- 1Science Department, Osa Conservation, Washington D.C., WA, United States
- 2Deanery of Biomedical Sciences, College of Medicine and Veterinary Medicine, University of Edinburgh, Edinburgh, United Kingdom
- 3Institute of Biodiversity, Animal Health and Comparative Medicine, College of Medical, Veterinary and Life Sciences, University of Glasgow, Glasgow, United Kingdom
- 4Department of Biology, Center for Energy, Environment, and Sustainability, Wake Forest University, Winston-Salem, NC, United States
For decades sea turtle projects around the world have monitored nesting females using labor-intensive human patrolling techniques. Here we describe the first empirical testing of a drone-mounted thermal infrared sensor for nocturnal sea turtle monitoring; on the Osa peninsula in Costa Rica. Preliminary flights verified that the drone could detect similar sea turtle activities as identified by on-the-ground human patrollers – such as turtles, nests and tracks. Drone observers could even differentiate tracks of different sea turtle species, detect sea turtle hatchlings, other wildlife, and potential poachers. We carried out pilot flights to determine optimal parameters for detection by testing different thermal visualization modes, drone heights, and gimbal angles. Then, over seven nights, we set up a trial to compare the thermal drone and operators’ detections with those observed by traditional patrollers. Our trials showed that thermal drones can record more information than traditional sea turtle monitoring methods. The drone and observer detected 20% more sea turtles or tracks than traditional ground-based patrolling (flights and patrols carried out across the same nights at the same time and beach). In addition, the drone operator detected 39 other animals/predators and three potential poachers that patrollers failed to detect. Although the technology holds great promise in being able to enhance detection rates of nesting turtles and other beach activity, and in helping to keep observers safer, we detail challenges and limiting factors; in drone imagery, current cost barriers, and technological advances that need to be assessed and developed before standardized methodologies can be adopted. We suggest potential ways to overcome these challenges and recommend how further studies can help to optimize thermal drones to enhance sea turtle monitoring efforts worldwide.
1 Introduction
Hundreds of projects around the world monitor nesting sea turtles, some for over 50 years, contributing to a global network (Meletis and Harrison, 2010; The State of the World’s Sea Turtles [SWOT], 2022). These projects monitor over 3,200 nesting beaches globally, with over 60 beaches to protect and survey sea turtle nesting beaches in Costa Rica alone (Fonseca et al., 2015; The State of the World’s Sea Turtles [SWOT], 2022). This long-term, global effort has enabled scientists to assess population trends of many species, provided evidence of recovering populations, and elucidated challenges (Mazaris et al., 2017; Piacenza et al., 2019; Godley et al., 2020; Mortimer et al., 2020). These efforts, however, rely upon traditional labor-intensive human patrollers, counting females, their nests, and/or tracks at night, usually relying upon volunteers, students, or young field scientists to gather the data (Shanker et al., 2003; Chacón et al., 2007; Quesada-Rodríguez et al., 2021).
There are various limitations and dangers associated with traditional monitoring methods, largely related to the sustainability of this labor demanding approach and sustainability of financial resources. These limitations were heightened by the COVID-19 pandemic, stressing both personnel and finances, leading to the inability to maintain data collection, nest management, and anti-poaching surveillance (Gardner, 2020; Quesada-Rodríguez et al., 2021). Poaching is a major threat for adult female sea turtles and their nests, and for the patrollers themselves. For example, 90% of leatherback turtle nests were poached before Marino Las Baulas National Park was established on the Costa Rican Pacific coast (Santidrian-Tomillo et al., 2008). Moreover, 202 green and 1,256 hawksbill females and clutches were poached between 2012 to 2019 along the 5 km beach of Pacuare (Costa Rican Caribbean coast), where patrol teams compete with poachers to relocate eggs to the hatchery (Pheasey et al., 2021). In addition, encounters with drug traffickers or feral dogs are further risks for patrollers, which has led to some nesting beaches being described as ‘remote and dangerous’, Moín beach on the Caribbean coast of Costa Rica for example (Smith, 2016). This beach was left unprotected after conservationist Jairo Mora was murdered by poachers while patrolling in 2013, resulting in a sharp rise in levels of egg poaching, close to 100% of nests (Fonseca et al., 2015).
However, modern technologies, such as drones or Unmanned Aerial Vehicles (UAVs), have already proven to be effective tools against poaching and surveying in remote and dangerous habitats (Hodgson et al., 2013; Mulero-Pázmány et al., 2014; Butcher et al., 2021). In South Africa, 1,215 rhinos were killed in 2014, the same year that the Game Rangers Association of Africa estimated that 1,000 rangers were murdered trying to protect wildlife in this continent (Wall, 2014; Department of Forestry, Fisheries and The Environment (DFFE), 2015). One project in South Africa conducted 3,000 flight hours over 20 months. In their first flight they arrested three people that climbed the park’s fence to kill a rhino and her calf that were close to a road. The drone camera recorded this episode and dozens more. With the ability to find poachers two kilometers from the rhinos, drone flights stopped poaching in every study area within 5-7 days (Snitch, 2015).
In addition to assisting with surveillance and keeping rangers or patrollers safer, drones can also carry out equally effective, and in some cases more accurate, population counts than traditional survey techniques (Chabot and Bird, 2015; Linchant et al., 2015; Gonzalez et al., 2016). For example, one study compared two methods to count birds in seabird colonies and showed that their drone was 43-96% more accurate than the traditional ground-based data collection method (Hodgson et al., 2018). Another study showed that UAV aerial surveys detected 26% more Nile crocodiles in a lake than ground surveys, with the ability to also obtain individuals’ length measurements (Ezat et al., 2018).
Promisingly, sea turtle projects are harnessing the power of drones, mostly using red-green-blue (RGB) cameras for diurnal sea turtle monitoring, research, and conservation efforts. Rees et al. (2018) reviewed the potential of drones to survey sea turtles and their behavior in the water, and along nesting beaches - suggesting their ability to increase surveillance. Drones can reveal adult sex ratios at breeding sites, identify individuals marked with satellite tags, and differentiate between some species by size (Schofield et al., 2017; Schofield et al., 2019). Other drone studies with sea turtles have led to greater population estimates compared with traditional observer methods (Dunstan et al., 2020). Gray et al. (2019) detected 8% more turtles than manual counts during a mass nesting event on Ostional beach, Costa Rica. However, the major limitation here is that RGB drones can typically only be used during the day, while most turtle nesting activity, the most suitable period for making counts, occurs at night (Miller, 2017).
Recently, thermal infrared (TIR) sensors mounted on drones have helped to both detect and count wildlife, and to uncover poaching activity. Such sensors detect the body heat of warm-blooded animals, or more specifically the thermal differences between animals and their surrounding environment (Hambrecht et al., 2019; Kays et al., 2019; Fust and Loos, 2020). Stander et al. (2021) utilized commercial drones with TIR systems to detect avian nests and compared results to traditional methods, showing that thermal imaging identified 77–100% of known nests. Witt et al. (2020) found that the TIR detection probability for koalas (83%) was higher than two traditional survey methods (38.9% for systematic spotlight and 4.2% for diurnal radial search). Despite this potential to utilize drones in darkness, only two sea turtle projects to date have investigated the potential of TIR technology as a conservation tool for sea turtle monitoring (Rees et al., 2018; Reischig et al., 2018; Reischig and Cordes, 2021).
Rees et al. (2018), in a broad review of the potential of unmanned aerial systems for sea turtle research and conservation, detail the ability of drones with TIR camera to detect nesting turtles at night, but only show a single case of a female nesting turtle returning to the sea post-nesting in Mexico. While in Cape Verde, one short note details how a thermal drone was used to perform 400 flights, covering approximately 30 km of high priority nesting beaches (Reischig et al., 2018; Reischig and Cordes, 2021). The authors suggest that although no arrests were made, this contributed to a decrease in poaching in the subsequent nesting season, however no data is detailed in the note. Neither of these works investigated in detail the potential of TIR drones as a monitoring tool, examined the effect of the different parameters that can influence detection ability, nor did they assess the ability to identify, or differentiate, between species tracks, or compare TIR’s efficacy to traditional surveying methods.
One location in which traditional patrol monitoring techniques are implemented across many beaches is the Osa Peninsula located in southern Pacific Costa Rica, which is home to four of the world’s seven sea turtle species nesting; the vulnerable Olive Ridley (Lepidochelys olivacea), the endangered Green turtle (Chelonia mydas), the critically endangered Hawksbill (Eretmochelys imbricata) and the vulnerable Leatherback (Dermochelys coriacea). The olive ridley is the most abundant sea turtle species in the eastern Pacific Ocean, which nests all year round, with a peak nesting season during the local rainy season from August to October, and occasionally extended into November (Pritchard, 1997; Marcovaldi, 1999; Maldonado et al., 2013; Hart et al., 2014; Dornfeld et al., 2015). The second most abundant species is the green sea turtle, which has a peak nesting season from November-March (Santidrian-Tomillo et al., 2014). Here we conduct the first standardized survey that investigates the potential for TIR-drones to survey and count nesting sea turtles, make observations on different settings of the camera and TIR-drone and compare the efficacy of drones with traditional human-led patrols. Specifically: 1) We carry out pilot flights to see how well we can detect sea turtles, general beach activity, and determine if we can differentiate between two sympatrically co-occurring species. 2) We detail observed-differences related to drone height, gimbal angle and TIR visualization mode. 3) We compare the detections from the TIR-drone with those from traditional on-the-ground patrollers. And finally, 4) We summarize our findings to provide methodological insights and identify key challenges for establishing TIR-drone monitoring protocols that could be developed and adopted for large-scale sea turtle nest monitoring programs around the world.
