- 1Department of Nephrology, The First Medical Center, Chinese PLA General Hospital, Chinese PLA Institute of Nephrology, State Key Laboratory of Kidney Diseases, National Clinical Research Center for Kidney Diseases, Beijing, China
- 2Beijing Computing Center, Beike Industry, Yongfeng Industrial Base, Beijing, China
- 3Department of Nephrology, Hainan Hospital of Chinese PLA General Hospital, the Hainan Academician Team Innovation Center, Sanya, China
Background: The disease pathology for diabetes mellitus patients with chronic kidney disease (CKD) may be diabetic nephropathy (DN), non-diabetic renal disease (NDRD), or DN combined with NDRD. Considering that the prognosis and treatment of DN and NDRD differ, their differential diagnosis is of significance. Renal pathological biopsy is the gold standard for diagnosing DN and NDRD. However, it is invasive and cannot be implemented in many patients due to contraindications. This article constructed a new noninvasive evaluation model for differentiating DN and NDRD.
Methods: We retrospectively screened 1,030 patients with type 2 diabetes who has undergone kidney biopsy from January 2005 to March 2017 in a single center. Variables were ranked according to importance, and the machine learning methods (random forest, RF, and support vector machine, SVM) were then used to construct the model. The final model was validated with an external group (338 patients, April 2017–April 2019).
Results: In total, 929 patients were assigned. Ten variables were selected for model development. The areas under the receiver operating characteristic curves (AUCROCs) for the RF and SVM methods were 0.953 and 0.947, respectively. Additionally, 329 patients were analyzed for external validation. The AUCROCs for the external validation of the RF and SVM methods were 0.920 and 0.911, respectively.
Conclusion: We successfully constructed a predictive model for DN and NDRD using machine learning methods, which were better than our regression methods.
Clinical Trial Registration: ClinicalTrial.gov, NCT03865914.
1 Introduction
As lifestyle changes, the number of diabetes mellitus (DM) patients increases globally, particularly in those with type 2 DM (T2DM) (1). The latest edition (10th edition) of the International Diabetes Federation Diabetes Atlas shows that 537 million adults are currently living with diabetes globally, and there are 140.9 million DM patients in China, which is the country with the largest number of DM patients (2). Diabetic nephropathy (DN) is one of the most important microvascular complications caused by DM, and approximately 30–40% of DM patients develop DN (3), while one-third of DN eventually develop into end-stage renal disease (ESRD) (4, 5). DM has become the major cause of ESRD worldwide (4). In 2018, ESRD was attributed to diabetes in Singapore (66.4%), Malaysia (66.2%), Qatar (63.9%), Hong Kong (52.0), and China (13.30%) (6, 7).
The disease pathology for DM patients with CKD may be DN, non-diabetic renal disease (NDRD), or DN combined with NDRD. Considering that the prognosis and treatment of DN and NDRD differ, their differential diagnosis is significant (8). Compared to DN, NDRD would have a better renal prognosis and longer survival in most cases. The 2007 Kidney Disease Outcomes Quality Initiative (KDOQI) guidelines are widely used for the clinical distinguish between DN and NDRD, but our previous study found that the specificity (40.63%) of the KDOQI guidelines was insufficient (9).
Some indicators, such as diabetic retinopathy (DR), DM course, and Hb could help in identifying DN and NDRD (10–12). However, the efficiency and accuracy of a single indicator may be insufficient. Currently, renal pathological biopsy is the gold standard for diagnosing DN and NDRD. However, its implementation in patients with diabetes-related CKD is subject to various contraindications, and the patient refused. Moreover, it is invasive and may cause complications.
In our previous study, we used the regression method to establish the distinguishing model (13, 14). Currently, machine learning methods have many advantages compared to traditional regression methods (15). In this study, we used support vector machine (SVM) and random forest (RF) methods to build new models (16, 17).
Using renal pathological biopsy as the gold standard, we established a noninvasive differential diagnostic model based on SMM and RF to distinguish DN and NDRD patients.
2 Materials and Methods
2.1 Patient Selection
This retrospective study included 1,030 consecutive patients with type 2 diabetes who required kidney biopsy at our institution from January 2005 to March 2017 for model development. Additionally, we screened 338 patients from April 2017 to April 2019 at our center for external validation. The variables used for external validation were the same as the variables in the development set. This study was attached to a registered clinical trial [ClinicalTrial.gov. Registry (NCT03865914)].
The study protocol was approved by the Medicine Ethics Committee of the Chinese People’s Liberation Army General Hospital (Approval No. S2014-012-01). Each patient provided written informed consent before their participation in the study. All patients were screened according to the protocol shown in Figure 1. Patients were classified as having DN, NDRD, or DN combined with NDRD based on the results of the kidney biopsy. Patients with both DN and NDRD were excluded from the modeling population (13).
