allowable distance between the sensor and the monitored concrete surface is limited (De Carufel, 2018).
Mechanical Properties
The compressive strength of concrete is certainly the most commonly used indicator of mechanical properties (Damineli et al., 2010; Yang et al., 2010; Ma et al., 2015). It is usually determined using a cube compression test, which is a time-consuming process. Consequently, several models have been created to reliably predict the strength without the need of physical testing. The ANNs method, described earlier, has been used in several studies (Lee, 2003; Kim et al., 2004; Yeh, 2006b; Prasad et al., 2009). It could estimate the compressive strength taking into account slump, air content, and fly ash amount as indicators in PreConS (intelligent system of strength). Unfortunately, the system showed a lower reliability at variable curing temperatures (Lee, 2003). Others used the ANN approach but based on different concrete mix proportions (Kim et al., 2004). In that case, literature data were used to estimate the compressive strength of SCC and high-performance concrete (HPC) taking into account the volume of fly ash and the water-to-cement ratio (Prasad et al., 2009). ANNs were also combined with the image processing technique and design of experiments to estimate the strength (Dogan et al., 2017; Waris et al., 2020). It enabled prediction of various mechanical properties, including compressive strength, modulus of elasticity, and maximum deformation, reaching 98.65% accuracy. ANNs were also efficiently incorporated in an approach based on utilizing data obtained from ultrasonic pulse velocity (UPV) measurements (Kewalramani and Gupta, 2006). Similarly, a neural expert system was used to predict the strength based on results from testing a total of 864 concrete specimens. The applied ANN model used a back-propagation learning algorithm, and the results were compared to a built-in expert system, which enabled prediction of the strength using rule-based knowledge representation techniques (Gupta et al., 2006). Both compressive and tensile strength of high-performance concrete were determined using a modified firefly algorithm–artificial neural network expert system. A good correlation between actual and predicted results was achieved (Bui et al., 2018).
A deep learning prediction method has been applied to predict the compressive strength of recycled aggregate concrete. The model used the water-to-cement ratio and the recycled aggregate replacement percentage as input parameters. Tests were performed on 74 concrete blocks. The achieved precision was higher than that of a traditional neural network (Deng et al., 2018). A machine learning approach has been utilized to predict the compressive strength at different ages for concrete with high fly ash content. The water cycle algorithm and the genetic algorithm showed a good correlation between the variation of fly ash content and compressive strength (Naseri, 2020).
Real-time prediction of the compressive strength has been carried out using data obtained using novel types of sensors (Providakis et al., 2011; Tareen et al., 2019; John et al., 2020). The early-age concrete strength was effectively estimated using data obtained from the active wireless sensing system (John et al., 2020). It used an electromechanical impedance measuring chip and a piezoelectric transducer installed in a Teflon-based (Providakis et al., 2011). Other approaches to predict the early strength used smart temperature (SmartRock) and PZT (piezoelectric) sensors with ultrasonic wave propagation combined with the concrete maturity concept (Tareen et al., 2019). Recently, the technology of Internet of things (IoT) was utilized to estimate the compressive strength using temperature sensors and Wi-Fi microcontrollers. The technology enabled real-time monitoring of strength (John et al., 2020). A summary of digitalization methods for prediction of compressive strength is shown in Table 6.
Crack Monitoring
Crack monitoring remains a major concern in the concrete industry, and it is crucial for safety and maintenance costs (Omondi et al., 2016). Concrete cracks are caused by two effects, that is, extrinsic and intrinsic (Li et al., 2018a). The former is induced by the application of excessive loads. The intrinsic effects are related to the hardening process and are considered as non-structural. Intrinsic cracks are controlled by the mix design, mixing method, ambient temperature, and humidity (Bolleni, 2009). Automated crack detection and monitoring are still in the developmental stage, and various approaches have been considered. Digital image processing is certainly one of the most used methods (Dare et al., 2002; Chen et al., 2006; Nagy, 2014; Gehri et al., 2020). An automated image processing technique with multitemporal crack measurements detected the extrinsic cracks in concrete. The automatic method accurately delineated cracks even when using poor-quality images (Dare et al., 2002). The same method was applied to study the relationship between the crack width and its expansion with multitemporal image processing. In that case, images were taken every 2 weeks with a high-resolution scanner. The method enabled automatic crack tracing and showed a good correlation between the estimated width and the manual measurement (Chen et al., 2006). Crack width was also measured by two emerging technologies, that is, the image digitalizing method and the digital image processing (DIP) method combined with a digital microscope that enabled mapping the tortuosity of cracks (Nagy, 2014). An example process of transforming crack monitoring data into a digital form is shown in Figure 2. The process starts by taking an image of the crack followed by adjustment and cropping of the crack line. In the next step, pixel coordinates are used to determine the crack width (Nagy, 2014).
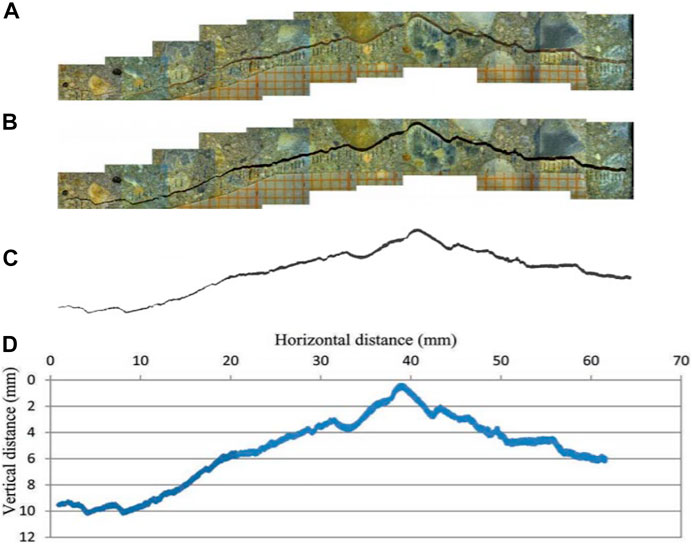
FIGURE 2. Example of crack width measurement using digital image processing (Nagy, 2014).