2 Materials and methods
2.1 Study site
This study was conducted at the Osa Conservation Campus (formerly known as Piro Biological Station; 8.40388 N, 83.33661 W) in the Osa Peninsula - Southwest Costa Rica (Figure 1). The campus comprises over 1,500 ha of lowland tropical rainforest (privately protected land) and two nesting sea turtle beaches along the Pacific coastline: 1) Piro beach - 2 km, and 2) Pejeperro beach - 4.5 km. Both beaches are highly inclined, washed over by tides that can reach 11.5 ft (according to tideschart.com), and the sand composition differs along the beaches between fine and pebbly (Ávila-Aguilar, 2015). Both beaches are divided into 100 m sectors using numbered markers for monitoring (Piro: 1-20 and Pejeperro: 1-45). On Piro beach, activity for olive ridley is 8 tracks/night during the peak season (Aug-Nov) and 3.7 tracks/night annually, for green it is 2.8 tracks/night during peak season (Nov-Mar), and 1.4 tracks/night annually. These relatively low numbers allow to easily detect each track in every day of survey. Piro beach incorporates a sea turtle hatchery and was the focal beach for the drone survey. Temperatures at the field site range 23.3-28.7°C and rainfall averages 3.584 mm yr-1, with a rainy season from June to November and a dry season from December to May (Whitworth et al., 2018). The drone study was executed during the rainy season (September-November), due to peak nesting activity.
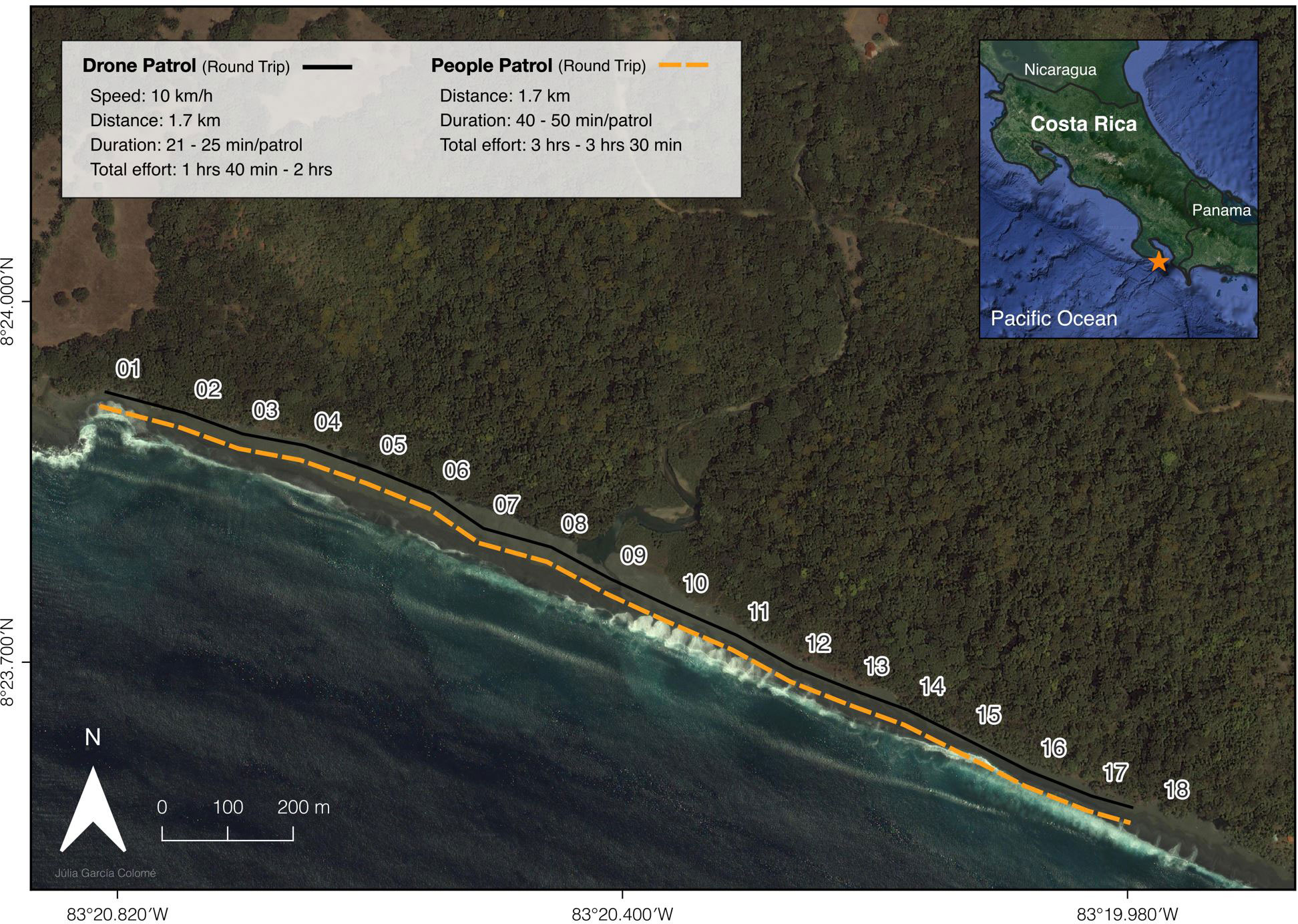
Figure 1 Map of the study beach - Piro (2 km). The 1.7 km transect (sectors 1-18) monitored by the drone identified by a black line and monitored by patrollers identified by an orange dotted line in the Osa Peninsula (identified by an orange star) in the Southwest Pacific of Costa Rica. Summary of drone and patroller efforts displayed in the white box.
2.2 Pilot drone flights
During September 2021 on Piro beach, we executed pilot drone flights across four nights (1st - 4th Sept), using a drone-mounted thermal camera (Autel Robotics EVO II Dual 8K Drone - takeoff weight is 1150 g). The goal of the pilot drone flights was to identify the ability of the drone to detect nesting sea turtles and their tracks at night by thermal imagery. From 8 pm - 4 am, two patrollers monitored an 800 m section along Piro beach, when fresh turtle tracks were encountered, the drone team (one pilot and one assistant) arrived at the patrollers’ location. The pilot launched the drone manually twenty-five meters from the sea turtle to hover above it, as the drone cannot be heard at this distance due to the loud wave noise (all videos were recorded along the twelve pilot drone flights we conducted). The drone team would observe the TIR images in real-time using the Autel Explorer App on a smartphone (Samsung A12) that was attached to the drone’s remote control. Additionally, we deployed the drone during an olive ridley nest release and over the hatchery to see if this activity could be observed by the thermal camera.
Upon confirming nesting sea turtles could be detected by the thermal drone, several variables were tested during the pilot flights to identify optimal drone configuration for sea turtle and track detection. In addition to detection, we also looked at how these variables affected the detail of information we could record, such as, identifying the different nesting stages. This included the thermal imagery mode of the drone camera, three settings were tested: 1) ‘Black Hot’, 2) ‘White Hot’, and 3) ‘Hottest’. Additionally, four camera gimbal angles were tested: 1) 90°, 2) 45°, 3) 35°, and 4) 18°. Finally, drone height was tested; the drone was flown at a starting height of 80 m and lowered by 10 m intervals going as low as 10 m. From 10 m and while the sea turtle was laying eggs, we continued decreasing the height slowly to identify the lowest height the drone could be flown without disturbing the turtle, as artificial lights can reduce nesting probability (Silva et al., 2017). When the landing lights were automatically triggered we would stop lowering the drone and record the height. This was the only time we flew the drone lower than 50 m.
2.3 Experimental surveys - Drone flights vs. sea turtle patrollers
Using the findings from the pilot drone flights, we established an experimental survey to compare the detections generated by two monitoring methods: TIR-drone flights versus traditional on-the-ground patrols, along the same stretch of beach over the same nights. We executed the experimental survey on Piro beach along a 1.7 km transect (sector 1-18; Figure 1), programmed by the Autel Explorer App. Only 1.7 km of the 2 km beach was surveyed to ensure that the transect could be completed and our drone would return to the start point home with at least 20% battery power for a safe manual landing. We executed the drone flights at a speed of 10 km/h (flight speed would vary between 10-11 km/h due to wind speed during flights) and a height of 50 m for enhanced detectability and to clear coastal forest vegetation. Based on the preliminary flight results, the TIR output was set to ‘Black Hot’ mode as this gave us the clearest images. In this thermal sensor mode, warm objects are depicted darker than cool objects.
During October-November 2021, we performed the experimental surveys across seven nights (19th - 20th Oct; 4th - 5th, 10th - 12th Nov). On each of these nights, the drone was flown over Piro beach every 1 - 1.5 hours from 8:30 pm - 3:30 am depending on the tides. Patrollers started their patrol simultaneously, as the drone was launched, walking the same transect. Groups of 2-3 people (trained and experienced in sea turtle monitoring and patrolling) executed the traditional sea turtle patrols walking along the latest high tide line, looking for tracks/nests and nesting females (Eckert et al., 1999). Upon finding a track/nest or nesting sea turtle, the patrol team collected nesting data (the sea turtle’s behavior, time of sighting, beach sector, and GPS location). The drone team and the patroller team did not communicate during the surveys to avoid any bias in comparative results.
The following day, drone footage from the previous night was analyzed by sea turtle biologist - BS-R. We reviewed the 30 videos collected (approximately 20-25 minutes long) that took 40-50 minutes each to analyze. Every time a detection was recorded, the video frame number and the time were recorded. Detections were divided into seven categories and were used to identify and compare detections from the drone flights and people patrols: i) sea turtle, ii) sea turtle and track, iii) track and/or fresh nest, iv) other wildlife (including moving animals along the beach or in the forest, birds perching on trees, but not bats), v) animals predating or animals close to predated nests, vi) people (non-patrollers), and vii) unidentified. When a track was re-identified in later videos at the same night or when a track was re-identified in the following nights, we compared the videos and if the track was the same, it was labeled as a recapture, so that only the first occurrence of a track was considered a detection to avoid duplicating results. For analysis we combined categories i)-iii) into a single category labeled ‘turtle activity’.
2.4 Experimental surveys - Covariate variables
Daily total rainfall (mm) and air temperature (maximum, minimum, and mean - °C) before and during patrols were measured using the Osa Conservation Campus weather station. Moonlight percentage and tides were obtained from moongiant.com and tideschart.com (Supplementary Table 1). In 2022, during normal patrol efforts, upon finding a sea turtle and/or track, temperature of the turtle, track and the sand (in two different beach zones; dry and recently washed over sand) were taken using the Etekcity Infrared Thermometer. This data was collected to monitor variables that have been proven to cause variation in sea turtle activity (Pike, 2008; Nakamura et al., 2019), but due to the short survey period we did not include this data in our analysis.
2.5 Data analysis
To identify differences in the number of detections by our thermal drone and the patrollers, we performed two paired t-tests using the R Studio software (R Core Team, 2022). One to observe differences in the number of nesting activity detections (sea turtle and/or tracks) and another for the total number of detections.