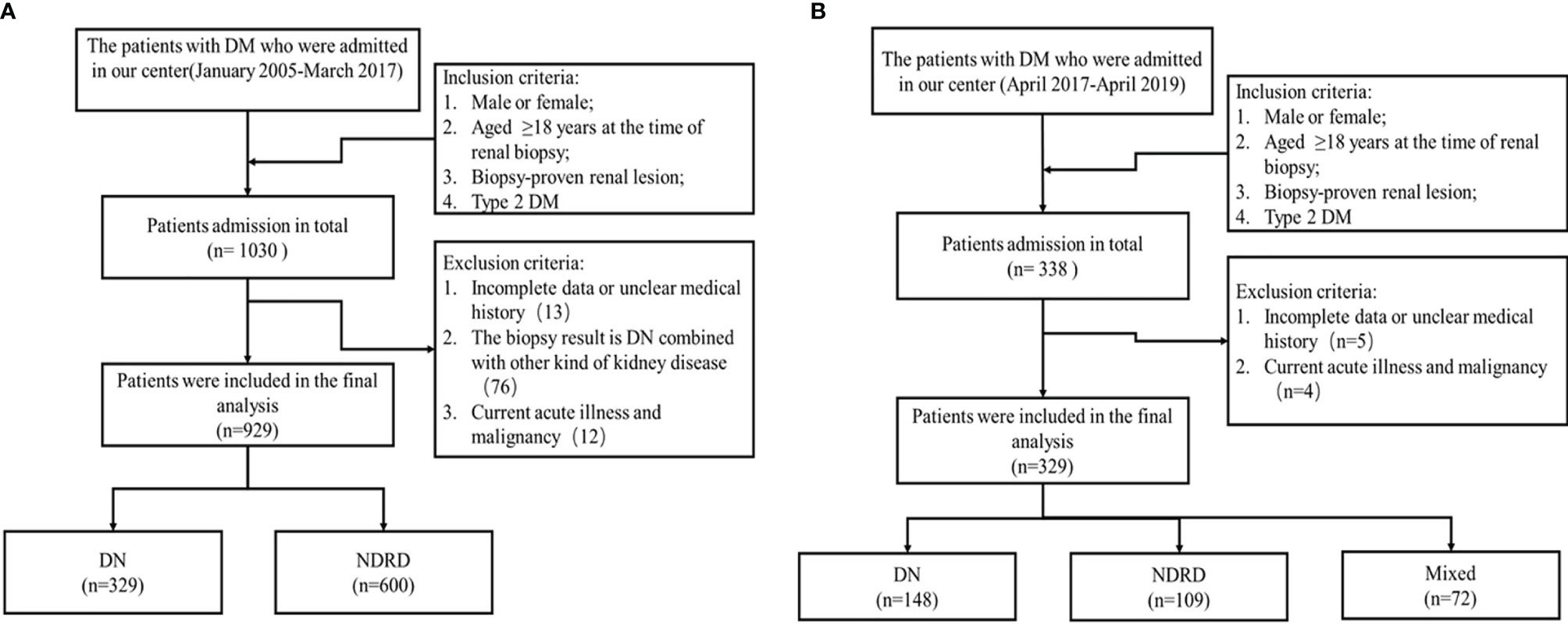
Figure 1 Patient screening process. (A) Screening process for the modeling group. (B) Screening process for the external validation group. Mixed: the biopsy result is DN combined with other kind of kidney disease.
All patients underwent kidney biopsy after signing the informed consent form. The indications for kidney biopsy were consistent with our previous standards (14). The kidney biopsy indications for the suspected diagnosis of NDRD at our center were in accordance with those listed in the 2007 KDOQI guidelines (18).
Type 2 diabetes was defined according to the World Health Organization criteria (19). DN was diagnosed on the basis of histological characteristics, such as glomerular hypertrophy, thickened capillary basement membranes, diffuse mesangial expansion, nodular mesangial sclerosis, exudative lesions such as capsular drop or fibrin cap, mesangiolysis, capillary microaneurysm, or hyalinosis of afferent and efferent arterioles (20). NDRD was diagnosed on the basis of the classical criteria (21). The results were obtained independently from two pathologists. Discordant results were solved through a discussion.
2.2 Data Collection
Baseline characteristics and clinical parameters were collected from all patients before kidney biopsy. Baseline characteristics included age, sex, body mass index (BMI), blood pressure (BP), and medical history of DM and/or hypertension. Laboratory data obtained included measurements of HbA1c, hemoglobin (Hb), serum creatinine (sCr), serum albumin (ALB), estimated glomerular filtration rate (eGFR), and serum lipid levels. Variable definitions have been provided in previous studies (9).
2.3 Variable Selection and Treatment of Missing Data
The variable importance of all 49 candidate variables (listed in Supplementary Table 1) was ranked using the RF classification method. Missing values were imputed by predictive mean matching. The Gini index was used for decisions related to variable ranking or node splitting. The importance and clinical significance of all variables were evaluated to rank the variables.
2.4 Model Building
All processes are shown in Figure 2. For new model development, SVM and RF methods were used.
Model construction steps: the entire modeling used RF and SVM models. RF modeling used the ranger module in the R language (parameter: importance = “impurity”), and SVM modeling used the kernellab module in the R language (parameters: kernel = “rbfdot”; C = 2, cross = 5). The detailed modeling steps are described as follows:
(1) Random forest and support vector machine modeling were performed for 929 samples in the training set (including 49 variables in total) (note: the steps were performed on the RF and SVM models respectively).
(2) The RF model was used to screen 49 variables, and finally, 10 features were determined as variables in the final modeling.
(3) The 929 samples of the training set and the 10 final selected variables were used to perform five-fold cross modeling (80% of the randomly divided data is used as the training set, and 20% as the verification set), and the modeling results were evaluated.
(4) Modeling was performed with all 929 samples.
(5) The final model was constructed using all the samples, and the 329 externally reserved samples were used as the test data set; model evaluation was performed, and the receiver operating characteristic (ROC) curve was drawn.
2.4.1 Random Forest
The RF machine learning algorithm is an ensemble tree method that can generate many regression trees to detect interactions. At each split, a candidate variable that maximizes the difference in cumulative hazard between the daughter nodes was chosen, and the splitting stopped at the terminal nodes when the data at hand can be split such that each terminal node has at least one unique outcome (22).
2.4.2 Support Vector Machine
The SVM algorithm was originally proposed by Vapnik and colleagues in 1963 (23). It creates a decision boundary, known as the hyperplane, between two classes (24). The input variables entered were either continuous or categorical data in the SVM, whereas the output variables entered were binary data. The dataset was further divided into training and validation subsets. The variables were entered into the classifier to yield the final model.
2.4.3 Logistic Regression
Logistic regression was performed using SPSS version 20.0 (IBM Corp., Armonk, NY). Accuracy and effectiveness were calculated using the formulas previously established by Zhou Jianhui (2008) and Liu Moyan (2014) at our center (9, 10), which were abbreviated as model-2008 and model-2014. The two formulas are listed in Supplementary Table 2.