The same technology has been used to monitor the crack behavior and the crack orientation by extracting images with the digital image correlation (DIC) method (Gehri et al., 2020). The obtained results were limited only to closely spaced cracks. DIC has been also used to study the fracture behavior of concrete interfaces (Shah and Chandra Kishen, 2011). The used optical and non-contact measurement tool analyzed the displacement of the surface using images obtained before and after the displacement occurred. Another application of DIC has been monitoring and measuring deformation developing in compression (Choi and Shah, 1997). Results showed a well-balanced image rate for both lateral and axial deformation after the peak load.
More advanced methods were applied to determine the crack width and length using a digital camera embedded in a calibrated cylindrical attachment. The crack width could be estimated reliably, but the obtained results strongly depended on the operator (Dare et al., 2002).
Other new technologies that have been used to detect and monitor cracking of concrete include thermography (Bolleni, 2009), combined acoustic emission and digital image correlation techniques (Omondi et al., 2016), local binarization algorithm (Li et al., 2018b), and ultrasound-excited thermography (Jia et al., 2019). Thermography uses a thermal camera based on the infrared radiation, and it does not require a direct access to concrete layers to detect the damage (Bolleni, 2009). This method has also been combined with the ultrasound-excited thermography and enabled detection of microcracks having width between 0.01 and 0.09 mm (Jia et al., 2019). DIC has been successfully combined with acoustic emission technology to detect cracks and determine their orientation (Omondi et al., 2016). Yet another tested approach is a technology based on a local binarization. The color of the image is transferred into a binary image that has two colors, typically black and white. The image is then processed to detect the surface and cross-sectional area of present cracks (Li et al., 2018b). A summary of digitalization of crack formation in concrete is shown in Table 7.
Discussion
More pieces of information were involved in the production of concrete, such as raw material characterizations, mix design, and properties of ready concrete, which are essential parameters used to envisage the quality of the end-product. Mostly, this information is acquitted manually in the laboratory. This process is time consuming, and technical experts need time to make quick judgments about modifying the mix design or developing the mix for specific use and environment condition. To save time and produce favorable and good-quality concrete, transforming information acquisition to real-time updates using digital technologies is preferred. The possibility of digital transformation of these essentials seems to be valid and possible; perhaps, more integration of different technologies can work efficiently to develop a system to obtain and communicate concrete information. The information needed from the source of raw materials at the quarry sites and the cement production plant by the engineer who develops the mix is surface area, specific gravity, shape, gradation, etc. Having this information on time will allow the mix design developer to adjust the proportions for the specific needs. Then, during the casting process, engineers need to monitor the concrete temperature, workability, formwork pressure, which is not discussed in this article, casting rate, maturity of the concrete to decide on the formwork removal time, mechanical properties, and crack monitoring. The question comes about merging all this information in one complete system using emerged technologies with embedded sensors and IoT for instantaneous communication, Figure 3. Extensive research has been carried out, as discussed in this article, to gradually transform the data acquisition into a digital form. Still, not all the attempts have been applied at the jobsite. There are reasons and challenges for low acceptance, and the process involves consideration of a multitude of stages. The first is the availability and accessibility of technology. Then, the question comes about the acceptance and confidence from the side of construction stakeholders of the technology, and that incurs some cost and expertise; these restrains need to be addressed through intensive research and full-scale experiments. It is suggested for future development to integrate the current technologies and applications into one integrated system for possible information acquisitions and instant communication.
Conclusion
Digitalization can be defined as converting information into a digital format and using these data to control, for example, the production and usage of concrete. Digital transformation enables us to save time and cost, facilitates access to information, and increases efficiency and readiness. In the concrete industry, the digital transformation of concrete properties and production helps to create a more consistent and faster construction process. Availability of real-time data enables engineers to follow and control the entire production process more efficiently and with higher reliability. Access to data is facilitated by, for example, cloud storage platforms. For example, the construction process can be accelerated and made safer by more accurate prediction of the formwork removal timing. In the current era, more advanced digital concrete has been introduced, and that technology needs to be coupled with the digitalized process of concrete data acquisition.
The real-time data assist engineers and managers in the decision-making process. The decision can be related, for example, to optimizing the mix design by reducing the usage of raw materials, thus leading to enhanced sustainability. On the negative side, the digital transformation, in the case of concrete technology, is a complex process due to not yet fully understood basic processes controlling, for example, hydration of Portland cement. An even worse situation is faced in the case of new ecological binders. Only for these reasons, it is extremely difficult to develop reliable models. Models which could be used to design concrete mixes predict strength development, crack formation, or deterioration due to various types of exposures. Another set of problems is related to the acceptance of the concrete and construction industry as well as compliance with current regulations and standards. There is also a need to ensure that the acquired data are communicated and stored correctly, analyzed, and interpreted by the responsible personnel. Other challenges include proper installation of sensors, data collection and storage devices, and data safety or data transmission.
There is still a significant amount of work to be completed before benefits of digitalization could be fully utilized in concrete technology. Problems to be solved are related not only to basic phenomena, for example, hydration of cement, but also to full-scale real-life applications with a number of factors not being present in laboratory settings.
