- 1Department of Breast and Thyroid Surgery, Affiliated Jinhua Hospital, Zhejiang University School of Medicine, Jinhua, Zhejiang, China
- 2Central Laboratory, Affiliated Jinhua Hospital, Zhejiang University School of Medicine, Jinhua, Zhejiang, China
Aims: The primary objective of this study was to investigate the relationship between the platelet/high-density lipoprotein cholesterol ratio (PHR) and the prevalence of nephrolithiasis within the adult population of the United States.
Methods: The data used in this study were obtained from the National Health and Nutrition Examination Survey (NHANES) conducted between 2007 and 2018. The analysis included a non-pregnant population aged 20 years or older, providing proper PHR index and nephrolithiasis data. The research utilized subgroup analyses and weighted univariate and multivariable logistic regression to evaluate the independent association between the PHR and the susceptibility to nephrolithiasis.
Results: The study comprised 30,899 participants with an average PHR value of 19.30 ± 0.11. The overall prevalence rate of nephrolithiasis was estimated at 9.98% with an increase in the higher PHR tertiles (T1, 8.49%; T2, 10.11%; T3, 11.38%, P < 0.0001). An elevated PHR level was closely linked with a higher susceptibility to nephrolithiasis. Compared with patients in T1, and after adjusting for potential confounders in model 2, the corresponding odds ratio for nephrolithiasis in T3 was 1.48 (95% CI: 1.06 to 2.08), with a P-value = 0.02. The results of the interaction tests revealed a significant impact of chronic kidney disease on the relationship between PHR and nephrolithiasis. Furthermore, the restricted cubic spline analyses exhibited a positive, non-linear correlation between PHR and the risk of nephrolithiasis.
Conclusion: A convenient biomarker, the PHR, was independently associated with nephrolithiasis and could be a novel biomarker in predicting occurrence in clinical decision.
Introduction
Nephrolithiasis has become more common throughout the world in the last few years. The prevalence of this condition has notably risen within the United States (US). Specifically, the incidence rate has surged from 3.2% in the 1980s to 10.1% in 2016 (1, 2). The occurrence of symptomatic nephrolithiasis is particularly high among adult males (3). Kidney stone disease (KSD), a major health concern, is expected to affect more than 15% of males and over 5% of females by the age of 70 (4). Furthermore, recurrences are common in patients with symptomatic nephrolithiasis after the initial stone episode, with a recurrence rate of up to 50% over a lifetime in both genders (5, 6). Nephrolithiasis has garnered significant attention in healthcare due to its substantial medical costs and societal impact, with annual healthcare expenditures exceeding $2 billion in the US alone (7). Therefore, there is a pressing need to focus on the prevention of nephrolithiasis.
Despite the increasing prevalence of nephrolithiasis, various risk factors have been identified, including obesity, diabetes mellitus (DM), excessive consumption of salt, animal protein, and sugary foods (8–11). Recent research suggests that multiple inflammatory processes are involved in kidney stone formation. For example, the retention of crystals is mediated by the excessive production of reactive oxygen species, leading to subsequent oxidative stress and inflammation (12–14). During this inflammatory response, the levels of neutrophils, platelets (PLT), lymphocytes and acute phase proteins change. Among these, PLTs have been identified as crucial modulators of the inflammatory response and can also accelerate inflammatory state. Activated PLTs trigger the intrinsic coagulation cascade, contributing to multiple diseases (15). The platelet lymphocyte ratio (PLR) has been explored as an accurate marker for systematic inflammatory response syndrome (SIRS) in patients who underwent percutaneous nephrolithotomy (PCNL) (16). Moreover, high-density lipoprotein cholesterol (HDL-C) has demonstrated anti-platelet and anti-thrombotic properties (17, 18) and has also been identified as an anti-inflammatory group of proteins (19). Low levels of HDL-C have been associated with an increased risk of nephrolithiasis. Jialal et al. initially demonstrated a significant correlation between the platelet -to-HDL-C ratio (PHR) and the severity of Metabolic Syndrome (MetS) (20). These findings have led to speculation about using a combination of PLT and HDL-C levels to detect groups at high risk for nephrolithiasis earlier so that interventions can be implemented in advance to reduce the development of nephrolithiasis. However, there is limited knowledge regarding its predictive capacity for nephrolithiasis.
The current study utilized data on nephrolithiasis from the National Health and Nutrition Examination Survey (NHANES) to examine the association between PHR and the risk of kidney stone development in US adults.
Materials and methods
Study participants and data collection
The data for this retrospective analysis were sourced from the NHANES, accessible via the official website of the Centers for Disease Control and Prevention (CDC, https://www.cdc.gov/nchs/nhanes/index.htm). The NHANES employs a systematic, multistage, probability-cluster method to collect data, which enables the assessment of health and nutritional trends in both adults and children in the US. The survey’s sampling strategy included an oversampling of participants from various racial and ethnic backgrounds such as non-Hispanic black, non-Hispanic white, Mexican American, etc. For the purposes of this study, NHANES data spanning from 2007 to 2018 were utilized. An initial review of the data for the specified study period provided records for 34,770 participants aged 20 years or older. However, the analysis excluded participants without nephrolithiasis data (n = 91), pregnant women (n = 374), those with missing platelet counts (PCs) (n = 2967), and those with missing HDL-C levels (n = 439). After applying the detailed inclusion and exclusion criteria, the study encompassed a total of approximately 30,899 participants (Figure 1). The NHANES protocol was approved by the National Center for Health Statistics Ethics Review Board, and the study was conducted in accordance with informed consent under ethical guidelines (21). The data utilized from the publicly available database was also openly accessible from other sources (22).