3 Results
3.1 Pilot drone flights
The pilot drone flights verified that sea turtles and their tracks can be reliably detected and distinguished between species (olive ridley and green) with a TIR-drone. Our flights determined the optimum flight height, camera angle, and TIR visualization mode for the study site beach. Moreover, the drone could detect people and other wildlife, both on the beach and in the vegetation. We detail the specifics of each of these results below.
3.1.1 Detecting sea turtles, their tracks, and other wildlife
Eight adult olive ridley turtles and one green sea turtle were detected via thermal imagery (Figures 2A–C). The TIR camera footage was clear enough to identify turtle species not only by body size and shape but also by track characteristics (Figures 2D, E). Green turtle tracks were identified by their characteristic symmetric front flipper marks and it was also viable to determine the direction of these tracks, coming up the beach toward the vegetation and down the beach toward the ocean. However, for olive ridley turtles their asymmetrical flipper marks are shallower and more uniform, and so the directionally up or down the beach could not be clearly determined.
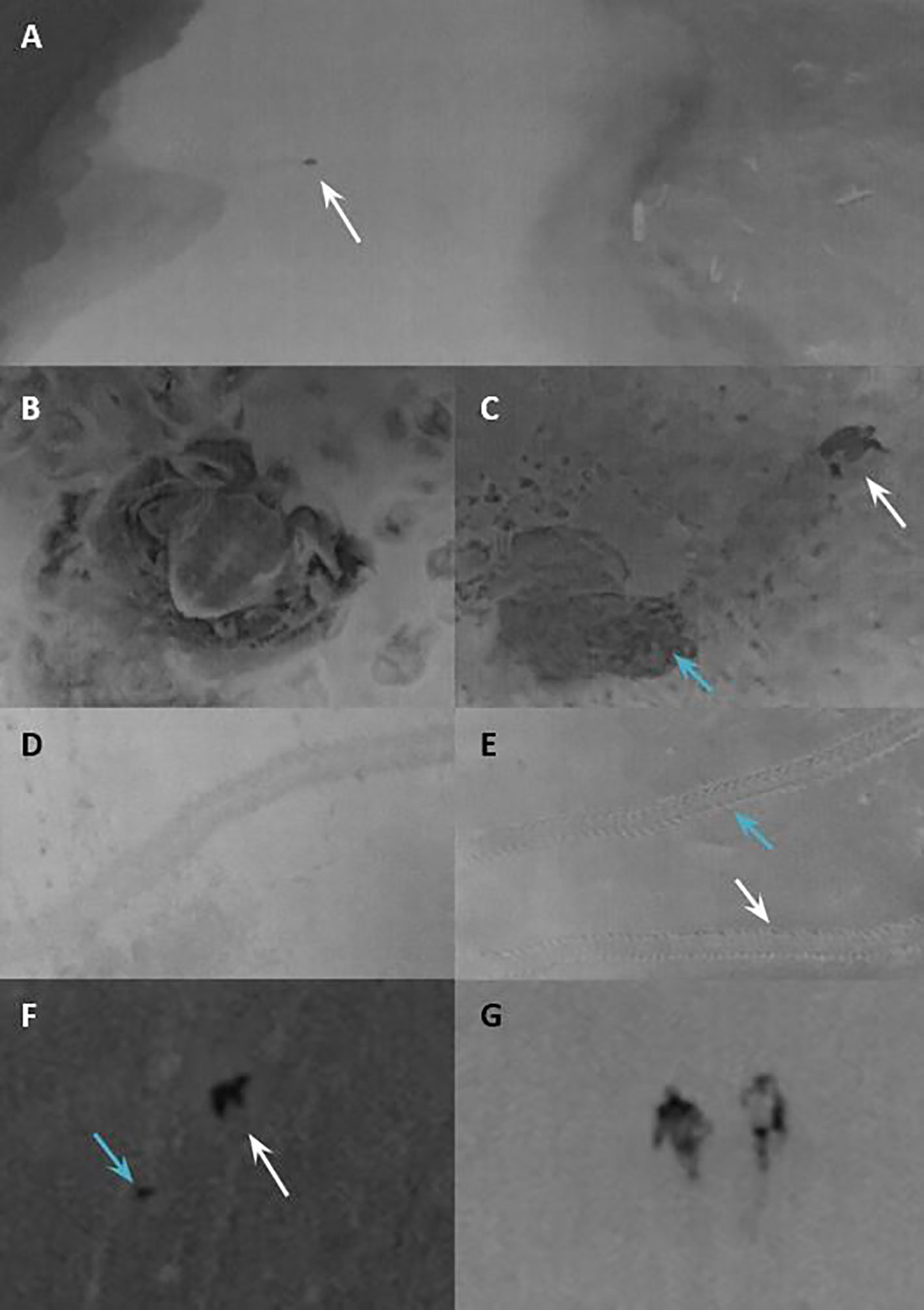
Figure 2 Still captures from the video imagery of the TIR-drone of (A) olive ridley turtle emerging from the sea, from a drone height of 60 m, (B) olive ridley camouflaging its nest, from a height of 4 m, (C) olive ridley turtle returning to the sea after nesting (blue arrow identifying its nest and white arrow identifying the turtle), from a drone height of 15 m, (D) olive ridley sea turtle track, (E) green sea turtle track with the front flipper marks that line up in pairs (blue arrow identifying the downward track and a white arrow identifying the upward track), (F) animal walking on the beach (identified by a white arrow) after predating a turtle nest (identified by a blue arrow), (G) two sea turtle team members patrolling the beach. Photos (D–G) were zoomed in.
When deploying the drone during an olive ridley nest release, hatchlings were visible as small gray spots moving towards the sea. The released hatchlings including crabs chasing hatchlings were identifiable from a height of seven meters, but not higher (Supplementary Video 1). Speeding up the videos enhanced the hatchlings’ detection. The thermal imaging from above the hatchery showed very dark signatures of temperature from the hatched nests (post-excavation). Warm-blooded animals were also identified using the drone’s thermal camera. We could observe animals predating turtle nests (Figure 2F) and some predated nests with no predator nearby. Flying bats were detected and identified by their rapid flight movement. Although no poacher was detected during the pilot flights, the TIR camera demonstrated that humans could be easily identified at all the tested heights (Figure 2G).
3.1.2 Selecting TIR imaging mode
Using the ‘Black Hot’ TIR camera mode, warm objects appear as darker shades of gray, and cooler objects appear lighter (Figure 3A). ‘White Hot’ is an inverted version of the ‘Black Hot’ mode, with cool objects on the black end of the scale (Figure 3B). We also tried a colored mode compared to the ‘White Hot’ and ‘Black Hot’ images of the same scene, where orange marks highlighted the warmest points (white arrow in Figure 3C). Differences in sand color were due to differences in temperature along the beach’s width. After trialing these three modes, we selected the ‘Black Hot’ mode for the remaining flights as it appeared to be the clearest to detect features.
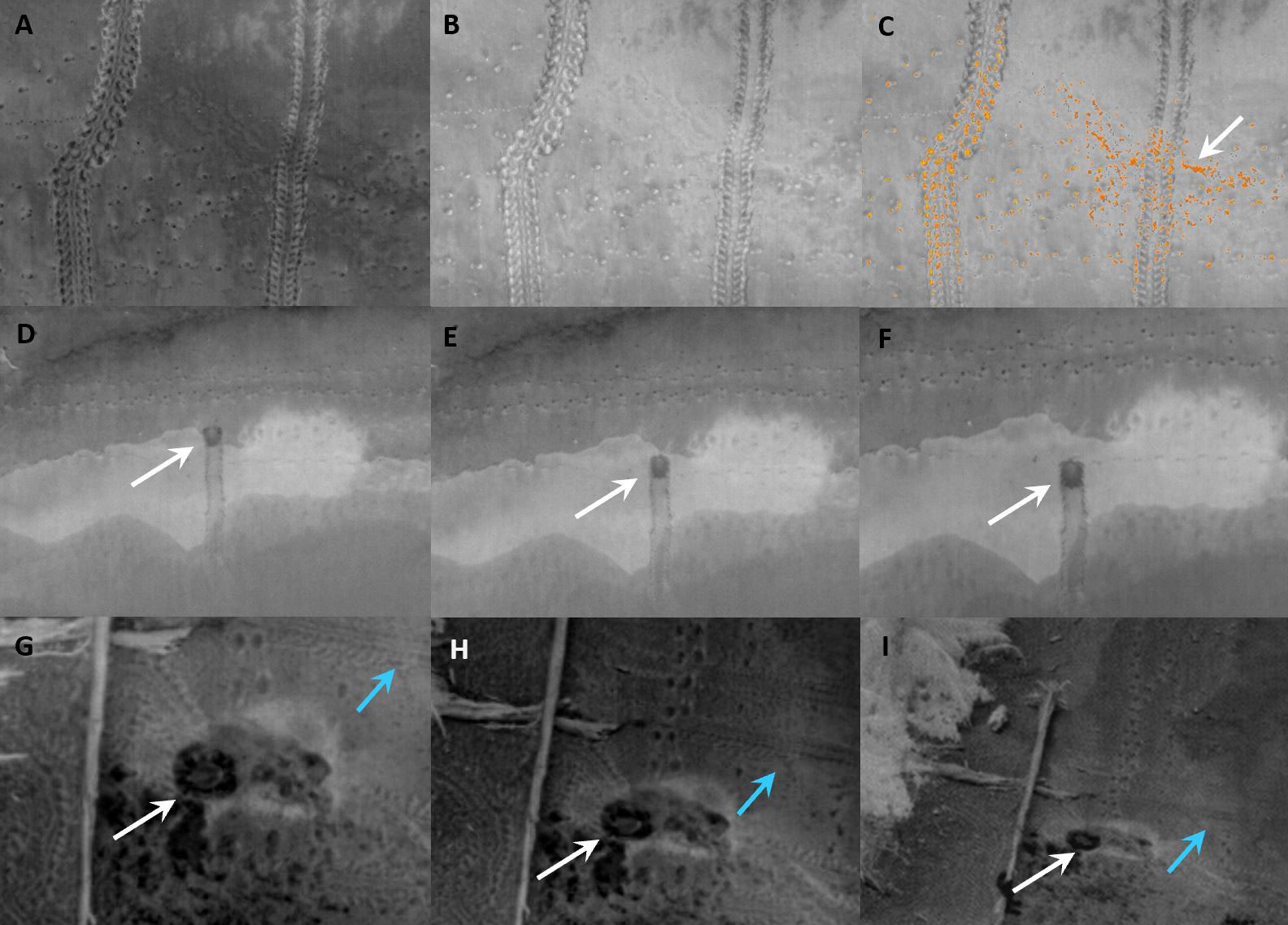
Figure 3 Testing different parameters that might affect detection rates. Different thermal imagery modes to detect the same green sea turtle track: (A) ‘Black Hot’, (B) ‘White Hot’, and (C) ‘Hottest’ with a white arrow showing the warmest sand). Green sea turtle (white arrow) emerging from the sea taken from different heights: (D) 70 m, (E) 60 m, and (F) 50 m. Olive ridley sea turtle (white arrow) covering her nest at a height of 50 m and different camera gimbal angles: (G) 90°, (H) 45°, and (I) 18°, with blue arrows pointing to an old green turtle track.