Author Contributions
YG has established the concept of the article, collected and analyze the data while AC has reviewed and supervise the work. He also contributed to proofreading and revising the article critically for important intellectual content.
Funding
This research was funded by the Development Fund of the Swedish Construction Industry (SBUF) and NCC construction company.
Conflict of Interest
The authors declare that the research was conducted in the absence of any commercial or financial relationships that could be construed as a potential conflict of interest.
Publisher’s Note
All claims expressed in this article are solely those of the authors and do not necessarily represent those of their affiliated organizations, or those of the publisher, the editors, and the reviewers. Any product that may be evaluated in this article, or claim that may be made by its manufacturer, is not guaranteed or endorsed by the publisher.
Acknowledgments
The authors acknowledge the financial support from the funding agencies of the project and Lulea University of Technology for the research material support.
References
Al-Rousan, T., Masad, E., Tutumluer, E., and Pan, T. (2007). Evaluation of Image Analysis Techniques for Quantifying Aggregate Shape Characteristics. Construction Building Mater. 21 (5), 978–990. doi:10.1016/j.conbuildmat.2006.03.005
Alhozaimy, A. M. (2009). Effect of Absorption of limestone Aggregates on Strength and Slump Loss of concrete. Cement and Concrete Composites 31 (7), 470–473. doi:10.1016/j.cemconcomp.2009.04.010
Arasan, S., Yenera, E., Hattatoglu, F., Hinislioglua, S., and Akbuluta, S. (2011). Correlation between Shape of Aggregate and Mechanical Properties of Asphalt Concrete. Road Mater. Pavement Des. 12 (2), 239–262. doi:10.1080/14680629.2011.9695245
Azenha, M., Faria, R., and Figueiras, H. (2011). Thermography as a Technique for Monitoring Early Age Temperatures of Hardening concrete. Construction Building Mater. 25 (11), 4232–4240. doi:10.1016/j.conbuildmat.2011.04.065
Babu, V. (2014). Mechanical Properties of High Strength concrete with Recycled Aggregate-Influence of Processing. Indian Concr J. 88 (5), 10–26.
Bai, J. (2003). Using Neural Networks to Predict Workability of concrete Incorporating Metakaolin and Fly Ash. Adv. Eng. Softw. 34 (11-12), 663–669. doi:10.1016/s0965-9978(03)00102-9
Ballim, Y., and Graham, P. C. (2009). The Effects of Supplementary Cementing Materials in Modifying the Heat of Hydration of concrete. Mater. Struct. 42 (6), 803–811. doi:10.1617/s11527-008-9425-3
Barroca, N., Borges, L. M., Velez, F. J., Monteiro, F., Górski, M., and Castro-Gomes, J. (2013). Wireless Sensor Networks for Temperature and Humidity Monitoring within concrete Structures. Construction Building Mater. 40, 1156–1166. doi:10.1016/j.conbuildmat.2012.11.087
Basyigit, C., Comak, B., K̓l̓nçarslan, Ş., and Üncü, İ. S. (2012). Assessment of concrete Compressive Strength by Image Processing Technique. Construction Building Mater. 37, 526–532.
Bois, K. J., Benally, A. D., Nowak, P. S., and Zoughi, R. (1998). Cure-state Monitoring and Water-To-Cement Ratio Determination of Fresh Portland Cement-Based Materials Using Near-Field Microwave Techniques. IEEE Trans. Instrum. Meas. 47 (3), 628–637. doi:10.1109/19.744313
Bois, K. J., Benally, A. D., and Zoughi, R. (2000). Microwave Near-Field Reflection Property Analysis of concrete for Material Content Determination. IEEE Trans. Instrum. Meas. 49 (1), 49–55. doi:10.1109/19.836308
Bolleni, N. K. (2009). Environmental Effects on Subsurface Defect Detection in concrete Structures Using Infrared Thermography. Columbia, MO: University of Missouri-Columbia.
Bowen, P. (2002). Particle Size Distribution Measurement from Millimeters to Nanometers and from Rods to Platelets. J. Dispersion Sci. Tech. 23 (5), 631–662. doi:10.1081/dis-120015368
Bui, D.-K., Nguyen, T., Chou, J.-S., Nguyen-Xuan, H., and Ngo, T. D. (2018). A Modified Firefly Algorithm-Artificial Neural Network Expert System for Predicting Compressive and Tensile Strength of High-Performance concrete. Construction Building Mater. 180, 320–333. doi:10.1016/j.conbuildmat.2018.05.201
Chang, C.-Y., and Hung, S.-S. (2012). Implementing RFIC and Sensor Technology to Measure Temperature and Humidity inside concrete Structures. Construction Building Mater. 26 (1), 628–637. doi:10.1016/j.conbuildmat.2011.06.066
Chen, F. Deng., and Wu, X. (2015). “A Passive Rfid Sensor Tag for Intelligent concrete Temperature Control,” in Proceedings of the International Conference on Mechatronics, Materials, Chemistry and Computer Engineering (Shanghai, China. doi:10.2991/icmmcce-15.2015.223
Chen, L.-C., Shao, Y.-C., Jan, H.-H., Huang, C.-W., and Tien, Y.-M. (2006). Measuring System for Cracks in concrete Using Multitemporal Images. J. Surv. Eng. 132 (2), 77–82. doi:10.1061/(asce)0733-9453(2006)132:2(77)
Chengju, G. (1989). Maturity of Concrete - Method for Predicting Early-Stage Strength. Aci Mater. J. 86 (4), 341–353.
