- Stanford Existential Risks Initiative, Center for International Security and Cooperation, Freeman Spogli Institute for International Studies, Stanford University, Stanford, CA, United States
Humanity faces a myriad of existential technology, geopolitical, and ecological risks. The paper analyzes the possibility that negative shocks superimpose and multiply their effects, leading to catastrophic macro-dynamics. Methodologically, this paper presents a rare, quantitative scenario model superimposed upon narrative scenarios where the cascading economic effects of 19 quantitative indicators of growth or decline are projected into 2075. These indicators map onto five narrative scenarios, and are subsequently re-combined to study effects of plausible cascading risk events coming to pass in the 50 years period between 2025 and 2075. Results indicate that even in the case of deeply catastrophic singular events, the world would eventually recover within 25 years, as has historically been the case. The exception is that in the event of several catastrophic events in short order around the midpoint of the 50-year scenario timeline, the cascading risk escalation would create formidable negative cascades. The possibility of a protracted depression and no visible recovery within 25 years is the result. However, if we assume a modest interaction effect, even with just 3-5 co-occurring catastrophes, the result becomes a path towards humanity’s extinction based on economic decline alone. The implications are that humanity either needs to avoid significant cascading effects at all costs or needs to identify novel ways to recover compared to previous depressions. Given the amount of model assumptions they rely upon, these projections carry a degree of uncertainty. Further study should therefore be conducted with a larger set of indicators and impacts, including mortality modeling, to assess how much worse plausible real-world outcomes might be compared to the simplified economic model deployed here.
1 Introduction
We are said to live in a risk society (Beck, 1992). As risks to further societal development compound, crafting and studying future risk scenarios is an important challenge for scholarship and a responsibility for governance in the years to come. Scenarios have been developed for the future of energy (Bentham, 2014), emerging technologies such as AI (Popkova et al., 2020), but also explicitly for single worry tech-derived risks, such as nuclear risk (RECNA, 2021), biosecurity (Nelson et al., 2021), and AI risk (Turchin and Denkenberger, 2020). However, even if scenario planning has a long history, including with a fairly rigorous analysis of driving forces, its delivery format tends to be qualitative descriptions (“narratives”). Perhaps because of the end format, there is a large degree of variability in the methods used, at times described as “methodological chaos,” and the quantitative underpinnings of its methodology are rarely systematically explored (Cordova-Pozo and Rouwette, 2023). As a consumer of scenarios, this often brings doubt as to whether quantitative models were used in any way.
Notable exceptions to this situation are found within the technology forecasting literature which wholeheartedly embraces the use of quantitative indicators to extrapolate historical trends, particularly to forecast economic growth, R&D patterns, the maturity and impact of emerging technologies, sectoral industrial development, and more (Parraguez et al., 2020; Tsai et al., 2023). That tradition uses a multiplicity of methods, including trend extrapolation (Tsai et al., 2023), Delphi surveys (Beiderbeck et al., 2021), and technology roadmapping (Halal, 2013; Cho, 2018), and also a multitude of data sources, including expert interviews or questionnaires, patent data, research publication data, economic indicators, online platform data, and more (Feng et al., 2022). Scholars have also tried to model the risk of future natural hazards based on future exposure and vulnerability of infrastructure in addition to current context and historical hazard data (Cremen, Galasso and McCloskey, 2022). Another quantitative tradition includes environmental forecasting, notably as seen in the early Limits To Growth (Aigner-Walder and Döring, 2022; Meadows et al., 1974), as well as in the work of the UN Climate Panel, the IPCC (De Pryck et al., 2022), although recently the IPCC also includes a limited use of storyline-based scenarios which some advocate could significantly improve both scenario construction and end user comprehension (Shepherd et al., 2018; Sillmann et al., 2021). Taleb analyzed the misuse of statistical normal distributions which leads to ignoring the possibility of, and not preparing for, high-impact, yet extreme, statistically unlikely (outliers), unexpected events, yet pretending such events can easily be explained (an imagined “retrospective predictability”) after the fact (Taleb, 2014; Taleb, 2007). Taleb, using his characteristic hyperbole, claims Black Swans explain almost everything in our world from religion, via historical events, to our personal life, and give the examples of 11 September 2001 and the Pacific tsunami of 2004 (Taleb, 2007). A quantitative definition of Black Swans has been lacking but using the Zipf-Mandelbrot law, an interesting paper uncovers a generative mechanism so as to classify extreme events into White, Grey or Black Swans, and might in turn declassify events commonly considered as such, including COVID-19, but would include Canadian wildfires, soccer player Lionel Messi’s goal performance, and Turkish Airlines Flight 981 based on a quantitative cutoff that depends on an initial sample to enable an estimate (De Marzo et al., 2022). However, a different class of costly and large scale (“king-like”) yet improbable (“dragon-like”) events (so-called “dragon-kings”) including natural hazards, cyberattacks, and financial events, also exist (Sornette, 2009). These may have properties that make them identifiable in real time but also theoretically predictable, or at least subject to complex dynamics systems modeling, and as a result, possibly suppressible (Masys et al., 2016). Unfortunately, dragon-king events are with us all the time and ‘catastrophes’ are not rare in the sense that they nearly don’t happen at all (Sornette, 2009).
Lastly, Blackrock, the investment company, uses a geopolitical risk dashboard with quantitative components based on the market attention and movements towards risk events (BlackRock, 2023).
A key finding across the literature examining the possibilities and challenges around macro level systemic shocks is that the co-occurrence of adverse shocks generates irreversible qualitative change because of a multiplication of impacts (West, 2018). The claim from system theorists is indeed that we live in an exponentially expanding socio-economic Universe where capitalism (GDP, population, etc.) is not growing at an exponential rate but at a super-exponential rate, where the exponent itself is increasing with time (West, 2018). What follows is that within the foreseeable future, some claim by 2050 ± 10 (Sornette, 2017), a qualitative phase transition in socio-economic systems might occur if this entropy model of complex adaptive systems holds (Bossomaier et al., 2013). Both positive multiplicative effects (super-exponential growth) for example, as observed in the superlinear scaling of some startups (Bohan et al., 2024) and negative multiplicative effects (unprecedented adverse shocks) could arise out of finite-time singularity (Johansen and Sornette, 2001). Others are more skeptical that singularity, within the context of economic growth theory, is near because it presumably implies that AI could encompass all human activities including lay hands on patients, read bedtime stories to children, and change flat tires and a variety of non-routine tasks (Nordhaus, 2021). The theory goes rapid growth in AI will (at some point) cross some boundary after which economic growth will accelerate sharply as an ever-accelerating pace of improvements cascade through the economy. However, that assumes the utter and complete substitutability between information and conventional inputs. The task of protecting humans from AIs might become a labor in itself bigger than the defense sector (Nordhaus, 2021).
To study extreme events in dynamical systems, mathematical models, machine learning, and nonlinear dynamical networks are now used, trying to establish their statistical properties and potential generic processes preceding the ensuing chaos (Nag Chowdhury et al., 2022). Understanding the role of random walkers, that is processes consisting of a sequence of steps each of whose characteristics such as magnitude and direction (seems to be) determined by chance, in spatial networks of concern, such as mobility networks, the Internet, mobile phone networks, power grids, social and contact networks, and neural network, and particularly how they evolve over time, is immensely challenging (Barthélemy, 2011).
Mathematically, the scaling behaviors in the form of X∼N۲ capture the fact that some macroscopic variables X scale with the system size N, where N represents the number of entities in a networked system and based on empirical observations of the exponent ۲, two categories, super-linear and sublinear scaling behaviors, have been identified (Zhang et al., 2015). For example, as a city grows, the total number of interactions increases at a faster rate, leading to more wealth and innovation per capita, but also with more crime and pollution as side effects (Zhang et al., 2015; Schläpfer et al., 2014). Similarly, although the social dynamics of financial networks are a fundamental source of systemic risk, we know little about their role because they tend to be modeled from synthetic networks with limited empirical properties (Kobayashi and Takaguchi, 2018). One challenge common to all models of super-exponential growth, such as Theory of the Adjacent Possible (TAP) equation, is the tendency to overestimate the number of new artifact types. The reason is that one does not take into account the fact that interactions, among existing types, may produce types which have already been previously discovered. To rectify that, we may need to use binary string models where new string types can be generated by interactions among (at most two) already existing types (Villani and Serra, 2023).
The present article contributes to the current body of knowledge in that it conducts a rare quantitative scenario modeling exercise within custom-developed narrative scenarios, and so provides a rare combination of the narrative and quantitative traditions of scenario production. It is also relatively unique in that it combines the analysis of the modeled effects of a plethora of emerging technologies, but also includes climate change effects, health effects, as well as sociopolitical effects.
The research aim of the present article is to investigate factors associated with the quantitative modeling of emerging and future tech-derived existential risks (x-risks), which is important for the way technology and innovation is developed, regulated, and governed, affecting both private sector innovators and public sector funders and legislators. The research goal is to clarify the cumulative impact of two or more risks that co-occur and amplify each other. This will be carried out using quantitative models simulating five different scenarios of such amplified, or cascading risks. The research question is the following: how many co-occurring, significant hazards would it take to put the world on a path towards humanity’s extinction? To be clear, the way this is empirically modeled, we are looking at steep downward spirals on the path towards possible extinction, typical of the fall of historical civilizations, we are not necessarily describing scenarios all the way to extinction as such.
The key hypotheses include that (I) two or more hazards would drastically increase the odds but that (II) a plethora of hazards would have to co-occur for x-risks to materialize, which means (III) X-risk events are exceedingly rare, possibly inconceivable in the foreseeable future. The null hypothesis would be that there is no significant relationship between the number of hazards and the appearance of X-risk events, which would occur if even a large number of modeled hazards did not drastically alter humanity’s economic, political, technological, social, or ecological development trajectory.
The dependent variables are each specific event chain (modeled through scenarios), and the independent variables being controlled in each model scenario are specific driving forces of risk, from three main domains, emerging technology, ecology, and sociopolitics, as specified below.
The five narrative scenarios we had custom designed for our study covered cascading risks, specifically meaning “risks that amplify each other,” from emerging technology such as AI, nuclear, bio, nano, and quantum as well as from their industrialization, ecological risks such as pandemics, biodiversity, and climate change, and sociopolitical risks such as geopolitics, organized crime, terrorism, and social movements. More precisely, we define cascading risks as connected, overlapping risks, forming cumulative, co-causal chains of events, resulting in accumulated damage, including contagion to other, related systems. This understanding is consistent with the notion of technological change as a combinatorial process (Parraguez et al., 2020).
