- 1Department of Parks, Recreation and Tourism Management, North Carolina State University, Raleigh, NC, United States
- 2School of Geography and the Environment, Oxford Martin School, University of Oxford, Oxford, United Kingdom
- 3System Dynamics Group, Department of Geography, University of Bergen, Bergen, Norway
Conservation practitioners routinely work within complex social-ecological systems to address threats facing biodiversity and to promote positive human-wildlife interactions. Inadequate understanding of the direct and indirect, short- and long-term consequences of decision making within these dynamic systems can lead to misdiagnosed problems and interventions with perverse outcomes, exacerbating conflict. Participatory system dynamics (SD) modeling is a process that encourages stakeholder engagement, synthesizes research and knowledge, increases trust and consensus and improves transdisciplinary collaboration to solve these complex types of problems. Tiger conservation exemplifies a set of interventions in a complex social-ecological system. Wild tigers remain severely threatened by various factors, including habitat constraints, human-wildlife conflict, and persistent consumer demand for their body parts. Opinions differ on whether commercial captive tiger facilities reduce or increase the threat from poaching for trade, resulting in policy conflict among diverse stakeholder groups. This paper explains how we are working with international conservation partners in a virtual environment to utilize a participatory SD modeling approach with the goal of better understanding and promoting coexistence of humans and wild tigers. We highlight a step-by-step process that others might use to apply participatory SD modeling to address similar conservation challenges, building trust and consensus among diverse partners to reduce conflict and improve the efficacy of conservation interventions.
Introduction
To navigate complex social-ecological systems and promote coexistence with wildlife, researchers and practitioners must focus on knowledge generation while increasing access to and use of information that already exists. Inadequate understanding of systems can lead to misdiagnosed problems and unintended outcomes (Larrosa et al., 2016). These misdiagnoses often create or exacerbate human-wildlife conflict (Hübschle, 2017). Conflict mitigation interventions typically focus on tangible disputes (e.g., livestock depredation and retaliatory killings, illegal poaching) without addressing root causes of problems such as inequitable social relationships and processes (Madden and McQuinn, 2014; Baynham-Herd et al., 2018). While a wealth of information about these social relationships and processes exists, this knowledge often remains on the periphery of decision-making that impacts wildlife management (Bennett et al., 2017). Interventions that neglect to consider social and political context, such as a singular “war on poachers” are therefore unlikely to succeed. In fact, such interventions may inadvertently fuel social-cultural tensions and subsequent conservation-related conflict (Brashares et al., 2014; Challender and MacMillan, 2014). Efforts to reduce human-wildlife conflict, especially for controversial carnivore species like tigers, rarely address these issues (Krafte Holland et al., 2018).
Madden and McQuinn (2014) argue that “conservation efforts would benefit from improved capacity and resources for understanding and transforming the complex drivers of deep-rooted social conflicts impacting wildlife conservation and management actions” (p. 104). Numerous scholars have made a strong case that biodiversity conservation is ultimately a social and political process (Brechin et al., 2002; Lele et al., 2010; Montgomery et al., 2020). Yet, despite a growing body of research focused on the social component of social-ecological systems (Ban et al., 2013), including systems with carnivore species such as tigers (Torri, 2011; Struebig et al., 2018), efforts to apply this research by re-conceptualizing and adapting conventional conservation approaches have been slow (Bennett et al., 2017). As recognition of these challenges grows, the key ingredients for change are already present. Leveraging them might simply require a shift toward systems-level thinking and adaptation.
Toward A Systems Approach
Conservation practitioners often acknowledge the complexity of the systems in which they operate, which span a wide array of habitats, stakeholder groups, communities, sectors, and political boundaries. Most adapt and respond to these systems, altering interventions based on data and experience to better achieve their goals. Yet dynamic social-ecological systems make it very difficult to grasp the long-term implications of management actions due to delays between cause and effect (Kim and Senge, 1994). Implementation itself may also change the nature of the problem, influencing the success of the solution (Game et al., 2014; Larrosa et al., 2016). New tools and approaches are needed to advance understanding of systems and build capacity for action (Mahajan et al., 2019).
To advance understanding of systems, researchers have employed modeling approaches such as bayesian belief networks (Bennett et al., 2021), agent-based modeling, social network analysis, and system dynamics (Frerichs et al., 2016). There are also approaches that focus more on planning to help managers improve decision making and outcomes, such as structured decision making (Gregory et al., 2012) and the Conservation Standards (CMP, 2020). For example, use of the Conservation Standards provides a number of benefits, including identifying potential interventions, clarifying theories of change and increasing collaboration; however, it does not explicitly incorporate system behavior such as feedbacks, non-linear behavior or the consequences of time delays. With such a diversity of tools that could be used for understanding complex systems (Voinov et al., 2018), it can be difficult for managers to know where to start.
The field of System Dynamics (SD) began in the early 1960s to better understand complex human and industrial dynamics (Forrester, 1971). Today, SD is used to inform decision making and policy in fields such as business (Ford, 1997; Sterman, 2000), health (Frerichs et al., 2016; Currie et al., 2018), social work (Trani et al., 2016; Appel et al., 2019; Fowler et al., 2019), and agriculture and natural resource management (Ford, 1999; Stave, 2010; Turner et al., 2016; Kopainsky et al., 2017). It has even been applied to species such as sage grouse (Beall and Zeoli, 2008), African penguins (Weller et al., 2014) and grizzly and spectacled bears (Faust et al., 2004).
While not suitable in all cases, SD offers a number of strengths in helping to understand the dynamic behavior of complex systems and test assumptions of different actions and policies with a focus on solving problems (Forrester, 1994; Sterman, 2000). SD traditionally uses two main modeling types: qualitative causal loop diagrams (CLDs) and quantitative simulation models. CLD's identify relationships and feedback mechanisms between elements. Simulation models incorporate the elements of a CLD into a stock-flow structure, where stocks represent what is accumulating in a system (e.g., number of tigers) and flows represent rates of change (e.g., birth or death rate). Structural (e.g. connections between elements) as well as numerical data are incorporated into simulation models to generate endogenous behavior over time under changing conditions and policy interventions. While not meant for predicting or forecasting, simulation models make it easier to explore the potential implications of changing conditions and selected policy interventions on system behavior (Sterman, 2000). SD simulation models run quickly and do not require high computing power; the approach is also particularly useful in environments where quantitative data is scarce and integration of qualitative data (e.g. expert opinion) could be used to address knowledge gaps (Luna-Reyes and Andersen, 2003; Gallagher et al., 2020).
SD is ideally used in participatory planning processes where it can support the negotiation of a shared understanding of a dynamic problem (Vennix, 1999). It facilitates exchange of ideas among participants (Sedlacko et al., 2014) and effectively integrates existing scientific research with local knowledge (Beall and Zeoli, 2008; Stave, 2010; Rouwette et al., 2016). Co-creating SD models necessitates turning implicit into explicit knowledge, so that participants are learning from each other and the model itself (Kopainsky et al., 2017). This also encourages participant ownership of the model and greater support of outputs to address the problem. Model creation can provide a laboratory for a group to examine policies and to visualize potential impacts of actions over time (Forrester, 1994; Sterman, 2000). This is an especially important benefit when working with endangered species or sensitive environments, where physical experiments are not always possible (Sterman et al., 2013; Turner, 2020). In addition to insights from the model, the model building process can increase the social capital of a group (Davies et al., 2015), strengthen relationships and improve communication (Beall and Zeoli, 2008; Stave, 2010). Although there are several terms used for conducting SD modeling with stakeholders, we use the term participatory SD modeling in this paper.
Despite calls to increase the overall use of models in decision making, resistance may persist for several reasons. Primary concerns include lack of transparency regarding model-building and outputs and weak communication between modelers and practitioners (Addison et al., 2013). Participatory SD modeling offers several advantages since models are designed to be built with stakeholders, using the language of people working on the chosen problem. The visual nature of the modeling software is more accessible to a lay audience, and easy-to-use interfaces help minimize technical barriers between modelers and the modeling groups (Sterman, 1994). Although the value of participatory SD is well-documented (Rouwette et al., 2011; Scott et al., 2016b; Andersen et al., 2017), adoption of this approach to address complex conservation problems has been slow. The time required of participants in the short term (Stave et al., 2019) and the need for a competent modeler and facilitator to coordinate the process (Andersen et al., 1997) are major barriers to adoption. More research and guidance are needed to help conservation practitioners explore the potential value of the participatory SD modeling approach.
This paper explores how a participatory SD modeling process can be used to address a particularly complex problem: conservation of wild tigers.