Choi, S., and Shah, S. P. (1997). Measurement of Deformations on concrete Subjected to Compression Using Image Correlation. Exp. Mech. 37 (3), 307–313. doi:10.1007/bf02317423
Courard, L., Piotrowski, T., and Garbacz, A. (2014). Near-to-surface Properties Affecting Bond Strength in concrete Repair. Cement and Concrete Composites 46, 73–80. doi:10.1016/j.cemconcomp.2013.11.005
Damineli, B. L., Kemeid, F. M., Aguiar, P. S., and John, V. M. (2010). Measuring the Eco-Efficiency of Cement Use. Cement and Concrete Composites 32 (8), 555–562. doi:10.1016/j.cemconcomp.2010.07.009
Daniotti, B., Gianinetto, M., and Della Torre, S. (2020). Digital Transformation of the Design, Construction and Management Processes of the Built Environment. Springer Nature.
Danish Technological Institute, C.C (2020). 4C-Rheometer – Measuring Rheology of Self Compacting Concrete - the Equipment. [cited 2021 August 2021]; Available from: https://www.dti.dk/4c-rheometer-8211-measuring-rheology-of-self-compacting-concrete/the-equipment/21743.
Dare, P., Hanley, H., Fraser, C., Riedel, B., and Niemeier, W. (2002). An Operational Application of Automatic Feature Extraction: The Measurement of Cracks in concrete Structures. Photogrammetric Rec. 17 (99), 453–464. doi:10.1111/0031-868x.00198
De Schutter, G., Bartos, P. J. M., Domone, P., Gibbs, J., and Concrete, S. C. (2008). Self-compacting concrete, 288. Scotland: Whittles Publishing Caithness, 288.
Deboucha, W., Leklou, N., Khelidj, A., and Oudjit, M. N. (2017). Hydration Development of mineral Additives Blended Cement Using Thermogravimetric Analysis (TGA): Methodology of Calculating the Degree of Hydration. Construction Building Mater. 146, 687–701. doi:10.1016/j.conbuildmat.2017.04.132
Deng, F., He, Y., Zhou, S., Yu, Y., Cheng, H., and Wu, X. (2018). Compressive Strength Prediction of Recycled concrete Based on Deep Learning. Construction Building Mater. 175, 562–569. doi:10.1016/j.conbuildmat.2018.04.169
Dittrich, S., Neubauer, J., and Goetz-Neunhoeffer, F. (2014). The Influence of Fly Ash on the Hydration of OPC within the First 44h-A Quantitative In Situ XRD and Heat Flow Calorimetry Study. Cement Concrete Res. 56, 129–138. doi:10.1016/j.cemconres.2013.11.013
Dogan, G., Arslan, M. H., and Ceylan, M. (2017). Concrete Compressive Strength Detection Using Image Processing Based New Test Method. Measurement 109, 137–148. doi:10.1016/j.measurement.2017.05.051
Erdogan, S. T. (2010). Micrometer-scale 3-D Shape Characterization of Eight Cements: Particle Shape and Cement Chemistry, and the Effect of Particle Shape on Laser Diffraction Particle Size Measurement. Cement Concrete Res. 40 (5), 731–739.
Erdogan, S. T., Quiroga, P. N., Fowler, D. W., Saleh, H. A., Livingston, R. A., Garboczi, E. J., et al. (2006). Three-dimensional Shape Analysis of Coarse Aggregates: New Techniques for and Preliminary Results on Several Different Coarse Aggregates and Reference Rocks. Cement Concrete Res. 36 (9), 1619–1627. doi:10.1016/j.cemconres.2006.04.003
Fang, G., Ho, W. K., Tu, W., and Zhang, M. (2018). Workability and Mechanical Properties of Alkali-Activated Fly Ash-Slag concrete Cured at Ambient Temperature. Construction Building Mater. 172, 476–487. doi:10.1016/j.conbuildmat.2018.04.008
Fares, G. (2015). Effect of Slump Cone Orientation on the Slump Flow Time (T50) and Stability of Sustainable Self-Compacting concrete Containing limestone Filler. Construction Building Mater. 77, 145–153. doi:10.1016/j.conbuildmat.2014.12.052
Ferrara, L., Shah, S. P., Ferron, R. P., Tregger, N., and Kwon, S. H. X. (2007). “Research on SCC: Some Emerging Themes,” in Scc2007, 5th International Rilem Conference on Self Compacting/Self Consolidating Concrete (RILEM PUBS), 3–14.
Ferraris, C. F., and Martys, N. S. (2012). “Concrete Rheometers,” in Understanding the Rheology of Concrete. Editor N. Roussel (Sawston, United Kingdom: Woodhead Publishing), 63–82. doi:10.1533/9780857095282.1.63
Ferraris, C., and Garboczi, E. (2013). Identifying Improved Standardized Tests for Measuring Cement Particle Size and Surface Area. Transportation Res. Rec. 2342 (2342), 10–16. doi:10.3141/2342-02
Gamil, Y., Bakar, I., and Loon, L. Y. (2019). Utilization of Normal and Treated Cement Kiln Dust as Cement Replacement Materials in Concrete. Pertanika J. Sci. Tech. 27 (1).