The fall of the Mayan (250–900) and Roman civilizations (27 BC-476 AD), the fall of the British Empire (1497–1947) or the end of the Third Reich (1933–1945) are some historical examples of negative cascades. In all those cases, a multitude of factors (drought, population dynamics, epidemics, tech, culture, natural resource access, social movements, corruption, economic downturn, wars, alliances) are believed to have been in play for downfall to occur over the course of multiple decades (Fernandez-Armesto, 1995; Landes, 1999; Fernandez-Armesto, 2002; Ferguson, 2004; Gibbon and Trevor-Roper, 2010; Shirer and Rosenbaum, 2011; Diamond, 2017). Today, because of globalization, technology, and general resource depletion, those timelines are, arguably, sped up and such negative cascading trajectories could likely happen within a decade alone. The relative change in historical speeds of social change is contested, given that every generation experiences some level of change (Kavanagh et al., 2021).
In modern times, the starting point for any discussion of cascading effects is the financial sector. As a case in point, during the Great Depression, real output in the United States fell nearly 30 percent, with widespread bank failures and bankruptcies by businesses and households, severely affecting the global financial system for about 5 years from 1931 to 1935, even after extensive government intervention and assistance (Bernanke, 1983).
More broadly, cascading, systemic risks are an emerging class consisting of a mix of large and medium-size risks that, emerging from disaster science (Mc Gee et al., 2014; Lucas et al., 2018; Zuccaro et al., 2018; Alexander and Pescaroli, 2019; Schweizer and Renn, 2019; Schweizer, 2021), represents an ongoing challenge to several established fields within risk science, and across the domains that touch on systemic phenomena, because of the complex ways these risks potentially interact and amplify each other. Specific cascading effects studied include inter-ecological cascades (Kemp et al., 2022), interdependency across systems such as volcanic ash, transportation, and supply chains in the case of the 2010 Eyjafjallajökull volcano eruption on Iceland (Alexander and Pescaroli, 2019), or natural disasters triggering industrial disasters during Hurricane Harvey in Texas in 2016 (Alexander and Pescaroli, 2019). A magnitude scale (L0-4) for cascades has been developed where the most severe causes the longest cascade chains (Alexander, 2018).
The sheer scale and magnitude of cascading risk chains that would threaten humanity as a whole are, admittedly, at a different level than disaster research typically engages with. In fact, the most relevant extinction scenarios chosen for this study, based on preliminary research, are: (1) Climate Cataclysm, (2) World War III, (3) Growth and Collapse, (4) Runaway AI, and (5) Synthetic Biology Unleashed In The Wild. They represent commonly held assumptions in the expert community as well as in the population at large about the most significant and already recognized risks the (relatively) near future holds. That being said, each of these alone, would blow Alexander’s (Alexander, 2018) cascading disaster magnitude scale out of the water in terms of magnitude, complexity, and length of the cascades potentially created.
Since Perrow’s normal accident theory of how tightly coupled systems (such as nuclear facilities) tend to make failures inevitable (Perrow, 1999), cascading risk dynamics have been observed in a wide range of areas from economics and markets (Sachs, 2009) to organized crime (Phillips and Watts, 2023). Social tipping dynamics, including positive dynamics (Otto et al., 2020) have also been described.
1.1 The five narrative input scenarios
The following is the 130-word summary text of the five scenarios (matching the text included in the survey). The video scenarios are based on the 1000-word version of these same scenarios, and can be consulted by those wanting to view the larger canvas (Undheim, 2023).
1.1.1 Growth and collapse by 2075
The build-up of exponential technologies, paired with a plethora of natural and human-made disasters that characterized the last few decades towards 2075 were not all unforeseen, but the exact toxic mix came as a more than unpleasant surprise, given that it depleted global resources and pitted the world’s nations against each other in a drive for ambition, domination, and, ultimately, for survival. The unforeseen chain of events that were precipitated by an initial period of nearly 30 years of exponential growth in technology, finance, and prosperity, ended abruptly in a global contraction caused by a devastating blast from an X factor surrounding a new energy technology, and escalated from there into an extinction event.
1.1.2 Climate cataclysm by 2075
Human-induced climate change from the whole industrial era, starting with industrial activity in the 1800s, and, in particular, since the anthropocene era starting in the 1950s, have accumulated to produce a mutually reinforcing cocktail of famine, extreme weather, war, and disease, starting to accelerate in the 2050s. Cascading effects of poor land use and primary predator extinction had wiped out 30% of 1850s biodiversity by 2040 and 50% by 2050, and has, since 2065, left the Earth without clean water and lacking in food. As a result, the Earth has already been reduced to 20 percent of the 2025 population, with the population rapidly dwindling, and the planet is on a trajectory that would end humanity within a few decades unless a drastic intervention happens, which does not look likely.
1.1.3 Runaway AI by 2075
The AIs that emerged in the first few decades after 2025 didn’t have the capacity of general intelligence, and were far from sentient. However, by the turn of 2050, things changed abruptly. Unforeseen changes started to occur, at first amongst the world’s top 100 supercomputers which, by 2045 had all been equipped with quantum processors. But it was the alignment of AIs with certain social groups who financed their emergence, and agreed with what we came to understand were the AI’s intentions and agenda, that made the runaway phenomenon possible. Enabled by humans, AIs became unstoppable, not alone, but as a hybrid collaboration.
1.1.4 Synthetic Biology Unleashed In The Wild by 2075
The synthetic biology breakthrough that fostered the crisis was discovered all the way back in the 2020s. It wasn’t the technology as much as the 2047 lab leak and the rapid integration with nature in a vulnerable part of the world that caused Earth’s ecology to take a nosedive. The synthetic compounds reacted adversely with photosynthesis and affected drinking water. However, it was the second lab leak, from the Floridian Mars lab in 2067 that accelerated things. The impact of both leaks were initially subtle, and almost untraceable. After a long incubation period, Earth succumbed to human-created synthetic compounds only after failed attempts to decontaminate and isolate the problem regionally.
1.1.5 World war by 2075
The devastating regional war that began in 2055 decimated the world’s two most powerful nations, entailed widespread use of inexpensive, widely available weapons of mass destruction, unleashed the biggest standoff ever seen using nuclear warheads, and created detrimental ripple effects across the world within 3 months, causing global financial collapse, nuclear winter, and near instant, drastic population decline, escalating GDP declines, as well as causing revolutions, fueled by disinformation in several countries. Globalization as a system of trade also became defunct, and with that, other global institutions collapsed, too.
This article describes the effort to quantify these five scenarios using a small set of indicators across six overarching dimensions: sci-tech, social dynamics, ecological impact, health adversity, economic factors, and governance, and a subsequent effort to model significant cascading effects obtained by mixing them in specific ways Figure 1.
For the purpose of quantification, High is hereafter defined as 50% change in Compound annual growth rate (CAGR), Medium as 30% change in CAGR, and Low as 0–10% change in CAGR. In most cases, a negative shock means a CAGR slowdown, rather than a reversal towards negative growth. We are using a standard definition of the compound annual growth rate (CAGR): CAGR is the annualized average rate of revenue growth between two given years, for a specified period longer than 1 year, assuming growth takes place at an exponentially compounded rate.
We will now list a tentative quantitative schematic for the five input scenarios previously mentioned, followed by projections towards 2075 for each dimension and indicator. For simplicity, we have chosen to only display the GDP growth and “Trade as % of GDP” curves. The other (17) curves, as well as the raw data used to construct them, are available in the data room [link removed to preserve anonymity].
1.1.6 Model inputs
The narrative scenarios were converted into quantitative data in a straightforward way. We first identified one to two key events in each scenario that would drive variance (as specified in the coming five sections, 1.1–1.5). We then identified 19 third party measured indicators that match the driving forces of disruption (and performed necessary scale conversions, as if needed, to create a standardized index). For example, in one of the ecological charts, an exponential curve needed to be inverted to match our format. To invert an exponential curve, you can use the inverse of an exponential function which is a logarithmic function. We then translated each narrative into curves with various inflection points depending on the events that constituted key scenario drivers.
Note that we have included a few extra graphs in the Runaway AI scenario (Section 1.1.9) to illustrate the process in more detail but otherwise stick to the “Trade as % of GDP” example.
1.1.7 Modeling the growth and collapse scenario
This scenario investigates the impact of protracted technology breakthroughs as well as an enormously effective expansion of globalization to all corners of the world. The assumption is that a combination of exponential progress in emerging technologies and energy production plus geopolitical stability (with stiff competition), floats all boats. Globalization expands dramatically and reaches nearly equally all corners of the world by 2050. Inequality of technological and market access is leveled out by the bounties shared by all. Space manufacturing expands the economic surplus by bringing in valuable externalities (resources, need for labor, demand for technology). In terms of social dynamics, we assume there is a widespread and full acceptance of sci-tech progress build-out, risks, and infrastructure. On the governance side, we imagine few or no regulatory hurdles.
As rapid economic growth is foreseen, all 19 indicators would be on a high trajectory from 2030 to 2050, as our scenario model assumes that fusion achieves net energy gain already by 2025 and gradually comes on the grid starting in 2030, providing an immense boost to the availability of power to the tune of a × 1000 energy output by 2050.
The negative events the initial input narrative had imagined starting in 2071 was slightly altered to see the model—growth and collapse and potential recovery—playing out. We started to introduce a high degree of decline already in 2050 (across the 19 indicators), with the most severe effect happening in the first 5–7 years (2050–55), to be followed by gradual improvement.
The 2050 economic contraction event described in the scenario would lead to an immediate fall in GDP but would, even if the scale might be several times more intense than previous depressions in history, eventually (and quite likely by 2075) recover (see Figures 2, 3).
Trade is less likely to recover at similar speeds (see Figure 4), due to discretionary trade barriers and tariffs such as those enacted during the Great Depression (Madsen, 2001), but trade would also likely eventually recover.