Conservation Context: Impacts of Tiger Farming On Wild Tiger Populations
Approximately 3,900 tigers remain in the wild worldwide (World Wildlife Fund, 2021), and they are found in <7% of their original global range (Dinerstein et al., 2007). Wild tiger populations are found in up to 13 countries: Bangladesh, Bhutan, Cambodia, China, India, Indonesia, Lao PDR, Malaysia, Myanmar, Nepal, Russia, and Thailand (Goodrich et al., 2015), although this list includes several countries where wild tiger may be functionally extint (EIA, 2017; Rasphone et al., 2019). The continued survival of tigers depends on a complex set of ecological, economic, and social factors across local and global scales. Because tigers need sufficient lands to roam where they can find adequate prey and live largely undisturbed by people, some experts believe that conservation efforts should focus on law enforcement and protection of habitat and corridors in and around key protected areas (Walston et al., 2010). In areas where tiger conservation succeeds and numbers grow, tigers increasingly come into conflict with growing rural human populations, threatening people and their livestock and potentially increasing revenge killings (Carter et al., 2014; Struebig et al., 2018).
In addition to the interrelated processes of human encroachment, habitat and prey loss, and human-tiger conflict, a persistent consumer market for tiger parts and products economically incentivizes poaching and makes the conservation of wild tigers even more challenging (Wong, 2016). In fact, there is growing consensus that the most urgent threat to wild tigers is poaching (Dinerstein et al., 2007; Chapron et al., 2008). International consumer demand for tiger parts (bones, hides, teeth, etc.) constitutes a major potential threat to wild tigers (Goodrich et al., 2015). Tiger parts are valued across Asia for their perceived health benefits and may confer status and wealth (Goodrich et al., 2015; EIA, 2017). As the species becomes rarer, illegal harvesting and trade in body parts are likely to increase alongside rising market values.
Reduction of the threat of poaching is difficult because tiger poaching crosses multiple countries with different cultures, laws, and policies, and it is influenced by complicated market behaviors (e.g., consumer demand for tiger parts) amidst a growing human population (Sharma et al., 2014). A feedback loop of inter-related increasing scarcity and rising prices can lead to a phenomenon termed the anthropogenic allee effect (Courchamp et al., 2006), which can drive a species to extinction or keep a population low. Under these conditions, drawing attention to the rarity of the species through a demand-reduction program can have the perverse effect of stimulating poaching (Courchamp et al., 2006; Hall et al., 2008). Poaching also appears to have a non-linear relationship with tiger survival, indicating that there are thresholds where even steady rates of poaching could suddenly cause an extinction risk to a tiger population (Kenney et al., 1995). Adding complexity, the tiger trade also potentially threatens other big cats around the world, as body parts from other species such as lions are being traded in the tiger parts market (Williams, 2015; Williams et al., 2017; Villalva and Moracho, 2019; Coals et al., 2020). To mitigate poaching, some have suggested that market demand for tiger products should be supplied from captive sources (Jiang et al., 2007), but this proposal is contested (Gratwicke et al., 2008).
As of 2017, at least 7,000 tigers were estimated to be held in captive facilities (hereafter “tiger farms”) across Asia, catering to growing demands for various products ranging from tiger body parts and derivatives to live tigers used for tourist attractions (EIA, 2017). The global captive tiger population is larger, with ~5,000 captive tigers in the United States alone (World Wildlife Fund, 2020). Many conservation organizations would like to see this practice end, but the potential impacts of closures of farming operations for species are not entirely clear or without risk (Kirkpatrick and Emerton, 2010; 't Sas-Rolfes, 2010). For the purpose of this study, we define a tiger farm as “a facility that keeps or breeds tigers in captivity with an intent (or reasonable probability) of supplying or directly engaging in the commercial trade in tigers or tiger products, be they body parts or derivatives. The application of this definition is not limited by the stated purpose of such facilities.”
As Asian economies grow, so might consumer demand for wildlife products such as skins and bones of tigers (Linkie et al., 2018). In one case, researchers found that nearly half (43%) of survey respondents in China (one of the largest consumer markets) had consumed a product that contained tiger parts (Gratwicke et al., 2008). There is uncertainty over the preference consumers may have for wild vs. farmed tiger products (Coals et al., 2020; Hinsley and 't Sas-Rolfes, 2020), with wild tigers possibly being prized more for their power and strength (EIA, 2017). Stronger preferences for wild vs. farmed animal parts have been reported for other species, such as bears farmed for bear bile (Dutton et al., 2011), but these preferences are dynamic and can shift based on access and availabilty (Davis et al., 2021; Rizzolo, 2021). This uncertainty raises questions about the relationship between tiger farms and demand for tiger parts and products (Song and Yao, 2021).
The challenge of enforcing global wildlife trade under the Convention on International Trade in Endangered Species (CITES) (Challender et al., 2015), combined with limited capacity to combat poachers, has led to some researchers to support limited tiger farming (Abbott and van Kooten, 2011). The argument for legalized tiger farming proposes that increasing the supply of parts will suppress the market price of illegally harvested tiger products (Abbott and van Kooten, 2011). While the demand for tiger parts would persist, diminishing financial incentives for illegally harvesting wild tigers could deter poaching. Based on this hypothesis, some scientists have advocated for humane, renewable harvest and legal trade of other endangered wildlife species facing similar predicaments—such as African rhinos (Biggs et al., 2013).
Conversely, many argue that farming tigers and facilitating the use of their parts for a consumer market fuels market demand and complicates enforcement efforts to reduce wild tiger poaching (Gratwicke et al., 2008; EIA, 2017). According to this argument, the presumed benefits of legal supply might be undermined by imperfections in the tiger parts market, including dominance of a small number of producers controlling prices, the luxury status of tiger parts, and the relatively high expense of farming tigers (Kirkpatrick and Emerton, 2010). Legal markets for farmed tiger products might also lead to greater social acceptability of the product, thereby suppressing a stigma effect considered necessary to prevent unsustainable demand levels (Fischer, 2004; Rizzolo, 2021).
Considering the growth in tiger farms and potential demand for tiger parts globally, many scientists and conservation managers are seeking to better understand the impacts of tiger farming on wild tiger populations. The complex dynamics surrounding tiger farms highlight the need for holistic, systems-based approaches to understand their full impact on wild tiger conservation (Rizzolo, 2021). Understanding complex systems such as those impacting tiger conservation efforts is exceptionally difficult (de Vos et al., 2019), but remains a global priority in conservation science. Participatory SD modeling offers a unique opportunity to understand the problems related to tiger farms and to evaluate the efficacy of proposed interventions.
Below, we describe the development and implementation of a participatory SD modeling process designed to explore the impact of tiger farming on wild tiger populations.
Applying The Participatory System Dynamics Modeling Process
There is a tendency when applying SD modeling to focus on what is perceived to be the final product: the model itself. Although the model can be an important decision-making tool, it is often not the most valuable outcome. The process itself is what creates an opportunity for conflict transformation (Madden and McQuinn, 2014). In the following sections, we discuss the primary steps in a participatory SD process and describe how we are currently applying them to improve understanding of the impact of tiger farming. Because our model building efforts are ongoing, the outcomes are not yet known and some aspects of our participatory approach continue to evolve. However, we have already learned multiple lessons that could help to inform participatory SD modeling in other contexts. Using tiger farming as a case study, the framework outlined in this paper illustrates how similar participatory SD approaches might be designed and implemented to build knowledge, trust and consensus among conservation partners with the goal of improving future conservation interventions.
Like any complex system, this process is not linear. At each step new information is learned and the identified problem may change, along with system components. Figure 1 depicts our participatory SD process in action (adapted from Beall and Ford, 2010). Key steps in Figure 1 are described in more detail below. Our process draws from many earlier examples of participatory modeling (Vennix et al., 1990; Sterman, 2000; Beall and Zeoli, 2008; Beall and Ford, 2010; Stave, 2010; Hovmand et al., 2012; Homer, 2019; Wilkerson et al., 2020), synthesizing and adapting these based on participant feedback and study context.