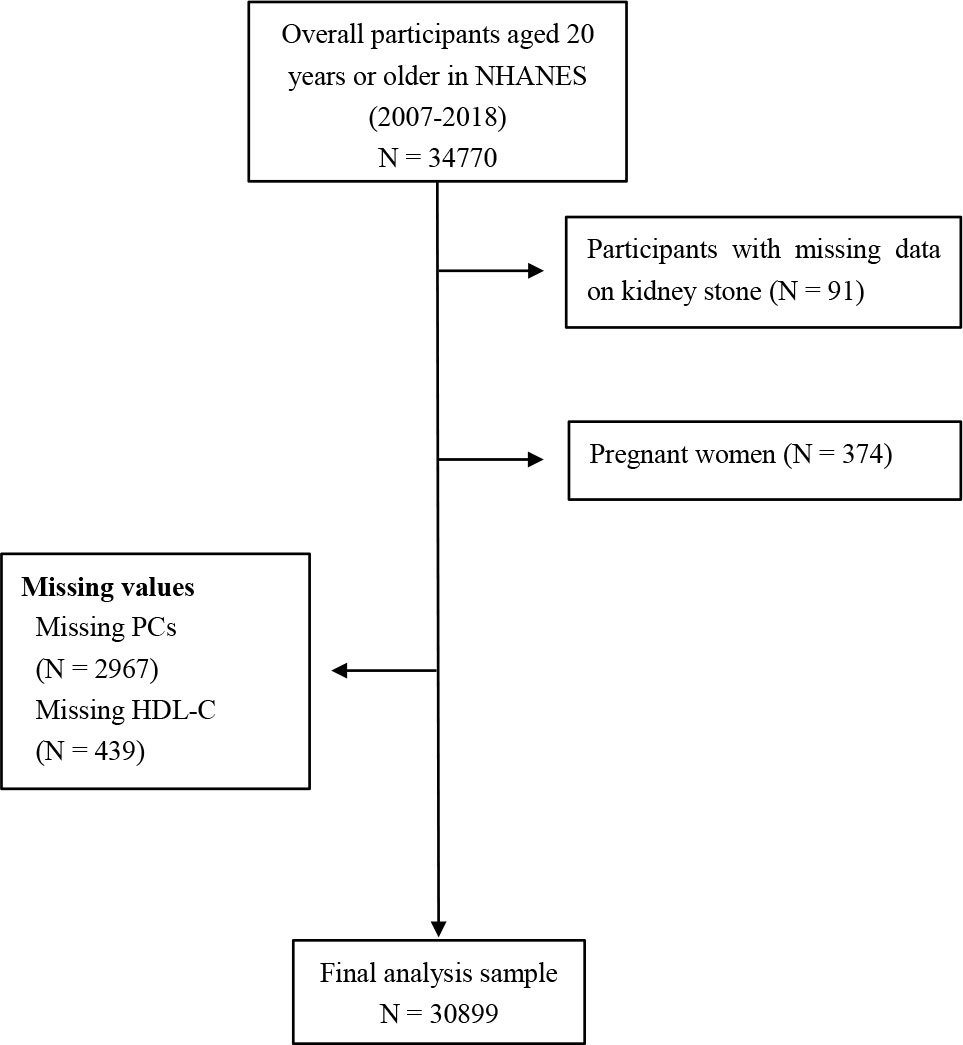
Figure 1 Representation of study design. NHANES, National Health and Nutrition Examination Survey; PCs, platelet counts; HDL-C, high-density lipoprotein cholesterol.
Exposure variables and outcomes
In this study, the PHR was specifically constructed to function as the exposure variable. Due to the extensive variability in PHR values, a transformation of the PHR was applied. The PHR was calculated by dividing the PCs by the HDL-C levels and then dividing the quotient by 10 (20).
The primary outcome was determined based on the presence or absence of a self-reported history of kidney stones, covering both symptomatic and asymptomatic cases. This was ascertained by the participant’s response to the question, “Have you/Has sample person (SP) ever had a kidney stone?” Those who answered ‘yes’ were categorized as having nephrolithiasis. The validity of self-reported data on the occurrence of kidney stones has been confirmed by previous studies (4, 23, 24).
Biochemical parameters
The study involved assessing various biochemical parameters in participants who voluntarily underwent blood sampling at a mobile examination center (MEC). The range of parameters measured included uric acid, fasting glucose, LDL-C, HDL-C, total cholesterol (TC), PCs, creatinine and blood urea nitrogen. The methodologies for these measurements were based on standardized procedures referenced from the current scientific literature (25, 26). For renal function assessment, the estimated glomerular filtration rate (eGFR) was calculated using the Chronic Kidney Disease Epidemiology Collaboration (CKD-EPI) equation based on creatinine levels, given by: eGFR = 141 × min(Scr/κ, 1)α × max(Scr/κ, 1)-1.209 × 0.993Age × 1.018 [if female] _ 1.159 [if black], where Scr is serum creatinine, κ is 0.7 for females and 0.9 for males, α is -0.329 for females and -0.411 for males, min indicates the minimum of Scr/κ or 1, and max indicates the maximum of Scr/κ or 1 (27).
Other covariates of interest
In this study, an array of potential confounding variables identified from epidemiological research related to nephrolithiasis was examined. These included sociodemographic factors such as marital status, education level, race, age, and gender. Physical measures addressed were body mass index (BMI), while lifestyle factors taken into account encompassed alcohol consumption, smoking status and total plain water intake. Furthermore, the presence of comorbid conditions such as DM, hypertension, hyperlipidemia, CKD and coronary heart disease (CHD) was also investigated. In addition, indices such as the composite dietary antioxidant index (CDAI), dietary inflammation index (DII), and systemic immune-inflammatory index (SII) were calculated to assess their potential roles as moderating factors.
Sociodemographic data were gathered through self-reported questionnaires. Age was categorized as < 45, 45–64, or ≥ 65 years. Individuals’ sex was documented as either male or female. Race/ethnicity was classified as non-Hispanic black, non-Hispanic white, Mexican-American, or other, which included those with multiracial backgrounds. Marital status was recorded as never married, married, or living separately. Participants who were divorced, widowed, or living separately were classified as residing in separate households. Education level was classified into three categories: less than high school, high school graduate, or education beyond high school. According to the established criteria by the World Health Organization (WHO), individuals with a BMI equal to or exceeding 30 kg/m^2 are considered obese. The family poverty-to-income ratio (PIR), when less than 1.00, indicates that the household income is below the poverty threshold, while a PIR exceeding 3.00 signifies that the income is more than three times the poverty threshold.
The lifestyle factors were assessed using self-reported questionnaires. Alcohol consumption was quantified based on established criteria and participants were divided into four categories: lifetime abstainers, defined as those who have consumed fewer than 12 drinks in their lifetime; former drinkers, those who have had at least 12 drinks in their lifetime but have not consumed alcohol in the past year; current light drinkers, consuming three or fewer drinks per week; and current heavy drinkers, consuming more than three drinks per week. According to the National Center for Health Statistics and the CDC, participants’ smoking history was classified into three groups. The first group comprised individuals who had never smoked or had smoked fewer than 100 cigarettes in their lifetime. The second category included those who had smoked 100 or more cigarettes but had quit by the time of the interview. The third group included people who were presently smoking. Total plain water intake, measured in milliliters per day (ml/day), was defined as the total volume of water consumed from all sources—including plain tap water, water from drinking fountains or water coolers, bottled water, and spring water—over a 24 hours period.
This study also investigated the comorbidities, including hypertension, hyperlipidemia, DM, CKD and CHD. The inclusion criteria for hypertension included a medical diagnosis of hypertension, the use of anti-hypertensive medication, or a measured systolic blood pressure equal to or exceeding 140 mmHg and/or diastolic blood pressure equal to or exceeding 90 mmHg. Hyperlipidemia was defined by an HDL-C level less than 40 mg/dL, LDL-C level of 160 mg/dL or higher, triglycerides of 200 mg/dL or higher, and TC of 240 mg/dL or higher, or by a previous diagnosis obtained during the NHANES blood test. The participants were identified with type 2 DM based on specific criteria, including a medical diagnosis of diabetes, oral glucose tolerance test results of 11.1 mmol/L or higher, random glucose levels of 11.1 mmol/L or higher, fasting glucose levels of 7.0 mmol/L or higher, Hemoglobin A1c (HbA1c) of 6.5% or more, or the use of antidiabetic medication. The CKD-EPI equation was used to assess the eGFR for CKD diagnosis, with CKD defined as an eGFR of less than 60 ml/min/1.73 m^2, or a urine albumin-to-creatinine ratio (UACR) exceeding 30 mg/g (28). The presence of CHD was determined through self-reported data, with participants indicating ‘Yes’ or ‘No’.
