- 1Post-graduate Programme in Wildlife Science, Wildlife Institute of India, Dehradun, India
- 2Project Elephant, Ministry of Environment, Forest and Climate Change, New Delhi, India
- 3Elephant Research and Conservation Division, Aaranyak, Guwahati, India
- 4Department of Endangered Species Management, Wildlife Institute of India, Dehradun, India
Physical barriers, like solar fences, elephant-proof trenches, stone, or rubble walls, are installed as conflict mitigation interventions in India’s major Asian elephant (Elephas maximus) ranges. However, installations lacking a priori scientific assessment of site specificity for reducing elephant incursion in the human settlements often fail in the intended goals of the resource-intensive management interventions. Since humans are central to conflict issues, research focusing on social aspects is essential for devising solutions. Despite the use of barriers in the Golaghat district in Assam, human–elephant conflict (HEC) cases are increasing, which offers an opportunity to evaluate their efficacy. Optimized hotspot analysis of the historic HEC records from 2010 to 2019 was done to check the spatial extent of the conflict and its dispersion in the district. We also assessed the efficiency of a 4.2-km long solar fence and a 2-km long elephant-proof trench through generalized linear modeling. We evaluated the encounter rates of elephant signs and other site covariates at the barrier and non-barrier sites. Multinomial logistic regression was applied to assess the perception of local people on the barriers and overall HEC in their areas based on their willingness to pay (WTP) for maintenance of the barriers. The highest cases occurred between 2016 and 2017, making up 25% of the total conflict cases. It was noticed that the extent of these hotspots was concentrated, and there was no dispersion of the conflict to other areas. Furthermore, a significant difference in the encounter rates of elephant signs between barriers and no barrier sites was observed. Solar fenced areas showed relatively lower encounter rates than areas with the trench, suggesting better efficiency of solar fencing than trench in the landscape. In addition, only five out of seven explanatory variables, viz., the persistence of HEC cases, amount of ex-gratia compensation, time taken in getting compensation, change in elephant behavior (incursion), and crop-raiding frequency significantly explained people’s perception of the barrier and non-barrier sites. According to local people, physical barriers are essential in managing HEC; hence, installing and maintaining solar fences in the high conflict hotspots is crucial for this landscape.
Introduction
The negative interactions between humans and elephants arising from shared space and overlapping food resources are commonly represented as human–elephant conflict (HEC) (Fernando et al., 2008). The global populations of Asian (Elephas maximus, Linnaeus, 1758) and African (Loxodonta spp., Anonymous, 1827) elephants are affected by HEC (Blanc et al., 2003), and regions where human settlements are close to elephant habitats tend to have high conflict incidences (Naughton et al., 1999). HEC is most common in areas where former elephant habitats have been reclaimed, encroached, and converted into farmlands (Osborn and Parker, 2002). The Asian elephant is one of Asia’s most important flagship species for biodiversity conservation (Venkataraman et al., 2002). India, being a country with an average human population density of 464 persons per km2 (The World Bank, 2020) and holding key elephant habitats, is among the HEC hotspots in the world (Naha et al., 2019; Naha et al., 2020; Tripathy et al., 2021). The States of Odisha, West Bengal, Jharkhand, and Assam bore a relatively higher number of HEC incidences in descending order (Jadhav, 2020; Akhtar, 2021). An increase in HEC cases is often due to crop raiding of agricultural fields by elephants falling within the elephant movement ranges (Venkataraman et al., 2002; Fernando et al., 2008). Most recent estimates of the damage caused by elephants in India record the loss of over 12,000 hectares of land by crop raiding and 800–1,000 houses between 2013 and 2014 (Agarwal, 2015).
The State of Assam supports a population of approximately 5,719 elephants (MoEFCC, 2017), second only to Karnataka. It is among the high conflict regions in India, where 875 people and 825 elephants succumbed to death due to HEC between 2010 and 2020 (Akhtar, 2021). The reason behind emerging HECs in Assam is the high encroachment of forests (Government of Assam, 2011; ISFR, 2019). For mitigation of HECs, physical barriers are installed on the forest–village edges. However, due to diffuse boundaries between human use areas and elephant habitats, the placement and construction of these physical barriers are complex and unjustified (Rangarajan et al., 2010). Therefore, installed barriers should be tested for their efficacy in deterring elephants away from human settlements. Moreover, the persistence of conflicts in an area affects attitudes and perceptions of local people toward wildlife and conservation efforts that heavily influence their willingness to engage in any conflict mitigation process (Ramkumar et al., 2013; Locke and Buckingham, 2016; Karanth and Kudalkar, 2017). However, recently, only a few studies have examined the social aspects of human–animal conflicts (Inskip and Zimmermann, 2009; Campbell-Smith et al., 2012; Sengupta et al., 2020).
It is vital to understand the spatiotemporal dispersion patterns of HECs by collating and comparing the past decade’s conflict instances concerning negative interactions, viz., crop losses, human/elephant casualties due to HEC, and property damage. According to Rangarajan et al. (2010), the intensity of HECs has increased and dispersed over the last two decades. Effective mitigation is vital for the long-term conservation of this endangered species with the increasing intensity of conflicts. The lack of a clear understanding of the principles of implementation and maintenance of mitigation tools such as barriers often leads to their failure (Desai and Riddle, 2015). In the Indian context, there is a lack of monitoring and assessment of the effectiveness of implemented HEC management approaches, including physical mitigation tools like electric fences, trenches, and walls (Rangarajan et al., 2010). Therefore, rigorous testing of these mitigation measures is needed for the successful and long-term management of conflicts (Nelson et al., 2003).
In order to fill the abovementioned existing gaps, the study was conducted to look at a) whether the extent and intensity of HECs in the Golaghat district were concentrated or dispersing spatially over time and b) whether the physical barriers effective in restricting the movement of elephants to human-use areas in Golaghat, and c) how do local people perceive the mitigation strategies involving physical barriers. It was hypothesized that with increasing cases of HECs, the spatial extent of conflict disperses over time; intensity and frequency of HECs were reduced by physical barriers, and elephant incursion was dependent on the types and placement of the barriers; and conflict-related (incursion rates, breaches, and damages/losses) and socio-economic variables (income level, gender, and educational qualification) affected local people’s perception towards the barriers.
Materials and methods
Study area
Located between 25°50'48"N–26°58'35"N and 93°19'11"E–94°14'24"E in central Assam, Golaghat is a homogeneous plain and low-lying area on the south bank of the river Brahmaputra covering an area of 3,502 km2. The altitudinal gradient of this site ranges from 40 to 360 m ASL. Golaghat district is home to two protected areas, viz., Kaziranga National Park (Kaziranga, 380 km2) on the west and the proposed Nambor Doigurung Wildlife Sanctuary (Nambor Doigurung), divided into Lower Doigurung Reserve Forest (13.5 km2) and Upper Doigurung Reserve Forest (9.3 km2), on the south. Both protected areas fall within the Kaziranga–Karbi-Anglong Elephant Reserve (Das et al., 2012). This district is a part of two major elephant corridors in north-eastern India, viz., number 20 (Kalapahar to Doigurung) and number 21 (Kaziranga to Karbi-Anglong and Panbari) (Wildlife Trust of India, 2019)1. The fringe villages of Kaziranga and Nambor Doigrung served as two study areas, where villages with barriers (either solar fence or trench) (Figure 1) were chosen as the treatment and villages without any barriers were the control of this study (Figure 2). The climate of Golaghat is highly humid, with a mean annual rainfall of up to 1,720.4 mm (Government of Assam, 2022) and the mean annual temperature between 10°C (50℉) and 38°C (100.4℉) (Government of Assam, 2022). This district has four major habitat types, i.e., tropical wet evergreen forests, tropical semi-evergreen forests, grassland, and savannahs (Champion and Seth, 1968). The forest cover of Golaghat is spread over 19.1% of the total area equaling 669.9 km2 (ISFR, 2019). The total human population of the district is 10,66,888, with a density of 305 people per km2 (Government of Assam, 2022). The villages of Golaghat are ethnically diverse, including Ahoms, Assamese, Tea-tribes, Mishing, Tamangs, and Karbis. A small population of migrants like Marwari, Bengali, and Biharis, and immigrants from Bangladesh and Nepal are among the other inhabitants (Government of Assam, 2022). Agriculture serves as the major livelihood option for people of this area, mainly cultivating paddy (Oryza sativa), tea (Camellia sinensis), mustard (Brassica juncea), and some vegetables (ICAR, 2020; Government of Assam, 2022). Physical barriers, such as solar fences, are installed in the park boundary of the Agoratoli and Kohora ranges of Kaziranga. Similarly, a stone wall was constructed by the Numaligarh refinery in the Numaligarh area. Trench(es) are also present in some tea estates and crop fields near Nambor Reserve Forest. This study included two barriers, viz., a 4.2-km long solar fence in the Agoratoli range of Kaziranga and a trench of 2 km in length in the Bokial range of the Nambor Doigurung. These two barriers are noted to have non-uniform landscape configurations at various parts. It is important to know that the study was conducted during the crop sowing season, and therefore, there were no ripe crops in the study areas.
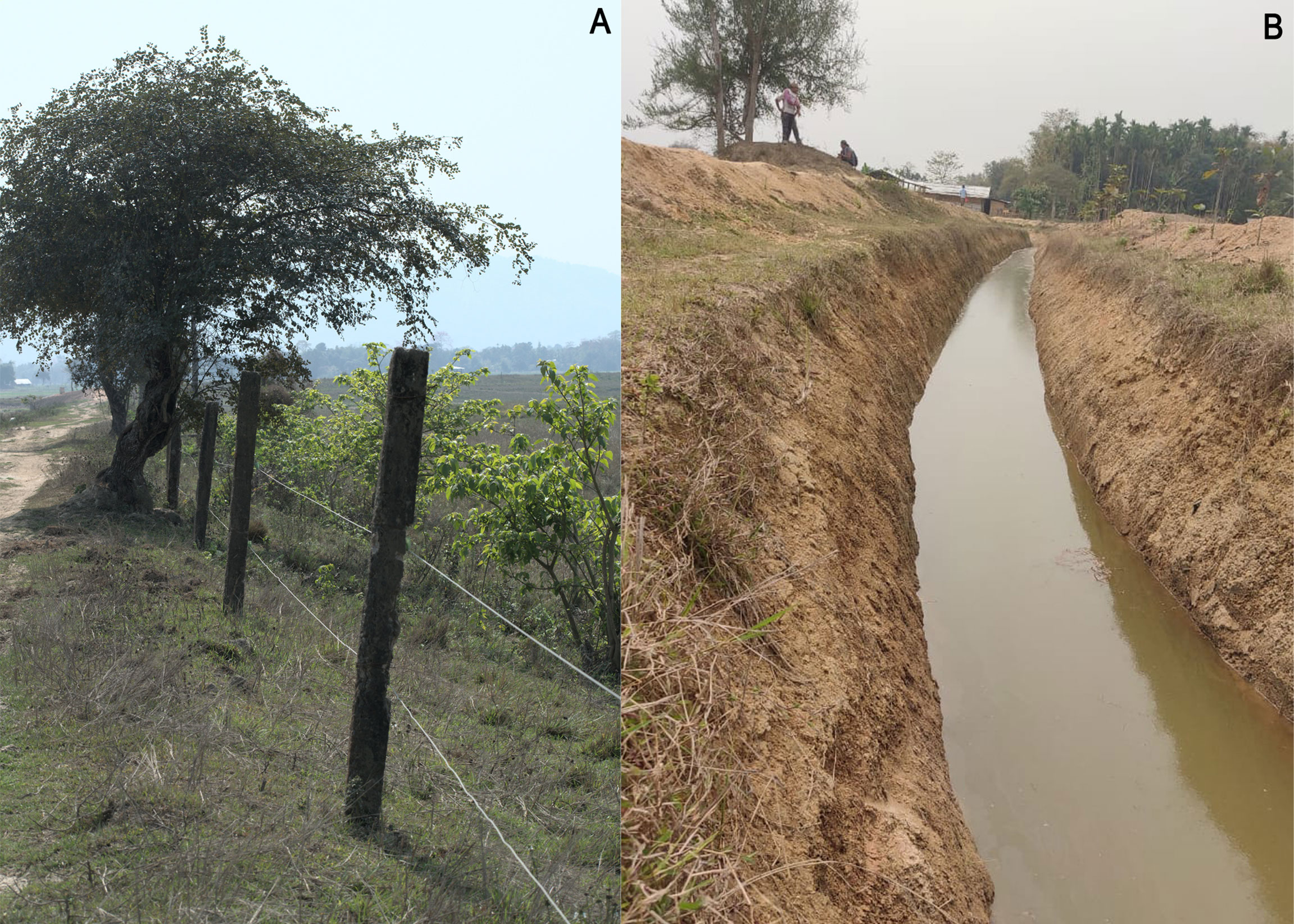
Figure 1 (A) Solar fence in Agoratoli range of Kaziranga National Park; (B) elephant-proof trench in Bokial range of Nambor Doigurung Wildlife Sanctuary.