2.4.4 Other Statistical Analyses
The remaining statistical analyses were performed using SPSS version 20.0 (IBM Corp., Armonk, NY) and R version 3.5.1 (R Foundation for Statistical Computing, Vienna, Austria) (25). Clinical and laboratory features were compared between the groups through analysis of variance. This information is presented as means and standard deviations. Kruskal–Wallis tests were performed to compare medians and interquartile ranges. Pearson’s chi-square test was used for numbers and proportions; the test was used to compare performances among models. A two-tailed P-value of <0.05 was considered statistically significant.
3 Results
3.1 Trial Population
In total, 929 patients were included in the development set and assigned to one of the two groups (DN and NDRD), based on the outcomes of the kidney biopsy. Patient characteristics are shown in Supplementary Table 3. The mean age (at the time of kidney biopsy) was 51.34 ± 10.02 (range, 19–85) years. Patients in the DN group had higher BP than those in the NDRD group (p <0.05). Patients with DN were more likely to experience DR and cardiovascular and cerebrovascular diseases (CCVD) than those with NDRD. Compared with patients with DN, patients with NDRD were more likely to have higher levels of Hb, BMI, ALB level, eGFR, Ucr, serum lipid, total cholesterol, and lower levels of SBP, DBP, MAP, pulse pressure, FBG, 24-hour proteinuria, and BUA.
3.2 Pathological Results of the NDRD Group
The results of the kidney biopsy indicated that 329 (35.41%) and 600 (64.59%) patients had DN and NDRD, respectively. Numerous pathological subtypes were observed in the NDRD group; membranous nephropathy was frequently observed (32.33%), followed by IgA nephropathy (30.83%), and then mesangial proliferative glomerulonephritis (6.00%) (Supplementary Table 4).
3.3 Importance Ranking
Various predictors were ranked based on their order of importance, using the RF method. DR was found to be the most important, followed by the duration of DM and then Hb, pulse pressure (PP), sCr, and ALB levels (Supplementary Table 5 lists the top 21).
3.4 Traversal Modeling and Selected Markers
We exhausted all combinations of variables (variables ranged from 6 to 12) for RF and SVM, and the best combinations are listed in Supplementary Table 6. Moreover, we observed that the increase in performance with ≥10 markers was marginal (Supplementary Figure 1). We then obtained the best combination of the variables, which consisted of the following: DR, DM course, Hb, PP, sCr, ALB, total cholesterol (TC) levels, sudden onset of heavy proteinuria, hematuria, and family history of DM.
3.5 Model Building
The performances of RF and SVM are shown in Tables 1, 2. The AUCROC for RF reached 0.953 (0.939–0.967), and that for the SVM method reached 0.947 (0.931–0.963).
3.6 External Validation
In total, 329 patients were enrolled for external validation, and their characteristics are presented in Supplementary Table 7. Validations were performed using the same variables as in the development set. We validated the four models under two conditions. First, the calculations were performed under ideal conditions (isolated DN vs. isolated NDRD). Second, the calculations were performed under actual conditions (DN patients vs. non-DN patients [NDRD with and without DN]) (9, 26). Regarding DN vs. NDRD, the AUCROC for the RF reached 0.920, and the SVM reached 0.911; model-2008 reached 0.886, and model-2014 reached 0.917. For DN vs. non-DN patients, the AUCROC for RF also reached 0.855, and SVM reached 0.846; model-2008 reached 0.821, and model-2014 reached 0.841 (Table 3; Supplementary Figure 2).
The comparison among the four models showed that RF and SVM do have better diagnostic performance than logistic regression models (Supplementary Table 8). The chi-square test also showed that there was no significant difference for each model between the two conditions (isolated DN vs. isolated NDRD; DN vs. non-DN), which means that these models also have good performances in the real world (Supplementary Table 9).
4 Discussion
Although some CKD patients have a clear history of diabetes and other complications (such as DR), which are clinically suspected to be diabetic nephropathy, there are still some kidney biopsy results of NDRD or DN combined with NDRD. The incidence of DN and NDRD varies greatly in different studies. The prevalence of DN ranges from 6.5 to 94% with an average of 41.3% (27–29); NDRD ranges from 3 to 93.5% with an average of 40.6% (29–31); and DN with NDKD ranges from 0 to 45.5%, with an average of 18.1% (29) in the CKD patients with T2DM history. Our study showed that 64.58% of biopsied type 2 diabetic patients were diagnosed with NDRD.
In our study, membranous nephropathy (MN) was the most common type, accounting for 32.33% of all NDRD cases, followed by IgA nephropathy, which accounted for 30.83%. In previous studies, MN (7 to 35%) was the most common cause of NDRD (32, 33), ranging from 7 to 35% (34–37). Some studies have also shown IgA nephropathy was the most common type of NDRD, which ranged from 3 to 59% (31, 38–40). Some researchers found that the prevalence of membranous nephropathy increased from 12 to 24% during the last decades (41), which might be due to the impact of air pollution and other factors (42).
DR, DM course, Hb levels, PP, and hematuria were among the most important indicators of diagnosis through importance ranking in our study. Same with the previous studies, which reported that the absence of DR (43), hematuria (44), and shorter course of DM (45) are risk factors for NDRD, while lower hemoglobin (32) and high BP (44) levels are risk factors for DN. We recently listed the published models for differential diagnosis of DN and NDRD (Supplementary Table 10) (13, 14, 46–48), which are most based on logistic regression analyses. Compared with the previous studies, we made use of larger samples and new methods to build our modes, and we compared two of them with our models in external groups.