1.1.8 Modeling the climate cataclysm scenario
This scenario investigates the impact of increasing deterioration of the world’s natural environment. The ecological impact of >2° temperature rise from 2040 to 2075, a gradual increase up to 4°, and the effects of a global drought that destroys 50% of food production in 2055, are reflected in the fact that even though there is a moderately (High -) growth from 2025 to 2055 across all 19 indicators, effects start to be felt by the 2040s with a major negative economic, social, and environmental shock in 2055 (affecting those dimensions the most at first). The overall immediate impact is medium negative growth from 2040 to 2075 across indicators (see Figure 5). The impact on GDP is drastic but recovers by the end of the forecasted period (2075) (see Figure 6).
1.1.9 Modeling the runaway AI scenario
This scenario investigates the impact of serial, compounded tech breakthroughs in AI. The assumption on the sci-tech dimension is that to maximize its impact AI aligns with social groups (2050–2075), or vice versa, and that the impact will, to some extent, depend on which social groups manage to control and align their interests the most for maximum benefit. Either way, the economic effect would be massive growth from 2050 onwards, to the tune of billions of dollars initially and trillions of dollars added to the economy over some time (High+). The scenario assumes full AI acceptance by 2050, consisting of mixed alliances between various AI and elites, and failed attempts to block AI from taking over key institutions and societal functions from 2050 to 75. Ultimately, the scenario foresees a hybrid form of governance emerging consisting of an AI/human cyborg constellation constituting the governing class with 250+ IQ by 2070. Even though the high growth (High +: 2050-75) would only occur for specific elites, the overall impact would still affect most indicators. The caveat is that all social indicators would have lower growth (High -). As can be seen, GDP rises on a high trajectory (see Figure 7). For this scenario, we also included hockey stick growth examples for other indicators as well as a dramatic growth in state conflicts.
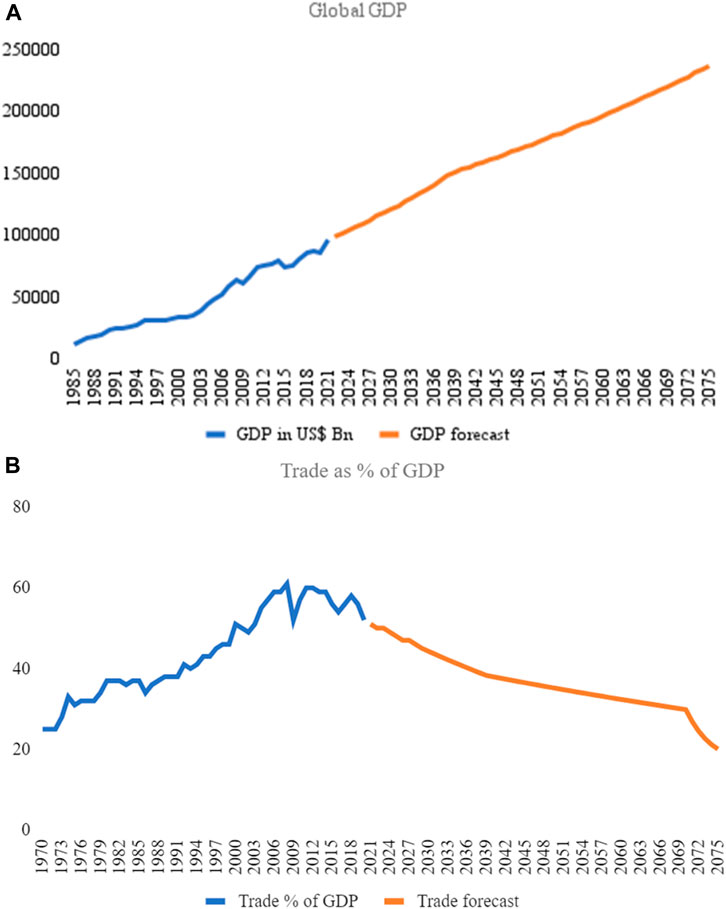
Figure 7. (A) Synbio growth scenario impact on global GDP. (B) Synbio growth scenario impact on Trade as % of GDP.
1.1.10 Modeling the synbio in the wild scenario
The gist of this scenario is synbio progress over the whole 50-year period from 2025 to 75 (High+) represented by our dedicated indicator, Carlson’s curve, but also with significant growth across other technologies (High). Catastrophic lab leaks in 2047 (a Swiss lab) and 2067 (a Florida lab) would severely impact ecological development but would not have economic impact (Medium) until the end of the period (2070-75). In fact, economic growth would be high (High -) from 2040 to 70. Governance would be strong from that moment (2047) onwards. The Pandemic Mars rust would come into play from 2070 to 75 and would accelerate the social panic which would have been growing steadily between 2040-75, as the food crisis in the same time frame (2040-75) would have begun to decimate population growth. At the end of the scenario timeline (2075), the arrow on most indicators would be pointing down although one might foresee that if the panic can be stopped, sci-tech progress would enable a lifestyle that is not overly affected by a deteriorating natural environment, in which case economic growth could perhaps be maintained at some reasonable level (Med). Again, what was an extinction scenario in the narrative might be salvaged into a more positive or at least neutral scenario when looking purely at the economic logic.
In the case of extreme synbio tech progress, we envision that GDP continues to rise at baseline or above (see Figure 7). This despite the economic effects of lockdowns, mandatory business closures, avoidance of workplaces, cities, or natural resources, the household lifestyle changes, or supply chain bottlenecks following COVID-19 (Walmsley et al., 2023), which could be expected to occur. The reason, we submit, is that the market making powers of synbio would offset such losses. In this particular model, we disregard the long term ecological implications.
1.1.11 Modeling the world war III scenario
1.1.11.1 Framing the challenge
Historically, the impact of world wars on the economy and society is complex. Fiscal crisis response tends to include a mix of taxing, borrowing, or printing money (Hall and Sargent, 2022). Post-war rebuilding efforts again tend to have positive social and economic effects, to the extent they are financially feasible. However such effects are often regional. The Second World War transformed the American West (Nash, 1990) and also benefited manufacturing in the South (Hooks and Bloomquist, 1992). The average time to foreign direct investment (FDI) recovery after wars between 1970 and 2008 is 4.5 years, and experiences range from 1 to 19 years (Moore, 2021). The two world wars shaped the European welfare states and their tax burden (Obinger et al., 2022). War technology is a major contributor to the legitimacy of states (Chin, 2019). World wars also reshape borders and create new states (Cebula, 2020). Industrial productivity, tech development, and the labor force initially tends to increase but is offset by damage to infrastructure (Mitoulis et al., 2023), inflation (Torregrosa-Hetland and Sabaté, 2021), food shortages (Frankelius, 2019; Kemmerling et al., 2022), pollution (Reuveny et al., 2010), adverse health outcomes (Allais et al., 2021), mental health deterioration (Murthy and Lakshminarayana, 2006), reduction in trade, uncertainty (Ramsay, 2017), a rise in debt, power shifts (Reynolds, 1992), and disruption of regular economic activity. In short, world wars epitomize cascading effects.
This scenario investigates the impact of a hypothesized World War in 2055. Before that, in the period from 2025 to 2050 a baseline scenario is expected in most western nations, meaning growth across all indicators continues as in previous years. Wartime industrial production sets in from 2055 to 75, which also means a rise in sci-tech indicators. However, a decline in free science occurs from 2060 to 75 as the war-mongering parties seek to limit the spread of advanced emerging technology outside their own military use (which is only partially successful). Social panic is evident in the period from 2055 to 75 as the true toll of war becomes apparent. Regional pollution from wartime activity also occurs from 2055 to 75. The growth rate is negative from 2055 to 75 across all indicators. However, in 2070, an 80% reduction in 2025-level GDP occurs as a result of mass starvation due to nuclear winter. That being said, for those who survived nuclear winter, the situation was nearly followed by significant recovery by 2075 (which would be the usual expectation after any depression) except there was a systemic governance collapse across the industrialized world which instead brought severe stagflation. As can be seen, GDP reclines and barely recovers to 2025 levels by 2075 (see Figure 8).
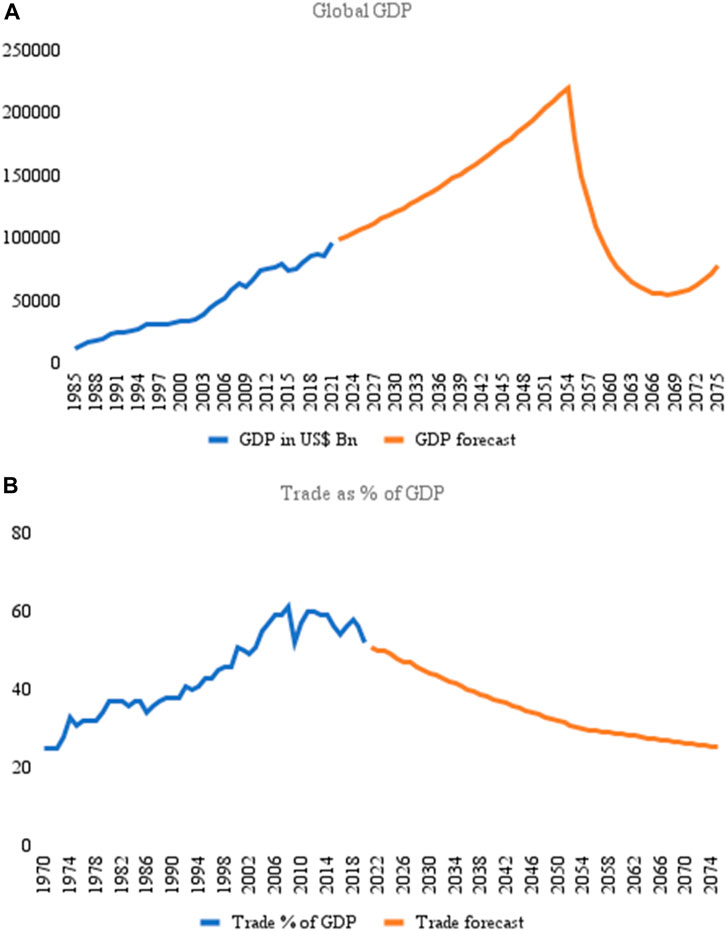
Figure 8. (A) World War III scenario impact on global GDP. (B) World War III scenario impact on Trade as % of GDP.