3.1.3 Testing drone flight heights
With the drone camera facing straight down (90°) and a flight height of 70 m and 80 m, the drone camera frame covers the entire beach, from the sea to the vegetation line, in most sectors. At these heights, the drone can distinguish between a traveling and a nesting sea turtle, however it cannot distinguish the sea turtle nesting phases (body pit creation, chamber digging, laying eggs, covering, and camouflaging). Flying at a lower height of 60 m, no trees were in the drone’s trajectory, but identifying nesting phases was still challenging. At a height of 50 m, we could identify nesting phases, however, the camera frame could not cover the whole beach in some sectors, where the beach width differs (Figures 3D–F).
At 50 m, we could detect sea turtle species of track and we could also distinguish the turtle track direction. The drone is inaudible for humans at heights over 50 m, and no turtles appeared to be disturbed. However, the small LED navigation lights (green and red) were visible in the sky during the whole flight. On three occasions, the videos showed the emerging or returning turtle stopping as the drone passed by. We also determined that the lowest height for flying above a turtle before the automatic white landing lights would turn on was 4 m, and it seemed that even at this height the nesting turtles showed little to no clear signs of disturbance (Supplementary Video 2). We concluded that our optimum flight height for the experimental surveys at this study site is 50 m to ensure detailed detections, although on a wider beach with very low tides, 60 m might be a more optimum flight height.
3.1.4 Testing camera gimbal angles
Positioning the drone camera at 90° enabled easier detection of sea turtles, their tracks, or other animals, which appeared the biggest in size (Figure 3G). There is a trade-off between vertical and near horizontal camera angles. Monitoring at 90°, we get the most information of an event but only for a relatively short time. Changing the camera angle to near horizontal - 18°, gives us longer observation time but less detailed information (Supplementary Video 3). For example, the sea turtle track identified by the blue arrow in Figures 3G–I was easily visible at every angle except from 18°. At 45°, the sea turtle reduced in size compared to flying the drone at 90°, although the difference was not as significant from the turtle size in the image at 18°. Therefore, the optimal camera angle is between 45° and 35° down from horizontal for sea turtle monitoring.
3.2 Experimental surveys - Drone flights vs. sea turtle patrollers
During 30 flights across seven nights the drone identified 60 sea turtle nesting activities, 25 wildlife, 14 predators, and three humans (who were not patrollers). During 23 patrols the patrollers identified 48 sea turtle nesting activities, but did not identify any other wildlife, predators or humans (Figure 4; Supplementary Video 4.1 and Video 4.2). Our TIR drone observed significantly more detections (sea turtle activity, predators, other wildlife and humans) than the patrollers (14.6 ± 6.16 vs. 6.86 ± 3.85; t(6) = 6.0883, p < 0.005). Specifically, the drone performed better than patrollers for detecting sea turtle nesting activity (8.57 ± 4.31 vs. 6.86 ± 3.85; t(6) = 4.7683, p < 0.005). See Supplementary Table 2 for detailed detections per night and per flight.
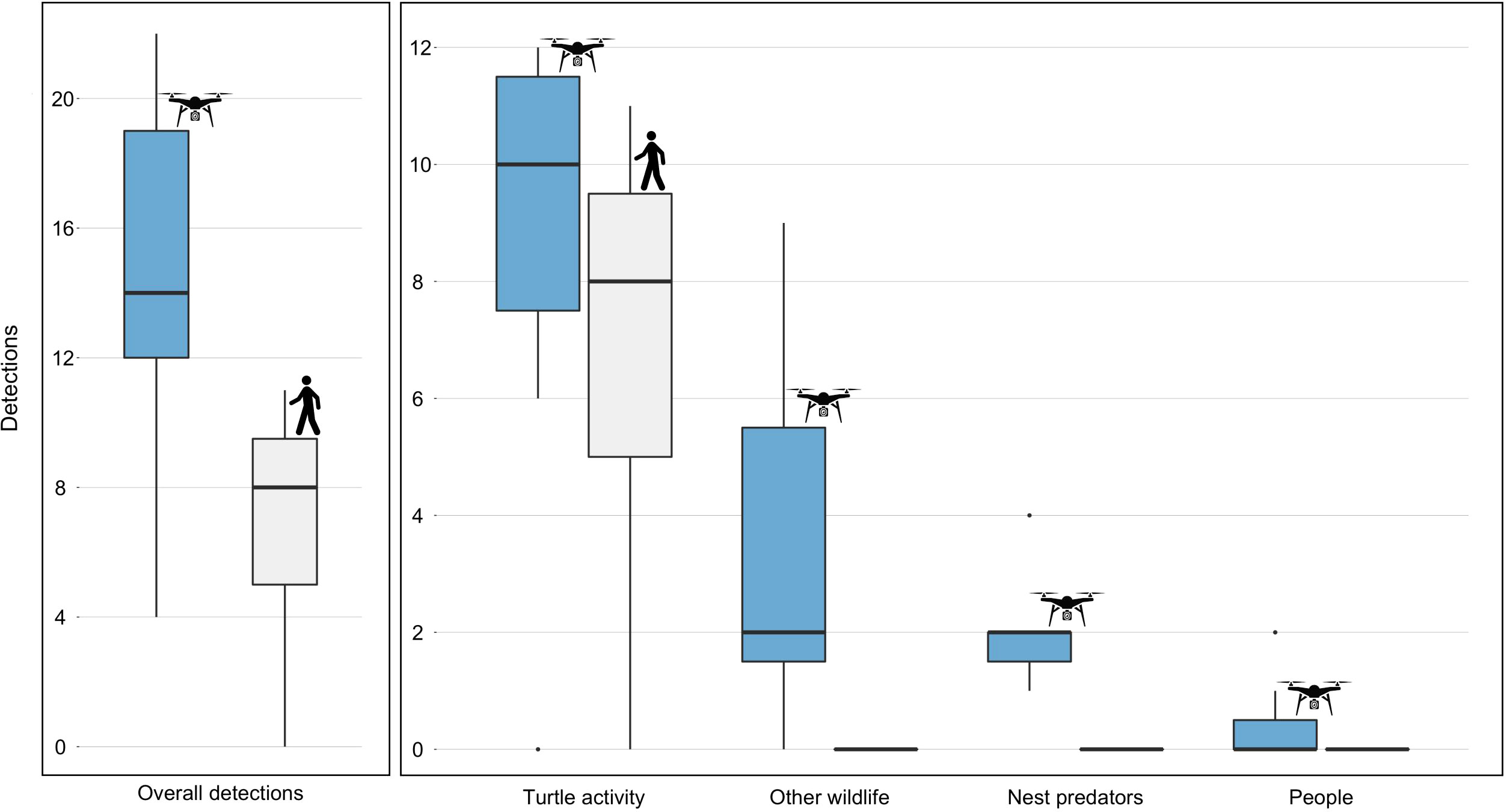
Figure 4 Comparison of the number of detections by our thermal camera drone (blue) and the patrollers (gray) across the seven experimental nights. Turtle activity includes any detected sea turtle or track. In the left panel we summarize the number of total observations from the four categories. Note that y-axises are differently scaled.
The drone videos showed that patrollers walked very close to the three humans and the animals, but they could only record the footprints of each - and did not directly encounter them. However, the drone was unable to identify the detected animals to species level.
3.2.1 Challenges detecting sea turtle tracks
During our study, several tracks (the whole track or a section of) were difficult or impossible to detect from the thermal image (Supplementary Video 5). This was due to the area of the beach being washed over by the tides creating perpendicular marks to the seashore that appeared darker with the thermal camera and could be confused with turtle tracks. Additionally, the sand closer to the sea was darker than the sand closer to the vegetation, as this lower sand retains the constant heat from the warm waves, which also made track ID difficult. The closest section to the vegetation is never washed over by the tides so stays warm at night (Figure 5). Therefore, tracks were easier detected in the middle section of the beach. These results are supported by the temperature values on Supplementary Table 3.