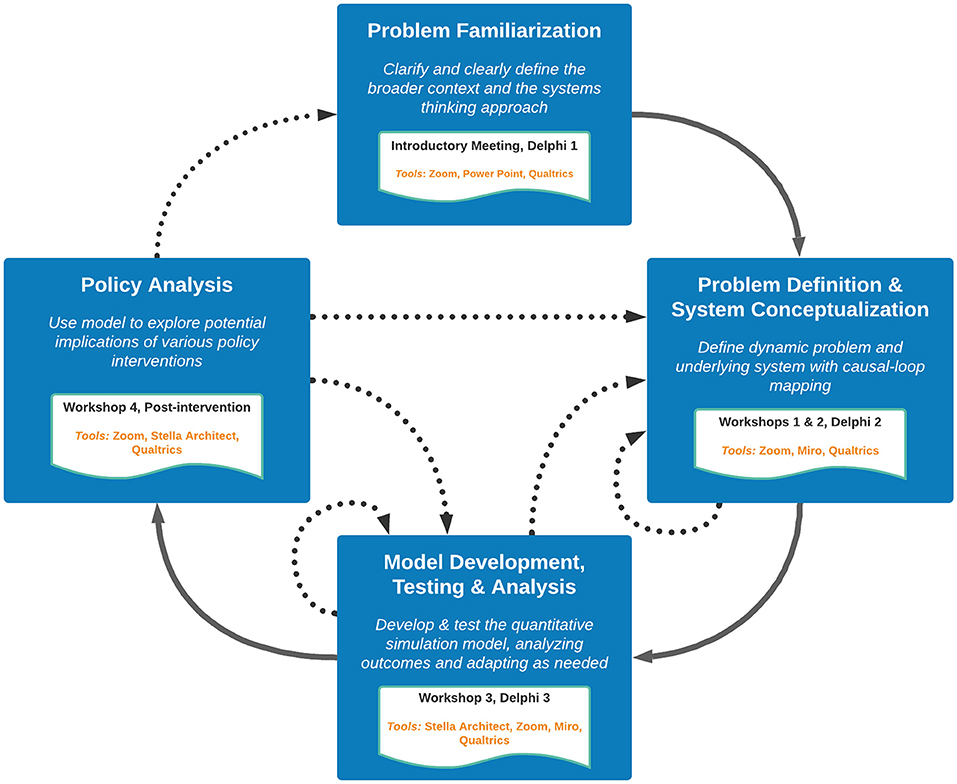
Figure 1. Diagram of the participatory system dynamics modeling process, highlighting key steps in the cyclical process and the activities and virtual tools that might be utilized at each stage. Adapted from Beall and Ford (2010). Solid lines depict direct paths as the stages progresses; dotted lines depict feedback loops and evolving conceptualizations that are a key component of the modeling process. The four workshop structure depicted here can be adapted based on groups' needs, availability, and problem complexity.
Setup and Design
The first step in a participatory SD project involves ensuring the right people are involved and that the process is tailored to match the scope of the problem. To develop a robust understanding of a complex problem, participants should bring diverse perspectives, knowledge, and expertise. This includes people who may not agree about a problem, its causes, or potential solutions. It is also important consider who is making policy and management decisions and involve these key actors in the process, if possible. This helps generate a model that is comprehensive, valuable to the individuals participating, and supported by leaders. Who participates also depends on the scope of the project (i.e., relevant geographic area, number of organizations or communities involved) and whether the model building will be done in person or virtually. There are benefits to convening in-person, however virtual platforms (e.g., Zoom) can engage more voices across a wider geographic area at a lower cost (Wilkerson et al., 2020). For either setting, group (or sub-group) size should be structured to make sure everyone can participate fully. Other factors to consider when designing the process include the experience level of facilitators and modelers, funding, and time available for both participants and facilitators/modelers.
Our tiger project was initiated by one organization (an international conservation NGO) starting in 2019, but the desire for diverse perspectives led to the creation of a four-person advisory group, each from varying backgrounds, perspectives, and organizations. This advisory group co-created the process with the research team, then selected and invited the rest of the participants. Throughout 2020, we devoted significant time to building understanding of the project within the advisory group and building trust among group members. Extensive conversations helped us reach consensus about which participants to invite, ensuring diverse perspectives regarding the costs and benefits of tiger farms while maintaining manageable group size for coordination purposes. To date, our participants include over 50 people spread across conservation NGOs (32 people from 20 different organizations), governmental or intergovernmental institutions (four people), research organizations or institutions (20 people), consultants (five people), and law enforcement (four people) (with participants able to identify multiple sectors). Participants live across Europe, Asia, North America and Africa. Expertise varies among participants, with self-reported knowledge being highest in wildlife trade (76% participants), law-enforcement and anti-poaching (40%), tiger farming in Asia (38%), and farming of non-tiger species (30%). Less than 20% of participants reported high confidence in systems thinking or participatory modeling, demonstrating that this process was relatively novel for most of these conservation practitioners.
Given the global network of experts involved, the costs and logistics made in-person meetings prohibitive (with challenges accentuated by the COVID-19 pandemic); thus, we made an early choice to adopt a completely virtual process. To operate in a virtual environment, the research team needed to learn new tools to be utilized at different stages (Figure 1). Recognizing not everyone could (or would) be interested in participating directly in the participatory modeling itself, we created two main groups: a modeling group (including the advisory group) and a consultation group (Table 1). To incorporate information from such a large group into the model and to support consensus building among the entire group in a virtual format, we decided to integrate a modified Delphi process into participatory SD modeling. The Delphi technique has been widely used for consensus building about topics ranging from program planning to policy development (Hsu and Sandford, 2007). It utilizes rounds of anonymous questionnaires to explore assumptions, illuminate diverse views, develop a range of possible alternatives, and to educate respondents about complex aspects of a topic. After each round, respondents review summarized responses and highlight areas of disagreement (Hsu and Sandford, 2007), as well as additional questions informed by the modeling (Vennix et al., 1990).
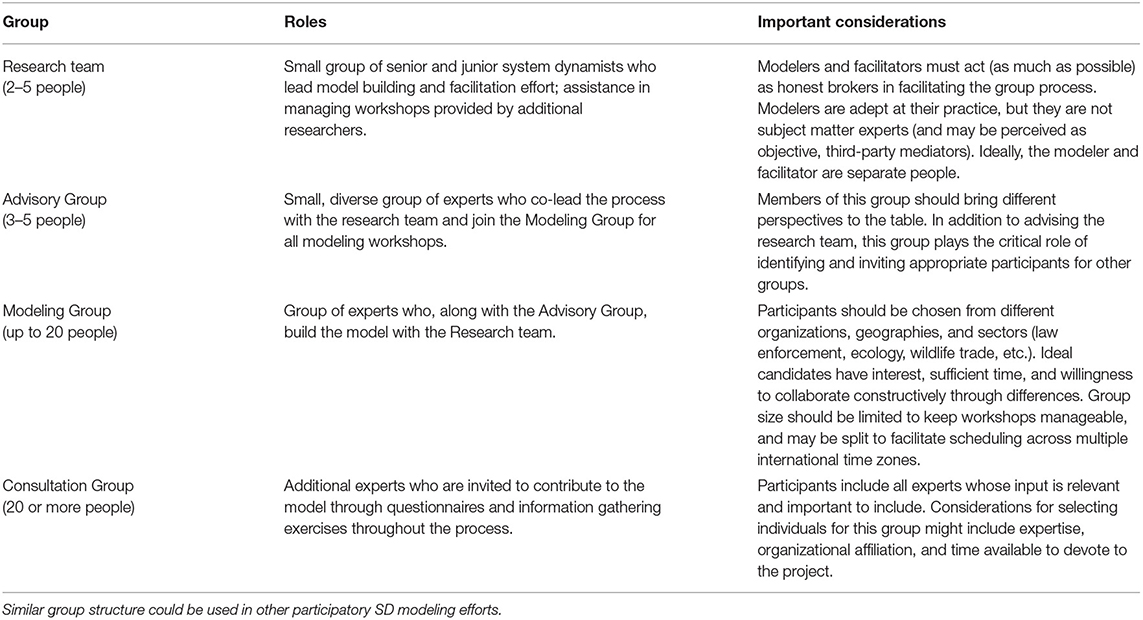
Table 1. Roles and responsibilities for different stakeholder groups engaged in the participatory system dynamics (SD) modeling process designed to improve wild tiger conservation.
Introduction and Problem Familiarization
Since many participants may not be familiar with systems thinking or the participatory SD process, it is critical to provide a road map to illustrate where the modeling process is going. This overview should include a basic review of systems concepts, such as the definition of a system and the concepts of reinforcing and balancing feedback loops and stocks (what is accumulating or declining) and flows (the rate of change). The introduction should also lay out the modeling process timeline, and show examples of what a model looks like to give participants an idea of where the process will end up. Example models should be relevant to participants, but unrelated to the conservation problem being tackled (Beall and Zeoli, 2008). Finally, it is important to get the group talking about the problem they want to address and to begin working toward defining that problem. The amount of time or focus this takes depends on the particular group, the nature of the problem, and how much clarity and agreement already exists.
The first step in our modeling process was an introductory meeting with all participants following official invitations. The meeting covered the history of the project, introductions, and an overview of the overall process. Basic systems concepts were introduced through real-world hypothetical examples. We used the iceberg model (Senge, 1990) to show that observable events, the tip of the iceberg, are part of larger patterns of change caused by unobservable relationships between elements in a system (also called “system structure”). These are further created and shaped by mental models at the bottom of the iceberg. Changing system structure and mental models produce long term change (Senge, 1990). A demonstration of a simple working dynamic simulation model provided participants with a vision of the end result of their efforts. The meeting ended with a group brainstorm around the broader issue of tiger conservation and the greatest concerns related to tiger farming (Figure 1, Problem Familiarization). The most important problems participants identified fell into the following major categories:
• Demand for tiger parts and products (or understanding drivers of demand)
• Lack of understanding the connections between wild tigers and tiger farms
• Market dynamics (price, supply of parts, diversity of products and consumers, etc.)