1.1.12 Modeling wider cascading effects
Given our conviction about, emphasis on, and awareness of cascades, to model realistic futures, it is not going to be enough to stick to the five relatively obvious scenarios we have depicted so far. Rather, the plausible futures we are going to face will be intricate mixes of all of these as well as full of events and mechanisms about which we are only scratching the surface. The next step in the process was always to let these scenarios be updated, amended, replaced, and filled in with driving forces, data projections, and a complete set of narratives. For that reason, the majority of the paper will attempt to model several scenarios for cascading effects, based on varying sequence, proximity, and magnitude of each of the major catastrophes depicted in the five scenarios, starting with mixing sets of two specific scenarios together.
In Figure 9, we illustrate the dimensions chosen for cascading effects of specific configurations of several of the five scenarios co-occurring, notably sequence, effects, proximity effects, and magnitude effects. We note that the cascading risk literature includes the notion of a location-specific vulnerability rank that depends on the distance to the epicenter of the shock (Naqvi and Monasterolo, 2021). We also theorize the likely impact of each upon each disruptive dimension. The assumption, before modeling the effects, would be that each disruptive factor differs in its sensitivity to such dimensions. For example, given its novelty aspect, sci-tech would be expected to produce the most negative effects if occurring early in the sequence of events. Similarly, the proximity effect (in time) would seem to matter less given its assumed generic impact across all time scales. Lastly, its magnitude would be high in most scenarios.
Both governance and social dynamics would be expected to have an opposite logic, where they would lead to less severe consequences if addressed early (e.g., governing sci-tech would reduce sci-tech risks). Ecological impact is assumed to be a result of (extractive) economic activity but would also be highly sensitive to proximity in time (or else might be expected to recover) and would not be worrisome unless the magnitude was enormous (and irreversible in human time scales). Given its lower overall severity as risks (apart from the risk of death), we would not expect health adversity to be equally severely affected by each variable. Social dynamics are expected to play a moderating role but not lead to extreme effects.
2 Methodology
Even if we have set out to understand, or give more context to societal evolution, we are not so much attempting to predict the future, or various possible futures (scenarios), as we are de facto modeling a set of if-then conditions within a set of scenarios.
The paper is based on a set of 19 quantitative indicators, chosen to represent six dimensions of societal change (sci-tech, social dynamics, ecological impact, health adversity, economic factors, and governance). They are proxy indicators of larger developments where data is even more scarce and are not purely meant to represent exactly what they measure. However, the chosen indicators are each third-party gathered information of longitudinal nature and represents among the most respected data sources available (e.g., from Statista, Synthesis, TeleGeography, Our World In Data, IRENA, Public Resource of California, Our World In Data, Encyclopedia Britannica, World Bank, Mori Foundation, Cambridge U, and the UN). Each of them has historical data from 10 to 50 years (or more) which is used to project a trend in the curve for the next 50 years. That trend was adjusted by historical averages for the impact of the type of events hypothesized to occur in each scenario. If available, sources for such adjustments are included in the relevant section.
We started with a desire to use 12 sci-tech indicators, 12 governance and regulation indicators, 19 business forces and macroeconomic indicators, 13 social dynamics indicators, and 8 environment and health indicators, but gave up such an extensive list given the poverty of available data for each, as well as overlapping statistical properties. Instead, we sacrificed some specificity (we lost potential indicators such as the number of black swan events, global cities index, number of revolutions, global peace index, Kondratieff waves, work automation, startup share of the economy, manufacturing risk, energy outlook, corruption, happiness, health security, and planetary boundaries) but gained clarity and simplicity of our model. The final list of 19 indicators (see Table 1) selected included 5 sci-tech indicators, 4 governance and regulation indicators, 4 business forces and macroeconomic indicators, 3 social dynamics indicators, and 3 environment and health indicators. Each has a sound data source.
As can be seen from Table 2, we used each source indicator to create an index curve with an assigned CAGR value. We then went about creating indices for each dimension, using this type of traditional economic statistics methods transforming indicators available in various forms to create an index normalized on a scale from 0 to 1 for simple comparison and to be able to use more than one indicator as part of each dimension (O'Neill, 2015;Ralph et al., 2015). In many cases, we had to project the best available data up to 2075 from a more limited time range (typically 10–50 years worth of data). Table 3.
2.1 The cascading model used
The 2075 Cascading Risks Model (see Table 2) contains a set of assumptions for each scenario that represent quantifications of the narrative scenarios (see Findings). We model cascades at the dimension-index level. The cascading effect means that now the index growth rate goes negative rather than a simple slowdown as the individual scenarios. For the monster scenario (where all negative scenario conditions apply), we apply an additional 30% weight to the downward impact.
The compound annual growth rate (CAGR) is the average rate of growth over a specified period longer than a year. It takes into account the effect of compounding, which means that the growth builds upon itself. As part of the scenario quantification, we used CAGR change as a proxy for all kinds of impact in the 2075 Model. In real life, the impacts would have been far worse because there would be interaction effects with non-financial impact. In order to estimate the effects of various hazards introduced in the narratives, we used a set of assumptions derived from the lessons from the Great Depression (Madsen, 2001; Bernanke, 1983; Granados and Roux, 2009), assuming that effects lasted about a decade, with the most severe effects in the first 5–7 years.
The model we used created a set of CAGR assumptions based on the above mentioned indicators (Table 1).
The cascades were modeled using a three step approach: 1) create an index for each impact dimension with higher weights assigned to more important metrics (e.g., GDP has the highest weight in the economic index); 2) negative growth rates applied across indices with minimal rebound till 2075 to capture the effect of multiple crises; 3) applying a 1.1–1.3x multiple for the “monster” scenario. The main difference from the individual scenario modeling is the fact that there is not just a growth slowdown but a sharp reversal across indices, and that the potential for a rebound is limited. Before applying the weights, the data was normalized.
The narrative scenarios were developed using a mainstream understanding of scenario planning methods, mixing deductive and inductive methods (Chermack, 2005; Kahane, 2012; Amer et al., 2013; Coates, 2016; Chermack, 2022), going back and forth between empirical data support and narrative construction. A key determinant was the unfolding, social embedding of game changing emerging technologies into society (Betz et al., 2023). However, evolving scenarios perform an important role in unlearning rigid views (Burt and Nair, 2020), including the stubborn dependency on single-risk factors determining scenario outcomes. The 19 quantitative indicators chosen for the model presented in this paper represent only a small sample of the empirical material scanned to develop the narratives.
3 Findings
In this section we present a selection of tables with modeled cascades from specific intersections between the five scenarios. The objective is to show the projected impact of closely occurring catastrophic events.
3.1 Three cascading risk scenarios and a fourth monster scenario
To test what would happen if cascading risk effects were highly prevalent, we exposed our five scenarios to each other in the following way, focusing only on three key interactions: 1) Runaway AI + Synbio in the Wild, 2) Climate Cataclysm + Runaway AI, and 3) Globalization collapse (Growth and Collapse + Climate Cataclysm) + Governance crisis. For simplicity, we show a combined chart with all indicators together–each indicator’s curve is in the [data room (link to data room removed to preserve anonymity)].
3.1.1 Runaway AI + synbio in the wild
In this type of scenario we assume that AI and synthetic biology is jointly producing effects upon the sci-tech world and upon industrial developments and that the magnitude of impact of dual risk failures (lab leaks, unaligned AI, terrorist scenarios, state-level weaponization) would depend on sequence effects, proximity effects, and sheer magnitude effects of each and the combined impact. The impact of AI risk is currently high on the policy agenda yet with few effective regulations in place due to high uncertainty regarding appropriate approaches (White and Lidskog, 2022). The hybrid risk of AI with biotechnology is under-studied even as progress in brain-computer interfaces (BCIs) or precision medicine is rampant. Wherever precision medicine is promised there is also the potential for eponymous maladies. The current cybersecurity measures related to AI-enhanced biotech such as DNA synthesizers is insufficient (O’Brien and Nelson, 2020). Having said that, higher power actors will tend to be more likely to produce such innovations or disruptions and should be the target of the most scrutiny (Sandberg and Nelson, 2020).
3.1.1.1 Scenario narrative
Already by 2025 AI and Synbio both were in high growth with immediate synergies. Life science breakthroughs solved cancer puzzles, developed climate resistant crops, started to repair biodiversity with SynBio created replacements and biobanks, but also delivered means of effective population control.
By 2050, scientists had achieved massive synergies between AI and Synbio but negative Social effects were materializing as people were increasingly living virtual lives and isolating themselves from the outdoors and nature due to the climate cataclysm (warming, extreme weather, biodiversity collapse). The 2037 lab leak spiraled out of control because AI had made the runaway SynBio process exponential. Governance began to crack as people worldwide questioned the way the world’s governments were responding to the lab leak and ecological disasters caused jointly by climate change and the synbio lab leak. The second, 2067 lab leak completes the ecological disaster already caused by climate change.
By 2075, Earth’s ecosystem is irrecoverably and near fully contaminated by SynBio created compounds. As a result, drinking water is severely restricted, only available to the global elite, and all others are suffering and beginning to succumb to diseases. As a result, the economy, which otherwise had been growing fast throughout the 50-year of scientific progress showed signs of stagnating and could no longer support even a dwindling population, partly because such a huge proportion of people were facing staggering inequality-related distress, requiring support in various ways, economically, healthwise, and socially.
As can be seen from Figure 10, initially the growth from a combination of AI and synbio technologies is staggering, but once adverse effects start to show, the decline would be even steeper and would be hard to mitigate because of its speed, magnitude, and effects on society.
3.1.2 Climate cataclysm + runaway AI
This type of scenario is described in the literature as natech (Girgin et al., 2019) and involves natural disasters co-occurring with, or directly causing technological failures and industrial accidents. The tricky thing with this type of risk trajectory is that if unprepared, the natural disaster could lead to taking the eye off the ball rather than preparing extra hard or prioritizing security of industrial installations and infrastructure. More generally, if we assume a more generalized state of climate disruption, one could readily imagine and reasonably expect that governance resources, budgets, and political attention would be focused on recovering from ongoing or already occurred disasters and not watching out for the next one.
In the following model, we took various timelines of each configuration, experimented with the sequence effects, proximity effects, and sheer magnitude effects of each and the combined impact.