Garboczi, E. J. (2002). Three-dimensional Mathematical Analysis of Particle Shape Using X-ray Tomography and Spherical Harmonics: Application to Aggregates Used in concrete. Cement Concrete Res. 32 (10), 1621–1638. doi:10.1016/s0008-8846(02)00836-0
Gawlicki, M., Nocuń-Wczelik, W., and Bąk, Ł. (2010). Calorimetry in the Studies of Cement Hydration. J. Therm. Anal. Calorim. 100 (2), 571–576. doi:10.1007/s10973-009-0158-5
Gehri, N., Mata-Falcón, J., and Kaufmann, W. (2020). Automated Crack Detection and Measurement Based on Digital Image Correlation. Construction Building Mater. 256, 119383. doi:10.1016/j.conbuildmat.2020.119383
Gupta, R., Kewalramani, M. A., and Goel, A. (2006). Prediction of concrete Strength Using Neural-Expert System. J. Mater. Civ. Eng. 18 (3), 462–466. doi:10.1061/(asce)0899-1561(2006)18:3(462)
Hackley, V. A. (2004). Particle Size Analysis by Laser Diffraction Spectrometry: Application to Cementitious Powders. US Department of Commerce, National Institute of Standards and Technology.
Hale, W. M., Bush, T. D., Russell, B. W., and Freyne, S. F. (2005). Effect of Curing Temperature on Hardened Concrete Properties. Transportation Res. Rec. 20051914, 97–104. doi:10.1177/0361198105191400112
Hamza, A. S. (2017). The Effect of Cement Type on Concrete Resistance to Sulphates. Leadership in Sustainable Infrastructure, 5691–5710.
Hughes, T. L., Methven, C. M., Jones, T. G. J., Pelham, S. E., Fletcher, P., and Hall, C. (1995). Determining Cement Composition by Fourier Transform Infrared Spectroscopy. Adv. Cement Based Mater. 2 (3), 91–104. doi:10.1016/1065-7355(94)00031-x
Ji, T., Lin, T., and Lin, X. (2006). A concrete Mix Proportion Design Algorithm Based on Artificial Neural Networks. Cement Concrete Res. 36 (7), 1399–1408. doi:10.1016/j.cemconres.2006.01.009
Ji-Zong, W., Hong-Guang, N., and Jin-Yun, H. (1999). The Application of Automatic Acquisition of Knowledge to Mix Design of concrete. Cement Concrete Res. 29 (12), 1875–1880. doi:10.1016/s0008-8846(99)00152-0
Jia, Y., Tang, L., Ming, P., and Xie, Y. (2019). Ultrasound-excited Thermography for Detecting Microcracks in concrete Materials. NDT E Int. 101, 62–71. doi:10.1016/j.ndteint.2018.10.006
John, S. T., Roy, B. K., Sarkar, P., and Davis, R. (2020). IoT Enabled Real-Time Monitoring System for Early-Age Compressive Strength of Concrete. J. Constr. Eng. Manage. 146 (2), 05019020. doi:10.1061/(asce)co.1943-7862.0001754
Kewalramani, M. A., and Gupta, R. (2006). Concrete Compressive Strength Prediction Using Ultrasonic Pulse Velocity through Artificial Neural Networks. Automation in Construction 15 (3), 374–379. doi:10.1016/j.autcon.2005.07.003
Kim, J.-H., and Park, M. (2018). Visualization of Concrete Slump Flow Using the Kinect Sensor. Sensors 18 (3), 771. doi:10.3390/s18030771
Kim, J.-I., Kim, D. K., Feng, M. Q., and Yazdani, F. (2004). Application of Neural Networks for Estimation of concrete Strength. J. Mater. Civ. Eng. 16 (3), 257–264. doi:10.1061/(asce)0899-1561(2004)16:3(257)
Kim, J., Luis, R., Smith, M. S., Figueroa, J. A., Malocha, D. C., and Nam, B. H. (2015). Concrete Temperature Monitoring Using Passive Wireless Surface Acoustic Wave Sensor System. Sensors Actuators A: Phys. 224, 131–139. doi:10.1016/j.sna.2015.01.028
Lediga, R., and Kruger, D. (2017). “Optimizing concrete Mix Design for Application in 3D Printing Technology for the Construction Industry,” in Solid State Phenomena (Switzerland: Trans Tech Publ). doi:10.4028/www.scientific.net/ssp.263.24
Lee, C. H., and Hover, K. C. (2015). Influence of Datum Temperature and Activation Energy on Maturity Strength Predictions. Aci Mater. J. 112 (6), 781–790. doi:10.14359/51687855
Lee, S.-C. (2003). Prediction of concrete Strength Using Artificial Neural Networks. Eng. Structures 25 (7), 849–857. doi:10.1016/s0141-0296(03)00004-x
Li, L., Wang, Q., Zhang, G., Shi, L., Dong, J., and Jia, P. (2018). A Method of Detecting the Cracks of concrete Undergo High-Temperature. Construction Building Mater. 162, 345–358. doi:10.1016/j.conbuildmat.2017.12.010
Li, W., Dong, B., Yang, Z., Xu, J., Chen, Q., Li, H., et al. (2018). Recent Advances in Intrinsic Self-Healing Cementitious Materials. Adv. Mater. 30 (17), e1705679. doi:10.1002/adma.201705679
Liu, C., Zhang, F. Q., and Zhang, H. (2020). Comparative Analysis of Off-Site Precast Concrete and Cast-In-Place Concrete in Low-Carbon Built Environment. Fresenius Environ. Bull. 29 (3), 1804–1812.
Liu, Y., Deng, F., He, Y., Li, B., Liang, Z., and Zhou, S. (2017). Novel Concrete Temperature Monitoring Method Based on an Embedded Passive RFID Sensor Tag. Sensors 17 (7), 1463. doi:10.3390/s17071463
Ma, Q., Guo, R., Zhao, Z., Lin, Z., and He, K. (2015). Mechanical Properties of concrete at High Temperature-A Review. Construction Building Mater. 93, 371–383. doi:10.1016/j.conbuildmat.2015.05.131
McCullough, B. F., and Rasmussen, R. O. (1999). Fast-track Paving: concrete Temperature Control and Traffic Opening Criteria for Bonded concrete Overlays, Volume I. Washington, DC: United States. Federal Highway Administration. Office of Infrastructure.