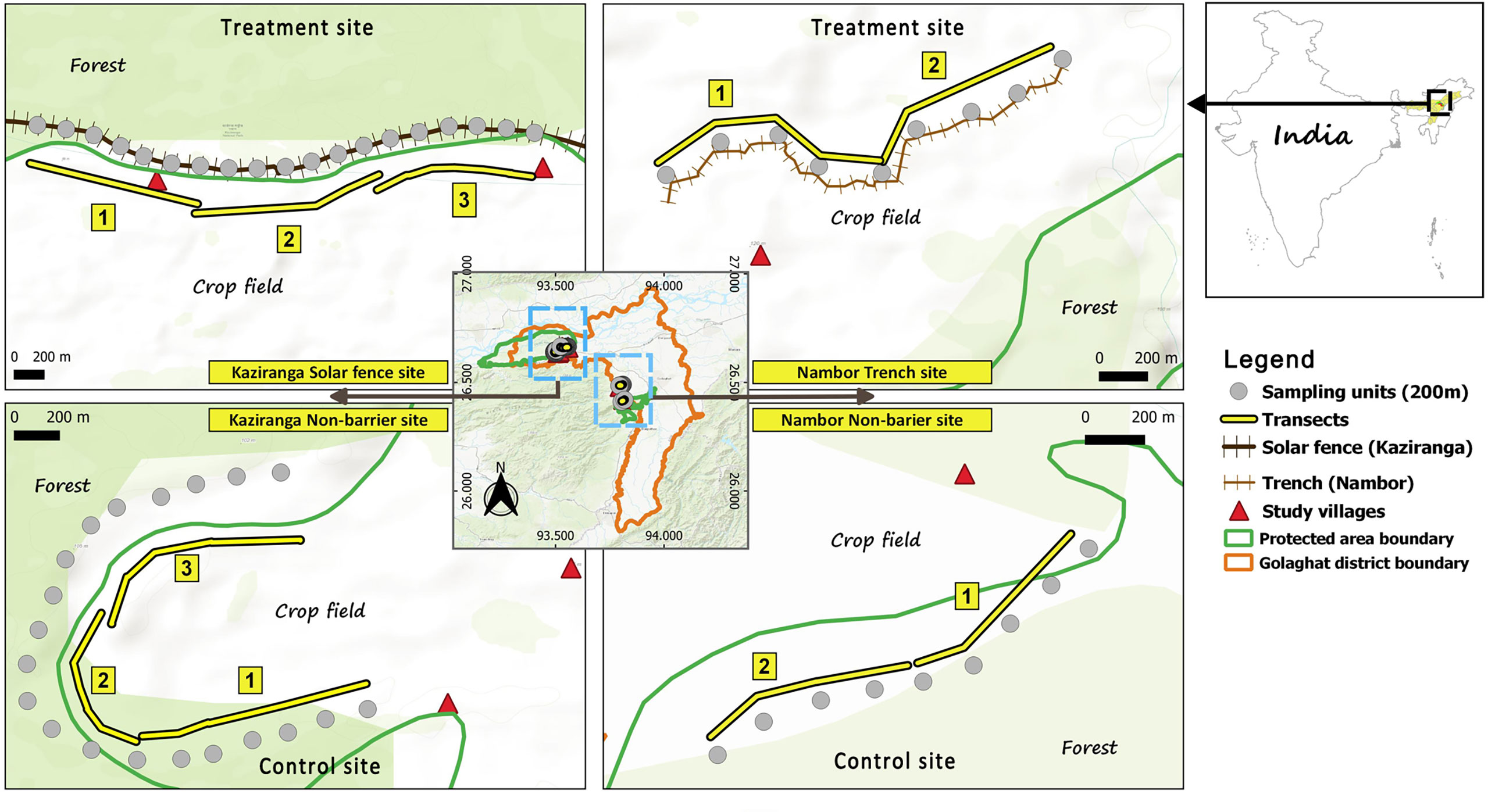
Figure 2 The study villages with transect layouts for elephant sign surveys along the barrier (treatment) and non-barrier (control) sites of Kaziranga National Park and Nambor Doigurung Wildlife Sanctuary.
Field methods
Data collection was done from January 2021 and March 2021. Historical conflict data of Golaghat district for the last decade, i.e., 2010–2019, involving i) crop/property damages, ii) loss of human lives, and iii) loss of elephant lives, were collected from the Assam Forest Department. Locations of villages were marked manually on Google Earth version 6.2.9200.0 and verified with village polygons acquired from NASA-SEDAC open-sourced portal (Meiyappan et al., 2018), and ground truthing was done during the field exercise with the help of local field assistants.
A reconnaissance survey was later conducted in the study sites after identifying villages based on i) absence/presence of physical barriers to compare control and treatment sites and ii) similar habitat characteristics on the part of the reserves. Six villages adjacent to the protected areas, namely, Diffloo Pathar, Tamuli Pathar, Japari Pathar and Agoratoli Mising gaon near Kaziranga, and Sundarpur and Kolbari near Nambor Doigurung were chosen. Tamuli Pathar and Agoratoli near Kaziranga had a barrier (i.e., solar fence), and the rest did not. Similarly, Sundarpur village near Nambor Doigurung had a barrier (i.e., trench), and the others did not. A total of 8.4 km (4.2×2) in the barrier and non-barrier study villages of Kaziranga were monitored through transects parallel to the barrier and parallel to the interface between the crop field and the natural habitat, respectively. Likewise, 4 km (2×2) in the barrier and non-barrier study villages of Nambor Doigurung was monitored through transects parallel to the barrier and parallel to the interface between the crop field and the natural habitat, respectively. Subsequently, the sites with solar fence in Kaziranga were divided into three equal parts with a distance of 1.4 km each. The site with a trench in Nambor Doigurung was divided into two equal parts of 1 km each. Briefly, in Kaziranga, a total effort of 33,600 m (16,800 m×2), i.e., 200 m×7 sampling units×3 transects×4 temporal replicates in both the barrier and non-barrier sites were made. In Nambor Doigurung, a total effort of 16,000 m (8,000 m×2), i.e., 200 m×5 sampling units×2 transects×4 temporal replicates in both the barrier and non-barrier sites, were made (Table 1). This study design was finalized based on i) length of the barriers, ii) accessibility for data collection, iii) time, and iv) logistic limitations with guided consultancies from local experts/scientists and forest department officials. Monitoring of the physical barriers throughout the sampling period ensured, viz., recording breaches in the barriers, direct or indirect signs of elephants (tracks and dung), using sign surveys at every 50 m of the sampling units within a transect (Appendix 1) (Kioko et al., 2008).
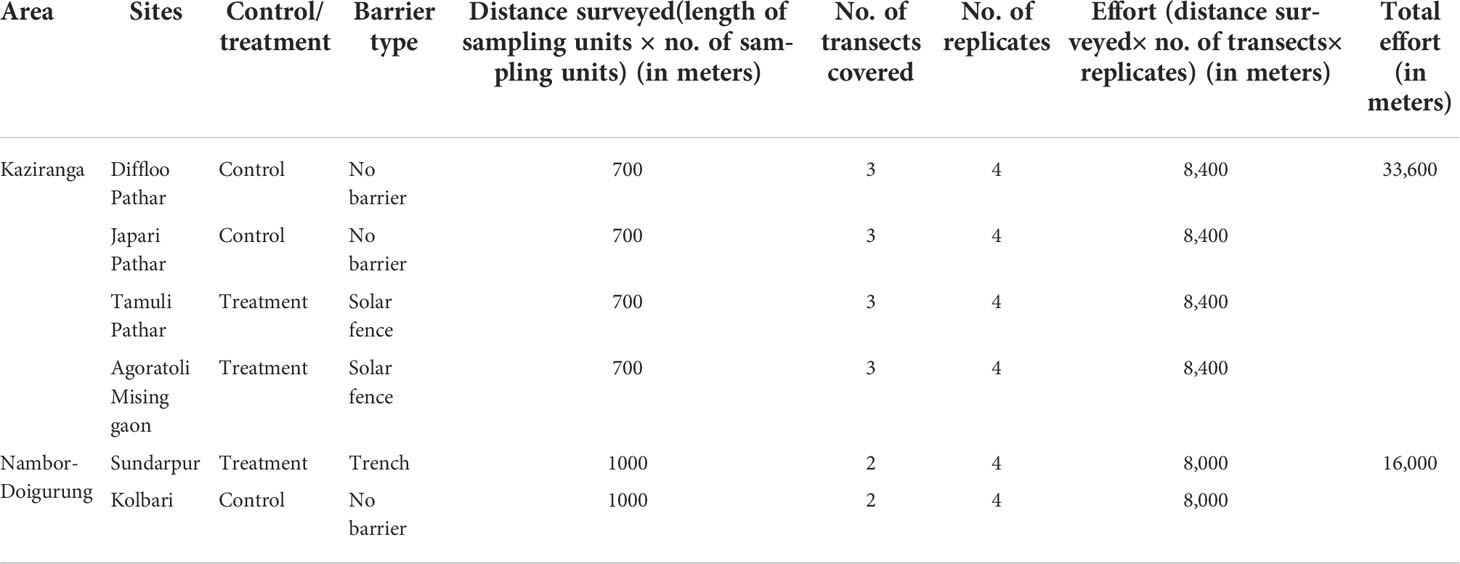
Table 1 List of control and treatment villages in Kaziranga and Nambor Doigurung areas of the Golaghat district depicting efforts done in each during the survey period.
Simultaneously, household interviews were done by convenience sampling, ensuring avoidance of continuously or tightly spaced households. Semi-structured questionnaire surveys of the chosen villages were conducted at both sites (Appendix 2) (Dunn, 2000; Rose et al., 2018). If a village had households <100, then 30% of the total households were covered; if they had ≥100, then 20% were covered, to be able to cover an array of respondents within the limited duration (CDC, 2008; Young et al., 2014). The first author did the interviews in the local language, i.e., Assamese. Local field assistants assisted in interviews conducted in Mishing and Adivasi (both tribal) languages. Questions were designed to understand people’s views on HECs, their losses, compensation scheme, change in instances of HECs before and after installation of the barriers, their willingness to actively participate in the maintenance of barriers, and other related socio-economic information. Here, the willingness to pay (WTP) for maintaining barriers was chosen as a proxy to assess people’s perceptions of the barriers. Willingness to pay was investigated in terms of hypothetical monetary inputs from the interviewees. The double bidding method collected a precise range of minimum and maximum financial WTP inputs (Hadker et al., 1997). The bidding was initiated from Indian rupees 10 and kept increasing until the “no” answer from the respondent ceased the bid, indicating hesitance in paying further. A total of n=249 interviews were taken. All the respondents were aged between 18 and 72 years, ensuring fair representation of all communities, genders, and occupations. Only one respondent from each household was interviewed, lasting for <20 min. Before the questionnaire, the interviewers sought verbal consent from the respondents regarding the survey. Participation in the survey was voluntary, and we assured the respondents that their information will be used for research purposes only.