DR ranked first in all indicators; DR was a co-occurrence in 79.3% of DN patients in this study. Previous studies ranged from 37 to 84% (49–51). In most cases, the kidney and eye were damaged at the same time for DM patients. It used to be emphasized that the absence of DR indicates a high likelihood of NDRD but not always, for organ heterogeneity, and they might have different protective factors. Some patients did not always have DR and DN at the same time. Studies showed that 10–70% of biopsied DN patients did not have DR, and 6–57.4% of biopsied NDRD patients had DR (48, 52–55). Thus, the single DR index may make mistakes in verifying DN and NDRD.
The presence of microhematuria in diabetic patients with proteinuria may range from 11 to 76–78% (11, 56, 57), while that in DN patients ranges from 5 to 75%. In our study, the prevalence of hematuria in the NDRD group was significantly higher than that in the DN group (5.8% versus 24.2%). It was believed that hematuria was of great significance in distinguishing between DN and NDRD. But recently, a meta-analysis concluded that type 2 diabetes patients presenting with hematuria may be slightly more likely to develop NDRD (58).
In our study, the DN group tended to have more severe hypertension than the NDRD group. The systolic and diastolic blood pressures (SBP and DBP) of the DN group were higher than those of the NDRD group, and this result is consistent with that in our previous study, which reported that lower BP was a good predictor of NDRD (10, 13, 59). In our study, lower PP was a better predictor of NDRD than both SBP and DBP in our study. DN, as the microvascular disease of DM, has many typical vascular lesions, such as arteriolar hyalinosis, arteriosclerosis, and the presence of large vessels (60), which might lead to a higher PP in DN patients.
Kidney biopsy is the gold standard method for diagnosis, but it cannot be performed on all patients because of contraindications (anticoagulation, active bleeding, and unilateral nephrectomy) and reluctance to undergo biopsy. As it is an invasive technique with certain risks, such as hematuria and perirenal hematoma, arterial embolization might be required (61, 62). Moreover, there is no consensus on the indications for biopsy in DM patients (63, 64). It is believed that the main purpose of biopsy is to detect cases of NDRD (63, 64). Nephrotomy was implemented to clarify its pathological type and implement earlier intervention (62). The rates of renal biopsies performed in patients with DN are variable, ranging from 12 to 80% (65–68). Thus, the limited accuracy of a single indicator and the implementation of kidney biopsy make it of great significance to establish a non-invasive differential diagnosis model for the diagnosis of DN and NDRD.
The previous non-invasive identification guidelines widely used internationally are the 2007 KDOQI guidelines (18), which were evaluated in Chinese patients and found that many of the predictors were binary categorical variables. The low specificity of the guidelines renders them not suitable for diagnostic criteria (9). So we built the models using the logistic regression method in 2008 and 2014 (13, 14). Recently, Jiang et al. [(47), n = 302)], Yang et al. [(46), n = 213)], and García-Martín [(48), n = 207)] also used the logistic regression method to build the diagnostic models, and all the models found the index like DR and DM course are important. In this study, we developed new models with machine learning and compared them with our previous models established in 2008 and 2014. The results showed that RF and SVM were superior to the traditional method.
Machine learning has been used in many diabetes-related CKD studies, such as genotype–phenotype risk patterns (69) and ensemble feature selection for clinical markers (70). It is also used to select the risk factors for diabetes-related CKD development (70, 71) or to construct a model to detect the progression of diabetes-related CKD (72). In this study, we used it to distinguish between DN and NDRD.
This study had several limitations. First, the time span of patients included is quite large (2005–2017); thus, the disease patterns for DM patients combined with CKD may change over this long period. Therefore, we built a new model using a new population (2015–2017), and it was verified externally. The 2015–2017 model did not show better performance (Supplementary Table 10) than the previous model (2005–2017), which indicates that the large time span of enrollment has little influence. Second, the model mainly included clinical predictors rather than some new predictors, which may limit the further improvement of the model accuracy. Finally, this is a retrospective and single-center study in a developing country like China. It may not be suitable for other countries.
Despite the above limitations, this model has provided a new method for the clinical differentiation between DN and NDRD. Our research has many advantages: 1. a large sample size avoids bias considerably; 2. all parameters were routine clinical variables that can be easily obtained and used by clinicians; 3. external verification shows that the models have low learning and test errors; 4. to enhance the effect of its practical application, we also verified patients with DN combined with NDRD, which indicated that the model has good feasibility in the real world; and 5. kidney biopsy results are the gold standard for verifying the accuracy of the models.
In conclusion, the main purpose of this study was to establish a noninvasive method to differentially diagnose DN and NDRD. As a result, fewer kidney biopsies can be performed, reducing the suffering and costs for patients.
Data Availability Statement
The original contributions presented in the study are included in the article/Supplementary Material. Further inquiries can be directed to the corresponding author.
Ethics Statement
Written informed consent was obtained from the individual(s) for the publication of any potentially identifiable images or data included in this article.
Author Contributions
WZ: Design of the experiment, data statistics, and model building. XL: Data collection, statistical analysis, and model building. ZD and QW: Data collection. ZP: Statistical analysis and model building. YC, YW, and YZ: Collecting clinical data. PC and ZF: Kidney biopsy. XS, GC, and XC: Design of the experiment. All authors listed have made a substantial, direct, and intellectual contribution to the work and approved it for publication.
Funding
This study was supported by the National Natural Science Foundation Of China (32141005), the Science & Technology Project of Beijing China (no. D171100002817002), the Beijing Municipal Science and Technology project (no. Z181100001918015), the National Basic Research Program of China (no. 2015CB553605), the National Key R&D Program of China (Nos. 2016YFC1305500, 2016YFC1305503), the Natural Science Foundation of China (Nos. 81601211, 8160050078), and the Specialized Scientific Program of the Hainan Province Academician Innovation Platform (YSPTZX202026).
Conflict of Interest
The authors declare that the research was conducted in the absence of any commercial or financial relationships that could be construed as a potential conflict of interest.