Dietary data in NHANES were obtained through a 24-hour dietary recall interview and have been validated by the Nutrition Methodology Working Group (29). The CDAI measures an individual’s antioxidant profile by considering dietary antioxidants such as vitamins A, C, E, as well as selenium, zinc, and carotenoids; we calculated the CDAI as proposed by Wright et al. (30) The DII was designed to evaluate the inflammatory potential of diets based on the proinflammatory and anti-inflammatory properties of the dietary components (31). The detailed method for calculating the CDAI and DII have been described in previous studies (30, 31). The concept of the SII was first introduced by Hu et al. (32) to evaluate the prognostic value for multiple diseases. The SII is composed of counts of peripheral neutrophil (N), lymphocyte (L), and PLT, and is defined as PLT multiplied N/L (expressed in 10^9/L) (33).
Statistical analysis
Data preparation and statistical analysis were carried out utilizing R software version 4.2 (http://www.R-project.org; The R Foundation). In accordance with NHANES recommendations, the study applied sampling weights to mitigate the purposeful oversampling of certain demographic categories. All tests were weighted for sample size and took into account the complex stratified, multistage, cluster sampling strategy used in NHANES (34). Continuous variables were represented as mean ± standard error (SE) with survey weights. Categorical variables were displayed as counts and percentages, adjusted for survey weights. The current study involved converting the variable corresponding to PHR from a continuous to a categorical scale. Subsequently, several models were developed to assess the individual impacts of PHR and nephrolithiasis on the outcome of interest. PHR was analyzed both as continuous and as categorical variables based on tertiles. Differences between groups, whether separated by PHR tertiles or by the presence versus absence of nephrolithiasis, were assessed using a weighted chi-square test for categorical variables and a weighted Student’s t-test for continuous variables.
The current study employed weighted univariate and multivariate logistic regression analyses to evaluate the relationship between PHR and nephrolithiasis across various models. Covariates with a P < 0.05 in the univariate analysis were included in the multivariate model as confounding factors. Crude Model did not incorporate flexible adjustments. Model 1 was adjusted for age, sex, and race. Model 2 included adjustments for age, sex, race, BMI, marital status, family PIR, smoking status, alcohol usage, hypertension, hyperlipidemia, CKD, CHD, DM, fasting glucose, uric acid, eGFR, creatinine, blood urea nitrogen, CDAI, DII and SII. The P-value for the trend was determined using a logistic regression model. Interaction analyses were also conducted to examine the heterogeneity of correlations among different subgroups. A restricted cubic spline (RCS) model with three knots was utilized to explore potential linear and non-linear relationships. Knot number 3 was selected by minimizing the Akaike information criterion (AIC) statistic. The log-likelihood ratio test was applied to assess the presence of linear or non-linear correlations. A P-value < 0.05 was considered statistically significant for all analyses.
Results
Personal characteristics of participants
The study included 30,899 participants, as indicated in Table 1. The mean age of the participants was 47.78 ± 0.23 years, and the population comprising 48.73% males and 51.27% females. The prevalence of nephrolithiasis accounted for 9.98% of the population. The average value of the PHR was 19.30 ± 0.11, and the observed PHR ranges for the three tertiles were as follows: tertile 1 ranged from 0.61 to 15.32, tertile 2 from 15.32 to 21.36, and tertile 3 from 21.36 to 183.52. The study observed that the individuals with higher PHR tertile tended to have a higher risk of nephrolithiasis (Tertile 1: 8.49%, Tertile 2: 10.11%, Tertile 3: 11.38%) (P < 0.0001). Significant variables across all tertiles of PHR included age, sex, ethnicity, marital status, educational level, household income, smoking status, alcohol use, BMI, PCs, fasting glucose, LDL-C, HDL-C, TC, uric acid, eGFR, blood urea nitrogen, CDAI, DII, SII, DM, hypertension, hyperlipidemia, CKD and CHD. Notably, participants in the higher PHR tertiles, compared to those in the lowest tertile, had increased levels of DII and SII and decreased levels of CDAI (P < 0.05). The clinical and biochemical characteristics of the participants with and without nephrolithiasis are shown in Supplementary Table 1. Cases of nephrolithiasis had higher levels of fasting glucose, uric acid, creatinine, blood urea nitrogen, DII, SII, and lower levels of eGFR, TC, and CDAI, and were more likely to be male, older, non-Hispanic White, married, more educated, of higher income, obese, non-drinkers, with DM, hypertension, hyperlipidemia, CKD and CHD (all P < 0.05).
Potential link between PHR and nephrolithiasis risk
As shown in Table 2, the results of the univariate analysis indicated that PHR, age, non-Hispanic White, having a BMI ≥ 30 kg/m^2, a family PIR of 1-3, history of smoking, former alcohol use, and a history of DM, hypertension, hyperlipidemia, CKD, CHD, high levels of fasting glucose, uric acid, creatinine, blood urea nitrogen, DII and SII were all positively associated with nephrolithiasis (P < 0.05). Conversely, being female, non-Hispanic Black, never married, heavy alcohol use, higher eGFR, and higher CDAI, were negatively associated with nephrolithiasis (P < 0.01).
The results of the multivariate logistic regression analysis demonstrated a significant relationship between the exposure and outcome variables, which persisted after adjusting for confounding factors (P-value < 0.05). The findings from the multivariate regression analyses are presented in Table 3. When treated as a continuous variable, PHR levels were positively associated with nephrolithiasis in adjusted model 2 (odds ratio [OR] = 1.02, 95% confidence interval [CI]: 1.00 – 1.04, P = 0.01). As categorical variables (divided into tertiles), the highest tertile of PHR was also positively associated with nephrolithiasis compared to the lowest tertile in adjusted model 2 (OR = 1.48, 95% CI: 1.06 – 2.08, P = 0.02). The P-value for the trend across tertiles was 0.02 in adjusted model 2.
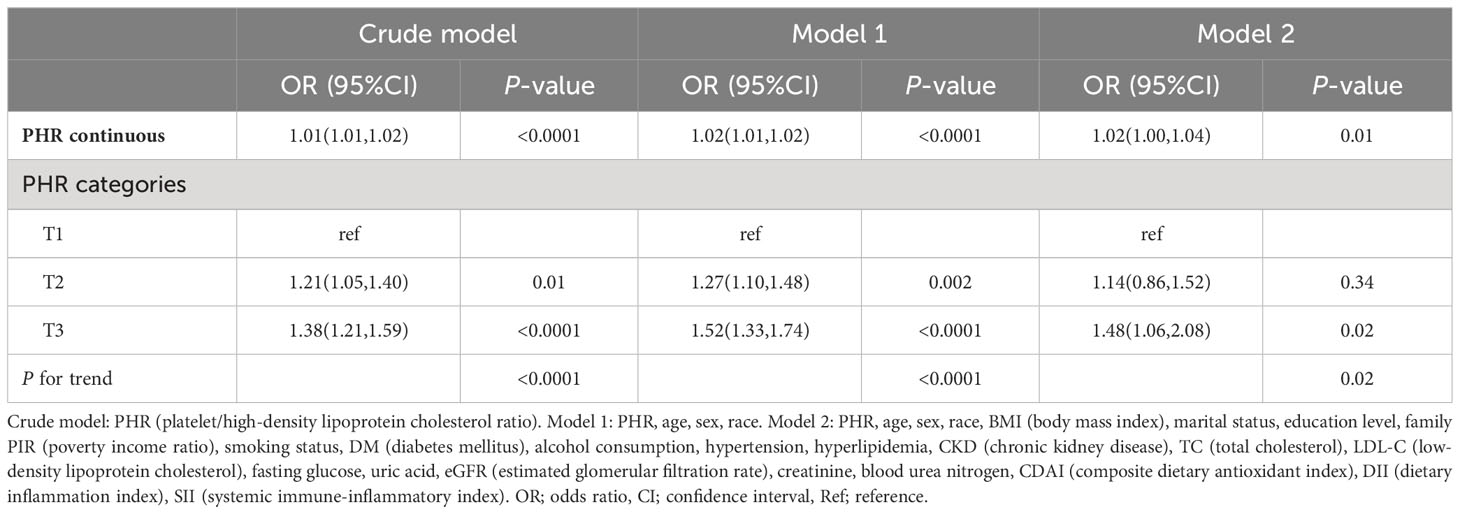
Table 3 Relationship between the risk of nephrolithiasis and PHR via weighted multivariable logistic regression.