Analytical methods
For visualization of historical spatiotemporal patterns of HECs in the study area, optimized hotspot analysis was carried out using the “Hotspot Analysis (Getis-Ord Gi*)” tool in ArcMap version 10.6. Input village features consisting of conflict incidents for all data types occurring each year from 2010 to 2019 (Getis and Ord, 1992) were made. The conflict data were divided into five groups with an interval of 1 year, i.e., 2010–2011, 2012–2013, 2014–2015, 2016–2017, 2018–2019, to reduce complexity. This hotspot analysis tool helps detect clusters of high values often indicative of a hotspot. The p- and z-values of each village input feature are either 90%, 95%, or 99% confident to determine the statistical significance of the potential optimized conflict clusters (Kalinic and Krisp, 2018). A feature with a high (positive) z-value is considered a statistically significant conflict hotspot and will be surrounded by other features with a high z-value. For larger z-scores, an intense clustering of high values (hotspot) is observed. A z-score ≥ 1.96 (≥ 95% CI) is the highest potential optimized conflict hotspot (Kalinic and Krisp, 2018). This tool interrogated the input data and determined settings that produced optimal hotspots of HECs for each year in the district. It helped determine conflict dispersion or persistence and changes in their relative intensity over the years.
In order to assess the effectiveness of the two types of barriers relative to the non-barrier sites, the difference in means of encounter rates of elephant signs of the three sites, i.e., solar fence, trench, and non-barrier areas, were determined. A Welch analysis of variance (ANOVA) was run using package “stats version 4.1.0” in R Studio version 1.4.1717 software due to small sample sizes having unequal variances. The categorical independent variable, i.e., “type of barrier” (divided into “solar fence,” “trench,” and “no barrier”) was modeled with the continuous dependent variable “encounter rate of elephant signs.” To determine statistically significant difference between mean encounter rates of the types of barriers, a pairwise post-hoc Games–Howell multiple comparison test was done using the package “rstatix version 0.7.0” in R Studio (Kassambara, 2021). Furthermore, to determine the relationships of predictor variables in driving the encounter rates of elephant signs, a generalized linear model (glm) with family “Poisson” was executed. The response variables were the encounter rates of direct and indirect elephant signs (signs/km). The predictor variables were distance from the nearest forest area, distance from the nearest water stream, and distance from the nearest human settlement. Multicollinearity between the predictor variables with respect to the response variables was checked by using the variance inflation factor (VIF) in Microsoft Excel. Given that the VIF values for each predictor variable were found close to 1, no multicollinearity was found.
Perception of people on the barriers was assessed based on their willingness to pay for the maintenance of the barriers used as a proxy. The continuous data of willingness to pay was categorized into three classes, i.e., “1” indicating protest zero (where the amount was zero, and the respondent was unwilling to pay as they were uninterested in paying), “2” for true zero (where the amount was zero, and the respondent was unwilling to pay due to low income), and “3” representing positive willingness to pay (where the amount was higher than zero). The third category, i.e., the willingness to pay category, was not categorized further to reduce complexity. A multinomial logistic regression in R Studio version 1.4.1717 was run using the package “nnet version 7.3” (Ripley and Venables, 2021) for barrier and non-barrier sites separately. The independent variables are all the socio-economic and conflict-related information that affects the willingness of people to pay for barriers at both the barrier and non-barrier sites (Appendices 3, 4). In total, 11 logistic regression models for barrier sites and 13 models for non-barrier sites were executed, having various socio-economic and conflict-related variables. The parsimonious models were selected based on the least AICc value among all the models (Burnham and Anderson, 2004). Multicollinearity between the predictor variables with respect to the response variables was checked using the variance inflation factor (VIF) in Microsoft Excel. Given that the VIF values for each predictor variable were found close to 1, no multicollinearity was found.
Results
Conflict hotspots and dispersion of HECs
The spatial configuration of HECs at the village level was patchy over the decade. High HEC hotspots were visualized by two clusters composed of different villages with significant (z-score≥1.96) spatial patterns throughout the decade (Figures 3–5). These hotspots were concentrated and persistent in villages around the western (near Kaziranga) and south-western (near Nambor Doigurung) regions of the Golaghat district. The optimized hotspot analysis indicated approximately a 16% increase in the conflicts hotspot emerging villages, shown by a significant z-score ≥ 1.96 (≥ 95% CI) from 2016–2017 to 2018–2019, including all types of conflicts in Golaghat. The period between 2014 and 2015 witnessed the highest number of crop/property damage incidents caused by elephants, with maximum reports from Kandulimari and Japori Pathar, identified as highly intensified conflict hotspots (with 95% CI) (Figure 3). Likewise, the highest number of human casualties caused by elephants occurred between 2012 and 2013, with Murphulani and Bogidhola Tea Estate becoming hotspots (with 95% CI) (Figure 4). Similarly, between 2014 and 2015, Koyabosti reported the highest elephant mortality incidents caused by HECs and was identified as the hotspot (with 95% CI) (Figure 5).
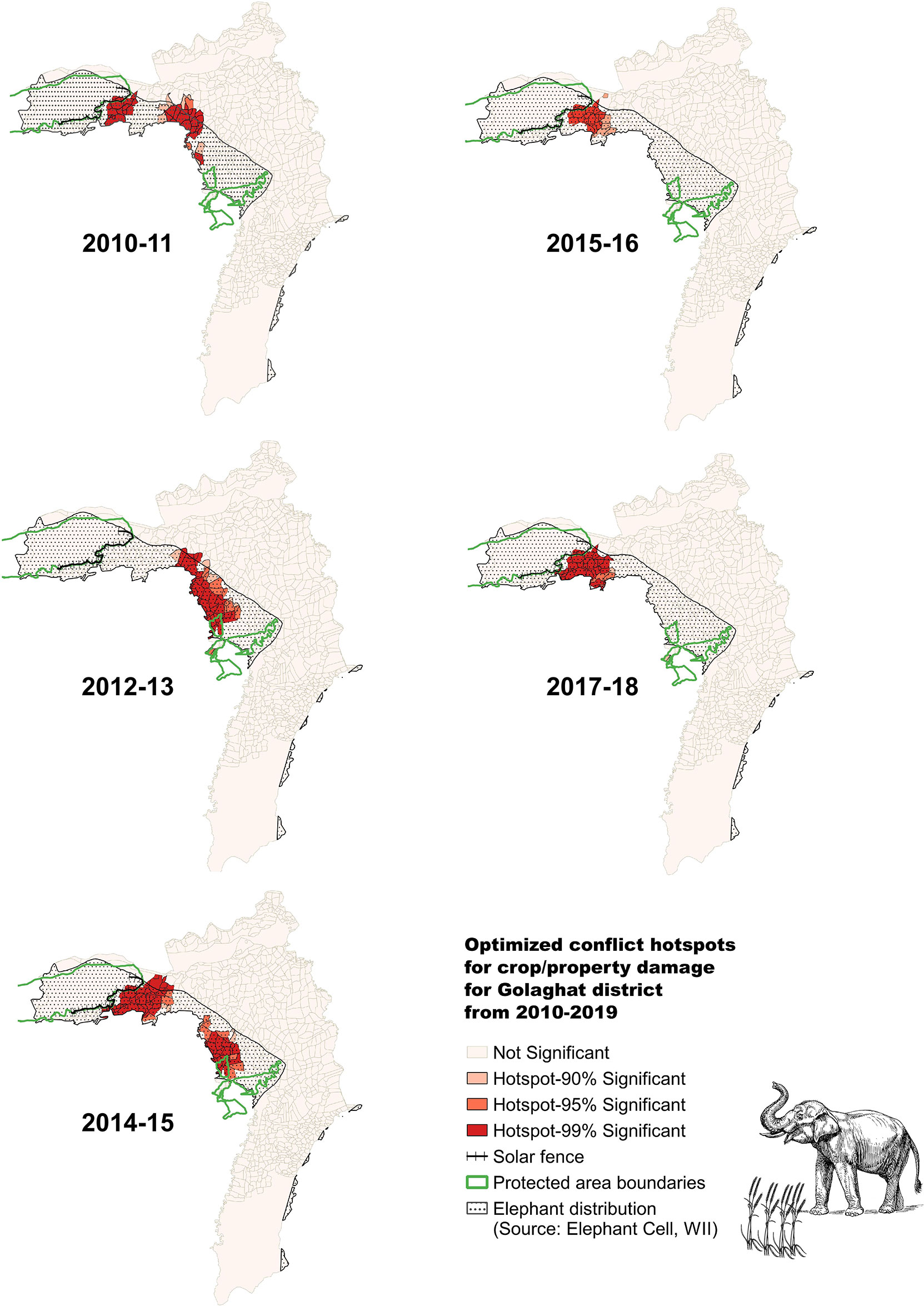
Figure 3 Conflict distribution and dispersion over the time period between 2010 and 2019 in the form of optimized crop/property damage hotspots for Golaghat district.
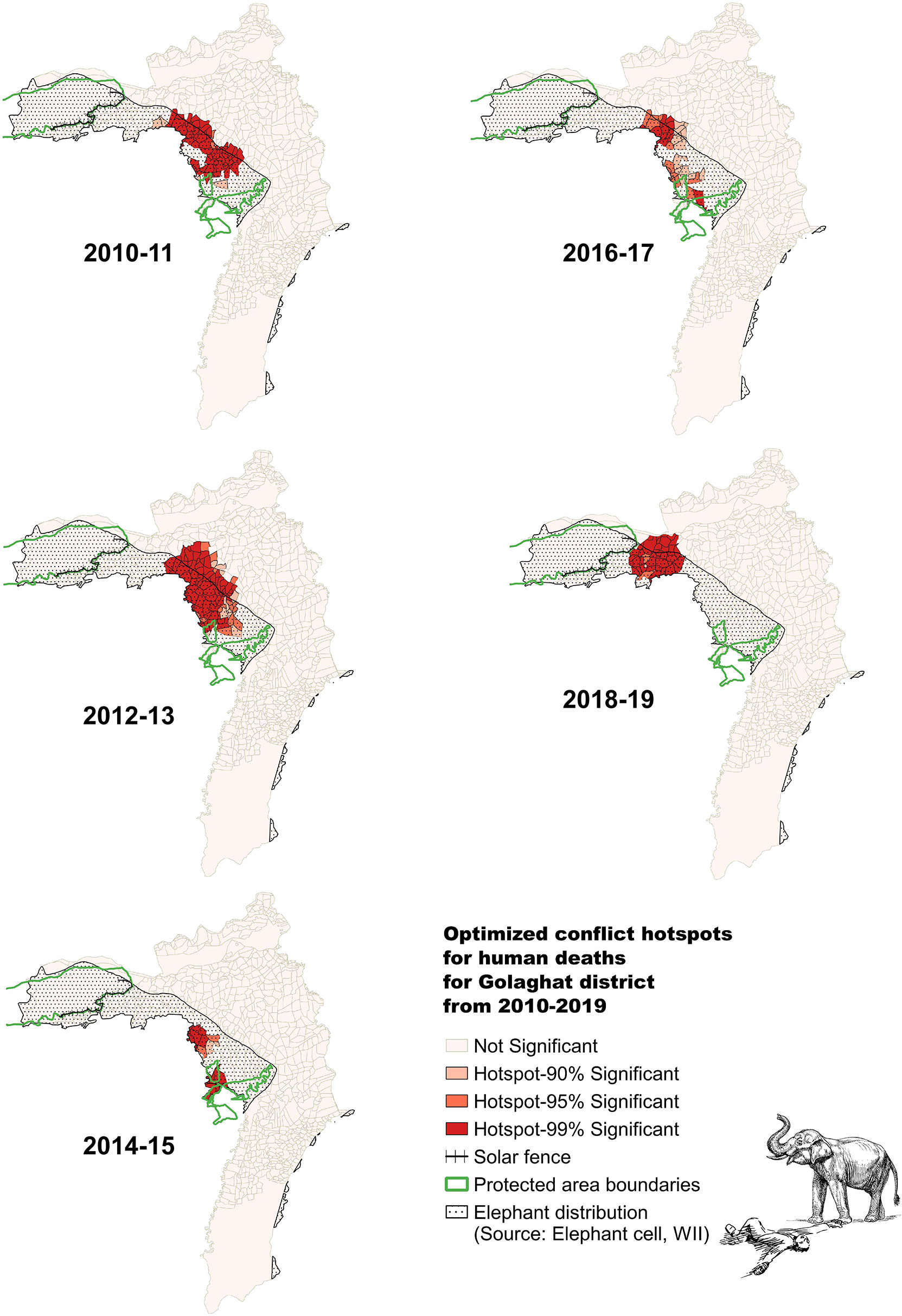
Figure 4 Conflict distribution and dispersion over the time period between 2010 and 2019 in the form of optimized human death hotspots for Golaghat district.