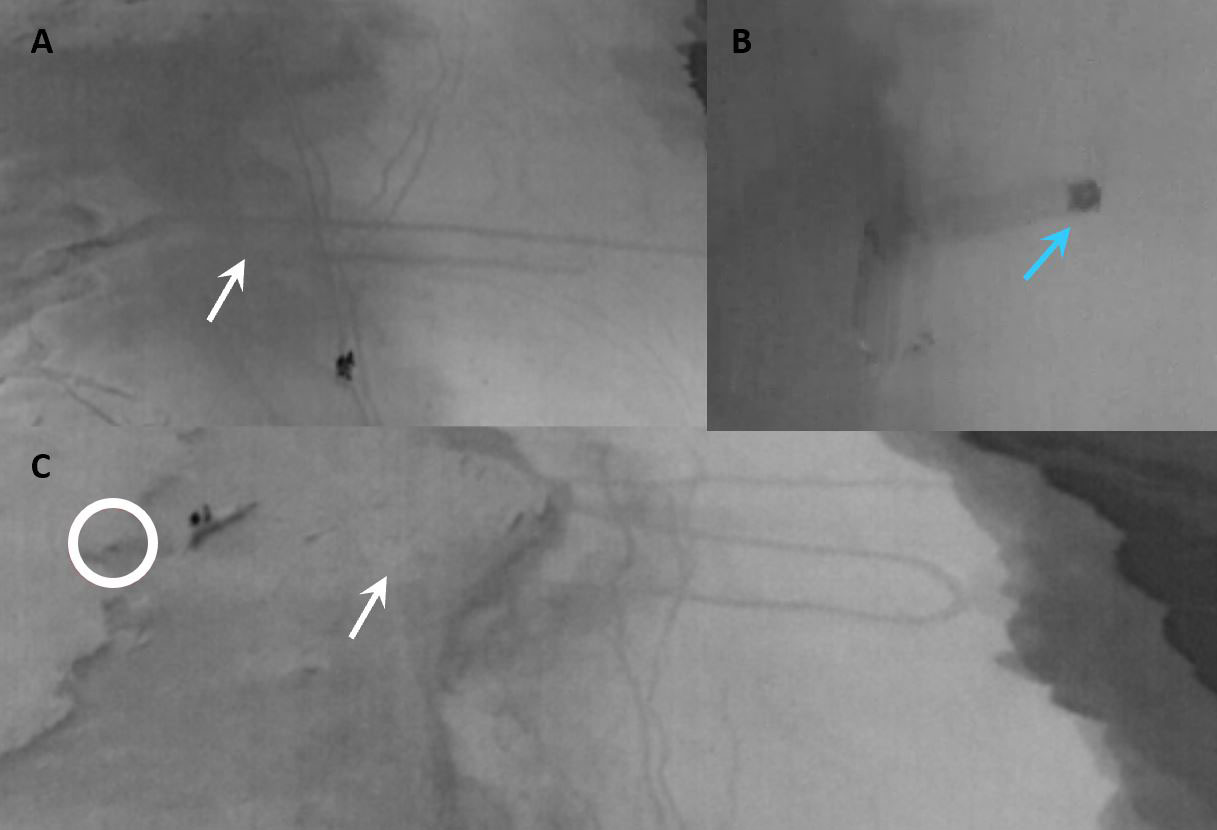
Figure 5 Some tracks disappear depending on the beach zone, making their detection challenging. In photos (A–C), white arrows point to where the turtle tracks are not visible using the thermal camera. Blue arrow in (B) points to an olive ridley turtle returning to the sea. The circle in photo (C) is around a nesting green sea turtle monitored by the patrollers. The vegetation in the three photos is on the left and the sea on the right.
4 Discussion
We show that in addition to being able to detect sea turtles at night on nesting beaches, it is also possible to differentiate between sea turtle species tracks. The TIR-mounted drone and observer outperformed traditional ground-based patrollers, with 20% more sea turtle activity detections. Additionally, the drone and observer detected other wildlife and poachers on the beach, all of which remained undetected by traditional patrollers. We tested different methodological factors related to drone settings, including camera visualization mode, height, and gimbal angle, all of which appear to affect detections, and the ability to distinguish tracks. We discuss each of our findings below in detail, related to both advantages and challenges, and highlight factors that need to be assessed more broadly so that TIR-mounted drones might be adopted to contribute to global monitoring efforts, research and protection of sea turtle nesting beaches.
Although Piro beach is not a ‘hot spot’ known for high levels of poaching, our drone experimental surveys were still able to detect unidentified people that remained hidden in the dark and vegetation from our human patrollers. As the drone flies along the coastline, although it cannot be heard above the sound of the waves, the multiple lights that indicate orientation are clearly visible, moving up and down the beach. As stated by Reischig et al. (2018), it is quite conceivable that the sight of the drone patrolling back and forth along the beach line could serve as an effective deterrent to discourage poachers, or other illicit activities. However, they also state that in the absence of consequences in terms of capture and prosecution, then reductions in illicit activities might be short lived, as perpetrators realize there are no consequences associated with the drones presence. As such, projects attempting to utilize TIR drone technology to tackle poaching along nesting beaches, would be most impactful working alongside local authorities to ensure that actions result in response to the drone detections, as has been achieved with great efficacy to tackle rhino poaching in South Africa (see Snitch, 2015).
One concern that we had in relation to using drone and TIR technology for nesting sea turtles was the ability to determine between different sea turtle species - not a problem for beaches frequented by single species. Our study shows for the first time that thermal drones can identify tracks to species level - at least when the most abundant species differ in their track shape. In this case, the olive ridley and green sea turtles, whose individual tracks are asymmetric and symmetric, respectively (Barquero-Edge, 2013). There are occasionally two other species that use Piro beach, albeit much more infrequently, the hawksbill and the leatherback. We did not appear to identify either within this study, which is not unexpected given that less than two to three leatherback nests are identified by the survey team each year, and fewer than 10 hawksbill nests. We are confident that a leatherback would have been easily distinguishable given the substantial difference in size from all other species, while hawksbill tracks are asymmetrical, like the olive ridley turtles, and might be indistinguishable (Rees and Baker, 2006). The imagery was also sufficiently detailed to determine directionality in symmetric tracks by the front flipper marks, as green sea turtles cut deeper into the sand (Pritchard and Mortimer, 1999), helping to identify individuals that could still be on the beach.
The ability to detect feral dogs and other wildlife, especially potential nest predators, such as racoons and coatis, could be useful in quantifying nest predation levels. These are almost never directly observed at night by human observers, and only the remnants of predated or partially predated nests are found. This is why most projects rely on animal track observation, scat analysis, and camera traps to identify the nest predator (Blamires, 2004; Lei and Booth, 2017). While we could see that some of these animals moved somewhat differently and showed some differences in size, we did not feel confident in being able to determine observations to species level. However, flying at lower heights than 50 m, or the use of a higher resolution TIR camera could likely help to determine the exact type of nest predator (Kays et al., 2019). This might be extremely useful in better understanding nest predation, and whether an intervention or control of such predators is necessary. Piro beach for example between the years 2015-2017 has displayed predation levels of natural nests of upwards of 27.6% (Ossmann, 2019). This is a far more concerning threat than poaching for this specific beach. The idea of predator control could be controversial, but if the nesting turtle species are of conservation concern while identified nest predators are common, least concern species, or feral domestic cats or dogs, then this tool might be useful in identifying what exactly the predators are, or in locating nests in process of being predated and deterring predators. Partially predated nests identified in real-time could also be quickly located and re-covered before further scavengers or insect infestations have the opportunity to spoil the rest of the nests (Fowler, 1979; Leighton et al., 2011).
The TIR drone outperformed traditional patrollers in detecting sea turtles and tracks across the seven nights by ~20%. One potential reason might explain this relates to a case where two sea turtles with no visible tracks were observed. This is because sea turtles can emerge and instantaneously return to the sea without leaving the shallows and also because tracks or nests can be quickly washed over by the tides. As traditional sea turtle monitoring protocols recommend that patrollers walk along the latest high tide line (Eckert et al., 1999), these events can often be missed by patrollers. Our TIR drone pilot recorded these turtles and tracks that patrollers could not see - providing more complete nesting activity counts, the cornerstone of any sea turtle monitoring program.
Despite some apparent advantages and the potential of TIR drones to enhance sea turtle monitoring programs there are also limitations, and advantages to having human observers on the ground. For example, one important aspect of monitoring is assessing turtle body condition through visual examination (Heithaus et al., 2007), an approach where humans likely have an advantage over a drone. There might however be ways in which videos of nesting females could be analyzed to assess nesting times, take temperature readings from the turtles, and assess nesting movements of females during the nesting process, that might give indications towards animal health and condition (Jorquera-Chavez et al., 2019; Ramos et al., 2022). Although during our standardized patrols we used pre-programmed flights for transects to make counts, the ability to take manual control, as we did in some of the pilot flights, is a valuable approach. Manual flights allow to gather this more specific information for maybe verifying an uncertain identification by flying lower, to gather temperature related data, or in being able to maintain visual information on other subjects, like predators or poachers. Further work to corroborate some form of agreement between the health assessments by human observers with data collected by drones, for the same individuals, might elucidate the viability for drones to gather this type of health-related data.
Another great value provided by traditional patrollers however, that likely wouldn’t be easily replaced by drones, is the ability to actively tag and mark individual turtles. This provides projects the ability to assess the return rate of adult females, and assess the fidelity of females, as marked individuals are recorded by different sea turtle programs under standardized international process. Although drones might never be successful in tagging turtles as human observers, they could still be adapted to detect the tags of marked individuals and be able to gather individual recognition of tagged females. For example, Mesquita et al. (2022) showed that drones could detect moving tracking devices in simulated target animals with a success of 90% in open savannah environments, and even 30% in forested habitat.
Additionally, if sea turtle programs only used TIR or RGB camera drones for daily monitoring, differentiating between new tracks and those from previous nights would be challenging. Especially if surveys were carried out on consecutive nights with high sea turtle nesting activity and a lack of rain to wash away old tracks. This could contribute to a biased, duplicated number of track detections by drones, whereas traditional patrollers rake out new turtle tracks on the sand to discern from old and new crawls to avoid double-counting (Eckert et al., 1999). Machine learning processing of video imagery however, might be useful to discern pre-counted tracks from standardized locations in flights from previous nights.
There are of course many other basic facets of drone application that need to be tested before standardized approaches might be adopted for sea turtle programs. For example, within our pilot flights we noticed how drone height, gimbal and angle and flight speed can each affect detection ability. Although we didn’t carry out intensive standardized approaches here to determine the most appropriate settings, we did carry out test flights to determine the most appropriate settings for our beach. For aerial surveys with aircrafts, one sea turtle manual suggests flying at 60 m to survey loggerhead turtles in the USA and 250 m for leatherbacks in Mexico (Eckert et al., 1999). This manual mentions that with lower aircraft altitudes, objects remain in the field of view for a shorter time, resulting in higher percent error for detection. By contrast, increasing flight height increases the field of view but lowers the resolution of the image and gives us lower detection probabilities (Hambrecht et al., 2019). For our study we found that 50 m above ground was the ideal height to better detect olive ridley turtles and their tracks, wildlife, and poachers.