Publisher’s Note
All claims expressed in this article are solely those of the authors and do not necessarily represent those of their affiliated organizations, or those of the publisher, the editors and the reviewers. Any product that may be evaluated in this article, or claim that may be made by its manufacturer, is not guaranteed or endorsed by the publisher.
Acknowledgments
We are thankful to Elsevier Webshop for providing language support. We thank all patients who participated in this study, as well as all doctors, pathologists, and nurses in the 301 Nephrology Department and the Beijing Computing Center.
Supplementary Material
The Supplementary Material for this article can be found online at: https://www.frontiersin.org/articles/10.3389/fendo.2022.913021/full#supplementary-material
Abbreviations
AUCROC, Area under the receiver operating characteristic curve; BP, Blood pressure; BMI, Body mass index; CKD, Chronic kidney disease; DM, Diabetes mellitus; DN, Diabetic nephropathy; DR, Diabetic retinopathy; Hb, Hemoglobin; KDOQI, Kidney Disease Outcomes Quality Initiative; NDRD, Nondiabetic renal disease; RF, Random forest; ALB, Serum albumin; sCr, Serum creatinine; SVM, Support vector machine.
References
2. Federation.ID. IDF Diabetes Atlas, 10th edn. (2021). https://diabetesatlas.org/atlas/tenth-edition/
3. Umanath K, Lewis JB. Update on Diabetic Nephropathy: Core Curriculum 2018. Am J Kidney Dis (2018) 71(6):884–95. doi: 10.1053/j.ajkd.2017.10.026
4. Jha V, Garcia-Garcia G, Iseki K, Li Z, Naicker S, Plattner B, et al. Chronic Kidney Disease: Global Dimension and Perspectives. Lancet (2013) 382(9888):260–72:382. doi: 10.1016/S0140-6736(13)60687-X
5. Zhang L, Long J, Jiang W, Shi Y, He X, Zhou Z, et al. Trends in Chronic Kidney Disease in China. N Engl J Med (2016) 375(9):905–6. doi: 10.1056/NEJMc1602469
6. Chinese National Renal Data System, C. In: Chinese National Renal Data System (2019). www.cnrds.net.
7. System USRD. 2020 usrds Annual Data Report: Epidemiology of Kidney Disease in the United States. (2020). national Institutes of Health, National Institute of Diabetes and Digestive and Kidney Diseasess:Bethesda, Md
8. Anders HJ, Huber TB, Isermann B, Schiffer M. CKD in Diabetes: Diabetic Kidney Disease Versus Nondiabetic Kidney Disease. Nat Rev Nephrol (2018) 14(6):361–77. doi: 10.1038/s41581-018-0001-y
9. Liu XM, Dong ZY, Zhang WG, Liu MY, Zhou JH, Wang Q, et al. Validation of the 2007 Kidney Disease Outcomes Quality Initiative Clinical Practice Guideline for the Diagnosis of Diabetic Nephropathy and Nondiabetic Renal Disease in Chinese Patients. Diabetes Res Clin Pract (2019) 147:81–6. doi: 10.1016/j.diabres.2018.11.008
10. Liang S, Zhang XG, Cai GY, Zhu HY, Zhou JH, Wu J, et al. Identifying Parameters to Distinguish non-Diabetic Renal Diseases From Diabetic Nephropathy in Patients With Type 2 Diabetes Mellitus: A Meta-Analysis. PLos One (2013) 8(5):e64184. doi: 10.1371/journal.pone.0064184
11. Oh SW, Kim S, Na KY, Chae DW, Kim S, Jin DC, et al. Clinical Implications of Pathologic Diagnosis and Classification for Diabetic Nephropathy. Diabetes Res Clin Pract (2012) 97(3):418–24. doi: 10.1016/j.diabres.2012.03.016
12. Dong Z, Wang Y, Qiu Q, Zhang X, Zhang L, Wu J, et al. Clinical Predictors Differentiating non-Diabetic Renal Diseases From Diabetic Nephropathy in a Large Population of Type 2 Diabetes Patients. Diabetes Res Clin Pract (2016) 121:112–8. doi: 10.1016/j.diabres.2016.09.005
13. Zhou J, Chen X, Xie Y, Li J, Yamanaka N, Tong X. A Differential Diagnostic Model of Diabetic Nephropathy and non-Diabetic Renal Diseases. Nephrol Dial Transplant (2008) 23(6):1940–5. doi: 10.1093/ndt/gfm897
14. Liu MY, Chen XM, Sun XF, Zhou JH, Zhang XG, Zhu HY, et al. Validation of a Differential Diagnostic Model of Diabetic Nephropathy and non-Diabetic Renal Diseases and the Establishment of a New Diagnostic Model. J Diabetes (2014) 6(6):519–26. doi: 10.1111/1753-0407.12150
15. Handelman GS, Kok HK, Chandra RV, Razavi AH, Lee MJ, Asadi H. Edoctor: Machine Learning and the Future of Medicine. J Intern Med (2018) 284(6):603–19. doi: 10.1111/joim.12822
16. Chuang LC, Kuo PH. Building a Genetic Risk Model for Bipolar Disorder From Genome-Wide Association Data With Random Forest Algorithm. Sci Rep (2017) 7:39943. doi: 10.1038/srep39943
17. Ahmad T, Lund LH, Rao P, Ghosh R, Warier P, Vaccaro B, et al. Machine Learning Methods Improve Prognostication, Identify Clinically Distinct Phenotypes, and Detect Heterogeneity in Response to Therapy in a Large Cohort of Heart Failure Patients. J Am Heart Assoc (2018) 7(8). doi: 10.1161/JAHA.117.008081
18. KDOQI. KDOQI Clinical Practice Guidelines and Clinical Practice Recommendations for Diabetes and Chronic Kidney Disease. Am J Kidney Dis (2007) 49(2 Suppl 2):S12–154. doi: 10.1053/j.ajkd.2006.12.005
19. Alberti KG, Zimmet PZ. Definition, Diagnosis and Classification of Diabetes Mellitus and its Complications. Part 1: Diagnosis and Classification of Diabetes Mellitus Provisional Report of a WHO Consultation. Diabetes Med (1998) 15(7):539–53. doi: 10.1002/(SICI)1096-9136(199807)15:7<539::AID-DIA668>3.0.CO;2-S
20. Shimizu M, Furuichi K, Toyama T, Kitajima S, Hara A, Kitagawa K, et al. Long-Term Outcomes of Japanese Type 2 Diabetic Patients With Biopsy-Proven Diabetic Nephropathy. Diabetes Care (2013) 36(11):3655–62. doi: 10.2337/dc13-0298
21. Churg J BJ, Glassock RJ. Renal Disease: Classification and Atlas of Glomerular Diseases. Igaku-Shoin Medical Pub (1995).