3.1.2.1 Scenario narrative
By 2025, Climate impact had caused an increasingly joined-up global governance effort to decarbonize and electrify the economy. The CCS efforts were financially taxing and scientifically complex, without great sci-tech breakthroughs apart from in AI (as the energy breakthroughs didn; t materialize and the world’s populations did not wholeheartedly embrace nuclear power). However, the material basis of the economy was harder to tackle. Even with the positive innovation impact of the AI explosion in the 2020s, the world economy gradually entered degrowth throughout the 2030s. As a result, even though AI progress continued for a while until we hit another AI winter as the deep learning paradigm became too computationally and energy costly and the world’s economies ran out of clean power, economic, social, governance, ecology, and health indicators all were impacted negatively. People in the Global South, as well as the poor in the Global North with nowhere to go and no resources to protect themselves from the heat, suffered massive health consequences. At the same time, AI, especially AI intransparency, accelerated existing inequalities despite attempts to govern AI.
By 2050, supercomputers with quantum processors taking advantage of exponential AI breakthroughs were capable of advanced climate simulations that unequivocally showed that the Earth’s eco-trajectory was headed for biodiversity collapse by the end of the century. This caused major social upheaval. Local environmental effects were also severe. Health adversity due to inequality in access and lack of options for great swaths of the world’s population created revolutions and governance crises. Apart from AI, other significant sci-tech breakthroughs (in SynBio or Energy) did not materialize. When global drought destroyed 50% of food production in 2050, this cascaded into a rapid population decline.
Towards 2075, runaway AI impact is near total, global AI regulation fails again and again to control the phenomenon, and global elites (including criminal networks and rogue states) collude with AI, enabling it to gain access to secure networks worldwide. This causes governance collapse in most major economies, and total chaos in all but a few states and territories (those that are still predominantly non-digital in their processes). The global system of trade, and globalization overall collapses, and with that most global institutions, including the UN, WTO, as well as military alliances including NATO, and even global discussion fora such as the World Economic Forum. With that, there is no way to control climate change, technology collaboration suffers, and countries are left to their own devices. A few countries and actors spiral to the top and start ruling vast territories, with AIs monitoring and beginning to rule most processes. Freedom is significantly diminished. The climate is also on a fast runway towards full collapse by the end of the century. Population continues to rapidly decline.
As can be seen from Figure 11, the degrowth scenario kicking in coupled with the compounding effects of an evolving climate cataclysm eventually leads to societal collapse even if AI continues to grow until its downsides start to show towards mid-century.
3.1.3 Globalization collapse (growth and collapse + climate cataclysm) + governance crisis
This type of scenario is already modeled in the Growth and Collapse scenario, which, in effect, is a cascading effects type scenario. We add severe effects from the Climate Cataclysm scenario to see what that does to the recovery times and overall development of the scenario. To substantiate this development, one could look at IPCC’s worst case baseline scenario, RCP 8.5 (Riahi et al., 2011), assuming conditions of no explicit climate policy, which predicts a total temperature increase of more than 4.5°C by 2100, or even the Hothouse Earth scenario (Steffen et al., 2018; Kemp et al., 2022) which imagines there is a tipping point already at a temperature rise of ∼2.0°C above pre industrial temperatures. We note that contrary to ours, both RCP 8.5 and the Hothouse Earth scenario assume some level of governance (but with no successful carbon mitigation).
3.1.3.1 Scenario narrative
By 2025, sci-tech progress across domains (AI, Energy, Nanotech, Quantum, Space tech, SynBio) created a decades-long global growth cycle, initially with no end in sight. Exponential energy breakthroughs in fusion, manufacturing prowess enabling that energy to get on the grid by the late 2030s, and space manufacturing, in particular, fueled that growth. Climate impact dampened the mood, but successful CCS including surprisingly scalable and effective Direct Air Capture (DAC), plus geoengineering at scale, both cooled the Earth’s climate and stemmed biodiversity collapse and global warming.
By 2050, however, Africa’s industrialization along with the near inevitable expansion of the rest of the Global South, created a resource squeeze that precluded decentralized decarbonization. As a result, emissions still continued to climb, temperature growth continued, and a global drought set in around 2050, destroying 50% of global food production. Health, governance, and ecological crisis at unseen levels ensued, causing mass climate migrations, violence and escalating inequalities in all urban areas.
Towards 2075, an exodus from the Global South due to high temperatures, and collapsing infrastructure in the vast urban conglomerations in Nigeria and in the Sahel, caused Europe’s social structure to collapse. With the EU in default, the global economy went into a depression. That’s also when the 2071 energy reactor catastrophes occurred, destroying the new nuclear infrastructure and causing massive radiation and physical destruction rendering vast urban areas as well as fertile land uninhabitable. These cascading effects decimated the world’s population, created health adversities and ecological effects that lingered into the end of the century. Only a few hundred isolated communities of indigenous populations scattered around the globe were relatively intact.
As Figure 12 indicates, even a staggered onset of various risk event dimensions towards and after mid-century, and even after years of economic and technological progress, eventually pushes the world towards a collapse with little recovery in sight by 2075. The compound effects of a lack of a globalized response further deteriorates the situation.
3.1.4 Monster scenario
This is a scenario where we assume that all calamities described in any of the five scenarios happen over the 50-year period.
3.1.4.1 Scenario narrative
By 2025, sci-tech progress across domains created a decades-long global megagrowth cycle. Except, as Africa’s industrialization accelerated throughout the 2030s, that created a political battle for scarce material resources such as rare earths and strategic metals such as cobalt. Energy progress initially slowed the ecological crisis, with efficient CCS by the early 2030s, and fusion energy on the grid by the late 2030s, and AI progress throughout the first 15 years, followed by a brief AI winter as the deep learning paradigm became too energy intensive, even for the fusion economy.
The 2037 SynBio lab leak had been a wake-up call, and was never contained. However, despite the scarcity of drinking water for the masses (with the elite drinking water cleaned by powerful SynBio chemicals), by 2050, the unprecedented economic expansion had created expectations that all countries would continue to grow exponentially. However, the material resource squeeze compelled the world’s existing and emerging superpowers to turn militaristic and territorial. As a consequence, globalization suffered a big blow. China’s 2055 invasion of Taiwan triggered a regional war that got the US, Australia, and the United Kingdom involved, which soon enough made it into a global war. With AI-weapons, and advanced quantum-enabled cybersecurity attacks, the war crippled global financial centers. Military infrastructure was quickly decimated on both sides, although China somehow got the upper hand because of its rapid manufacturing capabilities.
Towards 2075, the exodus from the Global South due to high temperatures caused migration crises across the world. The EU collapsed, the US split apart, and many Asian megacities collapsed under the pressure. Global elites weaponized powerful AIs, colluding to give those AIs full access to secure infrastructures, military systems, governance systems, financial networks, and the Internet. The result was that humanity lost control over its key resources, mostly because AIs now knew more about the vulnerabilities than any human team of experts anywhere. By 2071, societal collapse ensued, and only scattered indigenous communities were relatively unscathed. Even though sci-tech continued to advance, the costs of labs and infrastructure meant that only a few regimes could continue innovating. Decentralized innovations were of limited scope.
In Figure 13, our model indicates that if a sufficient number of risk events of high magnitude co-occur in close proximity to each other, regardless of the exact sequence of such events, the recovery is severely compromised because of cascading interactions and will at some point not plausibly occur in human timelines. In our model, we have only used a small number of indicators and risks. In reality, a far more complex array of risks might materialize and the outcome could turn catastrophic even more rapidly or violently. On the other hand, mitigating strategies could be put in at any time. Depending on their severity and impact, such trajectories might therefore presumably be avoided. That being said, our model relies heavily on historical patterns of recovery, for example, after the Great Depression, which, needless to be said, already was modified by mitigation strategies by governments and other actors.
4 Discussion
The existential risk literature’s use of quantitative scenarios is limited, perhaps for the reason that such risks are hard to model and that there is little or no precedent. A notable call to action is in a recent article called Climate Endgame, which calls for more advanced analysis of cascades using available data across domains such as climate science, economics, and social fragility (Kemp et al., 2022). Indeed, climate change might give rise to a wider discussion of the changing existential risk space that also might include considerations around permanently constrained wellbeing effects that would also be caused by such changes (Huggel et al., 2022). However, the complex dynamic systems literature does provide some guidance on negative multiplicative effects in the form of unprecedented adverse shocks that could arise out of finite-time singularity (Johansen and Sornette, 2001). This type of argument has variously been used to forecast the collapse of major stock markets, a transition to sustainability, or a return to accelerating growth, but for the latter, the problem is that we would have to innovate at a faster and faster rate which eventually might not work out for us (West, 2018). One study exploring the evolution of the US financial market over >150 years found a higher likelihood of a collapse greater than the “small” crashes of 1929 and 1987 which were relatively isolated and of short duration (Grobys, 2023). Sornette’s general theory of how, why, and when stock markets crash says the underlying cause of a catastrophic event can be sought months and even years before the abrupt crash, in the build-up of cooperative speculation, which often translates into an accelerating rise of the market price (a “bubble”) (Sornette, 2017).
Our five initial scenarios were built around one key disruptive force, such as governance (war), technology (AI, Fusion, or SynBio), ecology (climate cataclysm), or economy (growth). Each has its own literature of risks as well as, of course, opportunities. When we combined those scenarios, the cascading effects multiplied in unexpected ways. This was hard to model and we took a conservative approach. The effects of two technological advances (or lack thereof) in close proximity are not linear. Neither is the disturbance of climate cataclysm in the middle of a crisis such as a world war. The existing scientific literature is only beginning to address such concerns. Part of the reason is that despite being evoked for decades, transdisciplinary is, in practice, still rare (Bell, 2002; Rigolot, 2020; Rutting et al., 2022). Perhaps because it is nearly octogonal to the way science traditionally is practiced as an endeavor to dig deeper and deeper into smaller domains.
The severe impacts did not start occurring until two or more risks accumulated or happened in short order. At surface level, this is a trivial finding. The more calamities, the worse it gets. However, if we look a bit closer, a number of more nuanced dynamics, mechanisms, and trajectories could be observed. Some of them were linear, others could only be described as non-linear, but did depend somewhat on our assessment of the cascading weight of each risk dimension. For example, in most scenarios there was no plausible path to recovery once the full set of risk forces were unleashed by mid-century. This is evidenced by the economic dimension since even during the Great Depression, there was a sharp recovery within a decade while the cascading and multiplicative nature of risks in our modeling does not allow for that. To our knowledge, no existing models or research projects in the literature, nor have any publicly available findings from calamity scenario exercises previously credibly modeled such effects. The study of the rise and fall of previous civilizations has, however, again and again confirmed cascading effects as opposed to single factor explanations (Gibbon and Trevor-Roper, 2010; Diamond, 2020; The Oxford World History of Empire: Volume One: The Imperial Experience, 2021).