• Consumer behavior change
• Trade and criminality
• Governance and regulation
• Law enforcement
Setting expectations for participants about the importance of integrating diverse perspectives, including those of potential adversaries, was a key element of the first few meetings. We focused on creating an atmosphere of trying to understand the problem and not to debate specific positions.
Problem Definition and System Conceptualization
Modeling a system without a boundary or a clear focus would produce a model that was unnecessarily, and maybe impossibly, complex and impractical (Sterman, 2000). For this reason, the next step in the modeling process is defining the dynamic problem the group wants to address. A dynamic problem is composed of multiple variables that are changing over time (Homer, 2019), such as a declining wild tiger population, increasing demand for tiger products, and increasing tiger farms. Getting clarity on the problem can be one of the most challenging parts of the process, and may be revisited multiple times as knowledge increases (Mashayekhi and Ghili, 2012). Once the problem has been defined, then the system surrounding that problem can be conceptualized. A qualitative model known as a causal-loop diagram (CLD) is iteratively built based on expert judgment and opinion, followed by reflection about the problem and the system elements. The CLD is later used as the foundation of the simulation model. Approaches to eliciting this initial model vary, but efforts such as Scriptapedia (Hovmand et al., 2012) and the Online System Dynamics Collaborative (https://onlinesd.w.uib.no/) provide tested facilitation scripts to get started.
We are using a combination of questionnaires (that include an adapted Delphi process) and small group workshops to develop consensus around the problem and system components. We began with a pre-modeling survey that contained two open-ended questions focused on understanding the potential impacts of tiger farms on the illegal tiger trade and tiger conservation: (1) what is the most important problem to address within this system? and (2) what factors are contributing to this problem? Answers to these initial questions were summarized and used to inform discussions in the introductory meeting with the modeling group (See introduction and problem familiarization above).
After collecting and synthesizing this information, we hosted a longer workshop to begin the collaborative system conceptualization process. We did this with variable elicitation and behavior over time exercises adapted to the Miro online platform (https://miro.com) in Workshop #1. Participant-defined variables, along with relevant behavior over time graphs describing how variables have shifted in the past and predicting how they might shift in the future, were then worked into a CLD. The CLD was modified to include key stocks and flows important in the quantitative model (Figure 2) (Homer, 2019). The model was further developed in Workshop #2. Examples of key components of this model include: farmed tiger populations and farm capacity, connections between farmed tiger mortality and sales of products, consumer demand for products and factors that influence demand, and wild tiger population dynamics. Between workshops, the modeling team worked to refine the model and incorporate additional input through one-on-one conversations. When this paper was written, our modeling effort remained in this stage. The following sections outline next steps that could be taken in this, or any other, participatory SD modeling process.
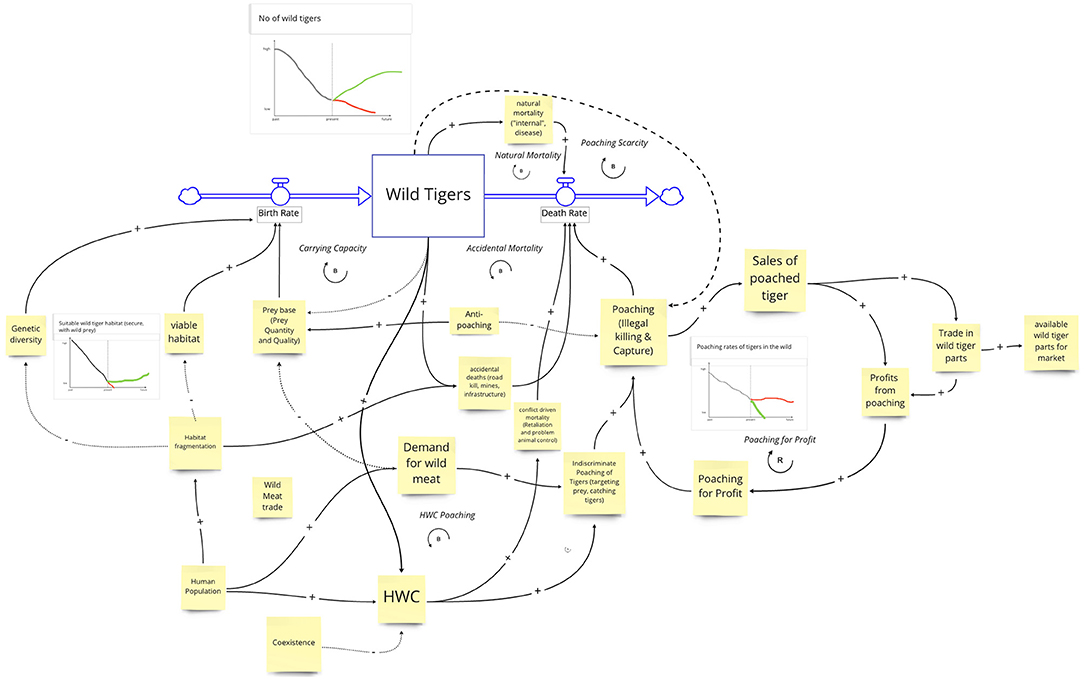
Figure 2. Example of causal-loop diagram (with stock-flow structure added) of wild tiger population developed in Miro during Workshops 1 & 2 of the participatory SD modeling process. Arrows depict causal relationships, with solid arrows representing a positive relationship and dotted representing negative relationships.
Model Development, Testing and Analysis
Once the initial qualitative CLD is developed around the problem, it is transferred to SD modeling software for creation of a dynamic simulation model. Popular software programs used for this purpose include Stella (https://www.iseesystems.com/store/products/) or Vensim (https://vensim.com). With this transition, additional questions as well as gaps in logic and knowledge become apparent, and changes to the problem definition or system components are common. Developing, testing and analyzing the quantitative simulation model happens through frequent dialogue between the research team and participants in the modeling group. As the modeler creates the model, they seek input and approval from the modeling group to refine overall system structure and to ensure necessary data are included. Data may include peer reviewed literature, public or private datasets, and local or expert knowledge. Not every relationship and variable within the system of interest will be captured by the simulation model. The focus of SD model building is to build as simple an explanation for the underlying historic behavior as possible. It is impossible to capture all relationships, but this is often unnecessary for understanding the major endogenous influences of problem behavior. As the model is being built, and before it is finalized, it should go through rigorous testing including structure and parameter confirmation, extreme condition testing, and sensitivity analysis (Forrester, 1980; Sterman, 2000).
For this study, we are using Stella Architect software for the simulation model and complementing this with Miro as a collaborative space for model development. Once the qualitative CLD is sufficiently complete in the previous step, the draft simulation model will be created in Stella (see simplified example in Figure 3). This model will be built sector by sector, starting with tigers in farms, then demand (and purchasing) of wild and farmed products, and finally linking to poaching of wild tigers. The model draft goes through a model review exercise (https://en.wikibooks.org/wiki/Scriptapedia/Model_Review), after which it is further refined by the modeler. After initial system structure has been determined, the second-round questionnaire using the Delphi process will ask stakeholders to review summarized feedback from round one and the logical integrity of the model, provide input into model parameters, and share additional relevant data sources. Information collected will then be summarized, shared with participants (in Workshop #3 and through another round of Delphi questionnaires in analysis of policy interventions) and used to revise the initial model presented in Workshop #3. In addition to these activities, one-on-one meetings will take place between the modeler and participants to answer questions as they arise.
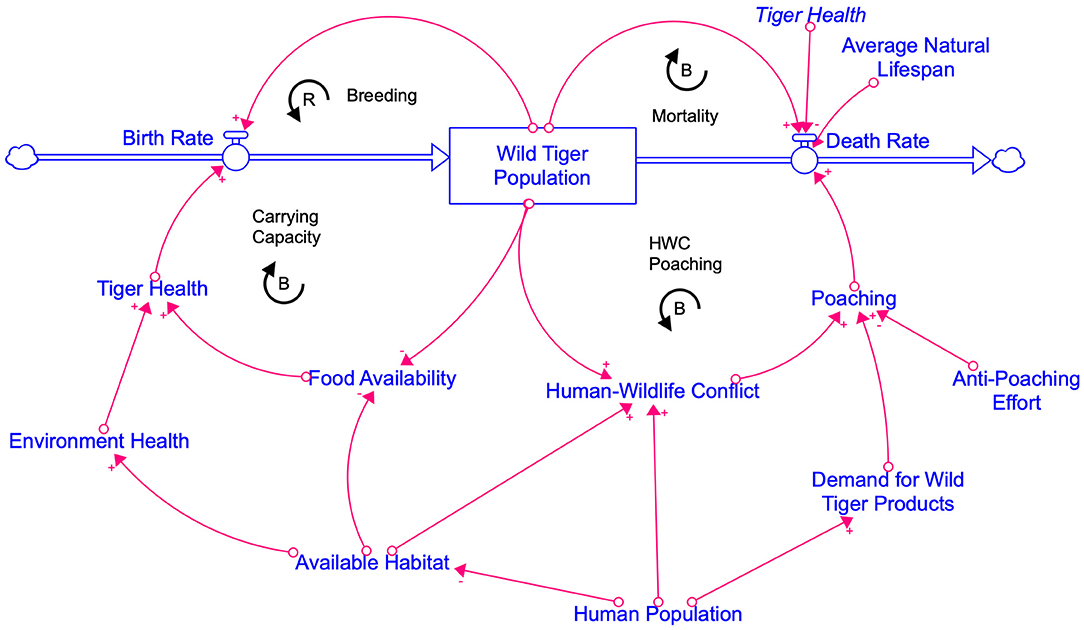
Figure 3. Hypothetical example of a stock-flow model of wild tiger population dynamics, developed using Stella Architect software.