22. Ambale-Venkatesh B, Yang X, Wu CO, Liu K, Hundley WG, McClelland R, et al. Cardiovascular Event Prediction by Machine Learning: The Multi-Ethnic Study of Atherosclerosis. Circ Res (2017) 121(9):1092–101. doi: 10.1161/CIRCRESAHA.117.311312
23. Salvatore C, Battista P, Castiglioni I. Frontiers for the Early Diagnosis of AD by Means of MRI Brain Imaging and Support Vector Machines. Curr Alzheimer Res (2016) 13(5):509–33. doi: 10.2174/1567205013666151116141705
24. Huang S, Cai N, Pacheco PP, Narrandes S, Wang Y, Xu W. Applications of Support Vector Machine (SVM) Learning in Cancer Genomics. Cancer Genomics Proteomics (2018) 15(1):41–51. doi: 10.21873/cgp.20063
25. Team RC. R: A Language and Environment for Statistical Computing. In: R Foundation for Statistical Computing. Vienna, Austria (2018).
26. Kurts C, Panzer U, Anders HJ, Rees AJ. The Immune System and Kidney Disease: Basic Concepts and Clinical Implications. Nat Rev Immunol (2013) 13(10):738–53. doi: 10.1038/nri3523
27. Zhuo L, Ren W, Li W, Zou G, Lu J. Evaluation of Renal Biopsies in Type 2 Diabetic Patients With Kidney Disease: A Clinicopathological Study of 216 Cases. Int Urol Nephrol (2013) 45(1):173–9. doi: 10.1007/s11255-012-0164-6
28. Fiorentino M, Bolignano D, Tesar V, Pisano A, Biesen WV, Tripepi G, et al. Renal Biopsy in Patients With Diabetes: A Pooled Meta-Analysis of 48 Studies. Nephrol Dial Transplant (2017) 32(1):97–110. doi: 10.1093/ndt/gfw070
29. Tong X, Yu Q, Ankawi G, Pang B, Yang B, Yang H. Insights Into the Role of Renal Biopsy in Patients With T2DM: A Literature Review of Global Renal Biopsy Results. Diabetes Ther (2020) 11(9):1983–99. doi: 10.1007/s13300-020-00888-w
30. Olsen S, Mogensen CE. How Often is NIDDM Complicated With non-Diabetic Renal Disease? An Analysis of Renal Biopsies and the Literature. Diabetologia (1996) 39(12):1638–45. doi: 10.1007/s001250050628
31. Mak SK, Gwi E, Chan KW, Wong PN, Lo KY, Lee KF, et al. Clinical Predictors of non-Diabetic Renal Disease in Patients With non-Insulin Dependent Diabetes Mellitus. Nephrol Dial Transplant (1997) 12(12):2588–91. doi: 10.1093/ndt/12.12.2588
32. Chang TI, Park JT, Kim JK, Kim SJ, Oh HJ, Yoo DE, et al. Renal Outcomes in Patients With Type 2 Diabetes With or Without Coexisting non-Diabetic Renal Disease. Diabetes Res Clin Pract (2011) 92(2):198–204. doi: 10.1016/j.diabres.2011.01.017
33. Liu D, Huang T, Chen N, Xu G, Zhang P, Luo Y, et al. The Modern Spectrum of Biopsy-Proven Renal Disease in Chinese Diabetic Patients-a Retrospective Descriptive Study. PeerJ (2018) 6:e4522. doi: 10.7717/peerj.4522
34. Richards NT, Greaves I, Lee SJ, Howie AJ, Adu D, Michael J. Increased Prevalence of Renal Biopsy Findings Other Than Diabetic Glomerulopathy in Type II Diabetes Mellitus. Nephrol Dial Transplant (1992) 7(5):397–9.
35. Castellano I, Covarsi A, Novillo R, Gomez-Martino JR, Ferrando L. [Renal Histological Lesions in Patients With Type II Diabetes Mellitus]. Nefrologia (2002) 22(2):162–9.