Additionally, the RCS analysis revealed a positive non-linear relationship between the risk of nephrolithiasis and PHR levels (P for nonlinearity = 0.0061), as illustrated in Figure 2.
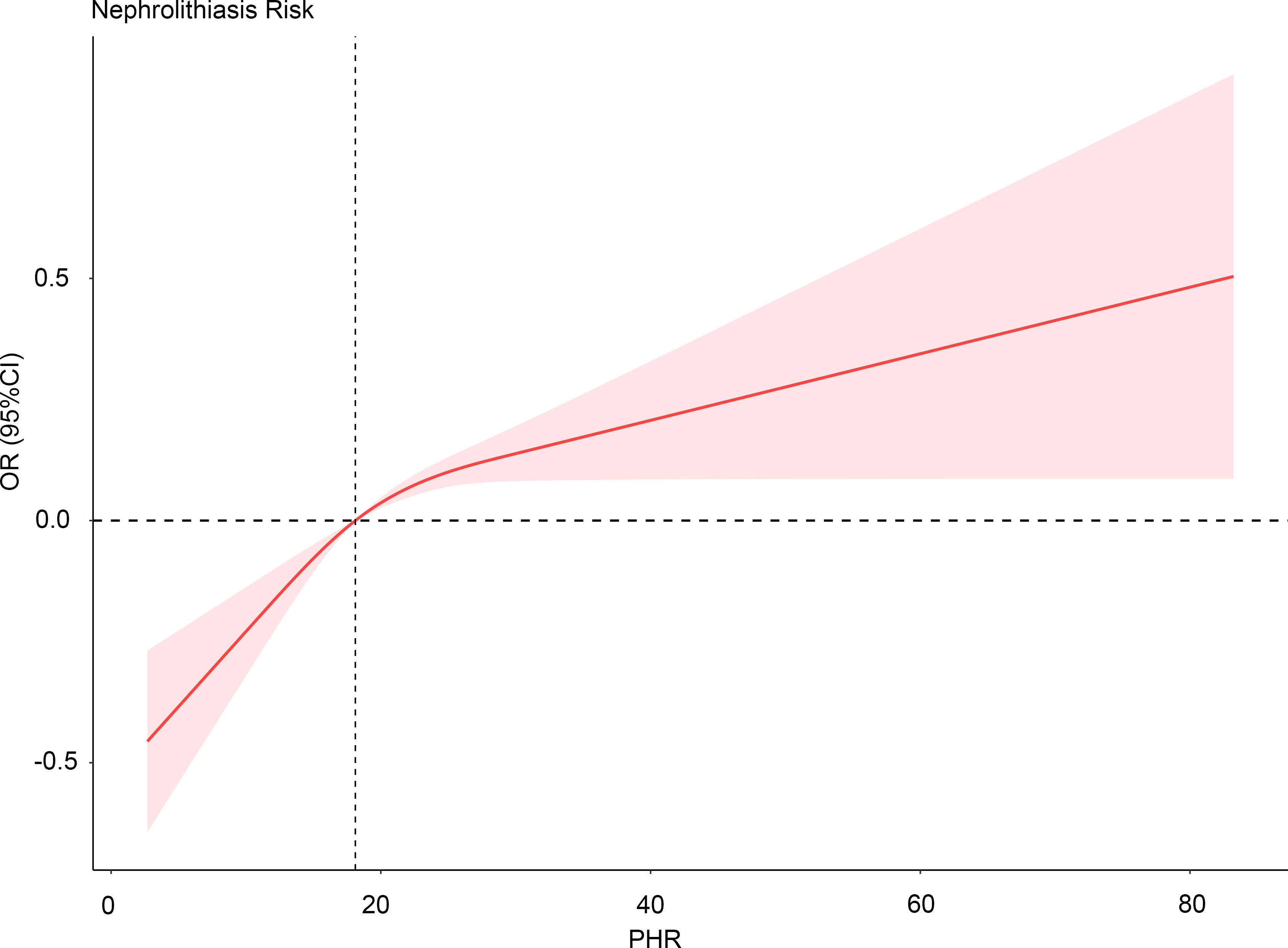
Figure 2 Restricted cubic spline analysis of the association between PHR values and the prevalence of nephrolithiasis. No variables have been adjusted. PHR, platelet/high-density lipoprotein cholesterol ratio; OR, odds ratio; CI, confidence interval; Ref, reference.
Stratified analyses
As shown in Table 4, a subgroup analysis stratified by various variables was conducted. Across all subgroups, participants in tertile 3 consistently had higher risks of nephrolithiasis compared to those in tertile 1 (all OR > 1). However, the association was not statistically significant in some subgroups. Notably, in the BMI-stratified analysis, the relationship between increased PHR levels and elevated risk of nephrolithiasis was significant for individuals with BMI ≥ 30 kg/m^2, with a 20.1% increase in risk for the T3 group compared to the T1 group, but this increase was not significant for individuals with BMI < 30 kg/m^2. In the analysis stratified by alcohol usage, there was a significant 55.5-89.7% increase in the risk of nephrolithiasis among moderate to heavy alcohol users; however, this increase was not significant in the never or former alcohol usage subgroup. In the CHD-stratified analysis, there was a significant 39.2% increase in the risk of nephrolithiasis in the group without CHD, but this increase was not significant in the CHD subgroup.