Analysis of Policy Interventions
One of the primary benefits of an SD model is that it can be used as an experimental platform to explore and evaluate the potential implications of policy interventions (Stave, 2010; Sterman et al., 2013; Turner, 2020). Once the model has been validated and can approximate historical behavior, policy interventions can be added and a more user-friendly interface can be built to help stakeholders interact with the model and discern the impacts of various policy options. An example demonstrating the potential of an SD simulation interface is the C-ROADS climate simulation model from Climate Interactive (Sterman et al., 2012; https://www.climateinteractive.org/tools/c-roads/). Stakeholders involved in the participatory SD process choose the policy interventions to be tested, in collaboration with the modeler.
For our case study, the ability to test potential implications of policies related to tiger farms is a main focus. Once the final draft of the model is ready for scrutiny, a final round in the Delphi process will create an opportunity for the full participant group to review summarized results earlier surveys, to provide input into key components and results of the model, and to suggest priority policy interventions. Input from the Delphi questionnaire will be summarized, reported back to the modeling group, and used to add policy interventions into the model. We anticipate this model could provide an opportunity to explore the potential implications of closing or phasing out tiger farms, or tightening restrictions to the trade or sale of tiger parts and products. Once policies and an interactive interface are added, Workshop #4 will give the modeling group an opportunity to test the model and explore the impacts of different scenarios. To mark the end of this stage and the whole process, a full-project presentation will share SD model results with all participants and provide a forum for reflecting on the process and discussing next steps for policy and practice.
Evaluating the Process, Outputs and Outcomes
A key benefit of participatory SD modeling is its potential impacts on shared knowledge building and social outcomes such as trust, communication, and consensus (Rouwette et al., 2002). Evaluation is necessary to verify outcomes and gather feedback to improve further participatory modeling efforts. Participatory approaches to SD have been evaluated in many cases, generally yielding positive outcomes (Rouwette et al., 2002; Rouwette, 2011; Hovmand et al., 2012; Scott et al., 2016b; Stave et al., 2019). Yet, to determine if the process is achieving desired goals, both output and outcome evaluation are an essential part of any modeling effort.
We are integrating evaluation throughout our modeling process. Following recommendations by Scott et al. (2013) we are employing a pre-post survey model. We adapted survey protocols from literature evaluating other collaborative model-building processes (Rouwette et al., 2007; Scott et al., 2016a, 2017), and we are distributing questionnaires to all groups of participants using Qualtrics XM software (https://www.qualtrics.com/). The pre-intervention questionnaire, which doubled as the first round of the Delphi process, included questions about participants' areas of expertise, specific perspectives on tiger conservation and tiger farms, and previous experience with systems thinking (see Supplemental survey instrument). We also included questions designed to measure key process outcomes such as knowledge and understanding (Rouwette et al., 2016; Scott et al., 2016a), trust (Stern and Coleman, 2014; Basco-Carrera et al., 2017), and consensus and commitment among conservation practitioners (Rouwette, 2011; Scott et al., 2016b; Basco-Carrera et al., 2017). We aim to integrate a post-intervention questionnaire that allows the research team to measure changes in responses from the beginning to the end of the modeling process. This final questionnaire will include additional questions to gather feedback about understanding of the dynamics in the tiger conservation system (i.e., connections between wild tigers, demand for tiger parts and products, and tiger farming), the utility of the final model, and perspectives on how the process itself influenced perceived outcomes such as knowledge, trust, communication and consensus and commitment (i.e., the same outcomes addressed on the pre-intervention questionnaire). Multiple rounds of Delphi questionnaires integrated throughout the process will help us track the evolution of participants' thinking regarding the problem(s) and the complex system surrounding tiger conservation.
Implications For Conservation Practice
Conservation practitioners work in complex social-ecological systems to address threats facing biodiversity, reduce conflict, and promote positive human-wildlife interactions. Inadequate understanding of the direct and indirect, as well as short- and long-term, consequences of decision making within these dynamic systems can lead to misdiagnosed problems and interventions with perverse outcomes, exacerbating conflict (Larrosa et al., 2016; Hübschle, 2017). Participatory SD provides an opportunity to minimize these risks through building a more complete shared understanding of a problem and potential implications of interventions. This is achieved while increasing trust and reducing conflict among stakeholders working to tackle these wicked problems. Once created, a simulation model can also be used as an experimental platform that is almost impossible to replicate in situ with threatened ecosystems and endangered species.
The process we have outlined in this paper shows how conservation researchers and practitioners can design and implement participatory SD modeling to address a complex problem such as wild tiger conservation. Throughout our ongoing modeling process, we have confronted conflicting perspectives and worked toward shared understandings of the tiger farming problem and its consequences. Through iterative meetings and conversations combining science with expert knowledge, we are building trust and fostering productive collaboration. As our simulation model progresses, it should yield insights regarding policy interventions that enhance the value of the process for participants. By strategically dissecting the social and political relationships that fuel many conservation conflicts (Madden and McQuinn, 2014), participatory SD processes like ours may be a key step on the path to sustainable coexistence between humans and tigers.
The participatory SD modeling process does not occur without challenges (Addison et al., 2013; Stave et al., 2019). It requires a large time commitment for both the research team and the modeling group. Our study, for example, will have taken multiple years from the initial advisory group meetings to development of the final policy model. The process at minimum requires a competent modeler and, ideally, a facilitator who has experience with systems modeling. Since participatory SD modeling is not common in the conservation world, there is a learning curve for participants to help them understand where the process is going and how to realize its value. Additionally, with a polarized topic such as tiger conservation, it is challenging to find diverse stakeholders willing to participate. Finally, while SD models provide insights that can help to guide management and policy, key decision makers must be willing and able to utilize these tools to initiate action. Strategies for addressing these potential barriers undoubtedly vary by context, but investments of time and resources into systems-based approaches could ultimately lead to long-term changes the way conservation efforts are conceptualized and carried out.
Despite challenges, enthusiasm with our effort to model the impacts of tiger farming on wild tiger conservation remains high. Some participants may not be able to engage in the whole process, but excitement has grown as conversations delve deeper into complex issues and the practical implications and potential policy impacts of the effort become more apparent. Our approach is showing how systems thinking and systems-based approaches can help to address the complex social, economic, political, and ecological problems that threaten the survival of wild tigers. Application of systems thinking could improve coexistence with other species where contentious policy choices are being critically evaluated, such as elephants (Mahajan et al., 2019), rhinos ('t Sas-Rolfes, 2016), and wild horses (BLM, 2020); it could also facilitate understanding of conservation issues that span multiple species and contexts, such as the substitutability of tiger and lion products across the farmed/wild nexus (Coals et al., 2020; Rizzolo, 2021). Additionally, SD approaches create unique opportunities to explore the effects of different property rights or management regimes on wildlife (Wilson et al., 2016). Regardless of geography, focal species, or management context, participatory SD modeling could represent a valuable tool in a conservation practitioner's toolbox to address conflict and improve coexistence with wildlife around the world.
Data Availability Statement
The raw data supporting the conclusions of this article will be made available by the authors, without undue reservation.
Ethics Statement
The studies involving human participants were reviewed and approved by Institutional Review Board of North Carolina State University (IRB 21209). The patients/participants provided their written informed consent to participate in this study.
Author Contributions
ER and LL are the principal investigators of the study and oversaw design of the conceptualization of the study, design and implementation of the process (including workshop facilitation), and the writing of this manuscript. BK helped design the participatory SD process, assisted with workshop facilitation, and assisted with manuscript writing. M'tS-R participated in the participatory SD process and assisted with manuscript writing. All authors contributed substantially to the article and approved the submitted version.
Conflict of Interest
The authors declare that the research was conducted in the absence of any commercial or financial relationships that could be construed as a potential conflict of interest.
Publisher's Note
All claims expressed in this article are solely those of the authors and do not necessarily represent those of their affiliated organizations, or those of the publisher, the editors and the reviewers. Any product that may be evaluated in this article, or claim that may be made by its manufacturer, is not guaranteed or endorsed by the publisher.