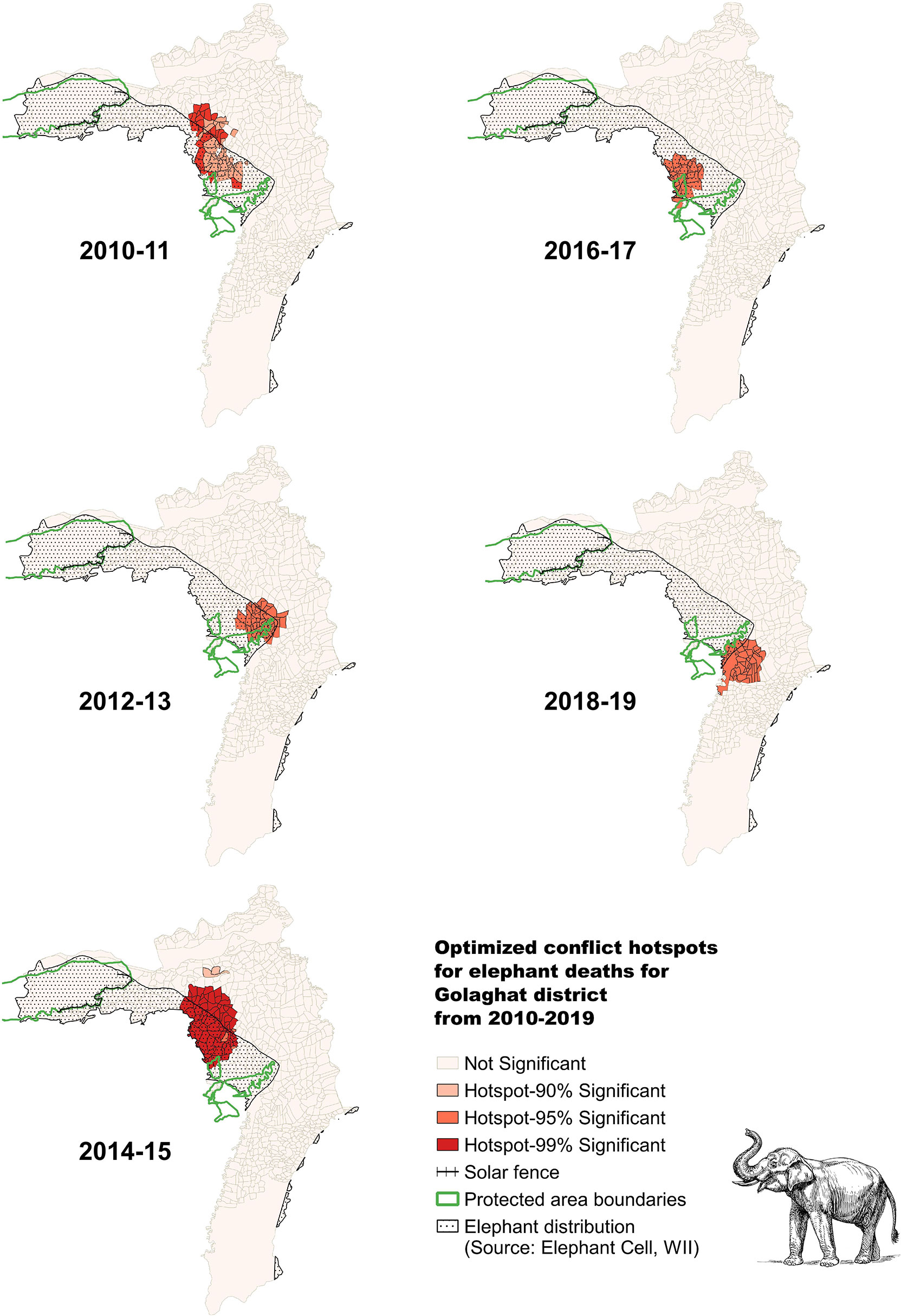
Figure 5 Conflict distribution and dispersion over the time period between 2010 and 2019 in the form of optimized elephant death hotspots for Golaghat district.
Effectiveness of physical barriers
The highest mean encounter rate was observed for the non-barrier sites (3.3 ± 0.6SE signs/km), followed by the trench near Nambor Doigurung (2.5 ± 0.9SE signs/km), and the solar fence in Kaziranga (0.7 ± 0.2SE signs/km). A significant difference among the encounter rates of sites with the solar fence, trench, and without barriers was found (F=13.7, df=2, p<0.01). The post-hoc analysis suggests that only the means of encounter rates of the solar fence and no barrier sites were significantly different [𝛽=1.09 (0.5−1.6SE, CI=0.95, adj p<0.01)] (Table 2). The difference between the means of encounter rates of elephant signs of solar fence–trench and trench–no barrier sites was not significantly different (p>0.05) (Table 2). It means that a solar fence may be relatively more effective than a trench in reducing elephant incursion into the human-settlement areas relative to the non-barrier sites, since fewer elephant signs were encountered. However, inference from the results indicates that the trench did not perform well compared to the solar fence, as the trench was found to have more encounter rates with a higher mean than the solar fence sites (Figure 6). Overall, barrier site encounter rates are relatively lower than non-barrier sites (Figure 7).

Table 2 Table representing the output of post-hoc Games–Howell multiple comparison test to look at the difference between mean encounter rates of all sites.
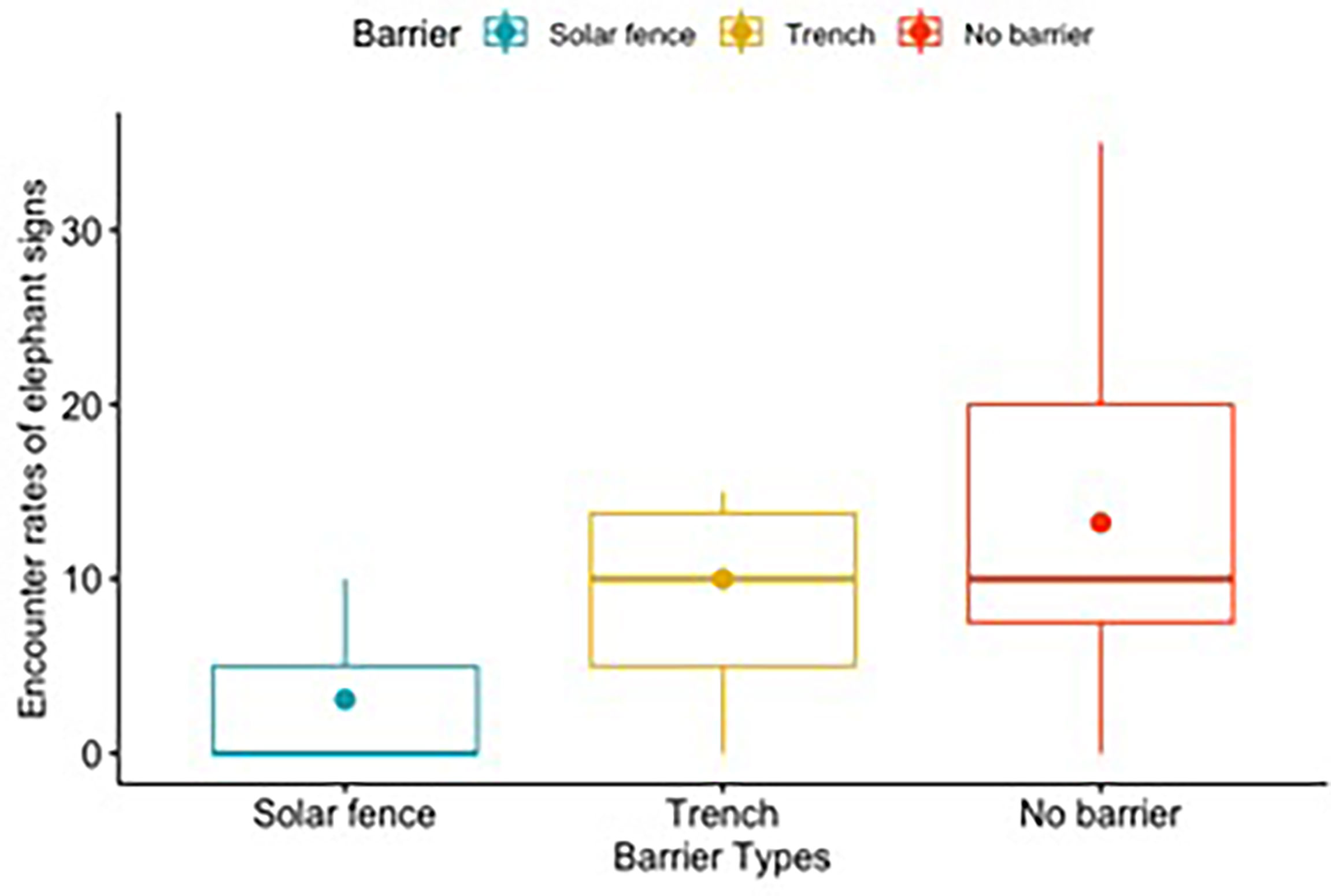
Figure 6 Box plot indicating quantiles and mean encounter rates for solar fence, trench, and no-barrier sites.
The model (mod6=encounter_rate~ barrier_occ+dist_settlement+dist_forest+dist_stream) was found to have the highest predictive power (Table 3). It suggested that in the presence of a solar fence, the encounter rates of elephant signs decreased significantly (𝛽=−1.7 ± 0.2SE, p<0.05) (Table 4). Likewise, in the presence of a trench, the encounter rates also decreased significantly (𝛽=−1.4 ± 0.4SE, p<0.01) but not as much as with the solar fence (Table 4). However, the encounter rates increased significantly with an increase in distance from the forest (𝛽=0.001 ± 0.0004SE, p<0.05) (Table 4). The other variables, such as distance from the nearest stream, suggest a decrease in encounter rates with increasing distance from streams and an increase in encounter rates with increasing distance from human settlements; however, both did not significantly affect the encounter rates (p>0.05) (Table 4).
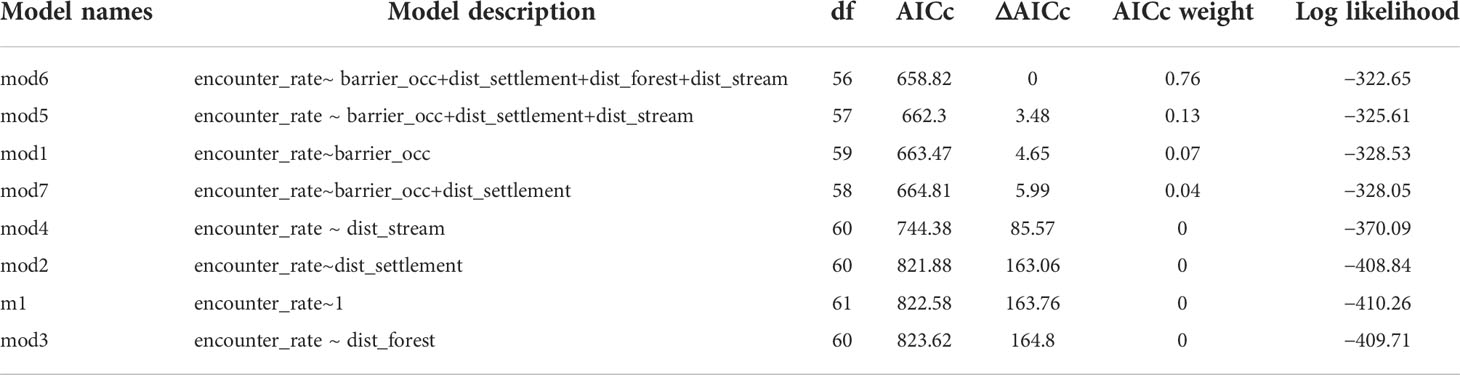
Table 3 Performed eight generalized linear models to check the effect of predictor variables on the encounter rates of elephant signs with their model estimates and AICc.