For studies involving drones and wildlife, measuring the level of disturbance should be a priority, although they are scarce (Smith et al., 2016). It is well known that sea turtles are more susceptible to being disturbed prior to nesting (Witherington, 1992). For example, the olive ridley turtle that we filmed at a drone height of 4 m was laying and camouflaging and showed no sign of disturbance and proceeded to nest successfully. Although some sea turtles stopped walking while the drone was flying, we cannot attribute this behavior to the drone sound or orientation lights. At 50 m height the drone sound was almost imperceivable to the human observers directly below due to the strong sound of the ocean. A study by Bevan et al. (2018) tested nesting sea turtle disturbance by drones at altitudes of 10-30 m and none flatback sea turtles were found to display avoidance behavior. Disturbance and avoidance should be tested by sea turtle programs for different sea turtle species and under different environmental conditions. It is also important to note that drone pilots and researchers need to consider the presence of other possible drone-sensitive animals and not only the target species (Raoult et al., 2020). For example, sea turtles may show no clear sign of disturbance with a flying height of 50 m, while in some circumstances nesting birds have displayed signs of disturbance at drone heights below 60 m (Bevan et al., 2018). To the best of our knowledge, bird attack reports on drones remain scarce (Lyons et al., 2018; Gallego and Sarasola, 2021) and no study has showcased drones falling on animals due to malfunctioning. Although failure rate for drones (expressed in flight hours) is estimated to be 1/1000 (Petritoli et al., 2018), with certified pilots and preventive and corrective maintenance measures, we could optimize safety and performance levels.
In terms of gimbal angle the camera pointing 90 degrees straight down might be the optimal angle for beaches with high nesting turtle density (such as the arribadas on Ostional in Costa Rica), where RGB drones have proven useful to make accurate nesting counts during the day (Gray et al., 2019). However, sea turtle nesting behaviors change across species; olive ridley turtles nest in open areas halfway up the beach, while green and hawksbill turtles nest closer to or in the vegetation (Whitmore and Dutton, 1985; López-Castro et al., 2004). In such cases, using inclined angles, as we did, allows for a wider field of view and enables the observer to see tracks all the way to the turtle path’s end - allowing observers to differentiate between a false crawl and a nest or nest attempt. Poachers usually hide in the vegetation, where the canopy can reduce or block the emitted radiation if the camera is facing straight down. Oblique angles like 18° below horizontal increase the probability of viewing sea turtles, poachers, or wildlife under trees. So, a standard camera angle of 35° up from vertical for object detection from the shore to the vegetation might work as a standard compromise to suit both cases.
These kinds of factors need to be tested across a number of beaches that likely vary in physical conditions, like temperature, which changes between regional climate, and general beach variability; such as width, inclination, sand color and grain size (Speakman et al., 1998; Wood and Bjorndal, 2000; Salleh et al., 2018). For example, darker sand beaches like Piro absorb more heat during the day than lighter sand (Hewitt, 2022), therefore we might expect that tracks are better detected in white sand beaches. During our flights we observed that the sand temperature can be the same, or very close to the turtle track temperature, resulting in reduced ability to detect tracks. For easier detection, the turtle track temperature needs to be different enough than the surrounding sand, which sometimes does not happen in the upper section of the beach. Higher TIR camera resolution and sensitivity could significantly increase animal detectability (Burke et al., 2019), and therefore enable the detection of smaller variations in temperature along the beach’s width. We suggest that sand and sea turtle temperatures should be considered for future long-term studies using TIR- drones for beach and sea turtle monitoring. This might be especially useful for understanding seasonal effects on detectability, understanding detectability between survey location, and for tracking annual variations in environmental conditions, even longer-term effects associated with climate change.
We also noticed during post-processing that TIR images revealed that the ability of detection could be influenced by humidity fogging up the camera lens. Some footage was more challenging to review as a result. Another study found fog to be a major issue for detection with aerial aircrafts like drones (Burke et al., 2019). Nano-coated lenses and regular lens cleaning between flights could help to tackle this in humid, rainy conditions. Additionally, as a remote beach, there is no light pollution on Piro beach and all light is starlight or moon light. In our study we did not test the effects of the moon illumination on detection, while according to Stander et al. (2021), lunar illumination can contribute to false positives when trying to detect bird nests. Until these kinds of factors are better understood, general standardized recommendations and practices for TIR drones to be applied across regions will be challenging to make.
In addition to providing complementary new approaches that can improve wildlife count accuracy, increase data gathering efforts and be used in potentially dangerous locations, conservation technology applications often aim to enhance efficiency and reduce associated monitoring costs (Linchant et al., 2015; Hodgson et al., 2018; Stephenson, 2019). Sea turtle programs usually rely on medium to large sized teams that are trained several days to handle the arduous field work of hiking up to 8 hours each night, resulting in high finance costs to cover living expenses. A thermal drone survey only requires two people. However, our study required significant time in the post-processing of videos. To process 24 minutes of footage the reviewer took 40-45 minutes, and so cumulatively, time and effort is comparable. To mitigate this arduous task, one other study used an application called ‘SPOT’ to automatically detect animals and poachers in near real time, performing better than EyeSpy, the world’s best object recognition mobile app (Bondi et al., 2018). With machine learning algorithms, models could be developed to identify tracks, sea turtles, predators and humans, significantly reducing the time spent in reviewing thermal footage. Moreover, during the pre-programmed flights we could only monitor 1.7 km of Piro beach in 24 minutes. As a proof-of-concept pilot study, during these flights we aimed to compare survey methods, but to apply aerial surveys on nesting beaches longer than Piro, the battery capacity would be a limiting factor. With two pilots at both ends of a beach and spare batteries, one could fly in one direction, land the drone, exchange the battery and fly back to the original starting point, thereby increasing the monitored area.
It is also worth mentioning that the investment in hardware, software and training could also be a challenge for many sea turtle projects. The cost of the commercially available off-the-shelf TIR drone used in the study for example, at the time of purchase was ~$10,000, a major barrier for many programs. RGB sensor drones are often more affordable, such as the DJI Mavic Air 2, which could incorporate a LED searchlight, although based on this spotlight characteristic and our established optimal height of 50 m, we would not expect it to illuminate the entire beach width. With more powerful lights and consequently increased carrying weight, the drone flight stability and batteries would be affected. In any case, if drone-mounted searchlights can illuminate the beach width, they must always be covered by a color filter red to prevent disturbance to sea turtles or be of a sensitivity level high enough that moonlight, or relatively soft floodlights, would be sufficient to gather detailed imagery. Further research would need to test if drones with RGB sensors could actually replace the TIR sensors in sea turtle nocturnal monitoring at a lower cost. However, a trained and certified drone pilot is essential. Such costs will likely reduce as technological advances continue and competitive models become available.
5 Conclusions
We find that the potential application of TIR drones for global sea turtle monitoring and research shows major promise in terms of its comparability in performance with traditional observers. There are many attractive potential developments that could allow TIR drones to provide data currently collected by traditional techniques, to provide new information that traditional surveys cannot provide, and with the right advancements in machine learning, can improve on the efficiency of data collection. The application for beaches that are dangerous to human patrollers is extremely attractive, especially if carried out with local enforcement authorities to help tackle illicit activities that impact threatened species, or along those beaches where it is challenging to gather data on populations because of the safety risk to patrollers (such as illicit narco activity), even if the turtles themselves are not necessarily at risk. However, the application is in early stages, and far more testing to understand regional condition variations is necessary, and enhancements in post- or real-time data processing are essential. Even then, we see that TIR drones will likely be best applied as a complementary tool to enhance traditional survey programs, as opposed to a complete replacement for human observers.
Data availability statement
The data that support the findings of this study are available upon request to authors, Bárbara Sellés Ríos and Dr. Andrew Whitworth, and meet requirements and permissions of Osa Conservation.
Ethics statement
Sea turtle monitoring is performed in accordance with the permit (research permit number: SINAC-ACOSA-DASP-PI-R-020-2021) granted by the National System of Conservation Areas (SINAC), part of the Ministry of Environment and Energy (MINAE) of Costa Rica. Osa Conservation’s Internal Science Committee approved the ethics associated with the methodology of this study.
Author contributions
BS-R, EF, and AW conceived and designed the experiments. JO-G and BS-R performed the drone experiments and surveys. BS-R, JG-C, and OL performed data analyses. BS-R, EF, and AW wrote the manuscript. All authors contributed to the article and approved the submitted version.
Funding
This work was supported by the Gordon and Betty Moore Foundation, and the International Conservation Fund for Canada.
Acknowledgments
Thanks to the Gordon and Betty Moore Foundation, Marisla Foundation, the International Conservation Fund of Canada, and the Troper Wojcicki Foundation for supporting this research, advancing the use of conservation technologies, and for their support of conservation in the Osa Peninsula. Thank you to the Derald H. Ruttenberg Foundation for supporting sea turtle monitoring and conservation efforts in the Osa. Thank you to Osa Conservation volunteers and interns for assisting with data collection in the field, with a special thanks to Frank Uhlig for reviewing the manuscript. Finally, we thank the reviewers and the handling editor for their time and comments.
Conflict of interest
The authors declare that the research was conducted in the absence of any commercial or financial relationships that could be construed as a potential conflict of interest.
Publisher’s note
All claims expressed in this article are solely those of the authors and do not necessarily represent those of their affiliated organizations, or those of the publisher, the editors and the reviewers. Any product that may be evaluated in this article, or claim that may be made by its manufacturer, is not guaranteed or endorsed by the publisher.
Supplementary material
The Supplementary Material for this article can be found online at: https://www.frontiersin.org/articles/10.3389/fcosc.2022.954791/full#supplementary-material
References
Ávila-Aguilar A. (2015). Nest-site selection of lepidochelys olivacea (Testudines: Cheloniidae) in the south pacific region of Costa Rica. Rev. Biol. Trop. 63, 375–381. doi: 10.15517/rbt.v63i1.23116
Barquero-Edge P. (2013). Trends in marine turtle nesting and egg predation on the osa peninsula, Costa Rica. Mar. Turt. Newsl. 138, 7–10.
Bevan E., Whiting S., Tucker T., Guinea M., Raith A., Douglas R. (2018). Measuring behavioral responses of sea turtles, saltwater crocodiles, and crested terns to drone disturbance to define ethical operating thresholds. PloS One 13 (3), e0194460. doi: 10.1371/journal.pone.0194460
Blamires S. J. (2004). Habitat preferences of coastal goannas (Varanus panoptes): are they exploiters of sea turtle nests at fog bay, Australia? Copeia 2004 (2), 370–377. doi: 10.1643/CH-03-016R1
Bondi E., Kapoor A., Dey D., Piavis J., Shah S., Hannaford R., et al. (2018). “’,” in Proceedings of the 27th International Joint Conference on Artificial Intelligence. 5814–5816 (Stockholm, Sweden: AAAI Press).