36. Akimoto T, Ito C, Saito O, Takahashi H, Takeda S, Ando Y, et al. Microscopic Hematuria and Diabetic Glomerulosclerosis–Clinicopathological Analysis of Type 2 Diabetic Patients Associated With Overt Proteinuria. Nephron Clin Pract (2008) 109(3):c119–26. doi: 10.1159/000145454
37. Mazzucco G, Bertani T, Fortunato M, Bernardi M, Leutner M, Boldorini R, et al. Different Patterns of Renal Damage in Type 2 Diabetes Mellitus: A Multicentric Study on 393 Biopsies. Am J Kidney Dis (2002) 39(4):713–20. doi: 10.1053/ajkd.2002.31988
38. Tone A, Shikata K, Matsuda M, Usui H, Okada S, Ogawa D, et al. Clinical Features of non-Diabetic Renal Diseases in Patients With Type 2 Diabetes. Diabetes Res Clin Pract (2005) 69(3):237–42. doi: 10.1016/j.diabres.2005.02.009
39. Wong TY, Choi PC, Szeto CC, To KF, Tang NL, Chan AW, et al. Renal Outcome in Type 2 Diabetic Patients With or Without Coexisting Nondiabetic Nephropathies. Diabetes Care (2002) 25(5):900–5. doi: 10.2337/diacare.25.5.900
40. Bi H, Chen N, Ling G, Yuan S, Huang G, Liu R. Nondiabetic Renal Disease in Type 2 Diabetic Patients: A Review of Our Experience in 220 Cases. Ren Fail (2011) 33(1):26–30. doi: 10.3109/0886022X.2010.536292
41. Xu X, Nie S, Ding H, Hou FF. Environmental Pollution and Kidney Diseases. Nat Rev Nephrol (2018) 14(5):313–24. doi: 10.1038/nrneph.2018.11
42. Xu X, Wang G, Chen N, Lu T, Nie S, Xu G, et al. Long-Term Exposure to Air Pollution and Increased Risk of Membranous Nephropathy in China. J Am Soc Nephrol (2016) 27(12):3739–46. doi: 10.1681/ASN.2016010093
43. Imtiaz S, Drohlia MF, Nasir K, Salman B, Ahmad A. Analysis of Renal Diseases Detected in Renal Biopsies of Adult Patients: A Single-Center Experience. Saudi J Kidney Dis Transpl (2017) 28(2):368–78. doi: 10.4103/1319-2442.202788
44. Wilfred DC, Mysorekar VV, Venkataramana RS, Eshwarappa M, Subramanyan R. Nondiabetic Renal Disease in Type 2 Diabetes Mellitus Patients: A Clinicopathological Study. J Lab Physicians (2013) 5(2):94–9. doi: 10.4103/0974-2727.119850
45. Bermejo S, Gonzalez E, Lopez-Revuelta K, Ibernon M, Lopez D, Martin-Gomez A, et al. Risk Factors for non-Diabetic Renal Disease in Diabetic Patients. Clin Kidney J (2020) 13(3):380–8. doi: 10.1093/ckj/sfz177
46. Yang Z, Feng L, Huang Y, Xia N. A Differential Diagnosis Model For Diabetic Nephropathy And Non-Diabetic Renal Disease In Patients With Type 2 Diabetes Complicated With Chronic Kidney Disease. Diabetes Metab Syndr Obes (2019) 12:1963–72. doi: 10.2147/DMSO.S223144
47. Jiang S, Fang J, Yu T, Liu L, Zou G, Gao H, et al. Novel Model Predicts Diabetic Nephropathy in Type 2 Diabetes. Am J Nephrol (2020) 51(2):130–8. doi: 10.1159/000505145
48. Garcia-Martin F, Gonzalez Monte E, Hernandez Martinez E, Bada Boch T, Bustamante Jimenez NE, Praga Terente M. When to Perform Renal Biopsy in Patients With Type2 Diabetes Mellitus? Predictive Model of non-Diabetic Renal Disease. Nefrologia (2020) 40(2):180–9. doi: 10.1016/j.nefro.2019.07.005
49. Chong YB, Keng TC, Tan LP, Ng KP, Kong WY, Wong CM, et al. Clinical Predictors of non-Diabetic Renal Disease and Role of Renal Biopsy in Diabetic Patients With Renal Involvement: A Single Centre Review. Ren Fail (2012) 34(3):323–8. doi: 10.3109/0886022X.2011.647302
50. Bermejo S, Soler MJ, Gimeno J, Barrios C, Rodriguez E, Mojal S\ , et al. Predictive Factors for non-Diabetic Nephropathy in Diabetic Patients. The Utility of Renal Biopsy. Nefrologia (2016) 36(5):535–44. doi: 10.1016/j.nefro.2016.06.006
51. Jiang S, Yu T, Zhang Z, Wang Y, Fang J, Yang Y, et al. Diagnostic Performance of Retinopathy in the Detection of Diabetic Nephropathy in Type 2 Diabetes: A Systematic Review and Meta-Analysis of 45 Studies. Ophthalmic Res (2019) 62(2):68–79. doi: 10.1159/000500833
52. Ahmed MH, Elwali ES, Awadalla H, Almobarak AO. The Relationship Between Diabetic Retinopathy and Nephropathy in Sudanese Adult With Diabetes: Population Based Study. Diabetes Metab Syndr (2017) 11 Suppl 1:S333–6. doi: 10.1016/j.dsx.2017.03.011
53. Ren D, Kang W, Xu G. Meta-Analysis of Diagnostic Accuracy of Retinopathy for the Detection of Diabetic Kidney Disease in Adults With Type 2 Diabetes. Can J Diabetes (2019) 43(7):530–7.e4. doi: 10.1016/j.jcjd.2019.04.002
54. Liu S, Guo Q, Han H, Cui P, Liu X, Miao L, et al. Clinicopathological Characteristics of non-Diabetic Renal Disease in Patients With Type 2 Diabetes Mellitus in a Northeastern Chinese Medical Center: A Retrospective Analysis of 273 Cases. Int Urol Nephrol (2016) 48(10):1691–8. doi: 10.1007/s11255-016-1331-y
55. Prakash J, Gupta T, Prakash S, Bhushan P, Usha Sivasankar M, et al. Non-Diabetic Renal Disease in Type 2 Diabetes Mellitus: Study of Renal - Retinal Relationship. Indian J Nephrol (2015) 25(4):222–8. doi: 10.4103/0971-4065.144420