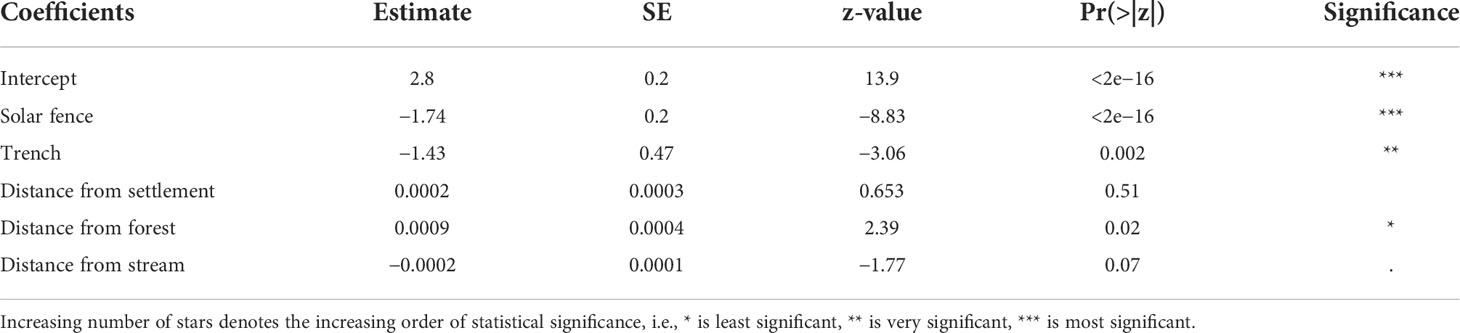
Table 4 Estimates of predictor variables towards the elephant encounter rate as per the least AICc-based best fit model (“mod6” global model).
People’s perceptions to physical barriers as a HEC mitigation measure
In areas having barriers, i.e., solar fence or trench, a total of 122 respondents were interviewed, out of which 44% people were uninterested in paying (“1,” protest zero), 3% people were unwilling due to low income (“2,” true zero), and remaining 53% were willing to pay (“3,” positive). Out of the 11 logistic regression models (Appendix 4), the model with the least AICc value (test_m2=wtp_cat~overall_powercut+human_elephant_conflict+net_loss+compensation+time_taken_compensation+change_ele_freq+change_cropraid_freq) suggested that as human–elephant conflict instances in the village persisted, people uninterested to pay were convinced to pay (𝛽=−6.2 ± 0.0003SE, p<0.05), but willingness to pay decreased with increasing HECs (𝛽=−4.4 ± 0.0003SE, p<0.05). It was found that if people get compensated, their unwillingness to pay decreased (𝛽=−19.7 ± 0.0006SE, p<0.05), and people became willing to pay (𝛽=20.7 ± 0.0006SE, p<0.05). However, if the payment of compensation took time, then unwillingness increased (𝛽=1.6 ± 0.02SE, p<0.05) and vice versa also held (𝛽=−0.06 ± 0.02SE, p<0.05). It was observed that with a change in elephant incursion frequency, both unwillingness (𝛽=−1.3 ± 0.0003SE, p<0.05) and willingness (𝛽=−1.1 ± 0.0003SE, p<0.05) of people to pay for maintenance of barrier reduced significantly. Furthermore, the change in crop-raiding frequency by elephants was found to have a negative relationship with the unwillingness of people to pay (𝛽= 0.1 ± 0.001SE, p<0.05); rather, people were willing to pay (𝛽=0.6 ± 0.001SE, p<0.05) (Table 5) (Figures 8, 9).
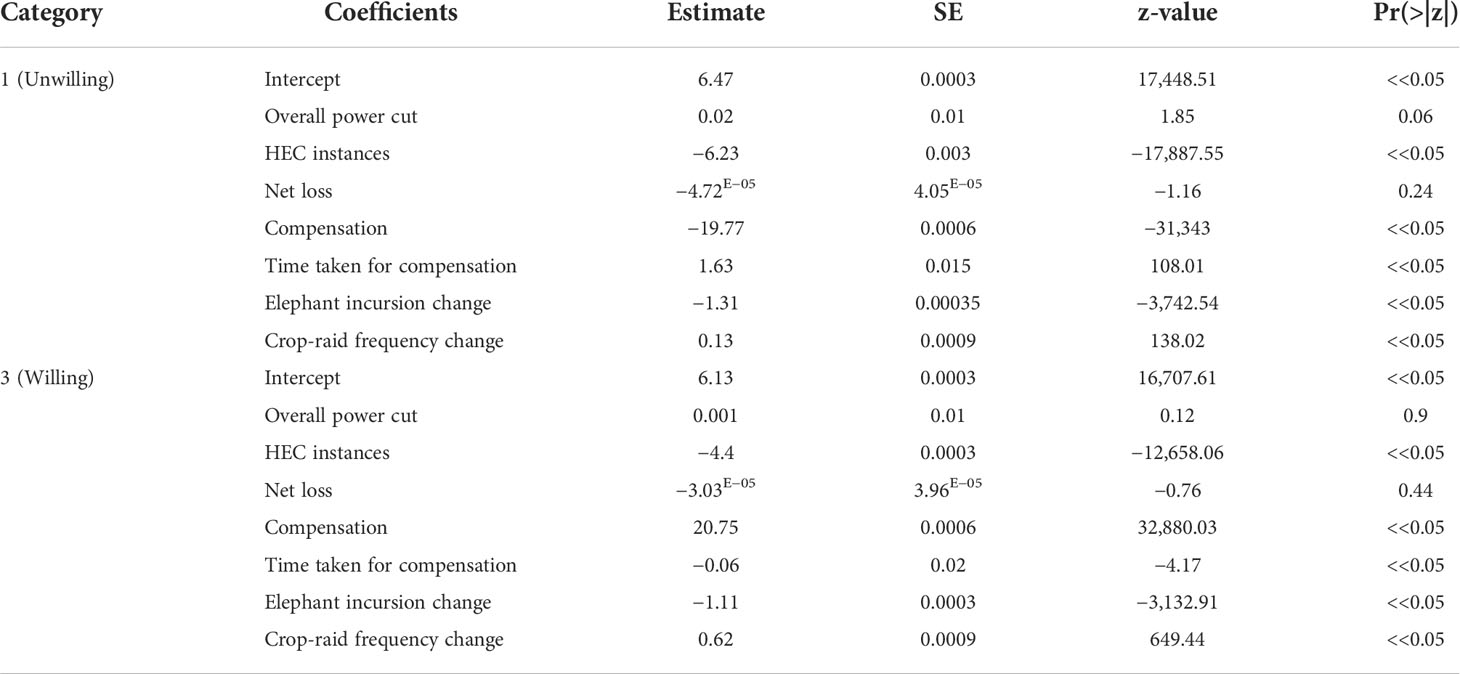
Table 5 Table representing estimates and level of significance of best fit multinomial logistic regression for describing the relationship with predictor variables and categorical variable of willingness to pay (“2”= true zero, as the reference category) on the barrier sites.
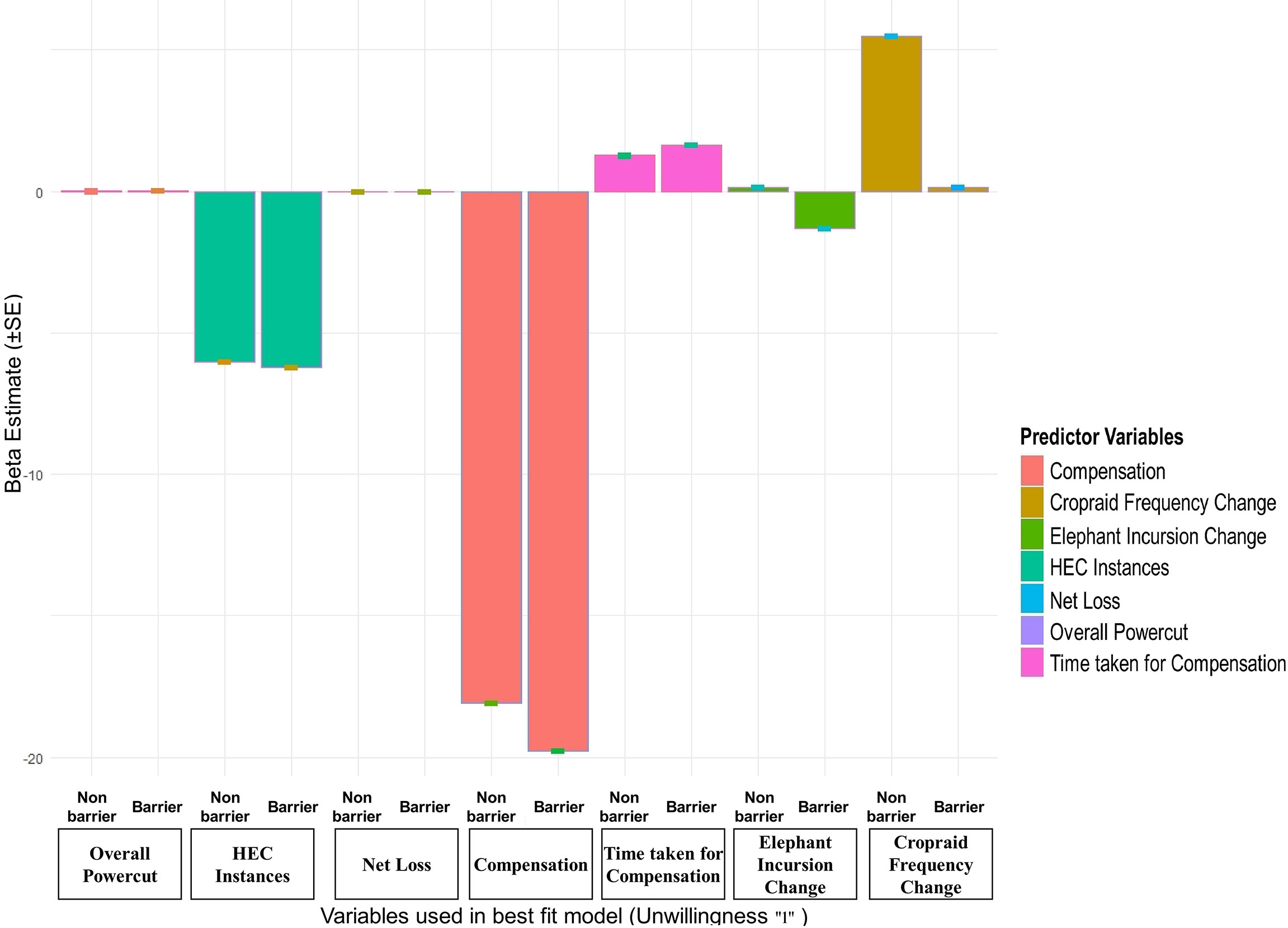
Figure 8 Comparisons of the relationship between beta estimates and different variables from least AICc model for category “1” (unwilling to pay for maintenance of barriers) for both barrier and non-barrier sites.