Acknowledgments
We would like to acknowledge the participants from NGOs and other organizations around the world who continue to contribute to our participatory modeling process as we work collectively to advance tiger conservation.
Supplementary Material
The Supplementary Material for this article can be found online at: https://www.frontiersin.org/articles/10.3389/fcosc.2021.696615/full#supplementary-material
References
Abbott, B., and van Kooten, G. C. (2011). Can domestication of wildlife lead to conservation? The economics of tiger farming in China. Ecol. Econ. 70, 721–728. doi: 10.1016/j.ecolecon.2010.11.006
Addison, P. F. E., Rumpff, L., Bau, S. S., Carey, J. M., Chee, Y. E., Jarrad, F. C., et al. (2013). Practical solutions for making models indispensable in conservation decision-making. Divers. Distrib. 19, 490–502. doi: 10.1111/ddi.12054
Andersen, D. F., Richardson, G. P., and Vennix, J. A. M. (1997). Group model building: adding more science to the craft. Syst. Dyn. Rev. 13, 187–201. doi: 10.1002/(SICI)1099-1727(199722)13:2<187::AID-SDR124>3.0.CO;2-O
Andersen, D. F., Vennix, J. A. M., Richardson, G. P., and Rouwette, E. A. J. A. (2017). Group model building: problem structuring, policy simulation and decision support. J. Oper. Res. Soc. 58, 691–694. doi: 10.1057/palgrave.jors.2602339
Appel, J. M., Fullerton, K., Hennessy, E., Korn, A. R., Tovar, A., Allender, S., et al. (2019). Design and methods of Shape Up Under 5: Integration of systems science and community-engaged research to prevent early childhood obesity. PLoS ONE 14:e0220169. doi: 10.1371/journal.pone.0220169
Ban, N. C., Mills, M., Tam, J., Hicks, C. C., Klain, S., Stoeckl, N., et al. (2013). A social–ecological approach to conservation planning: embedding social considerations. Front. Ecol. Environ. 11, 194–202. doi: 10.1890/110205
Basco-Carrera, L., van Beek, E., Jonoski, A., Benítez-Ávila, C., and Pj Guntoro, F. X. (2017). Collaborative modelling for informed decision making and inclusive water development. Water Resour. Manage. 31, 2611–2625. doi: 10.1007/s11269-017-1647-0
Baynham-Herd, Z., Redpath, S., Bunnefeld, N., Molony, T., and Keane, A. (2018). Conservation conflicts: behavioural threats, frames, and intervention recommendations. Biol. Conserv. 222, 180–188. doi: 10.1016/j.biocon.2018.04.012
Beall, A., and Ford, A. (2010). Reports from the field: assessing the art and science of participatory environmental modeling. Int. J. Inf. Syst. Soc. Change 1, 72–89. doi: 10.4018/jissc.2010040105
Beall, A., and Zeoli, L. (2008). Participatory modeling of endangered wildlife systems: simulating the sage-grouse and land use in Central Washington. Ecol. Econ. 68, 24–33. doi: 10.1016/j.ecolecon.2008.08.019
Bennett, E. L., Underwood, F. M., and Milner-Gulland, E. J. (2021). To trade or not to trade? Using Bayesian belief networks to assess how to manage commercial wildlife trade in a complex world. Front. Ecol. Evol. 9:587896. doi: 10.3389/fevo.2021.587896
Bennett, N. J., Roth, R., Klain, S. C., Chan, K., Christie, P., Clark, D. A., et al. (2017). Conservation social science: Understanding and integrating human dimensions to improve conservation. Biol. Conserv. 205, 93–108. doi: 10.1016/j.biocon.2016.10.006
Biggs, D., Courchamp, F., Martin, R., and Possingham, H. P. (2013). Legal trade of Africa's Rhino Horns. Science 339, 1038–1039. doi: 10.1126/science.1229998
Brashares, J. S., Abrahms, B., Fiorella, K. J., Golden, C. D., Hojnowski, C. E., Marsh, R. A., et al. (2014). Wildlife decline and social conflict. Science 345, 376–378. doi: 10.1126/science.1256734
Brechin, S. R., Wilshusen, P. R., Fortwangler, C. L., and West, P. C. (2002). Beyond the square wheel: toward a more comprehensive understanding of biodiversity conservation as social and political process. Soc. Nat. Resour. 15, 41–64. doi: 10.1080/089419202317174011
Bureau of Land Management (BLM) (2020). Washington, DC: United States Department of Interior. Retrieved from: https://www.blm.gov/sites/blm.gov/files/WHB-Report-2020-NewCover-051920-508.pdf
Carter, N. H., Riley, S. J., Shortridge, A., Shrestha, B. K., and Liu, J. (2014). Spatial assessment of attitudes toward tigers in Nepal. Ambio 43, 125–137. doi: 10.1007/s13280-013-0421-7
Challender, D. W. S., Harrop, S. R., and MacMillan, D. C. (2015). Understanding markets to conserve trade-threatened species in CITES. Biol. Conserv. 187, 249–259. doi: 10.1016/j.biocon.2015.04.015
Challender, D. W. S., and MacMillan, D. C. (2014). Poaching is more than an enforcement problem. Conserv. Lett. 7, 484–494. doi: 10.1111/conl.12082
Chapron, G., Miquelle, D. G., Lambert, A., Goodrich, J. M., Legendre, S., and Clobert, J. (2008). The impact on tigers of poaching versus prey depletion. J. Appl. Ecol. 45, 1667–1674. doi: 10.1111/j.1365-2664.2008.01538.x
CMP (2020). The Open Standards for the Practice of Conservation, Version 4.0. Conservation Measures Partnership. Accessed from: https://conservationstandards.org/wp-content/uploads/sites/3/2020/10/CMP-Open-Standards-for-the-Practice-of-Conservation-v4.0.pdf
Coals, P., Moorhouse, T. P., D'Cruze, N. C., Macdonald, D. W., and Loveridge, A. J. (2020). Preferences for lion and tiger bone wines amongst the urban public in China and Vietnam. J. Nat. Conserv. 57:125874. doi: 10.1016/j.jnc.2020.125874
Courchamp, F., Angulo, E., Rivalan, P., Hall, R. J., Signoret, L., Bull, L., et al. (2006). Rarity value and species extinction: the anthropogenic allee effect. PLoS Biol. 4:e415. doi: 10.1371/journal.pbio.0040415
Currie, D. J., Smith, C., and Jagals, P. (2018). The application of system dynamics modelling to environmental health decision-making and policy - a scoping review. BMC Public Health 18:402. doi: 10.1186/s12889-018-5318-8
Davies, K. K., Fisher, K. T., Dickson, M. E., Thrush, S. F., and Le Heron, R. (2015). Improving ecosystem service frameworks to address wicked problems. Ecol. Soc. 20:37. doi: 10.5751/ES-07581-200237
Davis, E., Veríssimo, D., Crudge, B., Sam, S., Cao, D., Ho, P., et al. (2021). How Will the End of Bear Bile Farming in Vietnam Influence Consumer Choice? Preprint. doi: 10.31235/osf.io/quyf2
de Vos, A., Biggs, R., and Preiser, R. (2019). Methods for understanding social-ecological systems: a review of place-based studies. Ecol. Soc. 24:16. doi: 10.5751/ES-11236-240416
Dinerstein, E., Loucks, C., Wikramanayake, E., Ginsberg, J., Sanderson, E., Seidensticker, J., et al. (2007). The fate of wild tigers. BioScience 57, 508–514. doi: 10.1641/B570608
Dutton, A. J., Hepburn, C., and Macdonald, D. W. (2011). A stated preference investigation into the Chinese demand for farmed vs. wild bear bile. PLoS ONE 6:e21243. doi: 10.1371/journal.pone.0021243
EIA (2017). Cultivating Demand: The Growing Threat of Tiger Farms. London: Environmental Investigation Agency.
Faust, L. J., Jackson, R., Ford, A., Earnhardt, J. M., and Thompson, S. D. (2004). Models for management of wildlife populations: lessons from spectacled bears in zoos and grizzly bears in Yellowstone. Syst. Dyn. Rev. 20, 163–178. doi: 10.1002/sdr.291
Fischer, C. (2004). The complex interactions of markets for endangered species products. J. Environ. Econ. Manage. 48, 926–953. doi: 10.1016/j.jeem.2003.12.003
Ford, A. (1997). System Dynamics and the Electric Power Industry. Syst. Dyn. Rev. 13, 57–85. doi: 10.1002/(SICI)1099-1727(199721)13:1<57::AID-SDR117>3.0.CO;2-B
Ford, A. (1999). Modeling the Environment. An introduction to System Dynamics Modeling of Environmental Systems. Washington, DC: Island Press.
Forrester, J. W. (1971). Counterintuitive behavior of social systems. Technol. Forecast. Soc. Change 73, 1–22. doi: 10.1016/S0040-1625(71)80001-X
Forrester, J. W. (1980). Tests for building confidence in system dynamics models. Syst. Dyn. TIMS Stud. Manage. Sci. 14, 209–228.