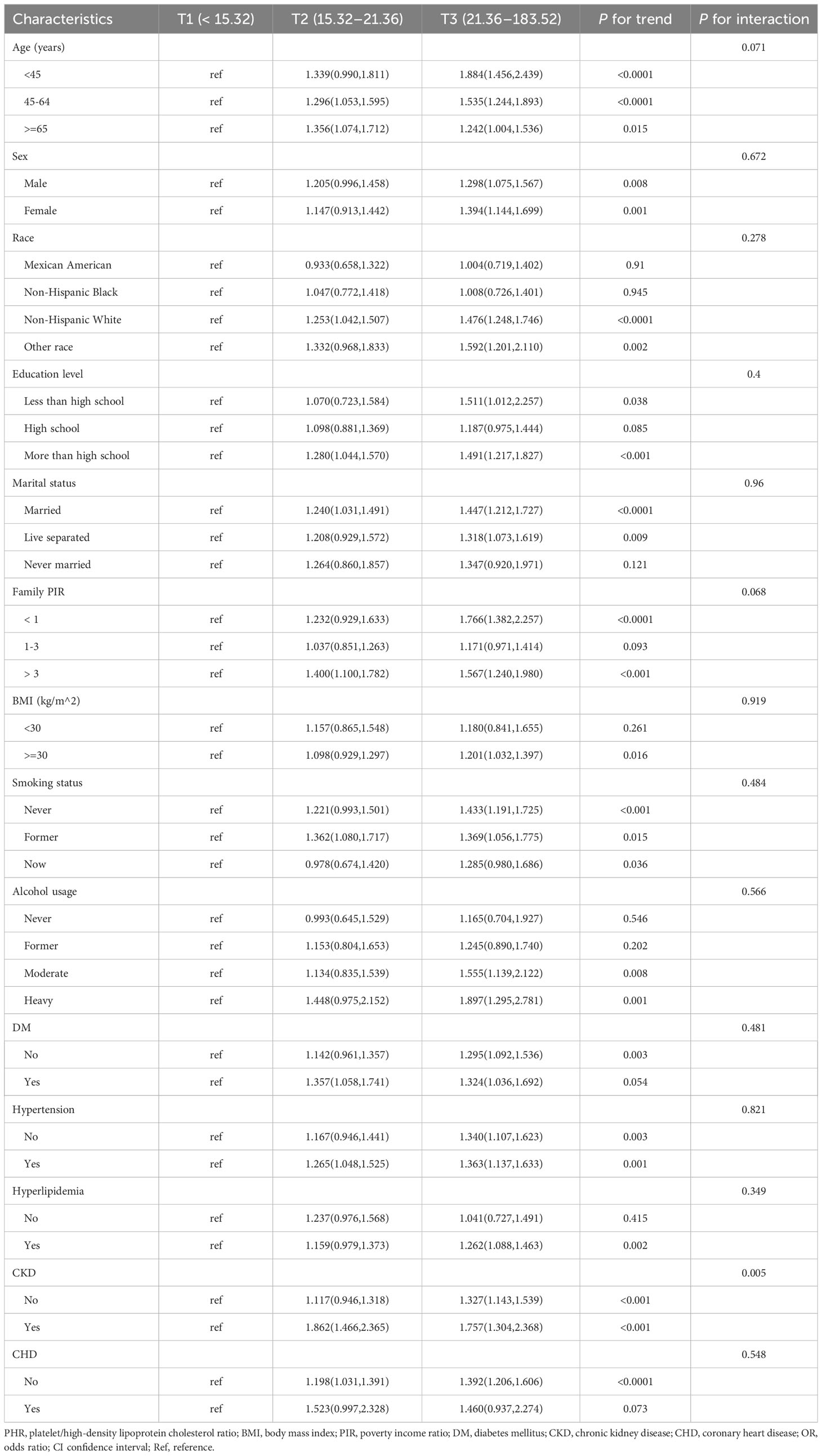
Table 4 Subgroup analyses of the relationship between PHR values and the prevalence of nephrolithiasis.
The interaction test indicated that, with the exception of CKD (P interaction < 0.05), there were no significant differences among the other stratifications in the association between PHR and nephrolithiasis prevalence. These results suggest that the positive association between PHR and the risk of nephrolithiasis was consistent across populations differing in alcohol usage, Family PIR, marital status, age, gender, race, education level, BMI, smoking status, DM, hypertension, hyperlipidemia and CHD (all P for the interaction > 0.05), indicating that the findings could be applicable in various population settings.
Discussion
In this observational study, we analyzed standardized data from a large cohort of participants in a US population sample. Our study found that the PHR was higher in patients with nephrolithiasis. Considering that an imbalance in the baseline characteristics of participants could modify the association between PHR and nephrolithiasis, adjustments were made for potential confounders in the regression analysis; nevertheless, we still observed a significant association of PHR with nephrolithiasis. This suggests that the association cannot be solely attributed to known risk factors and that PHR could independently predict the presence of nephrolithiasis. According to the subgroup analysis and interaction test, this connection was consistent across diverse demographic settings. In the RCS analysis, PHR demonstrated a pronounced non-linear association with the risk of nephrolithiasis, providing substantial evidence for further clinical and basic research.
This investigation reported the novel findings by representing the first examination of the correlation between PHR and nephrolithiasis. Researchers have recently suggested that kidney stone formation entails activating various inflammatory responses. We found that participants with kidney stones had lower levels of CDAI and higher levels of DII and SII, indicating that antioxidants, oxidants, and a pro-inflammatory diet may play a critical role in kidney stone formation. Dietary intake is an important source of antioxidants and oxidants. According to a study by Maddahi et al. (35), consuming a diet with an elevated DII is associated with a higher susceptibility to kidney stone formation in men. In another study, Zhang et al. (36) observed a positive association between an increased intake of a pro-inflammatory diet and a greater risk of kidney stone occurrence and recurrence. The DII provides a method for assessing the impact of diet on inflammation. However, anti-inflammatory diets, including the Dietary Approaches to Stop Hypertension (DASH) and the Mediterranean diet, may decrease systemic inflammation (37, 38). The regulation of inflammation requires various anti-inflammatory metabolites derived from dietary components, particularly short-chain fatty acids, tryptophan metabolites, and tyrosine metabolites, which have been identified as critical in the regulation of inflammation. Conversely, diets with a higher DII may potentially increase the overall level of systemic inflammation, which is involved in the development of nephrolithiasis. Cohen et al. (39) observed a potential beneficial effect of statin consumption on the development of urolithiasis. However, the precise mechanism remains elusive, and preliminary evidence suggests that statins possess anti-inflammatory and antioxidant properties that could potentially inhibit the incidence of nephrolithiasis. Elevated inflammation creates a conducive environment for the development and accumulation of renal tubular crystals, as verified in a murine model (40). Using statin can effectively reduce inflammatory mediators, thus inhibiting the retention of renal crystals (41). However, there are few studies on the role of immune responses and inflammatory cells in the formation of kidney stones. Idiopathic calcium oxalate stones often attach to Randall’s plaque, which are associated with the activation of M1 macrophages (42). While M2 macrophage-related genes are associated with the inhibition of stone formation (43). The renal crystal deposition is also related to the production of reactive oxygen species (ROS) production and inflammasome activation (44). Exosomes released by macrophage promote IL-8 production, facilitate neutrophil migration, and enhance the crystal inflammatory response (45). PLT interact with monocytes, neutrophils, and lymphocytes and regulate innate and adaptive responses. Thus, the imbalance of PHR may originate from the body’s immune and inflammatory response and may indirectly reflect the potential for kidney stone formation. However, the specific mechanistic relationship between PHR imbalance and nephrolithiasis formation needs further studied.
Furthermore, the risk of nephrolithiasis may be heightened by low levels of HDL-C. Torricelli et al. (46) reported that a significant correlation between reduced HDL-C levels and lower urinary pH. Moreover, a decreased urinary pH is implicated in the development of nephrolithiasis, particularly those characterized by uric acid stones (47–49). A urinary pH below 5.5 significantly increases the concentration of soluble non-dissociated uric acid, leading to the precipitation and formation of uric acid stones (47, 50). The primary concern stems from the potential link between low HDL levels and an increased susceptibility to nephrolithiasis, mediated by the influence of reduced HDL on urinary pH and thus facilitating the formation of uric acid-containing kidney stones. The PCs serves as a valuable marker for assessing systemic inflammation. Conversely, HDL-C plays a crucial role in attenuating inflammatory responses. Therefore, PHR could be considered an alternative marker for measuring inflammation, particularly in relation to nephrolithiasis.