Mehdipour, I., Zoughi, R., and Khayat, K. H. (2018). Feasibility of Using Near-Field Microwave Reflectometry for Monitoring Autogenous Crack Healing in Cementitious Materials. Cement and Concrete Composites 85, 161–173. doi:10.1016/j.cemconcomp.2017.10.014
Monteagudo, S. M., Moragues, A., Gálvez, J. C., Casati, M. J., and Reyes, E. (2014). The Degree of Hydration Assessment of Blended Cement Pastes by Differential thermal and Thermogravimetric Analysis. Morphological Evolution of the Solid Phases. Thermochim. Acta 592, 37–51. doi:10.1016/j.tca.2014.08.008
Mostafa, N. Y., and Brown, P. W. (2005). Heat of Hydration of High Reactive Pozzolans in Blended Cements: Isothermal Conduction Calorimetry. Thermochim. Acta 435 (2), 162–167. doi:10.1016/j.tca.2005.05.014
Mubarak, K., Bois, K. J., and Zoughi, R. (2001). A Simple, Robust, and On-Site Microwave Technique for Determining Water-To-Cement Ratio (W/c) of Fresh Portland Cement-Based Materials. IEEE Trans. Instrum. Meas. 50 (5), 1255–1263. doi:10.1109/19.963194
Naderpour, H., Rafiean, A. H., and Fakharian, P. (2018). Compressive Strength Prediction of Environmentally Friendly concrete Using Artificial Neural Networks. J. Building Eng. 16, 213–219. doi:10.1016/j.jobe.2018.01.007
Nagy, R. (2014). Image Digitalization as a Tool for Processing Experimental Data of Crack Width of concrete. Epitoanyag-Journal Silicate Based Compos. Mater. 66 (4). doi:10.14382/epitoanyag-jsbcm.2014.22
Naseri, H. (2020). Developing a Novel Machine Learning Method to Predict the Compressive Strength of Fly Ash concrete in Different Ages. AUT J. Civil Eng. 4 (4), 3.
Neshat, M. (2012). Predication of concrete Mix Design Using Adaptive Neural Fuzzy Inference Systems and Fuzzy Inference Systems. Int. J. Adv. Manufacturing Tech. 63 (1-4), 373–390. doi:10.1007/s00170-012-3914-9
Neville, A. M., and Brooks, J. J. (1987). Concrete Technology. Longman Scientific & Technical England.
Nguyen, H., Vu, T., Vo, T. P., and Thai, H.-T. (2021). Efficient Machine Learning Models for Prediction of concrete Strengths. Construction Building Mater. 266, 120950. doi:10.1016/j.conbuildmat.2020.120950
Norris, A., Saafi, M., and Romine, P. (2008). Temperature and Moisture Monitoring in concrete Structures Using Embedded Nanotechnology/microelectromechanical Systems (MEMS) Sensors. Construction Building Mater. 22 (2), 111–120. doi:10.1016/j.conbuildmat.2006.05.047
Olek, J., Cohen, M. D., and Lobo, C. (1990). Determination of Surface-Area of Portland-Cement and Silica Fume by Mercury Intrusion Porosimetry. Aci Mater. J. 87 (5), 473–478.
Omondi, B., Aggelis, D. G., Sol, H., and Sitters, C. (2016). Improved Crack Monitoring in Structural concrete by Combined Acoustic Emission and Digital Image Correlation Techniques. Struct. Health Monit. 15 (3), 359–378. doi:10.1177/1475921716636806
Ouchi, M., Self-compacting concrete-development, Applications and Investigations. NORDIC CONCRETE RESEARCH-PUBLICATIONS-, 2000. 23: p. 29–34.
Oztas, A. (2006). Predicting the Compressive Strength and Slump of High Strength concrete Using Neural Network. Construction Building Mater. 20 (9), 769–775.
Papadonikolaki, E., Krystallis, I., and Morgan, B. (2020). Digital Transformation in Construction-Systematic Literature Review of Evolving Concepts. Virtual Conference: Engineering Project Organization Society.
Parusheva, S. (2019). Digitalization and Digital Transformation in Construction-Benefits and Challenges. Information and Communication Technologies in Business and Education, 126–134.
Phang, T. C. H., Chen, C., and Tiong, R. L. K. (2020). New Model for Identifying Critical Success Factors Influencing BIM Adoption from Precast Concrete Manufacturers' View. J. Constr. Eng. Manage. 146 (4), 04020014. doi:10.1061/(asce)co.1943-7862.0001773
Polat, R. (2013). The Correlation between Aggregate Shape and Compressive Strength of concrete: Digital Image Processing Approach. Int. J. Struct. Civ. Eng. Res. 2, 63–80.
Prasad, B. K. R., Eskandari, H., and Reddy, B. V. V. (2009). Prediction of Compressive Strength of SCC and HPC with High Volume Fly Ash Using ANN. Construction Building Mater. 23 (1), 117–128. doi:10.1016/j.conbuildmat.2008.01.014
Providakis, C., Liarakos, E., and WiEYE, T. (2011). T-WiEYE: An Early-Age concrete Strength Development Monitoring and Miniaturized Wireless Impedance Sensing System. Proced. Eng. 10 (Icm11), 484–489. doi:10.1016/j.proeng.2011.04.082
Rao, C., and Tutumluer, E. (2000). Determination of Volume of Aggregates - New Image-Analysis Approach. Geomaterials 1721 (1721), 73–80. doi:10.3141/1721-09
Rasmussen, N. V., and Beliatis, M. J. (2019). IoT Based Digitalization and Servitization of Construction Equipment in concrete industryGlobal IoT Summit (GIoTS). IEEE.