Forrester, J. W. (1994). System dynamics, systems thinking and soft OR. Syst. Dyn. Rev. 10, 245–256. doi: 10.1002/sdr.4260100211
Fowler, P. J., Marcal, K. E., Chung, S., Brown, D. S., Jonson-Reid, M., and Hovmand, P. S. (2019). Scaling up housing services within the child welfare system: policy insights from simulation modeling. Child Maltreat 25, 51–60. doi: 10.1177/1077559519846431
Frerichs, L., Lich, K. H., Dave, G., and Corbie-Smith, G. (2016). Integrating systems science and community-based participatory research to achieve health equity. Am. J. Public Health 106, 215–222. doi: 10.2105/AJPH.2015.302944
Gallagher, L., Kopainsky, B., Bassi, A. M., Betancourt, A., Buth, C., Chan, P., et al. (2020). Supporting stakeholders to anticipate and respond to risks in a Mekong River water-energy-food nexus. Ecol. Soc. 25:29. doi: 10.5751/ES-11919-250429
Game E. T. Meijaard E. Sheil D. and McDonald-Madden, E. (2014). Conservation in a Wicked Complex World; Challenges and Solutions. Conserv. Lett. 7, 271–277. doi: 10.1111/conl.12050
Goodrich, J. M., Lynam, A., Miquelle, D. G., Wibisono, H. T., Kawanishi, K., Pattanavibool, A., et al. (2015). Panthera tigris, Tiger. The IUCN Red List of Threatened Species. IUCN (International Union for Conservation of Nature).
Gratwicke, B., Mills, J., Dutton, A., Gabriel, G., Long, B., Seidensticker, J., et al. (2008). Attitudes toward consumption and conservation of tigers in China. PLoS ONE 3:e2544. doi: 10.1371/journal.pone.0002544
Gregory, R., Failing, L., Harstone, M., Long, G., McDaniels, T., and Ohlson, D. (2012). Structured Decision Making: A Practical Guide to Environmental Management Choices. Hoboken, NJ: John Wiley & Sons. doi: 10.1002/9781444398557
Hall, R. J., Milner-Gulland, E. J., and Courchamp, F. (2008). Endangering the endangered: The effects of perceived rarity on species exploitation. Conserv. Lett. 1, 75–81. doi: 10.1111/j.1755-263X.2008.00013.x
Hinsley, A., and 't Sas-Rolfes, M. (2020). Wild assumptions? Questioning simplistic narratives about consumer preferences for wildlife products. People Nat. 2, 972–979. doi: 10.1002/pan3.10099
Homer, J. B. (2019). Best practices in system dynamics modeling, revisited: a practitioner's view. Syst. Dyn. Rev. 35, 177–181. doi: 10.1002/sdr.1630
Hovmand, P. S., Andersen, D. F., Rouwette, E., Richardson, G. P., Rux, K., and Calhoun, A. (2012). Group model-building ‘scripts' as a collaborative planning tool. Syst. Res. Behav. Sci. 29, 179–193. doi: 10.1002/sres.2105
Hsu, C.-C., and Sandford, B. A. (2007). The Delphi technique: making sense of consensus. Pract. Assess. Res. Eval. 12:10. doi: 10.7275/pdz9-th90
Hübschle, A. (2017). The social economy of rhino poaching: of economic freedom fighters, professional hunters and marginalized local people. Curr. Sociol. 65, 427–447. doi: 10.1177/0011392116673210
Jiang, Z., Li, C., Fang, H., Meng, Z., and Zeng, Y. (2007). Captive-bred tigers and the fate of wild tigers. BioScience 57, 725–725. doi: 10.1641/B570922
Kenney, J. S., Smith, J. L. D., Starfield, A. M., and Mcdougal, C. W. (1995). The long-term effects of tiger poaching on population viability. Conserv. Biol. 9, 1127–1133. doi: 10.1046/j.1523-1739.1995.9051116.x-i1
Kim, D. H., and Senge, P. M. (1994). Putting systems thinking into pratice. Syst. Dyn. Rev. 10, 277–290. doi: 10.1002/sdr.4260100213
Kirkpatrick, R. C., and Emerton, L. (2010). Killing tigers to save them: fallacies of the farming argument. Conserv. Biol. 24, 655–659. doi: 10.1111/j.1523-1739.2010.01468.x
Kopainsky, B., Hager, G., Herrera, H., and Nyanga, P. H. (2017). Transforming food systems at local levels: using participatory system dynamics in an interactive manner to refine small-scale farmers' mental models. Ecol. Model. 362, 101–110. doi: 10.1016/j.ecolmodel.2017.08.010
Krafte Holland, K., Larson, L. R., and Powell, R. B. (2018). Characterizing conflict between humans and big cats Panthera spp: a systematic review of research trends and management opportunities. PLoS ONE 13:e0203877. doi: 10.1371/journal.pone.0203877
Larrosa, C., Carrasco, L. R., and Milner-Gulland, E. J. (2016). Unintended feedbacks: challenges and opportunities for improving conservation effectiveness. Conserv. Lett. 9, 316–326. doi: 10.1111/conl.12240
Lele, S., Wilshusen, P., Brockington, D., Seidler, R., and Bawa, K. (2010). Beyond exclusion: alternative approaches to biodiversity conservation in the developing tropics. Curr. Opin. Environ. Sustain. 2, 94–100. doi: 10.1016/j.cosust.2010.03.006
Linkie, M., Martyr, D., Harihar, A., Mardiah, S., Hodgetts, T., Risdianto, D., et al. (2018). Asia's economic growth and its impact on Indonesia's tigers. Biol. Conserv. 219, 105–109. doi: 10.1016/j.biocon.2018.01.011
Luna-Reyes, L. F., and Andersen, D. L. (2003). Collecting and analyzing qualitative data for system dynamics: methods and models. Syst. Dyn. Rev. 19, 271–296. doi: 10.1002/sdr.280
Madden, F., and McQuinn, B. (2014). Conservation's blind spot: the case for conflict transformation in wildlife conservation. Biol. Conserv. 178, 97–106. doi: 10.1016/j.biocon.2014.07.015
Mahajan, S. L., Glew, L., Rieder, E., Ahmadia, G., Darling, E., Fox, H. E., et al. (2019). Systems thinking for planning and evaluating conservation interventions. Conserv. Sci. Pract. 1:e44. doi: 10.1111/csp2.44
Mashayekhi, A. N., and Ghili, S. (2012). System dynamics problem definition as an evolutionary process using the concept of ambiguity. Syst. Dyn. Rev. 28, 182–198. doi: 10.1002/sdr.1469
Montgomery, R. A., Borona, K., Kasozi, H., Mudumba, T., and Ogada, M. (2020). Positioning human heritage at the center of conservation practice. Conserv. Biol. 34, 1122–1130. doi: 10.1111/cobi.13483
Rasphone, A., Kéry, M., Kamler, J. F., and Macdonald, D. W. (2019). Documenting the demise of tiger and leopard, and the status of other carnivores and prey, in Lao PDR's most prized protected area: Nam Et - Phou Louey. Global Ecol. Conserv. 20:e00766. doi: 10.1016/j.gecco.2019.e00766
Rizzolo, J. B. (2021). Effects of legalization and wildlife farming on conservation. Global Ecol. Conserv. 25:e01390. doi: 10.1016/j.gecco.2020.e01390
Rouwette, E., Bleijenbergh, I., and Vennix, J. (2016). Group model-building to support public policy: addressing a conflicted situation in a problem neighbourhood. Syst. Res. Behav. Sci. 33, 64–78. doi: 10.1002/sres.2301
Rouwette, E. A. J. A. (2011). Facilitated modelling in strategy development: measuring the impact on communication, consensus and commitment. J. Oper. Res. Soc. 62, 879–887. doi: 10.1057/jors.2010.78
Rouwette, E. A. J. A., Korzilius, H., Vennix, J. A. M., and Jacobs, E. (2011). Modeling as persuasion: the impact of group model building on attitudes and behavior. Syst. Dyn. Rev. 27, 1–21. doi: 10.1002/sdr.441
Rouwette, E. A. J. A., Vennix, J. A. M., and Felling, A. J. A. (2007). On evaluating the performance of problem structuring methods: an attempt at formulating a conceptual model. Group Decis. Negot. 18, 567–587. doi: 10.1007/s10726-007-9100-z
Rouwette, E. A. J. A., Vennix, J. A. M., and Mullekom, T. V. (2002). Group model building effectiveness: a review of assessment studies. Syst. Dyn. Rev. 18, 5–45. doi: 10.1002/sdr.229
Scott, R. J., Cavana, R. Y., and Cameron, D. (2013). Evaluating immediate and long-term impacts of qualitative group model building workshops on participants' mental models. Syst. Dyn. Rev. 29, 216–236. doi: 10.1002/sdr.1505
Scott, R. J., Cavana, R. Y., and Cameron, D. (2016a). Mechanisms for understanding mental model change in group model building. Syst. Res. Behav. Sci. 33, 100–118. doi: 10.1002/sres.2303
Scott, R. J., Cavana, R. Y., and Cameron, D. (2016b). Recent evidence on the effectiveness of group model building. Eur. J. Oper. Res. 249, 908–918. doi: 10.1016/j.ejor.2015.06.078
Scott, R. J., Cavana, R. Y., and Cameron, D. (2017). Interpersonal success factors for strategy implementation: a case study using group model building. J. Oper. Res. Soc. 66, 1023–1034. doi: 10.1057/jors.2014.70
Sedlacko, M., Martinuzzi, A., Røpke, I., Videira, N., and Antunes, P. (2014). Participatory systems mapping for sustainable consumption: discussion of a method promoting systemic insights. Ecol. Econ. 106, 33–43. doi: 10.1016/j.ecolecon.2014.07.002
Senge, P. M. (1990). The Fifth Discipline: The Art and Practice of the Learning Organization. New York, NY: Doubleday/Currency 1994.