Previous epidemiological investigations have supported the link between various risk factors and the likelihood of developing. These risk factors include obesity, smoking, alcohol consumption, DM, hypertension, hyperlipidemia, CKD and CHD. The Taylor trial revealed a correlation between obesity and the development of kidney stones. Men with a BMI of 30 or higher exhibited a 1.33-fold increased risk of nephrolithiasis compared to men with a BMI of 21 to 22.9. Similarly, both elderly and young women in the same BMI category displayed a 1.90-fold increased risk (51). Past studies have examined the impact of body size on urine chemistry, revealing that an increase in BMI may potentially elevate lithogenic risk factors. These factors involve reducing urinary volume and citrate concentrations (52). Another study indicated that middle-aged, hypertensive white males might serve as an indicator for nephrolithiasis, which is characterized by the development of kidney stones and has been linked to increased and prolonged urinary calcium excretion in hypertensive patients (53). Kidney stone disease has also been associated with DM, specifically type 2 DM. This association suggests a beneficial connection between the severity of DM and the occurrence of kidney stones, potentially explainable by the effects of insulin resistance (IR) on urinary pH and the transport of ammonium and calcium into the kidneys (54). In their study, Sur et al. (55) conducted a retrospective analysis of medical histories to investigate the presence of stones in patients with hyperlipidemia. Their results revealed a significant association between statin use, which has cholesterol-lowering effects, and a lower risk of stone formation, suggesting a possible link between hyperlipidemia and nephrolithiasis. Khan et al. (56) explored the influence of various inflammatory markers on stone formation, hypothesizing that renal inflammation could damage epithelial tissue and create favorable conditions for crystallization. Ferraro et al. (57) reported that among women, a history of kidney stones was associated with a modest but statistically significantly increased risk of CHD; however, this association was no significant in men. The findings from the subgroup analysis indicated a beneficial relationship that was consistent across various subgroups when stratified by BMI, smoking status, alcohol consumption, DM, hypertension, hyperlipidemia, CKD and CHD. These results are in line with previous studies conducted on the same issue. Furthermore, except for CKD, where the interaction was significant (P for interaction = 0.005), no other clinical variables showed any significant dependency on this association (all P for interaction > 0.05). These findings suggest a beneficial relationship that may be applicable across different population settings.
The current inquiry boasts several notable strengths. First, the study utilized data from NHANES, and analyses were conducted with the appropriate NHANES sample weights taken into consideration. Second, confounding covariates were carefully adjusted to enhance the reliability of the results and to facilitate their generalizability to broader populations. Third, due to its cost-effectiveness, simplicity, and the breadth of informative parameters it provides, the inclusion of routine blood examination and blood biochemistry offers considerable potential for the diagnosis and management of nephrolithiasis. Consequently, this approach merits further exploration and detailed analysis.
Nevertheless, it is crucial to acknowledge the inherent limitations of this research. First, the identification of kidney stones was based on personal interviews, introducing the possibility of recall bias, recording errors, interviewer bias. Consequently, some asymptomatic kidney stones that would require physical examination for detection may have been overlooked in the database. Second, the database did not include detailed clinical variables such as personal medication histories or the types of kidney stones, both of which warrant further investigation. Moreover, blood samples were collected from one blood test only. Sequential testing could potentially be more indicative of true physiological states, given the lifespan of blood cells. Third, due to the limitations inherent to the NHANES database, several confounding factors that could influence the results were not considered in the analysis. Fourth, given the randomly missing data among the covariables and the large sample size, the study refrained from employing multiple imputation methods to handle the missing data, which may affect the precision of the findings. However, this approach may potentially impact the accuracy of the findings. Lastly, this study employed a cross-sectional study design, which limits the capacity to determine a causal connection between PHR and nephrolithiasis.
Conclusion
In summary, our study has demonstrated an independent and non-linear association between PHR and nephrolithiasis, suggesting that PHR could serve as a novel biomarker for predicting the occurrence of this condition and thereby informing individualized therapy and clinical decision-making. However, the establishment of PHR’s reliability as a predictive marker necessitates further validation through comprehensive prospective studies.
Data availability statement
The original contributions presented in the study are included in the article/Supplementary Material. Further inquiries can be directed to the corresponding author. The publicly available datasets presented in this study can be found in online repositories. These data can be found here: https://www.cdc.gov/nchs/nhanes/.
Ethics statement
The studies involving human participants were reviewed and approved by National Center for Health Statistics Research Ethics Review Board. The patients/participants provided their written informed consent to participate in this study. The studies were conducted in accordance with the local legislation and institutional requirements.
Author contributions
JN: Conceptualization, Writing – review & editing, Data curation, Formal analysis, Investigation, Methodology, Software, Visualization, Writing – original draft. LL: Data curation, Formal analysis, Writing – original draft. PW: Writing – original draft, Methodology. CX: Conceptualization, Supervision, Writing – review & editing. All authors contributed to the article and approved the submitted version.
Funding
The author(s) declare financial support was received for the research, authorship, and/or publication of this article. The work was supported by Jinhua Science and Technology Research Program (2022-3-073), Basic Research Foundation Project of Jinhua Central Hospital (JY2022-6-02).
Acknowledgments
Thanks to Zhang Jing (Second Department of Infectious Disease, Shanghai Fifth People’s Hospital, Fudan University) for statistical analysis support and for his work on the NHANES database. His outstanding work, nhanesR package and webpage, makes it easier for us to explore NHANES database.
Conflict of interest
The authors declare that the research was conducted in the absence of any commercial or financial relationships that could be construed as a potential conflict of interest.
Publisher’s note
All claims expressed in this article are solely those of the authors and do not necessarily represent those of their affiliated organizations, or those of the publisher, the editors and the reviewers. Any product that may be evaluated in this article, or claim that may be made by its manufacturer, is not guaranteed or endorsed by the publisher.
Supplementary material
The Supplementary Material for this article can be found online at: https://www.frontiersin.org/articles/10.3389/fendo.2024.1289553/full#supplementary-material
References
1. Sorokin I, Mamoulakis C, Miyazawa K, Rodgers A, Talati J, Lotan Y. Epidemiology of stone disease across the world. World J urol (2017) 35:1301–20. doi: 10.1007/s00345-017-2008-6.
2. Chewcharat A, Curhan G. Trends in the prevalence of kidney stones in the United States from 2007 to 2016. Urolithiasis (2021) 49:27–39. doi: 10.1007/s00240-020-01210-w.
3. Kittanamongkolchai W, Vaughan L, Enders F, Dhondup T, Mehta R, Krambeck A, et al. The changing incidence and presentation of urinary stones over 3 decades. Mayo Clinic Proc (2018) 93:291–9. doi: 10.1016/j.mayocp.2017.11.018.
4. Di XP, Gao XS, Xiang LY, Wei X. The association of dietary intake of riboflavin and thiamine with kidney stone: a cross-sectional survey of NHANES 2007-2018. BMC Public Health (2023) 23:964. doi: 10.1186/s12889-023-15817-2.
5. D’Costa MR, Haley WE, Mara KC, Enders FT, Vrtiska TJ, Pais VM, et al. Symptomatic and radiographic manifestations of kidney stone recurrence and their prediction by risk factors: A prospective cohort study. J Am Soc Nephrol: JASN (2019) 30:1251–60. doi: 10.1681/ASN.2018121241.