Rezaiee-Pajand, M., Karimipour, A., and Abad, J. M. N. (2021). Crack Spacing Prediction of Fibre-Reinforced concrete Beams with Lap-Spliced Bars by Machine Learning Models. Iran J. Sci. Technol. Trans. Civ Eng. 45 (2), 833–850. doi:10.1007/s40996-020-00441-6
Rizzo, P., Ni, X., Nassiri, S., and Vandenbossche, J. (2014). A Solitary Wave-Based Sensor to Monitor the Setting of Fresh concrete. Sensors 14 (7), 12568–12584. doi:10.3390/s140712568
Ruiz, J. (2001). “Concrete Temperature Modeling and Strength Prediction Using Maturity Concepts in the FHWA HIPERPAV Software,” in Seventh International Conference on Concrete Pavements. The Use of Concrete in Developing Long-Lasting Pavement Solutions for the 21st (Orlando, FL: CenturyInternational Society for Concrete Pavements).
Shah, S. G., and Chandra Kishen, J. M. (2011). Fracture Properties of Concrete-Concrete Interfaces Using Digital Image Correlation. Exp. Mech. 51 (3), 303–313. doi:10.1007/s11340-010-9358-y
Shoukry, S. N., William, G. W., Downie, B., and Riad, M. Y. (2011). Effect of Moisture and Temperature on the Mechanical Properties of concrete. Construction Building Mater. 25 (2), 688–696. doi:10.1016/j.conbuildmat.2010.07.020
Simonsson, P., and Emborg, M. (2009). Industrialized Construction: Benefits Using SCC in Cast In-Situ Construction. Nordic Concrete Res. 39 (1), 33–52.
Song, H., Ahmad, A., Farooq, F., Ostrowski, K. A., Maślak, M., Czarnecki, S., et al. (2021). Predicting the Compressive Strength of concrete with Fly Ash Admixture Using Machine Learning Algorithms. Construction Building Mater. 308, 125021. doi:10.1016/j.conbuildmat.2021.125021
Suryani, S., Heryanto, H., Rusdaeni, R., Fahri, A. N., and Tahir, D. (2020). Quantitative Analysis of Diffraction and Infra-red Spectra of Composite cement/BaSO4/Fe3O4 for Determining Correlation between Attenuation Coefficient, Structural and Optical Properties. Ceramics Int. 46 (11), 18601–18607. doi:10.1016/j.ceramint.2020.04.170
Tang, S. W., Cai, X. H., He, Z., Shao, H. Y., Li, Z. J., and Chen, E. (2016). Hydration Process of Fly Ash Blended Cement Pastes by Impedance Measurement. Construction Building Mater. 113, 939–950. doi:10.1016/j.conbuildmat.2016.03.141
Tareen, N., Kim, J., Kim, W-K., and Park, S. (2019). Comparative Analysis and Strength Estimation of Fresh Concrete Based on Ultrasonic Wave Propagation and Maturity Using Smart Temperature and PZT Sensors. Micromachines 10 (9), 559. doi:10.3390/mi10090559
Tesfamariam, S., and Najjaran, H. (2007). Adaptive Network-Fuzzy Inferencing to Estimate Concrete Strength Using Mix Design. J. Mater. Civ. Eng. 19 (7), 550–560. doi:10.1061/(asce)0899-1561(2007)19:7(550)
Thomas, J. J., Jennings, H. M., and Allen, A. J. (1998). The Surface Area of Cement Paste as Measured by Neutron Scattering: Evidence for Two C-S-H Morphologies. Cement Concrete Res. 28 (6), 897–905. doi:10.1016/s0008-8846(98)00049-0
Thomas, J. J., Jennings, H. M., and Allen, A. J. (1999). The Surface Area of Hardened Cement Paste as Measured by Various Techniques. Concrete Sci. Eng. 1 (1), 45–64.
Tomek, R. (2017). Advantages of Precast concrete in Highway Infrastructure Construction. Proced. EngineeringCcc 196, 176–180. doi:10.1016/j.proeng.2017.07.188
Topçu, İ. B., and Toprak, M. U. (2005). Fine Aggregate and Curing Temperature Effect on concrete Maturity. Cement Concrete Res. 35 (4), 758–762. doi:10.1016/j.cemconres.2004.04.023
Tran, Q. H., Han, D., Kang, C., Haldar, A., and Huh, J. (2017). Effects of Ambient Temperature and Relative Humidity on Subsurface Defect Detection in Concrete Structures by Active Thermal Imaging. Sensors 17 (8), 1718. doi:10.3390/s17081718
Van Damme, H. (2020). “Challenges and Opportunities for concrete in the Digital Era,” in Gulf Conference on Sustainable Built Environment (Cham: Springer). doi:10.1007/978-3-030-39734-0_3
Wallevik, O. H. (2003). “Rheology—a Scientific Approach to Develop Self-Compacting concrete,” in Proceedings of the 3rd International RILEM Symposium on Self-Compacting Concrete (Iceland; Reykjavik).
Walther, T. (2018). Digital Transformation of the Global Cement Industry. IEEE. IEEE-IAS/PCA Cement Industry Conference (IAS/PCA).