Burke C., Rashman M., Wich S., Symons A., Theron C., Longmore S. (2019). Optimizing observing strategies for monitoring animals using drone-mounted thermal infrared cameras. Int. J. Remote Sens. 40 (2), 439–467. doi: 10.1080/01431161.2018.1558372
Butcher P. A., Colefax A. P., Gorkin R. A., Kajiura S. M., López N. A., Mourier J., et al. (2021). The drone revolution of shark science: a review. Drones 5 (1), 8. doi: 10.3390/drones5010008
Chabot D., Bird D. M. (2015). Wildlife research and management methods in the 21st century: Where do unmanned aircraft fit in? J. Unmanned Veh. Syst. 3 (4), 137–155. doi: 10.1139/juvs-2015-0021
Chacón D., Sánchez J., Calvo J. J., Ash J. (2007). Manual para el manejo y la conservación de las tortugas marinas en Costa rica; con énfasis en la operación de proyectos en playa y viveros (San José, Costa Rica: Sistema Nacional de Áreas de Conservación, Ministerio de Ambiente y Energía).
Department of Forestry, Fisheries and The Environment (DFFE) (2015). Minister Edna molewa highlights progress in the war against poaching and plans for 2015 (Pretoria, South Africa: Department of Environmental Affairs. Forestry, Fisheries and the Environment). Available at: https://www.dffe.gov.za/mediarelease/molewa_waragainstpoaching2015.
Dornfeld T. C., Robinson N. J., Tomillo P. S., Paladino F. V. (2015). Ecology of solitary nesting olive ridley sea turtles at playa grande, Costa Rica. Mar. Biol. 162 (1), 123–139. doi: 10.1007/s00227-014-2583-7
Dunstan A., Robertson K., Fitzpatrick R., Pickford J., Meager J. (2020). Use of unmanned aerial vehicles (UAVs) for mark-resight nesting population estimation of adult female green sea turtles at raine island. PloS One 15 (6), e0228524. doi: 10.1371/journal.pone.0228524
Eckert K. L., Bjorndal K. A., Abreu-Grobois F. A., Donnelly M. (1999). Research and management techniques for the conservation of sea turtles Vol. vol. 4 (Washington DC: IUCN/SSC Marine Turtle Specialist Group Publication).
Ezat M. A., Fritsch C. J., Downs C. T. (2018). Use of an unmanned aerial vehicle (drone) to survey Nile crocodile populations: A case study at lake nyamithi, ndumo game reserve, south Africa. Biol. Conserv. 223, 76–81. doi: 10.1016/j.biocon.2018.04.032
Fonseca L. G., Arauz R., Chacon-Chaverri D., Mast R., Mario-Orrego C., Troëng S., et al. (2015). SWOT report X-special 10th anniversary Ed. (Ross, CA: Oceanic Society) 18–23.
Fowler L. E. (1979). Hatching success and nest predation in the green sea turtle, chelonia mydas, at tortuguero, Costa Rica. Ecology 60 (5), 946–955. doi: 10.2307/1936863
Fust P., Loos J. (2020). Development perspectives for the application of autonomous, unmanned aerial systems (UASs) in wildlife conservation. Biol. Conserv. 241, 108380. doi: 10.1016/j.biocon.2019.108380
Gallego D., Sarasola J. H. (2021). Using drones to reduce human disturbance while monitoring breeding status of an endangered raptor. Remote Sens. Ecol. Conserv. 7 (3), 550–561. doi: 10.1002/rse2.206
Gardner C. (2020). Nature’s comeback? no, the coronavirus pandemic threatens the world’s wildlife. Conversation. Available at: https://theconversation.com/natures-comeback-no-the-coronavirus-pandemic-threatens-the-worlds-wildlife-136209.
Godley B. J., Broderick A. C., Colman L. P., Formia A., Godfrey M. H., Hamann M., et al. (2020). Reflections on sea turtle conservation. Oryx 54 (3), 287–289. doi: 10.1017/S0030605320000162
Gonzalez L. F., Montes G. A., Puig E., Johnson S., Mengersen K., Gaston K. J. (2016). Unmanned aerial vehicles (UAVs) and artificial intelligence revolutionizing wildlife monitoring and conservation. Sensors 16 (1), 97. doi: 10.3390/s16010097
Gray P. C., Fleishman A. B., Klein D. J., McKown M. W., Bezy V. S., Lohmann K. J., et al. (2019). A convolutional neural network for detecting sea turtles in drone imagery. Methods Ecol. Evol. 10 (3), 345–355. doi: 10.1111/2041-210X.13132
Hambrecht L., Brown R. P., Piel A. K., Wich S. A. (2019). Detecting ‘poachers’ with drones: Factors influencing the probability of detection with TIR and RGB imaging in miombo woodlands, Tanzania. Biol. Conserv. 233, 109–117. doi: 10.1016/j.biocon.2019.02.017
Hart C. E., Ley-Quiñonez C., Maldonado-Gasca A., Zavala-Norzagaray A., Abreu-Grobois F. A. (2014). Nesting characteristics of olive ridley turtles (Lepidochelys olivacea) on El naranjo beach, nayarit, Mexico. Herpetol. Conserv. Biol. 9 (2), 524–534.
Heithaus M. R., Frid A., Wirsing A. J., Dill L. M., Fourqurean J. W., Burkholder D., et al. (2007). State-dependent risk taking by green sea turtles mediates top-down effects of tiger shark intimidation in a marine ecosystem. J. Anim. Ecol. 76, 837–844. doi: 10.1111/j.1365-2656.2007.01260.x
Hewitt C. (2022) Does sand hold heat? temperature master. Available at: https://temperaturemaster.com/does-sand-hold-heat/.
Hodgson A., Kelly N., Peel D. (2013). Unmanned aerial vehicles (UAVs) for surveying marine fauna: A dugong case study. PloS One 8 (11), e79556. doi: 10.1371/journal.pone.0079556
Hodgson J. C., Mott R., Baylis S. M., Pham T. T., Wotherspoon S., Kilpatrick A. D., et al. (2018). Drones count wildlife more accurately and precisely than humans. Methods Ecol. Evol. 9 (5), 1160–1167. doi: 10.1111/2041-210X.12974
Jorquera-Chavez M., Fuentes S., Dunshea F. R., Warner R. D., Poblete T., Jongman E. C. (2019). Modelling and validation of computer vision techniques to assess heart rate, eye temperature, ear-base temperature and respiration rate in cattle. Animals 9 (12), 1089. doi: 10.3390/ani9121089
Kays R., Sheppard J., Mclean K., Welch C., Paunescu C., Wang V., et al. (2019). Hot monkey, cold reality: surveying rainforest canopy mammals using drone-mounted thermal infrared sensors. Int. J. Remote Sens. 40 (2), 407–419. doi: 10.1080/01431161.2018.1523580
Lei J., Booth D. T. (2017). Who are the important predators of sea turtle nests at wreck rock beach? PeerJ 5, e3515. doi: 10.7717/peerj.3515
Leighton P. A., Horrocks J. A., Kramer D. L. (2011). Predicting nest survival in sea turtles: when and where are eggs most vulnerable to predation? Anim. Conserv. 14 (2), 186–195. doi: 10.1111/j.1469-1795.2010.00422.x
Linchant J., Lisein J., Semeki J., Lejeune P., Vermeulen C. (2015). Are unmanned aircraft systems (UAS s) the future of wildlife monitoring? a review of accomplishments and challenges. Mammal Rev. 45 (4), 239–252. doi: 10.1111/mam.12046
López-Castro M. C., Carmona R., Nichols W. J. (2004). Nesting characteristics of the olive ridley turtle (Lepidochelys olivacea) in cabo pulmo, southern Baja California. Mar. Biol. 145, 811–820. doi: 10.1007/s00227-004-1359-x
Lyons M., Brandis K., Callaghan C., McCann J., Mills C., Ryall S., et al. (2018). Bird interactions with drones, from individuals to large colonies. Aust. Field Ornithol. 35, 51–56. doi: 10.3316/informit.477965736750809
Maldonado T., Alfaro E., Fallas-López B., Alvarado L. (2013). Seasonal prediction of extreme precipitation events and frequency of rainy days over Costa Rica, central America, using canonical correlation analysis. Adv. Geosci. 33, 41–52. doi: 10.5194/adgeo-33-41-2013
Marcovaldi M. A. (1999). “Status and distribution of the olive ridley turtle, lepidochelys olivacea, in the Western Atlantic ocean,” in Proceedings of the regional meeting, marine turtle conservation in the wider Caribbean region: A dialogue for effective regional management. Eds. Eckert K. L., Abreu-Grobois K. A. (Santo Domingo, Dominican Republic: WIDECAST, IUCN-MTSG, WWF, and UNEP-CEP), 16–18.
Mazaris A. D., Schofield G., Gkazinou C., Almpanidou V., Hays G. C. (2017). Global sea turtle conservation successes. Sci. Adv. 3 (9), e1600730. doi: 10.1126/sciadv.1600730
Meletis Z. A., Harrison E. C. (2010). Tourists and turtles: Searching for a balance in tortuguero, Costa Rica. Conserv. Soc 8 (1), 26–43. doi: 10.4103/0972-4923.62678
Mesquita G. P., Mulero-Pázmány M., Wich S. A., Rodríguez-Teijeiro J. D. (2022). A practical approach with drones, smartphone and tracking tags for potential real-time tracking animal. Curr. Zool. 2022, 1–7. doi: 10.1093/cz/zoac029
Miller J. D. (2017). “Reproduction in sea turtles,” in The biology of sea turtles, vol. vol. 1 . Eds. Lutz P. L., Musick J. A. (Boca Raton, FL: CRC Press), 51–81.
Mortimer J. A., Esteban N., Guzman A. N., Hays G. C. (2020). Estimates of marine turtle nesting populations in the south-west Indian ocean indicate the importance of the chagos archipelago. Oryx 54 (3), 332–343. doi: 10.1017/S0030605319001108
Mulero-Pázmány M., Stolper R., Van Essen L. D., Negro J. J., Sassen T. (2014). Remotely piloted aircraft systems as a rhinoceros anti-poaching tool in Africa. PloS One 9 (1), e83873. doi: 10.1371/journal.pone.0083873
Nakamura M. F., Santos A. J. B., Lobão-Soares B., Corso G. (2019). Lunar phases and hawksbill sea turtle nesting. J. Ethol. 37 (3), 307–316. doi: 10.1007/s10164-019-00604-7
Ossmann M. (2019). Sea Turtle nesting trends from 2011-2017 on the osa peninsula, Costa Rica (Master’s thesis) (Duke University, NC).