6. Wang K, Ge J, Han W, Wang D, Zhao Y, Shen Y, et al. Risk factors for kidney stone disease recurrence: a comprehensive meta-analysis. BMC urol (2022) 22:62. doi: 10.1186/s12894-022-01017-4.
7. Thongprayoon C, Krambeck AE, Rule AD. Determining the true burden of kidney stone disease. Nat Rev Nephrol (2020) 16:736–46. doi: 10.1038/s41581-020-0320-7.
8. Piché M-E, Tchernof A, Després J-P. Obesity phenotypes, diabetes, and cardiovascular diseases. Circ Res (2020) 126:1477–500. doi: 10.1161/CIRCRESAHA.120.316101.
9. Geiss L, Wang J, Cheng Y, Thompson T, Barker L, Li Y, et al. Prevalence and incidence trends for diagnosed diabetes among adults aged 20 to 79 years, United States, 1980-2012. JAMA (2014) 312:1218–26. doi: 10.1001/jama.2014.11494.
10. Daniel C, Cross AJ, Koebnick C, Sinha R. Trends in meat consumption in the USA. Public Health Nutr (2011) 14:575–83. doi: 10.1017/S1368980010002077.
11. Bleich SN, Wang YC, Wang Y, Gortmaker SL. Increasing consumption of sugar-sweetened beverages among US adults: 1988-1994 to 1999-2004. Am J Of Clin Nutr (2009) 89:372–81. doi: 10.3945/ajcn.2008.26883.
12. Fujii Y, Okada A, Yasui T, Niimi K, Hamamoto S, Hirose M, et al. Effect of adiponectin on kidney crystal formation in metabolic syndrome model mice via inhibition of inflammation and apoptosis. PloS One (2013) 8:e61343. doi: 10.1371/journal.pone.0061343.
13. Khan SR. Reactive oxygen species as the molecular modulators of calcium oxalate kidney stone formation: evidence from clinical and experimental investigations. J urol (2013) 189:803–11. doi: 10.1016/j.juro.2012.05.078.
14. Lee HJ, Jeong SJ, Park MN, Linnes M, Han HJ, Kim JH, et al. Gallotannin suppresses calcium oxalate crystal binding and oxalate-induced oxidative stress in renal epithelial cells. Biol Pharm bullet (2012) 35:539–44. doi: 10.1248/bpb.35.539.
15. Mezger M, Nording H, Sauter R, Graf T, Heim C, von Bubnoff N, et al. Platelets and immune responses during thromboinflammation. Front Immunol (2019) 10:1731. doi: 10.3389/fimmu.2019.01731.
16. Cetinkaya M, Buldu I, Kurt O, Inan R. Platelet-to-lymphocyte ratio: A new factor for predicting systemic inflammatory response syndrome after percutaneous nephrolithotomy. Urol J (2017) 14:4089–93. doi: 10.22037/uj.v14i5.3749
17. van der Stoep M, Korporaal SJA, Van Eck M. High-density lipoprotein as a modulator of platelet and coagulation responses. Cardiovasc Res (2014) 103:362–71. doi: 10.1093/cvr/cvu137.
18. Nofer J--R, Brodde MF, Kehrel BE. High-density lipoproteins, platelets and the pathogenesis of atherosclerosis. Clin And Exp Pharmacol And Physiol (2010) 37:726–35. doi: 10.1111/j.1440-1681.2010.05377.x.
19. Pirillo A, Catapano AL, Norata GD. Biological consequences of dysfunctional HDL. Curr Medicinal Chem (2019) 26:1644–64. doi: 10.2174/0929867325666180530110543.
20. Jialal I, Jialal G, Adams-Huet B. The platelet to high density lipoprotein -cholesterol ratio is a valid biomarker of nascent metabolic syndrome. Diabetes-Metabolism Res And Rev (2021) 37(6):E3403. doi: 10.1002/dmrr.3403.
21. Ciardullo S, Monti T, Perseghin G. Prevalence of liver steatosis and fibrosis detected by transient elastography in adolescents in the 2017-2018 national health and nutrition examination survey. Clin Gastroenterol Hepatol (2021) 19:384–90.e1. doi: 10.1016/j.cgh.2020.06.048.
22. Vilar-Gomez E, Nephew L, Vuppalanchi R, Gawrieh S, Mladenovic A, Pike F, et al. High-quality diet, physical activity, and college education are associated with low risk of NAFLD among the US population. Hepatology (2022) 75:1491–506. doi: 10.1002/Hep.32207
23. Wang W, Lu X, Shi Y, Wei X. Association between food insecurity and kidney stones in the United States: Analysis of the National Health and Nutrition Examination Survey 2007-2014. Front Public Health (2022) 10:1015425. doi: 10.3389/fpubh.2022.1015425.
24. Di X, Xiang L, Jian Z, Xia Z, Luo D. Association between urinary phthalate metabolites and nephrolithiasis in adults: A cross-sectional analysis with NHANES 2007-2018. Chemosphere (2023) 337:139436. doi: 10.1016/j.chemosphere.2023.139436.
25. Ciardullo S, Perseghin G. Statin use is associated with lower prevalence of advanced liver fibrosis in patients with type 2 diabetes. Metabolism (2021) 121:154752. doi: 10.1016/j.metabol.2021.154752.
26. Zou B, Yeo Y, Nguyen V, Cheung R, Ingelsson E, Nguyen MH. Prevalence, characteristics and mortality outcomes of obese, nonobese and lean NAFLD in the United States, 1999-2016. J Intern Med (2020) 288:139–51. doi: 10.1111/joim.13069.
27. Levey A, Stevens L, Schmid C, Zhang Y, Castro A, Feldman H, et al. A new equation to estimate glomerular filtration rate. Ann Intern Med (2009) 150:604–12. doi: 10.7326/0003-4819-150-9-200905050-00006.
28. Kidney Disease: Improving Global Outcomes Glomerular Diseases Work G. KDIGO 2021 clinical practice guideline for the management of glomerular diseases. Kidney Int (2021) 100:S1–S276. doi: 10.1016/j.kint.2021.05.021.
29. Plan and operation of the Third National Health and Nutrition Examination Survey, 1988-94. Series 1: programs and collection procedures. Vital And Health Statistics Ser 1, Programs And Collection Procedures (1994) 1994:1–407.
30. Wright ME, Mayne ST, Stolzenberg-Solomon RZ, Li Z, Pietinen P, Taylor PR, et al. Development of a comprehensive dietary antioxidant index and application to lung cancer risk in a cohort of male smokers. Am J Epidemiol (2004) 160:68–76. doi: 10.1093/aje/kwh173.
31. Shivappa N, Steck SE, Hurley TG, Hussey JR, Hébert JR. Designing and developing a literature-derived, population-based dietary inflammatory index. Public Health Nutr (2014) 17:1689–96. doi: 10.1017/S1368980013002115.
32. Hu B, Yang XR, Xu Y, Sun YF, Sun C, Guo W, et al. Systemic immune-inflammation index predicts prognosis of patients after curative resection for hepatocellular carcinoma. Clin Cancer Res (2014) 20:6212–22. doi: 10.1158/1078-0432.CCR-14-0442.
33. Di X, Liu S, Xiang L, Jin X. Association between the systemic immune-inflammation index and kidney stone: A cross-sectional study of NHANES 2007-2018. Front Immunol (2023) 14:1116224. doi: 10.3389/fimmu.2023.1116224.