Wangler, T., Lloret, E., Reiter, L., Hack, N., Gramazio, F., Kohler, M., et al. (2016). Digital concrete: Opportunities and Challenges. RILEM Tech. Lett. 1, 67–75. doi:10.21809/rilemtechlett.2016.16
Wangler, T., Roussel, N., Bos, F. P., Salet, T. A. M., and Flatt, R. J. (2019). Digital Concrete: A Review. Cement Concrete Res. 123, 105780. doi:10.1016/j.cemconres.2019.105780
Waris, M. I., Mir, J., Plevris, V., and Ahmad, A. (2020). “Predicting Compressive Strength of CRM Samples Using Image Processing and ANN,” in IOP Conference Series: Materials Science and Engineering (IOP Publishing) Vol. 899, 012014. doi:10.1088/1757-899x/899/1/012014
Winslow, D. N., and Diamond, S. (1974). Specific Surface of Hardened Portland Cement Paste as Determined by Small-Angle X-Ray Scattering. J. Am. Ceram. Soc. 57 (5), 193–197. doi:10.1111/j.1151-2916.1974.tb10856.x
Wong, A. C. L., Childs, P. A., Berndt, R., Macken, T., Peng, G.-D., and Gowripalan, N. (2007). Simultaneous Measurement of Shrinkage and Temperature of Reactive Powder concrete at Early-Age Using Fibre Bragg Grating Sensors. Cement and Concrete Composites 29 (6), 490–497. doi:10.1016/j.cemconcomp.2007.02.003
Xu, Q., Hu, J., Ruiz, J. M., Wang, K., and Ge, Z. (2010). Isothermal Calorimetry Tests and Modeling of Cement Hydration Parameters. Thermochim. Acta 499 (1-2), 91–99. doi:10.1016/j.tca.2009.11.007
Xu, Q. W. (2011). Modeling Hydration Properties and Temperature Developments of Early-Age concrete Pavement Using Calorimetry Tests. Thermochim. Acta 512 (1-2), 76–85. doi:10.1016/j.tca.2010.09.003
Yang, Y., Divsholi, B. S., and Soh, C. K. (2010). A Reusable PZT Transducer for Monitoring Initial Hydration and Structural Health of concrete. Sensors 10 (5), 5193–5208. doi:10.3390/s100505193
Yaseen, Z. M., Deo, R. C., Hilal, A., Abd, A. M., Bueno, L. C., Salcedo-Sanz, S., et al. (2018). Predicting Compressive Strength of Lightweight Foamed concrete Using Extreme Learning Machine Model. Adv. Eng. Softw. 115, 112–125. doi:10.1016/j.advengsoft.2017.09.004
Yeh, I.-C. (2006). Analysis of Strength of concrete Using Design of Experiments and Neural Networks. J. Mater. Civ. Eng. 18 (4), 597–604. doi:10.1061/(asce)0899-1561(2006)18:4(597)
Yeh, I.-C. (1999). Design of High-Performance concrete Mixture Using Neural Networks and Nonlinear Programming. J. Comput. Civil Eng. 13 (1), 36–42. doi:10.1061/(asce)0887-3801(1999)13:1(36)
Yeh, I.-C. (2006). Exploring concrete Slump Model Using Artificial Neural Networks. J. Comput. Civ. Eng. 20 (3), 217–221. doi:10.1061/(asce)0887-3801(2006)20:3(217)
Yeh, I.-C. (2009). Simulation of concrete Slump Using Neural Networks. Proc. Inst. Civil Eng. - Construction Mater. 162 (1), 11–18. doi:10.1680/coma.2009.162.1.11
Yikici, T. A., and Chen, H.-L. (2015). Use of Maturity Method to Estimate Compressive Strength of Mass concrete. Construction Building Mater. 95, 802–812. doi:10.1016/j.conbuildmat.2015.07.026
Zavrtanik, N., Prosen, J., Tušar, M., and Turk, G. (2016). The Use of Artificial Neural Networks for Modeling Air Void Content in Aggregate Mixture. Automation in Construction 63, 155–161. doi:10.1016/j.autcon.2015.12.009
Zeltser, R. Y., au, fnm., Bielienkova, O. Y., Novak, Y. V., and Dubinin, D. V. (2019). Digital Transformation of Resource Logistics and Organizational and Structural Support of Construction. Sci. Innov. 15 (5), 34–46. doi:10.15407/scine15.05.034
Zhang, J., Cusson, D., Monteiro, P., and Harvey, J. (2008). New Perspectives on Maturity Method and Approach for High Performance concrete Applications. Cement Concrete Res. 38 (12), 1438–1446. doi:10.1016/j.cemconres.2008.08.001
Ziolkowski, P., and Niedostatkiewicz, M. (2019). Machine Learning Techniques in Concrete Mix Design. Materials 12 (8), 1256. doi:10.3390/ma12081256
Keywords: digital transformation, concrete properties, concrete technology, sustainability, advanced technology, monitoring
Citation: Gamil Y and Cwirzen A (2022) Digital Transformation of Concrete Technology—A Review. Front. Built Environ. 8:835236. doi: 10.3389/fbuil.2022.835236
Received: 14 December 2021; Accepted: 24 January 2022;
Published: 11 March 2022.
Edited by:
Maria Rashidi, Western Sydney University, AustraliaReviewed by:
Mahdi Kioumarsi, Oslo Metropolitan University, NorwayPeng Zhang, National Natural Science Foundation of China, China
Copyright © 2022 Gamil and Cwirzen. This is an open-access article distributed under the terms of the Creative Commons Attribution License (CC BY). The use, distribution or reproduction in other forums is permitted, provided the original author(s) and the copyright owner(s) are credited and that the original publication in this journal is cited, in accordance with accepted academic practice. No use, distribution or reproduction is permitted which does not comply with these terms.
*Correspondence: Yaser Gamil, eWFzZXIuZ2FtaWxAbHR1LnNl