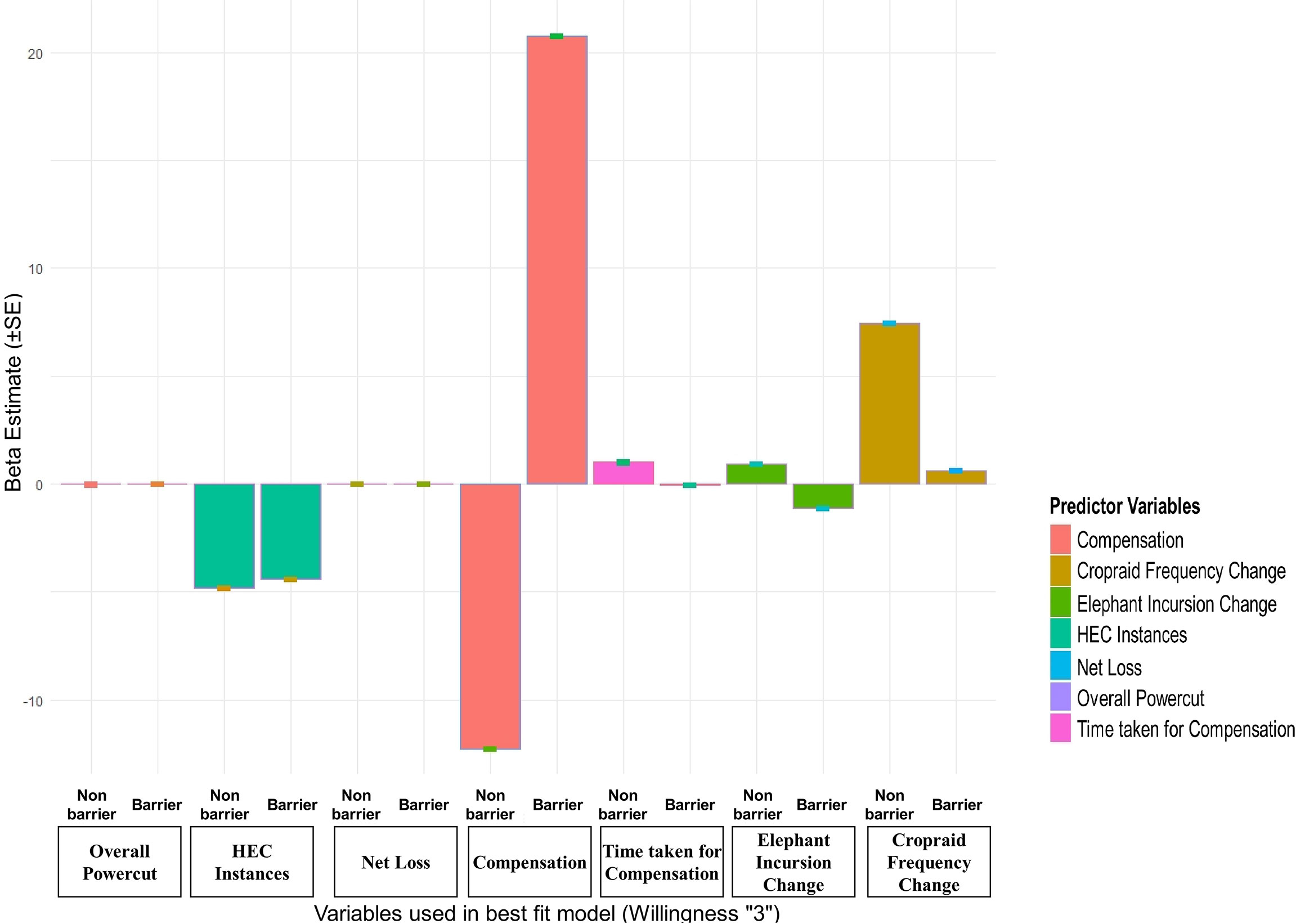
Figure 9 Comparisons of the relationship between beta estimates and different variables from least AICc model for category “3” (willing to pay for maintenance of barriers) for both barrier and non-barrier sites.
In the non-barrier sites, out of 127 respondents, 29% of them were unwilling to pay due to a lack of interest (“1,” protest zero), 7% people were unwilling due to low income (“2,” true zero), and the rest 64% were willing to pay (“3,” positive). Out of the 13 logistic regression models (Appendix 5), the best fit model (test_m2= wtp_cat ~ overall_powercut +human_elephant_conflict+net_loss+compensation+time_taken_compensation+change_ele_freq + change_cropraid_freq) suggested that as human–elephant conflict instances in the village persisted, uninterested people were convinced to pay (𝛽=−6.0 ± 0.0003SE, p<0.05), and willingness of people to pay decreased with increasing human–elephant conflicts (𝛽=−4.8 ± 0.0003SE, p<0.05). It was noted that if people got compensated, then both unwillingness (𝛽=−18.1 ± 0.0006SE, p<0.05) and willingness of people to pay decreased (𝛽=−12.27 ± 0.0006SE, p<0.05). However, if compensation payment took time, then the unwillingness (𝛽=1.26 ± 0.02SE, p<0.05) and willingness of people to pay increased (𝛽=1.01 ± 0.02SE, p<0.05). It was also observed that with a change in elephant incursion frequency, both unwillingness (𝛽=0.13 ± 0.001SE, p<0.05) and willingness (𝛽=0.9 ± 0.001SE, p<0.05) of people to pay increased significantly. Furthermore, with the change in crop-raiding frequency by elephants, the unwillingness of people increased (𝛽=5.4 ± 0.002SE, p<0.05), and people became more willing to pay (𝛽=7.4 ± 0.002SE, p<0.05) (Table 6) (Figures 8, 9).
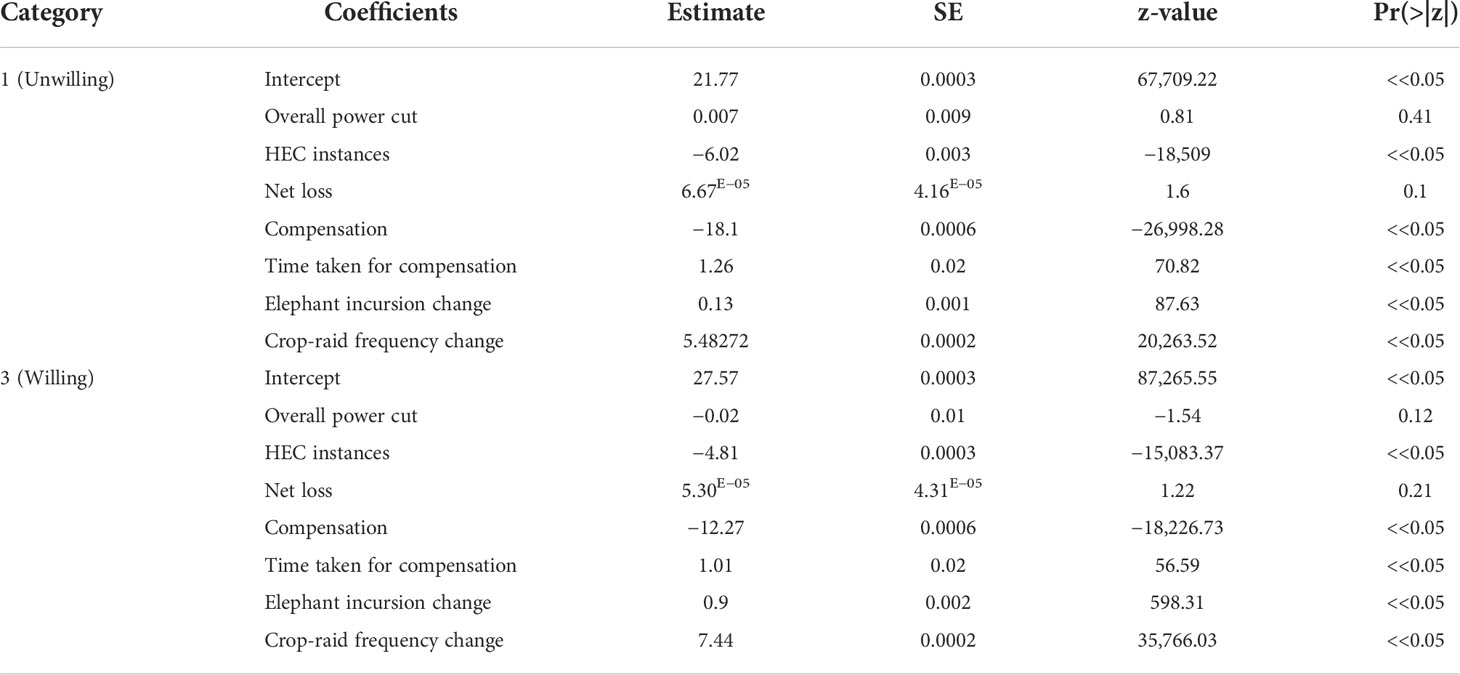
Table 6 Table representing estimates and level of significance of best fit multinomial logistic regression for describing the relationship with predictor variables and categorical variable of willingness to pay (“2” = true zero, as the reference category) on the non-barrier sites.
Variables such as overall power cut in the areas and net loss caused by the elephants did not significantly affect the willingness of people to pay for maintenance of the barriers. In addition, the average amount of money people were willing to pay from all three WTP categories for maintenance of existing barriers on the barrier sites was 18.4 ± 2.1 Indian Rupees (SE; 0–160 Indian Rupees). The mean amount of money people were willing to pay for the installation and subsequent maintenance of barriers on non-barrier sites was 27.9 ± 2.9 Indian Rupees (SE; 0–275 Indian Rupees).
Discussion
The optimized hotspot analysis showed an average of 16% increase in the emergence of high conflict hotspot villages from 2016 to 2017 and 2018 to 2019, including all types of HEC in the district of Golaghat. However, the number of conflict cases recorded from 2017 to 2019 sharply declined. These results can be attributed to either lack of conflict reporting or an actual decline in HEC cases due to the enhancement of traditional and personal mitigation methods, support from the forest department and non-governmental organizations, or the installation of physical barriers. Moreover, people in the area were observed investing in mitigation measures, such as mesh fences, lower wattage solar fences, makeshift watchtowers, group night guarding, and personal trenches around tea gardens, houses, and crop fields (personal observation by GD). The construction of a wall in 2011, followed by the felling of forested land in the No Development Zone (NDZ) for developmental activities by Numaligarh Refinery Limited (NRL), blocked the traditional moving paths of elephants (National Green Tribunal, 2016)2, attributing to decline in HECs. The presence of trenches in and around tea estates of Golaghat district and, likewise, the construction of a 2-km elephant-proof trench in the Bokial range of Lower Doigurng RF might be responsible for reducing conflict cases.
The high HEC hotspots were notably concentrated in clusters around the two reserved forests, i.e., Kaziranga on the western and Nambor Doigurung on the southwestern side, indicating less dispersion or shift in the extent of conflicts. These results were supported by the findings of Sukumar et al. (2020), showing an elephant density of 0.29 elephants per km2 in Karbi Anglong and Nagaon districts, including the Nambor Doigurung on the eastern side of Karbi-Anglong, while Kaziranga had 1.52 elephants per km2, which are relatively higher densities than other parts of Golaghat district. Sukumar et al. (2020) also showed records of the Golaghat district having elephant presence outside the forested areas but not beyond the high hotspot villages observed in this study. The low elephant presence and restricted conflict beyond these HEC hotspots, as shown in this study, can be due to the limited availability of forest cover and increasing density of urbanized areas with increasing distance from the protected areas, which are reported to be generally avoided by elephants (Evans et al., 2020; Sukumar et al., 2020).
This study showed a significant decline in encounter rates of elephants at barrier sites relative to the non-barrier sites, suggesting that the barriers help reduce conflicts, although not entirely (Neupane et al., 2018). The solar fence was relatively better than the trench and reduced the severity of the HEC instances. Previous studies suggested that mitigation measures, including physical barriers, should be implemented based on planned and scientifically based pilot surveys on the barrier’s performance (Rangarajan et al., 2010; Desai and Riddle, 2015). Likewise, in a study on the effectiveness of fences in Kenya, Kioko et al. (2008) suggested that the success of electric fences, or any physical barriers, should be based on on-site or region specificity, maintenance intervals, and proximity measures to highly dense elephant presence areas.