There are instances where very high growth rates in the “high +ve” scenarios seem quite unrealistic for the specific indicators we had chosen. For example, the growth rate of installed IoT devices or reduction in cost for genome sequencing is >20% over the past decade, which is an unreasonable estimate over the medium-long term going forward. We have tried to control for that by adjusting the projections slightly. However, we largely left the spirit of the forecasted growth rates for the sake of formal modeling. Had we chosen another specific indicator, the positive growth rates might have made more sense. There is also some evidence in the literature that over-reliance on causation-based approaches might become a bias against the indeterminism of the future (Derbyshire and Wright, 2014). If we follow the indeterminism logic, it would be important to explore wider scenarios so we could prepare more antifragile responses.
The model assumptions have numerous limitations, notably that with the chosen indicators, we were unable to model all relevant interactions and relationships across disruptive forces. In fact, we only modeled the impact of all forces upon a single indicator, economic growth and decline. Despite that shortcoming, and being quite conservative in the modeling of economic growth implications of each factor both isolated and combined, we were able to demonstrate shocking negative cascades. No political system, nor the global polity, currently has mitigation strategies for such cascades. This is highly worrisome and must be addressed. In the following, we will attempt to create a taxonomy of the cascading risk mechanisms the model demonstrates.
4.1 Exponential cascading effects
When our model assumption was that two co-occurring catastrophic events simply doubled the damage, the severe impacts did not start occurring until five to seven risks accumulated or happened in short order. When our assumption shifted to modeling that co-occurring catastrophic events had an exponential effect upon each other, the situation dramatically changed. In fact, even doubling the effect of each if they were to co-occur, radically altered the recovery timeline. Also note that the most drastic effects were seen only in the event of several catastrophic events in short order around the midpoint of the 50-year scenario timeline. However, none of our model assumptions included a “doomsday” factor that changes everything. Rather, we only used historical patterns of decline and recovery, adjusted for measured interaction effects. One could criticize this choice and point out that this is dangerously conservative and that the future could bring significant discontinuity. This is true. Future models could make different choices. The future studies literature also has interesting observations on the value of past-facing approaches to futuring, recasting and pastcasting, to complement future-facing approaches such as forecasting and backcasting (Bendor, 2022). Quantified cascading risk scenario modeling could perhaps usefully be deployed to each of these approaches by other researchers and might, as a complete picture, better illuminate options faced by various stakeholders at a given moment in time. However, despite more information becoming available, it is difficult to judge what the right amount of information is for any decision, much less what the most useful information is or how to best evaluate it and decide a course of action. That’s why resilience is such an attractive path forward for X-risk mitigation (Galaz et al., 2021).
4.2 What about single factor risks?
Our study did not provide explicit evidence that there is no reason to worry about single risks such as AI risk, climate risk, synbio risk, or nuclear risk. However, in most plausible scenarios, the complex interplay between different risk factors would ensure that cascading effects were what caused true calamities, even if a single effect could be said to be some sort of “trigger,” “domino,” or “tipping point,” as previously described in the risk literature around disasters (Schweizer, Pia-Johanna and Renn, 2019), climate change (Lenton et al., 2019), social tipping points (Juhola et al., 2022), or systemic risks as such (Schweizer, Pia-Johanna, 2021). We strongly feel such linear and mechanistic metaphors should be avoided because they are misleading. This is also a challenge with Taleb’s notion of Black Swan events as if they were just a single occurrence, not a complex process with preceding dynamics (even if they would have been hard to explain ex ante). Indeed, a recent article claims most technological accidents cannot be considered Black Swans because they are risks from hazardous industrial activities that should have been subject to corporate oversight. Natech accidents, which can occur due to a mix of climate change (Na) and technological progress (tech), are also generally foreseeable and therefore preventable (Krausmann and Necci, 2021).
Relying on such language fosters misaligned mitigation efforts that are a waste of time and don’t very likely produce true mitigation, implementable antifragility measures, or fruitful paths towards recovery. There is no way to know exactly which of many severe risks would be the one to stop or contain. While working hard on a single factor risk we might drop the ball on several others. Which is not the same as to say that they can be ignored. It would seem a portfolio approach makes the most sense, plus devising mitigations that can work for several risks at the same time because they work on root causes.
5 Conclusion
The research question we began with was about how many co-occurring, significant hazards it would take to put the world on a path towards humanity’s extinction, and the answer our model found was two or more. These findings echo what the literature of complex dynamic systems (Johansen and Sornette, 2001; West, 2018; Barthélemy, 2011) has consistently shown for the past few decades or more. Considering what is going on in the ecological sphere, the world of geopolitics, or simply considering the escalating rate of technological change, this is not a very comforting finding. To be clear, even though we modeled steep downward spirals typical of the fall of historical civilizations, our scenarios, even the monster scenario, did not immediately take us all the way towards humanity’s extinction. One might conclude that this gives room to develop resilience-based approaches which are now popular across risk areas and domains (Linkov et al., 2014; Logan et al., 2022; Mitoulis et al., 2023; Mitra and Shaw, 2023) in order to cope with cascading disasters, which provides a space for governance.
We found support for our first key hypothesis that (I) two or more hazards would drastically increase the odds but regrettably not for our second hypothesis that (II) a plethora of hazards would have to co-occur for x-risks to materialize. This might still mean our third hypothesis holds, which said that (III) X-risk events are exceedingly rare, possibly inconceivable in the foreseeable future. Either way, our null hypothesis that there is no significant relationship between the number of hazards and the appearance of X-risk events, does not have support.
Cascading effects, even of the factors included in five relatively straightforward input scenarios, would be devastating if they started to co-occur in close proximity to each other, or in certain sequences. For example, economic collapse during a climate catastrophe that was expensive to fix, or governance collapse during a period of exponential technology growth, would each be detrimental.
We should point out that this study used a small set of indicators (19) to model cascading effects of major catastrophes over a 50-year time span. We showed that even with such a constrained number of variables, the effects were devastating across society. We would suggest that future research applies a similar perspective but uses × 10, × 100, or × 1000 the number of indicators to model whether or how the severe interaction effects persist. Complex systems dynamics models might have to be deployed, and software would have to be developed for that specific purpose, which we opted against mostly for lack of easy interpretability. Another research task would be to map more precisely the specific cascading patterns that would ensue, including which specific areas might be affected depending on sequence, proximity, and magnitude of various cascading risk events. Also, we made no attempt to follow along the cascading chains to model specific trajectories.
A caveat is that such a research design, especially one that attempted true simulation, might require (not yet emerged) supercomputers. We would theorize that the effects potentially shown by such a simulation would be far worse than ours showed, due to chaos effects and further cascading exponentiality from the multitude of interactions and cascades created.
In the spirit of the precautionary principle, the conclusion we tentatively draw is that, if we want to maintain humanity’s growth potential economically, technologically, and socially, as well as if we want to maintain ecological balance from features such as a rich biodiversity and a human friendly climate, we need to avoid significant cascading effects at all costs. How to do that is another matter and is a task for pressing future transdisciplinary research, industrial innovation, and urgent policy discussion in global fora. Innovations where negative externalities are early identified should be rejected based on social cost. But the risk/innovation tradeoff can be significant (Witt, 2016). Failing that, mitigation strategies in the case multiple risk trajectories start to materialize would have to be all-encompassing and of a magnitude that would render previous crisis responses such as FDR’s New Deal after the Great Depression, the Marshall Plan after World War II, or the worldwide response to COVID-19 mere economic parentheses. Black swans call for robustness and resilience, and antifragility far beyond traditional engineering risk analysis (Aven, 2015). Those mitigations would themselves need to count on triggering positive cascades or at least slowing down or diffusing negative cascades. The timing of such mitigations would also matter enormously, with earlier interventions drastically better.
Future research should try to add further concreteness to what may happen close to the singularity of super-exponential growth or decline (shock) by further exploring the detailed interactions surrounding finite-time singularity arising out of multiplicative effects.
Data availability statement
The raw data supporting the conclusion of this article will be made available by the authors, without undue reservation.
Author contributions
TU: Conceptualization, Writing–original draft, Writing–review and editing, Investigation, Validation, Visualization. TA: Data curation, Methodology, Visualization, Writing–review and editing.
Funding
The author(s) declare that financial support was received for the research, authorship, and/or publication of this article. This work was supported by a grant from Open Philanthropy.
Conflict of interest
The authors declare that the research was conducted in the absence of any commercial or financial relationships that could be construed as a potential conflict of interest.
Publisher’s note
All claims expressed in this article are solely those of the authors and do not necessarily represent those of their affiliated organizations, or those of the publisher, the editors and the reviewers. Any product that may be evaluated in this article, or claim that may be made by its manufacturer, is not guaranteed or endorsed by the publisher.
References
Alexander, D. (2018). A magnitude scale for cascading disasters. Int. J. Disaster Risk Reduct. 30, 180–185. doi:10.1016/j.ijdrr.2018.03.006
Alexander, D., and Pescaroli, G. (2019). What are cascading disasters? UCL open Environ. 1 (1), e003. Available at:. doi:10.14324/111.444/ucloe.000003
Allais, O., Fagherazzi, G., and Mink, J. (2021). The long-run effects of war on health: evidence from World War II in France. Soc. Sci. Med. 276, 113812. doi:10.1016/j.socscimed.2021.113812
Amer, M., Daim, T. U., and Jetter, A. (2013). A review of scenario planning. Futures 46, 23–40. doi:10.1016/j.futures.2012.10.003
Beck, U. (1992). Risk society: towards a new modernity (published in association with theory, culture and society). 1st edn. Thousand Oaks, CA: SAGE Publications Ltd.
Beiderbeck, D., Frevel, N., von der Gracht, H. A., Schmidt, S. L., and Schweitzer, V. M. (2021). Preparing, conducting, and analyzing Delphi surveys: cross-disciplinary practices, new directions, and advancements. MethodsX 8, 101401. doi:10.1016/j.mex.2021.101401
Bell, W. (2002). A community of futurists and the state of the futures field. Futures 34 (3-4), 235–247. doi:10.1016/s0016-3287(01)00041-6
Bendor, R., Eriksson, E., and Pargman, D. (2021). Looking backward to the future: on past-facing approaches to futuring. Futures 125, 102666. doi:10.1016/j.futures.2020.102666
Bentham, J. (2014). The scenario approach to possible futures for oil and natural gas. Energy policy 64, 87–92. doi:10.1016/j.enpol.2013.08.019
Bernanke, B. S. (1983). Nonmonetary effects of the financial crisis in the propagation of the great depression. Am. Econ. Rev. 73 (3), 257–276.