56. Parving HH, Gall MA, Skott P, Jorgensen HE, Lokkegaard H, Jorgensen F, et al. Prevalence and Causes of Albuminuria in non-Insulin-Dependent Diabetic Patients. Kidney Int (1992) 41(4):758–62. doi: 10.1038/ki.1992.118
57. Moger V, Kumar SK, Sakhuja V, Joshi K, Walker R, Kohli HS, et al. Rapidly Progressive Renal Failure in Type 2 Diabetes in the Tropical Environment: A Clinico-Pathological Study. Ren Fail (2005) 27(5):595–600. doi: 10.1080/08860220500200205
58. Jiang S, Wang Y, Zhang Z, Dai P, Yang Y, Li W. Accuracy of Hematuria for Predicting non-Diabetic Renal Disease in Patients With Diabetes and Kidney Disease: A Systematic Review and Meta-Analysis. Diabetes Res Clin Pract (2018) 143:288–300. doi: 10.1016/j.diabres.2018.07.027
59. Dong ZY, Wang YD, Qiu Q, Hou K, Zhang L, Wu J, et al. Dysmorphic Erythrocytes are Superior to Hematuria for Indicating non-Diabetic Renal Disease in Type 2 Diabetics. J Diabetes Investig (2016) 7(1):115–20. doi: 10.1111/jdi.12371
60. Tervaert TW, Mooyaart AL, Amann K, Cohen AH, Cook HT, Drachenberg CB, et al. Pathologic Classification of Diabetic Nephropathy. J Am Soc Nephrol (2010) 21(4):556–63. doi: 10.1681/ASN.2010010010
61. Wang C, Yang Y, Jin L, Zhang Y, Chen G, Zhou Z, et al. Evaluating Renal Biopsy-Associated Hemorrhage Complications by the Equation and Providing an Early Intervention: A Single-Center Experience. J Nephrol (2015) 28(6):691–700. doi: 10.1007/s40620-015-0197-x
62. Gonzalez Suarez ML, Thomas DB, Barisoni L, Fornoni A. Diabetic Nephropathy: Is it Time Yet for Routine Kidney Biopsy? World J Diabetes (2013) 4(6):245–55. doi: 10.4239/wjd.v4.i6.245
63. Espinel E, Agraz I, Ibernon M, Ramos N, Fort J, Seron D. Renal Biopsy in Type 2 Diabetic Patients. J Clin Med (2015) 4(5):998–1009. doi: 10.3390/jcm4050998
64. Bermejo S, Pascual J, Soler MJ. The Current Role of Renal Biopsy in Diabetic Patients. Minerva Med (2018) 109(2):116–25. doi: 10.23736/S0026-4806.17.05446-5
65. Woo KT, Chan CM, Lim C, Choo J, Chin YM, Teng EWL, et al. The Value of Renal Biopsy in Non-Insulin-Dependent Diabetes Mellitus in Singapore Over the Past Two Decades. Kidney Dis (Basel) (2020) 6(4):284–98. doi: 10.1159/000505624
66. Yaqub S, Kashif W, Hussain SA. Non-Diabetic Renal Disease in Patients With Type-2 Diabetes Mellitus. Saudi J Kidney Dis Transpl (2012) 23(5):1000–7. doi: 10.4103/1319-2442.100882
67. Haider DG, Peric S, Friedl A, Fuhrmann V, Wolzt M, Horl WH, et al. Kidney Biopsy in Patients With Diabetes Mellitus. Clin Nephrol (2011) 76(3):180–5. doi: 10.5414/CN106955
68. Biesenbach G, Bodlaj G, Pieringer H, Sedlak M. Clinical Versus Histological Diagnosis of Diabetic Nephropathy–is Renal Biopsy Required in Type 2 Diabetic Patients With Renal Disease? QJM (2011) 104(9):771–4. doi: 10.1093/qjmed/hcr059
69. Leung RK, Wang Y, Ma RC, Luk AO, Lam V, Ng M, et al. Using a Multi-Staged Strategy Based on Machine Learning and Mathematical Modeling to Predict Genotype-Phenotype Risk Patterns in Diabetic Kidney Disease: A Prospective Case-Control Cohort Analysis. BMC Nephrol (2013) 14:162. doi: 10.1186/1471-2369-14-162
70. Song X, Waitman LR, Hu Y, Yu ASL, Robbins DC, Liu M. Robust Clinical Marker Identification for Diabetic Kidney Disease With Ensemble Feature Selection. J Am Med Inform Assoc (2019) 26(3):242–53. doi: 10.1093/jamia/ocy165
71. Rodriguez-Romero V, Bergstrom RF, Decker BS, Lahu G, Vakilynejad M, Bies RR. Prediction of Nephropathy in Type 2 Diabetes: An Analysis of the ACCORD Trial Applying Machine Learning Techniques. Clin Transl Sci (2019) 12(5):519–28. doi: 10.1111/cts.12647
Keywords: non-diabetic renal disease, diabetic nephropathies, diagnosis model, machine learning, renal biopsy
Citation: Zhang W, Liu X, Dong Z, Wang Q, Pei Z, Chen Y, Zheng Y, Wang Y, Chen P, Feng Z, Sun X, Cai G and Chen X (2022) New Diagnostic Model for the Differentiation of Diabetic Nephropathy From Non-Diabetic Nephropathy in Chinese Patients. Front. Endocrinol. 13:913021. doi: 10.3389/fendo.2022.913021
Received: 05 April 2022; Accepted: 20 May 2022;
Published: 30 June 2022.
Edited by:
Akira Sugawara, Tohoku University, JapanReviewed by:
Sheila Bermejo, Vall d’Hebron University Hospital, SpainLijun Zhao, Sichuan University, China
Copyright © 2022 Zhang, Liu, Dong, Wang, Pei, Chen, Zheng, Wang, Chen, Feng, Sun, Cai and Chen. This is an open-access article distributed under the terms of the Creative Commons Attribution License (CC BY). The use, distribution or reproduction in other forums is permitted, provided the original author(s) and the copyright owner(s) are credited and that the original publication in this journal is cited, in accordance with accepted academic practice. No use, distribution or reproduction is permitted which does not comply with these terms.
*Correspondence: XiangMei Chen, xmchen301@126.com; Guangyan Cai, caiguangyan@sina.com
†These authors have contributed equally to this work and share first authorship