Assam lies in the floodplains of the Brahmaputra river, where siltation is a recurring issue. Therefore, the functioning of elephant-proof trenches is bound to fail if not maintained timely (Borah et al., 2005). Mitigation measures like trenches, although not entirely effective for regions with high rainfall, might prove to be effective if well maintained and used in combination with a vegetative barrier, such as cultivation of lemon or cane along the trench (Wahed et al., 2016; Government of India, 2017). Similarly, due to overcast weather in the monsoon season, an increase in water level during flooding may also limit the functioning of solar fences, which is often problematic to restore, as stated by the forest staff and locals.
This study concludes that with increased distance from the forest, detection of elephant signs increases, indicating movement of the elephants out of the protected areas. The Kaziranga (Nagaon and Golaghat districts), Karbi-Anglong, and Nagaland make up the Kaziranga–Karbi-Anglong Elephant Reserve holding a population of 2,318 elephants, the highest in the state of Assam (Sukumar et al., 2020). However, these protected areas are infested with invasive species of plants, such as Mimosa diplotricha var. inermis, Rosa multiflora, and Lantana camara, that are degrading the habitat quality of grasslands and leading to a decline in palatable forage for elephants (WII et al., 2007; Sukumar et al., 2020). Meanwhile, forest degradation due to human encroachment, illegal felling, and land-use conversion in Assam are leading to a decline in the habitat of elephants and are forcing elephants to move out of the protected areas in search of food and space (Choudhary, 2004; Rangarajan et al., 2010). Golaghat district offers diffuse boundaries between tea estates, crop fields, and forests; they often act as a refuge during elephant’s movement from smaller forest patches to extensive and more habitable disjunct forest patches. Therefore, these agroforestry mosaics can serve as an opportunity for initiating and escalating conservation efforts beyond protected areas (Kshettry et al., 2020).
The logistic regression models evaluating the relationship between the three categories of willingness or unwillingness of people to pay for the maintenance of the barriers were seen to be influenced by different predictor variables. People were mostly unwilling to pay, as they thought maintenance and enhancement of the barrier was the role of the local forest department. People do not want to get involved or cannot pay due to low income. In contrast, only those people were willing to pay who were mindful for conservation of wildlife and hence supported the idea of modern mitigation techniques (Whitehead, 1992; Hadker et al., 1997; Pate and Loomis, 1997). It is important to note that not much difference was seen in the non-barrier sites because as HEC instances increased, unwilling people felt the need for a barrier. However, on the contrary, people who were initially willing to pay, lose their hopes in the authorities’ ability and their interventions in controlling the issue, similar to Neupane et al. (2017). In addition, it was seen that when people got compensation, then unwilling people wanted to have a barrier. However, people willing to pay do not want to invest in a barrier if they are already getting compensated. Interestingly, with an increase in elephant incursion and crop-raiding frequencies, unwilling people did not want to pay as they distrusted any intervention methods. However, people who were willing to pay were optimistic that any barrier might reduce the issue.
Most locals expressed dissatisfaction with different types of physical barriers, often shaping their decision regarding willingness to pay for the maintenance of the barriers or installation of barriers. Moreover, it is advocated that the involvement and consent of local communities is a must prior to the installation of physical barriers. An agreement of consent on active involvement in the maintenance and financial support for maintenance should be provided to the local people to evoke interest in the maintenance work (Government of India, 2017; Neupane et al., 2017). This study also showed that the local communities often demand equal involvement in policy-level HEC management and intervention plans, which are repeatedly ignored. Providing equal stake to local people in decision-making enhances people’s perception and imparts a sense of association toward species conservation (Neupane et al., 2017). Otherwise, improving the compensation payment system by ensuring transparency, decreased complication in filling the application, and timely payment will enhance people’s attitude toward the species and species conservation, leading to support by the local people and their participation in mitigation interventions. Thus, strengthening and aiding communication gaps between locals and forest officials can smoothen the management process. Training forest staff on quick response techniques, developing cost-effective early warning systems, communication with locals, and barrier maintenance can improve the mitigation process.
A few caveats of this study must be considered for future endeavors in this research field. The study was conducted during the sowing season; hence, elephants did not have a strong incentive to come into agricultural areas. Therefore, a similar study should also be conducted during the crop harvest season to compare and interpret the results and their implications. Although villages were identified considering the most similar habitat characteristics among all, villages are not entirely comparable to each other, since there are many idiosyncratic circumstances that might affect the presence of elephant signs irrespective of the barrier treatment. In addition, in the case of equal elephant densities, more elephant signs could be expected alongside a barrier than in a site without a barrier, since elephants would be blocked by the barrier and likely spend more time there, inflating the number of signs in that area.
Management implications
For the successful management of human–elephant conflicts, it is suggested that proper planning, experimentation, and a suitable scientific approach should be programmed pre- and post-implementation of any mitigation measures. In Golaghat, the solar fences were relatively more effective than the trenches in mitigating HECs. Therefore, combining both and their proper maintenance can be an effective solution. Furthermore, the government should be involved in using and installing physical barriers with the help and support of local people, based on robust studies on elephant movement and identification of elephant-use areas. Improvement of the compensation system by the forest department of Golaghat forest divisions is essential for conflict management success and gaining the trust of local people.
Short focus group discussions with community representatives and experts suggested cost-effective HEC preventive methods such as cultivating non-preferred crops by elephants like okra, lemon, and bitter gourd. In addition, night guarding, makeshift cost-effective community fencing, use of noise, visual and chemical repellents, and lemon/cane cultivation as bio-fences at the forest–village interface can drive away elephants. Installing hanging solar fences (Wildlife Trust of India, 2020)3, using kumkis (trained captive elephants) for driving away crop-raiding elephants into forests, and forming community-based anti-depredation squads (ADS) (WF-India, 2020) can notably reduce HECs. Forest department, non-government organizations (NGOs), and other organizations should associate with the local people to also use electric low-cost infrasonic audio detectors, which can act as early warning systems (Sayakkara et al., 2017). The abovementioned will lead to successful, sustainable, reliable, and long-term conflict prevention (Distefano, 2005; Wahed et al., 2016).
Appendices are provided in table format in the supplementary material WORD file (Appendices, Supplementary Material).
Data availability statement
The original contributions presented in the study are included in the article/Supplementary Material. Further inquiries can be directed to the corresponding author.
Ethics statement
This study was reviewed and approved by Wildlife Institute of India. The participants provided their verbal informed consent to participate in this study.
Author contributions
GD conceptualized the theory. All authors conceived the framework of the study. GD and GVG discussed and designed the methodology of the study. GVG, KS and BL provided necessary logistic support. GD performed data collection, data processing, spatial analysis, and validation. GVG, KS and BL did a major discussion of results and recommendations. GD wrote the manuscript. GVG, KS and BL supervised the finding of this work and manuscript improvement. GVG, KS and BL provided a factual review of the manuscript. All authors contributed to thearticle and approved the submitted version.
Funding
GD is a Masters student at the Wildlife Institute of India (WII), Dehradun. This study was conducted as part of her Master’s dissertation from WII. GD would like to thank the institute for supporting her research.
Acknowledgments
We thank the Director and Dean of WII for their support while carrying out this study. We thank Dr. N. Laxminarayanan of WII for his help and inputs to this work. We are grateful for all the guidance from faculties and researchers of the Wildlife Institute of India. We express our gratitude toward Assam Forest Department and all involved personnel of Kaziranga National Park and Nambor-Doigurung Wildlife Sanctuary for the logistic support and field guidance. The authors are indebted to all the field assistants, field collaborators, drivers, and local people of Golaghat district for their time, help, patience, and valuable inputs.
Conflict of interest
The authors declare that the research was conducted in the absence of any commercial or financial relationships that could be construed as a potential conflict of interest.
Publisher’s note
All claims expressed in this article are solely those of the authors and do not necessarily represent those of their affiliated organizations, or those of the publisher, the editors and the reviewers. Any product that may be evaluated in this article, or claim that may be made by its manufacturer, is not guaranteed or endorsed by the publisher.
Supplementary material
The Supplementary Material for this article can be found online at: https://www.frontiersin.org/articles/10.3389/fcosc.2022.956568/full#supplementary-material
Footnotes
- ^ https://www.wti.org.in/projects/right-of-passage/na
- ^ http://www.indiaenvironmentportal.org.in/files/Numaligarh%20refinery%20Kaziranga%20NGT%20Order.pdf
- ^ https://www.wti.org.in/news/hanging-fences-protect-people-and-elephants-in-manas/
References
Agarwal P. (2015) 350 deaths a year, thousands of hectares damaged in man-elephant conflict. Available at: https://timesofindia.indiatimes.com/india/350-deaths-a-year-thousands-of-hectares-damaged-in-man-elephant-conflict/articleshow/49915536.cms?from=mdr (Accessed July 18, 2022).
Akhtar M. (2021) Hundreds of casualties on both sides in world capital of human-elephant conflict. Available at: https://earthjournalism.net/stories/hundreds-of-casualties-on-both-sides-in-world-capital-of-human-elephant-conflict (Accessed May 12, 2022).
Blanc J. J., Thouless C. R., Hart J. A., Dublin H. T., Barnes R. F. W. (2003)An update from the African database. ICCN/SSC. In: African Elephant specialist group (U.K: IUCN, Gland, Switzerland and Cambridge). Available at: https://portals.iucn.org/library/efiles/documents/ssc-op-029.pdf (Accessed April 05, 2022).
Borah J., Thakuria K., Baruah K. K., Sarma N. K., Deka K. (2005). Man-elephant conflict problem: A case study. Zoos’ Print J. 20 (7), 22–24. Available at: http://www.zoosprint.org/ZooPrintMagazine/2005/July/22-24.pdf (Accessed on September 13, 2022).
Burnham K. P., Anderson D. R. (2004). Multimodel inference: Understanding AIC and BIC in model selection. Sociological Methods Res. 33 (2), 261–304. doi: 10.1177/0049124104268644
Campbell-Smith G., Sembiring R., Linkie M. (2012). Evaluating the effectiveness of human-orangutan conflict mitigation strategies in Sumatra. J. Appl. Ecol. 49, 367–375. doi: 10.1111/j.1365-2664.2012.02109.x
Centers for Disease Control and Prevention (2008) A guide to conducting household surveys for water safety plans. Atlanta: U. s. department of health and human services. Available at: https://www.cdc.gov/nceh/ehs/gwash/publications/guide_conducting_household_surveys_for_water_safety_plans.pdf (Accessed May 21, 2022).
Champion H. G., Seth S. K. (1968). “A revised forest types of India,” in Manager of publications (New Delhi: Government of India Press).
Choudhary A. (2004). Human–elephant conflicts in northeast India. Hum. Dimensions Wildlife: Int. J. 9 (4), 261–270. doi: 10.1080/10871200490505693
Das J. P., Lahkar B. P., Talukdar B. K. (2012)Increasing trend of human elephant conflict in golaghat district, Assam, India: Issues and concerns (Accessed July 08, 2021).
Desai A., Riddle H. (2015) Human-elephant conflict in asia. U.S fish and wildlife service. Available at: https://www.fws.gov/international/pdf/Human-Elephant-Conflict-in-Asia-June2015.pdf (Accessed June 10, 2021).
Distefano E. (2005)Human-wildlife conflict worldwide: Collection of case studies, analysis of management strategies and good practices. In: SARD initiative report (Rome: FAO) (Accessed June 01, 2021).
Dunn K. (2000). Qualitative research methods in human geography. Ed. Hay I. (Oxford, UK: Oxford University Press).
Evans L. J., Goossens B., Davies A. B., Reynolds G., Asner G. P. (2020). Natural and anthropogenic drivers of bornean elephant movement strategies. Global Ecol. Conserv. 22, 2351–9894. doi: 10.1016/j.gecco.2020.e00906
Fernando P., Kumar M. A., Williams A. C., Wikramanayake E., Aziz T., Singh S. M. (2008) Review of human-elephant conflict mitigation measures practiced in south asia. AREAS technical support document submitted to world bank, world wide fund for nature. Available at: http://awsassets.panda.org/downloads/review_of_human_elephant_final_reduced_01.pdf (Accessed November 27, 2020).
Getis A., Ord J. K. (1992). The analysis of spatial association by use of distance statistics. Geographical Anal. 24 (3), 189–206. doi: 10.1111/j.1538-4632.1992.tb00261.x
Government of Assam, Department of Environment & Forests (2011) Assam Forests at a glance. government of Assam (Accessed November 27, 2020).