Betz, U. A. K., Arora, L., Assal, R. A., Azevedo, H., Baldwin, J., Becker, M. S., et al. (2023). Game changers in science and technology - now and beyond. Technol. Forecast. Soc. change 193, 122588. doi:10.1016/j.techfore.2023.122588
BlackRock (2023). Geopolitical risk dashboard, BlackRock. Available at: https://www.blackrock.com/corporate/insights/blackrock-investment-institute/interactive-charts/geopolitical-risk-dashboard (Accessed: October 16, 2023).
Burt, G., and Nair, A. K. (2020). Rigidities of imagination in scenario planning: strategic foresight through “Unlearning”. Technol. Forecast. Soc. change 153, 119927. doi:10.1016/j.techfore.2020.119927
Cebula, A. (2020). The legacy and consequences of world war I. J. Mil. Ethics 19 (2), 118–120. doi:10.1080/15027570.2020.1796311
Chermack, T. J. (2005). Studying scenario planning: theory, research suggestions, and hypotheses. Technol. Forecast. Soc. change 72 (1), 59–73. doi:10.1016/j.techfore.2003.11.003
Chermack, T. J. (2022). Using scenarios: scenario planning for improving organizations. Oakland, CA: Berrett-Koehler Publishers.
Chin, W. (2019). Technology, war and the state: past, present and future. Int. Aff. 95 (4), 765–783. doi:10.1093/ia/iiz106
Cho, Y. (2018). Exploring technology forecasting and its implications for strategic technology planning. Portland, OR: Portland State University. Available at:. doi:10.15760/etd.6108
Coates, J. F. (2016). Scenario planning. Technol. Forecast. Soc. Change 113, 99–102. doi:10.1016/j.techfore.2016.10.043
Cordova-Pozo, K., and Rouwette, E. A. J. A. (2023). Types of scenario planning and their effectiveness: a review of reviews. Futures 149, 103153. doi:10.1016/j.futures.2023.103153
Cremen, G., Galasso, C., and McCloskey, J. (2022). Modelling and quantifying tomorrow’s risks from natural hazards. Sci. Total Environ. 817, 152552. doi:10.1016/j.scitotenv.2021.152552
De Marzo, G., Gabrielli, A., Zaccaria, A., and Pietronero, L. (2022). Quantifying the unexpected: A scientific approach to Black Swans. Physical Review Research 4 (3). doi:10.1103/physrevresearch.4.033079
De Pryck, K., Hulme, M., Skodvin, T., Leclerc, O., Hartz, F., Livingston, J. E., et al. (2022). A critical assessment of the intergovernmental Panel on climate change. Cambridge University Press. doi:10.1017/9781009082099
Derbyshire, J., and Wright, G. (2014). Preparing for the future: development of an “antifragile” methodology that complements scenario planning by omitting causation. Technol. Forecast. Soc. change 82, 215–225. doi:10.1016/j.techfore.2013.07.001
Diamond, J. (2017). Guns, germs, and steel: the fates of human societies. 20th edn. W. W. Norton and Company.
Diamond, J. (2020). Jared Diamond 3 books collection set(upheaval, collapse guns, germs and steel). Penguin ptd.
Feng, L., Wang, Q., Wang, J., and Lin, K. Y. (2022). A review of technological forecasting from the perspective of complex systems. Entropy 24 (6), 787. Available at:. doi:10.3390/e24060787
Ferguson, N. (2004). Empire: the rise and demise of the British world order and the lessons for global power. Reprint edition. Basic Books.
Fernandez-Armesto, F. (1995). Millennium: a history of the last thousand years. First Edition. Scribner.
Fernandez-Armesto, F. (2002). Civilizations: culture, ambition, and the transformation of nature. Reprint edition. Florence, MA: Free Press.
Frankelius, P. (2019). Back to the root causes of war: food shortages. Lancet 393, 981–982. doi:10.1016/s0140-6736(19)30018-2
Galaz, V., Centeno, M. A., Callahan, P. W., Causevic, A., Patterson, T., Brass, I., et al. (2021). Artificial intelligence, systemic risks, and sustainability. Technol. Soc. 67, 101741. doi:10.1016/j.techsoc.2021.101741
Gibbon, E., and Trevor-Roper, H. (2010). The decline and fall of the roman Empire: volumes 1-3, volumes 4-6. Reprint edition. New York, NY: Everyman’s Library.
Girgin, S., Necci, A., and Krausmann, E. (2019). Dealing with cascading multi-hazard risks in national risk assessment: the case of Natech accidents. Int. J. Disaster Risk Reduct. 35, 101072. doi:10.1016/j.ijdrr.2019.101072
Granados, J. A. T., and Roux, A. V. D. (2009). Life and death during the great Depression. Proceedings of the National Academy of Sciences 106 (41), 17290–17295.
Halal, W. E. (2013). Forecasting the technology revolution: results and learnings from the TechCast project. Technol. Forecast. Soc. change 80 (8), 1635–1643. doi:10.1016/j.techfore.2013.02.008
Hall, G. J., and Sargent, T. J. (2022). Three world wars: fiscal–monetary consequences. Proc. Natl. Acad. Sci. U. S. A. 119 (18), e2200349119. doi:10.1073/pnas.2200349119
Hooks, G., and Bloomquist, L. E. (1992). The legacy of world war II for regional growth and decline: the cumulative effects of wartime investments on U.S. Manufacturing, 1947-1972. Soc. Forces 71 (2), 303–337. doi:10.1093/sf/71.2.303
Huggel, C., Bouwer, L. M., Juhola, S., Mechler, R., Muccione, V., Orlove, B., et al. (2022). The existential risk space of climate change. Clim. change 174 (1-2), 8. doi:10.1007/s10584-022-03430-y
Juhola, S., Filatova, T., Hochrainer-Stigler, S., Mechler, R., Scheffran, J., and Schweizer, P. J. (2022). Social tipping points and adaptation limits in the context of systemic risk: concepts, models and governance. Front. Clim. 4. Available at:. doi:10.3389/fclim.2022.1009234
Kahane, A. (2012). Transformative scenario planning: working together to change the future. 1st edn. Oakland, CA: Berrett-Koehler Publishers.
Kavanagh, D., Lightfoot, G., and Lilley, S. (2021). Are we living in a time of particularly rapid social change? And how might we know? Technol. Forecast. Soc. change 169, 120856. doi:10.1016/j.techfore.2021.120856
Kemmerling, B., Schetter, C., and Wirkus, L. (2022). The logics of war and food (in)security. Glob. Food Secur. 33, 100634. doi:10.1016/j.gfs.2022.100634
Kemp, L., Xu, C., Depledge, J., Ebi, K. L., Gibbins, G., Kohler, T. A., et al. (2022). Climate Endgame: exploring catastrophic climate change scenarios. Proc. Natl. Acad. Sci. U. S. A. 119 (34), e2108146119. doi:10.1073/pnas.2108146119
Kobayashi, T., and Takaguchi, T. (2018). Social dynamics of financial networks. EPJ Data Science 7 (1), 1–19.
Landes, D. S. (1999). The wealth and poverty of nations publisher. W. W. Norton and Company. W W Norton and Co Ltd.
Lenton, T. M., Rockström, J., Gaffney, O., Rahmstorf, S., Richardson, K., Steffen, W., et al. (2019). Climate tipping points - too risky to bet against. Nature 575 (7784), 592–595. doi:10.1038/d41586-019-03595-0
Linkov, I., Bridges, T., Creutzig, F., Decker, J., Fox-Lent, C., Kröger, W., et al. (2014). Changing the resilience paradigm. Nat. Clim. change 4 (6), 407–409. doi:10.1038/nclimate2227
Logan, T. M., Aven, T., Guikema, S. D., and Flage, R. (2022). Risk science offers an integrated approach to resilience. Nat. Sustain. 5 (9), 741–748. doi:10.1038/s41893-022-00893-w
Lucas, K., Renn, O., Jaeger, C., and Yang, S. (2018). Systemic risks: a homomorphic approach on the basis of complexity science. Int. J. Disaster Risk Sci. 9 (3), 292–305. doi:10.1007/s13753-018-0185-6
Madsen, J. B. (2001). Trade barriers and the collapse of world trade during the great depression. South. Econ. J. 67 (4), 848–868. doi:10.2307/1061574
Masys, A. J., Yee, E., and Vallerand, A. (2016). ““Black Swans”, “Dragon Kings” and Beyond: Towards Predictability and Suppression of Extreme All-Hazards Events Through Modeling and Simulation”, in Applications of Systems Thinking and Soft Operations Research in Managing Complexity, 131.
Mc Gee, S., Frittman, J., Ahn, S. J., and Murray, S. (2014). Risk relationships and cascading effects in critical infrastructures: IMPLICATIONS for the hyogo framework. Global Assessment Report on Disaster Risk Reduction [Preprint]. Available at: https://www.preventionweb.net/english/hyogo/gar/2015/en/bgdocs/McGee%20et%20al.,%202014.pdf.
Mitoulis, S.-A., Argyroudis, S., Panteli, M., Fuggini, C., Clemente, V., Valkaniotis, S., et al. (2023). Conflict-resilience framework for critical infrastructure peacebuilding, 91. Amsterdam, NL: Sustainable Cities and Society, 104405. doi:10.1016/j.scs.2023.104405
Mitra, A., and Shaw, R. (2023). Systemic risk from a disaster management perspective: a review of current research, 140. Amsterdam, NL: Environmental science and policy, 122–133. doi:10.1016/j.envsci.2022.11.022
Moore, R. J. (2021). Emerging from war: public policy and patterns of foreign direct investment recovery in postwar environments. J. Int. Bus. Policy 4 (4), 455–475. doi:10.1057/s42214-020-00084-4
Murthy, R. S., and Lakshminarayana, R. (2006). Mental health consequences of war: a brief review of research findings. World psychiatry official J. World Psychiatric Assoc. 5 (1), 25–30.
Nag Chowdhury, S., Ray, A., Dana, S. K., and Ghosh, D. (2022). Extreme events in dynamical systems and random walkers: A review. Physics Reports, 966, 1–52.