Sharma, K., Wright, B., Joseph, T., and Desai, N. (2014). Tiger poaching and trafficking in India: estimating rates of occurrence and detection over four decades. Biol. Conserv. 179, 33–39. doi: 10.1016/j.biocon.2014.08.016
Song, A. Y., and Yao, Y. (2021). To ban or not to ban: china's trade in endangered species. J. Contemp. China 1–15. doi: 10.1080/10670564.2021.1926103
Stave, K. (2010). Participatory system dynamics modeling for sustainable environmental management: observations from four cases. Sustainability 2, 2762–2784. doi: 10.3390/su2092762
Stave, K., Dwyer, M., and Turner, M. (2019). Exploring the value of participatory system dynamics in two paired field studies of stakeholder engagement in sustainability discussions. Syst. Res. Behav. Sci. 36, 156–179. doi: 10.1002/sres.2576
Sterman, J. (1994). Learning in and about complex systems. Syst. Dyn. Rev. 10, 291–330. doi: 10.1002/sdr.4260100214
Sterman, J., Fiddaman, T., Franck, T., Jones, A., McCauley, S., Rice, P., et al. (2012). Climate interactive: the C-ROADS climate policy model. Syst. Dyn. Rev. 28, 295–305. doi: 10.1002/sdr.1474
Sterman, J. D. (2000). Business Dynamics: Systems Thinking and Modeling for a Complex World. New York, NY: Irwin/McGraw-Hill.
Sterman, J. D., Fiddaman, T., Franck, T., Jones, A., McCauley, S., Rice, P., et al. (2013). Management flight simulators to support climate negotiations. Environ. Model. Softw. 44, 122–135. doi: 10.1016/j.envsoft.2012.06.004
Stern, M. J., and Coleman, K. J. (2014). The multidimensionality of trust: applications in collaborative natural resource management. Soc. Nat. Resour. 28, 117–132. doi: 10.1080/08941920.2014.945062
Struebig, M. J., Linkie, M., Deere, N. J., Martyr, D. J., Millyanawati, B., Faulkner, S. C., et al. (2018). Addressing human-tiger conflict using socio-ecological information on tolerance and risk. Nat. Commun. 9:3455. doi: 10.1038/s41467-018-05983-y
't Sas-Rolfes, M. (2010). Tigers, Economics, and the Regulation of Trade. Tigers of the World. Amsterdam: Elsevier Inc. 477–490. doi: 10.1016/B978-0-8155-1570-8.00039-6
't Sas-Rolfes, M. (2016). Rhino poaching: what is the solution? Solut. J. Available online at: https://thesolutionsjournal.com/2016/03/20/rhino-poaching-what-is-the-solution/
Torri, M. (2011). Conservation, relocation and the social consequences of conservation policies in protected areas: case study of the Sariska Tiger Reserve, India. Conserv. Soc. 9:54. doi: 10.4103/0972-4923.79190
Trani, J. F., Ballard, E., Bakhshi, P., and Hovmand, P. (2016). Community based system dynamic as an approach for understanding and acting on messy problems: a case study for global mental health intervention in Afghanistan. Confl. Health 10:25. doi: 10.1186/s13031-016-0089-2
Turner, B., Menendez, H., Gates, R., Tedeschi, L., and Atzori, A. (2016). System dynamics modeling for agricultural and natural resource management issues: review of some past cases and forecasting future roles. Resources 5:40. doi: 10.3390/resources5040040
Turner, B. L. (2020). Model laboratories: a quick-start guide for design of simulation experiments for dynamic systems models. Ecol. Model. 434:109246. doi: 10.1016/j.ecolmodel.2020.109246
Vennix, J. A. M. (1999). Group model-building: tackling messy problems. Syst. Dyn. Rev. 15, 379–401.
Vennix, J. A. M., Gubbels, J. W., Post, D., and Poppen, H. J. (1990). A structured approach to knowledge elicitation in conceptual model building. Syst. Dyn. Rev. 6, 194–208. doi: 10.1002/sdr.4260060205
Villalva, P., and Moracho, E. (2019). Tiger trade threatens big cats worldwide. Science 364:743. doi: 10.1126/science.aax5200
Voinov, A., Jenni, K., Gray, S., Kolagani, N., Glynn, P. D., Bommel, P., et al. (2018). Tools and methods in participatory modeling: selecting the right tool for the job. Environ. Model. Softw. 109, 232–255. doi: 10.1016/j.envsoft.2018.08.028
Walston, J., Robinson, J. G., Bennett, E. L., Breitenmoser, U., da Fonseca, G. A., Goodrich, J., et al. (2010). Bringing the tiger back from the brink-the six percent solution. PLoS Biol. 8:e1000485. doi: 10.1371/journal.pbio.1000485
Weller, F. L.-,Cecchini, A., Shannon, L., Sherley, R. B., Crawford, R. J. M., Altwegg, R., Scott, L., et al. (2014). A system dynamics approach to modelling multiple drivers of the African penguin population on Robben Island, South Africa. Ecol. Model. 277, 38–56. doi: 10.1016/j.ecolmodel.2014.01.013
Wilkerson, B., Aguiar, A., Gkini, C., de Oliveira, I. C., Lunde Trellevik, L. K., and Kopainsky, B. (2020). Reflections on adapting group model building scripts into online workshops. Syst. Dy. Rev.. 36, 358–372. doi: 10.1002/sdr.1662
Williams, V. L., Loveridge, A. J., Newton, D. J., and Macdonald, D. W. (2017). A roaring trade? The legal trade in Panthera leo bones from Africa to East-Southeast Asia. PLoS ONE 12:e0185996. doi: 10.1371/journal.pone.0185996
Wilson, G. R., Hayward, M. W., and Wilson, C. (2016). Market-based incentives and private ownership of wildlife to remedy shortfalls in government funding for conservation. Conserv. Lett. 10, 485–492. doi: 10.1111/conl.12313
Wong, R. W. Y. (2016). The organization of the illegal tiger parts trade in China. Br. J. Criminol. 56, 995–1013. doi: 10.1093/bjc/azv080
World Wildlife Fund (2020). 5 Things Tiger Kind Doesn't Explain About Captive Tigers. Available online at: https://www.worldwildlife.org/stories/5-things-tiger-king-doesn-t-explain-about-captive-tigers (accessed June 20, 2021).
World Wildlife Fund (2021). Tiger. Available online at: https://www.worldwildlife.org/species/tiger (accessed June 20, 2021).
Keywords: human-wildlife conflict, systems thinking, wildlife trade, wildlife farming, participatory modeling
Citation: Rieder E, Larson LR, 't Sas-Rolfes M and Kopainsky B (2021) Using Participatory System Dynamics Modeling to Address Complex Conservation Problems: Tiger Farming as a Case Study. Front. Conserv. Sci. 2:696615. doi: 10.3389/fcosc.2021.696615
Received: 17 April 2021; Accepted: 26 August 2021;
Published: 22 September 2021.
Edited by:
Jenny Anne Glikman, Instituto De Estudios Sociales Avanzados (IESA), SpainReviewed by:
Laura Schmitt Olabisi, Michigan State University, United StatesJessica Bell Rizzolo, Michigan State University, United States
Elizabeth Davis, San Diego Zoo Institute for Conservation Research, United States
Copyright © 2021 Rieder, Larson, 't Sas-Rolfes and Kopainsky. This is an open-access article distributed under the terms of the Creative Commons Attribution License (CC BY). The use, distribution or reproduction in other forums is permitted, provided the original author(s) and the copyright owner(s) are credited and that the original publication in this journal is cited, in accordance with accepted academic practice. No use, distribution or reproduction is permitted which does not comply with these terms.
*Correspondence: Erica Rieder, ZXJpZWRlckBuY3N1LmVkdQ==