Petritoli E., Leccese F., Ciani L. (2018). Reliability and maintenance analysis of unmanned aerial vehicles. Sensors 18 (9), 3171. doi: 10.3390/s18093171
Pheasey H., Glen G., Allison N. L., Fonseca L. G., Chacón D., Restrepo J., et al. (2021). Quantifying illegal extraction of sea turtles in Costa Rica. Front. Conserv. Sci. 39. doi: 10.3389/fcosc.2021.705556
Piacenza S. E., Richards P. M., Heppell S. S. (2019). Fathoming sea turtles: monitoring strategy evaluation to improve conservation status assessments. Ecol. Appl. 29 (6), e01942. doi: 10.1002/eap.1942
Pike D. A. (2008). Environmental correlates of nesting in loggerhead turtles, caretta. Anim. Behav. 76 (3), 603–610. doi: 10.1016/j.anbehav.2008.04.010
Pritchard P. C. (1997). “Evolution, phylogeny, and current status,” in The biology of sea turtles, vol. vol. 1 . Eds. Lutz P. L., Musick J. A. (Boca Raton, FL: CRC Press), 1–28.
Pritchard P. C. H., Mortimer J. A. (1999). “Taxonomy, external morphology and species identification,” in Research and management techniques for the conservation of Sea turtles. Eds. Eckert K. L., Bjorndal K. A., Abreu-Grobois F. A., Donnelly M. (Washington DC: IUCN/Species Survival Commission Marine Turtles Specialist Group Publication), 31–48.
Quesada-Rodríguez C., Orientale C., Diaz-Orozco J., Sellés-Ríos B. (2021). Impact of 2020 COVID-19 lockdown on environmental education and leatherback sea turtle (Dermochelys coriacea) nesting monitoring in pacuare reserve, Costa Rica. Biol. Conserv. 255, 108981. doi: 10.1016/j.biocon.2021.108981
Ramos E. A., Landeo-Yauri S., Castelblanco-Martínez N., Arreola M. R., Quade A. H., Rieucau G. (2022). Drone-based photogrammetry assessments of body size and body condition of Antillean manatees. Mamm. Biol. (in prep.). doi: 10.1007/s42991-022-00228-4
Raoult V., Colefax A. P., Allan B. M., Cagnazzi D., Castelblanco-Martínez N., Ierodiaconou D., et al. (2020). Operational protocols for the use of drones in marine animal research. Drones 4 (4), 64. doi: 10.3390/drones4040064
R Core Team (2022) R: A language and environment for statistical computing. Available at: https://www.r-project.org/.
Rees A. F., Avens L., Ballorain K., Bevan E., Broderick A. C., Carthy R. R., et al. (2018). The potential of unmanned aerial systems for sea turtle research and conservation: a review and future directions. Endanger. Species Res. 35, 81–100. doi: 10.3354/esr00877
Rees A. F., Baker S. L. (2006). Hawksbill and olive ridley nesting on masirah island, sultanate of Oman: an update. Mar. Turt. Newsl. 113, 2–5.
Reischig T., Cordes H. (2021). “Drones for turtles,” in Nahill B Sea Turtle research and conservation (Washington DC: Academic Press), 57–67.
Reischig T., Resende E., Cordes H. (2018). Drones for turtles: Controlling poaching of nesting loggerhead sea turtles with night vision unmanned aerial vehicles on boavista island, cabo Verde. Afr. Sea Turt. Newsl. 10, 9–13. Washington DC. Available at: https://www.turtle-foundation.org/wp-content/uploads/documents/publications/African_Sea_Turtle_Newsletter_2018_Issue_010_pp9-13.pdf
Salleh S. M., Nishizawa H., Ishihara T., Sah S. A. M., Chowdhury A. J. K. (2018). Importance of sand particle size and temperature for nesting success of green turtles in penang island, Malaysia. Chelonian Conserv. Biol. 17 (1), 116–122. doi: 10.2744/CCB-1266.1
Santidrian-Tomillo P., Roberts S. A., Hernández R., Spotila J. R., Paladino F. V. (2014). Nesting ecology of east pacific green turtles at playa cabuyal, gulf of papagayo, Costa Rica. Mar. Ecol. 36 (3), 506–516. doi: 10.1111/maec.12159
Santidrian-Tomillo P., Saba V. S., Piedra R., Paladino F. V., Spotila J. R. (2008). Effects of illegal harvest of eggs on the population decline of leatherback turtles in las baulas marine national park, Costa Rica. Conserv. Biol. 22 (5), 1216–1224. doi: 10.1111/j.1523-1739.2008.00987.x
Schofield G., Esteban N., Katselidis K. A., Hays G. C. (2019). Drones for research on sea turtles and other marine vertebrates–a review. Biol. Conserv. 238, 108214. doi: 10.1016/j.biocon.2019.108214
Schofield G., Katselidis K. A., Lilley M. K. S., Reina R., Hays G. C. (2017). Detecting elusive aspects of wildlife ecology using UAVs: new insights on the mating dynamics and operational sex ratios of sea turtles. Funct. Ecol. 31, 2310–2319. doi: 10.1111/1365-2435.12930
Shanker K., Pandav B., Choudhury B. C. (2003). “Sea Turtle conservation: population census and monitoring,” in A GOI–UNDP project manual. centre for Herpetology/Madras crocodile bank trust (Tamil Nadu, India: Mamallapuram).
Silva E., Marco A., da Graça J., Pérez H., Abella E., Patino-Martinez J., et al. (2017). Light pollution affects nesting behavior of loggerhead turtles and predation risk of nests and hatchlings. J. Photochem. Photobiol. B: Biol. 173, 240–249. doi: 10.1016/j.jphotobiol.2017.06.006
Smith J. E. (2016) Murder on moín beach. turtle conservancy. Available at: https://www.turtleconservancy.org/news/2016/3/murder-on-moin-beach.
Smith C. E., Sykora-Bodie S. T., Bloodworth B., Pack S. M., Spradlin T. R., LeBoeuf N. R. (2016). Assessment of known impacts of unmanned aerial systems (UAS) on marine mammals: data gaps and recommendations for researchers in the united states. J. Unmanned Veh. Syst. 4 (1), 31–44. doi: 10.1139/juvs-2015-0017
Snitch T. (2015). Satellites, mathematics and drones take down poachers in Africa. Conversation. Available at: https://theconversation.com/satellites-mathematics-and-drones-take-down-poachers-in-africa-36638.
Speakman J. R., Hays G. C., Lindblad E. (1998). Thermal conductivity of sand and its effect on the temperature of loggerhead sea turtle (Caretta caretta) nests. J. Mar. Biol. Assoc. U. K. 78 (4), 1337–1352. doi: 10.1017/S0025315400044532
Stander R., Walker D. J., Rohwer F. C., Baydack R. K. (2021). Drone nest searching applications using a thermal camera. Wildl. Soc Bull. 45 (3), 371–382. doi: 10.1002/wsb.1211
Stephenson P. J. (2019). Integrating remote sensing into wildlife monitoring for conservation. Environ. Conserv. 46 (3), 181–183. doi: 10.1017/S0376892919000092
The State of the World’s Sea Turtles [SWOT] (2022) Online map & Sea turtle database. Available at: https://seamap.env.duke.edu/swot.
Wall B. M. (2014). Can drones help tackle africa’s wildlife poaching crisis (BBC News). Available at: https://www.bbc.com/news/business-28132521.
Whitmore C. P., Dutton P. H. (1985). Infertility, embryonic mortality and nest-site selection in leatherback and green sea turtles in Suriname. Biol. Conserv. 34, 251–272. doi: 10.1016/0006-3207(85)90095-3
Whitworth A., Beirne C., Flatt E., Pillco Huarcaya R., Cruz Diaz J. C., Forsyth A., et al. (2018). Secondary forest is utilized by great curassows (Crax rubra) and great tinamous (Tinamus major) in the absence of hunting. Ornithol. Appl. 120 (4), 852–862. doi: 10.1650/CONDOR-18-57.1
Witherington B. E. (1992). Behavioral responses of nesting sea turtles to artificial lighting. Herpetologica 48 (1), 31–39.
Witt R. R., Beranek C. T., Howell L. G., Ryan S. A., Clulow J., Jordan N. R., et al. (2020). Real-time drone derived thermal imagery outperforms traditional survey methods for an arboreal forest mammal. PloS One 15 (11), e0242204. doi: 10.1371/journal.pone.0242204
Keywords: sea turtle, nesting beach, wildlife monitoring, conservation technology, aerial survey, thermal imagery, drone, Unmanned Aerial Vehicle (UAV)
Citation: Sellés-Ríos B, Flatt E, Ortiz-García J, García-Colomé J, Latour O and Whitworth A (2022) Warm beach, warmer turtles: Using drone-mounted thermal infrared sensors to monitor sea turtle nesting activity. Front. Conserv. Sci. 3:954791. doi: 10.3389/fcosc.2022.954791
Received: 27 May 2022; Accepted: 01 July 2022;
Published: 28 July 2022.
Edited by:
Carlos R. Ruiz-Miranda, State University of the North Fluminense Darcy Ribeiro, BrazilReviewed by:
Gail Schofield, Queen Mary University of London, United KingdomFabiano Rodrigues de Melo, Universidade Federal de Viçosa, Brazil
Copyright © 2022 Sellés-Ríos, Flatt, Ortiz-García, García-Colomé, Latour and Whitworth. This is an open-access article distributed under the terms of the Creative Commons Attribution License (CC BY). The use, distribution or reproduction in other forums is permitted, provided the original author(s) and the copyright owner(s) are credited and that the original publication in this journal is cited, in accordance with accepted academic practice. No use, distribution or reproduction is permitted which does not comply with these terms.
*Correspondence: Bárbara Sellés-Ríos, YmFyYmFyYXNlbGxlc0Bvc2Fjb25zZXJ2YXRpb24ub3Jn; Andrew Whitworth, YW5keXdoaXR3b3J0aEBvc2Fjb25zZXJ2YXRpb24ub3Jn