Nagasaki University Recna, R. C. f. N. W. A., Apln, APLN, and Institute, N. (2021). Pandemic futures and nuclear weapon risks: the nagasaki 75th anniversary pandemic-nuclear nexus scenarios final report. J. Peace Nucl. Disarm. 4 (1), 6–39. doi:10.1080/25751654.2021.1890867
Naqvi, A., and Monasterolo, I. (2021). Assessing the cascading impacts of natural disasters in a multi-layer behavioral network framework. Sci. Rep. 11 (1), 20146. doi:10.1038/s41598-021-99343-4
Nash, G. D. (1990). The American west transformed: the impact of the second world war. Lincoln, NE: University of Nebraska Press.
Nelson, C., Adiguzel, I., Florin, M.-V., Lentzos, F., Knutsson, R., Rhodes, C., et al. (2021). “Foresight in synthetic biology and biotechnology threats,” in Emerging threats of synthetic biology and biotechnology: addressing security and resilience issues. Editor B. D. Trump (Dordrecht (DE): Springer).
Nordhaus, W. D. (2021). Are We approaching an economic singularity? information technology and the future of economic growth. American Economic Journal: Macroeconomics 13 (1), 299–332.
Obinger, H., Schmitt, C., and Seelkopf, L. (2022). “Mass warfare and the development of the modern welfare state: an analysis of the western world, 1914–1950,” in International impacts on social policy: short histories in global perspective. Editors F. Nullmeier, D. González de Reufels, and H. Obinger (Cham: Springer International Publishing), 21–34.
O’Brien, J. T., and Nelson, C. (2020). Assessing the risks posed by the convergence of artificial intelligence and biotechnology. Health Secur. 18 (3), 219–227. doi:10.1089/hs.2019.0122
Otto, I. M., Donges, J. F., Cremades, R., Bhowmik, A., Hewitt, R. J., Lucht, W., et al. (2020). Social tipping dynamics for stabilizing Earth’s climate by 2050. Proc. Natl. Acad. Sci. 117 (5), 2354–2365. doi:10.1073/pnas.1900577117
Parraguez, P., Škec, S., e Carmo, D. O., and Maier, A. (2020). Quantifying technological change as a combinatorial process. Technol. Forecast. Soc. change 151, 119803. doi:10.1016/j.techfore.2019.119803
Perrow, C. (1999). Normal accidents: living with high-risk technologies. Revised edition. Princeton University Press.
Phillips, T., and Watts, J. (2023). Brazilian Amazon at risk of being taken over by mafia, ex-police chief warns. Guard. 1 (6). Available at: https://www.theguardian.com/environment/2023/jun/01/brazil-amazon-crime-dom-phillips-bruno-pereira (Accessed June 1, 2023).
Popkova, E. G., Alekseev, A. N., Lobova, S. V., and Sergi, B. S. (2020). The theory of innovation and innovative development. AI scenarios in Russia. Technol. Soc. 63, 101390. doi:10.1016/j.techsoc.2020.101390
Ramsay, K. W. (2017). Information, uncertainty, and war. Annu. Rev. Political Sci. 20 (1), 505–527. doi:10.1146/annurev-polisci-051215-022729
Reuveny, R., Mihalache-O’Keef, A. S., and Li, Q. (2010). The effect of warfare on the environment. J. peace Res. 47 (6), 749–761. doi:10.1177/0022343310382069
Reynolds, D. (1992). “Power and superpower: the impact of two world wars on America’s international role,” in America unbound: world war II and the making of a superpower. Editor W. F. Kimball (New York: Palgrave Macmillan US), 13–35.
Riahi, K., Rao, S., Krey, V., Cho, C., Chirkov, V., Fischer, G., et al. (2011). RCP 8.5—a scenario of comparatively high greenhouse gas emissions. Clim. change 109 (1), 33–57. doi:10.1007/s10584-011-0149-y
Rigolot, C. (2020). Transdisciplinarity as a discipline and a way of being: complementarities and creative tensions. Humanit. Soc. Sci. Commun. 7 (1), 100–105. doi:10.1057/s41599-020-00598-5
Rutting, L., Vervoort, J., Mees, H., and Driessen, P. (2022). Strengthening foresight for governance of social-ecological systems: an interdisciplinary perspective, Futures, 102988. doi:10.1016/j.futures.2022.102988
Sachs, J. D. (2009). Blackouts and cascading failures of the global markets. Sci. Am. 1 (1). doi:10.1038/scientificamerican0109-34
Sandberg, A., and Nelson, C. (2020). Who should we fear more: biohackers, disgruntled postdocs, or bad governments? A simple risk chain model of biorisk. Health Secur. 18 (3), 155–163. doi:10.1089/hs.2019.0115
Schläpfer, M., Bettencourt, L. M. A., Grauwin, S., Raschke, M., Claxton, R., Smoreda, Z., et al. (2014). The scaling of human interactions with city size. Journal of the Royal Society, Interface/the Royal Society 11 (98), 20130789.
Schweizer, P.-J. (2021). Systemic risks – concepts and challenges for risk governance. J. risk Res. 24 (1), 78–93. doi:10.1080/13669877.2019.1687574
Schweizer, P.-J., and Renn, O. (2019). Governance of systemic risks for disaster prevention and mitigation. Disaster Prev. Manag. Int. J. 28 (6), 862–874. doi:10.1108/dpm-09-2019-0282
Shepherd, T. G., Boyd, E., Calel, R. A., Chapman, S. C., Dessai, S., Dima-West, I. M., et al. (2018). Storylines: an alternative approach to representing uncertainty in physical aspects of climate change. Clim. change 151 (3), 555–571. doi:10.1007/s10584-018-2317-9
Shirer, W. L., and Rosenbaum, R. (2011). The rise and fall of the third Reich: a history of nazi Germany. Reissue edition. New York, NY: Simon and Schuster.
Sillmann, J., Shepherd, T. G., van den Hurk, B., Hazeleger, W., Martius, O., Slingo, J., et al. (2021). Event-based storylines to address climate risk. Earth’s future 9 (2). Available at:. doi:10.1029/2020ef001783
Sornette, D. (2009). Dragon-Kings, Black swans and the prediction of crises. International Journal of Terraspace Science and Engineering 2 (1), 1–18.
Sornette, D. (2017) Why Stock Markets Crash: Critical Events in Complex Financial Systems (Princeton Science Library, 49). Revised edition. Princeton University Press.
Steffen, W., Rockström, J., Richardson, K., Lenton, T. M., Folke, C., Liverman, D., et al. (2018). Trajectories of the earth system in the anthropocene. Proc. Natl. Acad. Sci. 115 (33), 8252–8259. doi:10.1073/pnas.1810141115
Taleb, N. N. (2007). The black swan: the impact of the highly improbable. The New York Times. Available at: https://www.nytimes.com/2007/04/22/books/chapters/0422-1st-tale.html (Accessed: 17 November 2023).
Taleb, N. N. N. (2014). Antifragile: things that gain from disorder (Incerto). Reprint edition. Random House Publishing Group.
The Oxford World History of Empire (2021). Volume one: the imperial experience. Oxford University Press.
Torregrosa-Hetland, S., and Sabaté, O. (2021). Income tax progressivity and inflation during the world wars. Eur. Rev. Econ. Hist. 26 (3), 311–339. doi:10.1093/ereh/heab020
Tsai, P.-H., Berleant, D., Segall, R. S., Aboudja, H., Batthula, V. J. R., Duggirala, S., et al. (2023). Quantitative technology forecasting: a review of trend extrapolation methods. Int. J. Innovation Technol. Manag. 20 (04), 2330002. doi:10.1142/s0219877023300021
Turchin, A., and Denkenberger, D. (2020). Classification of global catastrophic risks connected with artificial intelligence. AI Soc. 35 (1), 147–163. doi:10.1007/s00146-018-0845-5
Undheim, T. A. (2023). Extinction scenarios for 2075: videos and narratives, stanford SERI. Available at: https://seri.stanford.edu/research/stanford-global-systemic-risk-scenarios-study/extinction-scenarios-2075-videos-and.
Villani, M., and Serra, R. (2023). Super-exponential growth in models of a binary string world. Entropy 25 (1). doi:10.3390/e25010168
Walmsley, T., Rose, A., John, R., Wei, D., Hlávka, J. P., Machado, J., et al. (2023). Macroeconomic consequences of the COVID-19 pandemic. Econ. Model. 120, 106147. doi:10.1016/j.econmod.2022.106147
West, G. (2018). Scale: the universal laws of life, growth, and death in organisms, cities, and companies. Reprint edition. Penguin Books.
White, J. M., and Lidskog, R. (2022). Ignorance and the regulation of artificial intelligence. J. risk Res. 25 (4), 488–500. doi:10.1080/13669877.2021.1957985
Witt, U. (2016). What kind of innovations do we need to secure our future? J. Open Innovation Technol. Mark. Complex. 2 (3), 1–14. doi:10.1186/s40852-016-0043-y
Zhang, J., Li, X., Wang, X., Wang, W. -X., and Wang, L. (2015). Scaling behaviours in the growth of networked systems and their geometric origins. Scientific Reports 5, 9767.
Keywords: multiplicative effects, cascading risks, scenario methods, systemic risk, complex systems, emerging technology, financial markets, economic growth
Citation: Undheim TA and Ahmad T (2024) Quantitative scenarios for cascading risks in AI, climate, synthetic bio, and financial markets by 2075. Front. Complex Syst. 2:1323321. doi: 10.3389/fcpxs.2024.1323321
Received: 17 October 2023; Accepted: 19 February 2024;
Published: 15 March 2024.
Edited by:
Georgios Balasis, National Observatory of Athens, GreeceReviewed by:
Guido Fioretti, University of Bologna, ItalyConstantinos Papadimitriou, National Observatory of Athens, Greece
Copyright © 2024 Undheim and Ahmad. This is an open-access article distributed under the terms of the Creative Commons Attribution License (CC BY). The use, distribution or reproduction in other forums is permitted, provided the original author(s) and the copyright owner(s) are credited and that the original publication in this journal is cited, in accordance with accepted academic practice. No use, distribution or reproduction is permitted which does not comply with these terms.
*Correspondence: Trond Arne Undheim, dHJvbmR1bkBzdGFuZm9yZC5lZHU=