34. Akinbami L, Chen T, Davy O, Ogden C, Fink S, Clark J, et al. National health and nutrition examination survey, 2017-march 2020 prepandemic file: sample design, estimation, and analytic guidelines. Vital Health Stat 1 (2022) 190:1–36.
35. Maddahi N, Yarizadeh H, Aghamir S, Alizadeh S, Yekaninejad M, Mirzaei K. The association of dietary inflammatory index with urinary risk factors of kidney stones formation in men with nephrolithiasis. BMC Res Notes (2020) 13:373. doi: 10.1186/s13104-020-05206-y
36. Zhang C, Qiu S, Bian H, Tian B, Wang H, Tu X, et al. Association between Dietary Inflammatory Index and kidney stones in US adults: data from the National Health and Nutrition Examination Survey (NHANES) 2007-2016. Public Health Nutr (2021) 24:6113–21. doi: 10.1017/S1368980021000793.
37. Saneei P, Hashemipour M, Kelishadi R, Esmaillzadeh A. The Dietary Approaches to Stop Hypertension (DASH) diet affects inflammation in childhood metabolic syndrome: a randomized cross-over clinical trial. Ann Nutr Metab (2014) 64:20–7. doi: 10.1159/000358341.
38. Chrysohoou C, Panagiotakos D, Pitsavos C, Das U, Stefanadis C. Adherence to the Mediterranean diet attenuates inflammation and coagulation process in healthy adults: The ATTICA Study. J Am Coll Cardiol (2004) 44:152–8. doi: 10.1016/j.jacc.2004.03.039.
39. Cohen AJ, Adamsky MA, Nottingham CU, Pruitt J, Lapin B, Wang CH, et al. Impact of statin intake on kidney stone formation. Urology (2019) 124:57–61. doi: 10.1016/j.urology.2018.01.029.
40. Taguchi K, Okada A, Hamamoto S, Iwatsuki S, Naiki T, Ando R, et al. Proinflammatory and metabolic changes facilitate renal crystal deposition in an obese mouse model of metabolic syndrome. J Urol (2015) 194:1787–96. doi: 10.1016/j.juro.2015.07.083.
41. Tsujihata M, Momohara C, Yoshioka I, Tsujimura A, Nonomura N, Okuyama A. Atorvastatin inhibits renal crystal retention in a rat stone forming model. J Urol (2008) 180:2212–7. doi: 10.1016/j.juro.2008.07.024.
42. Kumar P, Yang Z, Lever JM, Chávez MD, Fatima H, Crossman DK, et al. Hydroxyproline stimulates inflammation and reprograms macrophage signaling in a rat kidney stone model. Biochim Biophys Acta Mol Basis Dis (2022) 1868:166442. doi: 10.1016/j.bbadis.2022.166442.
43. Taguchi K, Okada A, Hamamoto S, Unno R, Moritoki Y, Ando R, et al. M1/M2-macrophage phenotypes regulate renal calcium oxalate crystal development. Sci Rep (2016) 6:35167. doi: 10.1038/srep35167.
44. Khan SR, Canales BK, Dominguez-Gutierrez PR. Randall’s plaque and calcium oxalate stone formation: role for immunity and inflammation. Nat Rev Nephrol (2021) 17:417–33. doi: 10.1038/s41581-020-00392-1.
45. Singhto N, Thongboonkerd V. Exosomes derived from calcium oxalate-exposed macrophages enhance IL-8 production from renal cells, neutrophil migration and crystal invasion through extracellular matrix. J proteomics (2018) 185:64–76. doi: 10.1016/j.jprot.2018.06.015.
46. Torricelli FC, De SK, Gebreselassie S, Li I, Sarkissian C, Monga M. Dyslipidemia and kidney stone risk. J Urol (2014) 191:667–72. doi: 10.1016/j.juro.2013.09.022.
47. Sakhaee K, Adams-Huet B, Moe O, Pak C. Pathophysiologic basis for normouricosuric uric acid nephrolithiasis. Kidney Int (2002) 62:971–9. doi: 10.1046/j.1523-1755.2002.00508.x.
48. Riese RJ, Sakhaee K. Uric acid nephrolithiasis: pathogenesis and treatment. J Urol (1992) 148:765–71. doi: 10.1016/S0022-5347(17)36715-0.
49. Ferraro PM, Curhan GC. Serum uric acid and risk of kidney stones. Am J Kidney Dis (2017) 70:158–9. doi: 10.1053/j.ajkd.2017.05.004.
50. Coe FL, Strauss AL, Tembe V, Le Dun S. Uric acid saturation in calcium nephrolithiasis. Kidney Int (1980) 17:662–8. doi: 10.1038/ki.1980.205.
51. Taylor E, Stampfer M, Curhan G. Obesity, weight gain, and the risk of kidney stones. JAMA (2005) 293:455–62. doi: 10.1001/jama.293.4.455.
52. Duffey BG, Pedro RN, Kriedberg C, Weiland D, Melquist J, Ikramuddin S, et al. Lithogenic risk factors in the morbidly obese population. J Urol (2008) 179:1401–6. doi: 10.1016/j.juro.2007.11.072.
53. Madore F, Stampfer MJ, Willett WC, Speizer FE, Curhan GC. Nephrolithiasis and risk of hypertension in women. Am J Kidney Dis (1998) 32:802–7. doi: 10.1016/S0272-6386(98)70136-2.
54. Weinberg AF, Patel CJ, Chertow GM, Leppert JT. Diabetic severity and risk of kidney stone disease. Eur Urol (2014) 65:242–7. doi: 10.1016/j.eururo.2013.03.026.
55. Sur RL, Masterson JH, Palazzi KL, L’Esperance JO, Auge BK, Chang DC, et al. Impact of statins on nephrolithiasis in hyperlipidemic patients: a 10-year review of an equal access health care system. Clin Nephrol (2013) 79:351–5. doi: 10.5414/CN107775.
56. Khan SR. Role of renal epithelial cells in the initiation of calcium oxalate stones. Nephron Exp Nephrol (2004) 98:e55–60. doi: 10.1159/000080257.
Keywords: platelet/high-density lipoprotein cholesterol ratio, nephrolithiasis, NHANES, cross-sectional study, United States
Citation: Ni J, Lv L, Wu P and Xu C (2024) Associations between the platelet/high-density lipoprotein cholesterol ratio and likelihood of nephrolithiasis: a cross-sectional analysis in United States adults. Front. Endocrinol. 15:1289553. doi: 10.3389/fendo.2024.1289553
Received: 06 September 2023; Accepted: 02 February 2024;
Published: 21 February 2024.
Edited by:
Kuanjun He, Inner Mongolia Minzu University, ChinaReviewed by:
Xingpeng Di, Sichuan University, ChinaQiu-li Zhang, Affiliated Hospital of Guangdong Medical College Hospital, China
Copyright © 2024 Ni, Lv, Wu and Xu. This is an open-access article distributed under the terms of the Creative Commons Attribution License (CC BY). The use, distribution or reproduction in other forums is permitted, provided the original author(s) and the copyright owner(s) are credited and that the original publication in this journal is cited, in accordance with accepted academic practice. No use, distribution or reproduction is permitted which does not comply with these terms.
*Correspondence: Chaoyang Xu, jinhuaxcy@126.com