Government of Assam, Golaghat District (2022) Population. Available at: https://golaghat.gov.in/about-us/population (Accessed May 10, 2022).
Government of India (2017) Guidelines for management of human elephant conflicts. Available at: http://moef.gov.in/wp-content/uploads/2017/08/HEC-management-guideline-Final1.pdf (Accessed July 12, 2021).
Hadker N., Sharma S., David A., Muraleedharan T. R. (1997). Willingness-to-pay for borivli national park: evidence from a contingent valuation. Ecol. Econ 21, 105–122. doi: 10.1016/S0921-8009(96)00094-8
Indian Council of Agricultural Research (2020) National agricultural scenario. Available at: https://icar.org.in/files/state-specific/chapter/3.htm (Accessed July 14,2021).
Indian State Forest Report (2019) Forest survey of india. edition 16, volume 1. Available at: https://fsi.nic.in/isfr19/vol1/cover-page.pdf (Accessed May 13, 2022).
Inskip C., Zimmermann A. (2009). Human-felid conflict: A review of patterns and priorities worldwide. Oryx 43, 18–34. doi: 10.1017/S003060530899030X
Jadhav R. (2020) Every day, India loses one person to human-elephant conflict. Available at: https://www.thehindubusinessline.com/data-stories/data-focus/every-day-india-loses-one-person-to-human-elephant-conflict/article33330536.ece (Accessed May 05, 2022).
Kalinic M., Krisp J. M. (2018). Kernel density estimation (KDE) vs. hot-spot analysis–detecting criminal hot spots in the city of San Francisco Proceeding of the 21st conference on geo-information science. AGILE 2018-Lund. Available at: https://www.agile-online.org/images/conferences/2018/documents/shortpapers/66%20Kernel%20Density%20Estimation%20(KDE)%20vs.%20Hot-Spot%20Analysis%20-%20Detecting%20Criminal%20Hot%20Spots%20in%20the%20City%20of%20San%20Francisco_UPDATE.pdf (Accessed on September 12, 2022).
Karanth K. K., Kudalkar S. (2017). History, location, and species matter: Insights for human–wildlife conflict mitigation from India. Hum. Dimensions Wildlife. 22, 331–346 doi: 10.1080/10871209.2017.1334106
Kassambara A. (2021) ). rstatix: Pipe-friendly framework for basic statistical tests. Available at: https://CRAN.R-project.org/package=rstatix R package version 0.3.0 (Accessed July 12, 2021).
Kioko J., Muruthi P., Omondi P., Chiyo P. I. (2008). The performance of electric fences as elephant barriers in amboseli, Kenya. South Afr. J. Wildlife Res. 38, 52–58. doi: 10.3957/0379-4369-38.1.52
Kshettry A., Vaidyanathan S., Sukumar R., Athreya V. (2020). Looking beyond protected areas: Identifying conservation compatible landscapes in agro-forest mosaics in north-eastern India. Global Ecol. Conserv. 22, e00905. doi: 10.1016/j.gecco.2020.e00905
Locke P., Buckingham J. (Eds.). (2016)Conflict, negotiation, and coexistence: Rethinking human–elephant relations in South Asia (Oxford University Press). Available at: https://oxford.universitypressscholarship.com/view/10.1093/acprof:oso/9780199467228.001.0001/acprof-9780199467228 (Accessed July 18, 2022).
Meiyappan P., Roy P. S., Soliman A., Li T., Mondal P., Wang S., et al. (2018). India Village-level geospatial socio-economic data set: 1991, 2001. palisades, NY: NASA socioeconomic data and applications center (SEDAC). doi: 10.7927/H4CN71ZJ
MoEFCC (2017) All India synchronized elephant population estimation. report, ministry of environment, forest and climate change. Available at: http://www.indiaenvironmentportal.org.in/content/446218/synchronized-elephant-population-estimation-india-2017/ (Accessed July 12, 2021).
Naha D., Dash S. K., Chettri A., Roy A., Sathyakumar S. (2020). Elephants in the neighborhood: Patterns of crop-raiding by Asian elephants within a fragmented landscape of Eastern India. PeerJ 8, e9399. doi: 10.7717/peerj.9399
Naha D., Sathyakumar S., Dash S., Chettri A., Rawat G. S. (2019). Assessment and prediction of spatial patterns of human-elephant conflicts in changing land cover scenarios of a human-dominated landscape in north Bengal. PloS One 14 (2), e0210580. doi: 10.1371/journal.pone.0210580
National Green Tribunal (2016) Before the national green tribunal. Available at: http://www.indiaenvironmentportal.org.in/files/Numaligarh%20refinery%20Kaziranga%20NGT%20Order.pdf (Accessed July 12, 2021).
Naughton L., Rose R., Treves A. (1999) The social dimensions of human–elephant conflict in Africa: a literature review and case studies from Uganda and cameroon. report to HEC task force, AFESG, IUCN, gland, Switzerland. Available at: https://www.iucn.org/sites/dev/files/import/downloads/hecugcarev.pdf (Accessed June 05, 2021).
Nelson A., Bidwell P., Sillero-Zubiri C. (2003)A review of human elephant conflict management strategies. In: People and wildlife initiative (Wildlife Conservation Research Unit, Oxford University) (Accessed January 05, 2021).
Neupane D., Johnson R. L., Risch T. S. (2017). How do land-use practices affect human-elephant conflict in Nepal? Wildlife Biol. 4 doi: 10.2981/wlb.00313
Neupane B., Khatiwoda B., Budhathoki S. (2018). Effectiveness of solar-powered fence in reducing human-wild elephant conflict (HEC) in northeast jhapa district, Nepal. Forestry: J. Institute Forestry Nepal 15, 13–27. doi: 10.3126/forestry.v15i0.24917
Osborn F. V., Parker G. E. (2002). Community based methods to reduce crop loss to elephants: experiments in the communal lands of Zimbabwe. Pachyderm 33, 32–38. Available at: https://www.researchgate.net/publication/284463354_Community-based_methods_to_reduce_crop_loss_to_elephants_Experiments_in_the_communal_lands_of_Zimbabwe (Accessed on September 13, 2022).
Pate J., Loomis J. (1997). The effect of distance on willingness to pay values: A case study of wetlands and salmon in California. Ecol. Economics 20, 199–207. doi: 10.1016/S0921-8009(96)00080-8
Ramkumar K., Ramakrishnan B., Saravanamuthu R. (2013). Human-elephant (Elephas maximus) conflict in southern India: People's perception on conflict and elephant conservation in coimbatore forest division. J. Sci. Trans. Environ. Technovation 7 (2), 69–76. doi: 10.20894/STET.116.007.002.002
Rangarajan M., Desai A. A., Sukumar R., Easa P. S., Menon V., Vincent S., et al. (2010)Gajah. securing the future of elephants in india. the report of the elephant task force. In: Ministry of environment and forests, government of India. Available at: http://www.environmentandsociety.org/node/2697 (Accessed November 25, 2020).
Ripley B., Venables W. (2021) Nnet: Feed-forward neural networks and multinomial log-linear models. r package version. Available at: https://cran.r-project.org/web/packages/nnet/index.html (Accessed July 05, 2021).
Rose D. C., Brotherton P., Owens S., Pryke T. (2018). Honest advocacy for nature: Presenting a persuasive narrative for conservation. Biodivers Conserv. 27, 1703–1723. doi: 10.1007/s10531-016-1163-1
Sayakkara A. P., Jayasuriya N., Ranathunga T., Suduwella C., Vithanage N., Keppitiyagama C., et al. (2017). “Eloc: Locating wild elephants using low-cost infrasonic detectors,” in 2017 13th international conference on distributed computing in sensor systems (DCOSS)(Ottawa, ON, Canada, Institute of Electrical and Electronics Engineers (IEEE)) 44–52. doi: 10.1109/DCOSS.2017.34
Sengupta A., Binoy V. V., Radhakrishna S. (2020). Human-elephant conflict in kerala, India: A rapid appraisal using compensation records. Hum. Ecol. 48 (6), 101–109. doi: 10.1007/s10745-020-00128-6
Sukumar R., Varma. S., Francis Ishmael S. A., Goswami A. A., Chatterjee S., Srinivasaiah N., et al. (2020). “Status and distribution of elephants in India- 2017,” in Asian Nature conservation foundation (ANCF) (New Delhi: Bengaluru, and Project Elephant Division, Ministry of Environment, Forest and Climate Change).
The World Bank (2020) Population density (people per sq. km of land area)-India. Available at: https://data.worldbank.org/indicator/EN.POP.DNST?locations=IN (Accessed July 19, 2022).
Tripathy B. R., Liu X., Songer M., Kumar L., Kaliraj S., Chatterjee N. D., et al. (2021). Descriptive spatial analysis of human-elephant conflict (HEC) distribution and mapping HEC hotspots in keonjhar forest division, India. Front. Ecol. Evol. vol.9. doi: 10.3389/fevo.2021.640624
Venkataraman A., Kumar V., Varma S., Sukumar R. (2002). Conservation of a flagship species:Prioritizing Asian elephant (Elephas maximus) conservation units in southern India. Curr. Sci. 82 (2), 1022–1023. Available at: http://eprints.iisc.ac.in/12294/1/Conservation_of_a_flagship_species.pdf (Accessed on September 13, 2022).
Wahed M. A., Ullah M. R., Irfanullah H. (2016) Human-elephant conflict mitigation measures: Lessons from bangladesh. IUCN, international union for conservation of nature, Bangladesh country office, Dhaka, Bangladesh. Available at: https://portals.iucn.org/library/sites/library/files/documents/2016-068.pdf (Accessed June 06, 2021).
WWF-India (2020) WWF-india’s interventions. Available at: https://www.wwfindia.org/about_wwf/priority_species/indian_elephant/interventions/ (Accessed Nov 10, 2020).
Whitehead J. C. (1992). Ex ante willingness to pay with supply and demand uncertainty: Implications for valuing a sea turtle protection programme. Appl. Economics 24, 981–988. doi: 10.1080/00036849200000075
WII (Wildlife Institute of India), UNESCO, UNF, IUCN, University of Queensland(2007)Final management effectiveness evaluation report, kaziranga national park, Assam, India. Available at: https://www.iucn.org/sites/dev/files/import/downloads/kaziranga_second_eoh_assessment_nov07.pdf (Accessed November 27, 2020).
Wildlife Trust of India (2019) Right of passage: National elephant corridors project. Available at: https://www.wti.org.in/projects/right-of-passage/ (Accessed November 27, 2020).
Wildlife Trust of India (2020) Hanging fences protect people and elephants in manas. Available at: https://www.wti.org.in/news/hanging-fences-protect-people-and-elephants-in-manas/ (Accessed July 21, 2022).
Keywords: Physical barriers, solar fencing, elephant-proof trench, people’s perception, optimized hotspot analysis, willingness to pay, human-elephant conflict (HEC)
Citation: Das G, Selvan K, Lahkar B and Gopi GV (2022) Effectiveness of physical barriers in mitigating human–elephant negative interactions in North-East India. Front. Conserv. Sci. 3:956568. doi: 10.3389/fcosc.2022.956568
Received: 30 May 2022; Accepted: 01 September 2022;
Published: 21 September 2022.
Edited by:
Ahimsa Campos-Arceiz, Center for integrative Conservation, (CAS), ChinaReviewed by:
Pranaya Swain, National Institute of Science Education and Research (NISER), IndiaJohn Kiringe, School for Field Studies, United States
Copyright © 2022 Das, Selvan, Lahkar and Gopi. This is an open-access article distributed under the terms of the Creative Commons Attribution License (CC BY). The use, distribution or reproduction in other forums is permitted, provided the original author(s) and the copyright owner(s) are credited and that the original publication in this journal is cited, in accordance with accepted academic practice. No use, distribution or reproduction is permitted which does not comply with these terms.
*Correspondence: Govindan Veeraswami Gopi, Z29waWd2QHdpaS5nb3YuaW4=